- 1Cooperative Institute for Research in Environmental Sciences, University of Colorado Boulder, Boulder, CO, United States
- 2National Snow and Ice Data Center, University of Colorado Boulder, Boulder, CO, United States
Polar lows (PLs) are intense maritime mesoscale weather systems that develop during marine cold air outbreaks at high latitudes. The objective of this review is to describe the advances in polar low research since the last literature review—published 3 years ago—, indicate the knowledge gaps that remain, and suggest promising courses of research. Among the breakthroughs identified here are the first climatology of PLs obtained with a global atmospheric model, and increased evidence showing that baroclinic instability is the main mechanism leading to PL development. Despite these advances, many challenges persist such as the lack of conventional observations of PLs and the need to better understand coupled atmosphere-ocean processes involved in PL development. With the rapid advances in deep learning, this method has the potential to be used for PL forecasting.
1 Introduction
Polar lows (PLs) are strong mesoscale maritime cyclones that form near the snow-covered continents or sea ice edge during marine cold air outbreaks (MCAOs) at high latitudes. PLs have a horizontal scale ranging from 200 to 1,000 km, and their associated near-surface winds are near or above gale force (Turner et al., 2003). Their typical lifetime ranges from 12 to 32 h (Stoll, 2022). The cloud signature of PLs is comma-shaped (e.g., Moreno-Ibáñez et al., 2023b) or spiraliform (e.g., Ganeshan et al., 2022; Figure 1), and can change during their lifetime (e.g., Lackner et al., 2023). The last comprehensive literature review on PL research was published 3 years ago by Moreno-Ibáñez et al. (2021). PL research has greatly advanced since then; for instance, a climatology of PLs has been obtained using a global atmospheric model for the first time (Bresson et al., 2022).
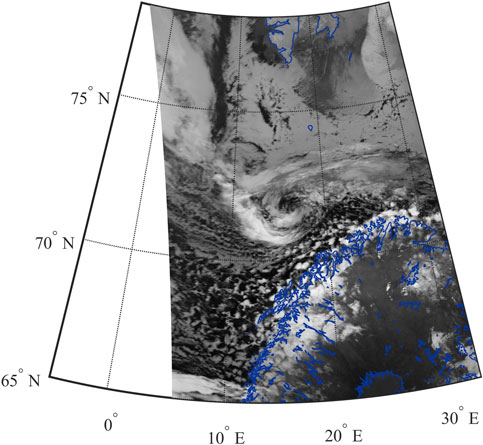
FIGURE 1. Polar low developed on 1 March 2021 captured by the Advanced Very High Resolution Radiometer (AVHRR) channel 4. The blue outlining represents the shoreline.
The objective of this review is to summarize the recent advances in polar low research since the review conducted by Moreno-Ibáñez et al. (2021) in order to highlight the knowledge gaps that still need to be addressed as well as promising directions of research. This review is relevant and timely given that a preparatory planning phase is currently undergoing for the 5th International Polar Year (IPY) 2032–20331. This manuscript is structured in five main sections: observations and numerical representation of PLs, present and future climatology of PLs, development mechanisms of PLs, other advances in PL research, and discussion.
2 Observations and numerical representation of PLs
2.1 Observations
Despite the wide variety of observations at the mesoscale (Bluestein et al., 2022), conventional observations over the ocean at high latitudes are scarce. The recent observations of PLs during the Cold-air Outbreaks in the Marine Boundary Layer Experiment (COMBLE, Geerts et al., 2022) have allowed the analysis of their vertical structure (Lackner et al., 2023). In 2022, the HALO-(AC)3 airborne field campaign, whose objective was to provide observations of air mass transformation during meridional transport to and from the Arctic, captured several MCAOs and a PL (Ehrlich et al., 2023). This year, the Cold Air Outbreak Experiment in the Sub-Arctic Region (CAESAR)2 will provide observations of clouds that form during MCAOs, and has therefore the potential to capture PLs. Regarding satellite observations, Synthetic Aperture Radar (SAR) observations provide details of the structure of PLs thanks to their high resolution (Tollinger et al., 2021). Atmospheric fronts and cyclonic centers are key features in SAR images that allow the identification of polar mesoscale cyclones using a deep learning algorithm (Grahn and Bianchi, 2022). However, SAR observations have rarely been used to study PLs (e.g., Hallerstig et al., 2021), probably because the current wind retrieval techniques perform poorly with highly variable wind (Tollinger et al., 2021).
2.2 Reanalyses
ERA5 (Hersbach et al., 2020; Bell et al., 2021), the fifth–generation reanalysis of the European Centre for Medium–Range Weather Forecasts, is a valuable global reanalysis for the study of PLs given its relatively high horizontal resolution and hourly output. It has a 31-km grid mesh and 137 vertical levels that extend to 0.01 hPa (Hersbach et al., 2020). Since its release, ERA5 has been used to develop climatologies of PLs (e.g., Stoll, 2022), to analyze their structure (e.g., Meyer et al., 2021) and the large–scale conditions associated with their development (e.g., Boyd et al., 2022), and as initial and boundary conditions for limited-area atmospheric models (e.g., Wu, 2021). ERA5 captures more PLs than its predecessor, ERA-Interim (Stoll et al., 2021). Nevertheless, ERA5 underestimates near–surface wind speed associated with PLs (Haakenstad et al., 2021; Gurvich et al., 2022; Køltzow et al., 2022). The Copernicus Arctic Regional Reanalysis (CARRA, Yang et al., 2020), which uses ERA5 as lateral boundary conditions, has a 2.5–km grid mesh and 65 vertical levels, and provides 3-hourly output. CARRA shows better performance than ERA5 (Isaksen et al., 2022; Køltzow et al., 2022). In particular, CARRA provides a better representation of the 10–m wind and 2–m temperature during the dissipation of PLs over land (Køltzow et al., 2022). The 3-km Norwegian Reanalysis (NORA3, Haakenstad et al., 2021) shows more skill than ERA5 at representing the 10–m wind speed, especially over the mountains and the coast.
