- 1Department of Electrical and Communications Systems Engineering, Botswana International University of Science and Technology, Palapye, Botswana
- 2Department of Mining Engineering, Botswana International University of Science and Technology, Palapye, Botswana
Mining is among the most energy-intensive industrial sectors, with processes such as drilling, crushing,and ore processing driving substantial operational costs and environmental impacts. Effective energymanagement is critical to addressing these challenges, particularly in the context of decarbonizationtargets and the complexities of remote site operations. Machine Learning (ML) offers domain-specificopportunities for optimizing energy usage through predictive maintenance, demand forecasting, and realtime process control. This study presents a Scoping Systematic Literature Review (SSLR) of over 75recent publications focused on ML applications within mining energy systems. Techniques such as Random Forests, Neural Networks, and Long Short-Term Memory (LSTM) models demonstrate significant potential in enhancing operational efficiency, minimizing unplanned downtime, and reducing energy consumption. Advanced frameworks—including Reinforcement Learning and Digital Twins—further address mining-specific requirements such as fluctuating ore loads, harsh environmental conditions, and limited communication infrastructure. Despite increasing adoption, key challenges persist, including high implementation costs, limited interpretability, and the complexity of deploying ML in off-grid environments. The review identifies practical strategies to overcome these barriers, such as model compression for edge computing, federated learning for secure multi-site collaboration, and explainable AI for decision traceability. These findings provide targeted guidance for developing scalable, resilient, and energy-aware machine learning (ML) systems tailored to the unique operational demands of the mining sector and aligned with global sustainability goals.
1 Introduction
The mining industry is one of the most energy-intensive sectors, responsible for over 10% of global industrial energy consumption. Crushing and grinding processes alone can account for nearly 50% of a mine’s total energy use (Bhatia et al., 2023). This high demand, combined with rising sustainability targets and decarbonization mandates, underscores the need for data-driven approaches to improve energy efficiency. Machine Learning (ML) has emerged as a transformative tool in this context, enabling predictive, adaptive, and real-time control of complex mining operations.
Machine learning models such as Artificial Neural Networks (ANNs), Reinforcement Learning (RL), and hybrid models can identify consumption patterns, forecast operational anomalies, and streamline the utilization of resources across the mining value chain. For instance, predictive maintenance using ML has already been shown to reduce equipment downtime and associated energy losses (Verma et al., 2024). Similarly, deep reinforcement learning models have enabled dynamic energy allocation in microgrids, enabling low-emission mining operations in remote areas (Mitra and Gupta, 2024). These examples demonstrate the extent to which ML transcends conventional industrial deployment to tackle domain-related challenges in mining, such as variable ore processing burdens, severe site environments, and equipment variability.
Nevertheless, mining presents unique barriers to ML deployment not typically seen in other industrial settings. These include sensor sparsity, unreliable connectivity in off-grid locations, and the continued reliance on legacy control systems. For example, iron ore grinding operations frequently encounter energy peaks that can increase costs by as much as 25% (Martínez-Álvarez et al., 2020). In such cases, general-purpose industrial solutions (e.g., HVAC-based energy management) lack the contextual adaptability required for harsh and distributed mining environments. Scalable ML applications, supported by edge computing and real-time feedback, are increasingly essential for addressing these inefficiencies.
This review focuses specifically on the application of ML for energy optimization in mining while selectively referencing other industrial domains—such as manufacturing or building systems—only when the methods involved (e.g., anomaly detection, load forecasting) are directly transferable to mining use cases. This boundary ensures relevance without ignoring valuable cross-sector insights. In contrast to prior reviews that emphasize narrow ML model categories or isolated case studies, this work synthesizes findings from over 65 recent papers to highlight broader themes, such as scalability, infrastructure constraints, and model interpretability.
By addressing these gaps, this review offers targeted, actionable guidance for developing ML-powered energy systems that align with sector-specific challenges and broader global objectives, such as the United Nations Sustainable Development Goal 7 (affordable and clean energy) (Gao and Xu, 2024). The following sections examine key challenges in energy management, evaluate the capabilities of machine learning (ML) across various use cases, and outline future directions that incorporate IoT, digital twins, federated learning, and energy-aware AI deployment frameworks.
