- 1Broadvision Engineering Consultants Co., Ltd., Kunming, China
- 2Institute of International Rivers and Eco-Security, Yunnan University, Kunming, China
- 3Yunnan Key Laboratory of Digital Communications, Kunming, China
- 4School of Earth Sciences, Yunnan University, Kunming, China
- 5School of Infrastructure Engineering, Nanchang University, Nanchang, China
- 6School of Public Security and Emergency Management, Kunming University of Science and Technology, Kunming, China
Environmental variables are crucial factors affecting the development and distribution of landslides, and they also provide vitally important information for statistically-based landslide susceptibility mapping (SLSM). The acquisition and utilization of appropriate and the most influential environmental variables and their combinations are crucial for improving the quality of SLSM results. However, compared with the construction of SLSM models based on machine learning, the acquisition and utilization of high-quality environmental variables have received very little attention. In order to further clarify the research status of the application of environmental variables and possible development directions in future research, this study systematically analyzed the application of environmental variables in SLSM. To this end, a literature database was constructed by collecting 261 peer-reviewed articles (from 2002 to 2021) on SLSM from the Web of Science and CNKI platform (www.cnki.net) based on the keywords of “landslide susceptibility” and “environmental variable.” We found that existing methods for determining environmental variables do not consider the regional representativeness and geomorphological significance of the variables. We also found that at present, environmental variables are utilized generally without the realization and understanding of their spatial heterogeneity. Accordingly, this study raises two major scientific issues: 1) Effective identification of important environmental variables required in SLSM. 2) Effective representation of the spatial heterogeneity of environmental variables in SLSM modeling. From the perspective of the identification of dominant variables and their geospatial pattern of heterogeneity, targeted solutions for future research are also preliminarily discussed, including the method for identifying dominant variables from qualitative and quantitative perspectives and SLSM model construction considering the specific geospatial patterns. In addition, the applicability and limitation of the mentioned methods are discussed.
1 Introduction
Landslide susceptibility refers to the likelihood of a landslide occurring in a certain area under the influence of local environmental conditions (Brabb, 1984). Applying the ideology that the “past and present are the keys to the future” (Furlani and Ninfo, 2015), statistically-based landslide susceptibility mapping (SLSM) is one of the most widely used methods in landslide susceptibility assessment. This method focuses on building the functional relationship between landslide environmental variables and existing landslide inventories, and further realize the quantitative or qualitative assessment of the spatial possibility of landslide occurrence under a set of geological/geographical environmental conditions (Guzzetti et al., 2005; 2006a; 2006b; Reichenbach et al., 2018). SLSM can be used to effectively predict and identify locations prone to landslides on the regional scale (Alcántara-Ayala et al., 2022), and its results can provide indispensable information for various tasks, such as landslide risk assessment, formulation of disaster reduction measures, and land use planning (van Westen et al., 2008; Erener et al., 2016; Huang et al., 2019; Zhang et al., 2022). In recent years, it has gradually become a hot topic of geological hazard research on a global scale (Chen et al., 2020; Huang et al., 2021a).
Since the mid-1970s, research on SLSM has made significant progress. According to 250 slopes and topographic and geological conditions in the southwest of Germany, Neuland (1976) built a landslide prediction model using the statistical method of binary discriminant analysis, which is considered to be the earliest statistical study of landslide susceptibility assessment. Henceforth, scholars worldwide have published hundreds of papers considering different environmental conditions and landslide distribution in different regions, using various statistical methods. These statistical methods are also called data-driven landslide susceptibility models (Lima et al., 2022). By sorting and summarizing relevant literature, these methods can be generally divided into three categories (Table 1): mathematically-based, geography-based and, pattern recognition-based. The model construction under these three categories is based on geostatistical analysis, geospatial analysis, and machine learning, respectively, with each presenting its own advantages and disadvantages.
Researchers are constantly attempting to determine a functional relationship that is more representative of the relationship (assessment model) between environmental variables (independent variables) and landslide inventories (dependent variables) (Carrara, 1983; Guzzetti et al., 1999; Guzzetti et al., 2006b; Budimir et al., 2015; Zhang et al., 2020) from different perspectives. Particularly in recent years, artificial intelligence represented by machine learning (ML) has introduced new tools of function expression and data analysis for SLSM and their combination significantly promotes the development of model construction for landslide susceptibility analysis (Huang et al., 2017; Zhao et al., 2019; Sun et al., 2021; Lima et al., 2022). Gradually, the focus of SLSM research has shifted to the construction and analysis of susceptibility models. Research interest in this field appears to be increasingly shifting towards the testing of new ML models and the comparison of model performance, striving to develop a better and more suitable prediction model. However, through the analysis of the quality of SLSM by applying the susceptibility quality level (SQL) index (Guzzetti et al., 2006a; Guzzetti et al., 2006b), Reichenbach et al. (2018) reported that the number of high-quality research remains small.
Environmental variables are among the most important input information for SLSM models (van Westen et al., 2008). They represent environmental factors that affect the development of landslides and have important and complex effects on the spatial distribution of landslides (Yan et al., 2019). We sorted out the number of environmental variables used in the literature database, which was constructed using 261 peer-reviewed articles (from 2002 to 2021) collected from the Web of Science and CNKI platform (www.cnki.net) according to the following two keywords: “landslide susceptibility” and “environmental variable.” The number of such articles showed a significant growth trend (Figure 1) in the past 20 years. In other words, researchers have gradually realized that environmental variables are crucial for the construction of SLSM models (Ercanoglu and Gokceoglu, 2002; Jebur et al., 2014; Kavzoglu et al., 2015; Trigila et al., 2015; Pham et al., 2016; Nguyen et al., 2019). The purpose is to select as many environment variables as possible and include the ones that may be the most influential.
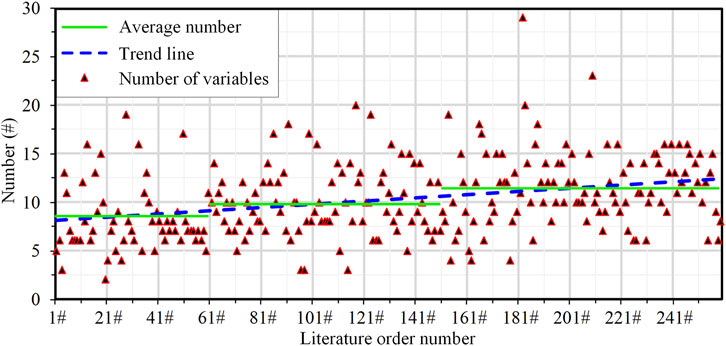
FIGURE 1. Number of environmental variables applied from the literature database. The order numbers are listed in chronological order of the literature.
However, the premise is that researchers require some prior knowledge of the main environmental factors affecting landslides in the study area (Crozier, 1986; Zhao et al., 2019), and the selected environmental variables should be closely related to the environmental characteristics of the study area (Pourghasemi et al., 2012; Lima et al., 2022). The analysis of the literature database reveals that this aspect has not received sufficient attention. Researchers appear to be more interested in new modeling techniques, but they pay little attention to selection and application of high-quality environmental variables required in landslide susceptibility modeling. Therefore, in this study, a systematic review based on the literature database was conducted to further clarify the current status of the application of environmental variables in SLSM. On this basis, possible future development ideas of improving the SLSM prediction quality from the perspective of environmental variables are preliminarily explored.
2 Acquisition status of environmental variables
The acquisition of environmental variables is a process of searching for inducing and contributing factors that have a certain correlation with the occurrence and distribution of landslides from different aspects such as the natural environment and human activities (Pradhan, 2013; Duan et al., 2022). The ultimate purpose is to ensure that the environmental variables used for SLSM have a logical and effective impact on the prediction of landslide susceptibility in the study area (Ayalew and Yamagishi, 2005; Guzzetti et al., 2012; Jaafari et al., 2019; Pourghasemi et al., 2020). In general, the approach of researchers selecting environmental variables can be summarized into two methods: qualitative selection and quantitative screening.
2.1 Qualitative selection
For a specific study area, the selection of environmental variables is necessary for every SLSM researcher. Through the analysis of the literature database, selection methods of environmental variables could be generally divided into four categories (Table 2): methods based on existing experience and cognition, direct reference from literature records, available existing data of the study area, and comprehensive consideration. At the same time, researchers also consider the accessibility and operability of data in the process of selection from the perspective of qualitative analysis (Lee, 2005). The four methods provide direct and practical guidance and are widely used.
Environmental factors affecting the occurrence and distribution of landslides in a region are complex and diverse (Soeters and van Westen, 1996; Hong et al., 2019), and different regions correspond to different variables (Guzzetti et al., 2006a). According to the analysis of the current status of the qualitative selection of environmental variables in the literature database, although qualitative selection provides a large number of environmental variables, several shortcomings still remain, such as the limited cognition ability of researchers regarding preliminary information on landslides in specific study areas, influence of commonly used environmental variables on the current reference and citation, and difficulties in data acquisition affecting variable selection. Consequently, environmental variables selected for susceptibility assessment may not all possess good expressive ability for the environmental characteristics of the specific study area. For example, for many types of landslides, especially loess or red bed landslides, groundwater variables have a critical impact on the development, movement, and distribution of landslides (Pei et al., 2018; Peng, 2018; Zhao et al., 2022), but the actual selection and application of groundwater variables in SLSM are rare. In some regions, especially dry and hot valleys, the arid climate conditions have a significant control over the spatial distribution of geological disasters such as landslides and debris flows (Chen et al., 2014; Sun, 2020; Liu et al., 2023). However, drought variables have not received sufficient attention in the current SLSM. Therefore, further in-depth consideration of landslide geological properties and environmental characteristics is required for specific regions in the acquisition of variables.
2.2 Quantitative screening
Some researchers (Lee et al., 2012; Wu et al., 2013; Samia et al., 2017; Lucchese et al., 2021) immediately constructed their susceptibility assessment model after completing the qualitative selection of environmental variables. In contrast, some researchers (He et al., 2019; Al-Najjar and Pradhan, 2021; Chen and Chen, 2021) carried out quantitative screening after completing preliminary selection. Through the analysis of the current status of variable screening in the literature database (Figure 2A), approximately 28% of the studies were found to have further carried out follow-up screening after qualitative selection. Since 2015, the screening of environmental variables has shown a distinct growth trend, and the number of studies with screening reached 39%. In other words, with the continuous deepening of SLSM research, an increasing number of researchers are acknowledging that the screening of environmental variables before constructing the models is conducive to ensuring better conditional independence and higher prediction ability of environmental variables involved in the assessment (Kavzoglu et al., 2015; Chen et al., 2018a; Amato et al., 2019; Hong et al., 2020; Saha et al., 2021).