2.3 Atmospheric models
With an increasing horizontal resolution, global atmospheric models will become a useful tool to study PLs. For example, Bresson et al. (2022) have provided the first climatology of PLs with a global atmospheric model. High-resolution atmospheric models are commonly used to study PLs because they provide details of their structure, which is essential to explain their genesis and intensification mechanisms (e.g., Moreno-Ibáñez et al., 2023a). The resolution of the model determines how certain processes associated with PL development are represented. For example, the higher the atmospheric resolution, the higher the maximum surface sensible and latent heat fluxes associated with MCAOs are (Spensberger and Spengler, 2021). Xue et al. (2021) recommend using a grid mesh of less than 3 km to simulate PLs. The representation of PLs by atmospheric models strongly depends on the initial conditions and on the initialization time (Revokatova et al., 2021; Xue et al., 2021; Moreno-Ibáñez et al., 2023b). High-resolution initial conditions are important to correctly simulate PLs (Xue et al., 2021). Moreover, high-resolution boundary conditions at the ocean surface, especially near the sea ice edge, are also likely to be important to correctly represent the atmospheric temperature gradients that contribute to PL genesis. For instance, Renfrew et al. (2021) have found that the gradients of the surface fluxes and near-surface atmospheric fields over the marginal ice zone simulated by Met Office’s Unified Model (MetUM, Bush et al., 2020), which has a 2.2-km grid mesh, were not as sharp as observed during the Iceland-Greenland Seas Project (Renfrew et al., 2019) since the sea ice field in the surface boundary conditions was too smooth. When simulating PLs, the use of data assimilation provides better results compared to a “cold start” (i.e., using analyses and reanalyses) (Kim et al., 2019; Xue et al., 2021), and the assimilation of satellite radiances is recommended (Xue et al., 2021). Assimilating adaptively thinned cloud-cleared hyperspectral infrared radiances from the Atmospheric Infrared Sounder instrument on board the NASA Aqua satellite can have a positive impact on the representation of PLs (Ganeshan et al., 2022).
2.4 Wave models
Kudryavtsev et al. (2022) suggest applying extended duration laws to provide first-guess wave parameters that can be used with a two-dimensional parametric wave model to simulate waves associated with PLs, and this method has been applied by Yurovskaya et al. (2023) to estimate the wavelength and significant wave height of waves associated with PLs.
3 Present and future climatology of PLs
MCAO indexes have been often used in climatology studies as indicator of favorable conditions for PL genesis (e.g., Landgren et al., 2019). In general, the indexes used to identify MCAOs are computed by subtracting the potential temperature at a certain pressure level from the sea surface potential temperature (e.g., Fletcher et al., 2016). Consequently, these indexes are very sensitive to the selected pressure level (Meyer et al., 2021). To tackle this limitation, Terpstra et al. (2021) and Meyer et al. (2021) have developed new MCAO indexes that take into account the vertical structure of the air masses. By using the PL list STARS-DAT (Noer et al., 2011; Eastwood et al., 2012), Meyer et al. (2021) have shown that their MCAO index has more skill at indicating the location and time of PL formation than the traditional MCAO indexes. Nevertheless, although MCAO is an essential condition for PL genesis, it is not sufficient (Terpstra et al., 2021; Boyd et al., 2022). A more adequate proxy for PLs is the PL genesis potential index (PGI) developed by Boyd et al. (2022), which is composed of an environmental baroclinicity index in addition to a MCAO index, and captures the observed climatology of PLs reasonably well.
Recently, a few subjective and objective climatologies of PLs have been produced. Using a subjective method, Golubkin et al. (2021) have obtained a PL list in the North Atlantic for 2015–2017. In contrast to previous studies (Moreno-Ibáñez et al., 2021), the authors have not found a high PL activity over the Irminger Sea, and they have found that the PL season is somewhat shorter than previously reported. Objective global (Stoll, 2022), Northern Hemisphere (Bresson et al., 2022), and Southern Ocean (Farjami and Kazemi, 2024) climatologies of PLs have also been developed. Stoll (2022) has obtained a global climatology of PLs with ERA5 reanalysis (Hersbach et al., 2020) for 1979–2020. Bresson et al. (2022) have obtained recent (1985–2010) and future (2085–2110) climatologies of PLs in the Northern Hemisphere for the cold season with the Hadley Centre Global Environment Model version 3 Global Atmosphere-only version 3 (HadGEM3-GA3, Walters et al., 2011; Williams et al., 2015) with a 25-km grid mesh and 85 vertical levels. Farjami and Kazemi (2024) have obtained a climatology of PLs in the Southern Ocean between 60°S and 85°S for 2000–2020 using ERA5 reanalysis (Hersbach et al., 2020) and other datasets. Regarding the recent climatology of PLs, the spatial distribution and seasonal cycle obtained by Stoll (2022), Bresson et al. (2022) and Farjami and Kazemi (2024) are qualitatively similar to those reported in the literature (Moreno-Ibáñez et al., 2021). In the Northern Hemisphere, most PLs develop at latitudes poleward of 50°N in the North Atlantic and 40°N in the North Pacific (Stoll, 2022; Figure 2A). Although rare, some PLs develop in the marginal seas of the Arctic Ocean (e.g., Gurvich et al., 2022). In the Southern Hemisphere, most PLs develop near the sea ice edge, between 50°S and 65°S (Stoll, 2022; Figure 2B). They are most frequent over the Amundsen, Bellingshausen, and Ross Seas (Stoll, 2022; Farjami and Kazemi, 2024).