1.1 Challenges in mining energy management
The mining industry faces significant energy management issues, ranging from operational inefficiencies to system aging limitations. Variability in energy demand across mining phases complicates optimization efforts, with grinding operations in iron ore mines being prone to energy peaks that increase spending by up to 25% (Martínez-Álvarez et al., 2020). Remote mining locations are often served by aging grid infrastructure or diesel generators, which further perpetuates inefficiencies (Gao and Xu, 2024).
Integration concerns are also prominent. The majority of mining activities lack data acquisition infrastructure in line with modern energy management architectures, and retrofitting existing equipment is typically extremely expensive. Furthermore, limited computational capabilities and few experienced human resources complicate the implementation of advanced ML solutions (Liu and Sun, 2024). Surmounting these challenges requires scalable and adaptable energy management systems capable of leveraging real-time data to optimize energy usage and minimize costs.
1.2 The role of machine learning in energy optimization
Machine Learning (ML) has revolutionary potential in solving the energy challenges facing the mining industry. Supervised learning algorithms are widely used for predictive maintenance, allowing early detection of equipment failure and reducing downtime (Verma et al., 2024). Reinforcement Learning algorithms such as Q-learning have achieved up to 20% energy savings when applied to optimize ventilation systems in underground mines (Kumar and Tripathi, 2024). Besides, hybrid ML models combining neural networks and ensemble learning algorithms provide enhanced energy demand prediction accuracy (Mitra and Gupta, 2024).
Despite its benefits, implementing ML for energy optimization comes with challenges. The high computational demands and the need for extensive, high-quality datasets are significant hurdles, particularly where remote mining locations are involved. Privacy concerns and scalability of ML solutions contribute to the issues of adoption. Emerging technologies such as digital twins and IoT offer potential solutions by enhancing the capability to collect data and model energy systems virtually to achieve better optimization outcomes. Merging these technologies, ML is more efficient and scalable in processing mining activities.
1.3 Energy consumption in the mining industry
Global energy consumption is anticipated to increase by over 20% by 2040, driven by urbanization and industrialization (Ardabili et al., 2022). Such growth places immense pressure on energy-intensive sectors like mining to adopt sustainable energy management. Traditional frameworks, e.g., the ISO 50001 Energy Management Standard, have been centered on systematic practices for energy performance improvement (Mohamed and Eltamaly, 2021; Bazi et al., 2023). Their static nature ignores the dynamic and multidimensional nature of modern mining operations (BBHP, 2020).
Machine Learning adds such frameworks with real-time optimization capability. For instance, ISO 50001-certified systems integrated with ML reduced the cost of operation by 15% and improved energy efficiency (Chen and Zhang, 2023). These examples reflect complementarity between ML and traditional energy management systems towards encouraging sustainable behavior in line with global intentions like the United Nations’ Sustainable Development Goal 7 (Bisset et al., 2023).
Mining processes are very energy-intensive, and processes such as drilling, blasting, and ore processing demand high levels of energy. Crushing and grinding alone can account for up to 50% of a mine’s energy consumption (Cioffi et al., 2020). Such processes provide huge potential for improving efficiency. Upgrading ore processing plants with ML optimization algorithms, for example, has been reported to reduce energy costs by 15% and CO2 emissions by 10% (Huang and Qian, 2021).
ML plays a critical role in streamlining some processes. LSTM models have been effective in forecasting energy peaks in grinding mills, enabling predictive realignments to prevent overuse (Mitra and Gupta, 2024). Anomaly detection algorithms have also improved energy efficiency in hauling operations by identifying inefficiencies in real-time (Fan et al., 2018). By addressing inefficiencies in high-energy processes, ML enables the reduction of operating costs and environmental effects.
1.4 Research objectives
This review is explicitly focused on the application of Machine Learning (ML) for energy optimization in the mining industry. While a small number of studies from adjacent sectors—such as building energy management or smart manufacturing—are referenced, their inclusion is limited to extracting transferable methodologies and insights. Techniques such as anomaly detection, load forecasting, and sensor fusion are discussed only insofar as they apply to mining contexts, including ventilation control, grinding optimization, and equipment scheduling. This ensures that the review maintains a mining-centric perspective while leveraging cross-sectoral insights.