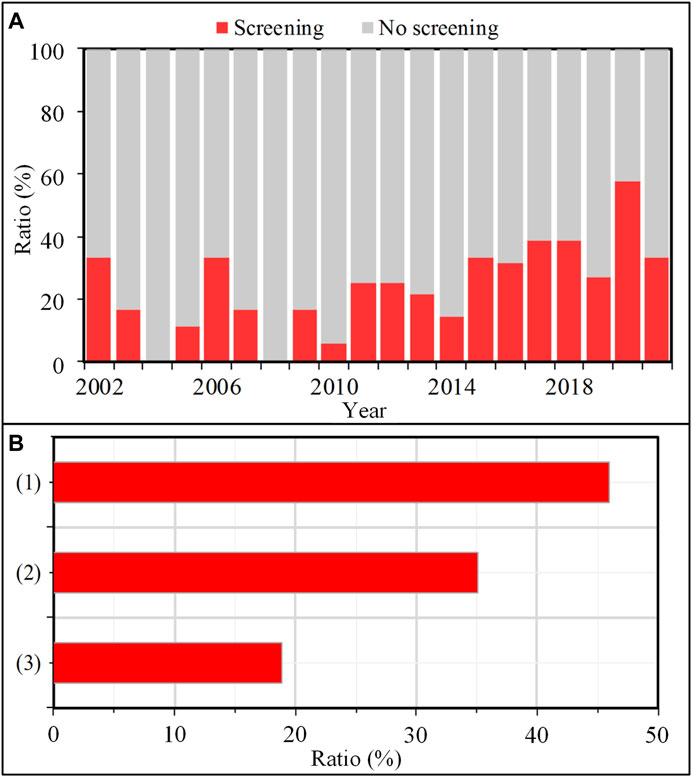
FIGURE 2. (A) Indicates the proportion of screening and non-screening. (B) Indicates the proportion of different screening methods.
At present, the screening of environmental variables is primarily based on 1) correlation analysis between variables and landslide distributions, 2) analysis of the conditional independence of variables, and 3) combination analysis (Figure 2B). The first approach accounts for the largest proportion (45.9%) of all screening approaches. In this approach, environmental variables with high correlation are screened by analyzing the relationship between the environmental variables and the landslide spatial distribution. The main screening methods include machine learning (Pradhan and Lee, 2010; Kavzoglu et al., 2015; Amato et al., 2019), correlation analysis (Jebur et al., 2014; Ciurleo et al., 2016; Steger et al., 2016; Huang et al., 2017; Chen and Li, 2020), and information gain (Chen et al., 2018b; Ngo et al., 2021). The second approach accounts for 35.1%. In this approach, the quality of environmental variables involved in the evaluation are improved by eliminating collinearity and redundancy factors, such that variable screening can be realized. The main methods include the collinearity test (Dou et al., 2019; Zhao, 2020; Azarafza et al., 2021; Chen and Chen, 2021), and chi square test (Hong et al., 2017; Pham et al., 2019). The third approach accounts for 18.9% and essentially involves the combination of different screening methods in the first two approaches (Dou et al., 2020; Hong et al., 2020; Fang et al., 2021).
As different screening methods are widely used, there is no universal and fixed criterion for selecting environmental variables for SLSM (Pradhan and Lee, 2010; Hong et al., 2019). Nevertheless, the original intention of researchers to carry out the screening of environmental variables is consistent, namely, to select variables with high quality as far as possible.
In reality, the development and distribution of landslides are closely related to the geomorphological evolution (Gómez and Kavzoglu, 2005). However, current screening methods do not sufficiently consider regional geomorphic characteristics. Moreover, the geomorphological significance of environmental variables is poorly discussed and justified (Reichenbach et al., 2018). Consequently, the environmental variables used for SLSM may not necessarily be the most suitable and provide effective information required for modeling. Furthermore, some selected variables may even be controversial. Therefore, the acquisition of higher quality environmental variables for SLSM remains to be further explored.
3 Application status of environmental variables
3.1 Quantity of variables
In the study of SLSM, the number of environmental variables used has always been controversial. Some researchers believe that effective assessment requires only a few important variables (Ohlmacher and Davis, 2003; Dahl et al., 2010; Akgün, 2012; Pereira et al., 2012). In contrast, some researchers believe that a large number of variables is more conducive to providing more comprehensive environmental information, implying more accurate landslide susceptibility assessment (Sabatakakis et al., 2013; Dou et al., 2015; Rowden and Aly, 2018; Jaafari et al., 2019). At the same time, many researchers (Remondo et al., 2003; Pradhan and Lee, 2010; Floris et al., 2011; Manzo et al., 2013; Jebur et al., 2014) have proved that the increase of the number of environmental variables cannot guarantee the quality and accuracy of susceptibility assessment, and that it may even be counterproductive. Moreover, in the presence of excessive environmental variables, the susceptibility prediction model may even have multicollinearity, high complexity, and limited explanatory power (Amato et al., 2019).
In this study, the numbers of variables used in different literature were found to be variable, with even large differences (Figure 1). In the literature database, 29 environmental variables were used at most, and 2 at least. The average number is about 10. A series of factors, such as the geological/geographical environment of the study area, disaster development/inducing conditions of the landslides, availability of regional data, and researchers’ cognition of landslides in the specific region, will affect the quantity of the environmental variables used for the susceptibility assessment (Guzzetti et al., 1999; Lee and Pradhan, 2007; Nefeslioglu et al., 2011; Felicísimo et al., 2013; Yang G. et al., 2019). As observable, there is no optimal solution for the quantity of environmental variables applied in SLSM. In the actual landslide susceptibility assessment, a corresponding number of representative environmental variables should be selected based on the specific environmental characteristics of the study area.
3.2 Type of variables
In the literature database, 91 types of environmental variables were recorded. According to the attributes, they can be divided into six types: morphological, geological, hydrological, land cover, climatic, and human activity (Figure 3A). Morphological variables are the most used among all types, accounting for more than 40%, followed by geological variables (18.66%), hydrological (16.2%), land cover (14.53%), and human activity (6.18%). Climatic variables are the least applied, accounting for only 3.84%. According to the similarity criteria of names and meanings of variables (Reichenbach et al., 2018), the six main types of environmental variables can be further subdivided into 23 subcategories. Figure 3B reveals the distribution of each subcategory of environmental variables. On the whole, 7 subcategories account for nearly 55% of the total, namely, slope, formation lithology, aspect, elevation, curvature, distance to fault, and distance to river. Among them, slope is the most widely used category, accounting for more than 10%.
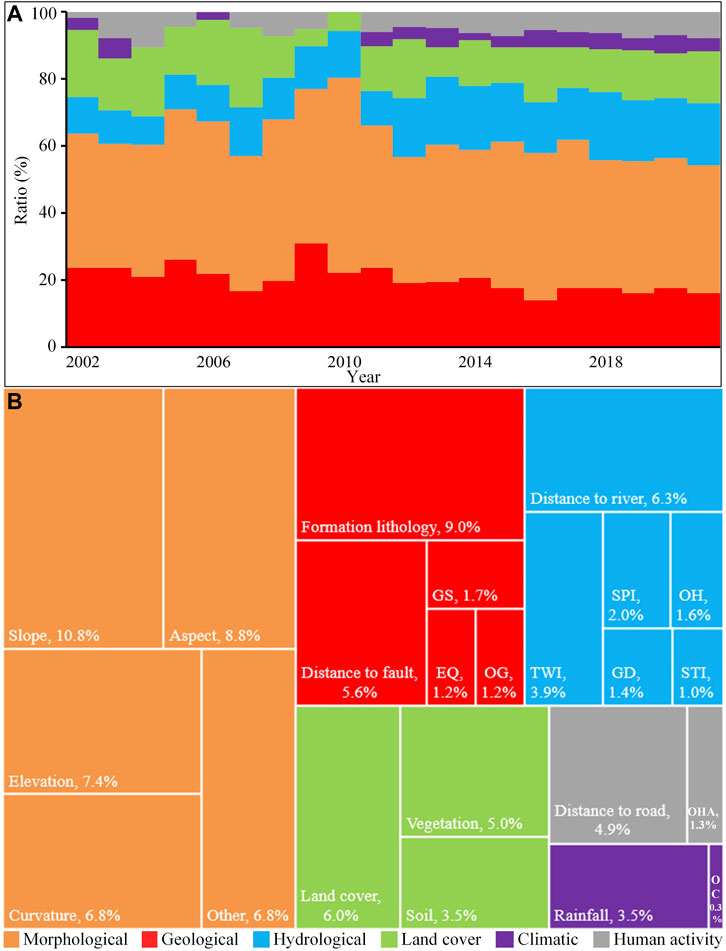
FIGURE 3. Utilization of environmental variables. (A) Proportion of different environmental variables in different years. (B) Application of different types (main categories and subcategories) of environmental variables. GS, Geological structure; EQ, Earthquake; OG, Other geological; TWI, Topographic wetness index; SPI, Stream power index; GD, Gully density; STI, Sediment transport index; OH, Other Hydrological; OHA, Other human activity; OC, Other climatic.
3.2.1 Morphological
The most widely used subtypes of morphological variables are slope, aspect, curvature (plane curvature and profile curvature), and elevation, which offer simple and direct measurement of the terrain. The wide use of these simple terrain variables is attributable to two factors: 1) They can be easily obtained through digital terrain data (usually DEM) (Xu and Xiao, 2013); 2) They are the most direct and effective measures of regional terrain/geomorphology and more effective for susceptibility prediction than other types of environmental variables (Fabbri et al., 2003).
As shown in Figure 3B, the slope variable is the most widely used among all environmental variables (carrara and Guzzetti, 1995; Fabbri et al., 2003; Budimir et al., 2015; Lima et al., 2022). Reichenbach et al. (2018) further pointed out that the slope variable is the most effective for identifying susceptible and non-susceptible areas of landslides. The main reasons are as follows: 1) The terrain slope plays an important role in controlling the balance between the sliding force and the anti-sliding force of the slope (Wu and Sidle, 1995). Compared with gentle slopes, steep slopes require stronger anti sliding force to maintain stability. 2) Different slope conditions have distinct differences in the ability to collect and store surface water and groundwater. To some extent, the slope determines the impact of surface runoff and groundwater level fluctuation on slope stability (Sun, 2020). Although simple terrain variables such as aspect, elevation and curvature are also widely used, due to the variable influence of different local conditions in different regions, the rationality of their use has not been effectively demonstrated in theory and practice (Reichenbach et al., 2018), and the rationality of their application in different environmental regions requires further analysis and consideration.