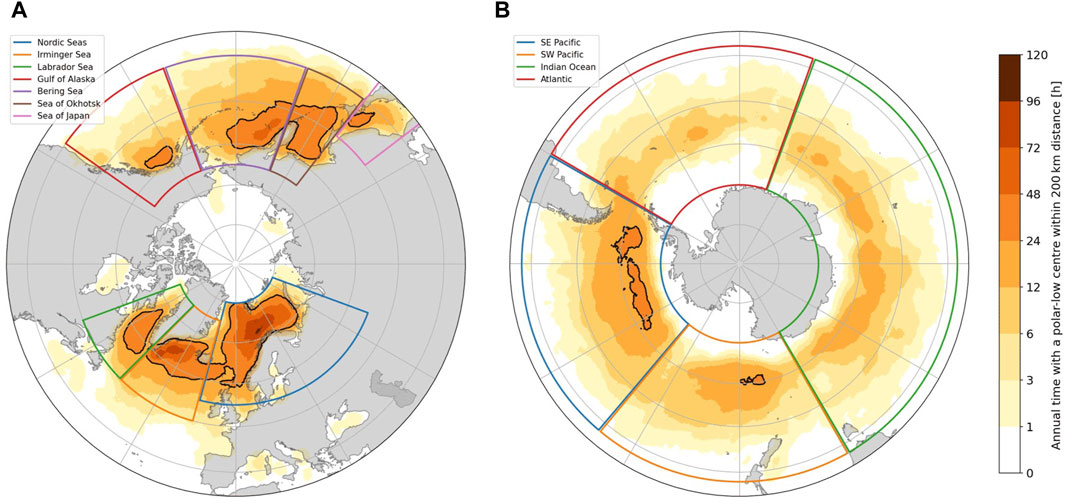
FIGURE 2. Annual mean density of polar low activity, defined as the number of polar low time steps within 200 km, for the (A) Northern Hemisphere and (B) Southern Hemisphere. The scale of the colorbar is logarithmic. Regions with polar low activity larger than 24 h yr–1 are delimited by a black contour. © Stoll (2022). This work is distributed under the Creative Commons Attribution 4.0 License (CC BY 4.0; https://creativecommons.org/licenses/by/4.0/). No changes have been made.
The impact of climate change on the frequency of PLs in the recent climate remains uncertain. In the Northen Hemisphere, Bresson et al. (2022) have not found a significant tendency in PL frequency, in agreement with previous studies (Moreno-Ibáñez et al., 2021). Nevertheless, Stoll (2022) has found a statistically significant increase in PL activity in the Northern Hemisphere for 1979–2020, and Chen and von Storch (2013) have found a slight increase in PL frequency in the North Pacific for 1948–2010. In the Southern Hemisphere, the PL frequency has not notably changed (Stoll et al., 2018; Stoll, 2022).
In the future, global warming is projected to lead to increased atmospheric stability (Landgren et al., 2019) and decreased Arctic sea ice extent (IPCC, 2021), which will affect the distribution of ocean surface heat fluxes (Moore et al., 2022). Like previous studies (Moreno-Ibáñez et al., 2021), Bresson et al. (2022) have found that there will be new areas of PL development where the sea ice retreats, and that the frequency of PLs will decrease in the Northern Hemisphere. The authors have also found a decrease in the intensity of PLs in the Northern Hemisphere, but the impact of global warming on the intensity of PLs remains uncertain. There is low confidence in the projected decline of Antarctic sea ice (IPCC, 2021), although the fact that there have been three record low summer sea ice extent in the last 7 years may indicate that the Antarctic sera ice has transitioned to a low-extent state (Purich and Doddridge, 2023). To the author’s best knowledge, no study has examined how climate change will affect the spatiotemporal distribution of PLs in the Antarctic. Given the low confidence in Antarctic sea ice projections, this area of research is best left for future research when models represent the Antarctic sea ice evolution more accurately.
4 Development mechanisms of PLs
In the last decades of the past century, there was a controversy regarding the mechanisms of PL development, with some authors arguing that PLs formed by baroclinic instability (e.g., Mansfield, 1974), and others affirming that PLs were convective systems (e.g., Rasmussen, 1979). Other studies pointed at the joint action of baroclinic instability and convection in PL development (e.g., Businger, 1985). In recent years, studies have provided evidence that moist baroclinic instability plays an important role in PL development (Terpstra et al., 2015; Haualand and Spengler, 2020; Stoll et al., 2021), and recent cases studies of PLs provide detailed examples of this mechanism (Moreno-Ibáñez et al., 2023a; Lackner et al., 2023). Moreover, the evidence does not support the existence of hurricane-like PLs (Kolstad and Bracegirdle, 2017; Stoll et al., 2021). Indeed, the spiraliform cloud signature of some PLs could be due to a warm seclusion process (Stoll et al., 2021; Lackner et al., 2023). Thus, strong baroclinicity in addition to low static stability are key ingredients for PL development (Boyd et al., 2022; Wang et al., 2023). There are other factors that contribute to PL development, such as the generation of convergence zones when MCAOs occur downstream of a sea ice edge with a certain shape (Watanabe et al., 2022), and the occurrence of weather events that can trigger PL formation, such as katabatic storms (e.g., Gutjahr et al., 2022). Although baroclinic eddies are the main responsible for poleward energy transport in mid-latitudes (Holton and Hakim, 2013), Stoll et al. (2023) have found that the meridional energy transport by mesoscale eddies—such as PLs—is negligible on annual and seasonal timescales.
Consistent with baroclinic instability as the main mechanism for PL development, PLs mainly form and intensify in environments with strong wind shear—forward-shear, reverse-shear, right-shear and left-shear—and dissipate in weak-shear environments (Stoll et al., 2021). Most often, PLs develop in forward-shear environments (Stoll, 2022). The type of environments where PLs form is closely related to their translation direction. PLs with north-eastward (southward) motion tend to develop in forward-shear (reverse-shear) environments, PLs with eastward motion are common in left-shear environments, and PLs with slow motion without a dominating direction occur in environments with any type of shear (Yan et al., 2023).
