- Department of Food Economics and Consumption Studies, University of Kiel, Kiel, Germany
Climate change remains a major challenge for farmers who rely on nature-based livelihoods such as livestock, which is a crucial aspect of income generation and food security in developing countries. In this study, we examine the determinants of livestock farmers’ adoption of climate-smart agricultural (CSA) practices and the impact of adoption on food security and household income in Punjab, Pakistan. The two CSA practices include livestock management (housing modification, livestock diversification, reducing herd size, and incorporating trees into livestock farming) and health and feed management (animal healthcare measures, feeding practices, enhanced fodder, and manure incorporation). We employ data from 428 livestock farmers in five districts of Punjab, employing a multinomial endogenous switching regression model to address potential selection bias. The results reveal that factors affecting CSA practice adoption include livestock units, landholdings, perception of climate change, climate indicators, veterinary center access, farming experience, and perception of increasing animal diseases. We also demonstrate that livestock farmers who adopt combined CSA practices benefit more than those who do not adopt any or adopt an individual practice, in terms of food security and household income. The findings also reveal that farmers’ perception of climate change and veterinary center access promote the adoption of CSA practices.
1 Introduction
Considerable climate change effects have occurred worldwide over the past few years, including variations in temperature and rainfall patterns, rising sea levels, and extreme weather events (IPCC, 2022). The frequency and severity of these changes are expected to increase in the near future, posing a serious threat to the global environment (Khan et al., 2021). Developing countries are more adversely affected by climate change than developed countries (Ankrah Twumasi and Jiang, 2021; IFAD, 2009), particularly agriculturally dependent developing countries such as Pakistan, where agriculture comprises 24% of the GDP and employs 37.4% of the population (GOP, 2024). Pakistan is among the top ten countries that have been most affected by climate change, although the nation’s global greenhouse gas (GHG) emissions are only approximately 0.8% of the global total GHG (CIAT and World Bank, 2017; Faisal et al., 2021b).
As the largest agricultural subsector, livestock farming is a major source of food security and is crucial to the economic well-being of rural households as 1.7 billion people generate income from livestock rearing globally (Shahbaz et al., 2020). Eight million families that are exposed to multiple climate change threats earn a major proportion of their income from livestock farming in the rural areas of Pakistan (GOP, 2023). The United Nations’ Food and Agriculture Organization (FAO) reports that livestock production makes up 40% of the total value of global agriculture and plays a vital role in supporting the livelihoods and food security of approximately 1.3 billion people (Bilotto et al., 2024). Climate change directly and indirectly affects livestock production with substantial economic and environmental implications, such as reduced water availability, increased water use, diminished quality and quantity of feed crops and foraging sites, metabolic changes in animals, reduced meat and milk production, pathogens, spread of vector-borne diseases, reduced reproductive performance, negative effects on animal immune systems, increased livestock mortality, and biodiversity loss (Cheng et al., 2022; Rojas-Downing et al., 2017; Thornton et al., 2015).
Agriculture has a two-way relationship with climate change, as this sector is also responsible for considerable GHG emissions. The sector’s performance can be improved by altering agricultural practices and adopting mitigation and adaptation measures. Such measures can advance the goals of climate-smart agriculture (CSA), which is resilient to climate change, reduces GHG emissions, and sustainably increases productivity for improved income and food security. In the agricultural sector, climate change adaptation and mitigation go hand-in-hand (FAO, 2008). In the current circumstances of climate change and rising population in Pakistan, it is crucial to manage livestock farms by employing CSA practices, including livestock diversification, destocking, agroforestry, animal healthcare measures, supplemental feeding, and other measures to improve farm performance (Cheng et al., 2022; Grossi et al., 2019; Rojas-Downing et al., 2017) and livestock farmers’ household welfare (Faisal et al., 2021a; Ndiritu and Muricho, 2021).
Most smallholder livestock farmers in Pakistan are extremely poor, with limited capacity to adopt CSA practices. Farmers generally employ traditional practices to navigate climate change, such as tree shading, mud floors and roofs, destocking, mixed farming, and increased drinking water, which are interrelated and adopted in combination (Faisal et al., 2021b; Shahbaz et al., 2020). Examining the adoption process requires an understanding of farmers’ perceptions of climate change and the factors that influence their decision to adopt CSA practices (Abbas et al., 2022). Adopting CSA practices has the potential to reduce the adverse impacts of climate variability and improve smallholder livestock farmers’ household welfare (Faisal et al., 2021b). Therefore, understanding the adoption process and how CSA practices affect rural household welfare will help identify effective CSA practices with considerable agricultural and environmental policy implications.
While the interaction between climate change and agricultural production, adoption behavior of CSA practices in response to climate change and their impact has gained research attention, the empirical literature has focused on crop production, with few studies examining the drivers and impact of adoption of CSA practices on livestock farming (Ranasinghe et al., 2023; Rojas-Downing et al., 2017). Most of these studies have focused on identifying the factors that affect livestock farmers’ adaptation to climate change (Ranasinghe et al., 2023; Abbas et al., 2022; Faisal et al., 2021b; Nganga et al., 2020), while very few studies have examined the impact of livestock farmers’ adoption of CSA practices on household welfare (Faisal et al., 2021a; Ndiritu and Muricho, 2021; Rahut and Ali, 2018). These studies demonstrate the positive impact of climate change adaptation strategies on household welfare. For instance, Rahut and Ali (2018) conducted a study in Pakistan using propensity score matching (PSM) analysis and reported that livestock insurance and allocating more land for fodder led to increase in household income and milk production. In contrast, coping strategies like migration and animal sales had negative impact on milk production. Similarly, Faisal et al. (2021a) study on Pakistan, employed Propensity Score Matching (PSM) and Poisson regression, examined farmers’ adaptation to climate change and its negative impacts on livestock losses and poverty levels. However, these studies failed to adequately address for unobserved selection bias, leading to biased or inconsistent impact estimates. Ndiritu and Muricho (2021), study on Kenya, applied the Endogenous Switching Regression (ESR) model and found positive impacts of CSA adoption on food security. While ESR is a more robust method than PSM as it accounts for unobserved selection bias, it is generally limited to binary treatment settings (Abdulai and Huffman, 2014; Shahzad et al., 2020).
A major limitation across these studies is their focus on single-adoption of practices, without considering the adoption of multiple practices. This overlook the potential complementarity or substitutability among different CSA practices when adopted in combination, which is critical for providing valuable insights into the true impact of CSA adoption on household welfare. To address these gaps our study contributes to the literature by analysing the drivers of adoption and the impact of CSA practices on the welfare of livestock farmers’ households in rural Pakistan. We employ the multinomial endogenous switching regression (MESR) approach to address selectivity bias due to observable and unobservable factors in multiple adoption context (Bourguignon et al., 2007). To the best of our knowledge, this approach has not been previously employed to assess the impact of CSA practices on livestock farmers’ welfare. The CSA practices examined in this study include the individual adoption of livestock management and health and feed management practices, as well as their combined adoption.
