- 1Lamont-Doherty Earth Observatory, Columbia University, Palisades, NY, United States
- 2Department of Biodiversity, Earth and Environmental Science, Drexel University, Philadelphia, PA, United States
- 3Department of Geological Engineering, Faculty of Engineering, Universitas Gadjah Mada, Yogyakarta, Indonesia
- 4School of Earth and Space Exploration, Arizona State University, Tempe, AZ, United States
- 5Istituto Nazionale di Geofisica e Vulcanologia—Sezione di Pisa, Pisa, Italy
Lava domes form by the effusive eruption of high-viscosity lava and are inherently unstable and prone to collapse, representing a significant volcanic hazard. Many processes contribute to instability in lava domes and can generally be grouped into two categories: active and passive. Active collapses are driven directly by lava effusion. In contrast, passive collapses are not correlated with effusion rate, and thus represent a hazard that is more difficult to assess and forecast. We demonstrate a new workflow for assessing and forecasting passive dome collapse by examining a case study at Sinabung Volcano (North Sumatra, Indonesia). We captured visual images from the ground in 2014 and from unoccupied aerial systems (UAS) in 2018 and used structure-from-motion photogrammetry to generate digital elevation models (DEMs) of Sinabung’s evolving lava dome. By comparing our DEMs to a pre-eruption DEM, we estimate volume changes associated with the eruption. As of June 2018, the total erupted volume since the eruption began is 162 × 106 m3. Between 2014 and 2018, 10 × 106 m3 of material collapsed from the lava flow due to passive processes. We evaluate lava dome stability using the Scoops3D numerical model and the DEMs. We assess the passive collapse hazard and analyze the effect of lava material properties on dome stability. Scoops3D is able to hindcast the location and volume of passive collapses at Sinabung that occurred during 2014 and 2015, and we use the same material properties to demonstrate that significant portions of the erupted lava potentially remain unstable and prone to collapse as of late 2018, despite a pause in effusive activity earlier that year. This workflow offers a means of quantitatively assessing passive collapse hazards at active or recently active volcanoes.
1 Introduction
The collapse of unstable lava dome and flow structures, generating block-and-ash style pyroclastic density currents (PDCs), is a dangerous and frequent hazard posed by the effusive eruption of high-silica, high-viscosity lavas. Dome-forming eruptions are often prolonged, lasting months to years, creating a persistent hazard for the surrounding region (Sparks and Aspinall, 2004; Wolpert et al., 2016). Anticipating the potential size or frequency of a collapse is a difficult task, as many different processes can contribute simultaneously to developing instabilities in the lava (Voight, 2000; Harnett et al., 2019). In some instances, the size or frequency of collapses can be correlated with effusion rate (Nakada et al., 1999; Calder et al., 2002; Carr et al., 2016), such that changes in effusion rate can be used to assess the variable hazard posed by dome collapses. However, this is not true for all processes driving the development of instabilities, and large collapses can occur when activity is otherwise low (Calder et al., 2002; Simmons et al., 2005; Carr et al., 2016; Carr et al., 2019a). Identifying the processes acting to destabilize erupted lava is crucial to understanding the collapse hazard.
Dome collapse events can be categorized as either “active” or “passive” (Calder et al., 2002). Active collapses are “pushed” by mechanisms associated with the ongoing growth of a lava dome or flow (e.g., oversteepening of a flow front or dome margin), or by gas overpressure within the lava. Active collapses are sourced from regions of new growth of the lava and their size and/or frequency generally correlates with the eruption rate. Passive collapses occur when internal or local processes (i.e., not related to addition of dome volume) occur in situ until failure takes place (Calder et al., 2002). These processes include failures due to internal dome weakening (e.g., due to cooling, fracturing, and/or hydrothermal alteration) to the point that the weight of the dome exceeds its internal strength, dome flow that leads to overtopping of a barrier, removal of confining topography, or external loading (e.g., by rainfall or earthquake shaking). The size and frequency of passive collapses does not correlate with eruption rate. Passive collapses often occur in older material and can occur during periods of no active effusion (Calder et al., 2002). During an eruption, passive collapses are generally associated with endogenous dome growth and slower extrusion rates while active collapses are generally associated with exogenous growth and faster extrusion rates (Calder et al., 2002; Harnett et al., 2019). We note here, however, that it is not always clear whether a collapse occurred due to an active or passive process. During endogenous dome growth, for example, it can be difficult to determine if an instability developed because of internal weaknesses (a passive process), or oversteepening of the dome margin as dome volume increases (an active process), or a combination of both.
Changes in the frequency of active collapses can be anticipated through monitoring of the effusion rate or lava flow advance rate. During lava flow emplacement at Santiaguito Volcano (Guatemala) and Sinabung Volcano (Indonesia), collapses were more frequent when flow advance rates were highest (Harris et al., 2002; Carr et al., 2019a). For dome-forming eruptions at the Soufrière Hills Volcano (SHV) (Calder et al., 2002), Unzen Volcano in Japan (Nakada et al., 1999), and Merapi Volcano in Indonesia (Voight et al., 2000; Carr et al., 2016), the frequency of collapse-generated PDCs increased during periods of higher effusion rate. This pattern allowed periods of increased PDC activity to be anticipated by observing tilt and seismic signals associated with pulses of magma supply that increased effusion rate (Voight et al., 2000; Calder et al., 2002). Pulses of increased effusion rate can also increase the gas flux into the dome and create overpressure conditions that can cause dome collapse and, in some cases, lead to explosive eruption phases (Voight and Elsworth, 2000).
The volumes of active collapses are widely variable, from small rockfalls to explosive events that can destroy the entire dome (Calder et al., 2002; Harnett et al., 2018; Harnett et al., 2019). Small active collapses are commonly related to exogenous dome growth and the advance of dome margins or flow fronts (Calder et al., 2002; Harnett et al., 2019). Many of the larger collapse events at SHV and Merapi were attributed to gas pressurization within the dome (Voight and Elsworth, 2000; Voight et al., 2000). While a global survey of dome collapse events by Harnett et al. (2019) did not find a correlation between effusion rate and collapse volume, this trend was observed for specific eruptions during periods when active collapse processes dominated dome instability (e.g., Unzen, SHV; Nakada et al., 1999; Calder et al., 2002; Simmons et al., 2005). This suggests that a correlation between collapse volume and effusion rate can be made under specific circumstances, primarily when the lava is viscous enough to increase in height (Simmons et al., 2005) and the source of collapses is the actively growing region of the dome (Calder et al., 2002).
Passive collapses, though rare and generally not the primary hazard during an effusive eruption, have one characteristic that makes them exceptionally dangerous—their unpredictability. Passive collapses do not correlate with effusion rate as active collapses often do, and so can occur with no obvious precursors. In 1994, the dome at Merapi collapsed due to a gravitational instability that developed slowly over months during a period of low but constant effusion rate (Voight et al., 2000). Though the resulting PDCs were less voluminous than the gas-driven collapses during the 1997 to 1998 eruption, the 1994 collapse caused 64 fatalities while the 1997 to 1998 collapses caused none (Voight et al., 2000). The key difference was that in 1997 and 1998, tilt and seismic instruments (installed in 1992) detected an increased effusion rate, prompting population evacuations (Voight et al., 2000); no such precursory signals were detected in 1994.
Large passive collapses are often associated with instabilities caused by the terrain confining erupted lava. These can also be compound processes, as a relatively small initial passive collapse can expose the gas-rich interior of the dome and trigger a depressurization explosion that causes more extensive dome collapse. The largest collapses at SHV that were not correlated with increased effusion rate were caused by the failure of a confining crater wall and/or lava overtopping a crater wall (Calder et al., 2002), resulting in passive collapses that depressurized a gas-rich dome (Voight and Elsworth, 2000; Calder et al., 2002). The collapse of a confining crater wall was also the cause of the largest and only deadly PDCs during the 2006 eruption at Merapi (Charbonnier and Gertisser, 2008), and crater wall instability likely played a role in the large dome collapse in 2015 at Colima Volcano (Mexico) (Lesage et al., 2018). Inflation of the lava flow at Sinabung caused lava to overtop two confining ridges and led to large collapses (Carr et al., 2019a; Carr et al., 2019b). The collapses at both Merapi and Sinabung also occurred in the weeks or months following the peak eruption phases when alert levels had been lowered (Carr et al., 2016; Carr et al., 2019a).
The timing of passive collapses is not limited to the duration of the eruption itself. At SHV, several of the largest collapses occurred during a period (July 1998 to July 1999) when effusion had paused (Calder et al., 2002). These collapses were caused by weakening of the dome structure associated with hydrothermal alteration and/or heavy rainfall (Calder et al., 2002; Elsworth et al., 2004). No precursory signals commonly observed prior to active collapses such as crater rim deformation, an increase in earthquake activity, or an increase in rockfalls were associated with these collapse events (Calder et al., 2002). Weakening of erupted lava remains a persistent hazard long after an eruption as well, as continued hydrothermal alteration and ground water flow can make volcanoes more prone to flank collapse (Calder et al., 2002; Ball et al., 2018; Harnett and Heap, 2021; Heap et al., 2021). Platz et al. (2012) described a dome collapse at Mt. Taranaki (New Zealand) that occurred decades after the eruption had ended, likely caused by progressive weakening of the dome material over time and eventually triggered by a heavy rainstorm or an earthquake.
Here, we assess the stability of erupted lava at Sinabung Volcano by combining digital elevation models (DEMs) generated through Structure-from-Motion (SfM) photogrammetry and the Scoops3D slope stability model (Reid et al., 2000; Reid et al., 2015). We first compare the collapse volume and location predicted by the models with events that already occurred. We then produce a passive collapse hazard assessment for Sinabung given its topography during our field campaign and investigate its sensitivity to input material properties. These steps provide a workflow for quantitatively assessing passive collapse hazards that complements existing methods for assessing active collapse hazards in order to develop a more complete assessment of the hazard presented by dome-forming volcanic eruptions. Lastly, we discuss the usefulness and limitations of this approach for assessing dome collapse hazards at active volcanoes.
2 Dome Collapse at Sinabung Volcano
Sinabung Volcano is a 2,460 m stratovolcano located in North Sumatra, Indonesia (Figure 1). Lava dome growth at Sinabung began in December 2013 (Gunawan et al., 2019; Pallister et al., 2019). Effusion of lava over the next 4 years resulted in thousands of collapse-generated PDCs and the emplacement of a 3 km long andesitic lava flow (Carr et al., 2019a; Nakada et al., 2019; Pallister et al., 2019; Kriswati and Solikhin, 2020). Nakada et al. (2019), Pallister et al. (2019), and Carr et al. (2019a); Carr et al. (2019b) detail the chronology of the eruption from late 2013 through 2017. We highlight here the overall trends relevant to our discussion of dome collapse.
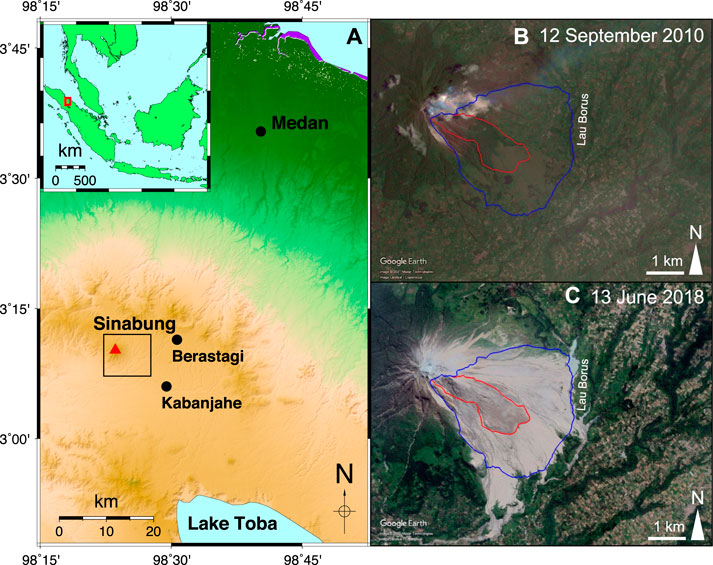
FIGURE 1. Location of Sinabung Volcano. (A) Sinabung Volcano is located in the Karo Regency of North Sumatra, Indonesia (modified from Carr et al., 2019a). Satellite images from before (B) and during (C) the eruption show the extensive coverage of deposits from PDCs and the 2014 lava flow (red outline). The blue outline shows the extent of our 2018 data and is the area used for the erupted volume calculation.
