- 1Centre for Glaciology, Department of Geography and Earth Sciences, Aberystwyth University, Aberystwyth, United Kingdom
- 2Centre for Arctic Gas Hydrate, Environment and Climate, Department of Geology, UiT The Arctic University of Norway, Tromsø, Norway
- 3Department of Glaciology and Climate, Geological Survey of Denmark and Greenland, Copenhagen, Denmark
- 4School of Geography, Geology and the Environment, Keele University, Keele, United Kingdom
- 5Institute of Biological, Environmental and Rural Sciences, Aberystwyth University, Aberystwyth, United Kingdom
- 6Department of Geography, University of Sheffield, Sheffield, United Kingdom
- 7Department of Geography, University of California, Los Angeles, Los Angeles, CA, United States
- 8Department of Geography, Rutgers, The State University of New Jersey, New Brunswick, NJ, United States
- 9Department of Ecology, Faculty of Science, Charles University, Prague, Czechia
- 10Department of Computer Science, Aberystwyth University, Aberystwyth, United Kingdom
Measurements of albedo are a prerequisite for modeling surface melt across the Earth's cryosphere, yet available satellite products are limited in spatial and/or temporal resolution. Here, we present a practical methodology to obtain centimeter resolution albedo products with accuracies of ±5% using consumer-grade digital camera and unmanned aerial vehicle (UAV) technologies. Our method comprises a workflow for processing, correcting and calibrating raw digital images using a white reference target, and upward and downward shortwave radiation measurements from broadband silicon pyranometers. We demonstrate the method with a set of UAV sorties over the western, K-sector of the Greenland Ice Sheet. The resulting albedo product, UAV10A1, covers 280 km2, at a resolution of 20 cm per pixel and has a root-mean-square difference of 3.7% compared to MOD10A1 and 4.9% compared to ground-based broadband pyranometer measurements. By continuously measuring downward solar irradiance, the technique overcomes previous limitations due to variable illumination conditions during and between surveys over glaciated terrain. The current miniaturization of multispectral sensors and incorporation of upward facing radiation sensors on UAV packages means that this technique could become increasingly common in field studies and used for a wide range of applications. These include the mapping of debris, dust, cryoconite and bioalbedo, and directly constraining surface energy balance models.
1. Introduction
The seasonal and interannual variability of melt on glaciers and ice sheets is primarily driven by the absorption of shortwave radiation at the surface (Braithwaite and Olesen, 1990; Munro, 1990; Arnold et al., 1996; Brock et al., 2000; van den Broeke et al., 2008). Albedo modulates the fraction of absorbed shortwave radiation and is known to be highly variable in space and time (e.g., Cutler and Munro, 1996; Jonsell et al., 2003; Klok et al., 2003). Quantifying albedo accurately and with high spatiotemporal resolution, is therefore critical for accurately determining the surface mass balance of glaciers and ice sheets (Gardner and Sharp, 2010). Despite this, patterns and dynamics of surface albedo remain one of the most prominent uncertainties in energy balance modeling (Hock, 2005; Noël et al., 2015).
Traditionally, energy balance models have relied on in-situ measurements, satellite imagery or numerical modeling for boundary condition albedo fields (e.g., Reijmer et al., 1999; van de Wal et al., 2005; Gardner and Sharp, 2010; van den Broeke et al., 2011; van As et al., 2012; Fettweis et al., 2013; Noël et al., 2015). However, the limitations of these methods, such as unrepresentative point measurements, coarse satellite pixel resolution, and incomplete representation of bare ice albedo in models, have motivated the development of new observation techniques. One such technique involves retrieving albedo from digital camera imagery (e.g., Corripio, 2004; Rivera et al., 2008; Dumont et al., 2011; Garvelmann et al., 2013; Rippin et al., 2015; Ayala et al., 2016). But while these approaches appear promising they range in complexity and suffer from large errors. For example, the relatively simple technique described by Rippin et al. (2015) for estimating an “albedo proxy” from the pixel values of RGB orthomosaics, likely suffers from high errors because, amongst other things, it does not account for variable illumination conditions. At the other extreme, Dumont et al. (2011) presented a theoretically rigorous approach for calculating albedo from oblique digital image pixels using a radiometrically calibrated camera, a bidirectional reflectance distribution function (BRDF) of snow and ice, a parametric transmittance model for downward spectral radiation, and a radiative transfer model for narrowband to broadband conversions. Although comprehensive, their methodology fails to retrieve robust albedo measurements under clouds and relies on assumptions about atmospheric transmittance and reflectance anisotropy of ice and snow, both of which are difficult to model or measure.
Accordingly, the primary aim of this study is to describe a technique for determining the albedo of snow and ice surfaces at high spatiotemporal resolution (i.e., centimeter to decimeter pixel resolution) and accuracy (i.e., ±5% or better) using a consumer-grade digital camera mounted on an unmanned aerial vehicle (UAV). The technique aims to be reproducible and practical under rapidly changing illumination conditions such as those commonly experienced in glaciated regions. The application of this method is demonstrated using digital images acquired by a UAV over the Kangerlussuaq sector of the Greenland Ice Sheet between June and July 2015. The accuracy of the albedo product is validated with near-simultaneous ground-based albedo measurements and the MOD10A1 satellite albedo product.
2. Methods
2.1. Assumptions
Estimating albedo from digital image pixels requires assumptions regarding the spectral reflectance of ice and snow and its relationship with the digital numbers of the image acquired by the camera. Our technique implicitly assumes that visible band (400–700 nm) digital imagery accurately captures the reflectance variability of surface types commonly found in glacier ablation areas. We evaluate this assumption by comparing albedo derived from the digital images to that obtained by a pair of Kipp & Zonen CM3 optical pyranometers (Section 3.3). We note that, at least for bare ice surfaces and snow with uniform grain size, most of the variability in reflected radiation lies in the visible band of the shortwave spectrum, between 350 and 695 nm (Wiscombe and Warren, 1980; Dozier et al., 1981; Cutler and Munro, 1996; Corripio, 2004) where a standard consumer-grade digital camera is most sensitive (e.g., Figure 1) (Jiang et al., 2013). In contrast, bare ice and snow albedo with uniform grain size does not display high variability in the near-infrared wavelengths (695–2,800 nm) (Warren and Wiscombe, 1980; Cutler and Munro, 1996).
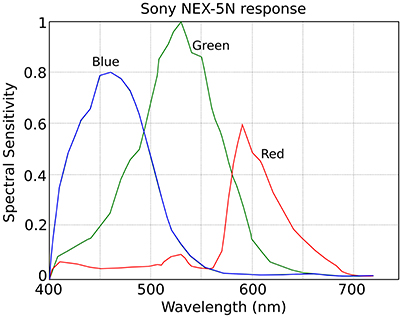
Figure 1. Spectral sensitivity of Sony NEX-5N digital camera (adapted from Jiang et al., 2013).
We also assume there is a direct relationship between the digital numbers of the camera image, which represent brightness, and surface reflectance. We argue this assumption can be justified if the camera is suitably calibrated and corrected using the methodology presented in this study. Furthermore, previous studies have demonstrated that it is possible to retrieve albedo from digital images with accuracies of ±10% or less (e.g., Corripio, 2004; Rivera et al., 2008; Dumont et al., 2011; Garvelmann et al., 2013; Ayala et al., 2016). Based on these assumptions, a procedure (Figure 2) to obtain and process imagery suitable for conversion to albedo is described in Sections 2.2–2.6.
2.2. Procedure Summary
Here, an example instrument configuration is outlined for obtaining albedo data using digital camera imagery and broadband pyranometers mounted on a UAV. Instruments include a standard consumer-grade digital camera, a pair of broadband silicon pyranometers and a white reference target (specific instruments used in our application are described in Section 3). Digital images of the white Teflon reference target, assumed to be a near-Lambertian reflector, were used to convert downward radiation measured by the upward facing pyranometer into digital numbers. Because the reflected radiation measured by the downward facing pyranometer is used to correct the digital images, both sensors were mounted in close proximity to each other in order to minimize the differences between their respective ground footprints. The instruments may be mounted on a multi-rotor UAV, but they could equally be mounted on a fixed-wing UAV, kite, blimp (e.g., Carrivick et al., 2013), fiberglass rod (e.g., van der Hage, 1992), step-ladder or other handheld device depending on the required resolution and spatial extent of the investigation.