The remainder of this paper is organized as follows. Section 2 presents the conceptual framework and methodology of the study. Section 3 describes the data and the variables used in this study. Section 4 examines and discusses the empirical results, and section 5 presents the conclusions and policy implications.
2 Materials and methods
2.1 Conceptual framework and methodology
The conceptual framework employed in this study allows us to examine the individual and joint impact of adopting livestock management and health and feed management CSA practices on livestock farmers’ food security and income. In this context, livestock management is considered a broad category that refers to physical housing modification, livestock diversification, reducing the number of weak or unproductive animals and minimizing herd sizes to mitigate potential losses from climate change, and incorporating trees into livestock farming systems to provide shade and create a more favorable environment. Health and feed management practices include animal healthcare measures, supplemental feeding, enhanced fodder availability through hay and silage storage, and manure incorporation to enhance the soil properties and feed crop productivity. These CSA practices were selected from previous studies (Cheng et al., 2022; Faisal et al., 2021b; Rojas-Downing et al., 2017) and observations made during our in-person survey of farmers.
These CSA practices are further categorized into four possible combinations: non-adopters, adoption of one or more health and feed management-related practices only (HFM), adoption of one or more livestock management-related practices only (LM), and joint adoption of at least one health and feed management-related practice alongside one livestock management-related practice.
Farmers endogenously self-select to adopt CSA practices, and adoption decisions may be influenced by farmers’ observed and unobservable characteristics, which may also correlate with particular outcomes of interest. Therefore, employing ordinary least squares (OLS) to analyze the impact of farmers’ CSA practice adoption may lead to biased and inconsistent estimates. Hence, we applied the multinomial endogenous switching regression (MESR) model to account for selection bias (Bourguignon et al., 2007). The MESR model is a two-step estimation procedure that is applied to examine the factors affecting the adoption of individual and combined CSA practices using a multinomial logit selection model in the first stage, and the impact of CSA practice adoption on food security and household income in the second stage using OLS, including selection bias correction.
2.1.1 Stage 1: multinomial logit selection model
In the first stage, adoption of combination of CSA practices is examined using multinomial logit selection model.
First, we assume that farmers adopt a combination of CSA practices to maximize their expected benefits. Although the expected benefits are unobservable, they can be represented with a latent variable and expressed as a function of the observed and unobserved factors. This can be specified as
The latent function indicates that farmer will adopt the CSA practice if that practice offers greater expected benefits than alternative option , where (Di Falco and Veronesi, 2013; Teklewold et al., 2013) for Equations 1, 2.
where .
Assuming that is independently and identically Gumbel distributed, the probability of the farmer selecting the CSA practice can be obtained using a multinomial logit (MNL) model as follows:
Parameters of Equation 3 are estimated employing the maximum likelihood method.
2.1.2 Stage 2: MESR model
The second stage of the model estimated the impact of all explanatory variables of interest on the outcome variables for each CSA practice, , where the outcome variables were household dietary diversity score (HDDS), household food insecurity access scale (HFIAS), and household income.
The outcome equation for each CSA practice choice is as follows.
where is the outcome variable of the farmer using the regime based on expected benefits, including non-adoption , adoption of health and feed management practices , adoption of livestock management practices , and joint adoption , and is a vector representing the parameters to be estimated.
As noted previously, farmers may endogenously self-select to adopt CSA practices, resulting in potential selection bias. Following Bourguignon et al. (2007), we assume that the error terms and are linearly correlated for each CSA practice choice and selection correction terms are required to obtain consistent estimates of .
To account for selection bias, Equation 4 can be re-specified as.
where is the error term with zero mean, is the inverse Mills ratio, which is evaluated from the estimated probabilities in the MNL model, is the covariance between , and , and is the correlation coefficient between and .
The selection and outcome equations were estimated simultaneously using the Full Information Maximum Likelihood (FIML) estimation method. The coefficients from the MESR model are then used to calculate the average treatment effect on the treated (ATT) by comparing the expected outcome values under both actual and counterfactual scenarios of adoption and non-adoption.
2.1.3 Estimating the average treatment effect of the treated (ATT)
To assess the impact of adopting CSA practices on the outcome variables, coefficients from the MESR model are used to estimate the treatment effects on the treated, from which we can compare the adopters’ expected outcomes and counterfactual outcomes for non-adopters with the same observable characteristics (Heckman et al., 2001; Teklewold et al., 2013) (Equation 8). We employed the MESR model to estimate the average treatment effects to address potential selection bias from the observed and unobserved factors.
The expected values of each adopter’s outcome variables (HDDS, HFIAS, household income) with adoption , can be predicted from Equation 5 as follows:
The counterfactual case of CSA practice non-adoption can be expressed as follows:
ATT indicates the impact of adopting the CSA practice on the outcome variables, which can be estimated by obtaining the differences between Equations 6, 7.
To address the identification issue of including the same covariates in selection and outcome equations, the selection equation should include at least one instrumental variable that directly affects participation in CSA practices, in the selection equation but does not directly affect the outcome variable. In line with previous studies (Issahaku and Abdulai, 2020; Ndiritu and Muricho, 2021; Shahzad et al., 2021), we hypothesize that farmers’ perception of climate change can directly influence the decision to adopt CSA practices but not the outcome variables of food security and household income. In addition to climate change perception, we used farmers’ perceptions of increased animal diseases in the last 20 years as identifying instruments. Our exclusion restriction is that farmers’ perceptions of climate change and increased animal disease incidence do not directly affect the outcome variable, but only indirectly through the decision to adopt CSA practices. These variables reflect long-term assessment of environmental changes and changes associated with animal diseases, rather than short-term shocks. As such, they are unlikely to directly influence the current outcome variables (food security and household income), which are more immediately affected by present conditions and economic circumstances. From a theoretical perspective, farmers who have observed changes over decades are more likely to engage in long-term adaptive measures, particularly the adoption of CSA practices, rather than altering daily consumption or income-earning activities. Thus, we hypothesize that farmers’ perceptions of climate change and the increased incidence of animal diseases over the past 20 years do not directly affect the outcome variables but rather influence them through the adoption of CSA practices.
A falsification test was conducted to confirm the validity of the instruments. The results presented in Supplementary Appendix Table 6 show that the instruments significantly impact the adoption of CSA practices but have no statistically significant impact on the outcome variables of non-adopters. We used a two-stage control function approach (Wooldridge, 2015) to address the potential endogeneity of farmers’ participation in off-farm activities, which may be endogenous because farmers involved in off-farm activities may not be able to adopt CSA practices because of the labor-intensive nature of some practices (Issahaku and Abdulai, 2020). We specify the potential endogenous variable as a function of all variables influencing the adoption of CSA practices along with the instrumental variables in the first stage of the logit regression, and calculate generalized residuals using the distance to the nearest town or city as an instrument for off-farm activity participation. This study included these estimated residuals in the MESR model to obtain a consistent estimation of off-farm activity participation.