Collapses of all sizes were most frequent in the initial months of the eruption, January—March 2014, coincident with the highest effusion rates and lava flow advance rates of the eruption (Figure 2) (Nakada et al., 2019; Carr et al., 2019b; Kriswati and Solikhin, 2020). A series of large collapses of the southwest side of the upper flow began on 30 September 2014. Instability in the lava developed due to gravitational loading as the flow inflated above a ridgeline that had initially confined the flow to a pre-existing ravine (Carr et al., 2019a; Carr et al., 2019b). A similar series of collapses occurred in June 2015 on the northeast side of the upper flow as that ridgeline was also overtopped. The collapses of the upper part of the flow in September-October 2014 and June 2015 ended lava flow emplacement and initiated a new phase of dome growth and collapse at the summit vent (Figure 2) (Carr et al., 2019b; Nakada et al., 2019; Pallister et al., 2019).
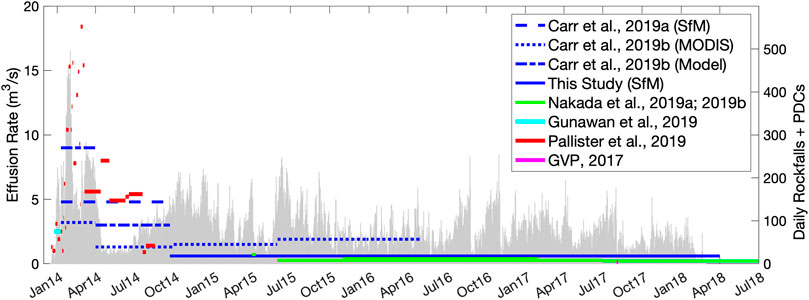
FIGURE 2. Sinabung Eruption History. Effusion rate at Sinabung (horizontal lines) increased rapidly in early 2014 before slowly decreasing over the next few years. The frequency of PDCs and rockfalls (gray bars, modified from Kriswati and Solikhin, 2020, data only through February 2018) was highest in the early months of 2014 when effusion rate and flow advance rate were highest and lowest in the middle of 2014 when the lava flow inflated. Increases in PDCs around October 2014 and June 2015 were caused by the instabilities that developed when lava overtopped confining ridgelines, generating passive collapses of the upper flow. Spikes in the frequency of collapses during 2016 and 2017 were active collapses associated with Vulcanian explosions caused by gas pressurization within the lava dome. These patterns demonstrate how both passive collapses and gas overpressure-driven active collapses can cause an increase in collapse activity without a corresponding increase in eruption rate.
Explosions began to occur frequently in August 2015 and continued through early 2018 (Nakada et al., 2019; Kriswati and Solikhin, 2020). Collapse activity during this period was characterized by relatively small, frequent events associated with dome growth or small vulcanian explosions (Figure 2) (Nakada et al., 2019). Major explosive and dome collapse events occurred every few months (Figure 2). The largest explosive event occurred 19 February 2018, and resulted in the complete destruction of the lava dome (Global Volcanism Program, 2018a). Following the February 2018 explosive event, the lava dome did not regrow-at least not to a size visible above the crater rim from the base of the volcano (Global Volcanism Program, 2018a; Global Volcanism Program, 2018b). This suggests that continuous effusion of lava at Sinabung likely ended sometime in the first half of 2018.
Dome growth and collapse resumed in August 2020 and continues as of this writing (Global Volcanism Program, 2020; Global Volcanism Program, 2021). Activity we observed during field work for this study in June 2018 (during the 2-year pause in dome growth) consisted of persistent degassing producing a diffuse plume and occasional ash-rich plumes rising no more than 1,000 m above the vent (Figure 3) (Global Volcanism Program, 2018b). Ash-rich plumes were initiated by small explosions and could persist for multiple hours.
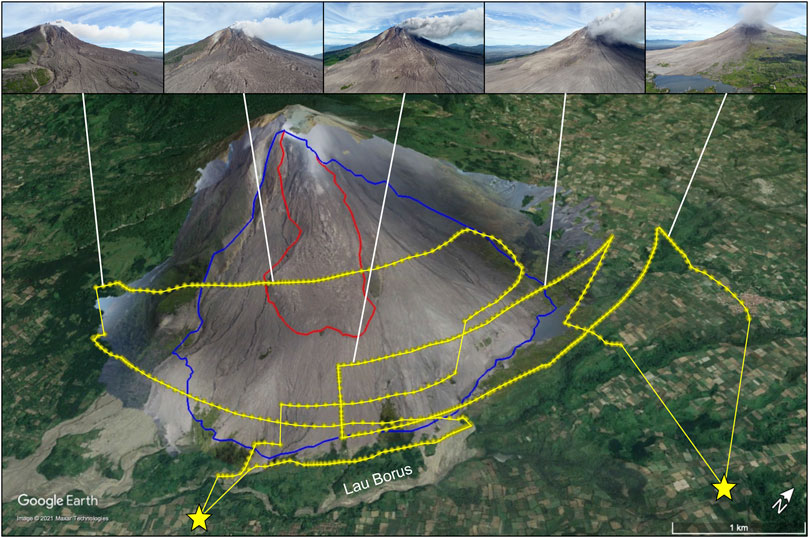
FIGURE 3. UAS flights at Sinabung. We collected oblique images (insets) of Sinabung at different elevations and perspectives using a UAS. Two flights (yellow lines) were needed to complete the full perspective of the flanks of Sinabung affected by the eruption. Take off locations are marked by stars; yellow dots are the locations where the UAS captured images. Lines from the inset photographs to an image location indicate where the images were taken. The orthophoto derived from these photos using Agisoft Metashape™ is shown draped over satellite imagery and topography in GoogleEarth™. A small ash-rich plume extending to the northeast was present during the flights, which limited the detail and extent of the orthophoto in this direction. The lake visible in the far right inset image and the orthophoto was formed when PDC deposits dammed the Lau Borus river. The lava flow is outlined in red and the area used for the volume calculation is outlined in blue.
Active collapse processes associated with dome growth or flow advance are the dominant cause of instability in erupted lava at Sinabung. In contrast, the collapses that occurred in late September to October 2014 and June 2015 in association with the inflating lava flow overtopping the confining ridgelines were passive collapses (Carr et al., 2019a; Carr et al., 2019b). No increase in effusion rate was noted prior to these collapses (Pallister et al., 2019), though the frequency of small rockfalls had been increasing since July (notably, larger collapses were absent) (Figure 2) (Nakada et al., 2019). Passive collapse processes, though rare over the course of the eruption, were responsible for significantly altering the character of the eruption in late 2014, 2015 from one of flow advance and inflation to once again dominated by frequent collapse of a lava dome growing at the summit vent.
3 Materials and Methods
3.1 Image Collection and Photogrammetric Processing
We conducted field work at Sinabung Volcano in September 2014 (prior to the collapse event that began on September 30) and June 2018. We collected optical photographs from which we created DEMs of the Sinabung edifice and lava flow by applying structure-from-motion (SfM) photogrammetry. The SfM technique solves simultaneously for camera parameters and the geometry of the features in a set of photographs, allowing detailed 3D information to be generated using images from standard digital cameras (Snavely et al., 2008). Compared to other methods of generating topographic data, SfM can be more time- and cost-effective while also offering a greater degree of user-control to define the resolution, coverage, and frequency at which the data are collected (James and Robson, 2012; Westoby et al., 2012). During field work in 2014 we collected ground-based photographs to generate DEMs; the subsequent data analysis and results are described in detail in Carr et al. (2019a); Carr et al. (2019b). Here, we use the DEM from “Model 4” of Carr et al. (2019a), available through OpenTopography (doi: 10.5069/G97S7KWC). This model was created using 54 images from a Nikon D40X DSLR camera taken on 22 September 2014, corresponding to a DEM with a spatial resolution of 3.8 m (Carr et al., 2019a).
In 2018, we collected images for SfM photogrammetry using a DJI Matrice 210 unoccupied aerial system (UAS). A Zenmuse X4S camera was mounted to the UAS; this camera has a 5,472 × 3,648 pixels 24 mm CMOS sensor with a mechanical shutter. We flew the UAS in an arc around the volcano to collect oblique images (Figure 3). We varied the distance from the vent and the elevation of the arcuate UAS flights to maximize the variety of perspectives that were captured. In total, it took two UAS flights, taking off from locations approximately 4 km apart, to complete this flight plan and capture a full perspective of the S-NE flanks of Sinabung that were affected by the eruption. Due to the exclusion zone enforced around the volcano during our field work and Sinabung’s ∼1000-m vertical prominence above the surrounding area, a near-nadir mapping flight plan was not possible.
We used Agisoft Metashape™ Pro (v. 1.6) for SfM photogrammetry processing and the creation of 3D models, point clouds, DEMs, and orthophotos from 454 UAS images taken during two flights on 20 June 2018. Prior to alignment of the images, each image was masked to eliminate foreground and sky captured in the oblique images (Figure 3). We also masked the persistent ashy plume being emitted and blown eastward during our flights, which resulted in reduced coverage of the NE side of the summit area compared to the S side. The ‘high’ setting (In Metashape™, meaning the full-resolution images were used for processing, with no downsampling) was used for all processing steps and resulted in a dense point cloud with 23, 386, 513 points and a DEM with 0.87 m per pixel spatial resolution. Initial spatial reference for the models was provided by the GPS onboard the UAS which geotagged each photograph. We estimated the precision of the points in the sparse cloud within Metashape™ following the workflow and using the code provided in James et al. (2020a). The point precision values were used to calculate the error in the volume estimates determined in the next processing step. The data products and images associated with 2018 field work at Sinabung are available from OpenTopography.org (doi: 10.5069/G9988568).
3.2 Volume Estimation
We estimated volume change at Sinabung by comparing our 2018 DEM of Sinabung to a 5 m pre-eruption DEM of Sinabung from 2010 (provided by the Badan Informasi Geospasial and the Center for Volcanology and Geological Hazard Management in Indonesia) and the 2014 DEM from Carr et al. (2019a). Differencing was performed using the open-source software CloudCompare (http://www.cloudcompare.org). First, we interpolated the point precision values calculated for the sparse cloud within Metashape™ onto the dense cloud for our 2018 model. Next, we aligned the 2018 dense point cloud to both a point cloud generated from the 2010 DEM and the 2014 dense point cloud using evenly distributed manually selected control points (Supplementary Table S1). We tested the iterative closest point (ICP) automated alignment method (Besl and McKay, 1992) supported by CloudCompare, but results were poor compared to the manual method due to the extent of significant topographic change that occurred within the survey area due to the ongoing eruption (Figure 1). By treating both the 2010 and 2014 point clouds as the “reference” dataset for the alignments, we eliminate potential registration errors that would arise if all point clouds were aligned to a shared reference frame.
We applied the M3C2 and M3C2-PM methods (Lague et al., 2013; James et al., 2017) to calculate the cloud-to-cloud distances. The Multiscale Model to Model Cloud Comparison (M3C2) technique calculates the surface normal distances between two point clouds and estimates a 95% confidence interval for that distance based on point cloud roughness and any known registration error (Lague et al., 2013). James et al. (2017) modified the M3C2 method to estimate the confidence interval based on point cloud precision maps (M3C2-PM). We performed M3C2 and M3C2-PM differencing within CloudCompare, using a diameter of 20 m for both the normal scale and projection scale (D and d; Lague et al., 2013). For a detailed description of determining user-defined input parameters required for M3C2-PM, the reader is referred to Lague et al. (2013) and James et al. (2017); James et al. (2020a). We restricted the differencing to only the vertical direction to make the results directly relatable to a raster representation of surface elevation change from which volume change is a straightforward calculation. We then rasterized and exported the cloud-to-cloud differences and confidence intervals with a 5-m cell size to match the resolution of the DEMs from 2010 to 2014.