2.3. Broadband Pyranometers
Upward and downward hemispherical reflectance were measured using broadband pyranometers, the ratio of which provides an estimate of the surface albedo. Downward radiation may be measured at a fixed ground station but if illumination conditions are rapidly changing, perhaps due to cloud cover, it may be more accurate to measure downward radiation from the aerial platform. In this case, we recommend using silicon pyranometers due to their low weight (~100 g per sensor) and rapid response time of 1 ms. The pyranometers must be precisely leveled with a clear view of the sky or surface to ensure accurate measurements.
The disadvantage of silicon pyranometers is that they are only sensitive to wavelengths between 300 and 1,100 nm, which excludes some of the solar energy contributing to the surface energy balance. We evaluate this source of uncertainty by comparing albedo derived from a pair of Apogee silicon pyranometers (https://www.campbellsci.com.au/sp-110) with albedo obtained by a pair of Kipp & Zonen CM3 optical pyranometers. The Kipp & Zonen pyranometers are based on carbon black thermopiles which have a flat spectral response for wavelengths between 300 and 2,800 nm and an accuracy of 2%. Comparison between the two sensors was made by simultaneously sampling 25 surfaces with an albedo of between 0.11 and 0.64 in a 20 × 20 m study area. The instruments were mounted on a 1 m aluminum rod and held 0.5 m above each surface for 1 min. Albedo was calculated as the mean of five 20 s samples. At this height, the pyranometers have a ground footprint of around 5.5 m in diameter. A root-mean-square difference (RMSD) of 3.6% between the two instruments justified that silicon pyranometers can accurately measure albedo of ice sheet surfaces between 0.11 and 0.64.
The Apogee silicon pyranometers were factory-adjusted to account for their partial spectral sensitivity under standard clear-sky atmospheric conditions. Therefore the upward facing pyranometer has an uncertainty of 5%, which includes a cosine-response error of 2% at solar zenith angles of 45°. The cosine-response error increases as solar zenith angle increases so we recommend acquiring measurements as close as possible to solar noon.
2.4. Digital Images
The digital images were acquired in RAW format with fixed exposure, ISO, and aperture. These settings are necessary to preserve linearity between RGB digital numbers acquired by the camera and the number of photons hitting the sensor. We recommend a relatively fast shutter speed to minimize image blur (when mounted on a moving UAV) and a low ISO and F-number to ensure that pixels do not saturate. Most software cannot read the RAW images so they should be converted to lossless 16-bit TIFF images using RAW decoding software such as dcraw (http://cybercom.net/~dcoffin/dcraw/) (Figure 2). When converting the RAW images it is important to use a fixed white level, or balance, and avoid using a gamma correction to ensure the reflectance histogram is not altered and the RGB digital numbers remain directly proportional to the brightness of the surface (Lebourgeois et al., 2008). A gamma correction is a non-linear function used to convert between the brightness measured by the camera's sensor and the output image pixel. In this study, we were only interested in the ratio between the total reflected and downward shortwave radiation and so the arithmetic mean of each pixel's RGB values was calculated.
Image brightness attenuation away from the center, vignetting, is inherent to all consumer-grade digital imagery and can be corrected by subtracting a vignette mask from the original image (Goldman, 2010). The vignette mask can be calculated by fitting a third-order polynomial function to multiple Gaussian smoothed images (e.g., Lebourgeois et al., 2008). The mean vignette polynomial function can then be applied to correct all the images from the survey. Finally, the geometric distortion of the images should be corrected to ensure that each image pixel represents the same ground sampling distance. We used Agisoft Lens 0.4.0 software and an on-screen checker board to automatically determine the camera calibration parameters and correct for this distortion. After vignette and geometric correction, the digital numbers of every pixel in the image are directly comparable.
2.5. Digital Image Illumination Correction
The radiation recorded by an image pixel is not only related to the reflectance of the surface, but also to the downward radiation. The digital numbers in an image can be corrected for variations in illumination by including a leveled white Teflon reference target in every digital image (e.g., Hakala et al., 2010). An illumination corrected image can be produced from the ratio between the digital numbers of the ice surface pixels and the digital numbers of the white reference target, which are a proxy for downward solar irradiance. After applying an illumination correction, the digital numbers of pixels are comparable between images including those obtained under very different weather or illumination conditions.
If survey area is large, it may not be possible to include a white reference target in every image. Therefore, to facilitate the correction of UAV acquired imagery there is a need to quantify the relationship between downward radiation measured by an upward facing pyranometer and the RAW digital numbers of a white reference target. If linearity between the reflectance of the surface and digital numbers of the RAW images is preserved, then the relationship can be statistically represented by an orthogonal distance linear regression.
An example of this relationship between the digital numbers of a white reference target obtained by a Sony NEX-5N camera in RAW mode from the ground against the downward radiation measured by a static, upward facing silicon pyranometer with a clear sky view is presented (Figure 3). Here, the orthogonal distance regression fits the data well with a R2 of 0.96 and an RMSD of 7.8%. The slope and intercept of the linear regression can subsequently be used to convert the downward radiation measured by the pyranometer to digital numbers. An illumination corrected image is then produced from the ratio between pyranometer to digital numbers.
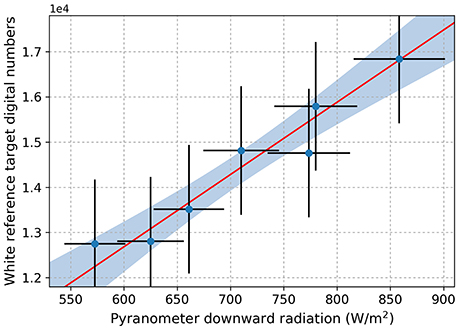
Figure 3. The relationship between the downward radiation measured by a broadband pyranometer and the digital numbers of a white reference target.
2.6. Digital Image Albedo Calibration
The final stage in the workflow is to calibrate the image using coincident measurements of albedo obtained from upward and downward facing pyranometer measurements. Snow and ice reflect incoming radiation anisotropically such that a nadir measurement of reflectance actually underestimates albedo by between 1 and 5% between 530 and 610 nm (i.e., the visible band) (Knap and Reijmer, 1998; Klok et al., 2003). The pyranometers account for the reflectance anisotropy of snow and ice because they measure hemispherical reflectance so can be used to correct the bidirectional reflectance measurements of the digital camera. This was achieved by multiplying the image pixel numbers by a factor calculated by dividing the mean pixel value of the illumination-corrected image by the albedo recorded by the pyranometers.
This step introduces uncertainty since the rectangular footprint of the digital image does not completely coincide with the circular footprint of the downward facing pyranometer (Figure 4). We overcome this issue by comparing the mean image pixel within the circular pyranometer footprint to the mean image pixel outside the pyranometer footprint for 300 representative sample images obtained from 600 m above the surface of the ablation area of the Greenland Ice Sheet. The silicon pyranometer has a circular FOV of approximately 60° whereas the Sony NEX-5N camera, used to test this uncertainty, has a rectangular FOV of 53.1 by 73.7°. The significant, strong positive correlation (R2 = 0.98; p < 0.01) between the mean pixel value inside and outside the pyranometer footprint, with an RMSD of 1.2%, demonstrates that the downward facing pyranometer can be used to correct the digital images over length scales in the order of hundreds of meters (Figure 4). We note that this relationship may not hold when there is a spatial trend at the scale of the image or extreme heterogeneity in the surface being measured.