Despite coupled atmosphere–ocean-sea ice interactions being involved in PL development, this area of research has been largely neglected. Since PLs form in MCAO, the coupled processes that take place during those events are relevant for their development. A case study of an observed MCAO in the western Nordic Seas has showed that the ocean mixed layer warmed in the boundary current region, whereas it cooled in the interior region of the Iceland Sea (Renfrew et al., 2023). Consistent with this finding, Wu (2021) has found that the large surface heat fluxes associated with PLs cause sea surface temperature (SST) cooling, but, in regions with an ocean temperature inversion—such as near the sea ice edge—the strong winds associated with PLs lead to SST warming through upper-ocean mixing. In turn, this SST warming or cooling influences PL development through surface heat fluxes (Wu, 2021).
5 Other advances in PL research
Subseasonal prediction of PL activity is an area of research that has gained increased attention. The Madden-Julian Oscillation has significant impacts on PL frequency in the Northern Hemisphere (Wang et al., 2023). In the Norwegian and Barents Seas, PLs are most frequent during the Atlantic Ridge regime and the negative phase of the North Atlantic Oscillation (NAO-) regime, and are less frequent during the Scandinavian blocking regime (Mallet et al., 2013; Yan et al., 2023; Boyd and Wang, 2024). In the Irminger Sea, PLs are more frequent during the positive phase of the NAO (NAO+) regime, and less frequent during the NAO- regime and the Scandinavian blocking regime (Boyd and Wang, 2024). In the Labrador Sea, PL activity is strongly decreased during the NAO- regime (Mallet et al., 2013; Chang et al., 2022; Yan et al., 2023; Boyd and Wang, 2024). Sudden stratospheric warmings (SSWs) are associated with a notable decrease in PL activity over the Labrador Sea during the following 3 weeks (Chang et al., 2022), which is consistent with the fact that SSWs are associated with a decreased frequency of MCAOs over this area (Afargan-Gerstman et al., 2020). In the Bering Sea, Sea of Okhotsk, and Gulf of Alaska, PL activity increases during the Arctic low regime, and decreases during the Pacific wave train regime (Boyd and Wang, 2024). A promising method to produce subseasonal forecasts of PL activity is the PGI (Boyd et al., 2023; Boyd and Wang, 2024), but its forecasting skill depends on the region, with predictability being highest over the Nordic, Irminger, Labrador, and Bering Seas (Boyd et al., 2023).
Machine learning¸ which has many applications in meteorology (Chase et al., 2022; Chase et al., 2023) has been used to study PLs. For instance, self-organizing maps have been used to identify typical environments where PLs develop by mapping PL-centered temperature anomaly fields to 3 × 3 nodes (Stoll et al., 2021) and deep learning has been used to detect maritime polar mesoscale cyclones in satellite images (Krinitskiy et al., 2018; Grahn and Bianchi, 2022).
6 Discussion
This review has shown that there have been important advances in PL research that address some of the challenges identified by Moreno-Ibáñez et al. (2021), such as the first climatology of PLs obtained with a global atmospheric model (Bresson et al., 2022), efforts to improve the representation of PLs in atmospheric models (e.g., Ganeshan et al., 2022), and a better understanding of PL development mechanisms (e.g., Stoll et al., 2021). Notwithstanding this, many challenges remain. The main advances in PL research and remaining challenges can be summarized as follows:
• Observations and numerical representation of PLs: PL research using ERA5 global reanalysis has been fruitful, but the potential of CARRA and NORA3 regional reanalyses for the study of PLs has not been explored yet. Despite efforts to improve the representation of PLs in high-resolution atmospheric models, it is unclear which variables in the initial conditions have the most important impact on PL forecasting. More conventional observations of PLs are needed to adequately assess the performance of high-resolution models.
• Present and future climatology of PLs: The first climatology of PLs with a global atmospheric model has opened new perspectives in the study of the impact of climate change on the spatio-temporal distribution of PLs, an area of research where many uncertainties remain. Although the need to conceive an intercomparison project of PL detection and tracking to determine the common characteristics of PLs obtained with different tracking methods and datasets has been highlighted in previous Polar Low Workshops (e.g., Spengler et al., 2017), such endeavor has not been undertaken yet.
• Development mechanisms of PLs: Recent research points at baroclinic instability having a major role in PL development. Knowledge about coupled atmosphere-ocean interactions involved in PL development is lacking.
• Other advances: The PGI is a new tool that can be used for subseasonal forecasting of PL activity. Despite its potential for the study PLs, machine learning has not been widely used in PL studies yet.
In what follows, the main key challenges in PL research and promising courses of research are discussed in more detail.
Conventional observations with high spatial and temporal resolution are needed to verify simulations of PLs with high-resolution atmospheric models. Therefore, planning for IPY 2032–2033 should ensure that targeted observations of PLs are made in regions where conventional observations are scarce. In addition, the investigation of the coupled atmosphere-ocean processes undoubtedly deserves more attention. The effect of atmosphere-ocean interactions in PL development remains to be elucidated. In the case of tropical cyclones, studies have shown that coupled atmosphere-ocean simulations lead to a decrease in their intensity compared to atmosphere-only simulations (e.g., Smith et al., 2018). Therefore, it is recommended to conduct such studies with PLs to see if the same results are obtained. The effect of atmosphere-ocean interactions on the ocean during PL development should also be investigated, with especial emphasis on the impact of PLs on the ocean circulation (e.g., Condron and Renfrew, 2013). Given that conducting high-resolution simulations with global coupled climate models is computationally expensive, a first step would be to conduct such a simulation for a short period of time. For instance, Gutjahr et al. (2022) have conducted a global coupled climate model simulation with a 5-km grid mesh covering 2 years, and they have found that katabatic storms lead to dense water formation in the western boundary current of the Irminger Sea.