We calculated the volume change for both the entire area affected by the Sinabung eruption and for only the lava flow (Figure 1; Figure 3). We clipped the difference and confidence interval maps using ArcMap™ 10.5.1 to eliminate areas where no volcanic deposits were present and the large errors associated with the edges of our models (Figure 3; Supplementary Figure S1). The volume change was determined by multiplying each cell in the clipped cloud-to-cloud difference maps by the pixel area (25 m2) and summing over the dataset.
3.3 Error Estimation
Methods for determining errors in point cloud-derived volume estimates often assume uniform data sources and ground control points (GCPs) measured with high precision. We had neither in this study, as we used both LiDAR (2010) and SfM (2014, 2018) point clouds and measuring ground control points within the surveyed area was not possible due to the ongoing eruption. The M3C2 method was developed for LiDAR point clouds, where the errors due to the surface roughness is primarily related to the instrument precision and can be considered random (James et al., 2017). This is not ideal for SfM point clouds, where error can vary in magnitude and be both localized and systematic (James et al., 2017). The M3C2-PM method was designed to address these types of errors in SfM point clouds, but the precision maps necessary for the workflow of James et al. (2017); James et al. (2020a) cannot be generated for LiDAR data. In cases where GCPs are not used, it is common for errors to be estimated based on the average vertical difference in areas where no topographic change occurred (e.g., Carr et al., 2019a; Civico et al., 2021). However, this method assumes a uniform error over all points and can result in an overestimate of the error (Carr et al., 2019a).
Our specific case at Sinabung is further complicated by two factors. First, there is not enough surface area unaffected by the eruption appearing in both the 2018 and 2014 surveys from which a representative vertical error could be calculated. Second, it was not possible to generate a point precision map for the 2014 SfM point cloud because it was made using Agisoft Photoscan™ v1.0.4, which did not create the metadata necessary for the precision map calculation (Carr et al., 2019a). We reprocessed the original 2014 images in Metashape™ Pro v1.6, but this changed the alignment properties and caused errors when comparing to the original published dataset. For consistency with the previously published data, we proceeded with the 2014 model available on OpenTopography.
We utilized the confidence intervals from both M3C2 and M3C2-PM cloud-to-cloud differencing methods to estimate an error that does not depend on GCPs and is sensitive to both localized and systematic errors in the point clouds. When applying M3C2-PM, we used a ‘null’ point precision map (all values equal to zero) for the 2010 and 2014 point clouds. The result was confidence intervals sensitive to only errors in the 2018 point cloud. The M3C2 differences (2010–2018 and 2014–2018) were sensitive to errors based on the roughness of all the point clouds. For each difference, we then compared the confidence intervals from both methods, and for each pixel, took the larger value (summing the confidence intervals was not appropriate as it would duplicate errors estimated for the 2018 cloud). A comparison of the magnitudes of the confidence intervals from the M3C2 and M3C2-PM methods is shown in Supplementary Figure S1. Cloud-to-cloud differences calculated by M3C2 and M3C2-PM were broadly equivalent. The total error for the volume change estimate was determined by summing the confidence intervals over the map area and multiplying by the pixel area.
3.4 Slope Stability Analysis With Scoops3D
Analysis of slope stability hazards is frequently done by calculating a Factor of Safety (FOS) for a potential collapse volume. The FOS is defined as the ratio between forces resisting and driving failure, or shear, along a potential failure surface. The resisting forces are due to the cohesion and friction of the material and represent the shear resistance (strength, s) of the slope. The driving forces (τ) are the shear stress due to gravity and the mass of the potential failure volume, such that
A FOS > 1 indicates stability and a FOS < 1 indicates instability. The further the FOS is above or below 1, the greater the stability or instability for that potential failure surface.
The shear strength (in Pa) is calculated by applying the Coulomb-Terzaghi failure rule
where c is cohesion (Pa), φ is the angle of internal friction (deg), and σn is the normal stress (Pa). The normal stress and shear stress acting on the slip surface are determined based on the volume and unit weight of the material (γ, kN m−3) in the potential failure region and the orientation of the potential failure surface.
Scoops3D is a 3D slope stability model that uses a DEM to calculate the failure hazard (i.e., FOS) for a spherical slip surface, approximating a rotational landslide (Reid et al., 2000; 2015). Stability is defined by the moment equilibrium between the shear stress imposed by gravity and the shear resistance of the potential failure mass (Figure 4). The program explores a range of potential spherical slip surfaces, each defined by the center of the axis of rotation and the radius of the sphere that forms that slip surface by intersecting with the topography. The input for Scoops3D includes: 1) a DEM, 2) the material properties (c, φ, and γ) for the terrain represented by the DEM, and 3) the lateral and vertical extent and spacing of the search grid for the axes of rotation and the increment of the radius for the sphere defining the failure surface. Scoops3D output includes the minimum FOS and the failure volume associated with that FOS for each DEM cell. Scoops3D also identifies the global minimum FOS (FOSmin), the location and volume of the associated failure, and can generate a new DEM with that volume removed (Figure 4).
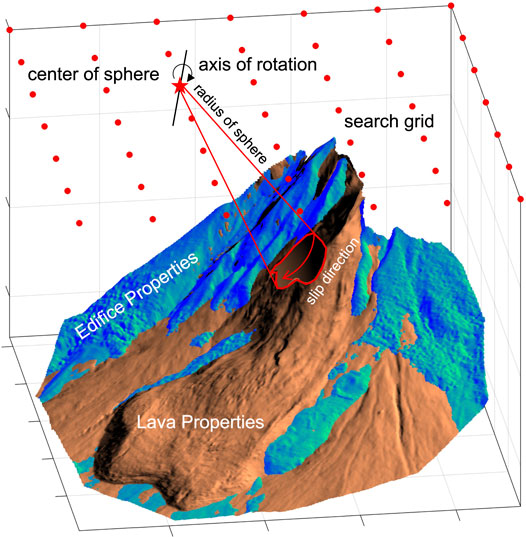
FIGURE 4. Visualization of Scoops3D analysis. A DEM of Sinabung from 2018 shows a ‘scoop’ (red outline) removed from the volcano representing a potential failure volume identified by Scoops3D. We define two sets of material properties for the terrain: 1) lava properties are assigned to material that erupted since 2010, shown here in brown shades, and 2) edifice properties are assigned everywhere else, shown here in blue/green shades. In this example, the red dots represent the grid of failure surface rotation centers defined by user input for one elevation. This grid also extends vertically with a user-defined spacing between the layers of grid nodes, but this is not shown in this schematic. The star is the center of the sphere that defines the failure slip surface marked in a red outline, and the straight lines are the radius of the sphere. The failure surface is where the sphere intersects the topography. The axis of rotation for the failure for this example is shown by the black line. Arrows indicate the direction of rotation and slip. Scoops3D iterates across the search grid, varying the radius of the sphere at each point, and calculates the FOS for hundreds of potential failure surfaces. Scoops3D output includes the minimum FOS and associated potential failure volume for each DEM cell and a new DEM with the FOSmin failure volume subtracted from the input DEM, as shown here.
The FOS is calculated through discretizing the collapse volume by a “method of columns” limit-equilibrium analysis, where each DEM cell is used to define a column of material between the terrain surface and the potential failure surface, and the driving and resisting moments for the entire potential failure are obtained by summing the parameters for each column. Thus, the FOS for a potential collapse volume is
where Ri,j is the distance (m) from the rotational axis of the spherical slip surface to the center of the slip area for the i,j column of the failure area, Ai,j is the area (m2) of the slip surface at the base of the column, Ni,j is the normal force, ui,j is the pore-water pressure acting on the slip surface, Wi,j is the weight of the column, αi,j is the apparent dip at the column base in the direction of slip (deg), and keqei,j is a term to account for horizontal loading due to an earthquake (Reid et al., 2015). In our application, we do not include pore-water pressure or earthquake loading, such that both the ui,jAi,j and keqei,j terms in Eq. 3 equal zero and can be ignored.
Scoops3D evaluates the FOS for many (generally thousands) potential slope failures. Using user-defined extent and spacing parameters, Scoops3D creates a search grid where the nodes of the grid serve as the axes of rotation for potential rotational failure surfaces. Scoops3D varies the radius of a sphere centered on each search node. Where a sphere intersects the land surface as defined by the DEM, Scoops3D calculates the FOS for the volume defined by the intersection (Figure 4). Scoops3D solves for FOS using both the Bishop’s simplified and the Ordinary (Fellenius) method (Reid et al., 2015). In this study, we use the results from the Bishop’s simplified method, which applies an iterative approach to produce results that are typically more accurate than the Ordinary method (Reid et al., 2015). For further description of the various capabilities of Scoops3D and how the program solves for the FOS of potential failure surfaces, the reader is referred to Reid et al. (2015).
We applied Scoops3D (v. 1.1) to the 2014 and 2018 DEMs of Sinabung. While the 2018 DEM has a resolution of 0.87 m, we exported it from Metashape™ for use in Scoops3D with a resolution of 5 m. This reduced the computational time of Scoops3D by orders of magnitude and made the model output directly comparable with the 5 m resolution of the 2014 DEM. We compared results from Scoops3D using both 5 and 1 m resolution versions of the 2018 DEM and found no significant differences in any of the output (i.e., FOS, collapse volumes, FOSmin). Additionally, the minimum surface area of a potential failure for Scoops3D analysis is ∼200 DEM cells (Reid et al., 2015), which at 5 m resolution equates to a circle with a radius of 40 m. Through visual observation of collapse sites captured in photographs (see Figure 2 in Carr et al., 2019b), this is similar in size to the initiation of the passive collapse sequence observed at Sinabung in late September - October 2014. Thus, reducing the resolution of our DEM improved computational time without sacrificing the ability to identify relevant potential failure volumes.
We selected values for the material properties of the Sinabung edifice and lavas based on previous studies of Sinabung and other stratovolcanoes. We assigned different properties to the pre-existing Sinabung edifice relative to the lava erupted since 2013 (Table 1). We created 3D material properties files for Sinabung by comparing the 2010 pre-eruption DEM to our 2014 and 2018 DEMs. Material properties representing the pre-existing edifice were assigned to all parts of the column for a given DEM cell with an elevation equal to or less than the elevation in the 2010 DEM. Material properties representing the newly erupted lava were assigned to the parts of the column with an elevation greater than the elevation in the 2010 DEM, representing emplacement of new material during the eruption. We used the same unit weight for both material categories. Nakada et al. (2019) reported that lava erupted at Sinabung during the current eruption had a unit weight of 24.5 kN m−3 and this lava—an andesite—is similar to lavas from previous eruptions. For the edifice, we chose a cohesion of 1,000 kPa and an angle of internal friction of 40° based on values for “strong (fractured)” volcanic rock in Reid et al. (2000). Similar values for stratovolcanoes were also applied in analyses by Simmons et al. (2005), Ball et al. (2018), Heap et al. (2021), and Kereszturi et al. (2021). For the material properties of erupted lava, Voight and Elsworth (2000), in an analysis of the active lava dome at Merapi Volcano (Java, Indonesia), tested cohesions of 0–500 kPa and angles of internal friction from 25 to 60° and found a best fit for a dome with a core temperature ∼800 °C when c = 500 kPa and φ = 25°. In an application of Scoops3D to Stromboli Volcano (Italy), Schaefer et al. (2019) used c = 200 kPa and φ = 35° for “lava + breccia” and c = 600 kPa and φ = 23° for “volcaniclastic deposits”. We define two end members for lava material properties– “stronger” with c = 500 kPa and φ = 25°, and “weaker”, with c = 100 kPa and φ = 25° (Table 1). Both end member parameter values fall within the range for “weak” to “very weak” volcanic rock (c = 10–500 kPa and φ = 15–27°) defined by Reid et al. (2000). The “weaker” properties are similar to the values of c = 200 kPa and φ = 20° used by Ball et al. (2018) for hydrothermally altered volcanic rock while the “stronger” properties are similar to those found by Voight and Elsworth (2000) for an active lava dome.
