- BEACON Center, Michigan State University, East Lansing, MI, United States
Evolutionary transitions occur when previously-independent replicating entities unite to form more complex individuals. Such transitions have profoundly shaped natural evolutionary history and occur in two forms: fraternal transitions involve lower-level entities that are kin (e.g., transitions to multicellularity or to eusocial colonies), while egalitarian transitions involve unrelated individuals (e.g., the origins of mitochondria). The necessary conditions and evolutionary mechanisms for these transitions to arise continue to be fruitful targets of scientific interest. Here, we examine a range of fraternal transitions in populations of open-ended self-replicating computer programs. These digital cells were allowed to form and replicate kin groups by selectively adjoining or expelling daughter cells. The capability to recognize kin-group membership enabled preferential communication and cooperation between cells. We repeatedly observed group-level traits that are characteristic of a fraternal transition. These included reproductive division of labor, resource sharing within kin groups, resource investment in offspring groups, asymmetrical behaviors mediated by messaging, morphological patterning, and adaptive apoptosis. We report eight case studies from replicates where transitions occurred and explore the diverse range of adaptive evolved multicellular strategies.
1. Introduction
An evolutionary transition in individuality is an event where independently replicating entities unite to replicate as a single, higher-level individual (Smith and Szathmary, 1997). These transitions are understood as essential to natural history's remarkable record of complexification and diversification (Smith and Szathmary, 1997). Likewise, artificial life researchers have highlighted transitions in individuality as a mechanism that is missing in digital systems, but necessary for achieving the evolution of complexity and diversity that we witness in nature (Banzhaf et al., 2016; Taylor et al., 2016).
Fraternal evolutionary transitions in individuality are transitions in which the higher-level replicating entity is derived from the combination of cooperating kin that have entwined their long-term fates (West et al., 2015). Multicellular organisms and eusocial insect colonies exemplify this phenomenon (Smith and Szathmary, 1997) given that both are sustained and propagated through the cooperation of lower-level kin. This work focuses on fraternal transitions. Although not our focus here, egalitarian transitions—events in which non-kin unite, such as the genesis of mitochondria by symbiosis of free-living prokaryotes and eukaryotes (Smith and Szathmary, 1997)—also constitute essential episodes in natural history.
In nature, major fraternal transitions occur sporadically with few extant transitional forms, making them challenging to study. For instance, on the order of 25 independent origins of Eukaryotic multicellularity are known (Grosberg and Strathmann, 2007) with most transitions having occurred hundreds of millions of years ago (Libby and Ratcliff, 2014). Recent work in experimental evolution (Koschwanez et al., 2013; Ratcliff and Travisano, 2014; Ratcliff et al., 2015; Gulli et al., 2019), mechanistic modeling (Hanschen et al., 2015; Staps et al., 2019), and digital evolution (Goldsby et al., 2012, 2014) complements traditional post hoc approaches focused on characterizing the record of natural history. These systems each instantiate the evolutionary transition process, allowing targeted manipulations to test hypotheses about the requisites, mechanisms, and evolutionary consequences of fraternal transitions. Digital evolution, computational model systems designed to instantiate evolution in abstract algorithmic substrates rather than directly emulating any specific biological system (Wilke and Adami, 2002; Dolson and Ofria, 2021), occupies a sort of middle ground between wet work and mechanistic modeling. This approach offers a unique conjunction of experimental capabilities that complements work in both of those disciplines. Like modeling, digital evolution affords rapid generational turnover, complete observability (every event in a digital system can be tracked), and complete manipulability (every event in a digital system can be arbitrarily altered). However, as with in vivo experimental evolution, digital evolution systems can exhibit rich evolutionary dynamics stemming from complex, rugged fitness landscapes (LaBar and Adami, 2017) and sophisticated agent behaviors (Grabowski et al., 2013).
Our work here follows closely in the intellectual vein of Goldsby's deme-based digital evolution experiments (Goldsby et al., 2012, 2014). In her studies, high-level organisms exist as a group of cells within a segregated, fixed-size subspace. High-level organisms that must compete for a limited number of subspace slots. Individual cells that comprise an organism are controlled by heritable computer programs that allow them to self-replicate, interact with their environment, and communicate with neighboring cells.
Goldsby's work defines two modes of cellular reproduction: tissue accretion and offspring generation. In this way, somatic and gametogenic modes of reproduction are explicitly differentiated. Within a group, cells undergo tissue accretion, whereby a cell copies itself into a neighboring position in its subspace. In the latter, a population slot is cleared to make space for a daughter organism then seeded with a single daughter cell from the parent organism.
Goldsby's model abstracts away developmental cost to focus on resource competition between groups. Cells grow freely within an organism, but fecundity depends on the collective profile of computational tasks (usually mathematical functions) performed within the organism. When an organism accumulates sufficient resource, a randomly chosen subspace is cleared and a single cell from the replicating organism is used as a propagule to seed the new organism. This setup mirrors the dynamics of biological multicellularity, in which cell proliferation may either grow an existing multicellular body or found a new multicellular organism.
Here, we take several steps to develop a computational environment that removes the enforcement and rigid regulation of multiple organismal levels. Specifically, we remove the explicitly segregated subspaces and we let multicells interact with each other more freely. We demonstrate the emergence of multicellularity where each organism manages its own spatial distribution and reproductive process. This spatially unified approach enables more nuanced interactions among organisms, albeit at the cost of substantially more complicated analyses. Instead of a single explicit interface to mediate interactions among high-level organisms, such interactions must emerge via many cell-cell interfaces. Novelty can occur in terms of interactions among competitors, among organism-level kin, or even within the building blocks that make up hierarchical individuality. Experimentally studying fraternal transitions in a digital system where key processes (reproductive, developmental, homeostatic, and social) occur implicitly within a unified framework can provide unique insights into nature. For example, pervasive, arbitrary interactions between multicells introduces the possibility for strong influence of biotic selection.
However, in our system, multicells do not emerge from an entirely impartial substrate. We do explicitly provide some framework to facilitate fraternal transitions in individuality by allowing cells to readily designate distinct hereditary groups. Offspring cells may either remain part of their parent's hereditary group or found a new group. Cells can recognize group members, thus allowing targeted communication and resource sharing with kin. We reward cells for performing tasks designed to require passive collaboration among hereditary group members. As such, cells that form hereditary groups to maximize advantage on those tasks stand to increase their inclusive fitness. In previous work introducing the DISHTINY (DIStributed Hierarchical Transitions in IndividualitY) framework we evolved parameters for manually designed cell-level strategies to explore fraternal transitions in individuality (Moreno and Ofria, 2019). In this work we extend DISHTINY to incorporate a more dynamic event-driven genetic programming representation called SignalGP, which was designed to facilitate dynamic interactions among agents and between agents and their environment (Lalejini and Ofria, 2018). As expected, with the addition of cell controllers capable of nearly arbitrary computation we see a far more diverse set of behaviors and strategies arise.
Here, we perform case studies to characterize notable multicellular phenotypes that evolved via this more dynamic genetic programming underpinning. Each case study strain was chosen by screening the entire set of replicate evolutionary runs for signs of the trait under investigation and then manually the most promising strain(s) for further investigation. Case studies presented therefore represent an anecdotal sampling, rather than an exhaustive summary, with respect to each trait of interest. Our goal is to explore a breadth of possible evolutionary outcomes under the DISHTINY framework. We see this as a precursory step toward hypothesis-driven work contributing to open questions about fraternal transitions in individuality.
2. Materials and Methods
We performed simulations in which cells evolved open-ended behaviors to make decisions about resource sharing, reproductive timing, and apoptosis. We will first describe the environment and hereditary grouping system cells evolved under and then describe the behavior-control system cells used.
