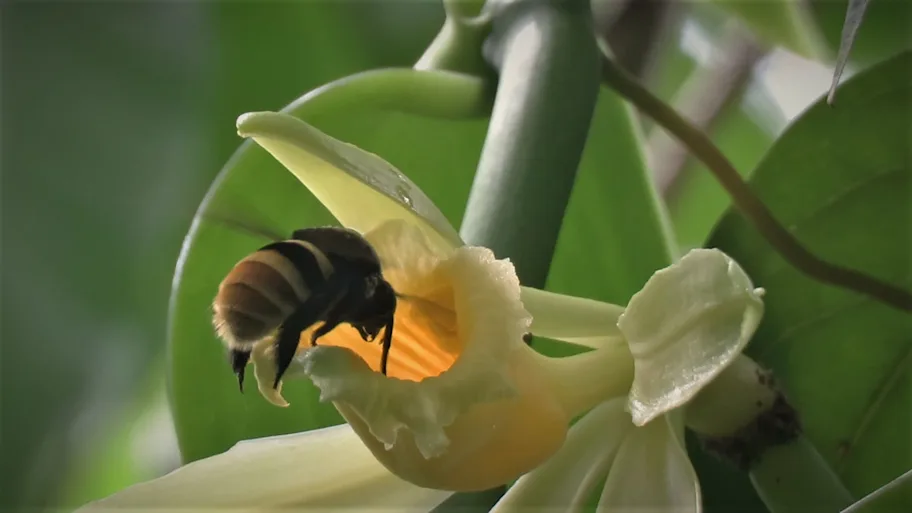
Featured news
Climate crisis could force wild vanilla plants and pollinating insects apart, threatening global supply
Researchers find that a reduced overlap of suitable habitats for vanilla plants and the insects that pollinate them could threaten the survival of wild vanilla