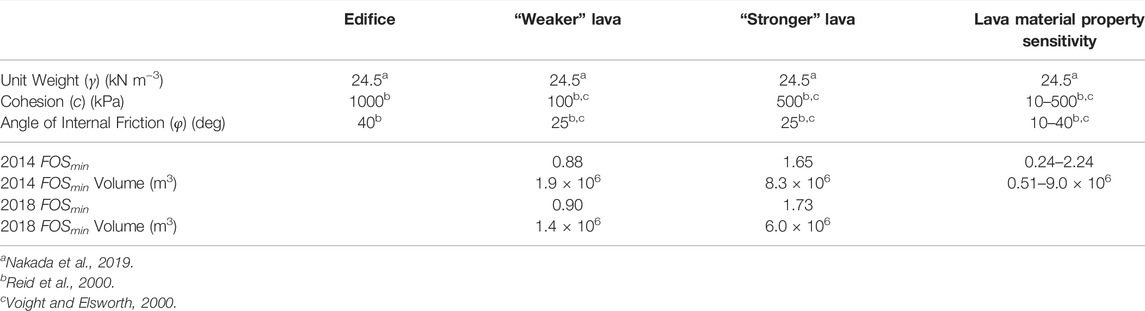
TABLE 1. Model input parameters and results. Material properties for the edifice (defined as the pre-eruption topography from a 2010 DEM) were held constant for all Scoops3D runs. Properties for the erupted lava used values of cohesion and angle of internal friction representing “stronger” and “weaker” end members for both the 2014 and 2018 DEMs. Lava properties were varied over their likely ranges to test the sensitivity of the resulting stability on these properties using the 2014 DEM as input. The results shown are the FOS for the least stable potential failure (FOSmin) and the volume of that potential failure.
We defined the horizontal and vertical extent of the search grid, the grid spacing, and the radius increment for potential spherical failure volumes based on the resolution and topographic relief represented by the input DEM and following the guidelines in Reid et al. (2015) for creating a thorough and computationally efficient search grid. We restricted the search to potential failures with volumes of 0.1–10 × 106 m3, corresponding to the approximate minimum detection volume for a 5-m DEM and the total volume of collapses that occurred at Sinabung between 2014 and 2018 (see Section 4.1). As the initial collapse at Sinabung in September 2014 was relatively small (Carr et al., 2019b), we further restricted the failure volume bounds and performed additional runs to investigate stability related specifically to individual small collapses (bounds of 0.1–0.3 × 106 m3). To ensure that our model set up captured the least stable potential failure for each DEM cell, we also tested a broader range of potential failure volumes spanning up to the total volume of the Sinabung eruption (105–108 m3). This run of Scoops3D had a significantly longer processing time and confirmed that our volume range based on observations (0.1–10 × 106 m3) both captured the least stable conditions and was more computationally efficient. Complete input files, DEMs, and material properties files for all the Scoops3D runs performed to evaluate stability in the 2014 and 2018 DEMs are included as Supplementary Material accompanying this paper.
We applied Scoops3D to investigate three main questions. First, we used the 2014 DEM as input and tested if unstable areas (FOS < 1) and collapse volumes calculated by Scoops3D accurately matched locations where collapses occurred between 2014 and 2018. This served as a benchmarking step for using Scoops3D to model instability in erupted lava and our selection of appropriate material properties. Second, we evaluated the stability of erupted lava at Sinabung using the 2018 DEM and identified the locations and volumes of areas most likely to collapse. Third, we investigated the effects of varying material properties on the stability, location, and volume of the FOSmin potential failure. To do this, we ran Scoops3D using the 2014 DEM as input for combinations of c = 10–500 kPa and φ = 10–40°- a total of 77 runs-for the erupted lava properties (Table 1). This range was chosen based on values used in previous studies for volcanic edifices or deposits (Reid et al., 2000; Voight and Elsworth, 2000; Simmons et al., 2005; Ball et al., 2018; Schaefer et al., 2019; Heap et al., 2021; Kereszturi et al., 2021).
4 Results
4.1 Eruption and Collapse Volumes
Differencing the 2010 DEM and our 2018 model produced a map of the thickness of the deposits from the Sinabung eruption (Figure 5C). As also observed in 2014 (Carr et al., 2019a), the lava flow front was approximately 100 m thick and lava thickness exceeded 150 m where the flow filled the pre-existing ravine. Deposits from PDCs were broadly ∼40 m thick on the north side of the lava flow (Figure 5C). We estimated the total erupted volume to be 161.8 ± 7.6 × 106 m3 (0.16 km3) through 20 June 2018. Of this volume, the lava flow accounted for 93.7 × 106 m3 and PDC deposits for 68.1 × 106 m3. This total volume likely represents a minimum estimate, as the DEM does not fully capture the vent area at the summit and does not account for material removed from the map area by lahars in the Lau Borus River (Figure 1) or distal ash fall. The 2018 volume we measured represents an increase by 59 ± 22 × 106 m3 relative to the 2014 volume of 103 ± 14 × 106 m3 (Carr et al., 2019a). Assuming continuous effusion ended at some point in the months prior to our 2018 UAS survey (Global Volcanism Program, 2018a; Global Volcanism Program, 2018b), we estimated the long-term average effusion rate during the dome growth and collapse phase of the Sinabung eruption (late 2014 through early 2018; 42 months) to be approximately 0.5 m3 s−1.
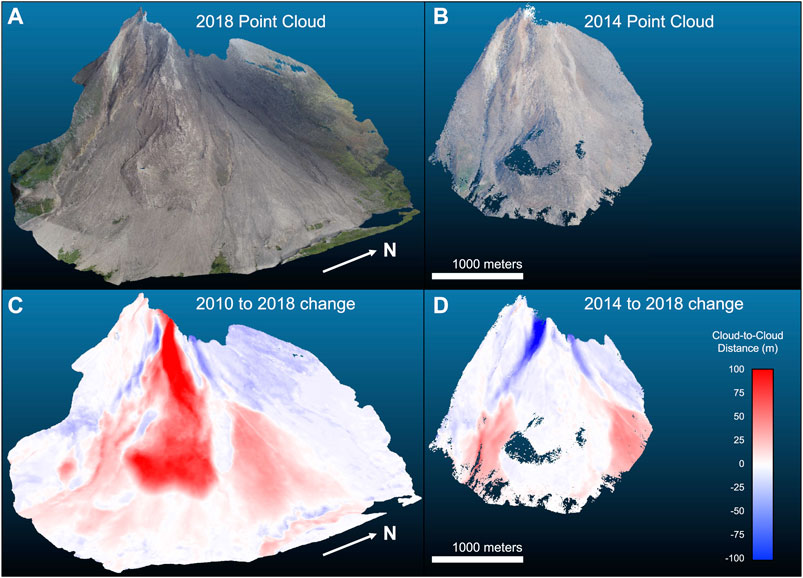
FIGURE 5. Thickness of eruption deposits at Sinabung. A 3D oblique view of the point clouds generated by SfM photogrammetry from the surveys of Sinabung in 2018 (A) and 2014 (B). The UAS survey in 2018 allowed for increased point density and coverage compared to the ground-based 2014 survey. (C) The eruption deposit thickness created by differencing the 2010 point cloud from the 2018 point cloud. PDC deposits on either side of the lava flow exceed 50 m in thickness. (D) The change in eruptive deposit thickness between the 2014 and 2018 point clouds. The region of the lava flow that collapsed in 2014–2015, removing over 100 m of material in some places, is clearly visible (dark blue). The 3D perspective and scale are identical for all four panels.
Volume loss due to collapse of the upper lava flow during 2014, 2015 was visible when differencing the 2014 and 2018 point clouds (Figure 5D). In the uppermost part of the flow, the region of material removed by collapse was over 100 m thick. Summing only the negative elevation changes in the 2018-2014 DEM difference (so as not to include regions where new lava was emplaced), we estimated a total collapsed volume of 10.3 ± 1.3 × 106 m3, or 10% of the pre-collapse flow volume.
4.2 Scoops3D Slope Stability for Sinabung Volcano
4.2.1 Validation Against 2014 Collapses
A large area of the erupted lava at Sinabung in 2014 had a FOS < 1 using the “weaker” lava material properties, including the location of the initial passive collapse in October 2014 (yellow dot, Figure 6A). The FOSmin was 0.88, located a few hundred meters downslope from the location of the initial collapse (black outline, Figure 6A). The volume associated with the FOSmin is 1.9 × 106 m3. For the “stronger” lava parameters, the FOSmin was 1.65, indicating generally stable conditions (Figure 6C). The FOSmin volume for the “stronger” lava was 8.3 × 106 m3 and was in a similar location to the “weaker lava” FOSmin volume (Figure 6). The volume of the potential failure associated with the lowest FOS for each DEM cell shows that smaller collapses are favored in less stable areas (Figures 6B,D), regardless of the material properties used. The weaker material properties matched the collapse observations better and are likely more representative values for the Sinabung lava flow at the time of the collapse. The weaker properties also likely represent a maximum instability case, as the properties are unlikely to be as uniformly weak over the entire volume of erupted lava as is represented by the simplified two-components (edifice and erupted lava) configuration we use.
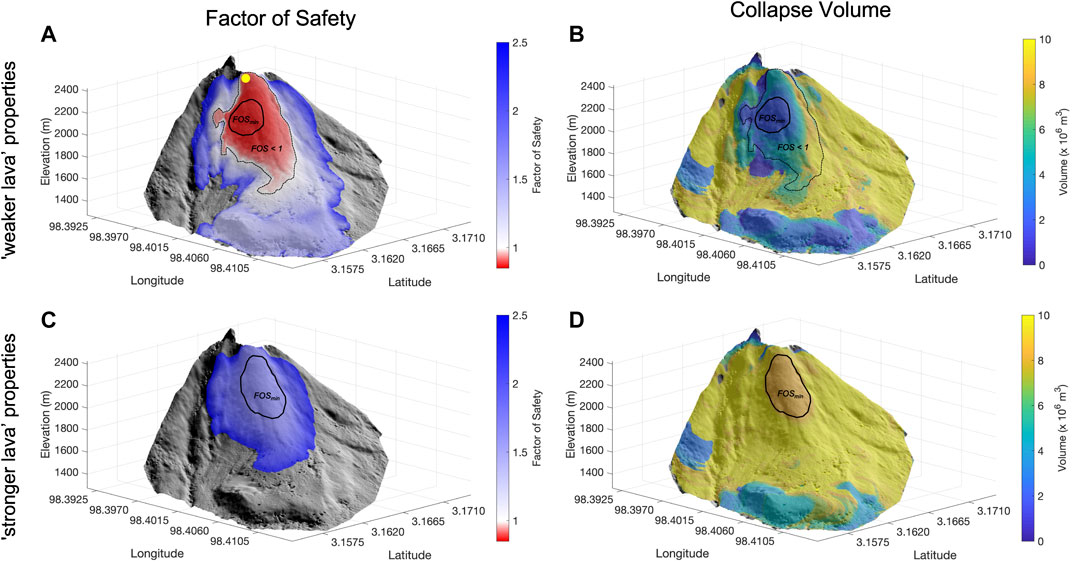
FIGURE 6. Slope stability analysis results for the 2014 topography (uncapped collapse volume). Output from the Scoops3D model shows the FOS for the least stable failure region (A,C) and the volume of that failure (B,D) for each DEM cell. Using material properties representing “weaker lava” (A,B), much of the upper half of the lava flow is potentially unstable, with FOS < 1 (black dashed line). The unstable area includes the location of the initial passive collapse in October 2014 (yellow dot in (A)). The least stable potential collapse surface (FOSmin = 0.88) is located a few hundred meters downslope from the passive collapse location (black outline). For material properties representing “stronger lava” (C,D), the entire edifice is stable, with FOSmin = 1.7. The least stable surface (black outline) is larger compared to the “weaker lava” material properties but includes much of the same area. Areas not colored in (A) and (C) have a FOS > 2.5. For both weaker and stronger lava properties, areas of lower FOS generally correspond with smaller predicted collapse volumes.
