- 1College of Energy, Chengdu University of Technology, Chengdu, China
- 2State Key Laboratory of Oil and Gas Reservoir Geology and Exploitation, Chengdu University of Technology, Chengdu, China
- 3Overseas Business Department, Geophysical Research Institute, Bureau of Geophysical Prospecting, CNPC, Zhuozhou, China
Predicting the distribution of fractured and dissolved reservoirs in granitic buried hills is a prerequisite for finding high-quality hydrocarbon reservoirs and improving the success rate of exploration activities. Granitic buried-hill reservoirs in the Bongor Basin are characterized by strong heterogeneity and poor lateral continuity. Through the analysis of seismic, core, logging and dynamic production data, this study predicts the distribution of fractured reservoirs and fractured-cave reservoirs in the granitic buried hills in the BC Block of the Bongor Basin. In this study, we predict fractured reservoirs using coherence cubes and linearly enhanced attributes and identify fractured-cave reservoirs with single-frequency attribute bodies. Integrated predictions of fractured reservoirs and fractured-cave reservoirs are conducted using attribute fusion techniques. With these methods, good results have been achieved in reservoir prediction in BC buried hills. Furthermore, this study summarizes a set of granitic buried-hill reservoir prediction techniques for densely inverted rift basins in the Central African Rift System.
1 Introduction
Granite, as a dense rock formed by magma crystallization, has no primary pores. In addition, granite is hard to detect when buried thousands of metres underground, resulting in little attention to be paid during hydrocarbon exploration (Ye et al., 2020; Yi et al., 2021). However, with the development of global oil and gas exploration, breakthroughs have been made in granitic buried hills worldwide, and a few medium- to large-sized oil and gas fields have been discovered, such as the BachHo field in Vietnam, the Dongping gas field in China, the Penglai oil field in China, and the BC oilfield discovered in the Bongor Basin in Chad (Cuong and Warren, 2009; Dou et al., 2015; Chen et al., 2018; Guo et al., 2019; Li et al., 2021a; Dai et al., 2021). The exploration of buried-hill reservoirs in the basement has gradually become a new exploration field (Cuong and Warren, 2009; Dou et al., 2020; Ye et al., 2020).
In 2013, the success of hydrocarbon drilling in the northern slope of the basin by the China National Petroleum Corporation (CNPC) targeting Precambrian granitic reservoirs expanded the boundary of petroleum exploration in the Bongor Basin, and industrial amounts of oil and gas were obtained from the granitic reservoirs of five buried hills (Dou et al., 2015; Chen et al., 2018). This reveals the huge exploration potential of the bedrock buried-hill reservoir in the Bongor Basin. Therefore, the Precambrian basement is a good reservoir for hydrocarbon accumulation (Li et al., 2017; Dou et al., 2018).
The Bongor Basin is an important hydrocarbon-rich basin in southwestern Chad. Preliminary exploration results reveal the huge exploration potential of bedrock buried-hill reservoirs in this area (Dou et al., 2015; Li et al., 2017; Chen et al., 2018; Dou et al., 2018). Scholars have reached an agreement that granitic reservoirs are mainly composed of secondary reservoir spaces, including structural fractures, weathering fractures, dissolved fractures and dissolved pores, and the physical properties change tremendously spatially, showing strong heterogeneity (Salah and Alsharhan, 1998; Cuong and Warren, 2009; Wang et al., 2018). Longitudinally, there is a certain zonality, but it is not obvious. Maerten and Panza proposed that the basement buried-hill reservoir consists mainly of structural fractures and caves formed by later modification. With the increase in depth below the buried hill surface, the type of basement buried-hill reservoir gradually transitions from a fractured-cave reservoir to a fractured reservoir. Through the calibration of the core and well seismic data, the oil-producing section of the well is mainly concentrated in the upper part of the buried hill (Wang et al., 2018; Dou et al., 2020). Therefore, the predictions of buried-hill reservoirs in the BC block are mainly fractured and fractured-cave reservoirs.
Fractures play a key role in basement buried-hill reservoirs and are important reservoirs and channels that communicate with reservoir space (Maerten et al., 2018; Panza et al., 2018; Shang et al., 2021). Therefore, the prediction of fractures is a key and difficult aspect of exploration and exploitation in basement buried-hill reservoirs (Salah and Alsharhan, 1998; Yan et al., 2019).
For the study of fractured reservoirs in the subsurface, outcrops and core observations were initially used, but they have the disadvantage of being less accurate and limited to areas with drilled wells. Logging evaluation is also an effective method for identifying and evaluating fractures. In recent years, emphasis has been placed on the combined application of multiple logging profiles to identify and evaluate fractured reservoirs (Li et al., 2021b). The combined application of multiple logs has improved the accuracy and precision compared to direct observation methods, but for areas with limited wells, only mathematical algorithms can be used to interpolate and predict the planimetric distribution of basement buried-hill reservoirs (Ye, 2019). The use of seismic detection technology for fracture prediction began later; however, because seismic data cover a much wider space than drilling data, have a greater range of applications and are more practical, they are more generally used (Gong et al., 2013; Zhang et al., 2022). At present, the main techniques for the fracture prediction of reservoirs using seismic data are shear wave exploration, multiwave and multicomponent detection, and three-dimensional p-wave fracture detection (Pu and Qing, 2008). The reservoir prediction in basement buried hills is limited by the heterogeneity of the reservoir and the quality of the seismic data, and the use of single-attribute prediction techniques will increase the multisolution nature of the reservoir prediction. Therefore, in this paper, multiple geophysical methods are first used to predict the spatial distribution of fractured and fractured-cave reservoirs in basement buried hills, and then they are combined to evaluate the granitic buried-hill reservoirs. This method achieves good results in predicting the granitic buried-hill reservoirs in the BC block of the Bongor Basin in southwestern Chad.
2 Geological Setting
The Bongor Basin in southwestern Chad is an important hydrocarbon-bearing basin (Figure 1A). Tectonically, it is in the northern part of the middle Central African shear zone and is a Mesozoic-Cenozoic intracontinental rift basin developed under the influence of the Central African shear zone (Genik, 1992; Li et al., 2017; Chen et al., 2018; Dou et al., 2018).
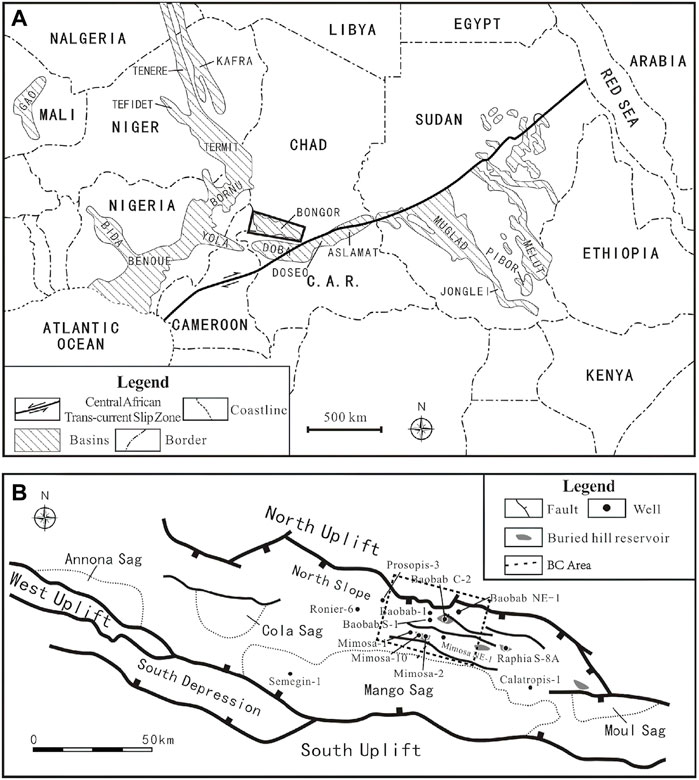
FIGURE 1. Location map of the study area and study area with main faults. (A) Location of the Bongor Basin and Central African Trans-Current Slip zone and adjacent basins, such as the Termit, Muglad, and Melut basins. (B) The main faults, tectonic division, and location of the BC block in the Bongor Basin and the discovered buried-hill hydrocarbon reservoirs with wells (Modified from Chen et al., 2018).