2.1. Cells and Hereditary Groups
Cells occupy individual tiles on a 60-by-60 toroidal grid. Over discrete time steps (“updates”), cells can collect a resource. Collected resource decays at a rate of 0.1% per update, incentivizing its quick use but gradual enough so as to not prevent the most naive cells from eventually accumulating enough resource to reproduce. Once sufficient resource accrues, cells may pay one unit of resource to place a daughter cell on an adjoining tile of the toroidal grid (i.e., reproduce), replacing any existing cell already there. Daughter cells inherit their parent's genetic program, except any novel mutations that may arise. Mutations included whole-function duplication and deletion, bit flips on tags for instructions and functions, instruction and argument substitutions, and slip mutation of instruction sequences. We used standard SignalGP mutation parameters from Lalejini and Ofria (2018), but only applied mutations to 1% of daughter cells at birth. Daughter cells may also inherit hereditary group ID, introduced and discussed below.
Cells accrue resource via a cooperative resource-collection process. The simulation distributes large amounts of resource within certain spatial bounds in discrete, intermittent events. Working in a group allows cells to more fully collect available resource during these events. Cooperating in medium-sized groups (on the order of 100 cells) accelerates per-cell resource collection rate. Unicellular, too-small, or too-large groups collect resource at a lesser per-cell rate. As an arbitrary side effect of the simulation algorithm employed to instantiate the cooperative resource distribution process, groups with a roughly circular layout collect resource faster than irregularly-shaped groups. Cooperative resource collection unfolds as an entirely passive process on the part of the cells, influenced only by a group's spatial layout. Full details on the simulation algorithm that determines cooperative resource collection rates appear in Supplementary Section 6.2.
Cells may grow a cooperative resource-collecting group through cell proliferation. We refer to these cooperative, resource-collecting groups as “hereditary groups.” As cells reproduce, they can choose to adsorb daughter cells onto the parent's hereditary group or expel those offspring to found a new hereditary group. These decisions affect the spatial layout of these hereditary groups and, in turn, affect individual cells' resource-collection rate.
To promote group turnover, we counteract the established hereditary groups' advantage with a simple aging scheme. As hereditary groups age over elapsed updates and somatic generations, their constituent cells lose the ability to regenerate somatic tissue and then, soon after, to collect resource. A complete description of group aging mechanisms used appears in Supplementary Section 6.3.
Because new hereditary group IDs arise first in a single cell and grow disseminate exclusively among direct descendants of that progenitor cell, hereditary groups are reproductively bottlenecked. This clonal (or “staying together”) multicellular life history stands in contrast with an aggregative (or “coming together”) life cycle where chimeric groups arise via fusion of potentially loosely-related lineages (Staps et al., 2019). Such clonal development is known to strengthen between-organism selection effects (Grosberg and Strathmann, 2007).
In this work, we screen for fraternal transitions in individuality with respect to these hereditary groups by evaluating three characteristic traits of higher-level organisms: resource sharing, reproductive division of labor, and apoptosis. We can further screen for the evolution of complex multicellularity by assessing cell-cell messaging, regulatory patterning, and functional differentiation between cells within hereditary groups (Knoll, 2011).
2.2. Hierarchical Nesting of Hereditary Groups
Successive fraternal transitions in natural history—for example, to multicellularity and then to eusociality (Smith and Szathmary, 1997)—underscores the constructive power of evolution to harness emergent structures as building blocks for further novelty. Such substructure can also provide scaffolding for differentiation and division of labor within an organism (Wilson, 1984). To explore these dynamics, in some experimental conditions we incorporated a hierarchical extension to the hereditary grouping scheme described above.
Hierarchical levels are introduced into the system by providing a mechanism to groups of hereditary groups to form. We accomplish this through two separate, but overlaid, instantiations of the hereditary grouping scheme. We refer to each independent hereditary grouping system as a “level.” The hierarchical extension allows two levels of hereditary grouping, identified here as L0 and L1. L0 instantiates smaller, inner grouping embedded inside of a L1 grouping. Without the hierarchical extension, only L0 is present1. We refer to the highest hereditary grouping level present in a simulation as the “apex” level.
Under the hierarchical extension, each cell contained a pair of separate hereditary group IDs—the first for L0 and the second for L1. During reproduction, daughter cells could either
1. Inherit both L0 and L1 hereditary group ID,
2. Inherit L0 hereditary group ID but not L1 hereditary group ID, or
3. Inherit neither hereditary group ID.
In order to enforce hierarchical nesting of hereditary group IDs, daughter cells could not inherit just the L1 hereditary group ID.
Hierarchical hereditary group IDs are strictly nested: all cells are members of one L0 hereditary group and L1 hereditary group. No cell can be a member of two L0 hereditary groups or two L1 hereditary groups. Likewise, no L0 hereditary group can appear within more than one L1 hereditary group. Useful as a concrete illustration of this scheme, Figure 6A depicts hierarchically-nested hereditary groupings assumed by an evolved strain.
2.3. Cell-Level Organisms
Our experiments use cell-level digital organisms controlled by genetic programs subject to mutations and selective pressures that stem from local competition for limited space.
We employ the SignalGP event-driven genetic programming representation. As sketched in Figure 1A, this representation is specially designed to express function-like modules of code in response to internal signals or external stimuli. This process can be considered somewhat akin to gene expression. In our experiments, virtual CPUs can execute responses to up to 24 signals at once, with any further signals usurping the longest-running modules. The event-driven framework facilitates the evolution of dynamic interactions between digital organisms and their environment (including other organisms) (Lalejini and Ofria, 2018).
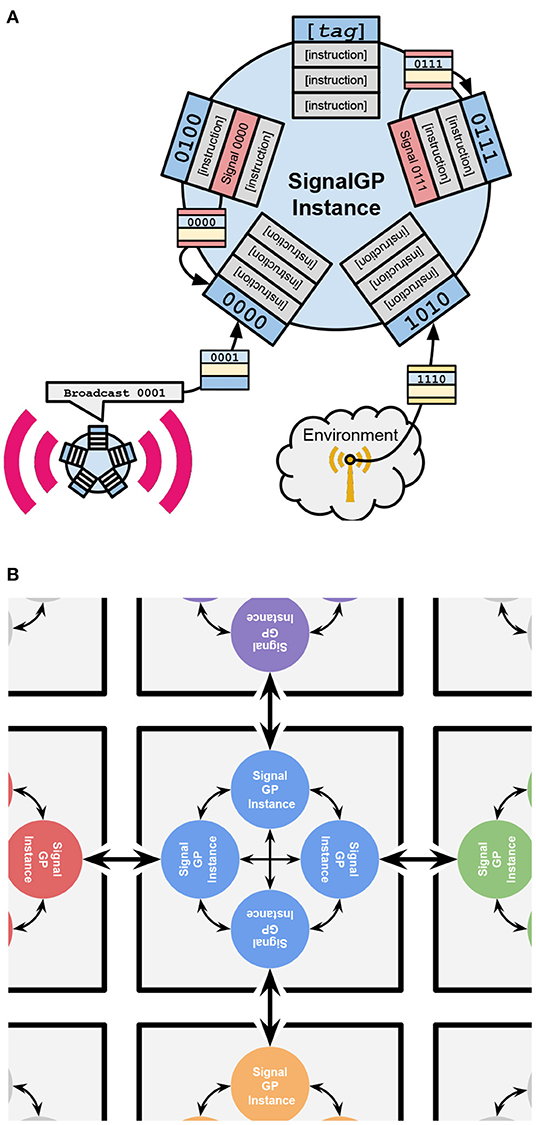
Figure 1. Schematic illustrations of how an individual SignalGP instance functions and how SignalGP instances control DISHTINY cells. Execution of cells' genetic programs on SignalGP instances controls cell behavior in our model. (A) provided courtesy Alexander Lalejini. (A) Overview of a single SignalGP instance. SignalGP program modules contain ordered sets of instructions that activate and execute independently in response to tagged signals. Above, these modules are shown as rectangular lists with bitstring tags protruding from the SignalGP instance. These signals can originate from any of three sources: (1) internally from execution of “Signal” instructions within a program's modules, (2) from the outside environment, (3) or from other agents executing “Message” instructions. (B) How individual SignalGP instances are organized into DISHTINY cells. Above, DISHTINY cells are depicted as gray squares. Each DISHTINY cell is controlled by independent execution of the cell's genetic program on four distinct SignalGP instances, depicted as colored circles. Each of four independent instances manages cell behavior with respect to a single cardinal direction: sensing environmental state, receiving intercellular messages, and determining cell actions. Above, the special role of each instance is depicted as a reciporical arrow to the neighboring instance in the neighboring cell. (All four instances sense non-directional environmental cues and non-directional actions may be taken by any instance.) These four instances can communicate with one another via intracellular messaging, indicated above by smaller reciprocal arrows among instances within a cell.