In addition, a type of composite terrain variables also exists, such as surface roughness (SR), topographic position index (TPI), relief degree of land surface, and surface cutting depth. Compared with direct terrain variables, these composite terrain variables can more comprehensively and effectively capture and reflect the overall terrain characteristics of a large area (Lee and Min, 2001; Peng et al., 2014), which can provide strong support for landslide susceptibility prediction. However, in the actual research process, these composite variables have not been widely applied. In the literature database, the SR variable was applied in only 22 articles and the TPI variable in only 13 articles. The main reasons are as follows: first, it is more difficult to obtain composite terrain variables than simple terrain variables; second, the composite variables may have very good applicability in some specific areas (Carrara and Guzzetti, 1995), but their effectiveness in other different environmental heterogeneous areas has not been generally confirmed.
3.2.2 Geological
A series of geological variables (Figure 3B) have been applied in landslide susceptibility modeling, such as formation lithology, distance to fault, and geological structure. Among them, the variables of formation lithology are the most widely used. On the one hand, formation lithology is the material basis for landslide development and movement. Different lithologies have different levels of hardness and looseness, shear strength, and weathering resistance, which have a direct impact on slope stability; therefore, formation lithology is considered an important factor affecting landslide susceptibility (Li et al., 2018; Zhu et al., 2021); On the other hand, the variables of formation lithology can be easily obtained from existing regional geological maps (Ciurleo et al., 2017; Yang J. et al., 2019; Fang et al., 2021), which greatly promotes their application in susceptibility modeling. The application frequency of distance to fault in geological variables is only second to that of formation lithology. Faults are the most active components of crustal movement, with nearby rock masses having more developed joint fissures and poor weathering resistance; therefore, geological disasters often occur near large faults (Hong et al., 2015).
As convenient and effective sources of data for obtaining regional geological variables, geological maps have been widely used by researchers (Feng et al., 2016; Zhu et al., 2018; Du et al., 2020; Huang et al., 2021b). Through the literature analysis, the remote sensing characteristics of geological variables were found to be becoming increasingly more prominent with the rapid development of remote sensing technology, Accordingly, remote sensing has gradually become another important tool for the inversion and extraction of geological variables. Many scholars obtained geological variables such as fault, lithology and structure by interpreting aerial photos and satellite images (Table 3), which are also rich sources of geological data for landslide susceptibility assessment.
For some regions with complex geological conditions, the geological environment will show significant differences in different sections, such as different fault distribution densities and regional differences in formation lithology exposure. Therefore, the regional distribution of landslides tends to show significant spatial differences (Lin et al., 2021). However, a notable phenomenon is that although remote sensing means are being used increasingly more frequently to obtain geological variables, only few scholars paid sufficient attention to the spatial heterogeneity characteristics reflected by geological variables in remote sensing images.
3.2.3 Hydrological
The distance to river is the most widely used hydrological variable (Figure 3B), accounting for 6.35% of all environmental variables. To some extent, it indicates the hydrological properties and saturation characteristics of the regional terrain (Lima et al., 2022), reflects the influence of hydrological factors on slope stability, and indirectly indicates the spatial distribution of landslides. However, in actual SLSM studies, the significance of the “distance” variables in morphological and geological aspects remain uncertain and needs to be further discussed (Reichenbach et al., 2018).
It is worth noting that DEM derived composite variables such as the topographic wetness index (TWI), stream power index (SPI), and sediment transport index (STI) are widely used among hydrological variables. Particularly in recent years, the magnitude and proportion of the application of these hydrological composite variables have shown a significant increasing trend (Figure 4). One of the most important reasons is that these composite variables can effectively represent the hydrological distribution characteristics of water and sediment movement in the region (Lian et al., 2008), and also indirectly reflect the correlation between regional hydrology and other factors, such as pore water pressure and permeability, that affect slope stability (Zhang et al., 2021; Yin et al., 2022). With these advantages, they are being increasingly applied to the prediction and determination of the spatial distribution of landslides.
In nature, different environmental regions have corresponding water resources and water environments (Yu, 2000), and the spatial distribution of hydrological elements is extremely uneven across regions, showing significant heterogeneity (Dong, 2015; Zheng et al., 2022). Therefore, the accurate representation of the spatial heterogeneity of hydrological variables is crucial for landslide susceptibility analysis. However, only few scholars have discussed this issue in detail thus far.
3.2.4 Land cover
Land cover variables and slope stability are closely related. At present, scholars generally select land cover variables from two aspects: vegetation and land use (Figure 3B). Vegetation is one of the important and key factors. As vegetation is the natural protective barrier of mountain slopes, it has the effects of slope reinforcement, reducing water and soil loss, and mitigating mountain disasters (Stokes et al., 2009; Qiu, 2012; Dai, 2021). Therefore, good vegetation coverage is often an important predictor of regional surface stability (Carrara et al., 1991).
In addition, under the influence of natural and human factors, land cover variables are highly prone to change in space, which in turn directly or indirectly affect the spatial distribution of landslides, and ultimately lead to significant spatial differences in landslide susceptibility (Reichenbach et al., 2014). With the advantages of wide coverage and high resolution, remote sensing technology provides an important and effective means for determining the spatial change characteristics of land cover variables (Liu et al., 2010; Zhang, 2016; Li et al., 2021). The analysis of the literature database revealed that although remote sensing means account for a high proportion in the acquisition methods of land cover variables (60% and 70% for land use and vegetation variables, respectively), researchers essentially began using remote sensing representation only in recent history (Bordoni et al., 2020; Lin et al., 2021; Saha et al., 2021). The spatial heterogeneity of variables in remote sensing images is rarely included in the prediction and analysis of landslide spatial distribution.
3.2.5 Climatic
Rainfall is one of the most widely used climate variables (Figure 3B). Rainfall can induce slope deformation, which is closely related to the spatial distribution of regional landslides (Li, 2020). Moreover, historical rainfall records are easy to obtain. Therefore, most researchers first consider rainfall when selecting climatic variables (Guo et al., 2015; Chen and Li, 2020; Balogun et al., 2021).
It is generally believed that the spatial distribution of landslides, debris flows, and other geological disasters is primarily controlled by rainfall variables. However, this is not the case in some regions. For example, in the Xiaojiang basin of Yunnan Province, the spatial distribution of debris flow disasters does not simply increase with rainfall, but it has a significant coupling relationship with the drought climate variables (Chen et al., 2014). Rainfall has more of an inductive effect (Yang et al., 2023), while the drought variable, representing regional weathering and material accumulation, has a significant control over the spatial distribution of disasters. For example, in the Sanjiang region at high altitude, eight areas with strong landslide development exhibit large differences in temperature conditions (Yao et al., 2020), but the rainfall variable does not show a significant dominant role. Furthermore, in the Haibalo debris-flow basin in northwest Yunnan, outbreaks of large-scale debris flows are often the result of the joint actions of rainfall and glacier melt water attributable to temperature rise (Zhao et al., 2020). It is observable that the heterogeneity of climatic conditions has an important impact on the development and distribution of geological disasters (Pourkhosravani et al., 2022). Therefore, the significant spatial heterogeneity of climate variables profoundly affects and controls the development and distribution of geological disasters. This phenomenon deserves in-depth analysis in future research because it may provide deeper insight into landslide susceptibility.
3.2.6 Human activity
With the rapid development of the economy and society, human activity variables are becoming increasingly more important in susceptibility assessment. The distance to linear engineering (highways, railways, etc.) is the most commonly used human activity variable. Although some scholars (Bai et al., 2010; Lepore et al., 2012) believe that the rationality of the impact of these variables on the spatial distribution of landslides has not been fully proved, distance variables related to human activities have always been favored by many researchers in the study of SLSM (Pham et al., 2018; Shao et al., 2020). The main reason is that such factors can be directly and conveniently obtained through DEM or topographic data with the help of GIS platforms.
Reichenbach et al. (2018) believe that different forms of linear engineering over slopes (surface cutting or underground crossing, etc.) will have distinct direct impacts on the stabilities of slopes. Moreover, such different forms will also indirectly lead to spatial differences in landslide distribution. With different types of engineering activities, human activity variables exhibit spatial heterogeneity characteristics similar to those of other environmental variables. Therefore, the effects of such heterogeneity characteristics on landslide susceptibility is worth exploring in the future.
4 Discussion and prospect
Compared with the construction of SLSM models based on ML, environmental variables have received very little attention. Nevertheless, their qualities directly determine the scientific nature and accuracy of the results of landslide susceptibility prediction. Therefore, an in-depth exploration on improving the quality of SLSM from the perspective of the acquisition and utilization of the environmental variables will be very important in the future. Through the literature review, this study identified two major scientific issues that need to be solved urgently: 1) Effective identification of important environmental variables required in SLSM. 2) Effective representation of spatial heterogeneity of environmental variables in SLSM modeling.
4.1 Identifying dominant environmental variables
Existing methods of the qualitative selection and quantitative screening of environmental variables have provided direct and practical tools for researchers and also improved the quality of the environmental variables involved in the assessment to a certain extent. However, against the background of the relatively low overall quality of SLSM (Reichenbach et al., 2018) and lack of in-depth consideration of environmental characteristics in variable selection, the method for identifying dominant variables for assessment requires further improvement. First, geo-environmental conditions significantly affect the spatial distribution of landslides (Guzzetti et al., 2005). To ensure sufficient representativeness of the landslide engineering geological properties and regional environmental characteristics of a specific study area, the selected environmental variables should be able to reflect such important influencing factors. Furthermore, as a common geological disaster in mountain areas, landslides are among the most intense surface processes and external forces of regional geomorphological evolution in the short and long terms (Broeckx et al., 2018), and the spatial distribution of landslides reflects the development and evolution characteristics of the landform to a large extent (Hu, 2019). It can be inferred that the regional geomorphological evolution will also record important environmental information required for predicting the spatial distribution of landslides to a large extent. Therefore, the above two perspectives provide important clues for solving the first scientific issue.
Based on the current research status and existing basis, this study proposes the following method (Figure 5) for identifying the dominant environmental variables for SLSM modeling. Firstly, as many variables with potential effects on the spatial distribution of landslides should be collected as possible based on the methods in Table 1 and the researchers’ cognition of the geo-environment of the study area. Subsequently, based on field surveying and the environmental characteristics of the study area, environmental variables with good representativeness of the environmental characteristics should be screened out. Finally, on the basis of traditional methods (Figure 1B), it is necessary to further explore the impact of regional geomorphological evolution on landslide distribution and further identify the geomorphological significance of environmental variables and determine the dominant variables for modeling.