3 Data and descriptive statistics
Data were obtained from a survey conducted in Punjab Province, Pakistan from December 2022 to February 2023. Face-to-face interviews were conducted in the local language because of the low literacy rate of the farmers. The prior informed consent was obtained from all respondents. The survey obtained comprehensive information on livestock farmers’ socioeconomic characteristics (e.g., household head age, education, family size, household income, farm experience), food security indicators (including HDDS and HFIAS), access to a veterinary center, perception of climate change and extreme weather events including floods, drought, changes in precipitation and temperature, perception of increased incidence of animal diseases, and current measures undertaken to navigate climate change.
Punjab is the most populous province in Pakistan, in terms of both humans and animals (Ahmad and Ma, 2020). The province includes northern and southern Punjab, with large ruminant populations of 64 and 36%, according to the 2006 Pakistan Livestock Census. We used a multistage sampling technique to collect primary data from two regions in Punjab (Figure 1). Based on the proportional share of the animal population, in the first stage, we selected three districts of northern Punjab (Faisalabad, Sheikhupura and Sahiwal) and two districts of southern Punjab (Rahim Yar Khan and Bahawalnagar) from arid and semiarid areas, which are more vulnerable to climate change (Ali and Erenstein, 2017). In the second stage, we randomly selected two tehsils (sub-districts) from each district and two union councils in the third stage, and then randomly chose three villages from each union council. In the last stage, we randomly selected seven to eight farmers from each village. Our survey included 428 livestock farmers, most of whom were involved in subsistence crop production.
We also conducted interviews with experts and government officials in the livestock and agriculture departments to understand the measures taken by the departments to address climate change, livestock health, and other challenges faced. We also used secondary data on the average daily temperature and precipitation from 1984 to 2022 for the selected districts to estimate climate indicators, using location-specific coordinates to interpolate climate data at the household level to merge with our survey data. We obtained climate data from the US National Aeronautics and Space Administration (NASA) Langley Research Center POWER Project for 2022 and long-term, averaging data from 1984 to 2021 to identify temperature and precipitation anomalies (Stackhouse et al., 2021). Weather anomalies were calculated by subtracting the long-term means (temperature/precipitation) from the mean of recent year (2022) and dividing them by the long-term mean (1984 to 2021).
The data revealed that 95.7% of households perceived climate change. Specifically, 92.5% of households perceived changes in average temperature, 94.2% perceived changes in rainfall, 90% perceived changes in rainfall patterns, and 73.8% perceived changes in extreme weather events such as floods and droughts over the past 20 years. These findings highlight the growing need to adopt CSA practices to address the challenges posed by climate change.
Table 1 indicates that 87.1% of the farmers adopted at least one CSA practice, while the remaining 12.9% were non-adopters; 8.9% adopted one or more health and feed management-related practices; 6.5% adopted one or more livestock management-related practices; and 71.7% adopted at least one health and feed management related practice and one livestock management-related practice simultaneously. The descriptive statistics of all the variables used in the current study are presented in Table 1.
Table 2 presents the different characteristics of individual and combined CSA practice adopters compared to non-adopters. Although a significant difference is evident among adopters of health and feed management practices compared to non-adopters in terms of HFIAS and household income and a significant difference is observed among joint adopters compared to non-adopters in terms of HDDS, HFIAS, and household income. These differences do not account for the potential selection bias caused by observed and unobserved factors. These differences also indicate that these variables may have different effects on the outcome variables, depending on farmers’ adoption of CSA practices, which justifies the application of MESR (Issahaku and Abdulai, 2020).
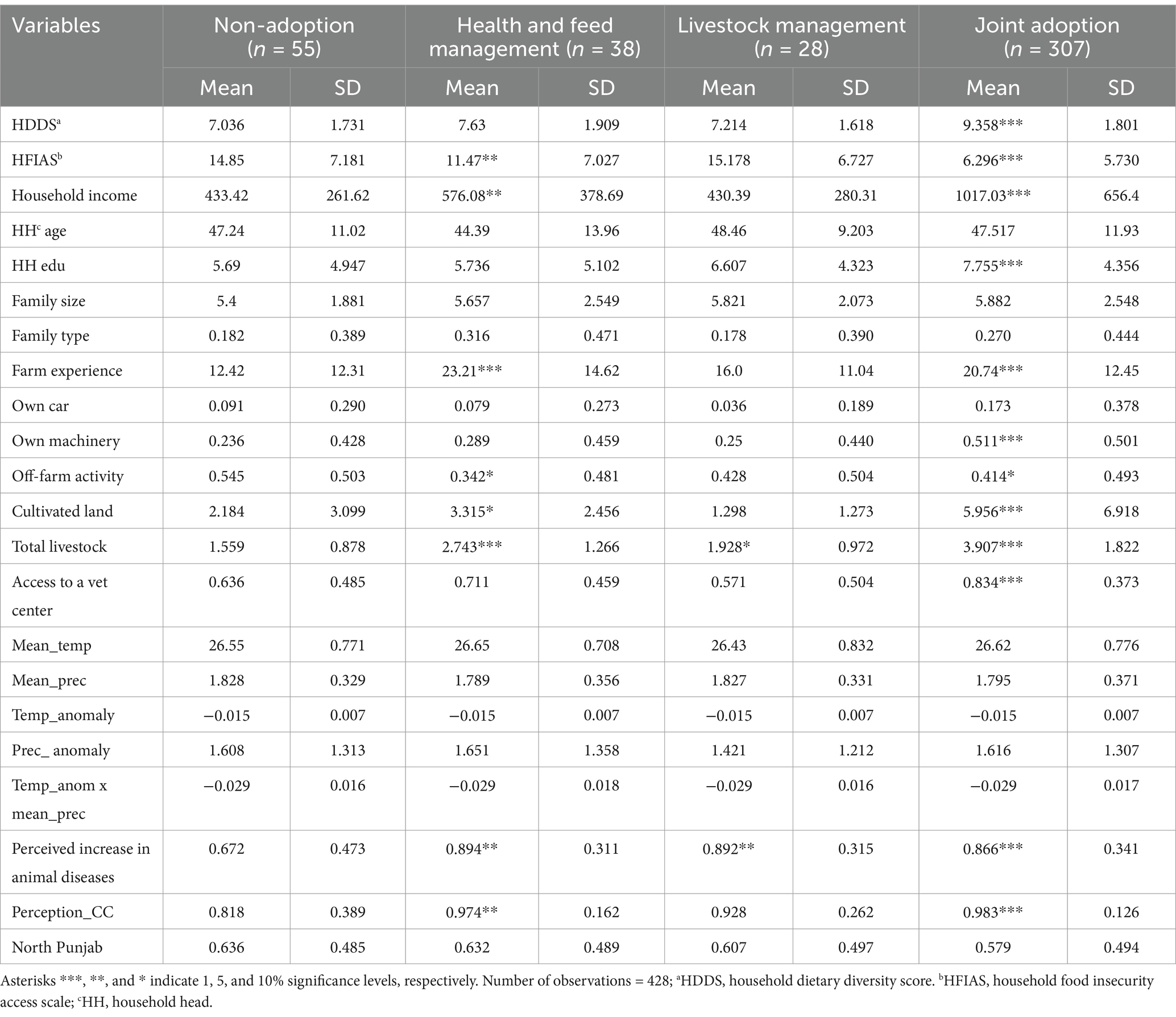
Table 2. Different characteristics of individual and combined CSA practice adopters and non-adopters.