The BC block is in the northeastern part of the Northern Slope belt of the Bongor Basin (Salah and Alsharhan, 1998) (Figure 1B). It developed at the bottom of the basin during the Precambrian period and shows the present structural-depositional morphology after long-term weathering denudation, subsidence, and sedimentary filling. Precambrian basement rock, Lower Cretaceous (P, M, K, R, and B formations) and Cenozoic strata are developed sequentially from bottom to top in the basin, while the Upper Cretaceous strata are absent after denudation (Chen et al., 2018) (Figure 2). According to the hydrocarbon exploration and investigation, three hydrocarbon plays are considered to have developed in the Bongor Basin, namely, the upper (R-K), lower (M-P) and basement rock plays (Figure 2). The target of this study is the basement rock play (Genik, 1992; Dou et al., 2014; Dou et al., 2018).
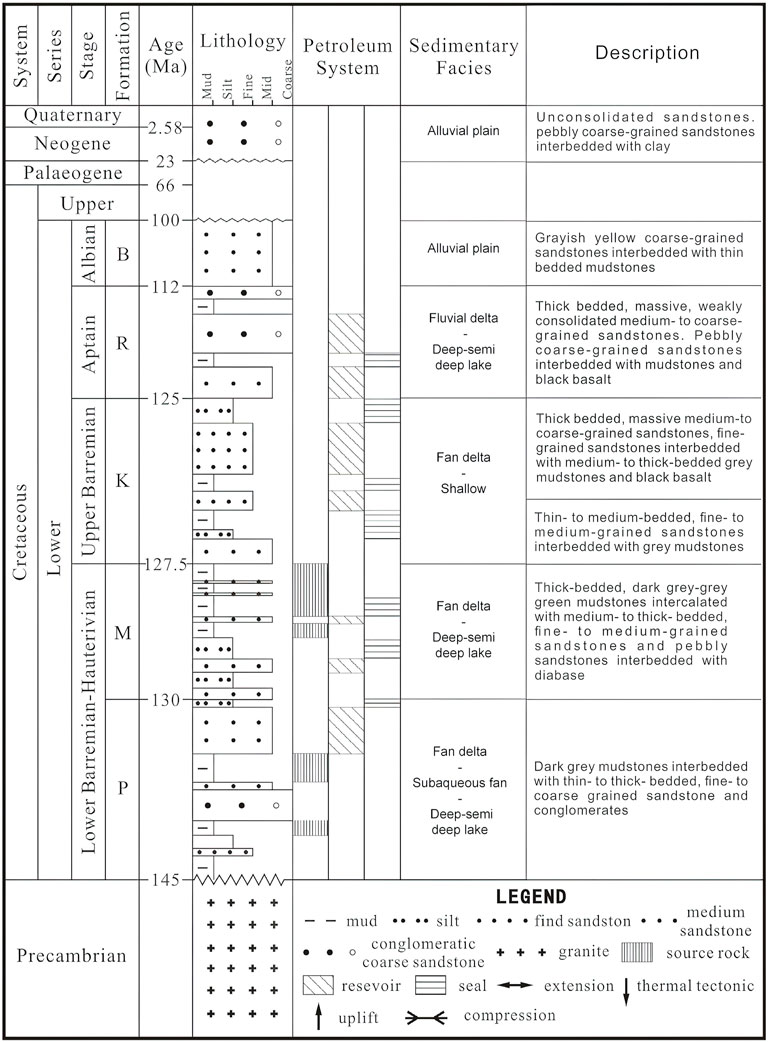
FIGURE 2. Comprehensive stratigraphic histogram of the Bongor Basin (Modified from Chen et al., 2018).
According to core observations, the lithology of the basement rock is mainly granite or mixed granite, and this rock features a brittle texture and is prone to develop structural fractures, joint fractures, and dissolution pores along these fractures. The crystalline basement of the Bongor Basin is mainly composed of metamorphic rocks with a high degree of metamorphism and magmatic rocks formed by remelting. Intercrystalline pores are difficult to retain, and the size of intercrystalline pores is small. In addition, due to the extent of drilling and coring, only a small amount of reservoir space of physical origin can be found in the cores of weathering crusts (Chen et al., 2018; Wang et al., 2018).
The FMI data show extensive development of horizontal to low-angle fractures in the basement rock (Figures 3A,B). The larger the fracture angle is, the more intense the dissolution is (Wang et al., 2018). The rocks at the intersection of faults are heavily fractured and easily form collapse caves (Dou et al., 2014; Dou et al., 2015; Li et al., 2017; Dou et al., 2020). Multistage and multiangle fractures and dissolution are superimposed on each other to form buried-hill fractured and fractured-cave reservoirs, which are often characterized by strong heterogeneity and limited distribution (Chen et al., 2018).
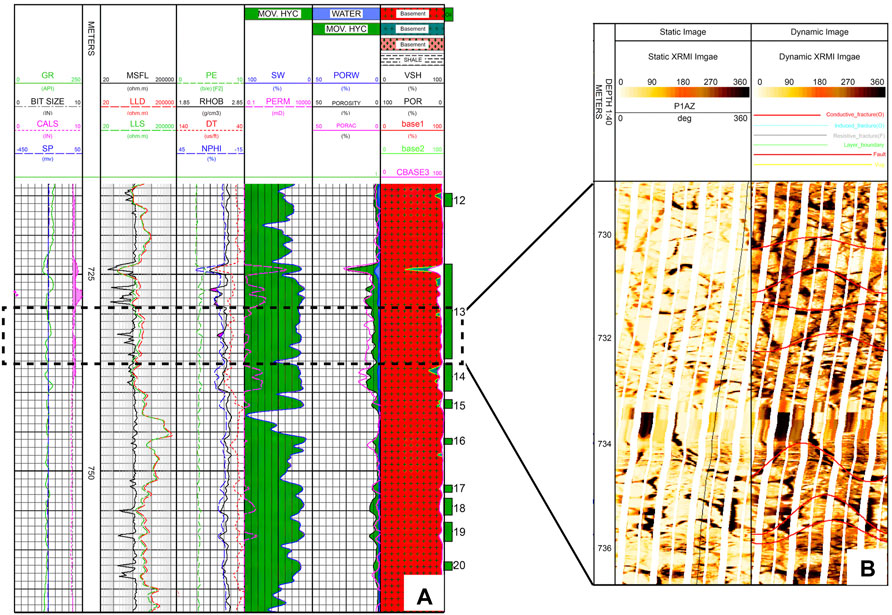
FIGURE 3. (A) Well log interpretation of well Baobab C-2. (B) Image log of well Babab C-2 (729–737 m).
The most developed reservoir spaces in the basement rock are mainly structural fractures, intergranular pores and dissolution pores formed by chemical dissolution (Dou et al., 2014). With the increase in depth below the buried hill surface, the type of basement buried-hill reservoir gradually transitions from a fractured-cave reservoir to a fractured reservoir. Through the calibration of the core and well seismic data, the oil-producing section of the well is mainly concentrated in the upper part of the buried hills (Wang et al., 2018; Dou et al., 2020). Therefore, the predicted types of pore space in the buried-hill reservoirs in the BC block are mainly fractures and dissolution pores.
At present, more than 30 wells exploring the basement rock of the BC block have obtained good oil and gas shows. Through years of research and drilling, it has been verified that basement buried-hill reservoirs in the BC block are typical fractured reservoirs with strong heterogeneity, large differences in oil-bearing layer thickness, and complex gas–water distributions (Chen et al., 2018). The accumulation degree of oil and gas is mainly controlled by the spatial distribution of reservoirs such as fractures and cavities. Therefore, the accurate prediction of the distributions of fractures and caves is key to the prediction of buried-hill reservoirs.