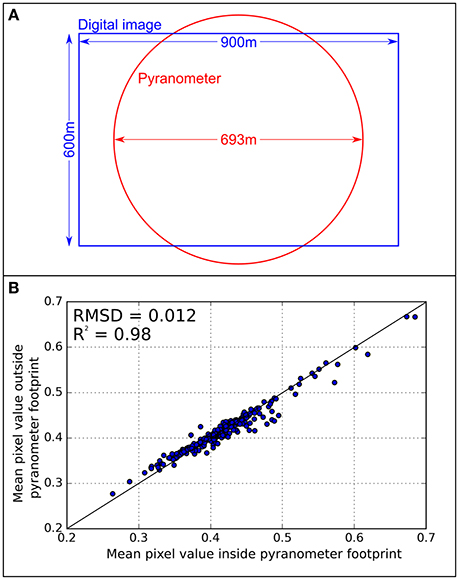
Figure 4. (A) Footprint difference between the downward facing pyranometer and digital camera. (B) Relationship between image pixels inside and outside the pyranometer footprint are well correlated.
3. Case Study: Kangerlussuaq Sector of the Greenland Ice Sheet
Our methodology is demonstrated using 7,378 digital camera images acquired from a fixed-wing UAV flow over the K-sector of the Greenland Ice Sheet between June and July 2015. We refer to this albedo product as “UAV10A1.” UAV10A1 covers Isunguata Sermia, herein called UAV10A1-Isunguata, and two regions of the ice sheet referred to as UAV10A1-S6 and UAV10A1-Behar (Figure 8). UAV10A1-S6 and UAV10A1-Behar are so-called because of their proximity to the weather station at Site 6 on the K-transect (van de Wal et al., 2005) and a large supraglacial river informally named Rio Behar described in Smith et al. (2015), respectively.
3.1. Vehicle and Instrument Set-up
The fixed-wing UAV used in this study was described by Ryan et al. (2015) and based on a Skywalker X8 airframe constructed from expanded polypropylene foam with a wingspan of 2.12 m (Figure 5). Autonomous control was provided by a Pixhawk autopilot module which utilizes an L1 GPS, two inertial measurement units (IMUs), a compass, and a barometer. A 30 Ah 14.4V lithium-ion battery pack provides power for the 715W electromagnetic motor, two servos, the receiver and the autopilot module. The UAV cruising speed is regulated by a digital differential airspeed sensor and targets 54 km h−1. The weight of the UAV without the sensor package was 4.79 kg. The sensor package weighs 0.715 kg and includes a Sony NEX-5N digital camera (0.345 kg), a Hobo micro data logger stripped of its case (0.075 kg) and powered externally, two Hobo pyranometers (0.170 kg), a temperature/humidity sensor (0.065 kg) and a GoPro HERO4 (0.060 kg). In this configuration, the UAV had an all-up-weight 5.5 kg and a 140 km range dependent on conditions.
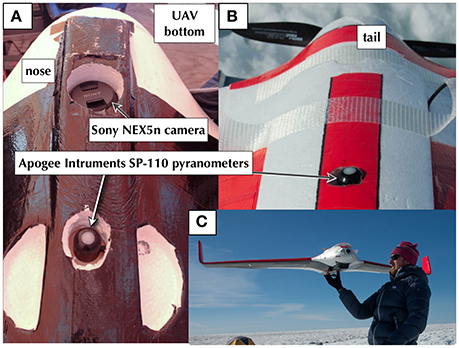
Figure 5. The UAV is equipped with (A) a downward facing digital camera and pyranometer, and (B) an upward facing pyranometer. (C) The UAV before launch.
The upward and downward facing Hobo silicon pyranometers were aligned within the airframe (Figure 5) so that they were level when the UAV was in stable flight. Errors due to instrument tilting were limited by omitting radiation data recorded when the pitch or roll of the UAV exceeded 3°. According to Bogren et al. (2016), this introduces a maximum error of 8.1%. At a flight altitude of 600 m above the surface, a 60° FOV gives the pyranometer an estimated 700 m diameter footprint. The pyranometers were sampled by a micro data logger at 1 Hz and, before each flight, the data logger was synchronized to GPS time to ensure that upward and downward shortwave radiation data coincided with GPS and attitude data recorded by the PixHawk in post-processing.
Digital images were obtained by a downward facing Sony NEX-5N digital camera mounted in the UAV airframe (Figure 5). The camera was pre-set for a fixed exposure of 1/1,000 s, ISO 100 and F-stop of 8. The camera has a 16 mm fixed-focus lens (equivalent to 24 mm for a 35 mm film camera) which gives an FOV of 53.1 by 73.7° and yields a ground footprint of approximately 900 × 600 m at an altitude of 600 m above the ice surface. Vignetting was as high as 17.6% at the corners of the images and was corrected with a vignette correction mask (Section 2.4). Each image was tagged with the geographic location and attitude data recorded by Pixhawk's two IMUs and L1 GPS. The camera was triggered every 50 m of horizontal displacement (about every 3 s) which provided a forward overlap of 92%.
Gridded flight patterns over the ice sheet were planned and uploaded to the UAV using the Mission Planner ground station software (http://ardupilot.org/planner/). The UAV was programmed to maintain a constant altitude above sea level during missions and was targeted at 600 m above the surface. For the purpose of our study, this altitude was deemed an optimal trade-off between ground sampling distance and coverage. The distance between flight lines was set to 300 m which provided a 50% horizontal or side overlap between the aerial images.
3.2. Orthomosaic Production
The images were corrected and calibrated using the workflow described in Section 2 and mosaicked using Agisoft PhotoScan 1.3.0, herein PhotoScan, to produce UAV10A1. The images were imported into PhotoScan and automatically aligned based on the georeferencing information. This approach avoids the need for GPS-surveyed ground control points and reduces processing time because the software pre-aligns the images instead of performing global point matching to achieve accurate image alignment. The orthomoasaics were produced in the software's “mosaic” mode, meaning that the pixels in the centers of the image were preferentially used to provide the output pixel value. The orthomosaics were nearest-neighbor resampled to a pixel resolution of 20 cm and exported as GeoTIFFs for analysis.
3.3. Validation and Comparison
Validation of UAV10A1 was undertaken by comparison with surfaces sampled by the Kipp & Zonen CM3 pyranometers (Section 2.3). Nine of the twenty five surfaces were identified in three images acquired when the UAV was close to the ground and a comparison, made by sampling pixels within a 5.5 m diameter circle in the images, indicates that the two measurements compared well with an RMSD of 4.9% and a bias of 4.1% (Figure 6). The slight underestimation of CM3 in comparison to UAV10A1 may be partly explained by the obscuring of reflected radiation by the observer holding the CM3 on the meter long rod (e.g., van der Hage, 1992). The difference between the range of wavelengths measured by the CM3 pyranometers (300–2,800 nm) and digital camera (300–700 nm) may be a source of error. For example, changes in snow grain size have less effect on visible wavelength albedo than in the near-infrared wavelengths (Wiscombe and Warren, 1980). The visible band digital camera is not sensitive to albedo variation in the near-infrared which likely introduces error when compared to CM3 albedo. Generally, UAV10A1 measures albedo of the ice sheet surface adequately between 0.11 and 0.64, and within our target accuracy of ±5%.
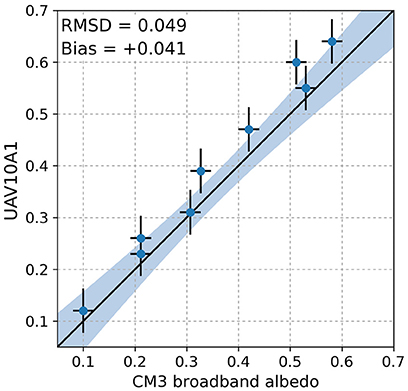
Figure 6. Scatter plot showing relationship between CM3 broadband albedo with albedo of pixels derived from the digital image albedo products.
UAV10A1 was also compared to the satellite-derived MOD10A1 Collection 6 daily albedo product (300–3,000 nm) obtained from the National Snow and Ice Data Center (NSIDC) (Hall and Riggs, 2016). The daily temporal resolution of MOD10A1 reduces uncertainties that may arise due to changes in ice surface albedo between data acquisition. MOD10A1. The value of each MOD10A1 pixel represents the best single albedo observation in the day, based on cloud cover and viewing and illumination angles (Stroeve et al., 2006). Retrieving the time of the MOD10A1 albedo observation was not possible and so we note that there may be up to a couple of hours difference between UAV and satellite data acquisition which may lead to discrepancies between the two data sources if there is a significant diurnal albedo cycle caused by changing solar azimuth and zenith angles or ice and snow metamorphosis during the day (Stroeve et al., 2006; Bogren et al., 2016). Comparison between both products was achieved by mean resampling UAV10A1 to the same pixel resolution as the MOD10A1 product (~463 m) using the average pixel value of UAV10A1. Both products were then clipped to the same extent and 347 cloud-free samples were compared. The mean RMSD between the two albedo products was 3.7% which is within the 6.7–7.0% estimated uncertainty of the MOD10A1 product (Figure 7) (Stroeve et al., 2006; Wang et al., 2011; Ryan et al., 2016).