4 Results and discussion
4.1 Determinants of CSA practices adoption
First, we discuss the results of the first stage estimation to reveal the determinants of farmers’ adoption of CSA practices. Diagnostic tests such as the Wald test for combining alternatives and a suest-based Hausman test of independence of irrelevant alternatives, are reported in Supplementary Appendix Tables 7, 8. The suest-based Hausman test of the independence of irrelevant alternatives indicates that the null hypothesis cannot be rejected, implying that the coefficients associated with adoption categories are independent of other alternatives. The Wald test for combining alternatives confirms that the farmers’ adoption categories cannot be collapsed into a single category and that the impact of adopting CSA practices varies across categories. Subsequently, we computed the marginal effects of the coefficients using the multinomial logit (MNL) model to better interpret the results, which are presented in Table 3.
Furthermore, we employ a control function approach to address the potential endogeneity arising from farmers’ participation in off-farm activities. The coefficient of the generalized residuals of off-farm activity participation is statistically insignificant for all choices, indicating the absence of detectable endogeneity in off-farm activity participation in the model (Wooldridge, 2015). The findings of Wald test reveal that , , indicating that all regression coefficients are jointly significant in the model. The instrumental variables used in the MESR model to address the issue of identification were statistically significant in the MNL model. Moreover, a falsification test for the validity of our instrumental variables demonstrates that the instrumental variables significantly affect the selection equations, but do not influence the outcomes, such as HDDS, HFIAS, and household income of non-adopters, further validating their appropriateness for the model, as reported in Supplementary Appendix Table 6.
The results of first stage multinomial logit selection model, reported in Table 3, reveal that the household head’s age negatively and significantly affects the adoption of health and feed management practices, implying that older farmers are less likely to adopt these practices, which is consistent with the findings of (Faisal et al., 2021a). However, the marginal effect of age on livestock management practice adoption was positive and significant, indicating that older farmers use these practices to mitigate climate change risks. The education level of the household head had a positive and significant impact on the adoption of livestock management practices. On the other hand, household heads’ previous farm experience negatively influences non-adoption and positively influences the individual adoption of health and feed management and livestock management practices indicating that more experienced farmers are more likely to adopt CSA practices, likely due to being more aware of climate change and its effects on livestock over the years and the subsequent implications of climate change practices (Shahbaz et al., 2020). The impact of cultivated land and total livestock is also significant for joint and individual adoption of livestock management practices, indicating that households with more cultivated land and livestock are more likely to adopt joint practices of livestock management and health and feed management, and are less likely to adopt livestock management practices solely. In the case of joint adoption, landholding and livestock units are symbols of wealth and assets that could lead to spending more time, effort, and money on farming. This finding aligns with the results of Shahbaz et al. (2020), who found that farmers with large landholdings were more likely to adopt CSA practices in combination. Another important factor influencing CSA adoption is access to a veterinary center. Our findings suggest that it is a negative and statistically significant determinant of livestock management practice adoption and a positive and statistically significant determinant for joint adoption.
To capture climate variability, we use average temperature and precipitation data, constructed from NASA’s climate datasets, along with their respective anomalies as key indicators. The coefficients of the variables representing climate indicators, such as mean temperature and precipitation, are positively and statistically significantly associated with non-adoption, negative and significant in the context of adopting livestock management practices, but show no significant influence on the adoption of other combinations of CSA practices. This suggests that households in areas with higher average temperatures were less likely to adopt livestock management practices. We use long-term temperature and precipitation anomalies as indicators of climate variability. These anomalies are critical for capturing environmental stressors that influence farm-level decision-making. Our analysis reveals that the coefficients of the variables representing temperature and precipitation anomalies show negative and significant impact on non-adoption, but exhibit a positive and statistically significant impact on the adoption of livestock management practices. This suggests that long-term deviations in temperature and precipitation tend to increase the probability that farmers adopt CSA practices such as adjusting herd size, diversifying livestock types, improving animal housing and incorporating trees into livestock farming systems to offer shade and reduce heat stress. However, it is important to note that these anomalies did not have any statistically significant impact on the adoption of other CSA practice combinations, such as improved feed and animal health care measures. This may indicate that certain practices are perceived as more directly responsive to climate-related stress, while others may be influenced by different factors such as access to a vet center, farm experience or total cultivated land.
To account for the combined impact of average precipitation and long-term deviations in temperature, we introduced an interaction term into the model. We find the interaction term to be positive and statistically significant for non-adoption of CSA practices, but negative and statistically significant for the adoption of livestock management practices. This indicates that increased precipitation generally moderates temperature extremes, which may negatively affect the adoption of livestock management practices. By illustrating the link between NASA derived climate indicators and CSA adoption decisions, our study contributes to a deeper understanding of how observable climate variability influences farmers’ decision-making, and highlights the importance of integrating spatial climate data into household level CSA adoption decision frameworks. The coefficient of the variable representing farmers’ perception of increased animal diseases has a negative and statistically significant impact on the non-adoption of CSA practices, and exerts a positive, albeit insignificant, impact on all adoption choices. Farmers’ perception of climate change negatively and significantly affects non-adoption, positively and significantly influences joint adoption, implying that farmers who are aware of climate change are less inclined toward non-adoption of CSA practices and are more likely to adopt multiple practices simultaneously. This finding aligns with that of Faisal et al. (2021b), who argued that livestock farmers who have experienced climate change are more likely to adopt multiple climate change practices.
4.2 The impact of CSA practices on welfare outcomes
The second stage of MESR provides estimates of the determinants and impacts on welfare outcomes. The results from the estimation, reported in Supplementary Appendix Tables 9–11, reveal that the selection correction terms are statistically significant for joint adoption in HFIAS, indicating the presence of sample selection bias and validating our use of the MESR model to obtain consistent estimates. The other selection correction terms are insignificant, indicating that estimated impact of CSA practice adoption would be similar for randomly chosen farmers’ choices to adopt any CSA practice (Dubbert and Abdulai, 2021).