Deep learning could become a useful tool for PL forecasting. The use of recurrent neural networks to forecast PL track and intensity, a method that has already been applied in tropical cyclone studies (e.g., Kapoor et al., 2023), is a promising area of research. Nevertheless, the application of deep learning to weather forecasting is in its beginnings (Schultz et al., 2021).
In summary, there have been important advances in PL research during the last 3 years, but many questions remain regarding, e.g., their impact on the ocean circulation. High-resolution coupled atmosphere-ocean-sea ice models, together with high-resolution conventional observations, are key to answering these questions.
Data availability statement
AVHRR channel 4 observations are available from EUMETSAT (2011) at https://navigator.eumetsat.int/product/EO:EUM:DAT:METOP:AVHRRL1. The dataset used to plot the shorelines is the Global Self-consistent, Hierarchical, High-resolution Geography Database (GSHHG; Wessel and Smith, 1996), available at https://www.ngdc.noaa.gov/mgg/shorelines/.
Author contributions
MM-I: Conceptualization, Investigation, Methodology, Visualization, Writing–original draft, Writing–review and editing.
Funding
The author(s) declare financial support was received for the research, authorship, and/or publication of this article. MM-I was supported by the CIRES Visiting Fellows Program, funded by NOAA Cooperative Agreement NA22OAR4320151. The statements, findings, conclusion, and recommendations are those of the author and do not necessarily reflect the views of NOAA or the U.S. Department of Commerce.
Conflict of interest
The author declares that the research was conducted in the absence of any commercial or financial relationships that could be construed as a potential conflict of interest.
Publisher’s note
All claims expressed in this article are solely those of the authors and do not necessarily represent those of their affiliated organizations, or those of the publisher, the editors and the reviewers. Any product that may be evaluated in this article, or claim that may be made by its manufacturer, is not guaranteed or endorsed by the publisher.
Footnotes
1The initial concept note of the IPY 2032–2033 is available at https://iasc.info/images/IPY/Initial_IPY_Concept_Note_-_Version_October_2023.pdf (accessed on 5 January 2024).
2Information about CAESAR is available at https://www.eol.ucar.edu/field_projects/caesar (accessed on 17 February 2024).
References
Afargan-Gerstman, H., Polkova, I., Papritz, L., Ruggieri, P., King, M. P., Athanasiadis, P. J., et al. (2020). Stratospheric influence on North Atlantic marine cold air outbreaks following sudden stratospheric warming events. Weather Clim. Dyn. 1 (2), 541–553. doi:10.5194/wcd-1-541-2020
Bell, B., Hersbach, H., Simmons, A., Berrisford, P., Dahlgren, P., Horányi, A., et al. (2021). The ERA5 global reanalysis: preliminary extension to 1950. Q. J. R. Meteorological Soc. 147 (741), 4186–4227. doi:10.1002/qj.4174
Bluestein, H. B., Carr, F. H., and Goodman, S. J. (2022). Atmospheric observations of weather and climate. Atmosphere-Ocean 60 (3-4), 149–187. doi:10.1080/07055900.2022.2082369
Boyd, K., and Wang, Z. (2024). Revisiting the relationship between polar lows and weather regimes. J. Clim. 37 (5), 1523–1538. doi:10.1175/JCLI-D-23-0309.1
Boyd, K., Wang, Z., Walsh, J., and Stoll, P. (2023). Subseasonal predictions of polar low activity using a hybrid statistical-dynamical approach. Geophys. Res. Lett. 50 (12), e2022GL102145. doi:10.1029/2022GL102145
Boyd, K., Wang, Z., and Walsh, J. E. (2022). A genesis potential index for polar lows. J. Clim. 35 (24), 7891–7902. doi:10.1175/JCLI-D-22-0100.1
Bresson, H., Hodges, K. I., Shaffrey, L. C., Zappa, G., and Schiemann, R. (2022). The response of northern Hemisphere polar lows to climate change in a 25 km high-resolution global climate model. J. Geophys. Res. Atmos. 127 (4), e2021JD035610. doi:10.1029/2021JD035610
Bush, M., Allen, T., Bain, C., Boutle, I., Edwards, J., Finnenkoetter, A., et al. (2020). The first Met Office unified model–JULES regional atmosphere and land configuration, RAL1. Geosci. Model Dev. 13 (4), 1999–2029. doi:10.5194/gmd-13-1999-2020
Businger, S. (1985). The synoptic climatology of polar low outbreaks. Tellus A Dyn. Meteorology Oceanogr. 37 (5), 419–432. doi:10.3402/tellusa.v37i5.11686
Chang, C.-C., Wang, Z., Walsh, J., and Stoll, P. J. (2022). Modulation of North Atlantic polar low activity and associated flow patterns by sudden stratospheric warmings. J. Clim. 35 (13), 4013–4026. doi:10.1175/jcli-d-21-0905.1
Chase, R. J., Harrison, D. R., Burke, A., Lackmann, G. M., and McGovern, A. (2022). A machine learning tutorial for operational meteorology. Part I: traditional machine learning. Weather Forecast. 37 (8), 1509–1529. doi:10.1175/WAF-D-22-0070.1
Chase, R. J., Harrison, D. R., Lackmann, G. M., and McGovern, A. (2023). A machine learning tutorial for operational meteorology. Part II: neural networks and deep learning. Weather Forecast. 38 (8), 1271–1293. doi:10.1175/WAF-D-22-0187.1
Chen, F., and von Storch, H. (2013). Trends and variability of North Pacific polar lows. Adv. Meteorology 2013, 1–11. doi:10.1155/2013/170387
Condron, A., and Renfrew, I. A. (2013). The impact of polar mesoscale storms on northeast Atlantic Ocean circulation. Nat. Geosci. 6 (1), 34–37. doi:10.1038/ngeo1661
Eastwood, S., Gusdal, Y., Drivdal, M., Furevik, B., Schyberg, H., and Tveter, F. T. (2012). STARS-DAT v3 user manual. Oslo, Bergen and Tromsø: Norwegian Meteorological Institute. Version 3.1.