Scoops3D produced a strong match to the initial passive collapse that occurred in October 2014 when we limited the potential failure volumes to 0.1–0.3 × 106 m3. This search range was based on our estimate of the collapsed volume using photographs (i.e., Figures 2B,C, Carr et al., 2019b) to identify the region that collapsed in the 2014 photogrammetric model. We approximated the collapse as a spherical volume with a diameter of ∼75 m, which results in a volume of ∼0.2 × 106 m3. The FOSmin for this application of Scoops3D is 0.92 and the FOSmin failure is in a nearly identical location to the observed collapse (Figure 7).
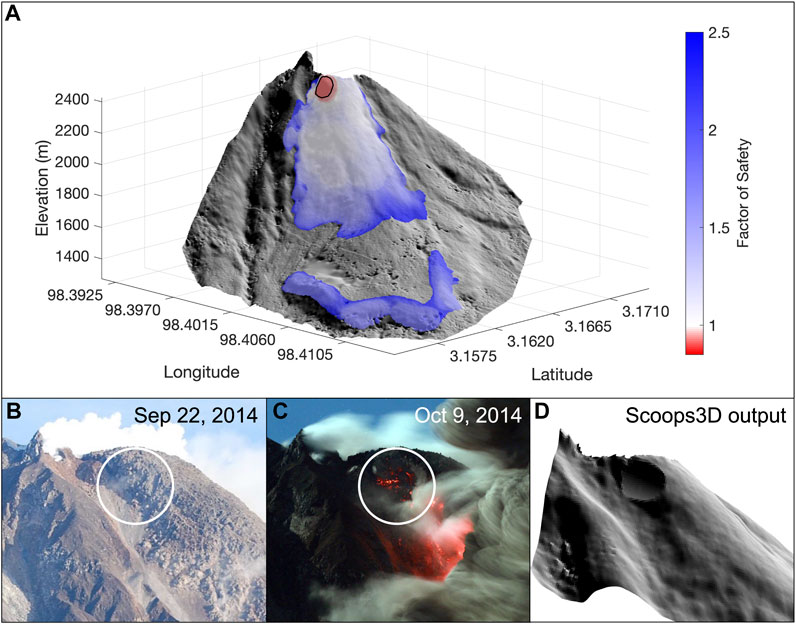
FIGURE 7. Slope stability analysis results for the 2014 topography (maximum collapse volume = 0.3 × 106 m3). Limiting the collapse volume in Scoops3D to a range approximating the size of the initial October 2014 passive collapse and assuming ʻweaker lava’ properties, reproduces the location and appearance of the observed collapse: (A) All unstable surfaces identified by Scoops3D (FOS < 1, red shades) are located where the October collapse occurred, and the FOSmin collapse size (black outline, a) is similar. (B,C) Visual images show the region of the collapse (white circle) before and after the event. The 3D perspective of the collapse produced by Scoops3D (D) shows the similarity of the modeled to observed collapse. Photo credits: L. Vanderkluysen (B); Dedi Sahputra/EPA/Shutterstock (C); (B) and (C) modified from Carr et al. (2019b).
4.2.2 2018 Hazard Assessment
Scoops3D results using the 2018 DEM indicated that a smaller, but still significant, portion of the upper flanks of Sinabung potentially remained unstable (Figure 8A). Using the weaker material properties as input, the FOSmin was 0.90, located on the downslope scarp of the lava flow which remained after the 2014–2018 collapses (Figures 8A,B). The potential collapse volume was 1.4 × 106 m3. As with the 2014 DEM, the stronger material properties do not identify any regions with FOS < 1 and had an FOSmin of 1.73 (Table 1). We also searched the 2018 DEM for smaller volume collapses (search range of 105–106) and found a FOSmin of 0.9 for a failure with a volume of 0.27 × 106 m3 located on the upper part of the downslope side of the collapse scarp in the lava flow (Figure 8C).
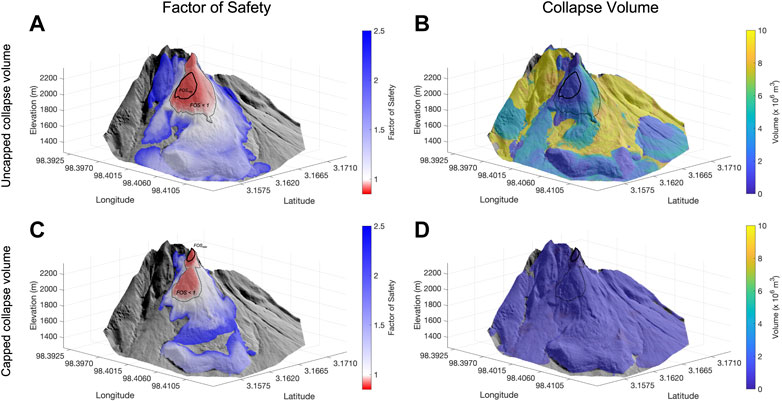
FIGURE 8. Slope stability analysis results for the 2018 topography. Output from the Scoops3D model shows the FOS for the least stable failure region (A,C) and the volume of that failure (B,D) for each DEM cell. Erupted lava at Sinabung remains potentially unstable when applying the 2018 DEM and “weaker lava” material properties (A). The potential failure volume (B) for the full search range is smaller than that for the 2014 DEM (Figure 6B). For potential collapse volumes restricted to 105–106 m3, the FOSmin moves upslope to the scarp remaining from previous collapses (C,D). The black outline in all panels marks the extent of the FOSmin collapse, the dashed black line encloses areas with FOS < 1.
4.2.3 Influence of Input Material Properties
The calculated stability of the erupted lava at Sinabung is greatly impacted by varying material properties within the range of feasible values (Table 1). For the 2014 DEM, the lava was broadly stable or broadly unstable depending on if the weaker or stronger material properties were used (Figure 6; Figure 8; Figure 9). Over the range of cohesion and angle of internal friction values we evaluated, FOSmin ranges from >2 to <0.3 (Table 1) and both parameters showed roughly equal influence on the value of FOSmin (Figure 9A). Low cohesion and low angle of internal friction resulted in the least stable failure surfaces and high values for both parameters resulted in the most stable surfaces (Figure 9A). The volume of the FOSmin collapse varied by approximately one order of magnitude over the range of values tested (0.51–9.0 × 106 m3; Table 1 and Figure 9B), and cohesion showed greater influence over the volume compared to the angle of internal friction. Lower cohesion values (<∼100 kPa) favored a smaller volume (∼1-2 x 106 m3) FOSmin failure and higher cohesion values (>∼200 kPa) favored a larger volume (∼8-9 x106 m3), with a sharp transition between two failure surface volumes at cohesion values of ∼100–200 kPa (Figure 9B). For FOSmin failures with similar volumes, the axis of rotation for the FOSmin failure surface was located in the same general area regardless of the FOSmin for that surface (Figure 9C).
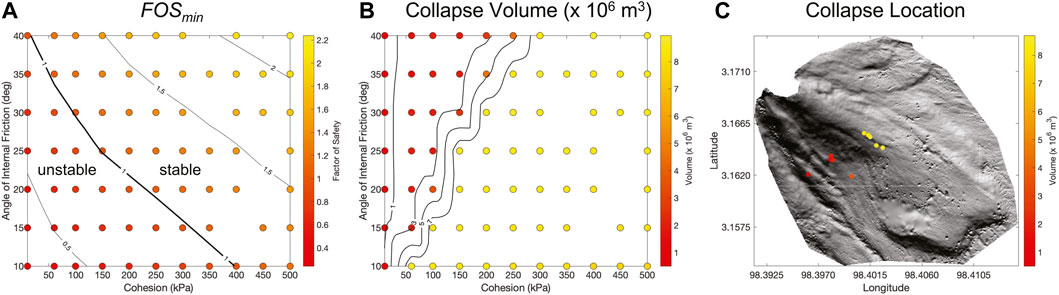
FIGURE 9. Sensitivity of model results to material properties input. Both cohesion and the angle of internal friction strongly affect the FOSmin for the 2014 Sinabung DEM (A). Contours in (A) show the FOS trend over the range of material properties. Each dot represents a run of Scoops3D, and the color corresponds to the FOS. The approximately diagonal orientation of the contours shows the two parameters have roughly equal impact on the FOS. The same plot is shown for the volume of the FOSmin surface in (B) and shows that volume varies by an order of magnitude over the range of input parameters. The more vertically oriented tightly spaced contours indicate a greater influence of a narrow range of cohesion values (∼50–250 kPa) on the volume compared to the angle of internal friction. The location of the axis of rotation for each unstable (FOS < 1) FOSmin failure is plotted in (C) and colored by the volume. This shows that, for an unstable potential failure of a given volume, the location of the collapse is not very sensitive to the input material properties.
5 Discussion
5.1 Erupted Volume and Duration of the Sinabung Eruption
Structure-from-motion photogrammetry has emerged over the past decade as an increasingly powerful and reliable means to survey and analyze lava dome growth (James and Varley, 2012; Thiele et al., 2017; Carr et al., 2019a; James et al., 2020b; Zorn et al., 2020; Andaru et al., 2021; Kelfoun et al., 2021; Moussallam et al., 2021). Our results based on differencing of DEMs created using SfM photogrammetry with images from UAS surveys serve to further constrain the erupted volume and effusion rates at Sinabung when compared to previous estimates using ground-based SfM photogrammetry (Carr et al., 2019a), laser distance measurements (Nakada et al., 2019), and satellite images (Yulianto et al., 2016; Nakada et al., 2019; Pallister et al., 2019; Kriswati and Solikhin, 2020).
Our estimate of the total erupted volume (161.8 ± 7.6 × 106 m3) through June 2018 is within error of the volume estimated by Nakada et al. (2019) for June 2015 (176 × 106 m3 with an estimated 15% error). That our value is less than but similar to that of Nakada et al. (2019) despite being measured 3 years later can be attributed to: 1) the significant slowing of the effusion rate during 2016–2017 (Figure 2) (Global Volcanism Program, 2017; Nakada, 2019), 2) our likely underestimate of the volume due to erupted material deposited or removed beyond the boundaries of our DEM by PDCs, lahars, and ash transport and 3) possible overestimation by Nakada et al. (2019) due to their use of a 30-m pre-eruption DEM compared to the 5-m DEM we use here. Pallister et al. (2019) and Yulianto et al. (2016) both estimate a total erupted volume as of late 2015 of nearly 300 × 106 m3 using DEMs derived from radar or optical satellite images. However, Nakada et al. (2019) visually identified numerous areas where the data of Yulianto et al. (2016) significantly overestimated deposit thicknesses, and both Yulianto et al. (2016) and Pallister et al. (2019) used a 30-m pre-eruption DEM. Thus, these volume estimates are likely overestimates. Kriswati and Solikhin (2020) used satellite thermal images to estimate a total erupted volume of ∼70 × 106 m3 through 2017, but this is a large underestimate caused by the thermal insulation of the lava flow crust, as explained by Carr et al. (2019b).
Our estimate for the lava flow volume of 94 × 106 m3 closely matches previously published estimates of 103 × 106 m3 (Carr et al., 2019a), 109 × 106 m3 (Nakada et al., 2019), 110 × 106 m3 (Pallister et al., 2019), and 90 × 106 m3 (Yulianto et al., 2016). Our volume is generally lower as the other measurements were made in late 2014 or 2015, and do not fully account for the collapse of the upper part of the lava flow as our 2018 measurement does.
Our volume estimates represent final volumes for this first effusive phase of the Sinabung eruption, given that dome growth paused between February 2018 and August 2020 (Global Volcanism Program, 2018a; Global Volcanism Program, 2018b; Global Volcanism Program, 2020). Continuous effusion at Sinabung Volcano thus lasted over 4 years (starting on 18 December 2013, Pallister et al., 2019), with an average effusion rate of ∼1.1 m3 s−1. Of note here is that Wolpert et al. (2016), using a statistical analysis of over 150 lava dome eruptions and data from the first 2 years of Sinabung’s eruption, predicted that Sinabung’s effusive eruption would last 4.19 years, or 1,529 days. This is only a 5-days difference from the span between 18 December 2013 and 19 February 2018 (1,524 days), a remarkable alignment of model with observations that lends strength to applying the statistical model of Wolpert et al. (2016) to assess the potential duration of hazards during dome-forming eruptions.