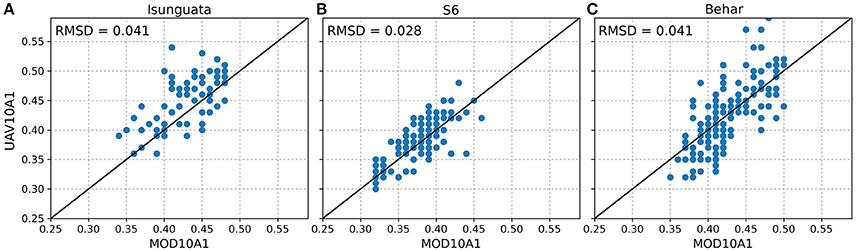
Figure 7. (A) Scatter plot showing the relationship between MOD10A1 and UAV10A1-Isunguata. (B) Same as (A) but for UAV10A1-S6. (C) Same as (A) but for UAV10A1-Behar.
3.4. High-Resolution Albedo Product
3.4.1. UAV10A1-Isunguata
UAV10A1-Isunguata is a 130 km2 map that covers the tongue of the land-terminating glacier in West Greenland approximately 26 km north-east of the town of Kangerlussuaq (Figures 8A,B). The most noticeable feature of UAV10A1-Isunguata is the variation in surface topography between highly crevassed and the lower relief, almost flat areas. The crevassed regions generally occur near the margin of the glacier presumably where the subglacial topography is steeper and longitudinal and transverse strain gradients are high. The surface topography appears to dominate the spatial albedo variability with crevassed areas having a lower mean albedo (0.39) than low-relief topography (0.50) (Figures 9A,B). Crevassed regions have a lower albedo because rugged topography increases the probability of a shading effect when radiation is reflected between surfaces and trapped in troughs (Pfeffer and Bretherton, 1987; Cutler and Munro, 1996). Additionally, crevasse troughs may appear to be darker than the peaks and plateaus of the bare ice because of the possibility of liquid water storage due to enhanced radiative melting in the depressions (Cathles et al., 2011). The slopes and troughs of the crevasses could also facilitate the transport and residence of impurities such as dust or ice algae. These impurities absorb downward radiation and darken the bare ice in the depressions (Figure 9B).
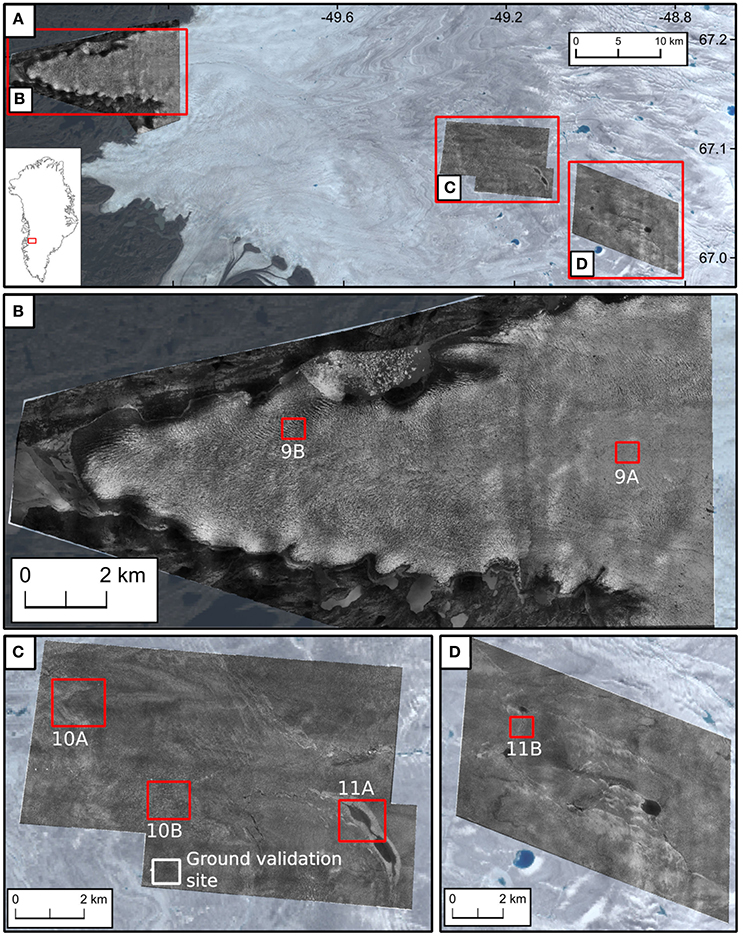
Figure 8. (A) Map showing locations of the three high resolution albedo products (UAV10A1) in West Greenland. (B) UAV10A1-Isunguata, a land-terminating outlet glacier. (C) UAV10A1-S6, an ablation area site at around 1,100 m a.s.l. (D) UAV10A1-Behar, an ablation area site between 1,200 to 1,350 m a.s.l. which encompasses the catchment of large supraglacial river informally known as Rio Behar.
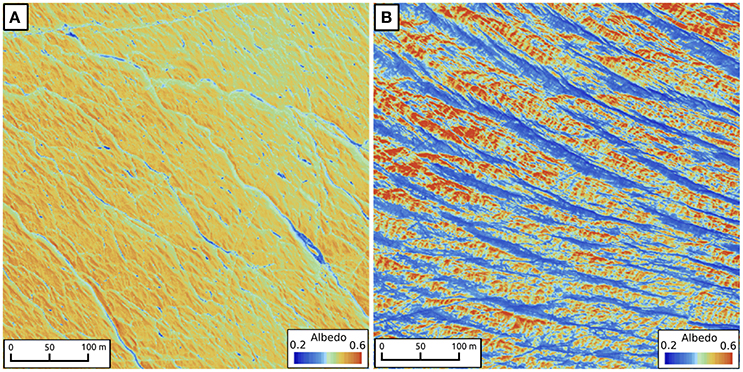
Figure 9. (A) Relatively clean bare ice with cryoconite holes potted on the surface. (B) Crevassed area of Isunguata Sermia.
Supraglacial meltwater ponds and channels also show low albedo and occupy areas of tens to hundreds of meters squared. Supraglacial meltwater flowing over relatively clean ice has been previously shown to have an albedo of between 0.19 and 0.26 (Ryan et al., 2016). But point sampling of surface water on Isunguata Sermia reveals that many streams have an albedo of between 0.09 and 0.12 due to the presence of underlying cryoconite deposits (Hodson et al., 2007) (Figure 9A). The cryoconite deposits cover the majority of the channel and pond beds and lower the albedo of the observed surface. Cryoconite holes, with a typical albedo of 0.11 when viewed from nadir, are also apparent across Isunguata Sermia, as evinced by the small quasi-circular black spots which are frequently observed on the bare ice (Figure 9A) (Cook et al., 2015). Cryoconite granules absorb solar radiation and melt down into the ice until they are in radiative and thermodynamic equilibrium (Takeuchi et al., 2001; MacDonell and Fitzsimons, 2008; Langford et al., 2010). Although the holes have very low albedo when seen from directly above, the cryoconite may become hidden at non-nadir viewing angles and from non-zenith solar illumination (Bøggild et al., 2010).