Special module components allow evolving programs to sense and interact with their environment, through mechanisms including resource sharing, hereditary group sensing, apoptosis, cell reproduction, and arbitrary cell-cell messaging. Modules can also include general purpose computational elements like conditionals and loops, which allows cells to evolve sophisticated behaviors conditioned on current (and even previous) local conditions. A simple “regulatory” system provides special CPU instructions that dynamically adjust which modules are activated by particular signals. In our simulation, directionality of some inputs and outputs must be accounted for (e.g., specifying which neighbor to share resource with). To accomplish this, we provide each cell an independent SignalGP hardware instance to manage inputs and outputs with respect to each specific cell neighbor. So there are four virtual hardware sets per cell, one for each cardinal direction2. Figure 1B overviews the configuration of the four SignalGP instances that constitute a single cell.
Supplementary Sections 6.4–6.7 provide full details of the digital evolution substrate underpinning this work.
2.4. Surveyed Evolutionary Conditions
To broaden our exploration of possible evolved multicellular behaviors in this system, we surveyed several evolutionary conditions.
In one manipulation, we explored the effect of enabling hierarchical structure within hereditary groups, such that parent cells can choose to keep offspring in their same sub-group, in just the same full group, or expel them entirely to start a new group. Cells can sense and react to the level of hereditary ID commonality shared with each neighbor. This manipulation presents opportunity for hierarchical individuality or for a mechanism to mediate differentiation within a multicell, but does not enforce it.
In a second manipulation, we explored the importance of explicitly selecting for medium-sized groups (as had been needed to maximize resource collection) by removing this incentive. Instead, the system distributed resource at a uniform per-cell rate.
We combined these two manipulations to yield four surveyed conditions:
1. “Flat-Even”: One hereditary group level (flat) with uniform resource inflow (even). In-browser simulation: https://hopth.ru/i,
2. “Flat-Wave”: One hereditary group level (flat) with group-mediated resource collection (wave); In-browser simulation: https://hopth.ru/j),
3. “Nested-Even”: Two hierarchically-nested hereditary group levels (nested) with uniform resource inflow (even). In-browser simulation: https://hopth.ru/k,
4. “Nested-Wave”: Two hierarchically-nested hereditary group levels (nested) with group-mediated resource collection (wave). In-browser simulation: https://hopth.ru/l.
Supplementary Section 6.8 provides full details for each of the four surveyed evolutionary conditions.
For each condition, we simulated 40 replicate populations for up to 1,048,576 (220) updates. During this time, on the order of 4,000 cellular generations and 500 apex-level group generations elapsed in runs (Full details appear in Supplementary Table 2). Due to variability in simulation speed, four replicates only completed 262,144 updates. All analyses involving inter-replicate comparisons were therefore performed at this earlier time point.
3. Results
To characterize the general selective pressures induced by surveyed environmental conditions, we assessed the prevalence of characteristic multicellular traits among evolved genotypes across replicates. In the case of an evolutionary transition of individuality, we would expect cells to modulate their own reproductive behavior to prioritize group interests above individual cell interests. In DISHTINY, cell reproduction inherently destroys an immediate neighbor cell. As such, we would expect somatic growth to occur primarily at group peripheries in a higher-level individual. Supplementary Figure 1 compares cellular reproduction rates between the interior and exterior of apex-level hereditary groups. For all treatments, phenotypes with depressed interior cellular reproduction rates dominated across replicates (non-overlapping 95% CI). By update 262,144 (about 1,000 cellular generations; see Supplementary Table 2), all four treatment conditions appear to select for some level of reproductive cooperation among cells.
Across replicate evolutionary runs in all four treatments, we also found that resource was transferred among registered kin at a significantly higher mean rate than to unrelated neighbors (non-overlapping 95% CI). Genetic programs controlling cells can sense whether any particular neighbor shares a common hereditary group ID. Thus, selective activation of resource sharing behavior to hereditary group members might have evolved, which would provide one possible explanation for this observation3. However, cells are also capable of conditioning behavior on whether a particular neighbor is direct kin (i.e., a parent or child). To test whether this resource-sharing was solely an artifact of sharing between direct cellular kin, we also assessed mean sharing to registered kin that were not immediate cellular relatives. Mean sharing between such cells also exceeded sharing among unrelated neighbors (non-overlapping 95% CI). Thus, all four treatments appear to select for functional cooperation among wider kin groups. Supplementary Section 6.12 presents these results in detail.
3.1. Qualitative Life Histories
Although cooperative cell-level phenotypes were common among evolved hereditary groups, across replicates functional and reproductive cooperation arose via diverse qualitative life histories. To provide a general sense for the types of life histories we observed in this system, Figure 2 shows time lapses of representative multicellular groups evolved in different replicates. Figure 2A depicts an example of a naive life history in which—beyond the cellular progenitor of a propagule group—the parent and propagule groups exhibit no special cooperative relationship. In Figure 2B, propagules repeatedly bud off of parent groups to yield a larger network of persistent parent-child cooperators. In Figure 2C, propagules are generated at the extremities of parent groups and then rapidly replace most or all of the parent group. Finally, in Figure 2D, propagules are generated at the interior of a parent group and replace it from the inside out.