5.2 Utilization of Slope Stability Modeling as a Monitoring Technique
We demonstrated that the Scoops3D slope stability model can be used to quantitatively assess the stability, location, and volume of potential lava dome collapses during an ongoing eruption. Scoops3D has been used previously to assess the stability of volcanic edifices (e.g., Reid et al., 2000; Ball et al., 2018; Schaefer et al., 2019; Kereszturi et al., 2021), but this is the first time the model has been applied to actively erupting or recently erupted lava. Our results show that active domes can be modeled similarly to other rock masses and that variations in material strength due to temperature, vesicularity, or other lava properties as the eruption progresses can be accounted for by applying a range of material properties as input to Scoops3D.
Scoops3D correctly identified as unstable (FOS < 1) a large portion of the 2014 lava flow that eventually collapsed over the following years (compare Figure 5 to Figure 6). The volume estimated for the least stable region (1.9 × 106 m3, FOS = 0.88) is less than the collapse volume estimated from DEM differencing (10.3 × 106 m3) but is within an order of magnitude. This is expected as Scoops3D output represents a single failure event, while the DEM difference volume captures the cumulative total between 22 September 2014 and 20 June 2018. The simplification of the volcano’s structure made by assuming that the edifice has only two components (pre-eruption and recently erupted material) in terms of material properties also likely contributes some error in the FOS and collapse volumes estimated by Scoops3D. Scoops3D may thus be more accurate for smaller failures, for which the assumed uniformity of material properties is likely more realistic. When the search was limited to smaller volumes, to match the volume of the collapse in early October 2014, Scoops3D identified as least stable nearly the exact location as that which collapsed (Figure 7).
The two-component model we applied for Sinabung’s structure also strongly controls the stability and potential collapse volume determined by Scoops3D. Generally, lower rock strength promotes larger failures (Eppler et al., 1987; Ball et al., 2018; Harnett and Heap, 2021). In this study however, we observed the opposite trend, with less stable conditions favoring smaller volumes (Figure 6; Figure 8). We attribute this discrepancy to the different material properties we use for the pre-eruption edifice and the erupted lava (Table 1). For the weaker lava properties, the lava is weak enough compared to the edifice that the recently erupted lava will fail in small volumes without incorporating much or any of the pre-eruption material. When the lava is stronger (and thus closer to the edifice properties), the erupted lava and edifice can act as one mass and the least stable failure surfaces extend into the edifice, leading to larger potential collapse volumes. In order to more accurately determine likely collapse volumes, it is helpful to identify the location and relative strength of the different materials within the terrain.
Scoops3D is also well-suited as a forward model during an ongoing eruption to assess the risk of lava dome collapse due to passive collapse processes. The software can be implemented and run relatively quickly (a single run from the results presented here typically took less than an hour on a standard laptop computer), making it appropriate for utilization by volcano observatories or other groups to assess evolving hazards. Similarly, UAS are also advantageous as a safe and cost-effective means to collect data in hazardous terrain. Creating SfM models from UAS-captured images can be time-intensive, but it is possible to modify a UAS survey (e.g., reduce the number of photos needed by taking photos from a greater distance or using a wider lens) and streamline the processing time (e.g., use lower quality settings in Metashape™) as part of a balance between the spatial and temporal (e.g., daily, weekly, monthly, etc.) resolution needed. Changes in stability during an eruption can be tracked by repeatedly creating DEMs as dome volume and geometry change and by varying the material properties to represent changes in dome strength due to cooling, heating associated with endogenous dome growth, or hydrothermal alteration. Additionally, Scoops3D output for the location and volume of the least stable potential failure can be used as input for models of PDCs such as VolcFlow (Kelfoun and Druitt, 2005) or Titan2D (Patra et al., 2005; Charbonnier and Gertisser, 2012) to quantify the runout distance and hazards associated with the block-and-ash flows that result from dome collapse (e.g., Kereszturi et al., 2021).
We use Scoops3D here to assess the potential for passive collapse due to the dome topography at a specific point in time. Forecasting active dome collapses associated with dome growth will require running Scoops3D on continuously updated topographic data and using time-dependent material properties. While possible, this would be a major undertaking. Alternative approaches such as using images from time lapse cameras or DEMs from frequent repeated surveys to track the velocity of the dome surface, as recently demonstrated by Zorn et al. (2020), Kelfoun et al. (2021), and Moussallam et al. (2021), could be more effective during active growth periods. Another limitation is that Scoops3D cannot currently assess instabilities specifically due to pressurization of gas. Gas overpressure can be partially accounted for by reducing the cohesion, but this effect is not separable from other processes that can reduce cohesion. While Scoops3D is capable of handling spatially variable pore pressure due to fluids such as ground water, this capability is not directly tunable to treat the effect of overpressured, gas-rich zones in recently erupted lava.
The ability to accurately constrain the material properties of the lava is the greatest source of uncertainty when applying Scoops3D to quantitatively assess dome collapse hazards. Here, we have shown that values for cohesion and angle of internal friction used in previous studies are likely reasonable values to use when applying Scoops3D to lava domes. Our results also highlight the importance of scale with respect to internal properties such as cohesion and internal friction angle. Laboratory measurements for the strength of andesite and other types of dome lava are 1-2 orders of magnitude greater than values for potential failure masses in nature (Heap et al., 2021; Kereszturi et al., 2021; Villeneuve and Heap, 2021). Our results agree more with material parameters estimated from analysis of natural failures (Heap et al., 2021; Kereszturi et al., 2021). Thus, laboratory measurements should be carefully ‘upscaled’ before being utilized in stability analysis, to account for large-scale fractures and/or alteration that do not appear in the small, intact samples used in laboratory analyses (Heap et al., 2021; Villeneuve and Heap, 2021).
Uncertainty can be further reduced by comparing model output to observed collapses, as we do here to demonstrate weaker material properties produced a better fit to observations. In cases where observations cannot be used to further constrain the most appropriate material properties for a specific case, forward modeling of dome stability should include scenarios with a range of lava strengths. The “weaker” scenarios can also be thought of as accounting for the potential of decreased stability due to gas overpressure, rainfall (e.g., Elsworth et al., 2004; Darmawan et al., 2018), or other external events (e.g., earthquake loading), in the absence of well-constrained values for the Scoops3D input parameters ui,j and keqei,j in Eq. 3.
We additionally demonstrated that while the FOS is strongly affected by the material properties, the location of FOSmin failures with similar volumes is not significantly impacted by the material strength. Material strength (primarily cohesion) controls whether the FOSmin surface has a relatively large or relatively small volume, but the location of the failure surface for a given volume range is generally constant (Figure 9C). Thus, even when quantitative assessment of stability is not accurate due to poorly constrained material properties, a relative assessment of lava dome stability remains possible. The output from Scoops3D is useful for hazard assessment in this case through its ability to identify the most likely location and potential range of collapse volume, if one were to occur.
Our results using our 2018 DEM highlight an often-overlooked hazard at dome-forming eruptions, which is the continued risk of collapse even after effusion has paused or stopped (e.g., Calder et al., 2002). Collapses can create oversteepened scarps in the remaining lava (Figure 7C), cooling of the lava can reduce the cohesion (Voight and Elsworth, 2000), and hydrothermal alteration from rainwater and gases percolating through fractures can weaken the material strength (Platz et al., 2012; Ball et al., 2018; Heap et al., 2021; Kereszturi et al., 2021). Post-eruptive collapse should be accounted for when considering persistent hazards at a volcano following its eruption and can be assessed using Scoops3D as we have demonstrated.
5.3 Dome Collapse Processes and the Sinabung Eruption
The material properties used to assess dome stability provide insight into the physical characteristics of lava at Sinabung. The porosity (i.e., vesicularity) of lava is a primary control on its strength (Zorn et al., 2018), with cohesion and the angle of internal friction decreasing as porosity increases (Heap et al., 2014; Villeneuve and Heap, 2021). Zorn et al. (2018) found that lower rock strength correlated with higher ascent rates, since faster ascent led to higher porosity and lower groundmass crystallinity in the erupted lava. The weaker material properties we used to represent the 2014 collapse at Sinabung (Table 1) support an interpretation that collapsed Sinabung dome lavas had higher porosity and ascended relatively quickly. Zorn et al. (2018) defines higher porosity as > 23% and describes an effusion rate of 4.6 m3/s at Unzen as high ascent rate. Nakada et al. (2019) measured up to 58% porosity in samples from PDC deposits at Sinabung, and Carr et al. (2019a) calculated an average effusion rate from January to September 2014 of 4.8 m3/s, in agreement with our interpretation based on the material properties.
Our stability assessment of Sinabung based on the 2018 DEM was valid for a little over 2 years. When a new dome grew at the summit in the second half of 2020, it changed the topography and stability conditions, rendering our analysis out-of-date. Based on observations of Sinabung during this 2018–2020 period (Global Volcanism Program, 2019; Global Volcanism Program, 2020; Global Volcanism Program, 2021), it does not appear any major collapses occurred from the regions identified as unstable in Figure 8. This suggests stable (i.e., FOS > 1) conditions existed following our 2018 field work and that the weaker material properties we apply to identify unstable areas in the 2014 DEM (Figure 6A) were less representative of the erupted lava during 2018–2020. One possible explanation is that heterogeneous distribution of porosity, internal gas pressure, and/or fracturing created more localized areas of weakness that collapsed in 2014–2018 while the remaining material was generally stable. Another possibility is that the material properties of the erupted lava strengthened as time increased since the lava was emplaced.
Many different processes contributed to the development of instabilities in erupted lava at Sinabung. The collapses observed during the eruption broadly reinforce existing understanding of the relationships between the driving mechanisms and the resulting collapses. Most of the collapses at Sinabung were caused by active processes associated with the exogenous growth of a lava dome or the advance of a lava flow front over steep topography (Carr et al., 2019a; Carr et al., 2019b; Nakada et al., 2019). These collapses were generally smaller compared to collapses driven by gas depressurization or overtopping of topography (Nakada et al., 2019) and were most common in early 2014 when effusion and flow advance rates were highest (Carr et al., 2019a; Carr et al., 2019b; Nakada et al., 2019; Pallister et al., 2019; Kriswati and Solikhin, 2020). These observations are consistent with previous studies showing that exogenous growth generates relatively small, frequent collapses that correlate to a varying effusion rate (Calder et al., 2002; Harnett et al., 2019). The largest collapse events at Sinabung were associated with explosions caused by gas pressurization within the erupted lava (Global Volcanism Program, 2019; Nakada et al., 2019; Pallister et al., 2019), a common trend observed at many other dome-forming eruptions (Voight and Elsworth, 2000; Calder et al., 2002; Harnett et al., 2019).
The passive process of a dome overtopping topography during endogenous growth also produced relatively large, though rare, collapses during Sinabung’s eruption. This is again consistent with Harnett et al. (2018); Harnett et al. (2019), who found large collapses (both active and passive) were more commonly associated with endogenous growth, and Calder et al. (2002), who described passive collapses occurring with lower frequency than active collapses. Passive collapses at Sinabung also further highlight the ability of passive processes to significantly change eruption hazards, due to their ability to generate instability in regions away from the location of active growth and gas pressurization in the dome.
A crucial observation of dome collapse of all styles during Sinabung’s eruption is that the potential hazard from PDCs did not decrease along with decreasing eruption rate. While the frequency of collapses decreased as the eruption rate decreased (Figure 2), the volumes of the collapses and the runout distances of the associated PDCs did not (Kriswati and Solikhin, 2020). This agrees with the observations of Harnett et al. (2019) in their survey of data from multiple lava dome eruptions. At Sinabung, the processes of gravitational loading and gas pressurization (a passive and an active process, respectively) remained capable of producing PDCs with runouts approaching 5 km throughout the course of the eruption, including after dome growth paused (Global Volcanism Program, 2019; Nakada et al., 2019; Pallister et al., 2019; Kriswati and Solikhin, 2020).