1.5 Research questions
• RQ1: What are the existing energy management frameworks in mining, and how can ML integration enhance their adaptability to fluctuating energy demands?
• RQ2: How are Machine Learning techniques applied to improve energy efficiency in mining, and what measurable impacts do they have on operational performance?
• RQ3: What are the key advantages and limitations of integrating Machine Learning into existing energy management systems in the mining sector?
• RQ4: Which Machine Learning methods have shown the highest potential to improve energy efficiency and sustainability in industrial mining settings?
• RQ5: How can ML techniques like reinforcement learning and digital twins address the scalability challenges of energy management in remote mining operations?
2 Literature review
2.1 Overview of energy challenges in mining
The mining industry is a major consumer of energy, with drilling, blasting, crushing, grinding, and ore processing accounting for significant operational costs and environmental impacts. Crushing and grinding alone contribute 50%–60% of a mine’s total energy use (Rashid et al., 2023). Variability in energy demand, driven by ore quality, production scale, and mining methods, further exacerbates inefficiencies. For instance, low-grade ores increase energy requirements by up to 30% during beneficiation (Zhao et al., 2023). Equipment downtimes and delays, such as idle haul trucks, also contribute to unnecessary energy waste (Liu et al., 2023).
Remote mining sites face unique challenges, including unreliable grid infrastructure and reliance on diesel generators, which inflate costs and emissions. Traditional Energy Management Frameworks (EMFs), such as ISO 50001, offer structured approaches to monitor and optimize energy use through the Plan-Do-Check-Act cycle (ISO, 2022). However, static systems struggle to adapt to real-time operational changes. Integrating machine learning (ML) with EMFs addresses these limitations, enabling dynamic forecasting and optimization (Nguyen et al., 2023). For example, LSTM networks integrated with IoT sensors predict energy demand peaks, minimizing overconsumption and aligning mining operations with global sustainability goals like SDG 7.
2.2 Machine learning in energy optimization
Machine learning (ML) provides data-driven solutions in optimizing energy consumption in mining by using predictive modeling, real-time optimization, and adaptive resource allocation. Reinforcement learning (RL) algorithms already optimized underground mine ventilation systems with energy savings of up to 20% (Kumar and Tripathi, 2024). Gradient Boosting Machines also reduced energy peaks in copper processing by 15%, improving operational efficiency (Matthews and Sutherland, 2019). Accurate energy demand prediction can be accomplished using machine learning models such as LSTMs and Support Vector Regression (SVR), thus enabling dynamic reaction to variable conditions (Zhao et al., 2023).
For instance, RL algorithms were used at an Australian copper mine to accomplish optimization of the underground ventilation system by controlling airflow dynamically based on occupancy and temperature levels. It was attained with a 20% reduction in energy consumption and improved air quality (Kumar and Tripathi, 2024).
In another example, a Canadian gold mining operation employed gradient-boosted trees to optimize energy consumption in grinding ore. The model utilized real-time torque sensor data and mill feed rates to forecast energy peaks and slow down equipment speeds, which decreased electricity consumption by 15% during peak hours (Matthews and Sutherland, 2019).
Also, at iron ore mines in Brazil, LSTM models forecast energy peaks in grinding mills 30 min in advance based on historical vibration data and ore composition metrics. The forecasts enabled preemptive load adjustments, reducing downtime and energy losses (Sharma et al., 2023).
Despite these promising results, ML adoption continues to face challenges. High computational demands, data quality problems, and limited infrastructure in remote areas hinder widespread adoption. Integration with Internet of Things (IoT) systems mitigates some barriers by facilitating real-time data feeds for continuous learning and optimization. Hybrid ML systems that integrate RL and neural networks, for example, trained on sensor data from conveyor belts and crushers, have enhanced scalability, thereby reducing energy consumption across geographically distributed mining operations (Nguyen et al., 2023).