Ehrlich, A., Wendisch, M., Klingebiel, M., Mech, M., Crewell, S., Herber, A., et al. (2023). HALO-(AC)3: airborne observations of arctic clouds in airmass transformations. Vienna, Austria: EGU General Assembly.
Farjami, H., and Kazemi, A. F. (2024). Spatiotemporal patterns of polar low activity over the Southern Ocean. J. Geophys. Res. Atmos. 129 (4), e2023JD039832. doi:10.1029/2023JD039832
Fletcher, J., Mason, S., and Jakob, C. (2016). The climatology, meteorology, and boundary layer structure of marine cold air outbreaks in both hemispheres. J. Clim. 29 (6), 1999–2014. doi:10.1175/jcli-d-15-0268.1
Ganeshan, M., Reale, O., McGrath-Spangler, E., and Boukachaba, N. (2022). Impact of assimilating adaptively thinned AIRS cloud-cleared radiances on the analysis of polar lows and mediterranean sea tropical-like cyclone in a global modeling and data assimilation framework. Weather Forecast. 37 (7), 1117–1134. doi:10.1175/WAF-D-21-0068.1
Geerts, B., Giangrande, S. E., McFarquhar, G. M., Xue, L., Abel, S. J., Comstock, J. M., et al. (2022). The COMBLE campaign: a study of marine boundary layer clouds in arctic cold-air outbreaks. Bull. Am. Meteorological Soc. 103 (5), E1371–E1389. doi:10.1175/BAMS-D-21-0044.1
Golubkin, P., Smirnova, J., and Bobylev, L. (2021). Satellite-derived spatio-temporal distribution and parameters of North Atlantic polar lows for 2015-2017. Atmosphere 12 (2), 224. doi:10.3390/atmos12020224
Grahn, J., and Bianchi, F. M. (2022). Recognition of polar lows in Sentinel-1 SAR images with deep learning. IEEE Trans. Geoscience Remote Sens. 60, 1–12. doi:10.1109/TGRS.2022.3204886
Gurvich, I., Pichugin, M., and Baranyuk, A. (2022). Satellite multi-sensor data analysis of unusually strong polar lows over the Chukchi and Beaufort Seas in October 2017. Remote Sens. 15 (1), 120. doi:10.3390/rs15010120
Gutjahr, O., Jungclaus, J. H., Brüggemann, N., Haak, H., and Marotzke, J. (2022). Air-Sea interactions and water mass transformation during a katabatic storm in the Irminger Sea. J. Geophys. Res. Oceans 127 (5), e2021JC018075. doi:10.1029/2021JC018075
Haakenstad, H., Breivik, Ø., Furevik, B. R., Reistad, M., Bohlinger, P., and Aarnes, O. J. (2021). NORA3: a nonhydrostatic high-resolution hindcast of the North Sea, the Norwegian Sea, and the Barents Sea. J. Appl. Meteorology Climatol. 60 (10), 1443–1464. doi:10.1175/JAMC-D-21-0029.1
Hallerstig, M., Magnusson, L., Kolstad, E. W., and Mayer, S. (2021). How grid-spacing and convection representation affected the wind speed forecasts of four polar lows. Q. J. R. Meteorological Soc. 147 (734), 150–165. doi:10.1002/qj.3911
Haualand, K. F., and Spengler, T. (2020). Direct and indirect effects of surface fluxes on moist baroclinic development in an idealized framework. J. Atmos. Sci. 77 (9), 3211–3225. doi:10.1175/jas-d-19-0328.1
Hersbach, H., Bell, B., Berrisford, P., Hirahara, S., Horányi, A., Muñoz-Sabater, J., et al. (2020). The ERA5 global reanalysis. Q. J. R. Meteorological Soc. 146 (730), 1999–2049. doi:10.1002/qj.3803
Holton, J. R., and Hakim, G. J. (2013). An introduction to dynamic meteorology. San Diego, USA: Elsevier Inc.
IPCC (2021). “Summary for policymakers,” in Climate change 2021: the physical science basis. Contribution of Working Group I to the Sixth Assessment Report of the Intergovernmental Panel on Climate Change. Editors V Masson-Delmotte, P Zhai, A Pirani, SL Connors, C Péan, and S Berger.