3 Materials and Methods
3.1 Structure-Oriented Filtering Technology
A structure-oriented filter is a diffusion filtering process for seismic data anisotropy under the control of dip and azimuth bodies. The seismic data after structure-oriented filtering have the functions of directivity and edge detection directivity. It smooths the information parallel to the seismic in-phase axis and does not deal with the seismic information perpendicular to the fault or the main tectonic direction. This technology was first applied in the field of image processing and analysis (Höcker and Fehmers, 2002; Saleh et al., 2002; Fehmers and Höcker, 2003; Wang et al., 2021; Luo et al., 2022). During the early stage, linear operators were used to filter noisy images. However, although this method was good at reducing noise, edge information of images would be damaged. Later, a nonlinear operator defined by a nonlinear partial differential equation was developed to convolve the original image with a series of Gaussian kernel functions with different noise scales to obtain smooth images of the original image at different scales, which can effectively protect the structural information of the image (Höcker and Fehmers, 2002; Fehmers and Höcker, 2003; Zhou and Li, 2018; Luo et al., 2022). Structure-oriented filters have the advantages of reducing invalid signals, increasing effective signals, and highlighting small and microscale faults, which can effectively reduce the noise of seismic profiles, improve the quality of seismic data, improve the signal-to-noise ratio, improve the accuracy of small and microscale fault identification, and guide oil and gas exploration (Höcker and Fehmers, 2002; Chen, 2015; Zhou and Li, 2018; Luo et al., 2022).
3.2 Fractured Reservoir Prediction Technology
The BC buried-hill fractured reservoir prediction technique is based on poststack migration seismic data. First, spectrum analysis is used to determine the reasonable calculation parameters to fabricate coherence. Then, linear enhancement of the AFE module in Paradigm software is used to enhance the resolution of microfault recognition by coherence. Finally, the coherent slices of a certain time window are extracted from the linear enhanced coherence to predict fractured reservoirs based on seismic geometry attributes.
3.2.1 Coherence Attributes Technique
Coherence attributes reflect the similarity of adjacent seismic trace waveforms in the time window centered on the target point and can be used to obtain the discontinuous variation characteristics of the stratum through the similarity between waveforms (Bahorich and Farmer, 1995; Marfurt et al., 1999; Wang et al., 2016; Gao et al., 2018). The coherence data reflect the difference between adjacent seismic tracks. Mathematically, the difference value is mainly expressed by variance. The worse the continuity of the strata is, the greater the variance value. Coherence highlights discontinuities by emphasizing unrelated anomalies (Zhang et al., 2002; Wang et al., 2012; Gong et al., 2013; Wang et al., 2015). When the continuity of a stratum is broken, such as by a fault, pinching, intrusion, deformation, etc. causing seismic waves to change, sudden changes are evident at the edges.
The data used are the coherence attributes extracted from the resulting data after structure-oriented filtering using the eigenvalue algorithm of coherence attribute technology of GeoEast software. The number of coherence channels is 5 × 5, and the coherent time window is selected as 25 ms after the test (approximately 2/3 wavelength, the main frequency of the data is approximately 30 Hz).
3.2.2 Coherent Linear Enhancement Technique
The main function of the AFE module in Paradigm software is automatic fault extraction. The intermediate part of this module, linear reinforcement processing, supports the search for microcracks and can help predict the planar distribution of cracks. The discontinuity of seismic reflection events mainly contains two kinds of information: noise irrelevant to geological factors and fractures and faults. The main effect of linear reinforcement in the AFE module is to suppress noise and enhance and amplify the desired geological information. First, linear filtering is carried out; that is, according to the actual geological conditions, the noise distribution is correctly understood, and INLINE, XLINE, and BOTH are flexibly used in three ways to remove noise, save related geological information as much as possible, and improve the quality of the data. Then, through the line detection method and reasonable selection of the detection line length, linear strengthening is conducted to highlight the fracture information and solve the actual geological problems (Zhang et al., 2013; Shang et al., 2021).
The fabrication of coherence is the basis of the linear strengthening technique for crack detection. To obtain a higher-quality data volume sensitive to fractures, the coherence data volume should be further linearly enhanced. In the process of linear strengthening, noise suppression is mainly carried out along the direction of noise in the plane, and the direction perpendicular to the main measuring line is usually considered the main direction of noise. Its purpose is mainly to filter the noise that can be seen in the coherence data. The effect of noise filtering can be achieved by selecting the length of the noise filtering factor. In the process of linear strengthening, fracture seismic reflection in the direction of the main survey line is retained, and the noise information of fractures in other directions is suppressed to enhance the fracture information in the study area.
3.3 Spectral Decomposition
Fractures inside a buried hill are the main cause for the formation of a cavity, and the response characteristics of seismic data with different acquisition directions vary. The collection direction vertical to the cavity is most sensitive to the response characteristics of the cavity (Lu et al., 2007; Liu and Ning, 2009; Su et al., 2020). The technique of spectral decomposition transforms the seismic signal from the time domain to the frequency domain by mathematical transformation. The reservoir is characterized in the frequency domain to avoid the mutual interference of different frequencies in the time domain (Sinha et al., 2005; Zhang et al., 2017; Li et al., 2019; Wu et al., 2020). Therefore, the seismic response characteristics of the same cavity to seismic waves with different frequencies are also different. The low-frequency amplitude energy of the reservoir increases when oil and gas are present (Li et al., 2019; Luo et al., 2020). In other words, the energy of the low-frequency component of seismic waves passing through oil–gas reservoirs is stronger than that of seismic waves passing through nonreservoirs (Liu, 2013; Tian et al., 2016; Zheng et al., 2019). It is possible to predict the distribution of fractured-cave reservoirs by using weak seismic spectral variation (Wang et al., 2004; Liu, 2013). Based on the above understanding, it can be considered that seismic waves in the low-frequency segment have a clearer representation of fractures and caves. To clarify the distribution rules of buried hill cavities, the following research ideas are designed: the short-time Fourier transform (STFT) is applied to seismic data to obtain a single frequency attribute body of multiple frequencies, combined with along-layer slicing technology, to identify the cavity plane distribution of various time windows (theoretically, the frequency division information can reflect the aperture of the 1/4 wavelength specification) (Raeesi et al., 2012; Tian et al., 2016; Li et al., 2019; Yang et al., 2021).
3.4 Attribute Fusion Technology
The extraction of seismic attributes is mainly based on mathematical transformation, and the geological significance of a single attribute may be relatively fuzzy, which requires geological researchers to determine the attributes comprehensively according to experience and various data (Zhou et al., 2021). However, the mixed attribute generated after seismic attribute fusion has no specific geological meaning. The purpose is to enable different attributes to be displayed simultaneously in the same palette mode, which is convenient for researchers to conduct comprehensive analysis and consideration of multiple attributes.
The GeoEast system attribute fusion technology is, according to a certain proportion relationship, to fuse attribute A and attribute B to generate a new attribute C. An appropriate dividing line is selected within the value range of attribute C, and the value range to be displayed by data A and that by data B are placed on either side of the boundary point of data C (Meng et al., 2018) (Figure 4).
Amax, Amin: the maximum and minimum values of seismic attribute data A; Data between (A1, A2) in data A are selected for fusion; Amin ≤ A1 ≤ A2 ≤ Amax.
Bmax, Bmin: the maximum and minimum values of seismic attribute data B; Data between (B1, B2) in data B are selected for fusion; Bmin ≤ B1 ≤ B2 ≤ Bmax.
The maximum and minimum of data C after fusion are Cmax and Cmin.
In the program, the maximum and minimum of data C are designed to be equal to the maximum and minimum of attribute data A, that is, Cmax = Amax, Cmin = Amin.