Table 4 presents the impact of the adoption of individual and combined CSA practices on the outcome variables of food security and household income under actual and counterfactual conditions. The actual case represents the expected outcome of farmers who adopted a specific or combined CSA practice, and the counterfactual case shows that they had not adopted it.
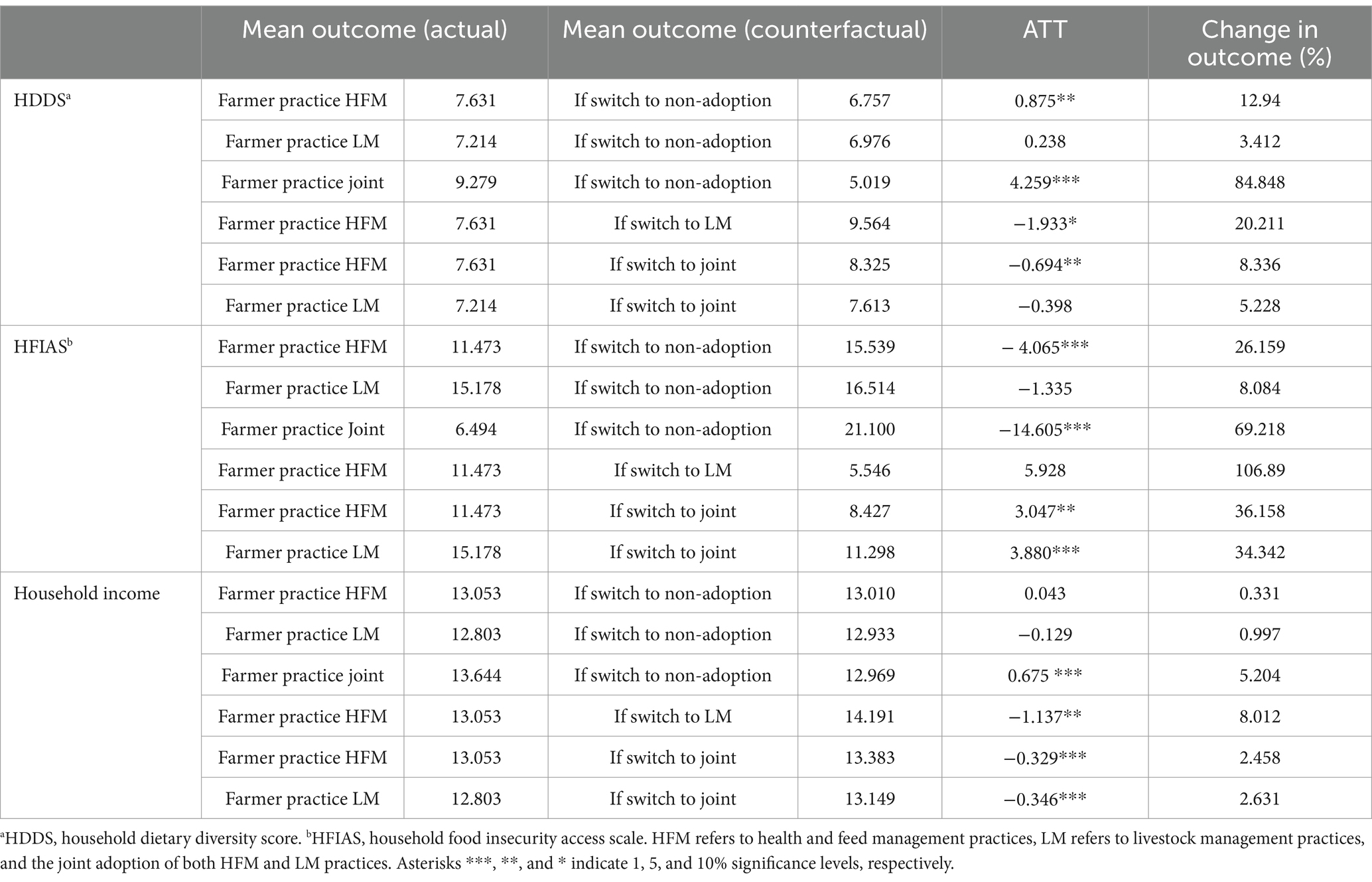
Table 4. Average treatment effects of adopting individual and combined CSA practices on HDDS, HFIAS, and household income.
The results show that the adoption of feed and health management practices has a statistically significant positive effect on HDDS, a significant negative effect on HFIAS, and an insignificant positive impact on household income compared to non-adopters, whereas the adoption of livestock management practices has an insignificant impact on all outcome variables when compared with non-adopters. However, combined CSA practice adoption results in a significant improvement in HDDS and household income and a substantial decrease in HFIAS compared to non-adopters. These results indicate that farmers who adopt feed and health management practices would experience a statistically significant decrease of 13% in HDDS and an increase of 26% in HFIAS if they had not adopted these practices. However, the adoption of these practices was associated with a statistically insignificant increase in household income. Farmers who adopt joint CSA practices, if they had not adopted them, would experience a significant decrease in HDDS and household income of 85 and 5%, respectively, and a 69% increase in HFIAS. These results are consistent with the findings of Issahaku and Abdulai (2020), Kabubo-Mariara and Mulwa (2019) and Ndiritu and Muricho (2021).
The results also show that farmers who switch from individual CSA practices (health and feed management) to joint adoption will significantly improve HDDS and household income by 8 and 2%, respectively, while reducing HFIAS by 36%. Farmers who switch from individual livestock management CSA practices to joint adoption experience a significant decrease in HFIAS by 34% and an increase in household income by 3%. This indicates that a farmer who adopts joint practices is better equipped to cope with climate variability than one who adopts individual practice. For instance, joint adoption of livestock diversification and health measures, such as timely vaccination, can reduce reliance on a single source of income, mitigate the effects of disease outbreaks, or mitigate the scarcity of feed, and thus, can more effectively help diversify the risk associated with climate change than single adoption. Furthermore, farmers who switch from individual health and feed management practices to livestock management practices would experience significantly increased food security (HDDS) and household income by 20 and 8%, respectively. The rationale for these findings is probably because livestock management practices such as destocking (selling animals makes farmers more financially strong), livestock diversification, and incorporating trees into livestock farming provide farmers with higher incomes and more diversified diets.