Isaksen, K., Nordli, Ø., Ivanov, B., Køltzow, M. A. Ø., Aaboe, S., Gjelten, H. M., et al. (2022). Exceptional warming over the Barents area. Sci. Rep. 12 (1), 9371. doi:10.1038/s41598-022-13568-5
Kapoor, A., Negi, A., Marshall, L., and Chandra, R. (2023). Cyclone trajectory and intensity prediction with uncertainty quantification using variational recurrent neural networks. Environ. Model. Softw. 162, 105654. doi:10.1016/j.envsoft.2023.105654
Kim, D.-H., Kim, H. M., and Hong, J. (2019). Evaluation of wind forecasts over Svalbard using the high-resolution Polar WRF with 3DVAR. Arct. Antarct. Alp. Res. 51 (1), 471–489. doi:10.1080/15230430.2019.1676939
Kolstad, E. W., and Bracegirdle, T. J. (2017). Sensitivity of an apparently hurricane-like polar low to sea-surface temperature. Q. J. R. Meteorological Soc. 143 (703), 966–973. doi:10.1002/qj.2980
Køltzow, M., Schyberg, H., Støylen, E., and Yang, X. (2022). Value of the Copernicus Arctic Regional Reanalysis (CARRA) in representing near-surface temperature and wind speed in the north-east European Arctic. Polar Res. 41, 8002. doi:10.33265/polar.v41.8002
Krinitskiy, M., Verezemskaya, P., Grashchenkov, K., Tilinina, N., Gulev, S., and Lazzara, M. (2018). Deep convolutional neural networks capabilities for binary classification of polar mesocyclones in satellite mosaics. Atmosphere 9 (11), 426. doi:10.3390/atmos9110426
Kudryavtsev, V., Cheshm Siyahi, V., Yurovskaya, M., and Chapron, B. (2022). On surface waves in Arctic Seas. Boundary-Layer Meteorol. 187 (1-2), 267–294. doi:10.1007/s10546-022-00768-9
Lackner, C. P., Geerts, B., Wang, Y., Juliano, T. W., Xue, L., Kosović, B., et al. (2023). Insights into the relation between vertical cloud structure and dynamics of three polar lows: observations from COMBLE. Q. J. R. Meteorological Soc. 149, 2992–3013. doi:10.1002/qj.4543
Landgren, O. A., Seierstad, I. A., and Iversen, T. (2019). Projected future changes in marine cold-air outbreaks associated with polar lows in the northern North-Atlantic Ocean. Clim. Dyn. 53 (5), 2573–2585. doi:10.1007/s00382-019-04642-2
Mallet, P.-E., Claud, C., Cassou, C., Noer, G., and Kodera, K. (2013). Polar lows over the Nordic and Labrador Seas: synoptic circulation patterns and associations with North Atlantic-Europe wintertime weather regimes. J. Geophys. Res. Atmos. 118 (6), 2455–2472. doi:10.1002/jgrd.50246
Mansfield, D. A. (1974). Polar lows: the development of baroclinic disturbances in cold air outbreaks. Q. J. R. Meteorological Soc. 100 (426), 541–554. doi:10.1002/qj.49710042604
Meyer, M., Polkova, I., Modali, K. R., Schaffer, L., Baehr, J., Olbrich, S., et al. (2021). Interactive 3-D visual analysis of ERA5 data: improving diagnostic indices for marine cold air outbreaks and polar lows. Weather Clim. Dyn. 2 (3), 867–891. doi:10.5194/wcd-2-867-2021
Moore, G. W. K., Våge, K., Renfrew, I. A., and Pickart, R. S. (2022). Sea-ice retreat suggests re-organization of water mass transformation in the Nordic and Barents Seas. Nat. Commun. 13 (1), 67. doi:10.1038/s41467-021-27641-6
Moreno-Ibáñez, M., Laprise, R., and Gachon, P. (2021). Recent advances in polar low research: current knowledge, challenges and future perspectives. Tellus A Dyn. Meteorology Oceanogr. 73 (1), 1890412–1890431. doi:10.1080/16000870.2021.1890412
Moreno-Ibáñez, M., Laprise, R., and Gachon, P. (2023a). Analysis of the development mechanisms of a polar low over the Norwegian Sea simulated with the Canadian Regional Climate Model. Atmosphere 14 (6), 998. doi:10.3390/atmos14060998
Moreno-Ibáñez, M., Laprise, R., and Gachon, P. (2023b). Assessment of simulations of a polar low with the Canadian Regional Climate Model. PLOS ONE 18 (10), e0292250. doi:10.1371/journal.pone.0292250
Noer, G., Saetra, Ø., Lien, T., and Gusdal, Y. (2011). A climatological study of polar lows in the Nordic Seas. Q. J. R. Meteorological Soc. 137 (660), 1762–1772. doi:10.1002/qj.846
Purich, A., and Doddridge, E. W. (2023). Record low Antarctic sea ice coverage indicates a new sea ice state. Commun. Earth Environ. 4 (1), 314. doi:10.1038/s43247-023-00961-9
Rasmussen, E. (1979). The polar low as an extratropical CISK disturbance. Q. J. R. Meteorological Soc. 105 (445), 531–549. doi:10.1002/qj.49710544504
Renfrew, I. A., Barrell, C., Elvidge, A. D., Brooke, J. K., Duscha, C., King, J. C., et al. (2021). An evaluation of surface meteorology and fluxes over the Iceland and Greenland Seas in ERA5 reanalysis: the impact of sea ice distribution. Q. J. R. Meteorological Soc. 147 (734), 691–712. doi:10.1002/qj.3941
Renfrew, I. A., Huang, J., Semper, S., Barrell, C., Terpstra, A., Pickart, R. S., et al. (2023). Coupled atmosphere–ocean observations of a cold-air outbreak and its impact on the Iceland Sea. Q. J. R. Meteorological Soc. 149 (751), 472–493. doi:10.1002/qj.4418
Renfrew, I. A., Pickart, R. S., Våge, K., Moore, G. W. K., Bracegirdle, T. J., Elvidge, A. D., et al. (2019). The Iceland Greenland seas project. Bull. Am. Meteorological Soc. 100 (9), 1795–1817. doi:10.1175/bams-d-18-0217.1
Revokatova, A., Nikitin, M., Rivin, G., Rozinkina, I., Nikitin, A., and Tatarinovich, E. (2021). High-resolution simulation of polar lows over Norwegian and Barents Seas using the COSMO-CLM and ICON models for the 2019–2020 cold season. Atmosphere 12 (2), 137. doi:10.3390/atmos12020137