Amax and Amin: maximum and minimum values of seismic attribute data A, respectively. Data between (A1, A2) in data A are selected for fusion, and Amin ≤ A1 ≤ A2 ≤ Amax.
Bmax and Bmin: the maximum and minimum values of seismic attribute data B; data between (B1, B2) in data B are selected for fusion, and Bmin ≤ B1 ≤ B2 ≤ Bmax.
The maximum and minimum of data C after fusion are Cmax and Cmin. In the program, the maximum and minimum of data C are designed to be equal to the maximum and minimum of attribute data A; that is, Cmax = Amax, Cmin = Amin.
To scale the attribute data A and B into data C, an appropriate value between the maximum and minimum of data C is selected as the cut-off point Cx, and Cmin < Cx < Cmax (or Amin < Cx < Amax). Then, the selected part of data A (A1, A2) is fused into the (Cmin, Cx) segment of data C, and the selected part of data B (B1, B2) is fused into the (Cx, Cmax) segment of data C. The specific calculation formula is as follows (Zhang et al., 2015; Meng et al., 2018) (Formulas 1, 2):
Cmin < Cx < Cmax, Amin ≤ A1 ≤ A2 ≤ Amax, Bmin ≤ B1 ≤ B2 ≤ Bmax.
4 Results and Discussion
4.1 Seismic Data Preprocessing
In the BC block, there is a large difference in wave impedance between the granitic buried hill and the overlying clastic rocks in the P formation, which has a certain shielding effect on the seismic reflection characteristics of the underlying basement rock. As a result, the reflection signal is lost, and the resolution gradually decreases as the depth inside the buried hill increases. Seismic interpretation requires enhancement of the seismic reflections within the buried hill to maximize the accuracy of fracture prediction. Currently, the structure-oriented filtering technique is widely used for the preprocessing of seismic data because of its simplicity and effectiveness.
The signal-to-noise ratio of seismic data can be improved by structure-oriented filtering, thus improving the quality of the seismic data. Although the raw seismic data can reflect fault and fracture information to some extent, the resolution of the breakpoints is low (Figure 5A). Parameters suitable for the area were selected for structure-oriented filtering (Figure 5B). After filtering, the seismic profiles and breakpoints are clear. In addition, the continuity of seismic reflection events was improved, which provides a basis for subsequent prediction studies of buried-hill reservoirs.
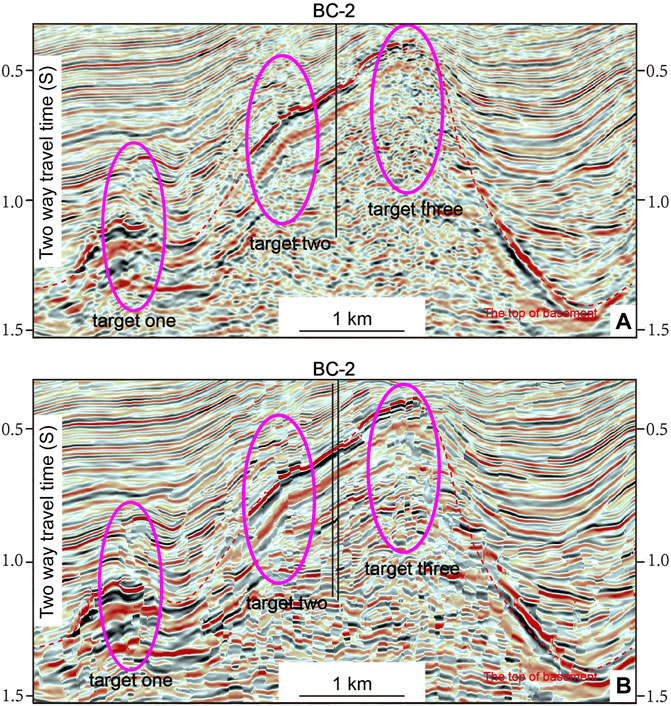
FIGURE 5. Seismic profile before and after structure-oriented filtering. (A) Original seismic profile; (B) Seismic profile after structure-oriented filtering.
4.2 Coherent Linear Enhancement
Figure 6A is the coherence attribute map along the top of the buried hill in the test area of the BC block, in which dark shadows represent low coherence, and bright areas represent high coherence. Because of the poor continuity of the reflected wave at the fault, it shows dark lines or curved features. Superposed with the fault system, the faults in the buried hill are found to have strikes of NW–SE and nearly E–W. This attribute is good for identifying large fractures but has a weak sensitivity response to fractures. Therefore, further linear strengthening technology is needed to improve the prediction accuracy of fractures.
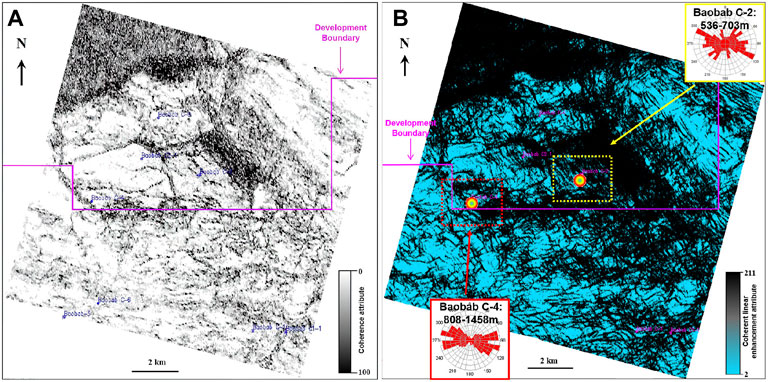
FIGURE 6. Coherence attribute properties and coherent linear enhancement properties. (A) Coherence attribute properties on the top of the granitic buried hill in the BC2 well area. (B) Coherent linear enhancement properties of cracks on the top of granitic buried hill in the BC2 well area.
Figure 6B shows the prediction plan of fracture development after coherent linear enhancement within 100 m below the upper surface of the buried hill in the test area of the BC block. In the figure, black areas represent areas with well-developed fractures, and blue areas represent areas with poorly developed fractures. The results of fracture prediction are verified by the high oil and gas production of well BC-4 and well BC-2 in the buried hill section. The results show that the degree of coincidence is high, which suggests the feasibility of this fracture prediction method, which can effectively predict the planar distribution of fractured reservoirs.
4.3 Fractured-Cave Reservoir Prediction
Combined with accurate reservoir calibration, the development location and seismic reflection characteristics of the cavity were determined. The GeoEast time-frequency analysis module was used to obtain the highest coincidence between the 5 Hz single frequency attribute body and the actual drilling core calibration, which can accurately reflect the plane distribution of the fractured-cave reservoir in the buried hill (Figures 7A–C).
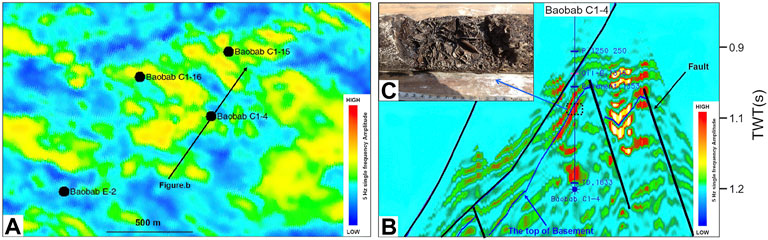
FIGURE 7. (A) Plan view of the single-frequency volume in the area of well Baobab C1-4 (5 Hz). (B) Single-frequency profile of well Baobab C1-4. (C) Image of the core of well Baobab C1-4 (1,419.72 m–1420 m).
Figure 7B shows the core photos of Well Baobab C1-4 and the 5 Hz frequency volume profile of well Baobab C1-4. Red and yellow represent abnormal bodies such as cavities, and green represents conventional strata. In the drilling of Well Baobab C1-4, multistage caving and mud leakage occurred, and holes were found in the drilling core at 1,419.72 m, which was consistent with the characteristics of the frequency attribute body at the corresponding position, indicating the sensitivity of low-frequency hole detection. Therefore, 5 Hz single-frequency attribute body data can be used to predict the distribution of fractures or cavities.