3.4.2. UAV10A1-S6
UAV10A1-S6 is a 70 km2 map between 1,060 and 1,200 m a.s.l. which includes the Institute for Marine and Atmospheric (IMAU), Utrecht University weather station at S6 (Figure 8C). The region is situated in an area of particularly low albedo which has been defined as the “dark zone” (Wientjes and Oerlemans, 2010; Wientjes et al., 2012; Shimada et al., 2016). UAV10A1-S6 is predominantly characterized by a low-relief surface topography and variable bare ice albedo ranging between 0.27 and 0.58. The variable bare ice albedo appears to cause the striking foliation patterns in the ice which are also observed in satellite imagery (Figure 10A) (Wientjes and Oerlemans, 2010; Wientjes et al., 2012; Shimada et al., 2016). Possible causes of variable bare ice albedo include liquid water content of the ice and/or the presence of light absorbing impurities (Greuell, 2000; Wientjes et al., 2012; Lüthi et al., 2015).
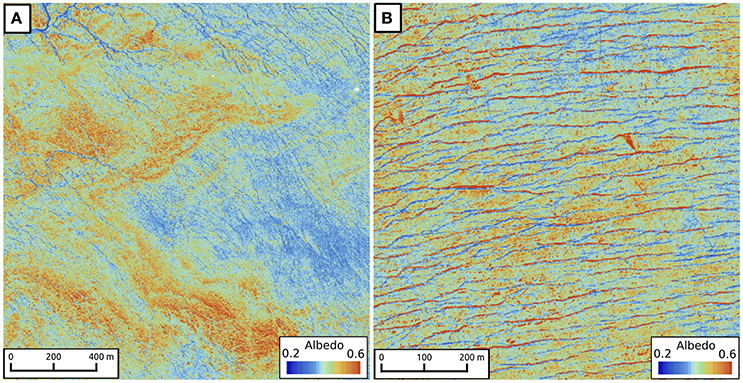
Figure 10. (A) Foliation patterns in the dark region. (B) Fractures filled with remnant snow cornices.
UAV10A1-S6 is dominated by the presence of bare ice, but remnant snow can persist within ice fractures and supraglacial channel incisions exhibiting local albedo of between 0.65 and 0.71. The likely persistence of snow in these locations is due to a thicker snowpack and the redistribution of snow by the wind (Figure 10B) (van den Broeke et al., 2008). A 0.6 km2 supraglacial lake in the south-west corner of UAV10A1-S6 (Figure 11A) is characterized by albedo ranging from 0.17 to 0.28. The difference in the lake's albedo is a consequence of varied water depth and the bed reflectance. At the lake's edge, slabs of bright ice are visible which are interpreted as grounded lake ice and a possible lake drainage event. Lake ice forms annually as a floating layer when the temperature of the water drops below freezing. It has therefore existed for less than a year and appears relatively impurity free with a mean albedo of 0.61.
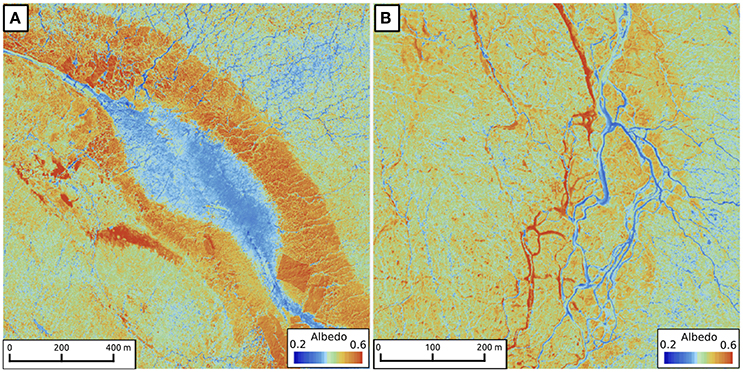
Figure 11. (A) Supraglacial lake with lake ice grounded on the shore. (B) Braided supraglacial rivers.
3.4.3. UAV10A1-Behar
UAV10A1-Behar is an 80 km2 map 10 km east of the S6 weather station between 1,200 and 1,350 m a.s.l. UAV10A1-Behar is at the edge of the dark zone but still within the ablation area (Figure 8D). UAV10A1-Behar is characterized by similar bare ice variability as UAV10A1-S6 but the former contains four supraglacial lakes and a more active drainage system. Large braided and single channel meltwater streams with widths of up to 25 m are common features in this area and have albedo values between 0.16 and 0.24, similar to supraglacial lakes (Figure 11B). UAV10A1-Behar concurs with recent studies which reveal that the channels are characterized by dendritic drainage patterns and ultimately terminate in a large moulin located at 67.05° N, −49.03° E (e.g., Yang and Smith, 2013; Smith et al., 2015; Yang et al., 2016). Although the lakes ephemerally store large fluxes of water over weekly to seasonal time-scales (Fitzpatrick et al., 2014), all the lakes in UAV10A1-Behar have laterally draining outlet streams showing that these features provide little obstruction to meltwater flow through the supraglacial hydrologic system (Smith et al., 2015).
4. Discussion
Developments in economical remote sensing tools and the rapidly evolving field of UAVs are enabling glaciologists to obtain surface topographic and reflectance data at unprecedented spatial and temporal resolutions (Carrivick et al., 2013, 2016; Bhardwaj et al., 2016). In particular, structure-from-motion software has facilitated the collection of topographic data using consumer-grade digital cameras (e.g., Westoby et al., 2012; Whitehead et al., 2013; Immerzeel et al., 2014; Tonkin et al., 2014; Ryan et al., 2015; Smith et al., 2016). More recently, there has been an interest in quantifying the reflectance of surfaces using similarly low-cost sensors (e.g., Hakala et al., 2010; Di Mauro et al., 2015; Rippin et al., 2015). This study has shown that it is possible to obtain albedo measurements over ice sheet surfaces with high spatial resolution and accuracy using a standard consumer-grade RGB digital camera mounted on a UAV.
The technique described has several advantages over current methods to generate background albedo fields. We overcome the commonly identified, but little addressed, challenge of variable illumination during surveying (e.g., Dumont et al., 2011; Rippin et al., 2015). Cloud cover is typical over glaciated regions and, during surveys lasting more than a couple of hours, solar zenith angles can change significantly. By continuously measuring downward radiation on the UAV, we account for these changes and can obtain high-quality reflectance data regardless of the meteorological conditions. By using the hemispherical reflectance measured by the downward facing silicon pyranometer, we account for the anisotropic reflectance of the surface and correct the bidirectional reflectance recorded by the digital images. The result is that we measure albedo with accuracies of better than ±5%, regardless of changing meteorological conditions. Although the described method relies on data collection during low solar zenith angles, to minimize the cosine response errors of pyranometer and specular reflection, an albedo field can be generated at short (i.e., daily) intervals allowing unprecedented mapping of evolving snow and ice surfaces over the melt-season. The technique and workflow outlined in this study should therefore have interest for users of UAVs including the latest proximal remote sensing solutions, such as MicaSense's RedEdge (https://www.micasense.com/rededge/) and Sequoia (https://www.micasense.com/sequoia/) multispectral cameras, which include upward facing radiation sensors.
The visible band digital camera detects albedo variations which occur between 400 and 700 nm. However, UAV10A1 is not sensitive to albedo variations, such as those caused by changes in snow grain size, which only have an effect on albedo in the near-infrared wavelengths (Wiscombe and Warren, 1980). Improvements to the method presented would be to incorporate new instruments that are sensitive to a wider portion of the electromagnetic spectrum and are hence able to detect changes in surface reflectance at wavelengths shorter and longer than that recorded by standard digital cameras confined to the visible band (e.g., MacArthur et al., 2014; Von Bueren et al., 2015). Both the weight and cost of these sensors, such as Tetracam's ADC Snap (Tetracam, California, USA) and MicaSense's RedEdge and Sequoia (MicaSense, Seattle, USA), is decreasing and their use could become increasingly common in glaciological field studies or any other field that rely on precise high-resolution near-surface albedo data.
The methodology and UAV application described in this study can be used to evaluate and improve the parameterization of albedo in surface mass balance models. At a regional scale (e.g., the entire Greenland Ice Sheet), contemporary models either prescribe a background bare ice albedo using MODIS data (e.g., van Angelen et al., 2012; Noël et al., 2015) or simulate it as a function of melt (e.g., Zuo and Oerlemans, 1996; Lefebre et al., 2003; Alexander et al., 2014). However, these schemes may not adequately represent the sub-grid albedo variability of the ice sheet surface, leading to discrepancies between the modeled and observed surface mass balance. The high spatial resolution of UAV10A1 therefore presents an opportunity to assess the representativeness of albedo parameterizations and determine a grid scale at which ice albedo is sufficiently represented.