Overall, the results suggest that livestock farmers who adopt either individual or combined CSA practices experience better outcomes than they would have if they had not adopted any practice. Furthermore, farmers who adopt combined CSA practices are better off than if they had only adopted individual practices in terms of food security and household income. This is consistent with the findings of Issahaku and Abdulai (2020), who demonstrated that higher crop revenue can be attained from the joint adoption of the CSA practice. These findings also align with Shahbaz et al. (2022), who found that livestock farmers who adopted combined CSA livestock practices consumed higher per capita dietary intake than those who adopted CSA livestock practices in isolation.
To provide further location-specific information concerning the impact of the adoption of CSA practices in the two regions of Punjab, Table 5 presents a disaggregated analysis of the adoption impact. The results for northern Punjab are almost the same as the baseline results above, indicating that farmers who adopt either individual or combined CSA practices are better off than those who have not adopted any practice. When comparing the adoption of individual versus combined CSA practices, we found that adopting a combination of CSA practices leads to greater improvements in farmers’ welfare. Farmers also achieve better outcomes in terms of HDDS and household income if they switch from the adoption of health and feed management to livestock management practices.
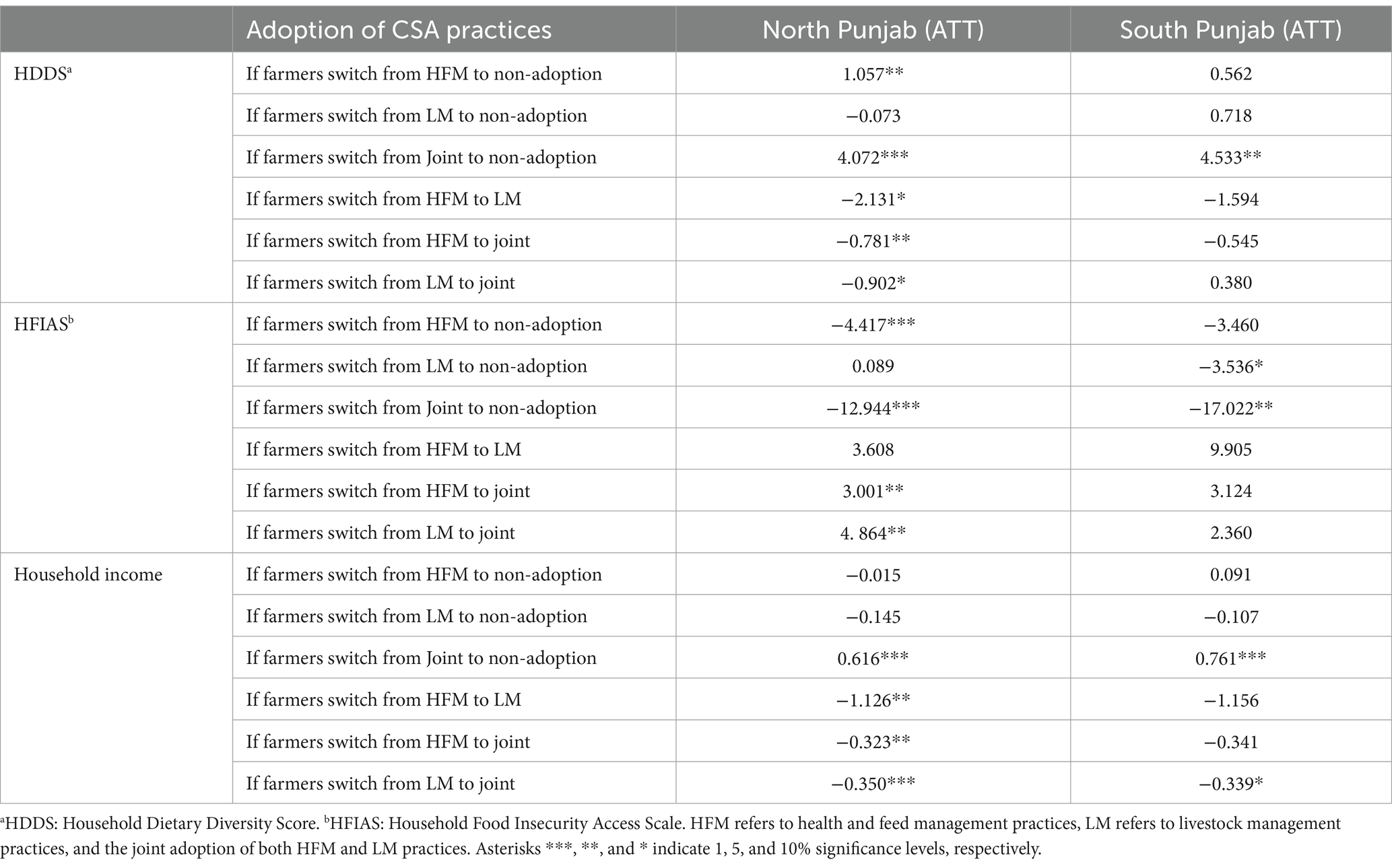
Table 5. Average treatment effects of the adoption of individual and combined CSA practices on HDDS, HFIAS, and household income by location.
The results for southern Punjab indicate that farmers who adopt joint CSA practices experience significantly higher HDDS and household income, as well as reduced HFIAS, compared to those who did not adopt these practices. Farmers who adopt livestock management practices have a statistically significant decrease in HFIAS compared to farmers who have not adopted these practices. Additionally, the results indicate that if farmers switch from adopting individual livestock management practices to the joint adoption of CSA practices, this leads to an increase in household income. However, no statistically significant differences were found for other combinations of CSA practices in southern Punjab. This is probably because of poor infrastructure and higher vulnerability to climate change compared with northern Punjab. Livestock farmers in Pakistan often depend on conventional methods to cope with changing weather conditions. This approach is likely, as the onset of climate change has compounded existing challenges in the sector. Many farmers lack adequate access to essential resources such as productive assets, availability of credit, and technical training. Furthermore, inadequate infrastructure, such as unreliable electricity, poor roads, and limited access to internet further restrict their ability to adapt effectively. Economic challenges, including inflation and high poverty levels, further exacerbates these barriers to adoption of CSA practices. Thus, the ability to adopt innovative approaches is still largely hindered, particularly in remote and rural areas.
5 Conclusion and policy implications
This study examined the determinants of farmers’ adoption of the two CSA practices and their impact on food security and household income of livestock farmers in Punjab, Pakistan. Survey data from 428 livestock farmers in five districts of Punjab were used in the empirical analysis, employing a multinomial endogenous switching regression (MESR) model to account for potential selection bias. The empirical results showed that factors such as livestock units, landholdings, perception of climate change, climate indicators, veterinary center access, farming experience, and perception of increased incidence of animal diseases tend to influence CSA practice adoption. We also found that livestock farmers who adopted combined CSA practices were better off in terms of food security and household income than those who did not adopt CSA practices or adopted only individual practice.