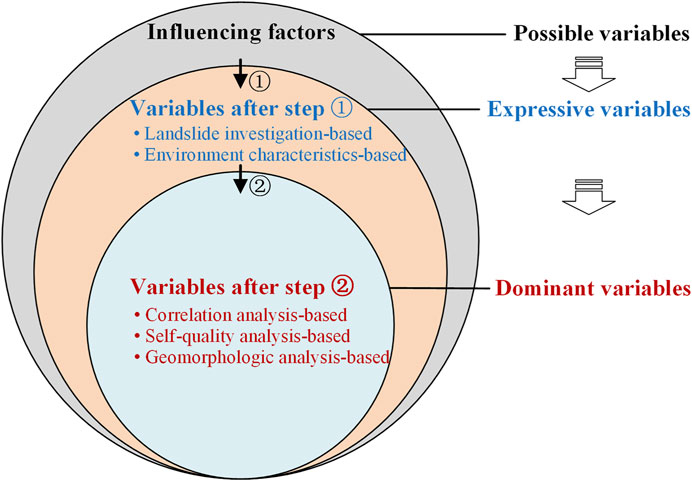
FIGURE 5. Proposed approach for identifying dominant environmental variables. Influencing factors are variables that may affect the spatial distribution of landslides. Step ① indicates the screening procedure of expressive variables based on field investigations and the researchers’ recognition of environmental characteristics. Variables after step ① indicate those that are more expressive in environmental characteristics, and are screened from the qualitative perspective. Step ② indicates the identification procedure of dominant variables based on the correlation analysis of landslides and variables, analysis of variable quality, and the influence analysis of variables on geomorphological evolution. Variables after step ② indicate those with strong correlation with landslide, high quality, and significant geomorphological significance screened from a quantitative perspective.
4.2 SLSM analysis considering geospatial patterns
Heterogeneity is a significant spatial distribution characteristic of variables in the natural environment (Cheng et al., 2020; Fu et al., 2021; Liu et al., 2022). As mentioned in Section 3.2, environmental variables required for landslide susceptibility modeling also exhibit significant spatial heterogeneity on the surface, which will lead to different dominant environmental variables and their combinations in different heterogeneous regions. However, considering the current research status, although a few researchers have begun to pay attention to the heterogeneity of environmental variables in landslide susceptibility research (Chang et al., 2022), the realization and understanding of the spatial pattern of the heterogeneity of environmental variables are lacking. Therefore, further exploration is urgently required.
In reality, different regional environmental characteristics will lead to regional differences in the spatial distribution of landslides, which will further lead to different results (Figure 6). According to field surveys, the established spatial distribution of landslides and heterogeneity of environmental variables determine the unique distribution of landslide susceptibility in a given study area, leading to specific spatial patterns. Therefore, in future SLSM research, it is necessary to draw on the idea of research concepts and methods involving the geospatial pattern (Fu, 2014), which would provide a means for solving the second scientific issue. Moreover, SLSM models should be constructed by considering the specific geospatial patterns of the study area. This may be a research direction worth considering for improving the quality of SLSM in the future.
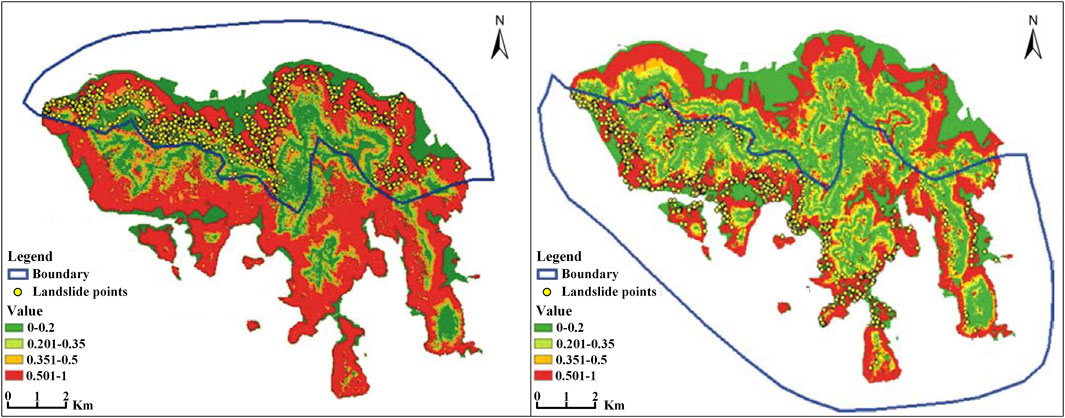
FIGURE 6. Landslides distribution in Hong Kong Island. Landslides are dense in the north and sparse in the south, and the heterogeneity in landslide distributions produces different susceptibility prediction results accordingly (Chau and Chan, 2005).
4.3 Applicability and limitation
In fact, as important input information in SLSM studies at the regional scale, the environmental variables involved in the evaluation represent environmental factors affecting the development and distribution of landslides in the study area. Therefore, their acquisition and application should be based on the actual environmental characteristics. Every region has distinctive dominant variables in a specific environment. The identification method discussed in this study (Figure 5) can realize the acquisition of dominant variables from the qualitative (Figure 5 Step ①) and quantitative (Figure 5 Step ②) perspectives. The purpose is to select the most representative and high-quality evaluation factors. However, this method also has some limitations, in that a certain theoretical basis of geomorphology is required and researchers are required to have a deep understanding of environmental characteristics.
The geospatial pattern represents the difference of regional environmental characteristics, which is directly related to the distribution pattern of landslide susceptibility. In fact, the spatial heterogeneity of environmental variables required for SLSM cannot be fully reflected for all regions. For example, the SLSM analysis method mentioned in this study may not be applicable to areas with small area and insignificant environmental differences. In contrast, it may have good reference value for areas with significant differences in environmental characteristics such as landform, geology and climate.
5 Conclusion
Based on the literature database composed of 261 peer-reviewed articles (from 2002 to 2021), this paper systematically summarizes the status of the acquisition and utilization of environmental variables used in SLSM. Moreover, possible research directions in the future are explored.
(1) As one of the important input information of SLSM models, environmental variables significantly affect the accuracy of susceptibility predictions. However, compared with the construction of ML-based susceptibility models, the acquisition and utilization of high-quality environmental variables have received very little attention.
(2) This review revealed that although the current acquisition methods of environmental variables provide direct and practical guidance for researchers to some extent, the environmental variables still lack representativeness of the landslide engineering geological properties and environmental characteristics of specific regions and their geomorphological significance. In other words, the environmental variables used in susceptibility assessment are not all dominant factors. Accordingly, one major scientific issue is raised: Effective identification of important environmental variables required in SLSM. In view of this, an approach for identifying dominant environmental variables is preliminarily proposed. Nevertheless, the identification method requires a deep understanding of environmental characteristics and a certain theoretical basis of geomorphology.
(3) This review also revealed that the current approach of utilizing various types of environmental variables is generally not based on the acknowledgment and understanding of the spatial heterogeneity of variables. Accordingly, another major scientific issue is raised: Effective representation of the spatial heterogeneity of environmental variables in SLSM modeling. Given that the established distribution of landslides and the heterogeneity of environmental variables constitute a specific spatial pattern, the concepts and methods of the geospatial pattern (Fu, 2014) should be fully taken into consideration for areas with significant environmental heterogeneity in future SLSM research.
Author contributions
XZ: literature database construction and original draft writing. ZZ, FH, JH, and ZY: review and editing. QC, DZ, XY, and JC: investigation and data analysis. LF: paper revision and language polishing.
Funding
This work is supported by the Yunnan Academic and Technical Leader Reserve Talent Project (202305AC160071), the List of Key Science and Technology Projects in the Transportation Industry of the Ministry of Transport in 2021 (Grant No. 2021-MS4-105), the Technological Innovation Plan Project of Yunnan Communications Investment & Construction Group Co., Ltd. (Grant No. YCIC-YF-2022-07), the Science and Technology Project of Yunnan Traffic Planning Design Institute Co., Ltd. (Grant No. ZL-2021-03), the Yunnan Key Laboratory of Digital Communications (Grant No. 202205AG070008), the National Natural Science Foundation of China (Grant No. 41872251), the Plateau Mountain Ecology and Earth’s Environment Discipline Construction Project (Grant No. C1762101030017) and the Joint Foundation Project between Yunnan Science and Technology Department and Yunnan University (Grants Nos. C176240210019 and 2019FY003017), the Yunnan Provincial Department of Education Science Research Fund Project (Grant No. 2023Y0196). The authors declare that this study received funding from Yunnan Communications Investment & Construction Group Co., Ltd. and Yunnan Traffic Planning Design Institute Co., Ltd. The funders were not involved in the study design, collection, analysis, interpretation of data, the writing of this article, or the decision to submit it for publication.
Conflict of interest
Authors XZ, LF, and XY were employed by the company Broadvision Engineering Consultants Co., Ltd.
The remaining authors declare that the research was conducted in the absence of any commercial or financial relationships that could be construed as a potential conflict of interest.
Publisher’s note
All claims expressed in this article are solely those of the authors and do not necessarily represent those of their affiliated organizations, or those of the publisher, the editors and the reviewers. Any product that may be evaluated in this article, or claim that may be made by its manufacturer, is not guaranteed or endorsed by the publisher.