Another application of the technique is in the collection of UAV-based albedo data over areas where surface instruments cannot be deployed. Access to glaciers and ice sheets is often hindered by steep slopes, crevasses, and impassable meltwater channels. But small UAVs can be deployed to survey these areas without risk to the observer. UAV10A1 may also be used to investigate the robustness of in-situ albedo observations for validating satellite-derived albedo. The calibration and validation of satellite albedo products relies upon in-situ measurements but direct comparison between the two is only valid when the footprint of the in-situ measurement is either the same as the corresponding satellite image pixel or when the surface is sufficiently homogeneous at the scale of both the in-situ measurement and satellite data pixel (Román et al., 2009, 2013). UAV10A1 provides an opportunity to assess whether the spatial heterogeneity of ice sheet and glacier surfaces is captured by the in-situ measurement footprint and whether direct comparison of such a “ground truth” with satellite-derived albedo data is fully justified.
5. Conclusion
The technique presented in this study demonstrates that an accurate (uncertainty less than 5%), high resolution and spatially continuous albedo product can be derived using a combination of a digital camera, broadband pyranometers, and UAV platform. This technique is reproducible and provides a practical approach for overcoming the limitations of previous studies caused by variable illumination. The method is demonstrated using measurements collected by a fixed-wing UAV across the Kangerlussuaq sector of the Greenland Ice Sheet in the 2015 melt-season. Validation of the albedo product, UAV10A1, indicates it has an RMSD of 4.9% in comparison to ground-based albedo measurements and an RMSD of 3.7% when compared to MOD10A1. UAV10A1 has numerous applications such as evaluating the accuracy of albedo fields used in energy balance modeling and understanding spatial and temporal variability of ice sheet albedo. The miniaturization of multispectral sensors and incorporation of upward facing radiation sensors on UAV packages means that this technique could become increasingly common in glaciological field studies and further developed to measure a wider range of wavelengths.
Author Contributions
JR, AH, JB, and NS conceived the study. NS, JR, SB, and AH designed, tested and built the UAV used for data collection. JR and JB led data acquisition. JR developed and implemented the technique and wrote the article. AH provided supervision. JB collected handheld CM3 albedo measurements. AH, JB, MC, LP, AR, LS, MS, KC, TH, CJ, and SD provided support in the field and contributed to acquisition of data. AH, JB, SB, AE, SD, JC, and TI gave conceptual and technical advice and supported the interpretation of data. All authors edited and critically revised the article.
Funding
Fieldwork was supported by Dark Snow Project crowd funding (http://www.darksnow.org/), a grant from the Leonardo DiCaprio Foundation and an Aberystwyth University Research Fund. JR was supported by an Aberystwyth University Doctoral Career Development Scholarship (DCDS). AH gratefully acknowledges support from the Centre for Arctic Gas Hydrate, Environment and Climate, funded by the Research Council of Norway through its Centres of Excellence (No. 223259). AR was funded by NASA grant NNX14AH93G.
Conflict of Interest Statement
The authors declare that the research was conducted in the absence of any commercial or financial relationships that could be construed as a potential conflict of interest.
Acknowledgments
We thank Daniel Vegh and Leo Nathan who worked tirelessly building, repairing and operating the UAVs.
References
Alexander, P. M., Tedesco, M., Fettweis, X., Van De Wal, R. S. W., Smeets, C. J. P. P., and Van Den Broeke, M. R. (2014). Assessing spatio-temporal variability and trends in modelled and measured Greenland Ice Sheet albedo (2000-2013). Cryosphere 8, 2293–2312. doi: 10.5194/tc-8-2293-2014
Arnold, N. S., Willis, I. C., Sharp, M. J., Richards, K. S., and Lawson, W. J. (1996). A distributed surface energy-balance model for a small valley glacier. I. Development and testing for Haut Glacier d'Arolla, Valais, Switzerland. J. Glaciol. 42, 77–89. doi: 10.1017/S0022143000030549
Ayala, A., Pellicciotti, F., MacDonell, S., McPhee, J., Vivero, S., Campos, C., et al. (2016). Modelling the hydrological response of debris-free and debris-covered glaciers to present climatic conditions in the semiarid Andes of central Chile. Hydrol. Process. 30, 4036–4058. doi: 10.1002/hyp.10971
Bhardwaj, A., Sam, L., Martín-torres, F. J., and Kumar, R. (2016). UAVs as remote sensing platform in glaciology: present applications and future prospects. Remote Sens. Environ. 175, 196–204. doi: 10.1016/j.rse.2015.12.029
Bøggild, C. E., Brandt, R. E., Brown, K. J., and Warren, S. G. (2010). The ablation zone in Northeast Greenland: Ice types, albedos and impurities. J. Glaciol. 56, 101–113. doi: 10.3189/002214310791190776
Bogren, W. S., Burkhart, J. F., and Kylling, A. (2016). Tilt error in cryospheric surface radiation measurements at high latitudes: a model study. Cryosphere 10, 613–622. doi: 10.5194/tc-10-613-2016
Braithwaite, R. J., and Olesen, O. B. (1990). A simple energy-balance model to calculate ice ablation at the margin of the Greenland ice sheet. J. Glaciol. 36, 222–228. doi: 10.1017/S0022143000009473
Brock, B. W., Willis, I. C., Sharp, M. J., and Arnold, N. S. (2000). Modelling seasonal and spatial variations in the surface energy balance of Haut Glacier d'Arolla, Switzerland. Ann. Glaciol. 31, 53–62. doi: 10.3189/172756400781820183
Carrivick, J. L., Smith, M. W., and Quincey, D. J. (2016). Structure from Motion in the Geosciences. Chichester: John Wiley & Sons.
Carrivick, J. L., Smith, M. W., Quincey, D. J., and Carver, S. J. (2013). Developments in budget remote sensing for the geosciences. Geol. Today 29, 138–143. doi: 10.1111/gto.12015
Cathles, L. M., Abbot, D. S., Bassis, J. N., and MacAyeal, D. R. (2011). Modeling surface-roughness/solar-ablation feedback: application to small-scale surface channels and crevasses of the Greenland ice sheet. Ann. Glaciol. 52, 99–108. doi: 10.3189/172756411799096268
Cook, J., Edwards, A., Takeuchi, N., and Irvine-Fynn, T. (2015). Cryoconite: the dark biological secret of the cryosphere. Prog. Phys. Geogr. 40, 66–111. doi: 10.1177/0309133315616574
Corripio, J. G. (2004). Snow surface albedo estimation using terrestrial photography. Int. J. Remote Sens. 25, 5705–5729. doi: 10.1080/01431160410001709002
Cutler, P. M., and Munro, D. S. (1996). Visible and near-infrared reflectivity during the ablation period on Peyto Glacier, Alberta, Canada. J. Glaciol. 42, 333–340. doi: 10.1017/S0022143000004184
Di Mauro, B., Fava, F., Ferrero, L., Garzonio, R., Baccolo, G., Delmonte, B., et al. (2015). Mineral dust impact on snow radiative properties in the European Alps combining ground, UAV, and satellite observations. J. Geophys. Res. Atmospher. 120, 6080–6097. doi: 10.1002/2015JD023287
Dozier, J., Schneider, S. R., and McGinnis, D. F. Jr. (1981). Effect of grain size and snowpack water equivalence on visible and near-infrared satellite observations of snow. Water Resour. Res. 17, 1213–1221. doi: 10.1029/WR017i004p01213
Dumont, M., Sirguey, P., Arnaud, Y., and Six, D. (2011). Monitoring spatial and temporal variations of surface albedo on Saint Sorlin Glacier (French Alps) using terrestrial photography. Cryosphere 5, 759–771. doi: 10.5194/tc-5-759-2011
Fettweis, X., Franco, B., Tedesco, M., van Angelen, J. H., Lenaerts, J. T. M., van den Broeke, M. R., et al. (2013). Estimating the greenland ice sheet surface mass balance contribution to future sea level rise using the regional atmospheric climate model mar. Cryosphere 7, 469–489. doi: 10.5194/tc-7-469-2013
Fitzpatrick, A. A. W., Hubbard, A. L., Box, J. E., Quincey, D. J., Van As, D., Mikkelsen, A. P. B., et al. (2014). A decade (2002-2012) of supraglacial lake volume estimates across Russell Glacier, West Greenland. Cryosphere 8, 107–121. doi: 10.5194/tc-8-107-2014
Gardner, A. S., and Sharp, M. J. (2010). A review of snow and ice albedo and the development of a new physically based broadband albedo parameterization. J. Geophys. Res. Earth Surf. 115, 1–15. doi: 10.1029/2009JF001444
Garvelmann, J., Pohl, S., and Weiler, M. (2013). From observation to the quantification of snow processes with a time-lapse camera network. Hydrol. Earth Syst. Sci. 17, 1415–1429. doi: 10.5194/hess-17-1415-2013
Goldman, D. B. (2010). Vignette and exposure calibration and compensation. IEEE Trans. Patt. Anal. Mach. Intell. 32, 2276–2288. doi: 10.1109/TPAMI.2010.55
Greuell, W. (2000). Melt-water accumulation on the surface of the Greenland Ice Sheet: effect on albedo and mass balance. Geografiska Ann. Ser. A Phys. Geogr. 82, 489–498. doi: 10.1111/j.0435-3676.2000.00136.x
Hakala, T., Suomalainen, J., and Peltoniemi, J. I. (2010). Acquisition of bidirectional reflectance factor dataset using a micro unmanned Aaerial vehicle and a consumer camera. Remote Sens. 2, 819–832. doi: 10.3390/rs2030819
Hall, D. K., and Riggs, G. A. (2016). MODIS/Terra Snow Cover Daily L3 Global 500m Grid, Version 6. Boulder, CO: NASA National Snow and Ice Data Center Distributed Active Archive Center.