4.4 Comprehensive Prediction of Buried-Hill Reservoirs
The planar prediction results (as shown in Figures 8A,B) of the buried hill fractures and fractured caves in the BC block described above are used for attribute fusion. Figure 9A is a comprehensive evaluation diagram of buried-hill reservoirs in the BC block after splicing and fusion of the two types of reservoirs at depths of 0–100 m below the buried hill surface. The blue values indicate the development area of fractured reservoirs, which are mainly concentrated in the higher area in the northeast. The distribution characteristics are influenced by the NW–SE strike. Because the northeastern part of the section is strongly affected by tectonic stress, the buried hill is steep and narrow, and a series of large first-order faults are developed in the NW–SE direction. The strata adjacent to the large faults are seriously broken, and a fracture zone is developed. The buried hill landform in the southwestern section is relatively broad and has been weakly affected by tectonic stress. It is located at the tail end of the large fault, the strata are relatively intact, and the fracture development intensity is relatively weak. The fracture system around the long-term active fault associated with multistage tectonic movement is dense and complex and represents the final result of the crossing and superposition of multistage fractures (Sun et al., 2013). The red and yellow values indicate the development zones of fractured-cave reservoirs. Figure 8B shows that the basement rock pores in this area are mainly developed in the southwest of the section (West of the black dotted line in Figure 8B), are weakly developed in the East and are least developed in the North. The development degree of basement rock pores weakens from SW to NE.
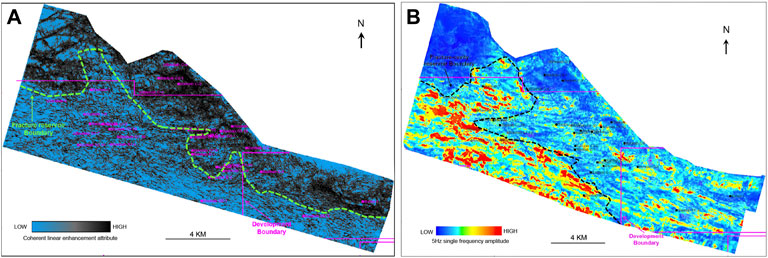
FIGURE 8. (A) Prediction map of the fractured granitic buried-hill reservoirs in the BC block (100 m below buried-hill surface). (B) Prediction map of the fractured-cave granitic buried-hill reservoirs in the BC block (0–100 m below the buried-hill surface).
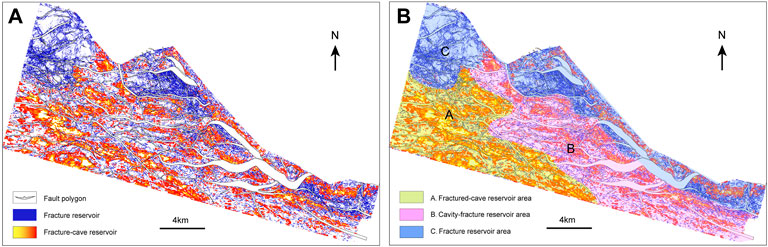
FIGURE 9. (A) Overlap map of the buried-hill reservoirs in the BC block (0–100 m below the buried hill surface). (B) Planar distribution of the three types of buried-hill reservoirs in the BC block (0–100 m below the buried hill surface).
Comprehensive analysis shows that the granitic rocks mainly host fractured reservoirs with little difference in development intensity. During the formation of the central African shear zone, the uplift height in the northeast of the section was greater than that in the southwest due to tectonic stress (Cuong and Warren, 2009). As the large fault developed, the formation fragmentation was more severe in the northeast than in the southwest. In the southwestern part of the section, the stress intensity has remained low, and the strata are relatively intact. Later developed faults or fractures intersect with early fractures, which is conducive to local formation dissolution and fractured-cave formation. The reservoir connectivity of the whole area gradually increases from southwest to northeast.
According to the above observations, the types of buried-hill reservoirs in the BC block are divided according to the relative development degree of fractures and caves. From southwest to northeast, the reservoirs gradually transition from the fractured-cave type to the fracture type. With gradually decreasing porosity, reservoirs are divided as follows: A) fractured-cave reservoir, B) cavity-fracture reservoir, and C) fracture reservoir. Finally, based on the comprehensive consideration of porosity and connectivity, it is considered that the B type (cavity-fracture reservoir) is the preferred high-quality reservoir (Figure 9B).
4.5 Application Effect Evaluation
Reservoir production dynamic data can effectively reflect the production characteristics of a large section of the play zone, which can further verify the reservoir prediction results (Guo et al., 2019). Wells BC1-10 and BC1-13 are located in the high part of the buried hill, and the fracture and frequency data are displayed in the fusion profile and on the horizontal plane (Figures 10A,B). Black represents the fractured reservoir response, and yellow–green represents the fractured-cave reservoir response. Fractures are well developed in both wells. However, the amplitude energy of the 5 Hz single-frequency attribute body in BC1-13 is stronger, suggesting that the fractured-cave reservoir is more developed, with better reservoir connectivity and better reservoir evaluation. The logging interpretation of the BC1-13 well buried-hill oil layer is 142.69 m, and that of the BC1-10 well buried-hill oil layer is 16 m. Based on the dynamic production curve statistics of the past year (Figure 10C), well BC1-10 averaged 90 m3 of oil per day, while well BC1-13, which was predicted to have a more developed buried-hill formation, averaged 180 m3/day, in line with the predrilling forecast.
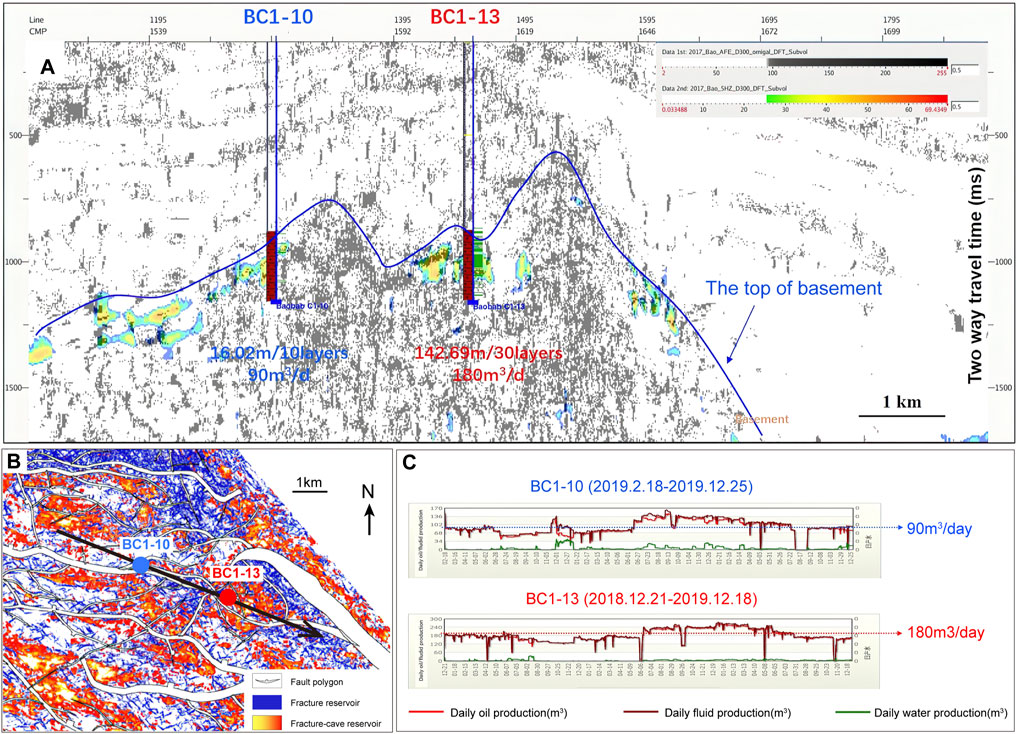
FIGURE 10. (A) Fusion profile of fracture data and fractured-cave data through wells BC1-10 and BC1-13 (coherent linear enhancement attribute and 5 Hz frequency volume). (B) Reservoir overlap diagram of buried hills in the BC block. (C) Dynamic production curves of wells BC1-10 and BC1-13.