The elevated persistent hazard that results from processes capable of producing large collapses at infrequent intervals demonstrates the importance of identifying and understanding all potential collapse hazards during and after a dome-forming eruption. The method we apply in this study presents a means to assess hazards from passive collapses both during and after an eruption through a powerful combination of repeated high-resolution topographic surveys and slope stability analysis. Thanks to the advances and increased availability of tools such as UAS and SfM, repeat DEM generation can now be conducted daily if necessary. With these repeated topographic models as input, passive processes related to both confining topography and internal dome strength can be assessed using slope stability models such as Scoops3D. This combination allows continuous assessment of changing hazards during and after an eruption (e.g., if the lava is becoming more or less stable and how fast this change is occurring), representing a useful addition to monitoring techniques for dome-forming volcanic eruptions.
6 Conclusion
We develop a new workflow for using topographic models derived by photogrammetry from UAS-captured imagery and the Scoops3D slope stability model to assess the passive collapse hazard of lava domes. We find that the Scoops3D model can accurately identify as unstable (FOS < 1) regions of erupted lava at Sinabung Volcano that later collapsed. By restricting the size of potential failure volumes, Scoops3D finds a FOSmin indicative of instability (0.92) with a failure surface located in the same location as the initial passive collapse that occurred in early October 2014. Thus, Scoops3D was able to accurately hindcast the collapse hazard and location at Sinabung. Additionally, we find that passive collapse of erupted lava at Sinabung remains a potentially significant hazard, with an FOSmin of 0.9 and potential collapse volume of 1.4 × 106 m3 based on the 2018 DEM. While the material properties of an active lava dome or flow are difficult to accurately constrain, we demonstrate that the location of the most likely collapse site for a given volume range is not sensitive to the material properties within a feasible range. While the likelihood of collapse (based on whether or not FOS is < or >1) may not be possible to know with certainty, the most likely location and potential size of a collapse can still be identified and used for hazard assessment and mitigation planning.
Dome collapses during the eruption of Sinabung Volcano occurred due to many different processes and remained a significant hazard as the eruption waned. Passive collapse due to lava overtopping topography produced large collapses and led to a change in eruption style in late 2014. Our method is especially useful here as passive collapses are often difficult to identify and anticipate as they do not correlate with other measures of eruptive activity as active collapse processes do. It therefore complements the monitoring of effusion rate as a proxy for the frequency of active collapses and allows for a more complete assessment of lava collapse hazards at active or recently active volcanoes.
Data Availability Statement
The images used for photogrammetry, DEMs, and point clouds for Sinabung Volcano are available through OpenTopography.org at https://doi.org/10.5069/G97S7KWC (2014) and https://doi.org/10.5069/G9988568 (2018).
Author Contributions
BC and EL conceptualized and designed the study. BC, LV, GM and AC planned and facilitated the field work. BC, LV and DM participated in the field work and collected data. BC processed the data, conducted the modeling, and wrote the first draft of the manuscript. All authors contributed to manuscript revision, read, and approved the submitted version.
Funding
This work was supported by NSF EAR Postdoctoral Fellowship Award #1725768. EL acknowledges support from NSF EAR Award #1654588. LV and DM were supported by the 2017 Drexel University Antelo Devereux award. Field work in Indonesia was conducted under a memorandum of understanding between Arizona State University (Tempe, AZ, United States) and Universitas Gadjah Mada (Yogyakarta, Indonesia). The 2010 DEM was provided by the Badan Informasi Geospasial and the Center for Volcanology and Geological Hazard Management (CVGHM) in Indonesia. Christine Sealing and Emily Carey of Drexel University (Philadelphia, PA, United States) and Annisa Nurina Adani and Riski Budi Pratiwi of Universitas Gadjah Mada (Yogyakarta, Indonesia) assisted with data collection at Sinabung Volcano.
Conflict of Interest
The authors declare that the research was conducted in the absence of any commercial or financial relationships that could be construed as a potential conflict of interest.
Publisher’s Note
All claims expressed in this article are solely those of the authors and do not necessarily represent those of their affiliated organizations, or those of the publisher, the editors, and the reviewers. Any product that may be evaluated in this article, or claim that may be made by its manufacturer, is not guaranteed or endorsed by the publisher.
Supplementary Material
The Supplementary Material for this article can be found online at: https://www.frontiersin.org/articles/10.3389/feart.2022.813813/full#supplementary-material
References
Andaru, R., Rau, J.-Y., Syahbana, D. K., Prayoga, A. S., and Purnamasari, H. D. (2021). The Use of UAV Remote Sensing for Observing Lava Dome Emplacement and Areas of Potential Lahar Hazards: An Example from the 2017-2019 Eruption Crisis at Mount Agung in Bali. J. Volcanology Geothermal Res. 415, 107255. doi:10.1016/j.jvolgeores.2021.107255
Ball, J. L., Taron, J., Reid, M. E., Hurwitz, S., Finn, C., and Bedrosian, P. (2018). Combining Multiphase Groundwater Flow and Slope Stability Models to Assess Stratovolcano Flank Collapse in the Cascade Range. J. Geophys. Res. Solid Earth 123, 2787–2805. doi:10.1002/2017JB015156
Besl, P. J., and McKay, N. D. (1992). A Method for Registration of 3-D Shapes. IEEE Trans. Pattern Anal. Mach. Intell. 14, 239–256. doi:10.1109/34.121791
Calder, E. S., Luckett, R., Sparks, R. S. J., and Voight, B. (2002). Mechanisms of Lava Dome Instability and Generation of Rockfalls and Pyroclastic Flows at Soufrière Hills Volcano, Montserrat. Geol. Soc. Lond. Mem. 21, 173–190. Druitt, T. H., Kokelaar, B. P. (Eds.). doi:10.1144/GSL.MEM.2002.021.01.08
Carr, B. B., Clarke, A. B., and Vanderkluysen, L. (2016). The 2006 Lava Dome Eruption of Merapi Volcano (Indonesia): Detailed Analysis Using MODIS TIR. J. Volcanology Geothermal Res. 311, 60–71. doi:10.1016/j.jvolgeores.2015.12.004
Carr, B. B., Clarke, A. B., Arrowsmith, J. R., Vanderkluysen, L., and Dhanu, B. E. (2019a). The Emplacement of the Active Lava Flow at Sinabung Volcano, Sumatra, Indonesia, Documented by Structure-From-Motion Photogrammetry. J. Volcanology Geothermal Res. 382, 164–172. doi:10.1016/j.jvolgeores.2018.02.004
Carr, B. B., Clarke, A. B., Vanderkluysen, L., and Arrowsmith, J. R. (2019b). Mechanisms of Lava Flow Emplacement during an Effusive Eruption of Sinabung Volcano (Sumatra, Indonesia). J. Volcanology Geothermal Res. 382, 137–148. doi:10.1016/j.jvolgeores.2018.03.002
Charbonnier, S. J., and Gertisser, R. (2008). Field Observations and Surface Characteristics of Pristine Block-And-Ash Flow Deposits from the 2006 Eruption of Merapi Volcano, Java, Indonesia. J. Volcanology Geothermal Res. 177, 971–982. doi:10.1016/j.jvolgeores.2008.07.008
Charbonnier, S. J., and Gertisser, R. (2012). Evaluation of Geophysical Mass Flow Models Using the 2006 Block-And-Ash Flows of Merapi Volcano, Java, Indonesia: Towards a Short-Term hazard Assessment Tool. J. Volcanology Geothermal Res. 231-232, 87–108. doi:10.1016/j.jvolgeores.2012.02.015
Civico, R., Ricci, T., Scarlato, P., Andronico, D., Cantarero, M., Carr, B. B., et al. (2021). Unoccupied Aircraft Systems (UASs) Reveal the Morphological Changes at Stromboli Volcano (Italy) Before, Between, and After the 3 July and 28 August 2019 Paroxysmal Eruptions. Remote Sensing 13, 2870. doi:10.3390/rs13152870
Darmawan, H., Walter, T. R., Brotopuspito, K. S., Subandriyo , , and Nandaka, I. G. M. A. (2018). Morphological and Structural Changes at the Merapi Lava Dome Monitored in 2012-15 Using Unmanned Aerial Vehicles (UAVs). J. Volcanology Geothermal Res. 349, 256–267. doi:10.1016/j.jvolgeores.2017.11.006
Elsworth, D., Voight, B., Thompson, G., and Young, S. R. (2004). Thermal-Hydrologic Mechanism for Rainfall-Triggered Collapse of Lava Domes. Geol. 32, 969–972. doi:10.1130/g20730.1
Eppler, D. B., Fink, J., and Fletcher, R. (1987). Rheologic Properties and Kinematics of Emplacement of the Chaos Jumbles Rockfall Avalanche, Lassen Volcanic National Park, California. J. Geophys. Res. 92, 3623. doi:10.1029/jb092ib05p03623
Global Volcanism Program (2017). “Report on Sinabung (Indonesia),” in Bulletin of the Global Volcanism Network. Editors A.E. Crafford, and E. Venzke (Washington, DC: Smithsonian Institution), 42, 12. doi:10.5479/si.GVP.BGVN201712-261080
Global Volcanism Program (2018a). “Report on Sinabung (Indonesia),” in Bulletin of the Global Volcanism Network. Editors A.E. Crafford, and E. Venzke (Washington, DC: Smithsonian Institution), 43, 4. doi:10.5479/si.GVP.BGVN201804-261080
Global Volcanism Program (2018b). “Report on Sinabung (Indonesia),” in Bulletin of the Global Volcanism Network. Editors J.B. Krippner, and E. Venzke (Washington, DC: Smithsonian Institution), 43, 9. doi:10.5479/si.GVP.BGVN201809-261080
Global Volcanism Program (2019). “Report on Sinabung (Indonesia),” in Bulletin of the Global Volcanism Network. Editors A.E. Crafford, and E. Venzke (Washington, DC: Smithsonian Institution), 44, 8. doi:10.5479/si.GVP.BGVN201908-261080
Global Volcanism Program (2020). “Report on Sinabung (Indonesia),” in Bulletin of the Global Volcanism Network. Editors A. E. Crafford, and E. Venzke (Washington, DC: Smithsonian Institution), 45, 11. doi:10.5479/si.GVP.BGVN202011-261080
Global Volcanism Program (2021). “Report on Sinabung (Indonesia),” in Bulletin of the Global Volcanism Network. Editors A. E. Crafford, and E. Venzke (Washington, DC: Smithsonian Institution), 46, 8. doi:10.5479/si.GVP.BGVN202108-261080
Gunawan, H., SuronoBudianto, A., BudiantoPrambada, A., Kristianto, W., Prambada, O., McCausland, W., et al. (2019). Overview of the Eruptions of Sinabung Volcano, 2010 and 2013-Present and Details of the 2013 Phreatomagmatic Phase. J. Volcanology Geothermal Res. 382, 103–119. doi:10.1016/j.jvolgeores.2017.08.005
Harnett, C. E., and Heap, M. J. (2021). Mechanical and Topographic Factors Influencing Lava Dome Growth and Collapse. J. Volcanology Geothermal Res. 420, 107398. doi:10.1016/j.jvolgeores.2021.107398