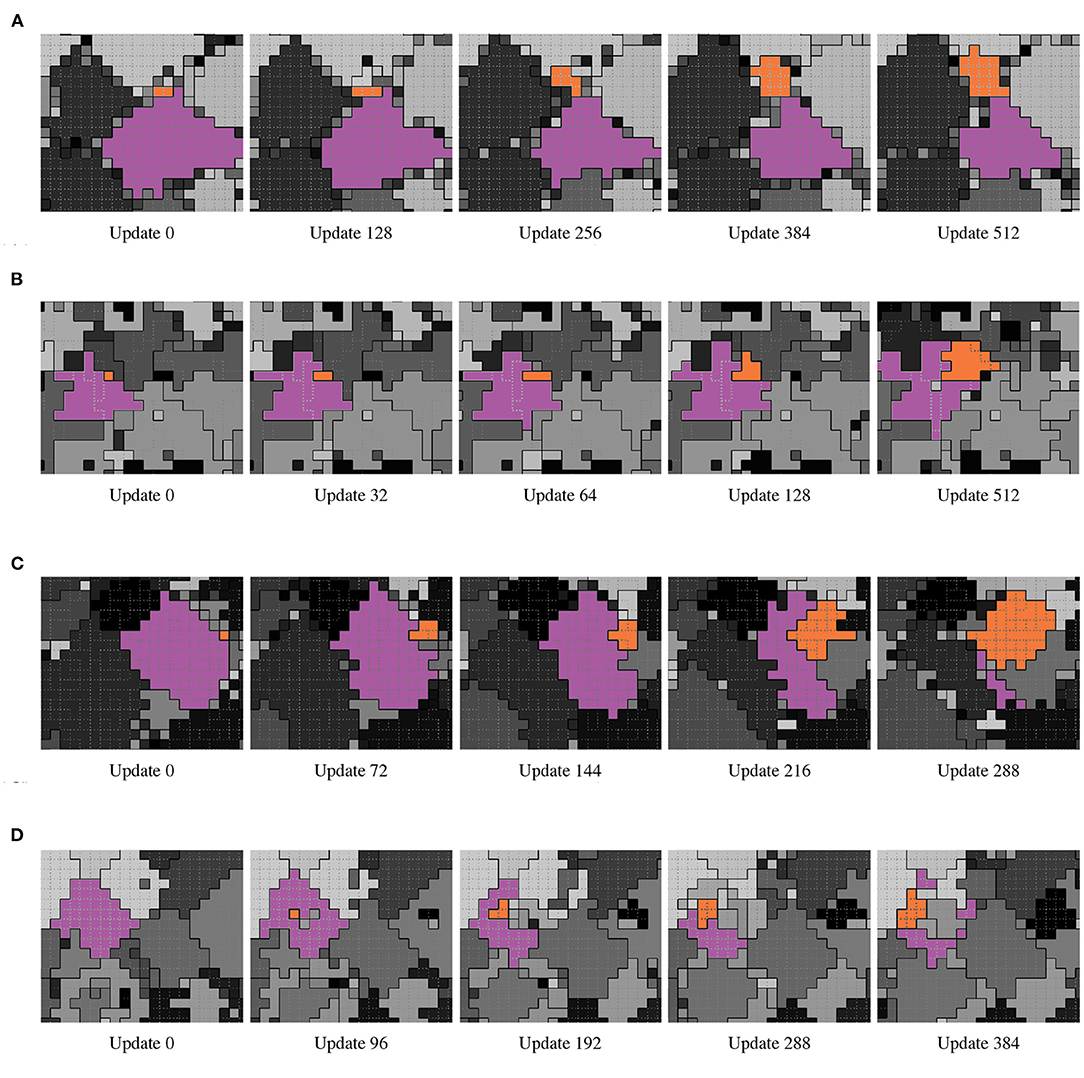
Figure 2. Time lapse examples of qualitative life histories evolved under the Nested-Wave treatment. From left to right within each row, frames depict the progression of simulation state within a subset of the simulation grid. L1 hereditary groups are by differentiated by grayscale tone and separated by solid black borders. L0 hereditary groups are by separated by dashed gray borders. In each example, the focal parent L1 group is colored purple and the focal offspring group orange. (A) Naive (animation: https://hopth.ru/x, in-browser simulation: https://hopth.ru/1). The offspring group is birthed at the exterior of the parent group. Parent and offspring groups then compete with each other for space just the same as they do with other groups. (B) Adjoin (animation: https://hopth.ru/y, in-browser simulation: https://hopth.ru/2). The offspring group begins as a single cell at the exterior of the parent group. Parent and offspring groups then exclusively expend reproductive effort to compete with other groups. This results in a stable interface between the parent and offspring groups as the offspring group grows over time. (C) Sweep (animation: https://hopth.ru/z, in-browser simulation: https://hopth.ru/3). The offspring group begins as a single cell at the exterior of the parent group. The offspring group then grows rapidly into the parent group, resulting in a near-complete transfer of simulation space into the offspring group. Multiple offspring groups may simultaneously grow over the parent, as is the case here. (D) Burst (animation: https://hopth.ru/0, in-browser simulation: https://hopth.ru/4). The offspring group begins as a single cell at the interior of the parent group. Over time, the offspring group grows over the parent group from the inside out. Multiple offspring groups may develop simultaneously, as is the case here.
To better understand the multicellular strategies that evolved in this system, we investigated the mechanisms and adaptiveness of notable phenotypes that evolved in several individual evolutionary replicates. In the following sections, we present these investigations as a series of case studies.
3.2. Case Study: Burst Lifecycle
We wondered how the strain exhibiting the “burst” lifecycle in Figure 2D determined when and where to originate its propagules. To assess whether gene regulation instructions played a role in this process, we prepared two knockout strains. In the first, gene regulation instructions were replaced with no-operation (Nop) instructions (so that gene regulation state would remain baseline). In the second, the reproduction instructions to spawn a propagule were replaced with Nop instructions. Figure 3A depicts the gene regulation phenotypes of these strains.
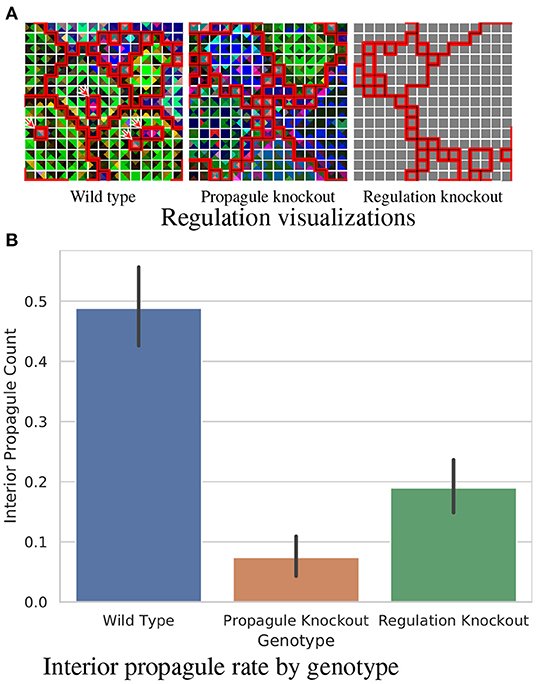
Figure 3. Analysis of a wild type strain exhibiting a “burst” lifecycle evolved under the “Nested-Wave” treatment exhibiting interior propagule generation. (A) compares gene regulation between analyzed strains. Group layouts are overlaid via borders between cells. Black borders divide L1 groups and white borders divide L0 groups. Borders between L1 groups are underlined in red for greater visibility. Within these group layouts, regulation state for each cell's four directional SignalGP instances is color coded using a PCA mapping from regulatory state to three-dimensional RGB coordinates. (The PCA mapping is calculated uniquely for each L1 hereditary group.) Within a L1 hereditary group, color similarity among tile quarters indicates that the corresponding SignalGP instances exhibit similar regulatory state. However, the particular hue of a SignalGP instance has no significance. In the case of identical regulatory state (here, due to the absence of genetic regulation in a knockout strain) this color coding appears gray. Wild type interior propagules are annotated with red arrows. (B) compares the mean number of interior propagules observed per L1 hereditary group. Error bars indicate 95% confidence. View an animation of wild type gene regulation at https://hopth.ru/t. View the wild type strain in a live in-browser simulation at https://hopth.ru/g.
Figure 3B compares interior propagule generation between the strains, confirming the direct mechanistic role of gene regulation in promoting interior propagule generation (non-overlapping 95% CI).
In head-to-head match-ups, the wild type strain outcompetes both the regulation-knockout (20/20; p < 0.001; two-tailed Binomial test) and the propagule-knockout strains (20/20; p < 0.001; two-tailed Binomial test). The deficiency of the propagule-knockout strain confirms the adaptive role of interior propagule generation. Likewise, the deficiency of the regulation-knockout strain affirms the adaptive role of gene regulation in the focal wild type strain.
3.3. Case Study: Cell-Cell Messaging
We discovered adaptive cell-cell messaging in two evolved strains. Here, we discuss a strain evolved under the Flat-Wave treatment where cell-cell messaging disrupts directional and spatial uniformity of resource sharing. Supplementary Section 6.13 overviews an evolved strain where cell-cell messaging appears to intensify expression of a contextual tit-for-tat policy between hereditary groups.