Hock, R. (2005). Glacier melt: a review of processes and their modelling. Progr. Phys. Geogr. 29, 362–391. doi: 10.1191/0309133305pp453ra
Hodson, A., Anesio, A. M., Ng, F., Watson, R., Quirk, J., Irvine-Fynn, T., et al. (2007). A glacier respires: quantifying the distribution and respiration CO2 flux of cryoconite across an entire Arctic supraglacial ecosystem. J. Geophys. Res. 112:G04S36. doi: 10.1029/2007JG000452
Immerzeel, W., Kraaijenbrink, P., Shea, J., Shrestha, A., Pellicciotti, F., Bierkens, M., et al. (2014). High-resolution monitoring of himalayan glacier dynamics using unmanned aerial vehicles. Remote Sens. Environ. 150, 93–103. doi: 10.1016/j.rse.2014.04.025
Jiang, J., Liu, D., Gu, J., and Süsstrunk, S. (2013). “What is the space of spectral sensitivity functions for digital color cameras?,” in What is the space of spectral sensitivity functions for digital color cameras?, (Florida: IEEE), 168–179.
Jonsell, U., Hock, R., and Holmgren, B. (2003). Spatial and temporal variations in albedo on storglacia¨ ren, sweden. J. Glaciol. 49, 59–68. doi: 10.3189/172756503781830980
Klok, E. J. L., Greuell, W., and Oerlemans, J. (2003). Temporal and spatial variation of the surface albedo of Morteratschgletscher, Switzerland, as derived from 12 Landsat images. J. Glaciol. 49, 491–502. doi: 10.3189/172756503781830395
Knap, W. H., and Reijmer, C. H. (1998). Anisotropy of the reflected radiation field over melting glacier ice: measurements in Landsat TM bands 2 and 4. Remote Sens. Environ. 65, 93–104. doi: 10.1016/S0034-4257(98)00015-7
Langford, H., Hodson, A., Banwart, S., and Bøggild, C. (2010). The microstructure and biogeochemistry of Arctic cryoconite granules. Ann. Glaciol. 51, 87–94. doi: 10.3189/172756411795932083
Lebourgeois, V., Bégué, A., Labbé, S., Mallavan, B., Prévot, L., and Roux, B. (2008). Can commercial digital cameras be used as multispectral sensors? A crop monitoring test. Sensors 8, 7300–7322. doi: 10.3390/s8117300
Lefebre, F., Gallée, H., VanYpersele, J., and Greuell, W. (2003). Modeling of snow and ice melt at ETH Camp (West Greenland): a study of surface albedo. J. Geophys. Res. 108:4231. doi: 10.1029/2001JD001160
Lüthi, M., Ryser, C., Andrews, L., Catania, G., Funk, M., Hawley, R., et al. (2015). Heat sources within the greenland ice sheet: dissipation, temperate paleo-firn and cryo-hydrologic warming. Cryosphere 9, 245–253. doi: 10.5194/tc-9-245-2015
MacArthur, A., Robinson, I., Rossini, M., Davis, N., and MacDonald, K. (2014). “A dual-field-of-view spectrometer system for reflectance and fluorescence measurements (piccolo doppio) and correction of etaloning,” in Proceedings of the Fifth International Workshop on Remote Sensing of Vegetation Fluorescence, (Paris), 22–24.
MacDonell, S., and Fitzsimons, S. (2008). The formation and hydrological significance of cryoconite holes. Prog. Phys. Geogr. 32, 595–610. doi: 10.1177/0309133308101382
Munro, D. S. (1990). Comparison of melt energy computations and ablatometer measurements on melting ice and snow. Arctic Alpine Res. 22, 153–162. doi: 10.2307/1551300
Noël, B., Van De Berg, W., Van Meijgaard, E., Kuipers Munneke, P., Van De Wal, R., and Van Den Broeke, M. (2015). Evaluation of the updated regional climate model RACMO2. 3: summer snowfall impact on the Greenland Ice Sheet. Cryosphere 9, 1831–1844. doi: 10.5194/tc-9-1831-2015
Pfeffer, W. T., and Bretherton, C. S. (1987). The effect of crevasses on the solar heating of a glacier surface. IAHS Publ. 170, 191–205.