These findings generally indicate that the adoption of CSA practices can mitigate the adverse impacts of climate change on livestock farmers. Furthermore, CSA practice adoption can also contribute to climate change mitigation because climate change adaptation and mitigation reinforce each other, particularly in the agricultural sector (FAO, 2008). Based on the findings of this study, several policy recommendations are proposed to enhance the resilience of livestock farming to climate change. At the provincial and regional levels, the Government of Pakistan should develop targeted policies addressing climatic risks and promote CSA practices, particularly those that are poorly adopted by farmers due to limited awareness and constraints arising from poverty, low financial resources, and poor access to institutional services. Extension services must be extended to remote areas, providing small dairy farmers with the necessary guidance to the adoption of climate-smart practices. Training programs aimed at improving livestock feeding practices should be implemented, particularly for rural communities with limited resources, ensuring knowledge transfer and cooperation in adopting appropriate methods. Strengthening institutional services to increase farmers’ awareness of climate change impacts on livestock (food security) and the role of livestock in climate change would help support resilience among livestock farmers and increase farmers’ productivity and welfare. Since many livestock farmers are unaware of disease transmission, early detection, and persistence, new methods for managing and controlling livestock diseases should be introduced to reduce losses. Educational programs for herders should be tailored to encourage the implementation of optimal combinations of practices rather than relying on a single practice, thereby increasing overall farm resilience. Policymakers and development agencies could also promote the adoption of CSA practices by improving farmers’ access to credit to purchase farm inputs and diversify income by engaging in other livelihood activities to reduce their dependence on livestock farming. Furthermore, encouraging collaboration between key sectors, such as rural development, agriculture, and environmental protection, is crucial to create a supportive environment for the adoption of CSA practices.
Although our study contributes to the scant literature on the adoption of CSA practices by livestock farmers, future research could expand on this by considering other types of ruminants, such as goats and sheep. Although, our study, which is based on Punjab province, provides valuable insights into the specific challenges and opportunities faced by farmers in one of the most agriculturally important regions of Pakistan, the regional focus limits the generalizability of the findings to other geographic contexts. However, it can be useful to address similar issues in comparable agricultural areas. Future research could extend this work to other provinces, or use nationally representative data to test the broader applicability of the findings. Moreover, as in other studies, data used in the study are self-reported, which may introduce concerns regarding recall bias, while the cross-sectional nature of the analysis limits the ability to capture potential dynamics over time. We suggest that future research could address these limitations by exploring longitudinal data to better assess the evolving effects of CSA adoption.
Data availability statement
The raw data supporting the conclusions of this article will be made available by the authors, without undue reservation.
Author contributions
MA: Conceptualization, Data curation, Formal analysis, Methodology, Writing – original draft. AA: Conceptualization, Methodology, Resources, Supervision, Writing – review & editing.
Funding
The author(s) declare that financial support was received for the research and/or publication of this article. The authors acknowledge financial support by DFG within the funding program Open Access Publikationskosten. The first author also acknowledges the scholarship funding from the Higher Education Commission (HEC) of Pakistan, in collaboration with the German Academic Exchange Service (DAAD), Germany (Ref. no. 91821259).
Conflict of interest
The authors declare that the research was conducted in the absence of any commercial or financial relationships that could be construed as a potential conflict of interest.
Generative AI statement
The authors declare that no Gen AI was used in the creation of this manuscript.
Publisher’s note
All claims expressed in this article are solely those of the authors and do not necessarily represent those of their affiliated organizations, or those of the publisher, the editors and the reviewers. Any product that may be evaluated in this article, or claim that may be made by its manufacturer, is not guaranteed or endorsed by the publisher.
Supplementary material
The Supplementary material for this article can be found online at: https://www.frontiersin.org/articles/10.3389/fsufs.2025.1604899/full#supplementary-material
References
Abbas, Q., Han, J., Bakhsh, K., Ullah, R., Kousar, R., Adeel, A., et al. (2022). Adaptation to climate change risks among dairy farmers in Punjab, Pakistan. Land Use Policy 119:106184. doi: 10.1016/j.landusepol.2022.106184
Abdulai, A., and Huffman, W. (2014). The adoption and impact of soil and water conservation technology: an endogenous switching regression application. Land Econ. 90, 26–43. doi: 10.3368/le.90.1.26
Ahmad, M. I., and Ma, H. (2020). Climate change and livelihood vulnerability in mixed crop–livestock areas: the case of province Punjab, Pakistan. Sustain. For. 12:586. doi: 10.3390/su12020586
Ali, A., and Erenstein, O. (2017). Assessing farmer use of climate change adaptation practices and impacts on food security and poverty in Pakistan. Clim. Risk Manag. 16, 183–194. doi: 10.1016/j.crm.2016.12.001
Ankrah Twumasi, M., and Jiang, Y. (2021). The impact of climate change coping and adaptation strategies on livestock farmers’ technical efficiency: the case of rural Ghana. Environ. Sci. Pollut. Res. 28, 14386–14400. doi: 10.1007/s11356-020-11525-1
Bilotto, F., Harrison, M. T., Vibart, R., Mackay, A., Christie-Whitehead, K. M., Ferreira, C. S., et al. (2024). Towards resilient, inclusive, sustainable livestock farming systems. Trends Food Sci Tech 152:104668. doi: 10.1016/j.tifs.2024.104668
Bourguignon, F., Fournier, M., and Gurgand, M. (2007). Selection bias corrections based on the multinomial logit model: Monte Carlo comparisons. J. Econ. Surv. 21, 174–205. doi: 10.1111/j.1467-6419.2007.00503.x
Cheng, M., McCarl, B., and Fei, C. (2022). Climate change and livestock production: a literature review. Atmos. 13:140. doi: 10.3390/atmos13010140
CIAT and World Bank (2017). Climate-smart agriculture in Pakistan. CSA country profiles for Asia series. Washington, DC: International Center for Tropical Agriculture (CIAT) and the World Bank, 28.
Di Falco, S., and Veronesi, M. (2013). How can African agriculture adapt to climate change? A counterfactual analysis from Ethiopia. Land Economics, 89, 743–766.
Dubbert, C., and Abdulai, A. (2021). Does the contract type matter? Impact of marketing and production contracts on cashew farmers’ farm performance in Ghana. J. Agric. Food Ind. Organ. 20, 119–134. doi: 10.1515/jafio-2020-0040
Faisal, M., Abbas, A., Xia, C., Raza, M. H., Akhtar, S., Ajmal, M. A., et al. (2021a). Assessing small livestock herders’ adaptation to climate variability and its impact on livestock losses and poverty. Clim. Risk Manag. 34:100358. doi: 10.1016/j.crm.2021.100358
Faisal, M., Chunping, X., Abbas, A., Raza, M. H., Akhtar, S., Ajmal, M. A., et al. (2021b). Do risk perceptions and constraints influence the adoption of climate change practices among small livestock herders in Punjab, Pakistan? Environ. Sci. Pollut. Res. 28, 43777–43791. doi: 10.1007/s11356-021-13771-3
FAO (2011). Guidelines for the preparation of livestock sector reviews. Animal Production and Health Guidelines No. 5. Rome: Food and Agriculture Organization of the United Nations.