Figure 4 depicts the cell-cell messaging, resource sharing, and resource stockpile phenotypes of the wild type strain side-by-side with corresponding phenotypes of a cell-cell messaging knockout strain. In the wild type strain, cell-cell messaging emanates from irregular collection of cells—in some regions, grid-like and in others more sparse—broadcasting to all neighboring cells. Resource sharing appears more widespread in the knockout strain than in the wild type. However, messaging's effects suppressing resource sharing is neither spatially nor directionally homogeneous. Relative to the knockout strain, cell-cell messaging increases variance in cardinal directionality of net resource sharing (WT: mean 0.28, S.D. 0.07, n = 54; KO: mean 0.17, S.D. 0.07, n = 69; p < 0.001, bootstrap test). Cell-cell messaging also increases variance of resource sharing density with respect to spatial quadrants demarcated by the hereditary group's spatial centroid (WT: mean 0.23, S.D. 0.07, n = 52; KO: mean 0.16, S.D. 0.08, n = 68; p < 0.001, bootstrap test). We used competition experiments to confirm the fitness advantage both of cell-cell messaging (20/20; p < 0.001; two-tailed Binomial test) and (using a separate knockout strain) resource sharing (20/20; p < 0.001; two-tailed Binomial test). The fitness advantage of irregularities sharing might stem from a corresponding increase in the fraction of cells with enough resource to reproduce stockpiled (WT: mean 0.18, S.D. 0.11, n = 54; KO: mean 0.06, S.D. 0.08, n = 69; p < 0.001, bootstrap test).
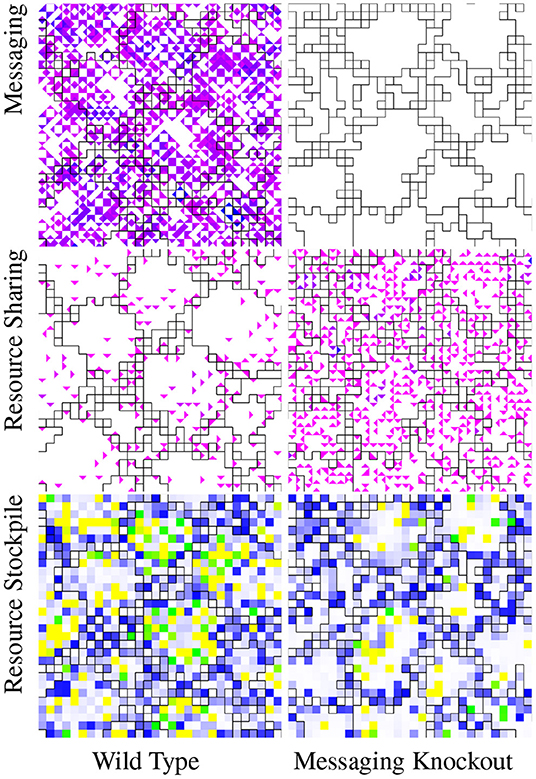
Figure 4. Visualization of phenotypic traits of a wild type strain evolved under the “Flat-Wave” treatment and corresponding intercell messaging knockout strain. For these visualizations, group layouts are overlaid via borders between cells. Black borders divide L0 hereditary groups. In the messaging visualization, color coding represents the volume of incoming messages. White represents no incoming messages and the magenta to blue gradient runs from one incoming message to the maximum observed incoming message traffic. Unlike the wild type strain, as expected the messaging knockout strain exhibits no messaging activity. In the resource sharing visualization, color coding represents the amount of incoming resource. White represents no incoming resource and the magenta to blue gradient runs from the minimum to the maximum observed incoming resource. The wild type strain exhibits much more sparse resource sharing than the messaging knockout strain. In the resource stockpile visualization, white represents zero-resource stockpiles, blue represents stockpiles with just under enough resource to reproduce, green represents stockpiles with enough resource to reproduce, and yellow represents more than enough resource to reproduce. The wild type groups contain more cells with rich resource stockpiles (green and yellow) than the messaging knockout strain. View an animation of the wild type strain at https://hopth.ru/p. View the wild type strain in a live in-browser simulation at https://hopth.ru/e.
3.4. Case Study: Gradient-Conditioned Cell Behavior
To further assess how multicellular groups process and employ spatial and directional information, we investigated whether successful multicellular strategies evolved where cells condition their behavior based on the resource concentration gradient within a multicellular group. We discovered a strain that employs a dynamic strategy where cells condition their own resource-sharing behavior based on the relative abundance of their own resource stockpiles compared to their neighbors. This strain appears to use this information to selectively suppress resource sharing. This strain's wild type outcompeted a variant where cells' capacity to assess relative richness of neighboring resource stockpiles was knocked out (20/20; p < 0.001; two-tailed Binomial test). Figure 5 contrasts the wild type resource-sharing phenotype with the more sparse knockout resource-sharing phenotype.
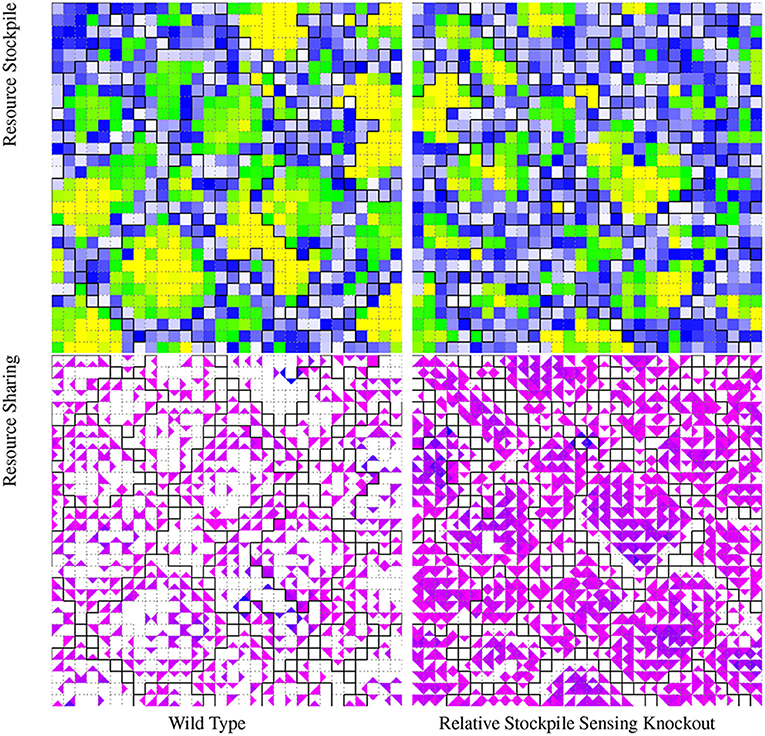
Figure 5. Visualization of phenotypic traits of a wild type strain evolved under the “Nested-Wave” treatment and corresponding resource-sensing knockout strain. For these visualizations, group layouts are overlaid via borders between cells. Black borders divide L1 hereditary groups and dashed gray borders divide L0 hereditary groups. In the resource stockpile visualization, white represents zero-resource stockpiles, blue represents stockpiles with just under enough resource to reproduce, green represents stockpiles with enough resource to reproduce, and yellow represents more than enough resource to reproduce. The wild type groups contain more cells with rich resource stockpiles (green and yellow) than the knockout strain. In the resource-sharing visualization, white represents no incoming resource and the magenta to blue gradient runs from the minimum to the maximum observed amount of incoming shared resource. The wild type strain exhibits less resource sharing than the knockout strain. View an animation of the wild type strain at https://hopth.ru/s. View the wild type strain in a live in-browser simulation at https://hopth.ru/h.
This result raises the question of whether more sophisticated morphological patterning might evolve within the experimental system. Next, in Section 3.5, we examine a strain that exhibited striking genetically driven morphological patterning of hereditary groups.