Reijmer, C. H., Knap, W. H., and Oerlemans, J. (1999). The surface albedo of the Vatnajökull ice cap, Iceland: a comparison between satellite-derived and ground-based measurements. Boundary Layer Meteorol. 92, 123–143. doi: 10.1023/A:1001816014650
Rippin, D. M., Pomfret, A., and King, N. (2015). High resolution mapping of supra-glacial drainage pathways reveals link between micro-channel drainage density, surface roughness and surface reflectance. Earth Surf. Process. Landforms 40, 1279–1290. doi: 10.1002/esp.3719
Rivera, A., Corripio, J. G., Brock, B., Clavero, J., and Wendt, J. (2008). Monitoring ice-capped active Volćan Villarrica, southern Chile, using terrestrial photography combined with automatic weather stations and global positioning systems. J. Glaciol. 54, 920–930. doi: 10.3189/002214308787780076
Román, M. O., Gatebe, C. K., Shuai, Y., Wang, Z., Gao, F., Masek, J. G., et al. (2013). Use of in situ and airborne multiangle data to assess modis-and landsat-based estimates of directional reflectance and albedo. IEEE Trans. Geosci. Remote Sens. 51, 1393–1404. doi: 10.1109/TGRS.2013.2243457
Román, M. O., Schaaf, C. B., Woodcock, C. E., Strahler, A. H., Yang, X., Braswell, R. H., et al. (2009). The modis (collection v005) brdf/albedo product: assessment of spatial representativeness over forested landscapes. Remote Sens. Environ. 113, 2476–2498. doi: 10.1016/j.rse.2009.07.009
Ryan, J. C., Hubbard, A., Stibal, M., Box, J. E., and team, T. D. S. P. (2016). Attribution of greenland's ablating ice surfaces on ice sheet albedo using unmanned aerial systems. Cryosph. Discuss. 2016, 1–23. doi: 10.5194/tc-2016-204
Ryan, J. C., Hubbard, A. L., Box, J. E., Todd, J., Christoffersen, P., Carr, J. R., et al. (2015). UAV photogrammetry and structure from motion to assess calving dynamics at Store Glacier, a large outlet draining the Greenland ice sheet. Cryosphere 9, 1–11. doi: 10.5194/tc-2016-204
Shimada, R., Takeuchi, N., and Aoki, T. (2016). Inter-annual and geographical variations in the extent of bare ice and dark ice on the Greenland Ice Sheet derived from MODIS satellite images. Front. Earth Sci. 4:43. doi: 10.3389/feart.2016.00043
Smith, L. C., Chu, V. W., Yang, K., Gleason, C. J., Pitcher, L. H., Rennermalm, A. K., et al. (2015). Efficient meltwater drainage through supraglacial streams and rivers on the southwest Greenland ice sheet. Proc. Natl. Acad. Sci. U.S.A. 112, 1001–1006. doi: 10.1073/pnas.1413024112
Smith, M. W., Quincey, D. J., Dixon, T., Bingham, R. G., Carrivick, J. L., Irvine-Fynn, T. D., et al. (2016). Aerodynamic roughness of glacial ice surfaces derived from high-resolution topographic data. J. Geophys. Res. Earth Surf. 121, 748–766. doi: 10.1002/2015JF003759
Stroeve, J. C., Box, J. E., and Haran, T. (2006). Evaluation of the MODIS (MOD10A1) daily snow albedo product over the Greenland ice sheet. Remote Sens. Environ. 105, 155–171. doi: 10.1016/j.rse.2006.06.009
Takeuchi, N., Kohshima, S., and Seko, K. (2001). Structure, formation, and darkening process of albedo-reducing material (cryoconite) on a Himalayan glacier: a granular algal mat growing on the glacier. Arctic Antarctic Alpine Res. 33, 115–122. doi: 10.2307/1552211
Tonkin, T. N., Midgley, N. G., Graham, D. J., and Labadz, J. (2014). The potential of small unmanned aircraft systems and structure-from-motion for topographic surveys: a test of emerging integrated approaches at cwm idwal, north wales. Geomorphology 226, 35–43. doi: 10.1016/j.geomorph.2014.07.021
van Angelen, J. H., Lenaerts, J. T. M., Lhermitte, S., Fettweis, X., Kuipers Munneke, P., van den Broeke, M. R., et al. (2012). Sensitivity of Greenland Ice Sheet surface mass balance to surface albedo parameterization: a study with a regional climate model. Cryosphere 6, 1175–1186. doi: 10.5194/tc-6-1175-2012
van As, D., Hubbard, A. L., Hasholt, B., Mikkelsen, A. B., van den Broeke, M. R., and Fausto, R. S. (2012). Large surface meltwater discharge from the Kangerlussuaq sector of the Greenland ice sheet during the record-warm year 2010 explained by detailed energy balance observations. Cryosphere 6, 199–209. doi: 10.5194/tc-6-199-2012
van de Wal, R. S. W., Greuell, W., Van den Broeke, M. R., Reijmer, C. J., and Oerlemans, J. (2005). Surface mass-balance observations and automatic weather station data along a transect near Kangerlussuaq, West Greenland. Ann. Glaciol. 42, 311–316. doi: 10.3189/172756405781812529
van den Broeke, M. R., Smeets, C. J. P. P., and van de Wal, R. S. W. (2011). The seasonal cycle and interannual variability of surface energy balance and melt in the ablation zone of the west Greenland ice sheet. Cryosphere 5, 377–390. doi: 10.5194/tc-5-377-2011
van den Broeke, M. R., Smeets, P., Ettema, J., van der Veen, C., van de Wal, R. S. W., and Oerlemans, J. (2008). Partitioning of melt energy and meltwater fluxes in the ablation zone of the west Greenland ice sheet. Cryosphere 2, 179–189. doi: 10.5194/tc-2-179-2008
van der Hage, J. C. H. (1992). Interpretation of field measurements made with a portable albedometer. J. Atmospher. Oceanic Technol. 9, 420–425.
Von Bueren, S., Burkart, A., Hueni, A., Rascher, U., Tuohy, M., and Yule, I. (2015). Deploying four optical UAV-based sensors over grassland: challenges and limitations. Biogeosciences 12:163. doi: 10.5194/bg-12-163-2015
Wang, J., Ye, B., Cui, Y., Yang, G., He, X., and Sun, W. (2011). “Accuracy assessment of MODIS daily snow albedo product based on scaling transformation,” in 2011 International Conference on Remote Sensing, Environment and Transportation Engineering, RSETE 2011 - Proceedings, (Nanjing), 2865–2870.
Warren, S. G., and Wiscombe, W. J. (1980). A model for the spectral albedo of snow. II: snow containing atmospheric aerosols. J. Atmospher. Sci. 37, 2734–2745.
Westoby, M., Brasington, J., Glasser, N., Hambrey, M., and Reynolds, J. (2012). Structure-from-Motion photogrammetry: a low-cost, effective tool for geoscience applications. Geomorphology 179, 300–314. doi: 10.1016/j.geomorph.2012.08.021
Whitehead, K., Moorman, B. J., and Hugenholtz, C. H. (2013). Brief Communication: low-cost, on-demand aerial photogrammetry for glaciological measurement. Cryosphere 7, 1879–1884. doi: 10.5194/tc-7-1879-2013
Wientjes, I., De Van Wal, R., Schwikowski, M., Zapf, A., Fahrni, S., and Wacker, L. (2012). Carbonaceous particles reveal that Late Holocene dust causes the dark region in the western ablation zone of the Greenland ice sheet. J. Glaciol. 58, 787–794. doi: 10.3189/2012JoG11J165
Wientjes, I. G. M., and Oerlemans, J. (2010). An explanation for the dark region in the western melt zone of the Greenland ice sheet. Cryosphere 4, 261–268. doi: 10.5194/tc-4-261-2010
Wiscombe, W. J., and Warren, S. G. (1980). A model for the spectral albedo of snow. I: Pure snow. J. Atmospher. Sci. 37, 2712–2733.
Yang, K., and Smith, L. C. (2013). Supraglacial streams on the greenland ice sheet delineated from combined spectral-shape information in high-resolution satellite imagery. IEEE Geosci. Remote Sens. Lett. 10, 801–805. doi: 10.1109/LGRS.2012.2224316
Yang, K., Smith, L. C., Chu, V. W., Pitcher, L. H., Gleason, C. J., Rennermalm, A. K., et al. (2016). Fluvial morphometry of supraglacial river networks on the southwest greenland ice sheet. GISci. Remote Sens. 53, 459–482. doi: 10.1080/15481603.2016.1162345
Keywords: albedo, digital camera, unmanned aerial vehicle, remote sensing, Greenland Ice Sheet, energy balance modeling
Citation: Ryan JC, Hubbard A, Box JE, Brough S, Cameron K, Cook JM, Cooper M, Doyle SH, Edwards A, Holt T, Irvine-Fynn T, Jones C, Pitcher LH, Rennermalm AK, Smith LC, Stibal M and Snooke N (2017) Derivation of High Spatial Resolution Albedo from UAV Digital Imagery: Application over the Greenland Ice Sheet. Front. Earth Sci. 5:40. doi: 10.3389/feart.2017.00040
Received: 24 February 2017; Accepted: 09 May 2017;
Published: 23 May 2017.
Edited by:
Christoph Schneider, Humboldt University of Berlin, GermanyReviewed by:
Martin Truffer, University of Alaska Fairbanks, United StatesCarleen Reijmer, Utrecht University, Netherlands
Joseph Michael Shea, University of Saskatchewan, Canada
Copyright © 2017 Ryan, Hubbard, Box, Brough, Cameron, Cook, Cooper, Doyle, Edwards, Holt, Irvine-Fynn, Jones, Pitcher, Rennermalm, Smith, Stibal and Snooke. This is an open-access article distributed under the terms of the Creative Commons Attribution License (CC BY). The use, distribution or reproduction in other forums is permitted, provided the original author(s) or licensor are credited and that the original publication in this journal is cited, in accordance with accepted academic practice. No use, distribution or reproduction is permitted which does not comply with these terms.
*Correspondence: Alun Hubbard, YWh1MDEyQHVpdC5ubw==