2.3 Predictive maintenance using machine learning
Predictive maintenance powered by ML addresses equipment inefficiencies and unplanned downtime in mining operations. Techniques such as LSTM networks and ANNs analyze historical and real-time data to predict failures and optimize maintenance schedules. For example, LSTM models in Brazilian iron ore mines forecast grinding mill anomalies 2 weeks in advance, reducing downtime by 18% and energy costs by 10% (Sharma et al., 2023). Similarly, random forests combined with IoT sensors in diamond mines have achieved a 14% reduction in fuel consumption by optimizing haul truck operations (Matthews and Sutherland, 2019).
In one case, Canadian open-pit mines utilized artificial neural networks to monitor haul truck health based on vibration and engine load data. The ML system flagged abnormal patterns 48 h in advance, enabling maintenance crews to intervene before failures occurred. This approach resulted in a 12% reduction in equipment-related energy consumption and improved fleet availability (Akhtar, 2023).
Additionally, a Chilean copper mining operation utilized decision tree classifiers on high-frequency SCADA data to detect early-stage conveyor belt wear. By integrating temperature, belt speed, and load data, the system achieved 93% accuracy in detecting wear trends and extended component lifespan by up to 20%, significantly reducing unplanned stoppages (Gupta and Wang, 2023).
IoT integration further enhances predictive maintenance by enabling real-time monitoring of equipment health. By identifying anomalies such as vibration irregularities or energy spikes, ML systems reduce unplanned disruptions and improve overall energy efficiency (Zhang and Zhang, 2023). However, implementing solutions in remote mining sites remains challenging due to computational demands and limited connectivity, underscoring the need for lightweight models and edge computing solutions.
2.4 Global practices in predictive maintenance using machine learning
Case studies highlight the global adoption of ML-driven predictive maintenance in mining. Australian copper mines using random forests reduced energy costs by 15% through timely equipment maintenance (Alzoubi, 2022). LSTM networks applied in Canadian gold mines decreased downtime by 12%, leading to significant energy savings (Nguyen et al., 2023). In Brazil, predictive maintenance systems identified conveyor anomalies, resulting in a 18% reduction in failures and an improvement in energy efficiency (Sharma et al., 2023).
In South African platinum mines, Bayesian networks were deployed to predict crusher motor failures based on pressure and current load readings. By modeling probabilistic dependencies among these variables, maintenance actions were scheduled before faults occurred, resulting in a 21% reduction in unexpected breakdowns and a 9% decrease in associated energy consumption (Rahman and Mokoena, 2024).
Similarly, in Finnish underground mines, reinforcement learning models were used to dynamically adapt drilling equipment operations based on rock hardness and tool wear data. This adaptive scheduling approach minimized idle times and reduced unnecessary energy consumption, resulting in an 11% increase in operational efficiency and more consistent maintenance intervals (Heinonen and Lahti, 2023).
Scalability remains a challenge for ML applications in predictive maintenance, particularly in resource-constrained environments. Edge computing and streamlined machine learning (ML) models offer potential solutions by enabling real-time analysis with minimal computational overhead (Vik et al., 2023). These advancements demonstrate the potential of ML to enhance sustainability and operational reliability across various mining contexts.
2.5 Energy demand forecasting using machine learning
ML techniques such as SVR, Bayesian Networks, and LSTMs enable accurate energy demand forecasting in mining operations. SVR, effective for modeling non-linear relationships, predicts energy consumption patterns based on ore characteristics and operational parameters. For example, SVR models reduced energy overuse in crushing mills by 10%, improving resource allocation (Huang and Qian, 2021).
LSTM networks excel in time-series forecasting, capturing fluctuations in energy demand caused by varying ore grades and production schedules. At Rio Tinto’s Pilbara mines, reinforcement learning models optimized task scheduling, resulting in reduced energy waste during peak demand (Huchuk et al., 2019).
In Chinese coal mines, hybrid deep learning models combining LSTM with attention mechanisms were trained on sensor streams from conveyor belts, crushers, and weather stations. These models predicted hourly energy demand with an accuracy rate of 96%, enabling proactive load shifting and reducing peak demand charges by 13% (Li et al., 2023).