The three types of reservoir areas are compared with the previous prediction results (Figure 11), combined with dynamic data from all producing wells in the field. The average daily oil production per well in the buried-hill type B reservoir is 253 m3/day; the average daily oil production per well in the buried-hill type C reservoir area is 161 m3/day; the average daily oil production per well in the buried-hill type A reservoir area is 66 m3/day; and the average daily production of oil and gas per well decreases successively. This is consistent with the comprehensive prediction results of basement buried-hill reservoirs in the BC block area, which confirms the effectiveness of this method. Buried-hill oil and gas accumulations are controlled by structural location and reservoir type. In a buried-hill fault block with a high structure, the type of buried-hill reservoir is the main controlling factor affecting productivity change. The cavity-fracture reservoirs are characterized by the highest oil production.
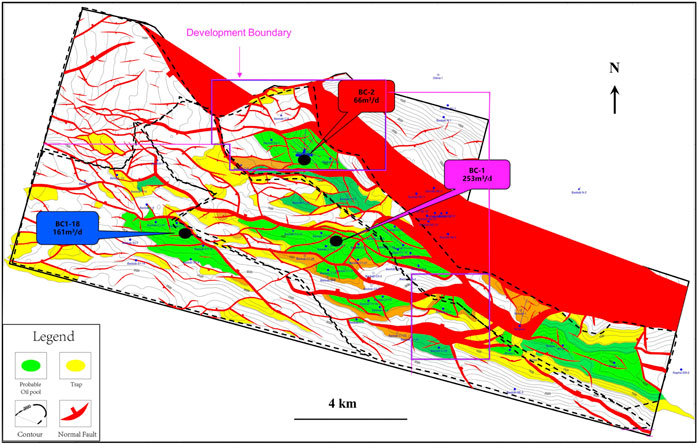
FIGURE 11. Structural diagram of the top of the buried hill in the BC block (green indicates oil-bearing areas and yellow indicates traps).
5 Conclusion
(1) A set of reservoir prediction methods suitable for granitic buried-hill reservoirs is summarized. These methods have been successfully applied to predict the most favourable development zones in the BC block. According to the different types of basement buried-hill reservoirs, different methods can be used to predict the spatial distribution of fracture reservoirs and fractured-cave reservoirs in complicated and heterogeneous basement buried-hill reservoirs.
(2) The basement buried-hill reservoirs in the BC block are dominated by fractured reservoirs, with locally developed fractured-cave reservoirs. The fractured-cave reservoirs formed from fractured reservoirs and are mainly associated with tectonic faults in the southwestern part. The tectonic faults in the northeastern part are close to the tectonic stress release zone. The formation is heavily fractured. The reservoirs are mainly fractured. The overall connectivity is better in the northeast than in the southwest.
(3) A comprehensive study shows that the basement buried-hill reservoirs in the BC block can be divided into three types: A) fractured-cave reservoirs; B) cavity-fracture reservoirs; and C) fracture-type reservoirs. From the southwest to the northeast, the reservoirs gradually transition from the fractured-cave type to the fracture type, with a gradual decrease in porosity and a gradual increase in connectivity. Based on the comprehensive evaluation of porosity and connectivity, it is concluded that the B-type cavity-fracture reservoir is the best reservoir type. Actual drilling data are consistent with the predictions.
(4) The comprehensive reservoir prediction results show that the method proposed in this study can effectively predict the reservoir distribution in the depth range of 0–100 m from the top of the buried hill but still has some limitations (e.g., it cannot distinguish effective fractures from ineffective fractures).
(5) The reservoir potential of the Precambrian basement has been greatly underestimated. This favourable finding has potential implications for exploration in the future. The oil discovery in the fractured buried hills in the Precambrian basement of the Bongor Basin will aid the hydrocarbon exploration of new plays in the central African rift system. It is also a milestone in the onshore exploration of the central African rift system.
Data Availability Statement
The datasets presented in this article are not readily available because 1. The original data involves trade secrets and shall not be disclosed without authorization. 2. The original data sets take up too much disk space to be easily transferred over the Internet. Requests to access the datasets should be directed to d2FuZ3lhamllMDFAY25wYy5jb20uY24=.
Author Contributions
YW: Writing Original Draft, Formal analysis; Methodology, GX: Conceptualization; Reviewing and Editing. WZ: Verified the analytical methods. JL, FX, and SH: Technology support: provided critical feedback.
Conflict of Interest
The authors declare that the research was conducted in the absence of any commercial or financial relationships that could be construed as a potential conflict of interest.
Publisher’s Note
All claims expressed in this article are solely those of the authors and do not necessarily represent those of their affiliated organizations, or those of the publisher, the editors and the reviewers. Any product that may be evaluated in this article, or claim that may be made by its manufacturer, is not guaranteed or endorsed by the publisher.
Acknowledgments
We thank the editors for their constructive recommendations that have improved this manuscript. We also thank the CNPCIC for providing the data and allowing the publication of this article. Zhao Lin-Hai of the College of Energy, Chengdu University of Technology and senior engineer Hou Yun-Peng of the Geophysical Research Institute, Bureau of Geophysical Prospecting of Petro China have been of great assistance during the data analysis.
References
Bahorich, M., and Farmer, S. (1995). 3-D Seismic Discontinuity for Faults and Stratigraphic Features: The Coherence Cube. Lead. edge 14, 1053–1058. doi:10.1190/1.1437077
Chen, C. (2015). Key Technique and Application of Structure Oriented Filter for Seismic Wave Field. Doctor’s thesis. Changchun (China): Jilin University.
Chen, L., Ji, H., Dou, L., Du, Y., Xu, Z., Zhang, L., et al. (2018). The Characteristics of Source Rock and Hydrocarbon Charging Time of Precambrian Granite Reservoirs in the Bongor Basin, Chad. Mar. Petroleum Geol. 97, 323–338. doi:10.1016/j.marpetgeo.2018.06.003
Cuong, T. X., and Warren, J. K. (2009). BACH HOFIELD, A FRACTURED GRANITIC BASEMENT RESERVOIR, CUU LONG BASIN, OFFSHORE SE VIETNAM: A "BURIED-HILL" PLAY. J. Petroleum Geol. 32, 129–156. doi:10.1111/j.1747-5457.2009.00440.x
Dai, J., Ni, Y., Dong, D., Qin, S., Zhu, G., Huang, S., et al. (2021). 2021-2025 Is a Period of Great Development of China's Natural Gas Industry: Suggestions on the Exploration and Development of Natural Gas during the 14th Five-Year Plan in China. J. Nat. Gas Geoscience 6, 183–197. doi:10.1016/j.jnggs.2021.08.001
Dou, L., Li, W., and Cheng, D. (2020). Hydrocarbon Accumulation Period and Process in Baobab Area of Bongor Basin. J. Afr. Earth Sci. 161, 103673. doi:10.1016/j.jafrearsci.2019.103673
Dou, L., Shrivastava, C., Dai, C. S., Wang, J., Hammond, N., Anoliefo, C., et al. (2014). “Better Exploitation of Granitic Reservoirs: Understanding the Role of Stress Regime and Fractures,” in Unconventional Resources Technology Conference, Denver, CO. doi:10.1306/02061817090
Dou, L., Wang, J., Wang, R., Wei, X., and Shrivastava, C. (2018). Precambrian Basement Reservoirs: Case Study from the Northern Bongor Basin, the Republic of Chad. Bulletin 102, 1803–1824. doi:10.1306/02061817090
Dou, L., Wei, X., Wang, J., Li, J., Wang, R., and Zhang, S. (2015). Characteristics of Granitic Basement Rock Buried-Hill Reservoir in Bongor Basin, Chad. Acta Pet. Sin. 36, 897–904+925.