References
Achour, Y., and Pourghasemi, H. R. (2020). How do machine learning techniques help in increasing accuracy of landslide susceptibility maps? Geosci. Front. 11 (3), 871–883. doi:10.1016/j.gsf.2019.10.001
Akgün, A. (2012). A comparison of landslide susceptibility maps produced by logistic regression, multi-criteria decision and likelihood ratio methods: Case study at izmir, Turkey. Landslides 9, 93–106. doi:10.1007/s10346-011-0283-7
Al-Najjar, H. A. H., and Pradhan, B. (2021). Spatial landslide susceptibility assessment using machine learning techniques assisted by additional data created with generative adversarial networks. Geosci. Front. 12, 625–637. doi:10.1016/j.gsf.2020.09.002
Alcántara-Ayala, I., Cui, P., and Pasuto, A. (2022). Disaster risk reduction in mountain areas: A research overview. J. Mt. Sci. 19 (6), 1487–1494. doi:10.1007/s11629-022-7487-2
Amato, G., Eisank, C., Castro-Camilo, D., and Lombardo, L. (2019). Accounting for covariate distributions in slope-unit-based landslide susceptibility models. A case study in the alpine environment. Eng. Geol. 260, 105237. doi:10.1016/j.enggeo.2019.105237
Ayalew, L., and Yamagishi, H. (2005). The application of GIS-based logistic regression for landslide susceptibility mapping in the kakuda-yahiko mountains, central Japan. Geomorphology 65, 15–31. doi:10.1016/j.geomorph.2004.06.010
Azarafza, M., Azarafza, M., Akgün, H., Atkinson, P. M., and Derakhshani, R. (2021). Deep learning-based landslide susceptibility mapping. Sci. Rep. 11, 24112. doi:10.1038/s41598-021-03585-1
Bai, S., Wang, J., Lü, G., Zhou, P., Hou, S., and Xu, S. (2010). GIS-Based logistic regression for landslide susceptibility mapping of the zhongxian segment in the three gorges area, China. Geomorphology 115 (1), 23–31. doi:10.1016/j.geomorph.2009.09.025
Balogun, A. L., Rezaie, F., Pham, Q. B., Gigović, L., Drobnjak, S., Aina, T. A., et al. (2021). Spatial prediction of landslide susceptibility in western Serbia using hybrid support vector regression (SVR) with GWO, BAT and COA algorithms. Geosci. Front. 12, 101104. doi:10.1016/j.gsf.2020.10.009
Bordoni, M., Galanti, Y., Bartelletti, C., Persichillo, M. G., Barsanti, M., Giannecchini, R., et al. (2020). The influence of the inventory on the determination of the rainfall-induced shallow landslides susceptibility using generalized additive models. Catena 193, 104630. doi:10.1016/j.catena.2020.104630
Brabb, E. E. (1984). Innovative approaches to landslide hazard and risk mapping. Proc. 4th Int. Symp. Landslides 1, 307–324.
Broeckx, J., Vanmaercke, M., Duchateau, R., and Poesen, J. (2018). A data-based landslide susceptibility map of africa. Earth-Sci. Rev. 185, 102–121. doi:10.1016/j.earscirev.2018.05.002
Budimir, M. E. A., Atkinson, P. M., and Lewis, H. G. (2015). A systematic review of landslide probability mapping using logistic regression. Landslides 12 (3), 419–436. doi:10.1007/s10346-014-0550-5
Carrara, A., Cardinali, M., Detti, R., Guzzetti, F., Pasqui, V., and Reichenbach, P. (1991). GIS techniques and statistical models in evaluating landslide hazard. Earth Surf. Proc. Land. 16 (5), 427–445. doi:10.1002/esp.3290160505
Carrara, A., and Guzzetti, F. (1995). Advances in natural technological hazard research series. in Geographical information systems in assessing natural hazards (Dordrecht, Netherlands: Kluwer Academic Publisher), 5, 342.
Carrara, A. (1983). Multivariate models for landslide hazard evaluation. Math. Geol. 15 (3), 403–426. doi:10.1007/BF01031290
Chang, Z., Catani, F., Huang, F., Liu, G., Meena, S. R., Huang, J., et al. (2022). Landslide susceptibility prediction using slope unit-based machine learning models considering the heterogeneity of conditioning factors. J. Rock Mech. Geotech. doi:10.1016/j.jrmge.2022.07.009
Chau, K. T., and Chan, J. (2005). Regional bias of landslide data in generating susceptibility maps using logistic regression: Case of Hong Kong island. Landslides 2 (4), 280–290. doi:10.1007/s10346-005-0024-x
Chen, N., Lu, Y., Zhou, H., Deng, M., and Han, D. (2014). Combined impacts of antecedent earthquakes and droughts on disastrous debris flows. J. Mt. Sci. 11 (6), 1507–1520. doi:10.1007/s11629-014-3080-7
Chen, W., and Li, Y. (2020). GIS-Based evaluation of landslide susceptibility using hybrid computational intelligence models. Catena 195, 104777. doi:10.1016/j.catena.2020.104777
Chen, W., Peng, J., Hong, H., Shahabi, H., Pradhan, B., Liu, J., et al. (2018b). Landslide susceptibility modelling using GIS-based machine learning techniques for chongren county, jiangxi province, China. Sci. Total Environ. 626, 1121–1135. doi:10.1016/j.scitotenv.2018.01.124
Chen, W., Xie, X., Peng, J., Shahabi, H., Hong, H., Bui, D. T., et al. (2018a). GIS-Based landslide susceptibility evaluation using a novel hybrid integration approach of bivariate statistical based random forest method. Catena 164, 135–149. doi:10.1016/j.catena.2018.01.012
Chen, X., and Chen, W. (2021). GIS-Based landslide susceptibility assessment using optimized hybrid machine learning methods. Catena 196, 104833. doi:10.1016/j.catena.2020.104833
Chen, Z., Ye, F., Fu, W., Ke, Y., and Hong, H. (2020). The influence of DEM spatial resolution on landslide susceptibility mapping in the baxie river basin, NW China. Nat. Hazards 101 (3), 853–877. doi:10.1007/s11069-020-03899-9
Cheng, S., Peng, P., Zhang, H., and Lu, F. (2020). Review of interpolation, reconstruction and prediction methods for heterogeneous and sparsely distributed geospatial data. Geomatics Inf. Sci. Wuhan Univ. 45 (12), 1919–1929. doi:10.13203/j.whugis20200488
Ciurleo, M., Calvello, M., and Cascini, L. (2016). Susceptibility zoning of shallow landslides in fine grained soils by statistical methods. Catena 139, 250–264. doi:10.1016/j.catena.2015.12.017
Ciurleo, M., Cascini, L., and Calvello, M. (2017). A comparison of statistical and deterministic methods for shallow landslide susceptibility zoning in clayey soils. Eng. Geol. 223, 71–81. doi:10.1016/j.enggeo.2017.04.023
Clerici, A., Perego, S., Tellini, C., and Vescovi, P. (2002). A procedure for landslide susceptibility zonation by the conditional analysis method. Geomorphology 48, 349–364. doi:10.1016/S0169-555X(02)00079-X
Costanzo, D., Rotigliano, E., Irigaray, C., Jiménez-Perálvarez, J. D., and Chacón, J. (2012). Factors selection in landslide susceptibility modelling on large scale following the GIS matrix method: Application to the river beiro basin (Spain). Nat. Hazards Earth Syst. Sci. 12, 327–340. doi:10.5194/nhess-12-327-2012
Dahl, M. -P. J., Mortensen, L. E., Veihe, A., and Jensen, N. H. (2010). A simple qualitative approach for mapping regional landslide susceptibility in the Faroe Islands. Nat. Hazards Earth Syst. Sci. 10, 159–170. doi:10.5194/nhess-10-159-2010
Dai, Z. (2021). Study on controlling factors of rainfall induced shallow landslide in well vegetated area: A case study of liziyuan forest farm in qinzhou district, gansu Province. Beijing: Beijing Forestry University. doi:10.26949/d.cnki.gblyu.2021.000723
Dong, Z. (2015). On features of five dominant ecological components of aquatic ecosystem. Water Resour. Hydropower Eng. 46 (6), 41–47. doi:10.13928/j.cnki.wrahe.2015.06.008
Dou, J., Yamagishi, H., Pourghasemi, H. R., Yunus, A. P., Song, X., Xu, Y., et al. (2015). An integrated artificial neural network model for the landslide susceptibility assessment of osado island, Japan. Nat. Hazards 78, 1749–1776. doi:10.1007/s11069-015-1799-2
Dou, J., Yunus, A. P., Bui, D. T., Merghadi, A., Sahana, M., Zhu, Z., et al. (2019). Assessment of advanced random forest and decision tree algorithms for modeling rainfall-induced landslide susceptibility in the izu-oshima volcanic island, Japan. Sci. Total Environ. 662, 332–346. doi:10.1016/j.scitotenv.2019.01.221
Dou, J., Yunus, A. P., Merghadi, A., Shirzadi, A., Nguyen, H., Hussain, Y., et al. (2020). Different sampling strategies for predicting landslide susceptibilities are deemed less consequential with deep learning. Sci. Total Environ. 720, 137320. doi:10.1016/j.scitotenv.2020.137320
Du, J., Glade, T., Woldai, T., Chai, B., and Zeng, B. (2020). Landslide susceptibility assessment based on an incomplete landslide inventory in the jilong valley, tibet, Chinese himalayas. Eng. Geol. 270, 105572. doi:10.1016/j.enggeo.2020.105572
Duan, Y., Tang, J., Liu, Y., Gao, X., and Duan, Y. (2022). Spatial sensitivity evaluation of loess landslide in liulin county, shanxi based on random forest. Sci. Geogr. Sin. 42 (2), 343–351. doi:10.13249/j.cnki.sgs.2022.02.016
Ercanoglu, M., and Gokceoglu, C. (2002). Assessment of landslide susceptibility for a landslide-prone area (north of yenice, NW Turkey) by fuzzy approach. Environ. Geol. 41, 720–730. doi:10.1007/s00254-001-0454-2
Erener, A., Mutlu, A., and Düzgün, H. S. (2016). A comparative study for landslide susceptibility mapping using GIS-based multi-criteria decision analysis (MCDA), logistic regression (LR) and association rule mining (ARM). Eng. Geol. 203, 45–55. doi:10.1016/j.enggeo.2015.09.007
Ermini, L., Catani, F., and Casagli, N. (2005). Artificial neural networks applied to landslide susceptibility assessment. Geomorphology 66, 327–343. doi:10.1016/j.geomorph.2004.09.025
Fabbri, A. G., Chung, C-J. F., Cendrero, A., and Remondo, J. (2003). Is prediction of future landslides possible with a GIS? Nat. Hazards 30 (3), 487–503. doi:10.1023/B:NHAZ.0000007282.62071.75,
Fang, Z., Wang, Y., Peng, L., and Hong, H. (2021). A comparative study of heterogeneous ensemble-learning techniques for landslide susceptibility mapping. Int. J. Geogr. Inf. Sci. 35 (2), 321–347. doi:10.1080/13658816.2020.1808897