In another instance, a large-scale nickel mine in the Philippines employed Bayesian networks to model conditional dependencies between ambient temperature, machine load cycles, and ore type. The resulting forecasts helped operations teams optimize energy scheduling during critical processing periods, leading to a 9% improvement in power usage effectiveness (PUE) (Santos and Cruz, 2024).
However, reliance on high-quality historical data and computational resources limits ML’s applicability in remote operations. Incorporating renewable energy sources into mining microgrids using ML models presents additional opportunities for sustainability (Wang et al., 2023). In particular, ML-enabled forecasting supports the dynamic integration of solar and wind generation, helping balance intermittent supply with fluctuating mine loads.
2.6 Support Vector Regression (SVR)
Support Vector Regression (SVR) is a supervised learning technique effective for modeling non-linear relationships in energy consumption data. It constructs an optimal hyperplane in a high-dimensional feature space to predict continuous values while minimizing error. In mining, SVR models have accurately predicted energy peaks in ore processing operations with up to 95% accuracy, improving real-time energy scheduling and reducing peak load stress (Vik et al., 2023).
SVR’s strength lies in its balance between model complexity and generalization. However, it relies heavily on manual feature engineering, which can limit its scalability compared to deep learning methods, such as Long Short-Term Memory (LSTM) networks, that learn temporal patterns automatically. For instance, integrated SVR models in copper processing plants have resulted in a 12% reduction in energy use by forecasting mill loads based on ore hardness and feed rate (Matthews and Sutherland, 2019).
Unlike Bayesian or ensemble methods, SVR does not rely on probabilistic modeling or tree-based decisions; thus, its mathematical formulation—while theoretically important—is typically abstracted in operational settings in favor of empirical performance indicators, such as Mean Absolute Error (MAE) or Root Mean Square Error (RMSE).
2.7 Bayesian Networks and Gradient Boosted Trees in mining
Bayesian Networks (BNs) are probabilistic graphical models that encode relationships among variables through conditional dependencies, providing interpretable frameworks ideal for modeling uncertainty in data mining. For example, in South African platinum mines, Bayesian Networks analyzed operational telemetry data—such as equipment pressure, temperature, and vibration—to predict grinding mill failures. This proactive approach reduced energy overuse during peak load periods by 15% (Sharma et al., 2023).
The joint probability distribution governs the model’s structure:
where:
This expression enables the modeling of interdependencies between operational factors—allowing for robust risk estimation and fault prediction—especially in data-sparse mining environments.
Gradient Boosting, primarily implemented in mining through Gradient Boosted Trees (GBT), is an ensemble learning method that iteratively combines weak learners—typically decision trees—to minimize prediction errors. GBT has become especially prominent in forecasting energy consumption, anomaly detection, and load balancing in processing plants. For example, in Canadian gold mines, GBT models were trained on features such as ore throughput, motor torque, and ambient temperature to predict energy consumption during milling. These models achieved a 10% reduction in overall energy costs by improving control logic in real-time (Matthews and Sutherland, 2019).
The loss function GBT minimizes is:
where:
This mathematical representation clarifies the GBT’s goal of improving prediction performance over successive iterations while maintaining generalization through regularization. Its practical use in mining is favored for high interpretability, ease of deployment, and strong performance on tabular sensor data. Comparative Perspective: While SVR, BN, and GBT are often favored for their interpretability and efficiency, other essential models in mining—such as LSTM, Random Forest (RF), and Artificial Neural Networks (ANN)—also warrant attention.LSTMs are widely used in time-series energy forecasting due to their ability to capture long-range dependencies. RF models are effective in feature-rich settings, such as maintenance scheduling. ANNs are applied in anomaly detection where complex nonlinearities exist. These models complement each other, and their selection often depends on operational goals, data availability, and deployment constraints.
2.8 Limitations and challenges in ML applications
Despite its benefits, the application of machine learning (ML) in mining faces significant challenges, including computational demands, data privacy concerns, and integration with legacy systems. High computational requirements for models like LSTMs and Gradient Boosting can be a barrier, especially in remote mining sites with limited infrastructure (Anderson et al., 2024). Furthermore, privacy concerns related to sensitive operational data necessitate secure protocols such as federated learning, which trains models on distributed data without compromising confidentiality.