Fehmers, G. C., and Höcker, C. F. W. (2003). Fast Structural Interpretation with Structure‐oriented Filtering. Geophysics 68, 1286–1293. doi:10.1190/1.1598121
Gao, M., Cui, G., Liu, B., Han, J., Tian, Y., and Xu, L. (2018). Fault Block Trap Evaluation in Maozhou Oilfield. Oil Geophys. Prospect. 53, 170–178+113114. doi:10.13810/j.cnki.issn.1000-7210.2018.S1.028
Genik, G. J. (1992). Regional Framework, Structural and Petroleum Aspects of Rift Basins in Niger, Chad and the Central African Republic (C.A.R.). Tectonophysics 213, 169–185. doi:10.1016/0040-1951(92)90257-7
Gong, D., Xu, G., Xu, S., Wang, X., Zheng, L., Fu, H., et al. (2013). Seismic Geomorphology Analysis and its Application in Llanos Basin, Columbia. Prog. Geophys. 28, 2569–2578.
Guo, K., Fan, L., Li, Y., Zhang, M., Zhang, C., and Li, L. (2019). Comprehensive Prediction of Fracture of Tight Carbonate Reservoir in the H Block of Amu Darya Right Bank. Geophys. Prospect. Petroleum 58, 112–122+138.
Höcker, C., and Fehmers, G. (2002). Fast Structural Interpretation with Structure-Oriented Filtering. Lead. edge 21, 238–243. doi:10.1190/1.1463775
Li, H., Lin, C., Ren, L., Zhang, G., Chang, L., and Dong, C. (2021a). Quantitative Prediction of Multi-Period Tectonic Fractures Based on Integrated Geological-Geophysical and Geomechanics Data in Deep Carbonate Reservoirs of Halahatang Oilfield in Northern Tarim Basin. Mar. Petroleum Geol. 134, 105377. doi:10.1016/j.marpetgeo.2021.105377
Li, H., Niu, C., Xu, P., Liu, Q., Zhang, X., and Cui, H. (2021b). Discovery of Bozhong 13-2 Archean Large Monoblock Volatile Buried Hill Oilfield and its Oil and Gas Exploration Significance. Nat. Gas. Ind. B 8, 376–383. doi:10.1016/j.ngib.2021.07.008
Li, W., Dou, L., Wen, Z., Zhang, G., and Cheng, D. (2017). Use of a Geochemical Method to Analyze the Hydrocarbon Accumulation Process in the Bongor Basin, Chad. Petroleum Sci. Technol. 35, 2133–2138. doi:10.1080/10916466.2017.1386678
Li, W., Yue, D., Wang, W., Wang, W., Wu, S., Li, J., et al. (2019). Fusing Multiple Frequency-Decomposed Seismic Attributes with Machine Learning for Thickness Prediction and Sedimentary Facies Interpretation in Fluvial Reservoirs. J. Petroleum Sci. Eng. 177, 1087–1102. doi:10.1016/j.petrol.2019.03.017
Liu, A. (2013). Comprehensive Evaluation and Fluid Identification of Fracture-Cavity Carbonate Reservoir. Doctor’s thesis. Chengdu (China): Chengdu University of Technology.
Liu, X., and Ning, J. (2009). A Review of Time Frequency Attributes and Their Applications in Seismic Data Interpretation for Oil & Gas Geology. Prog. Explor. Geophys. 32 (1), 18–22+83. (in Chinese with English abstract).
Lu, P., Yang, C., and Guo, A. (2007). The Present Research on Frequency-Spectrum Imaging Technique. Prog. Geophys. 22 (5), 1517–1521. (in Chinese with English abstract).
Luo, J., Wu, F., Zhang, D., Xu, M., Chen, H., Fan, J., et al. (2020). A Frequency-Divided Gas Prediction Technology Based on Resistivity Constraint. Nat. Gas. Ind. B 7, 127–132. doi:10.1016/j.ngib.2019.09.003
Luo, X., Chen, X., Zhang, J., Li, K., Lv, B., and Wen, H. (2022). A Target-Oriented Integrated Workflow for Seismic Delineation of Thin Tight Reservoirs in the Eastern Sichuan Basin, China. J. Petroleum Sci. Eng. 208, 109246. doi:10.1016/j.petrol.2021.109246
Maerten, L., Maerten, F., and Lejri, M. (2018). Along Fault Friction and Fluid Pressure Effects on the Spatial Distribution of Fault-Related Fractures. J. Struct. Geol. 108, 198–212. doi:10.1016/j.jsg.2017.10.008
Marfurt, K. J., Sudhaker, V., Gersztenkorn, A., Crawford, K. D., and Nissen, S. E. (1999). Coherency Calculations in the Presence of Structural Dip. Geophysics 64, 104–111. doi:10.1190/1.1444508
Meng, Y., Xu, Y., Li, J., Xu, T., Wang, H., and Wang, X. (2018). Fault Identification with OVT-Domain Seismic Attribute Analysis. Oil Geophys. Prospect. 53, 289–294+219. doi:10.13810/j.cnki.issn.1000-7210.2018.S2.045
Panza, E., Sessa, E., Agosta, F., and Giorgioni, M. (2018). Discrete Fracture Network Modelling of a Hydrocarbon-Bearing, Oblique-Slip Fault Zone: Inferences on Fault-Controlled Fluid Storage and Migration Properties of Carbonate Fault Damage Zones. Mar. Petroleum Geol. 89, 263–279. doi:10.1016/j.marpetgeo.2017.09.009
Pu, J., and Qing, Q. (2008). An Overview of Fracture Prediction Methods for Oil and Gas Reservoirs. Special Oil Gas Reservoirs 15 (3), 9–13+106. (in Chinese with English abstract).
Raeesi, M., Moradzadeh, A., Doulati Ardejani, F., and Rahimi, M. (2012). Classification and Identification of Hydrocarbon Reservoir Lithofacies and Their Heterogeneity Using Seismic Attributes, Logs Data and Artificial Neural Networks. J. Petroleum Sci. Eng. 82-83, 151–165. doi:10.1016/j.petrol.2012.01.012
Salah, M. G., and Alsharhan, A. S. (1998). The Precambrian Basement: A Major Reservoir in the Rifted Basin, Gulf of Suez. J. Petroleum Sci. Eng. 19, 201–222. doi:10.1016/S0920-4105(97)00024-7
Saleh, A. D., Kurt, J. M., and Luo, Y. (2002). 3‐D Edge Preserving Smoothing for Seismic Edge Detection. Seg. Tech. Program Expand. Abstr., 524–527. doi:10.1190/1.1817300
Shang, X., Long, S., and Duan, T. (2021). Fracture System in Shale Gas Reservoir: Prospect of Characterization and Modeling Techniques. J. Nat. Gas Geoscience 6, 157–172. doi:10.1016/j.jnggs.2021.06.001
Sinha, S., Routh, P. S., Anno, P. D., and Castagna, J. P. (2005). Spectral Decomposition of Seismic Data with Continuous-Wavelet Transform. GEOPHYSICS 70, P19–P25. doi:10.1190/1.2127113
Su, Z., Liu, Y., Han, J., Luo, H., Yang, F., Cui, Y., et al. (2020). Application of Ultra-deep Sandstone Reservoirs Prediction Technology under Controlled Seismic Facies in Yudong Block of Tabei Uplift, Tarim Basin, China. J. Nat. Gas Geoscience 5, 157–167. doi:10.1016/j.jnggs.2020.05.001
Sun, W., Li, Y., He, W., Hei, J., and Kong, K. (2013). Using P-Wave Azimuthal Anisotropy to Predict Fractures in Carbonate Reservoirs of the ZY Block. Geophys. Prospect. Petroleum 34 (1), 137–144. (in Chinese with English abstract).