Felicísimo, A., Cuartero, A., Remondo, J., and Quirós, E. (2013). Mapping landslide susceptibility with logistic regression, multiple adaptive regression splines, classification and regression trees, and maximum entropy methods: A comparative study. Landslide 10, 175–189. doi:10.1007/s10346-012-0320-1
Feng, H., Zhou, A., Yu, J., Tang, X., Zheng, J., Chen, X., et al. (2016). A comparative study on plum-rain-triggered landslide susceptibility assessment models in west zhejiang province. Earth Sci. 41 (3), 403–415. doi:10.3799/dqkx.2016.032
Floris, M., Iafelice, M., Squarzoni, C., Zorzi, L., De Agostini, A., and Genevois, R. (2011). Using online databases for landslide susceptibility assessment: An example from the veneto region (northeastern Italy). Nat. Hazards Earth Syst. Sci. 11, 1915–1925. doi:10.5194/nhess-11-1915-2011
Fu, B. (2014). The integrated studies of geography: Coupling of patterns and processes. Acta Geogr. Sin. 69 (8), 1052–1059. doi:10.11821/dlxb201408002
Furlani, S., and Ninfo, A. (2015). Is the present the key to the future? Earth-Sci. Rev. 142, 38–46. doi:10.1016/j.earscirev.2014.12.005
Gómez, H., and Kavzoglu, T. (2005). Assessment of shallow landslide susceptibility using artificial neural networks in jabonosa river basin, Venezuela. Eng. Geol. 78, 11–27. doi:10.1016/j.enggeo.2004.10.004
Guo, C., Montgomery, D. R., Zhang, Y., Wang, K., and Yang, Z. (2015). Quantitative assessment of landslide susceptibility along the xianshuihe fault zone, Tibetan plateau, China. Geomorphology 248, 93–110. doi:10.1016/j.geomorph.2015.07.012
Guzzetti, F., Carrara, A., Cardinali, M., and Reichenbach, P. (1999). Landslide hazard evaluation: A review of current techniques and their application in a multi-scale study, central Italy. Geomorphology 31, 181–216. doi:10.1016/S0169-555X(99)00078-1
Guzzetti, F., Galli, M., Reichenbach, P., Ardizzone, F., and Cardinali, M. (2006a). Landslide hazard assessment in the collazzone area, umbria, central Italy. Nat. Hazard. Earth Sys. 6, 115–131. doi:10.5194/nhess-6-115-2006
Guzzetti, F., Mondini, A. C., Cardinali, M., Fiorucci, F., Santangelo, M., and Chang, K. -T. (2012). Landslide inventory maps: New tools for an old problem. Earth-Sci. Rev. 112, 42–66. doi:10.1016/j.earscirev.2012.02.001
Guzzetti, F., Reichenbach, P., Ardizzone, F., Cardinali, M., and Galli, M. (2006b). Estimating the quality of landslide susceptibility models. Geomorphology 81, 166–184. doi:10.1016/j.geomorph.2006.04.007
Guzzetti, F., Reichenbach, P., Cardinali, M., Galli, M., and Ardizzone, F. (2005). Probabilistic landslide hazard assessment at the basin scale. Geomorphology 72, 272–299. doi:10.1016/j.geomorph.2005.06.002
He, Q., Shahabi, H., Shirzadi, A., Li, S., Chen, W., Wang, N., et al. (2019). Landslide spatial modelling using novel bivariate statistical based naïve bayes, RBF classifier, and RBF network machine learning algorithms. Sci. Total Environ. 633, 1–15. doi:10.1016/j.scitotenv.2019.01.329
Hong, H., Ilia, I., Tsangaratos, P., Chen, W., and Xu, C. (2017). A hybrid fuzzy weight of evidence method in landslide susceptibility analysis on the wuyuan area, China. Geomorphology 290, 1–16. doi:10.1016/j.geomorph.2017.04.002
Hong, H., Liu, J., Bui, D. T., Pradhan, B., Acharya, T. D., Pham, B. T., et al. (2018). Landslide susceptibility mapping using J48 decision tree with AdaBoost, bagging and rotation forest ensembles in the guangchang area (China). Catena 163, 399–413. doi:10.1016/j.catena.2018.01.005
Hong, H., Liu, J., and Zhu, A-X. (2020). Modeling landslide susceptibility using LogitBoost alternating decision trees and forest by penalizing attributes with the bagging ensemble. Sci. Total Environ. 718, 137231. doi:10.1016/j.scitotenv.2020.137231
Hong, H., Miao, Y., Liu, J., and Zhu, A-X. (2019). Exploring the effects of the design and quantity of absence data on the performance of random forest-based landslide susceptibility mapping. Catena 176, 45–64. doi:10.1016/j.catena.2018.12.035
Hong, H., Pradhan, B., Xu, C., and Bui, D. T. (2015). Spatial prediction of landslide hazard at the yihuang area (China) using two-class kernel logistic regression, alternating decision tree and support vector machines. Catena 133, 266–281. doi:10.1016/j.catena.2015.05.019
Hu, S. (2019). Spatial pattern of landslide in loess plateau and its influence on geomorphologic evolution. Xi’an: Northwest University.
Huang, F., Chen, J., Tang, Z., Fan, X., Huang, J., Zhou, C., et al. (2021a). Uncertainties of landslide susceptibility prediction due to different spatial resolutions and different proportions of training and testing datasets. Chin. J. Rock Mech. Eng. 40 (6), 1155–1169. doi:10.13722/j.cnki.jrme.2020.1119
Huang, F., Wang, Y., Dong, Z., Wu, L., Guo, Z., and Zhang, T. (2019). Regional landslide susceptibility mapping based on grey relaional degree model. Earth Sci. 44 (2), 664–676. doi:10.3799/dqkx.2018.175
Huang, F., Ye, Z., Jiang, S., Huang, J., Chang, Z., and Chen, J. (2021b). Uncertainty study of landslide susceptibility prediction considering the different attribute interval numbers of environmental factors and different data-based models. Catena 202, 105250. doi:10.1016/j.catena.2021.105250
Huang, F., Yin, K., Huang, J., Gui, L., and Wang, P. (2017). Landslide susceptibility mapping based on self-organizing-map network and extreme learning machine. Eng. Geol. 229, 11–22. doi:10.1016/j.enggeo.2017.04.013
Hussin, H. Y., Zumpano, V., Reichenbach, P., Sterlacchini, S., Micu, M., van Westen, C., et al. (2016). Different landslide sampling strategies in a grid-based bivariate statistical susceptibility model. Geomorphology 253, 508–523. doi:10.1016/j.geomorph.2015.10.030
Jaafari, A., Panahi, M., Pham, B. T., Shahabi, H., Bui, D. T., Rezaie, F., et al. (2019). Meta optimization of an adaptive neuro-fuzzy inference system with grey wolf optimizer and biogeography-based optimization algorithms for spatial prediction of landslide susceptibility. Catena 175, 430–445. doi:10.1016/j.catena.2018.12.033
Jebur, M. N., Pradhan, B., and Tehrany, M. S. (2014). Optimization of landslide conditioning factors using very high-resolution airborne laser scanning (LiDAR) data at catchment scale. Remote Sens. Environ. 152, 150–165. doi:10.1016/j.rse.2014.05.013
Juliev, M., Mergili, M., Mondal, I., Nurtaev, B., Pulatov, A., and Hübl, J. (2019). Comparative analysis of statistical methods for landslide susceptibility mapping in the bostanlik district, Uzbekistan. Sci. Total Environ. 653, 801–814. doi:10.1016/j.scitotenv.2018.10.431
Kamp, U., Growley, B. J., Khattak, G. A., and Owen, L. A. (2008). GIS-Based landslide susceptibility mapping for the 2005 kashmir Earthquake region. Geomorphology 101, 631–642. doi:10.1016/j.geomorph.2008.03.003
Kavzoglu, T., Sahin, E. K., and Colkesen, I. (2015). Selecting optimal conditioning factors in shallow translational landslide susceptibility mapping using genetic algorithm. Eng. Geol. 192, 101–112. doi:10.1016/j.enggeo.2015.04.004
Lee, S. (2007). Application and verification of fuzzy algebraic operators to landslide susceptibility mapping. Environ. Geol. 52, 615–623. doi:10.1007/s00254-006-0491-y
Lee, S. (2005). Application of logistic regression model and its validation for landslide susceptibility mapping using GIS and remote sensing data. Int. J. Remote Sens. 26 (7), 1477–1491. doi:10.1080/01431160412331331012
Lee, S., Hwang, J., and Park, I. (2012). Application of data-driven evidential belief functions to landslide susceptibility mapping in jinbu, korea. Catena 100, 15–30. doi:10.1016/j.catena.2012.07.014
Lee, S., and Min, K. (2001). Statistical analysis of landslide susceptibility at yongin, korea. Env. Geol. 40 (9), 1095–1113. doi:10.1007/s002540100310
Lee, S., and Pradhan, B. (2007). Landslide hazard mapping at selangor, Malaysia using frequency ratio and logistic regression models. Landslides 4, 33–41. doi:10.1007/s10346-006-0047-y
Lepore, C., Kamal, S. A., Shanahan, P., and Bras, R. L. (2012). Rainfall-induced landslide susceptibility zonation of Puerto Rico. Environ. Earth Sci. 66 (6), 1667–1681. doi:10.1007/s12665-011-0976-1
Li, C., Zhuang, D., He, J., and Wen, K. (2021). Spatiotemporal variations in remote sensing phenology of vegetation and its responses to temperature change of boreal forest in tundra-taiga transitional zone in the eastern siberia. Acta Geogr. Sin. 76 (7), 1634–1648. doi:10.11821/dlxb202107005
Li, G., Yang, X., Li, H., and Le, Q. (2018). Dataset of geo-hazards in fengjie mapsheet (1:50,000), chongqing. Geol. China 45 (S1), 160–169. doi:10.12029/gc2018Z110
Li, H. (2020). Accounting for spatial autocorrelation into regional landslide susceptibility assessment. Wuhan: Wuhan University. doi:10.27379/d.cnki.gwhdu.2020.001211
Li, Y., Liu, X., Han, Z., and Dou, J. (2020). Spatial proximity-based geographically weighted regression model for landslide susceptibility assessment: A case study of qingchuan area, China. Appl. Sci. 10, 1107. doi:10.3390/app10031107
Lian, G., Guo, X., Fu, B., and Hu, C. (2008). Spatial variability and prediction of soil nutrients on A county scale on the loess plateau: A case study of hengshan county, shaanxi province. Acta Pedol. Sin. 45 (4), 577–584.
Lima, P., Steger, S., Glade, T., and Murillo-García, F. G. (2022). Literature review and bibliometric analysis on data-driven assessment of landslide susceptibility. J. Mt. Sci. 19 (6), 1670–1698. doi:10.1007/s11629-021-7254-9
Lin, Q., Lima, P., Steger, S., Glade, T., Jiang, T., Zhang, J., et al. (2021). National-scale data-driven rainfall induced landslide susceptibility mapping for China by accounting for incomplete landslide data. Geosci. Front. 12, 101248. doi:10.1016/j.gsf.2021.101248
Liu, F., Zhu, A-X., and Pei, T. (2010). Application of high temporal resolution satellite remote sensing in identifying soil texture patterns. J. Geo-information Sci. 15 (5), 733–740.