3.5. Case Study: Morphology
Figure 6A shows one of the more striking examples of genetically encoded hereditary group patterning we observed. In this strain, which arose in a Nested-Even treatment replicate, L0 hereditary groups arrange as elongated, one-cell-wide strands.
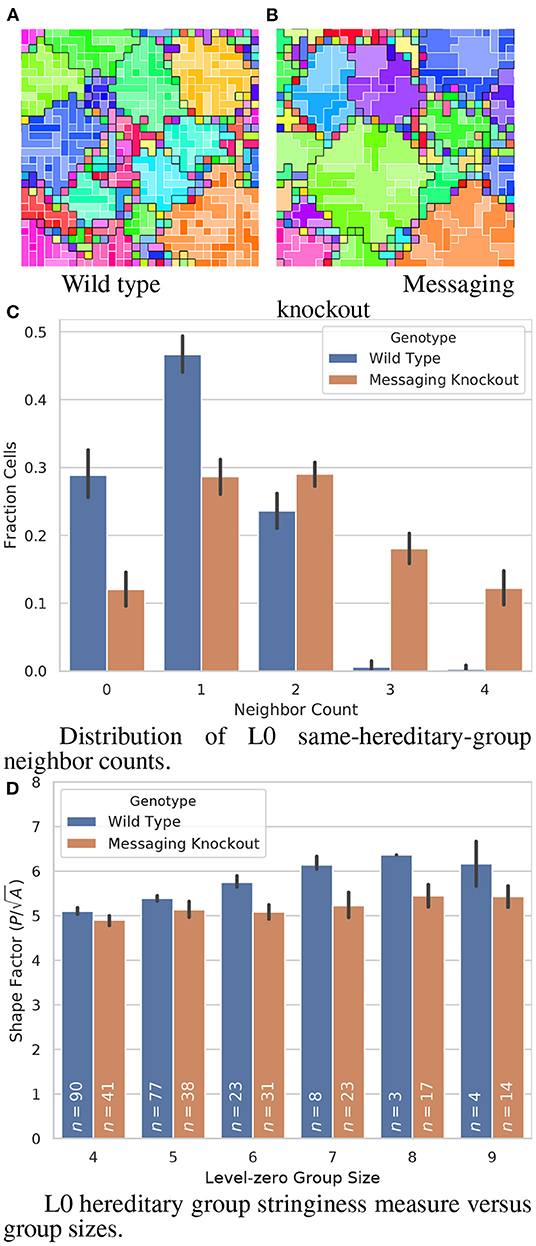
Figure 6. Comparison of a wild type strain evolved under the “Nested-Even” treatment with stringy L0 hereditary groups and the corresponding intracellular-messaging knockout strain. (A,B) visualize hereditary group layouts; color hue denotes and black borders divide L1 hereditary groups while color saturation denotes and white borders divide L0 hereditary groups. Smaller, thinner, and more elongated L0 groups can be seen in the wild type strain than in the knockout strain. (C,D) quantify the morphological effect of the intracellular-messaging knockout. In the formula for Shape Factor given in (C), P refers to group perimeter and A refers to group area. Error bars indicate 95% confidence. View an animation of the wild type strain at https://hopth.ru/q. View the wild type strain in a live in-browser simulation at https://hopth.ru/f.
Knocking out intracell messaging disrupts the stringy arrangement of L0 hereditary groups, shown in Figure 6B. Figure 6C compares the distribution of cells' L0 same-hereditary-group neighbor counts for L1 groups of nine or more cells. Compared to the knockout variant, many fewer wild-type cells are have three or four L0 same-hereditary-group neighbors, consistent with the one-cell-wide strands (non-overlapping 95% CI). However, we also observed that wild-type L0 hereditary groups were overall smaller than the knockout strain (WT: mean 2.1, S.D. 1.5; messaging knockout: mean 4.3, S.D. 5.1; p < 0.001; bootstrap test).
So, we set out to determine whether smaller L0 group size alone was sufficient to explain these observed differences in neighbor count. We compared a dimensionless shape factor describing group stringiness (perimeter divided by the square root of area) between the wild type and messaging knockout strains. Between L0 group size four (the smallest size stringiness can emerge at on a grid) and L0 group size six (the largest size we had sufficient replicate wild type observations for), wild type exhibited significantly greater stringiness (Figure 6D; 4: p < 0.01, bootstrap test; 5: p < 0.01, bootstrap test; 6: non-overlapping 95% CI). This confirms that more sophisticated patterning beyond just smaller L0 group size is at play to create the observed one-cell-wide L0 strand morphology.
Competition experiments failed to show a fitness effect of this strain's morphological patterning. The wild type strain won competitions about as often as the knockout strain (6/20). Thus, it seems this trait emerged either by drift, as the genetic background of a selective sweep, or was advantageous against a divergent competitor earlier in evolutionary history.
3.6. Case Studies: Apoptosis
Finally, we assessed whether cell self-sacrifice played a role in multicellular strategies evolved across our survey. Screening replicate evolutionary runs by apoptosis rate flagged two strains with several orders of magnitude greater activity. In strain A, evolved under the Nested-Even treatment, apoptosis accounts for 2% of cell mortality. In strain B, evolved under the Nested-Flat treatment, 15% of mortality is due to apoptosis.
To test the adaptive role of apoptosis in these strains, we performed competition experiments against apoptosis knockout strains, in which all apoptosis instructions were substituted for Nop instructions. Figure 7 compares the wild type hereditary group structures of these strains to their corresponding knockouts.
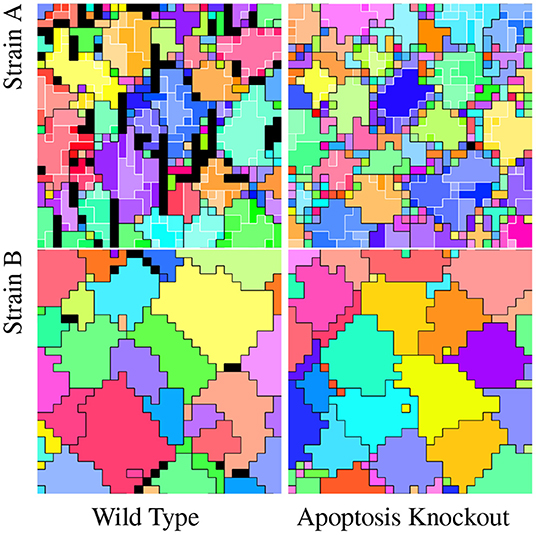
Figure 7. Comparison of wild type strains and corresponding apoptosis knockout strains. In all visualizations, color hue denotes and black borders divide apex-level hereditary groups. In Strain A visualizations, color saturation denotes and white borders divide L0 hereditary groups. (Strain B evolved under the flat treatment.) Black tiles are dead. These dead tiles, all due to apoptosis, can be seen in both strain's wild type. Dead tiles appear to be clustered contiguously or near contiguously at group peripheries in both strains, with more dead tiles apparent in Strain A than Strain B. View an animation of wild type strain A at https://hopth.ru/m. View an animation of wild type strain B at https://hopth.ru/n. View wild type strain A in a live in-browser simulation at https://hopth.ru/b. View wild type strain B in a live in-browser simulation at https://hopth.ru/c.
Apoptosis contributed significantly to fitness in both strains (strain A: 18/20, p < 0.001, two-tailed Binomial test; strain B: 20/20, p < 0.001, two-tailed Binomial test). The success of strategies incorporating cell suicide is characteristic of evolutionary conditions favoring altruism, such as kin selection or a transition from cell-level to collective individuality.