Tian, F., Jin, Q., Lu, X., Lei, Y., Zhang, L., Zheng, S., et al. (2016). Multi-layered Ordovician Paleokarst Reservoir Detection and Spatial Delineation: A Case Study in the Tahe Oilfield, Tarim Basin, Western China. Mar. Petroleum Geol. 69, 53–73. doi:10.1016/j.marpetgeo.2015.10.015
Wang, J., Dou, L., Xu, J., Wei, X., Wang, Z., and Chen, H. (2018). Granitic Buried-Hill Reservoir Characterization Based on Broadband, Wide-Azimuth and High-Density Seismic Data:A Case Study of Bongor Basin in Chad. Oil Geophys. Prospect. 53, 320–329+222. doi:10.13810/j.cnki.issn.1000-7210.2018.02.013
Wang, S., Guan, L., and Zhu, H. (2004). Prediction of Fracture-Cavity System in Carbonate Reservoir: A Case Study in the Tahe Oilfield. Appl. Geophys. 1, 56–62. doi:10.1007/s11770-004-0032-y
Wang, S., Yuan, S., Yan, B., He, Y., and Sun, W. (2016). Directional Complex-Valued Coherence Attributes for Discontinuous Edge Detection. J. Appl. Geophys. 129, 1–7. doi:10.1016/j.jappgeo.2016.03.016
Wang, X., Gao, J., Chen, W., and Song, Y. (2012). An Efficient Implementation of Eigenstructure-Based Coherence Algorithm Using Recursion Strategies and the Power Method. J. Appl. Geophys. 82, 11–18. doi:10.1016/j.jappgeo.2012.03.009
Wang, X., Zhang, X., Zhao, X., Hu, F., Ren, C., Wang, G., et al. (2021). Key Technologies for Complex Surface Seismic Acquisition in the Sichuan Basin and Their Application Effect. Nat. Gas. Ind. B 8, 552–561. doi:10.1016/j.ngib.2021.11.003
Wang, Y., Lu, W., and Zhang, P. (2015). An Improved Coherence Algorithm with Robust Local Slope Estimation. J. Appl. Geophys. 114, 146–157. doi:10.1016/j.jappgeo.2015.01.015
Wu, D., Wu, Z., and Wu, Y. (2020). A New Method for High Resolution Well-Control Processing of Post-stack Seismic Data. Nat. Gas. Ind. B 7, 215–223. doi:10.1016/j.ngib.2019.11.003
Yan, L., Chang, Y., Tian, Z., Li, X., and Jin, J. (2019). Characteristics of Reservoir Spaces and Influencing Factors for Basement Rock of Bongor Basin in Chad. J. Northeast Petroleum Univ. 43 (2), 59–67+5859. doi:10.19509/j.cnki.dzkq.2019.0608
Yang, Y., Xie, J., Zhao, L., Huang, P., Zhang, X., Chen, C., et al. (2021). Breakthrough of Natural Gas Exploration in the Beach Facies Porous Dolomite Reservoir of Middle Permian Maokou Formation in the Sichuan Basin and its Implications: A Case Study of the Tridimensional Exploration of Well JT1 in the Central-Northern Sichuan Basin. Nat. Gas. Ind. B 8, 393–401. doi:10.1016/j.ngib.2021.07.010
Ye, T., Niu, C., and Wei, A. (2020). Characteristics and Genetic Mechanism of Large Granitic Buried-Hill Reservoir, a Case Study from PengLai Oil Field of Bohai Bay Basin, North China. J. Petroleum Sci. Eng. 189, 106988. doi:10.1016/j.petrol.2020.106988
Ye, Y. (2019). Comprehensive Prediction of Precise Reservoir Fracture and the Application Stduy on the Gas-Bearing Detection. Doctor’s thesis. Chengdu (China): Chengdu University of Technology.
Yi, S., Li, M., Xu, S., Guo, X., Cui, B., Meng, Q. A., et al. (2021). Accumulation Condition and Model of Buried Hill in the Central Uplift, Songliao Basin, China. J. Nat. Gas Geoscience 6, 121–135. doi:10.1016/j.jnggs.2021.06.002
Zhang, H., Ma, J., Jiang, Z., Wang, T., Zhao, Z., and Li, B. (2017). Application of Spectral Decomposition Technique in Reservoir Characterization and Fluid Identification. Complex Hydrocarb. reserv. 10, 31–33. doi:10.16181/j.cnki.fzyqc.2017.01.006
Zhang, J., Liu, Y., Luo, X., Zhao, L., Li, S., and Yin, N. (2015). Application of Complex Fracture Characterization Technique provided by GeoEast in Dawan Oil-Field. Petroleum Geol. Englineering 29 (5), 31–32+37. (in Chinese).
Zhang, J., Tian, S., Zheng, D., and Qu, X. (2013). Seismic Attribute Predication Method for Fractured Reservoirs. Jourmal Oil Gas Technol. 35 (3), 79–84+166. (in Chinese with English abstract).
Zhang, K., Lin, N., Tian, G., Yang, J., Wang, D., and Jin, Z. (2022). Unsupervised-learning Based Self-Organizing Neural Network Using Multi-Component Seismic Data: Application to Xujiahe Tight-Sand Gas Reservoir in China. J. Petroleum Sci. Eng. 209, 109964. doi:10.1016/j.petrol.2021.109964
Zhang, Y., Li, S., Huang, G., Zhang, F., and Chi, Y. (2002). The Classification and Application Value of Seismic Slice. Petroleum Geology and Recovery Efficiency 9 (3), 67–69+61. (in Chinese with English abstract).
Zheng, S., Yang, M., Kang, Z., Liu, Z., Long, X., Liu, K., et al. (2019). Controlling Factors of Remaining Oil Distribution after Water Flooding and Enhanced Oil Recovery Methods for Fracture-Cavity Carbonate Reservoirs in Tahe Oilfield. Petroleum Explor. Dev. 46, 786–795. doi:10.1016/s1876-3804(19)60236-3
Zhou, L., Qian, Y., Zhang, L., Lan, X., Wu, Y., Wang, Q., et al. (2021). Seismic Prediction of Oolitic Beach Thin-Bed Reservoir Based on Favorable Facies Belt Constraints: Taking the Second Member of Feixianguan Formation in Jiulongshan Area, Northwest Sichuan, China. J. Nat. Gas Geoscience 6, 329–344. doi:10.1016/j.jnggs.2021.12.002
Keywords: granitic buried-hills, fractured reservoir prediction, fracture-cavity reservoir prediction, attribute fusion, inverted rift basin, Bongor Basin
Citation: Wang Y, Xu G, Zhou W, Liang J, Xu F and He S (2022) Predicting Granitic Buried-Hill Reservoirs Using Seismic Reflection data—A Case Study From the Bongor Basin, Southwestern Chad. Front. Earth Sci. 10:949660. doi: 10.3389/feart.2022.949660
Received: 21 May 2022; Accepted: 21 June 2022;
Published: 12 July 2022.
Edited by:
Dongming Zhi, Turpan-Hami oil company, PetroChina, ChinaReviewed by:
Qi Li, China University of Geosciences, ChinaNan Jia, Liaoning Technical University, China
Copyright © 2022 Wang, Xu, Zhou, Liang, Xu and He. This is an open-access article distributed under the terms of the Creative Commons Attribution License (CC BY). The use, distribution or reproduction in other forums is permitted, provided the original author(s) and the copyright owner(s) are credited and that the original publication in this journal is cited, in accordance with accepted academic practice. No use, distribution or reproduction is permitted which does not comply with these terms.
*Correspondence: Yajie Wang, d2FuZ3lhamllMDFAY25wYy5jb20uY24=; Guosheng Xu, eGdzQGNkdXQuZWR1LmNu