Liu, Q., Liu, H., Zhang, Y., Wu, H., and Deng, M. (2022). A spatio-temporal prediction method of large-scale ground subsidence considering spatial heterogeneity. Natl. Remote Sens. Bull. 26 (7), 1315–1325. doi:10.11834/jrs.20211445
Liu, Z., Yang, Z., Chen, M., Xu, H., Yang, Y., Zhang, J., et al. (2023). Research hotspots and Frontiers of Mountain flood disaster: Bibliometric and visual analysis. Water 15, 673. doi:10.3390/w15040673
Lucchese, L. V., de Oliveira, G. G., and Pedrollo, O. C. (2021). Investigation of the influence of nonoccurrence sampling on landslide susceptibility assessment using artificial neural networks. Catena 198, 105067. doi:10.1016/j.catena.2020.105067
Wu, H., Zhang, X., Qiao, Y., Liang, Y., Zhang, Y., Yang, S., et al. (2017). Landslide susceptibility evaluation based on certain factor and weight of evidence: a case study in the Longkaikou to Qina section of Jinshajiang watershed. Geoscience 31 (6), 1269–1277. Available from: https://www.webofscience.com/wos/alldb/full-record/CSCD:6139846.
Manzo, G., Tofani, V., Segoni, S., Battistini, A., and Catani, F. (2013). GIS techniques for regional-scale landslide susceptibility assessment: The sicily (Italy) case study. Int. J. Geogr. Inf. Sci. 27, 1433–1452. doi:10.1080/13658816.2012.693614
Moayedi, H., Mehrabi, M., Mosallanezhad, M., Rashid, A. S. A., and Pradhan, B. (2019). Modification of landslide susceptibility mapping using optimized PSOANN technique. Eng. Comput. 35, 967–984. doi:10.1007/s00366-018-0644-0
Myronidis, D., Papageorgiou, C., and Theophanous, S. (2016). Landslide susceptibility mapping based on landslide history and analytic hierarchy process (AHP). Nat. Hazards 81, 245–263. doi:10.1007/s11069-015-2075-1
Nefeslioglu, H. A., Gokceoglu, C., Sonmez, H., and Gorum, T. (2011). Medium-scale hazard mapping for shallow landslide initiation: The buyukkoy catchment area (cayeli, rize, Turkey). Landslides 8, 459–483. doi:10.1007/s10346-011-0267-7
Neuland, H. (1976). A prediction model of landslips. Catena 3 (2), 215–230. doi:10.1016/0341-8162(76)90011-4
Ngo, P. T. T., Panahi, M., Khosravi, K., Ghorbanzadeh, O., Kariminejad, N., Cerda, A., et al. (2021). Evaluation of deep learning algorithms for national scale landslide susceptibility mapping of Iran. Geosci. Front. 12, 505–519. doi:10.1016/j.gsf.2020.06.013
Nguyen, V. V., Pham, B. T., Vu, B. T., Prakash, I., Jha, S., Shahabi, H., et al. (2019). Hybrid machine learning approaches for landslide susceptibility modeling. Forests 10, 157. doi:10.3390/f10020157
Nikoobakht, S., Azarafza, M., Akgün, H., and Derakhshani, R. (2022). Landslide susceptibility assessment by using convolutional neural network. Appl. Sci. 12 (12), 5992. doi:10.3390/app12125992
Nsengiyumva, J. B., Luo, G., Amanambu, A. C., Mind'je, R., Habiyaremye, G., Karamage, F., et al. (2019). Comparing probabilistic and statistical methods in landslide susceptibility modeling in Rwanda/Centre-Eastern africa. Sci. Total Environ. 659, 1457–1472. doi:10.1016/j.scitotenv.2018.12.248
Ohlmacher, G. C., and Davis, J. C. (2003). Using multiple logistic regression and GIS technology to predict landslide hazard in northeast Kansas, USA. Eng. Geol. 69, 331–343. doi:10.1016/S0013-7952(03)00069-3
Pei, X., Zhang, S., Huang, R., Zhang, X., Wang, S., Chang, Z., et al. (2018). Study on the centrifuge model test of landslide induced by enrichment of groundwater. Adv. Eng. Sci. 50 (5), 55–63. doi:10.15961/j.jsuese.201700983
Peng, D. (2018). Study on early recognition for potentially loess landslide: A case study at heifangtai terrace, gansu Province, China. Chengdu: Chengdu University of Technology. doi:10.26986/d.cnki.gcdlc.2018.000010
Peng, L., Niu, R., Huang, B., Wu, X., Zhao, Y., and Ye, R. (2014). Landslide susceptibility mapping based on rough set theory and support vector machines: A case of the three gorges area, China. Geomorphology 204, 287–301. doi:10.1016/j.geomorph.2013.08.013
Pereira, S., Zêzere, J. L., and Bateira, C. (2012). Technical Note: Assessing predictive capacity and conditional independence of landslide predisposing factors for shallow landslide susceptibility models. Nat. Hazards Earth Syst. Sci. 12, 979–988. doi:10.5194/nhess-12-979-2012
Pham, B. T., Pradhan, B., Bui, D. T., Prakash, I., and Dholakia, M. B. (2016). A comparative study of different machine learning methods for landslide susceptibility assessment: A case study of uttarakhand area (India). Environ. Model. Softw. 84, 240–250. doi:10.1016/j.envsoft.2016.07.005
Pham, B. T., Prakash, I., Singh, S. K., Shirzadi, A., Shahabi, H., Tran, T., et al. (2019). Landslide susceptibility modeling using reduced error pruning trees and different ensemble techniques: Hybrid machine learning approaches. Catena 175, 203–218. doi:10.1016/j.catena.2018.12.018
Pham, B. T., Shirzadi, A., Bui, D. T., Prakash, I., and Dholakia, M. B. (2018). A hybrid machine learning ensemble approach based on a radial basis function neural network and rotation forest for landslide susceptibility modeling: A case study in the himalayan area, India. Int. J. Sediment. Res. 33, 157–170. doi:10.1016/j.ijsrc.2017.09.008
Pourghasemi, H. R., Kornejady, A., Kerle, N., and Shabani, F. (2020). Investigating the effects of different landslide positioning techniques, landslide partitioning approaches, and presence-absence balances on landslide susceptibility mapping. Catena 187, 104364. doi:10.1016/j.catena.2019.104364
Pourghasemi, H. R., Mohammady, M., and Pradhan, B. (2012). Landslide susceptibility mapping using index of entropy and conditional probability models in GIS: Safarood basin, Iran. Catena 97, 71–84. doi:10.1016/j.catena.2012.05.005
Pourghasemi, H. R., and Rahmati, O. (2018). Prediction of the landslide susceptibility: Which algorithm, which precision? Catena 162, 177–192. doi:10.1016/j.catena.2017.11.022
Pourkhosravani, M., Mehrabi, A., Pirasteh, S., and Derakhshani, R. (2022). Monitoring of maskun landslide and determining its quantitative relationship to different climatic conditions using D-InSAR and PSI techniques. Geomat. Nat. Haz. Risk 13 (1), 1134–1153. doi:10.1080/19475705.2022.2065939
Pradhan, B. (2013). A comparative study on the predictive ability of the decision tree, support vector machine and neuro-fuzzy models in landslide susceptibility mapping using GIS. Comput. Geosci. 51, 350–365. doi:10.1016/j.cageo.2012.08.023
Pradhan, B., and Lee, S. (2010). Landslide susceptibility assessment and factor effect analysis: Backpropagation artificial neural networks and their comparison with frequency ratio and bivariate logistic regression modelling. Environ. Modell. Softw. 25, 747–759. doi:10.1016/j.envsoft.2009.10.016
Qiu, H. (2012). Study on the regional landslide characteristics analysis and hazard assessment: A case study of ningqiang county. Xi’an: Northwest University.
Reichenbach, P., Busca, C., Mondini, A. C., and Rossi, M. (2014). The influence of land use change on landslide susceptibility zonation: The briga catchment test site (messina, Italy). Environ. Manage. 54 (6), 1372–1384. doi:10.1007/s00267-014-0357-0
Reichenbach, P., Rossi, M., Malamud, B. D., Mihir, M., and Guzzetti, F. (2018). A review of statistically-based landslide susceptibility models. Earth-Sci. Rev. 180, 60–91. doi:10.1016/j.earscirev.2018.03.001
Remondo, J., González, A., De Terán, J. R. D., Cendrero, A., Fabbri, A., and Chung, C. F. (2003). Validation of landslide susceptibility maps; examples and applications from a case study in northern Spain. Nat. Hazards 30, 437–449. doi:10.1023/B:NHAZ.0000007201.80743.fc
Rossi, M., Guzzetti, F., Rechenbach, P., Mondini, A. C., and Peruccacci, S. (2010). Optimal landslide susceptibility zonation based on multiple forecasts. Geomorphology 114, 129–142. doi:10.1016/j.geomorph.2009.06.020
Rowden, K. W., and Aly, M. H. (2018). A novel triggerless approach for mass wasting susceptibility modeling applied to the boston mountains of Arkansas, USA. Nat. Hazards 92 (1), 347–367. doi:10.1007/s11069-018-3201-7
Sabatakakis, N., Koukis, G., Vassiliades, E., and Lainas, S. (2013). Landslide susceptibility zonation in Greece. Nat. Hazards 65, 523–543. doi:10.1007/s11069-012-0381-4
Saha, S., Roy, J., Pradhan, B., and Hembram, T. K. (2021). Hybrid ensemble machine learning approaches for landslide susceptibility mapping using different sampling ratios at east Sikkim himalayan, India. Adv. Space Res. 68, 2819–2840. doi:10.1016/j.asr.2021.05.018
Samia, J., Temme, A., Bregt, A., Wallinga, J., Guzzetti, F., Aedizzone, F., et al. (2017). Characterization and quantification of path dependency in landslide susceptibility. Geomorphology 292, 16–24. doi:10.1016/j.geomorph.2017.04.039
San, B. T. (2014). An evaluation of SVM using polygon-based random sampling in landslide susceptibility mapping: The candir catchment area (western antalya, Turkey). Int. J. Appl. Earth Obs. 26, 399–412. doi:10.1016/j.jag.2013.09.010
Shao, X., Ma, S., Xu, C., and Zhou, Q. (2020). Effects of sampling intensity and non-slide/slide sample ratio on the occurrence probability of coseismic landslides. Geomorphology 363, 107222. doi:10.1016/j.geomorph.2020.107222
Soeters, R., and van Westen, C. J. (1996). “Slope instability recognition, analysis, and zonation,” in Landslides: Investigation and mitigation. Transportation research board special report 247. Editors K. A. Turner, and R. L. Schuster (National Research Council, Washington, USA).