Schultz, M. G., Betancourt, C., Gong, B., Kleinert, F., Langguth, M., Leufen, L. H., et al. (2021). Can deep learning beat numerical weather prediction? Philosophical Trans. R. Soc. A Math. Phys. Eng. Sci. 379 (2194), 20200097. doi:10.1098/rsta.2020.0097
Smith, G. C., Bélanger, J.-M., Roy, F., Pellerin, P., Ritchie, H., Onu, K., et al. (2018). Impact of coupling with an ice–ocean model on global medium-range NWP forecast skill. Mon. Weather Rev. 146 (4), 1157–1180. doi:10.1175/MWR-D-17-0157.1
Spengler, T., Claud, C., and Heinemann, G. (2017). Polar low workshop summary. Bull. Am. Meteorological Soc. 98 (6), ES139–ES142. doi:10.1175/bams-d-16-0207.1
Spensberger, C., and Spengler, T. (2021). Sensitivity of air-sea heat exchange in cold-air outbreaks to model resolution and sea-ice distribution. J. Geophys. Res. Atmos. 126 (5), e2020JD033610. doi:10.1029/2020JD033610
Stoll, P. J. (2022). A global climatology of polar lows investigated for local differences and wind-shear environments. Weather Clim. Dyn. 3 (2), 483–504. doi:10.5194/wcd-3-483-2022
Stoll, P. J., Graversen, R. G., and Messori, G. (2023). The global atmospheric energy transport analysed by a wavelength-based scale separation. Weather Clim. Dyn. 4 (1), 1–17. doi:10.5194/wcd-4-1-2023
Stoll, P. J., Graversen, R. G., Noer, G., and Hodges, K. (2018). An objective global climatology of polar lows based on reanalysis data. Q. J. R. Meteorological Soc. 144 (716), 2099–2117. doi:10.1002/qj.3309
Stoll, P. J., Spengler, T., Terpstra, A., and Graversen, R. G. (2021). Polar lows – moist-baroclinic cyclones developing in four different vertical wind shear environments. Weather Clim. Dyn. 2 (1), 19–36. doi:10.5194/wcd-2-19-2021
Terpstra, A., Renfrew, I. A., and Sergeev, D. E. (2021). Characteristics of cold-air outbreak events and associated polar mesoscale cyclogenesis over the North Atlantic region. J. Clim. 34 (11), 1–52. doi:10.1175/jcli-d-20-0595.1
Terpstra, A., Spengler, T., and Moore, R. W. (2015). Idealised simulations of polar low development in an Arctic moist-baroclinic environment. Q. J. R. Meteorological Soc. 141 (691), 1987–1996. doi:10.1002/qj.2507
Tollinger, M., Graversen, R., and Johnsen, H. (2021). High-resolution polar low winds obtained from unsupervised SAR wind retrieval. Remote Sens. 13 (22), 4655. doi:10.3390/rs13224655
Turner, J., Rasmussen, E. A., and Carleton, A. M. (2003). “Introduction,” in Polar lows: mesoscale weather systems in the polar regions. Editors E. A. Rasmussen, and J. Turner (Cambridge: Cambridge University Press), 1–51.
Walters, D. N., Best, M. J., Bushell, A. C., Copsey, D., Edwards, J. M., Falloon, P. D., et al. (2011). The Met Office unified model global atmosphere 3.0/3.1 and JULES global land 3.0/3.1 configurations. Geosci. Model Dev. 4 (4), 919–941. doi:10.5194/gmd-4-919-2011
Wang, Z., Boyd, K., and Walsh, J. E. (2023). Modulation of polar low activity by the Madden-Julian Oscillation. Geophys. Res. Lett. 50 (12), e2023GL103719. doi:10.1029/2023GL103719
Watanabe, S.-i.I., Niino, H., and Spengler, T. (2022). Formation of maritime convergence zones within cold air outbreaks due to the shape of the coastline or sea ice edge. Q. J. R. Meteorological Soc. 148 (746), 2546–2562. doi:10.1002/qj.4324
Wessel, P., and Smith, W. H. F. (1996). A global, self-consistent, hierarchical, high-resolution shoreline database. J. Geophys. Res. Solid Earth 101 (B4), 8741–8743. doi:10.1029/96JB00104
Williams, K. D., Harris, C. M., Bodas-Salcedo, A., Camp, J., Comer, R. E., Copsey, D., et al. (2015). The Met Office global coupled model 2.0 (GC2) configuration. Geosci. Model Dev. 8 (5), 1509–1524. doi:10.5194/gmd-8-1509-2015
Wu, L. (2021). Effect of atmosphere-wave-ocean/ice interactions on a polar low simulation over the Barents Sea. Atmos. Res. 248, 105183. doi:10.1016/j.atmosres.2020.105183
Xue, J., Bromwich, D. H., Xiao, Z., and Bai, L. (2021). Impacts of initial conditions and model configuration on simulations of polar lows near Svalbard using Polar WRF with 3DVAR. Q. J. R. Meteorological Soc. 147 (740), 3806–3834. doi:10.1002/qj.4158
Yan, Z., Wang, Z., Peng, M., and Ge, X. (2023). Polar low motion and track characteristics over the North Atlantic. J. Clim. 36 (13), 4559–4569. doi:10.1175/JCLI-D-22-0547.1
Yang, X., Schyberg, H., Palmason, B., Bojarova, J., Box, J., Pagh Nielsen, K., et al. (2020). C3S Arctic regional reanalysis—full system documentation. Reading, UK: Copernicus Climate Change Service.
Keywords: polar low, mesoscale cyclone, cold air outbreak, Arctic, Antarctic, severe weather, baroclinic instability
Citation: Moreno-Ibáñez M (2024) Polar low research: recent developments and promising courses of research. Front. Earth Sci. 12:1368179. doi: 10.3389/feart.2024.1368179
Received: 10 January 2024; Accepted: 20 February 2024;
Published: 06 March 2024.
Edited by:
Bin Yu, Environment and Climate Change Canada (ECCC), CanadaReviewed by:
Thomas Ballinger, University of Alaska Fairbanks, United StatesCopyright © 2024 Moreno-Ibáñez. This is an open-access article distributed under the terms of the Creative Commons Attribution License (CC BY). The use, distribution or reproduction in other forums is permitted, provided the original author(s) and the copyright owner(s) are credited and that the original publication in this journal is cited, in accordance with accepted academic practice. No use, distribution or reproduction is permitted which does not comply with these terms.
*Correspondence: Marta Moreno-Ibáñez, bWFydGEubW9yZW5vaWJhbmV6QGNvbG9yYWRvLmVkdQ==