To discern whether spatial or temporal targeting of apoptosis contributed to fitness, we competed wild type strains with apoptosis-knockout strains on which we externally triggered cell apoptosis with spatially and temporally uniform probability. In one set of competition experiments, the knockout strain's apoptosis probability was based on the observed apoptosis rate of the wild type strain's monoculture. In a second set of competition experiments, the knockout strain's apoptosis probability was based on the observed apoptosis rate of the population in the evolutionary run the wild type strain was harvested from. In both sets of experiments on both strains, wild type strains outcompeted knockout strains with uniform apoptosis probabilities (strain A monoculture rate: 18/20, p < 0.001, two-tailed Binomial test; strain A population rate: 19/20, p < 0.001, two-tailed Binomial test; strain B monoculture rate: 20/20, p < 0.001, two-tailed Binomial test; strain B population rate: 20/20, p < 0.001, two-tailed Binomial test).
4. Discussion
In this work, we selected for fraternal transitions in individuality among digital organisms controlled by genetic programs. Because—unlike previous work (Goldsby et al., 2012, 2014)—we provided no experimentally prescribed mechanism for collective reproduction, we observed the emergence of several distinct life histories. Evolved strategies exhibited intercellular communication, coordination, and differentiation. These included endowment of offspring propagule groups, asymmetrical intra-group resource sharing, asymmetrical inter-group relationships, morphological patterning, gene-regulation mediated life cycles, and adaptive apoptosis.
Across treatments, we observed resource-sharing and reproductive cooperation among registered kin groups. These outcomes arose even in treatments where registered kin groups lacked functional significance (i.e., resource was distributed evenly), suggesting that reliable kin recognition alone might be sufficient to observe aspects of fraternal collectivism evolve in systems where population members compete antagonistically for limited space or resources and spatial mixing is low. In addition to their functional consequences, perhaps the role of physical mechanisms such as cell attachment simply as a kin recognition tool might merit consideration.
In future work, we are eager to undertake experiments investigating open questions pertaining to major evolutionary transitions such as the role of pre-existing phenotypic plasticity (Clune et al., 2007; Ofria and Lalejini, 2016), pre-existing environmental interactions, pre-existing reproductive division of labor, and how transitions relate to increases in organizational (Goldsby et al., 2012), structural, and functional (Goldsby et al., 2014) complexity. Expanding the scope of our existing work to directly study evolutionary dynamics and evolutionary histories will be crucial to such efforts.
In particular, we plan to investigate mechanisms to evolve greater collective sophistication among agents. The modular design of SignalGP lends itself to the possibility of exploring sexual recombination. We are interested in exploring extensions to allow cell groups to develop neural and vascular networks (Moreno and Ofria, 2020). We hypothesize that selective pressures related to intra-group coordination and inter-group conflict might spur developmental and structural infrastructure that could be co-opted to evolve agents proficient at unrelated tasks like navigation, game-playing, or reinforcement learning.
Unfortunately, however, experiments with multicellularity are specially constrained by a fundamental limitation of digital evolution research: processing power (Moreno, 2020). This limitation, which commonly manifests as smaller population sizes than natural populations (Liard et al., 2018), only compounds when the unit of selection shifts to computationally expensive groups of dozens or hundreds of component individuals. Ongoing work with DISHTINY is testing approaches to harness increasingly abundant parallel processing power for digital evolution simulation (Moreno et al., 2021). The spatial, distributed nature of our approach potentially affords a route to achieve large-scale digital multicellularity experiments consisting of millions, instead of thousands, of cells via high-performance parallel computing.
We hope that such technical efforts will also benefit other computational work exploring a broader range of conceptual models of multicellularity. For instance, this work assumes incessant, pervasive biotic interaction via competition for space. However, many natural systems exhibit more intermittent, sparse encounters between multicells and such selective interactions have been hypothesized as key to the evolution of complexity and diversity (Soros and Stanley, 2014). Also crucial to explore, and unaccounted for in this work, are dynamics of cell migration in development (Horwitz and Webb, 2003) and motility of multicells (Arnellos and Keijzer, 2019). It seems certain that the varied conditions and mechanistic richness of biological reality can only be fully explored through a plurality of conceptual models and model systems.
Data Availability Statement
The datasets presented in this study can be found in online repositories. The names of the repository/repositories and accession number(s) can be found below: https://osf.io/g58xk/.
Author Contributions
MAM wrote simulation software, collected data, performed the statistical analysis. and wrote the first draft of the manuscript. All authors contributed to conception and design of the study. All authors contributed to manuscript revision, read, and approved the submitted version.
Funding
This research was supported in part by NSF grants DEB-1655715 and DBI-0939454 as well as by Michigan State University through the computational resources provided by the Institute for Cyber-Enabled Research. This material is based upon work supported by the National Science Foundation Graduate Research Fellowship under Grant No. DGE-1424871. Any opinions, findings, and conclusions or recommendations expressed in this material are those of the author(s) and do not necessarily reflect the views of the National Science Foundation.
Conflict of Interest
The authors declare that the research was conducted in the absence of any commercial or financial relationships that could be construed as a potential conflict of interest.
Publisher's Note
All claims expressed in this article are solely those of the authors and do not necessarily represent those of their affiliated organizations, or those of the publisher, the editors and the reviewers. Any product that may be evaluated in this article, or claim that may be made by its manufacturer, is not guaranteed or endorsed by the publisher.
Acknowledgments
Thanks to members of the DEVOLAB, in particular Nathan Rizik for help implementing gene regulation features in SignalGP and Alexander Lalejini for help navigating the SignalGP programming API and graphically depicting SignalGP. Thanks also to Ben Kerr for comments on our manuscript.
Supplementary Material
The Supplementary Material for this article can be found online at: https://www.frontiersin.org/articles/10.3389/fevo.2022.750837/full#supplementary-material
Footnotes
1. ^We chose to number these levels using the computer science convention of zero-based indexing (as opposed to everyday practice of counting up from one) to maintain consistency with source code and data sets associated with this work.
2. ^This approach differs from existing work evolving digital organisms in grid-based problem domains, where directionality is managed by a within-cell “facing” state that determines the source direction for inputs and the target direction for outputs (Grabowski et al., 2010; Biswas et al., 2014; Goldsby et al., 2014, 2018; Lalejini and Ofria, 2018); see Supplementary Section 6.4 for further detail.
3. ^Alternately to the same end, resource sharing behavior could be instead suppressed in the opposite case, when a neighbor holds a different hereditary group ID.
References
Arnellos, A., and Keijzer, F. (2019). Bodily complexity: integrated multicellular organizations for contraction-based motility. Front. Physiol. 10, 1268. doi: 10.3389/fphys.2019.01268
Banzhaf, W., Baumgaertner, B., Beslon, G., Doursat, R., Foster, J. A., McMullin, B., et al. (2016). Defining and simulating open-ended novelty: requirements, guidelines, and challenges. Theory Biosci. 135, 131–161. doi: 10.1007/s12064-016-0229-7
Biswas, R., Bryson, D., Ofria, C., and Wagner, A. (2014). “Causes vs benefits in the evolution of prey grouping,” in ALIFE 14: The Fourteenth International Conference on the Synthesis and Simulation of Living Systems, ALIFE 2021: The 2021 Conference on Artificial Life, 641–648. doi: 10.7551/978-0-262-32621-6-ch103
Clune, J., Ofria, C., and Pennock, R. T. (2007). “Investigating the emergence of phenotypic plasticity in evolving digital organisms,” in Advances in Artificial Life, eds F. Almeida e Costa, L. M. Rocha, E. Costa, I. Harvey, and A. Coutinho (Berlin; Heidelberg: Springer), 74–83. doi: 10.1007/978-3-540-74913-4_8
Dolson, E., and Ofria, C. (2021). Digital evolution for ecology research: a review. Front. Ecol. Evol. 9, 750779. doi: 10.3389/fevo.2021.750779
Goldsby, H. J., Dornhaus, A., Kerr, B., and Ofria, C. (2012). Task-switching costs promote the evolution of division of labor and shifts in individuality. Proc. Natl. Acad. Sci. U.S.A. 109, 13686–13691. doi: 10.1073/pnas.1202233109
Goldsby, H. J., Knoester, D. B., Ofria, C., and Kerr, B. (2014). The evolutionary origin of somatic cells under the dirty work hypothesis. PLoS Biol. 12, e1001858. doi: 10.1371/journal.pbio.1001858
Goldsby, H. J., Young, R. L., Schossau, J., Hofmann, H. A., and Hintze, A. (2018). “Serendipitous scaffolding to improve a genetic algorithm's speed and quality,” in Proceedings of the Genetic and Evolutionary Computation Conference, GECCO '18, (New York, NY: Association for Computing Machinery), 959–966. doi: 10.1145/3205455.3205617
Grabowski, L. M., Bryson, D. M., Dyer, F. C., Ofria, C., and Pennock, R. T. (2010). “Early evolution of memory usage in digital organisms,” in Artificial Life XII: Proceedings of the Twelfth International Conference on the Synthesis and Simulation of Living Systems, eds H. Fellermann, M. Dorr, M. M. Hanczyc, L. L. Laursen, S. E. Maurer, D. Merkle, P. Monnard, K. Støy, and S. Rasmussen (Odense: MIT Press), 224–231.