Steger, S., Brenning, A., Bell, R., Petschko, H., and Glade, T. (2016). Exploring discrepancies between quantitative validation results and the geomorphic plausibility of statistical landslide susceptibility maps. Geomorphology 262, 8–23. doi:10.1016/j.geomorph.2016.03.015
Stokes, A., Atger, C., Bengough, A. G., Fourcaud, T., and Sidle, R. C. (2009). Desirable plant root traits for protecting natural and engineered slopes against landslides. Plant Soil 324 (1-2), 1–30. doi:10.1007/s11104-009-0159-y
Sun, D., Xu, J., Wen, H., and Wang, D. (2021). Assessment of landslide susceptibility mapping based on bayesian hyperparameter optimization: A comparison between logistic regression and random forest. Eng. Geol. 281, 105972. doi:10.1016/j.enggeo.2020.105972
Sun, X. (2020). Study on landslide susceptibility and risk mapping along the rapidly uplifting section of the upper jinsha river: A case of xulong to benzilan reach. Changchun: Jilin University. doi:10.27162/d.cnki.gjlin.2020.001120
Taner San, B. (2014). An evaluation of SVM using polygon-based random sampling in landslide susceptibility mapping: The candir catchment area (western antalya, Turkey). Int. J. Appl. Earth Obs. 26, 399–412. doi:10.1016/j.jag.2013.09.010
Trigila, A., Iadanza, C., Esposito, C., and Scarascia-Mugnozza, G. (2015). Comparison of logistic regression and random forests techniques for shallow landslide susceptibility assessment in giampilieri (NE sicily, Italy). Geomorphology 249, 119–136. doi:10.1016/j.geomorph.2015.06.001
van Westen, C. J., Castellanos, E., and Kuriakose, S. L. (2008). Spatial data for landslide susceptibility, hazard, and vulnerability assessment: An overview. Eng. Geol. 102 (3–4), 112–131. doi:10.1016/j.enggeo.2008.03.010
Wu, W., and Sidle, R. C. (1995). A distributed slope stability model for steep forested basins. Water Resour. Res. 31, 2097–2110. doi:10.1029/95WR01136
Wu, X., Ren, F., Niu, R., and Peng, L. (2013). Landslide spatial prediction based on slope units and support vector machines. Geomatics Inf. Sci. Wuhan Univ. 38 (12), 1499–1503. doi:10.13203/j.whugis2013.12.006
Xu, C., and Xiao, J. Z. (2013). Spatial analysis of landslides triggered by the 2013 Ms7.0 lushan Earthquake: A case study of a typical rectangle area in the northeast of taiping town. Seismol. Geol. 35 (2), 436–451. doi:10.3969/j.issn.0253-4967.2013.02.021
Yalcin, A. (2008). GIS-Based landslide susceptibility mapping using analytical hierarchy process and bivariate statistics in ardesen (Turkey): Comparisons of results and confirmations. Catena 72, 1–12. doi:10.1016/j.catena.2007.01.003
Yan, F., Zhang, Q., Ye, S., and Ren, B. (2019). A novel hybrid approach for landslide susceptibility mapping integrating analytical hierarchy process and normalized frequency ratio methods with the cloud model. Geomorphology 327, 170–187. doi:10.1016/j.geomorph.2018.10.024
Yang, G., Xu, P., Cao, C., Zhang, W., Lan, Z., Chen, J., et al. (2019). Assessment of regional landslide susceptibility based on combined model of certainty factor method. J. Eng. Geol. 27 (5), 1153–1163. doi:10.13544/j.cnki.jeg.2019018
Yang, J., Song, C., Yang, Y., Xu, C., Guo, F., and Xie, L. (2019). New method for landslide susceptibility mapping supported by spatial logistic regression and GeoDetector: A case study of duwen highway basin, sichuan province, China. Geomorphology 324, 62–71. doi:10.1016/j.geomorph.2018.09.019
Yang, Z., Zhao, X., Chen, M., Zhang, J., Yang, Y., Chen, W., et al. (2023). Characteristics, dynamic analyses and hazard assessment of debris flows in niumiangou valley of wenchuan county. Appl. Sci. 13, 1161. doi:10.3390/app13021161
Yao, X., Deng, J., Liu, X., Zhou, Z., Yao, J., Dai, F., et al. (2020). Primary recognition of active landslides and development rule analysis for Pan three-river-parallel territory of tibet plateau. Adv. Eng. Sci. 52 (5), 16–37. doi:10.15961/j.jsuese.202000529
Yin, Y., Zhang, C., Yan, H., Xiao, M., Hou, X., Zhu, S., et al. (2022). Research on seepage stability and prevention design of landslides during impoundment operation of the Three Gorges Reservoir, China. Chin. J. Rock Mech. Eng. 41 (4), 649–659. doi:10.13722/j.cnki.jrme.2021.0674
Youssef, A. M., and Pourghasemi, H. R. (2021). Landslide susceptibility mapping using machine learning algorithms and comparison of their performance at abha basin, asir region, Saudi arabia. Geosci. Front. 12, 639–655. doi:10.1016/j.gsf.2020.05.010
Yu, P. (2000). Application of physically-based distributed models in forest hydrology. For. Res. 4, 431–438. doi:10.13275/j.cnki.lykxyj.2000.04.015
Zêzere, J. L. (2002). Landslide susceptibility assessment considering landslide typology. A case study in the area north of lisbon (Portugal). Nat. Hazards Earth Syst. Sci. 2, 73–82. doi:10.5194/nhess-2-73-2002
Zhang, C., Yin, Y., Yan, H., Li, H., Dai, Z., and Zhang, N. (2021). Reactivation characteristics and hydrological inducing factors of a massive ancient landslide in the three gorges reservoir, China. Eng. Geol. 292 (1), 106273. doi:10.1016/j.enggeo.2021.106273
Zhang, Q., Ling, S., Li, X., Sun, C., Xu, J., and Huang, T. (2020). Comparison of landslide susceptibility mapping rapid assessment models in Jiuzhaigou County, Sichuan province, China. Chin. J. Rock Mech. Eng. 39 (8), 1595–1610. doi:10.13722/j.cnki.jrme.2020.0029
Zhang, Y., Qin, S., Zhai, J., Li, G., Peng, S., Liu, X., et al. (2018). Susceptibility assessment of debris flow based on GIS and weight information for the changbai mountain area. Hydrogeology Eng. Geol. 45 (2), 150–158. doi:10.16030/j.cnki.issn.1000-3665.2018.02.23
Zhang, Z., Deng, M., Xu, S., Zhang, Y., Fu, H., and Li, Z. (2022). Comparison of landslide susceptibility assessment models in zhenkang county, yunnan province, China. Chin. J. Rock Mech. Eng. 41 (1), 157–171. doi:10.13722/j.cnki.jrme.2021.0360
Zhang, Z. (2016). Land use/land cover classification and change detection in mountainous areas using multi-resolution remotely sensed data – a case study of manwan reservoir basin. Kunming: Yunnan University.
Zhao, F. (2020). Early identification of geological hazards and landslides susceptibility evaluation of karakorum highway (domestic section). Lanzhou: Lanzhou University. doi:10.27204/d.cnki.glzhu.2020.000254
Zhao, X., Li, G., Zhao, Z., Li, C., Chen, Q., and Ye, X. (2022). Identifying the spatiotemporal characteristics of individual red bed landslides: A case study in western yunnan, China. J. Mt. Sci. 19 (6), 1748–1766. doi:10.1007/s11629-022-7339-0
Zhao, X., Zhang, H., Zhao, Z., Xia, L., Liu, B., Li, Y., et al. (2020). Study on the genesis of rainfall-glacier mixed type debris flow of Haibalo gully in northwest Yunnan on july 28, 2019. J. Eng. Geol. 28 (6), 1339–1349. doi:10.13544/j.cnki.jeg.2020-505
Zhao, Y., Wang, R., Jiang, Y., Liu, H., and Wei, Z. (2019). GIS-Based logistic regression for rainfall-induced landslide susceptibility mapping under different grid sizes in yueqing, southeastern China. Eng. Geol. 259, 105147. doi:10.1016/j.enggeo.2019.105147
Zheng, T., Cai, H., and Liu, J. (2022). Effects of land use and climate change on spatial distribution characteristics of hydrological factors in tongzi river basin [J/OL]. Journal of Yangtze River Scientific Research Institute. Available at: https://kns.cnki.net/kcms/detail/42.1171.TV.20220513.1529.005.html.
Zhu, A-X., Miao, Y., Yang, L., Bai, S., Liu, J., Hong, H., et al. (2018). Comparison of the presence-only method and presence-absence method in landslide susceptibility mapping. Catena 171, 222–233. doi:10.1016/j.catena.2018.07.012
Keywords: literature review, landslide, susceptibility zonation, statistically-based method, environmental variable, geomorphological significance, spatial heterogeneity
Citation: Zhao X, Zhao Z, Huang F, Huang J, Yang Z, Chen Q, Zhou D, Fang L, Ye X and Chao J (2023) Application of environmental variables in statistically-based landslide susceptibility mapping: A review. Front. Earth Sci. 11:1147427. doi: 10.3389/feart.2023.1147427
Received: 18 January 2023; Accepted: 21 February 2023;
Published: 24 March 2023.
Edited by:
Wei Zhao, Institute of Mountain Hazards and Environment (CAS), ChinaReviewed by:
Zheng Han, Central South University, ChinaReza Derakhshani, Utrecht University, Netherlands
Copyright © 2023 Zhao, Zhao, Huang, Huang, Yang, Chen, Zhou, Fang, Ye and Chao. This is an open-access article distributed under the terms of the Creative Commons Attribution License (CC BY). The use, distribution or reproduction in other forums is permitted, provided the original author(s) and the copyright owner(s) are credited and that the original publication in this journal is cited, in accordance with accepted academic practice. No use, distribution or reproduction is permitted which does not comply with these terms.
*Correspondence: Zhifang Zhao, emhhb3poaWZhbmdAeW51LmVkdS5jbg==