GOP (2023). Economic survey of Pakistan 2022-2023, Finance and Economic Affairs Division, Ministry of Finance, Islamabad, Government of Pakistan (GOP). Available online at: https://www.finance.gov.pk/survey_2023.html
GOP (2024). Economics Survey of Pakistan 2023-2024, Finance and Economic Affairs Division, Ministry of Finance, Islamabad, Government of Pakistan (GOP). Available online at: https://www.finance.gov.pk/survey_2024.html
Grossi, G., Goglio, P., Vitali, A., and Williams, A. G. (2019). Livestock and climate change: impact of livestock on climate and mitigation strategies. Anim. Front. 9, 69–76. doi: 10.1093/af/vfy034
Heckman, J., Tobias, J. L., and Vytlacil, E. (2001). Four parameters of interest in the evaluation of social programs. Southern Economic Journal, 68, 210–223.
IFAD. (2009). Livestock and climate change. Livestock thematic papers. Tools for project design, International Fund for Agricultural Development (IFAD). Rome, Italy.
IPCC (2022). Climate change 2022: Impacts, adaptation and vulnerability. Cambridge, NY, USA: Cambridge University Press, 3056.
Issahaku, G., and Abdulai, A. (2020). Adoption of climate-smart practices and its impact on farm performance and risk exposure among smallholder farmers in Ghana. Aust. J. Agric. Resour. Econ. 64, 396–420. doi: 10.1111/1467-8489.12357
Kabubo-Mariara, J., and Mulwa, R. (2019). Adaptation to climate change and climate variability and its implications for household food security in Kenya. Food Secur. 11, 1289–1304. doi: 10.1007/s12571-019-00965-4
Khan, N. A., Qiao, J., Abid, M., and Gao, Q. (2021). Understanding farm-level cognition of and autonomous adaptation to climate variability and associated factors: evidence from the rice-growing zone of Pakistan. Land Use Policy 105:105427. doi: 10.1016/j.landusepol.2021.105427
Ndiritu, S. W., and Muricho, G. (2021). Impact of climate change adaptation on food security: evidence from semi-arid lands, Kenya. Clim. Chang. 167, 1–20. doi: 10.1007/s10584-021-03180-3
Nganga, T. W., Coulibaly, J. Y., Crane, T. A., Gachene, C. K., and Kironchi, G. (2020). Propensity to adapt to climate change: insights from pastoralist and agro-pastoralist households of Laikipia County. Kenya. Clim. Chang. 161, 393–413. doi: 10.1007/s10584-020-02696-4
Rahut, D. B., and Ali, A. (2018). Impact of climate-change risk-coping strategies on livestock productivity and household welfare: empirical evidence from Pakistan. Heliyon 4:e00797. doi: 10.1016/j.heliyon.2018.e00797
Ranasinghe, R. D. A. K., Korale-Gedara, P. M., and Weerasooriya, S. A. (2023). Climate change adaptation and adaptive capacities of dairy farmers: evidence from village tank cascade systems in Sri Lanka. Agric. Syst. 206:103609. doi: 10.1016/j.agsy.2023.103609
Rojas-Downing, M. M., Nejadhashemi, A. P., Harrigan, T., and Woznicki, S. A. (2017). Climate change and livestock: impacts, adaptation, and mitigation. Clim. Risk Manag. 16, 145–163. doi: 10.1016/j.crm.2017.02.001
Shahbaz, P., Abbas, A., Aziz, B., Alotaibi, B. A., and Traore, A. (2022). Nexus between climate-smart livestock production practices and farmers’ nutritional security in Pakistan: exploring level, linkages, and determinants. Int. J. Environ. Res. Public Health 19:5340. doi: 10.3390/ijerph19095340
Shahbaz, P., Boz, I., and ul Haq, S. (2020). Adaptation options for small livestock farmers having large ruminants (cattle and buffalo) against climate change in Central Punjab Pakistan. Environ. Sci. Pollut. Res. 27, 17935–17948. doi: 10.1007/s11356-020-08112-9
Shahzad, M. F., and Abdulai, A. (2020). Adaptation to extreme weather conditions and farm performance in rural Pakistan. Agric. Syst. 180:102772. doi: 10.1016/j.agsy.2019.102772
Shahzad, M. F., Abdulai, A., and Issahaku, G. (2021). Adaptation implications of climate-smart agriculture in rural Pakistan. Sustain. For. 13:11702. doi: 10.3390/su132111702
Stackhouse, P. W., Macpherson, B., Broddle, M., McNeil, C., Barnett, A. J., Mikovitz, C., et al. (2021). Introduction to the Prediction of Worldwide Energy Resources (POWER) Project: NASA Applied Sciences Week 2021. Available at: https://power.larc.nasa.gov/
Teklewold, H., Kassie, M., Shiferaw, B., and Köhlin, G. (2013). Cropping system diversification, conservation tillage and modern seed adoption in Ethiopia: Impacts on household income, agrochemical use and demand for labor. Ecological Economics, 93, 85–93.
Thornton, P. K., Boone, R. B., and Ramírez Villegas, J. (2015). Climate change impacts on livestock. CGIAR Research Program on Climate Change, Agriculture and Food Security (CCAFS). CCAFS Working Paper no. 120. Copenhagen, Denmark. Available online at: https://ccafs.cgiar.org/resources/publications/climate-change-impacts-livestock
Keywords: climate-smart agricultural practices, livestock farming, household welfare, impact assessment, Pakistan
Citation: Arshad M and Abdulai A (2025) The drivers of adoption and impact of climate-smart agricultural practices on livestock farmers’ household welfare in Pakistan. Front. Sustain. Food Syst. 9:1604899. doi: 10.3389/fsufs.2025.1604899
Edited by:
Francesco Bozzo, University of Bari Aldo Moro, ItalyReviewed by:
Biagia De Devitiis, University of Foggia, ItalyTommaso Fantechi, University of Florence, Italy
Copyright © 2025 Arshad and Abdulai. This is an open-access article distributed under the terms of the Creative Commons Attribution License (CC BY). The use, distribution or reproduction in other forums is permitted, provided the original author(s) and the copyright owner(s) are credited and that the original publication in this journal is cited, in accordance with accepted academic practice. No use, distribution or reproduction is permitted which does not comply with these terms.
*Correspondence: Mahwish Arshad, bWFyc2hhZEBmb29kLWVjb24udW5pLWtpZWwuZGU=