Grabowski, L. M., Bryson, D. M., Dyer, F. C., Pennock, R. T., and Ofria, C. (2013). A case study of the de novo evolution of a complex odometric behavior in digital organisms. PLoS ONE 8, e60466. doi: 10.1371/journal.pone.0060466
Grosberg, R. K., and Strathmann, R. R. (2007). The evolution of multicellularity: a minor major transition? Annu. Rev. Ecol. Evol. Syst. 38, 621–654. doi: 10.1146/annurev.ecolsys.36.102403.114735
Gulli, J. G., Herron, M. D., and Ratcliff, W. C. (2019). Evolution of altruistic cooperation among nascent multicellular organisms. Evolution 73, 1012–1024. doi: 10.1111/evo.13727
Hanschen, E. R., Shelton, D. E., and Michod, R. E. (2015). “Evolutionary transitions in individuality and recent models of multicellularity,” in Evolutionary Transitions to Multicellular Life, eds I. Ruiz-Trillo and A. M. Nedelcu (Dordrecht: Springer), 165–188. doi: 10.1007/978-94-017-9642-2_9
Horwitz, R., and Webb, D. (2003). Cell migration. Curr. Biol. 13, R756–R759. doi: 10.1016/j.cub.2003.09.014
Knoll, A. H. (2011). The multiple origins of complex multicellularity. Annu. Rev. Earth Planet. Sci. 39, 217–239. doi: 10.1146/annurev.earth.031208.100209
Koschwanez, J. H., Foster, K. R., and Murray, A. W. (2013). Improved use of a public good selects for the evolution of undifferentiated multicellularity. eLife 2, e00367. doi: 10.7554/eLife.00367
LaBar, T., and Adami, C. (2017). Evolution of drift robustness in small populations. Nat. Commun. 8, 1–12. doi: 10.1038/s41467-017-01003-7
Lalejini, A., and Ofria, C. (2018). “Evolving event-driven programs with signalGP,” in Proceedings of the Genetic and Evolutionary Computation Conference (New York, NY: ACM Press), 1135–1142. doi: 10.1145/3205455.3205523
Liard, V., Parsons, D., Rouzaud-Cornabas, J., and Beslon, G. (2018). “The complexity ratchet: Stronger than selection, weaker than robustness,” in ALIFE 2018: The 2018 Conference on Artificial Life (Tokyo), 250–257. doi: 10.1162/isal_a_00051
Libby, E., and Ratcliff, W. C. (2014). Ratcheting the evolution of multicellularity. Science 346, 426–427. doi: 10.1126/science.1262053
Moreno, M. A. (2020). Profiling Foundations for Scalable Digital Evolution Methods. Available online at: https://doi.org/10.17605/OSF.IO/TCJFY
Moreno, M. A., and Ofria, C. (2019). Toward open-ended fraternal transitions in individuality. Artif. Life 25, 117–133. doi: 10.1162/artl_a_00284
Moreno, M. A., and Ofria, C. (2020). Practical Steps Toward Indefinite Scalability: In Pursuit of Robust Computational Substrates for Open-Ended Evolution. Available online at: https://doi.org/10.17605/OSF.IO/53VGH
Moreno, M. A., Papa, S. R., and Ofria, C. (2021). “Conduit: a c++ library for best-effort high performance computing,” in Proceedings of the Genetic and Evolutionary Computation Conference Companion, GECCO '21 (New York, NY: Association for Computing Machinery), 1795–1800. doi: 10.1145/3449726.3463205
Ofria, C., and Lalejini, A. (2016). “The evolutionary origins of phenotypic plasticity,” in ALIFE 2016, the Fifteenth International Conference on the Synthesis and Simulation of Living Systems (Cancun), 372–379. doi: 10.7551/978-0-262-33936-0-ch063
Ratcliff, W. C., Fankhauser, J. D., Rogers, D. W., Greig, D., and Travisano, M. (2015). Origins of multicellular evolvability in snowflake yeast. Nat. Commun. 6, 6102. doi: 10.1038/ncomms7102
Ratcliff, W. C., and Travisano, M. (2014). Experimental evolution of multicellular complexity in saccharomyces cerevisiae. Bioscience 64, 383–393. doi: 10.1093/biosci/biu045
Soros, L., and Stanley, K. (2014). “Identifying necessary conditions for open-ended evolution through the artificial life world of chromaria,” in ALIFE 14: The Fourteenth International Conference on the Synthesis and Simulation of Living Systems (New York, NY: MIT Press), 793–800. doi: 10.7551/978-0-262-32621-6-ch128
Staps, M., van Gestel, J., and Tarnita, C. E. (2019). Emergence of diverse life cycles and life histories at the origin of multicellularity. Nat. Ecol. Evol. 3, 1197–1205. doi: 10.1038/s41559-019-0940-0
Taylor, T., Bedau, M., Channon, A., Ackley, D., Banzhaf, W., Beslon, G., et al. (2016). Open-ended evolution: perspectives from the OEE workshop in York. Artif. Life 22, 408–423. doi: 10.1162/ARTL_a_00210
West, S. A., Fisher, R. M., Gardner, A., and Kiers, E. T. (2015). Major evolutionary transitions in individuality. Proc. Natl. Acad. Sci. U.S.A. 112, 10112–10119. doi: 10.1073/pnas.1421402112
Wilke, C. O., and Adami, C. (2002). The biology of digital organisms. Trends Ecol. Evol. 17, 528–532. doi: 10.1016/S0169-5347(02)02612-5
Keywords: digital evolution, artificial life, major transitions in evolution, multicellularity, evolution
Citation: Moreno MA and Ofria C (2022) Exploring Evolved Multicellular Life Histories in a Open-Ended Digital Evolution System. Front. Ecol. Evol. 10:750837. doi: 10.3389/fevo.2022.750837
Received: 31 July 2021; Accepted: 06 April 2022;
Published: 13 May 2022.
Edited by:
Michael Travisano, University of Minnesota Twin Cities, United StatesReviewed by:
Enrico Sandro Colizzi, Leiden University, NetherlandsCharles Rocabert, University of Helsinki, Finland
Copyright © 2022 Moreno and Ofria. This is an open-access article distributed under the terms of the Creative Commons Attribution License (CC BY). The use, distribution or reproduction in other forums is permitted, provided the original author(s) and the copyright owner(s) are credited and that the original publication in this journal is cited, in accordance with accepted academic practice. No use, distribution or reproduction is permitted which does not comply with these terms.
*Correspondence: Matthew Andres Moreno, bW1vcmU1MDBAbXN1LmVkdQ==