Harnett, C. E., Thomas, M. E., Purvance, M. D., and Neuberg, J. (2018). Using a Discrete Element Approach to Model Lava Dome Emplacement and Collapse. J. Volcanology Geothermal Res. 359, 68–77. doi:10.1016/j.jvolgeores.2018.06.017
Harnett, C. E., Thomas, M. E., Calder, E. S., Ebmeier, S. K., Telford, A., Murphy, W., et al. (2019). Presentation and Analysis of a Worldwide Database for Lava Dome Collapse Events: the Global Archive of Dome Instabilities (GLADIS). Bull. Volcanol. 81, 16. doi:10.1007/s00445-019-1276-y
Harris, A. J. L., Flynn, L. P., Matías, O., and Rose, W. I. (2002). The thermal Stealth Flows of Santiaguito Dome, Guatemala: Implications for the Cooling and Emplacement of Dacitic Block-Lava Flows. GSA Bull. 114, 533–546. doi:10.1130/0016-760610.1130/0016-7606(2002)114<0533:ttsfos>2.0.co;2
Heap, M. J., Lavallée, Y., Petrakova, L., Baud, P., Reuschlé, T., Varley, N. R., et al. (2014). Microstructural Controls on the Physical and Mechanical Properties of Edifice‐Forming Andesites at Volcán de Colima, Mexico. J. Geophys. Res. Solid Earth 119, 2925–2963. doi:10.1002/2013jb010521
Heap, M. J., Baumann, T. S., Rosas‐Carbajal, M., Komorowski, J. C., Gilg, H. A., Villeneuve, M., et al. (2021). Alteration‐Induced Volcano Instability at La Soufrière de Guadeloupe (Eastern Caribbean). JGR Solid Earth 126, e2021JB022514. doi:10.1029/2021jb022514
James, M. R., and Robson, S. (2012). Straightforward Reconstruction of 3D Surfaces and Topography with a Camera: Accuracy and Geoscience Application. J. Geophys. Res. 117, F03017. doi:10.1029/2011JF002289
James, M. R., and Varley, N. (2012). Identification of Structural Controls in an Active lava Dome with High Resolution DEMs: Volcán de Colima, Mexico. Geophys. Res. Lett. 39, L22303. doi:10.1029/2012GL054245
James, M. R., Robson, S., and Smith, M. W. (2017). 3-D Uncertainty-Based Topographic Change Detection with Structure-From-Motion Photogrammetry: Precision Maps for Ground Control and Directly Georeferenced Surveys. Earth Surf. Process. Landforms 42, 1769–1788. doi:10.1002/esp.4125
James, M. R., Carr, B., D'Arcy, F., Diefenbach, A., Dietterich, H., Fornaciai, A., et al. (2020b). Volcanological Applications of Unoccupied Aircraft Systems (UAS): Developments, Strategies, and Future Challenges. Volcanica 3, 67–114. doi:10.30909/vol.03.01.67114
James, M. R., Antoniazza, G., Robson, S., and Lane, S. N. (2020a). Mitigating Systematic Error in Topographic Models for Geomorphic Change Detection: Accuracy, Precision and Considerations Beyond Off‐Nadir Imagery. Earth Surf. Process. Landforms 45, 2251–2271. doi:10.1002/esp.4878
Kelfoun, K., and Druitt, T. H. (2005). Numerical Modeling of the Emplacement of Socompa Rock Avalanche, Chile. J. Geophys. Res. 110, B12202. doi:10.1029/2005jb003758
Kelfoun, K., Santoso, A. B., Latchimy, T., Bontemps, M., Nurdien, I., Beauducel, F., et al. (2021). Growth and Collapse of the 2018-2019 Lava Dome of Merapi Volcano. Bull. Volcanol. 83, 8. doi:10.1007/s00445-020-01428-x
Kereszturi, G., Schaefer, L., Mead, S., Miller, C., Procter, J., and Kennedy, B. (2021). Synthesis of Hydrothermal Alteration, Rock Mechanics and Geophysical Mapping to Constrain Failure and Debris Avalanche Hazards at Mt. Ruapehu (New Zealand). New Zealand J. Geology. Geophys. 64, 1–22. doi:10.1080/00288306.2021.1885048
Kriswati, E., and Solikhin, A. (2020). Lava Discharge Rate of Sinabung Volcano Obtained from Modis Hot Spot Data. Indonesian J. Geosci. 7, 241–252. doi:10.17014/ijog.7.3.241-252
Lague, D., Brodu, N., and Leroux, J. (2013). Accurate 3D Comparison of Complex Topography with Terrestrial Laser Scanner: Application to the Rangitikei Canyon (N-Z). ISPRS J. Photogrammetry Remote Sensing 82, 10–26. doi:10.1016/j.isprsjprs.2013.04.009
Lesage, P., Carrara, A., Pinel, V., and Arámbula-Mendoza, R. (2018). Absence of Detectable Precursory Deformation and Velocity Variation Before the Large Dome Collapse of July 2015 at Volcán de Colima, Mexico. Front. Earth Sci. 6, 93. doi:10.3389/feart.2018.00093
Moussallam, Y., Barnie, T., Amigo, Á., Kelfoun, K., Flores, F., Franco, L., et al. (2021). Monitoring and Forecasting Hazards from a Slow Growing Lava Dome Using Aerial Imagery, Tri-Stereo Pleiades-1A/B Imagery and PDC Numerical Simulation. Earth Planet. Sci. Lett. 564, 116906. doi:10.1016/j.epsl.2021.116906
Nakada, S., Shimizu, H., and Ohta, K. (1999). Overview of the 1990-1995 Eruption at Unzen Volcano. J. Volcanology Geothermal Res. 89, 1–22. doi:10.1016/S0377-0273(98)00118-8
Nakada, S., Zaennudin, A., Yoshimoto, M., Maeno, F., Suzuki, Y., Hokanishi, N., et al. (2019). Growth Process of the Lava Dome/Flow Complex at Sinabung Volcano During 2013-2016. J. Volcanology Geothermal Res. 382, 120–136. doi:10.1016/j.jvolgeores.2017.06.012
Nakada, S. (2019). “Causes and Consequences of Lava Dome Eruptions,” in IUGG General Assembly, Montréal, QC, July 8 - 18, 2019. IUGG19-2760.
Pallister, J., Wessels, R., Griswold, J., McCausland, W., Kartadinata, N., Gunawan, H., et al. (2019). Monitoring, Forecasting Collapse Events, and Mapping Pyroclastic Deposits at Sinabung Volcano with Satellite Imagery. J. Volcanology Geothermal Res. 382, 149–163. doi:10.1016/j.jvolgeores.2018.05.012
Patra, A. K., Bauer, A. C., Nichita, C. C., Pitman, E. B., Sheridan, M. F., Bursik, M., et al. (2005). Parallel Adaptive Numerical Simulation of Dry Avalanches Over Natural Terrain. J. Volcanology Geothermal Res. 139, 1–21. doi:10.1016/j.jvolgeores.2004.06.014
Platz, T., Cronin, S. J., Procter, J. N., Neall, V. E., and Foley, S. F. (2012). Non-Explosive, Dome-Forming Eruptions at Mt. Taranaki, New Zealand. Geomorphology 136, 15–30. doi:10.1016/j.geomorph.2011.06.016
Reid, M. E., Christian, S. B., and Brien, D. L. (2000). Gravitational Stability of Three-Dimensional Stratovolcano Edifices. J. Geophys. Res. 105, 6043–6056. doi:10.1029/1999JB900310
Reid, M. E., Christian, S. B., Brien, D. L., and Henderson, S. T. (2015). Scoops3D: Software to Analyze 3D Slope Stability Throughout a Digital Landscape, 14. Reston, VA: U.S. Geological Survey Techniques and Methods, 218. Book Chap. A1. doi:10.3133/tm14A1
Schaefer, L. N., Di Traglia, F., Chaussard, E., Lu, Z., Nolesini, T., and Casagli, N. (2019). Monitoring Volcano Slope Instability with Synthetic Aperture Radar: A Review and New Data from Pacaya (Guatemala) and Stromboli (Italy) Volcanoes. Earth-Science Rev. 192, 236–257. doi:10.1016/j.earscirev.2019.03.009
Simmons, J., Elsworth, D., and Voight, B. (2005). Classification and Idealized Limit-Equilibrium Analyses of Dome Collapses at Soufrière Hills Volcano, Montserrat, During Growth of the First Lava Dome: November 1995-March 1998. J. Volcanology Geothermal Res. 139, 241–258. doi:10.1016/j.jvolgeores.2004.08.009
Snavely, N., Seitz, S. M., and Szeliski, R. (2008). Modeling the World from Internet Photo Collections. Int. J. Comput. Vis. 80, 189–210. doi:10.1007/s11263-007-0107-3
Sparks, R. S. J., and Aspinall, W. P. (2004). “Volcanic Activity: Frontiers and Challenges in Forecasting, Prediction and Risk Assessment,” in The State of the Planet: Frontiers and Challenges in Geophysics, IUGG Monograph. Editors R. S. J. Sparks, and C. J. Hawkesworth (New York: AGU), 19, 359–373. doi:10.1029/150gm28
Thiele, S. T., Varley, N., and James, M. R. (2017). Thermal Photogrammetric Imaging: A New Technique for Monitoring Dome Eruptions. J. Volcanology Geothermal Res. 337, 140–145. doi:10.1016/j.jvolgeores.2017.03.022
Villeneuve, M., and Heap, M. (2021). Calculating the Cohesion and Internal Friction Angle of Volcanic Rocks and Rock Masses. Volcanica 4, 279–293. doi:10.30909/vol.04.02.279293
Voight, B., and Elsworth, D. (2000). Instability and Collapse of Hazardous Gas-Pressurized Lava Domes. Geophys. Res. Lett. 27, 1–4. doi:10.1029/1999GL008389
Voight, B., Constantine, E. K., Siswowidjoyo, S., and Torley, R. (2000). Historical Eruptions of Merapi Volcano, Central Java, Indonesia, 1768-1998. J. Volcanology Geothermal Res. 100, 69–138. doi:10.1016/S0377-0273(00)00134-7
Voight, B. (2000). Structural Stability of Andesite Volcanoes and Lava Domes. Phil. Trans. R. Soc. Lond. Ser. A: Math. Phys. Eng. Sci. 358, 1663–1703. doi:10.1098/rsta.2000.0609
Westoby, M. J., Brasington, J., Glasser, N. F., Hambrey, M. J., and Reynolds, J. M. (2012). ‘Structure-from-Motion' Photogrammetry: A Low-Cost, Effective Tool for Geoscience Applications. Geomorphology 179, 300–314. doi:10.1016/j.geomorph.2012.08.021
Wolpert, R. L., Ogburn, S. E., and Calder, E. S. (2016). The Longevity of Lava Dome Eruptions. J. Geophys. Res. Solid Earth 121, 676–686. doi:10.1002/2015JB012435
Yulianto, F., Suwarsono , , and Sofan, P. (2016). The Utilization of Remotely Sensed Data to Analyze the Estimated Volume of Pyroclastic Deposits and Morphological Changes Caused by the 2010-2015 Eruption of Sinabung Volcano, North Sumatra, Indonesia. Pure Appl. Geophys. 173, 2711–2725. doi:10.1007/s00024-016-1342-8
Zorn, E. U., Rowe, M. C., Cronin, S. J., Ryan, A. G., Kennedy, L. A., and Russell, J. K. (2018). Influence of Porosity and Groundmass Crystallinity on Dome Rock Strength: A Case Study from Mt. Taranaki, New Zealand. Bull. Volcanol 80, 35. doi:10.1007/s00445-018-1210-8
Keywords: lava domes, lava dome collapse, Sinabung volcano, hazard assessment, unoccupied aerial systems, structure-from-motion photogrammetry
Citation: Carr BB, Lev E, Vanderkluysen L, Moyer D, Marliyani GI and Clarke AB (2022) The Stability and Collapse of Lava Domes: Insight From Photogrammetry and Slope Stability Models Applied to Sinabung Volcano (Indonesia). Front. Earth Sci. 10:813813. doi: 10.3389/feart.2022.813813
Received: 12 November 2021; Accepted: 05 April 2022;
Published: 27 May 2022.
Edited by:
Emma J. Liu, University College London, United KingdomReviewed by:
Lucia Capra, National Autonomous University of Mexico, MexicoClaire E. Harnett, University of Leeds, United Kingdom
Karim Kelfoun, Université Clermont Auvergne, France
Edgar Ulrich Zorn, GFZ German Research Centre for Geosciences, Germany
Copyright © 2022 Carr, Lev, Vanderkluysen, Moyer, Marliyani and Clarke. This is an open-access article distributed under the terms of the Creative Commons Attribution License (CC BY). The use, distribution or reproduction in other forums is permitted, provided the original author(s) and the copyright owner(s) are credited and that the original publication in this journal is cited, in accordance with accepted academic practice. No use, distribution or reproduction is permitted which does not comply with these terms.
*Correspondence: Brett B. Carr, YmJjYXJyQGFyaXpvbmEuZWR1
†Present address: Brett B. Carr, Lunar and Planetary Laboratory, University of Arizona, Tucson, AZ, United States