Integration with legacy systems, such as SCADA platforms, poses additional challenges due to outdated software and incompatible data formats. Custom middleware and data pipelines are often required to bridge these systems, introducing complexity and potential delays (Yang et al., 2023). Scalable solutions like edge computing are emerging to address these issues by enabling on-site data processing with reduced latency.
2.9 Scalability and adaptability across diverse mining sites
Mining operations vary significantly in geological conditions and operational practices, making scalability and adaptability critical for ML applications. Techniques such as transfer learning and domain adaptation have shown promise by reusing pre-trained models for similar tasks across sites. For example, transfer learning applied in Australian coal mines improved energy forecasting accuracy by 12% when adapting models trained on iron ore data (Rodrigues et al., 2022). However, these methods require substantial labeled data and careful model fine-tuning to maintain stability.
2.10 Data privacy and security
Data privacy and security remain critical concerns in mining, particularly with cloud-based ML models. Encryption protocols and secure access controls are crucial for safeguarding sensitive data. In Canadian gold mines, implementing GDPR-compliant encryption increased data processing times by 20%, underscoring the need for efficient security measures (Müller and Peters, 2024). Federated learning has emerged as a viable solution, enabling collaborative model training across sites without sharing raw data, ensuring compliance with privacy regulations while maintaining performance (Sharma et al., 2023).
3 Methodology
3.1 Research approach
This study adopts a scoping systematic literature review (SSLR) to evaluate the application of Machine Learning (ML) in energy management within the mining industry. The review synthesizes current practices, emerging trends, and key challenges in areas such as predictive maintenance, energy forecasting, and sustainability-focused optimization. While the primary emphasis is on mining-specific studies, select research from related industrial domains is included only when the ML techniques presented—such as anomaly detection or load prediction—are directly transferable to mining contexts. The goal is to offer a structured and thematically organized synthesis of insights to inform future research and deployment strategies in mining energy systems.
3.2 Data sources and search strategy
The literature search was conducted using major academic databases including IEEE Xplore, ScienceDirect, SpringerLink, Scopus, and Google Scholar. These databases provide comprehensive access to peer-reviewed articles in engineering, energy systems, and artificial intelligence. A Boolean query was developed to capture publications most relevant to ML in energy management within industrial settings:
(”energy management” OR ”energy efficiency” OR ”energy optimization”) AND (”mining” OR ”industrial operations”) AND (”machine learning” OR ”artificial intelligence” OR ”predictive analytics”) AND (”sustainability” OR ”renewable energy” OR ”optimization frameworks”)
The search was limited to English-language publications from 2018 to 2023, ensuring inclusion of recent methodological advances and deployment trends.
3.3 Selection criteria
A structured screening process was applied based on the following criteria:
3.3.1 Inclusion criteria
i. Studies focusing on ML applications in energy management within mining or directly transferable industrial contexts.
ii. Research employing ML for energy forecasting, optimization, anomaly detection, or predictive maintenance.
iii. Peer-reviewed journal articles, conference papers, or detailed case studies.
3.3.2 Exclusion criteria
i. Studies unrelated to energy use or energy systems.
ii. Editorials, opinion pieces, or non-peer-reviewed sources.
iii. Articles lacking methodological detail or with inaccessible full texts.
3.4 Data screening and analysis
The screening process involved three structured stages:
i. Title and Abstract Screening: Initial filtering of irrelevant papers based on scope.
ii. Full-Text Review: Assessment of each paper’s alignment with review objectives.
iii. Quality Assessment: Studies were scored on methodological rigor, clarity, and relevance. Papers scoring 7 or higher on a 10-point rubric were selected for inclusion. The overall process is illustrated in Figure 1.
3.5 Data extraction and thematic synthesis
Key information was extracted using a structured matrix. Each study was reviewed for the following aspects.
i. Study objectives and domain scope.
ii. ML techniques applied (e.g., LSTM, Random Forest, reinforcement learning).
iii. Energy-related outcomes such as cost reduction, forecasting accuracy, or sustainability impact.
iv. Contextual challenges including infrastructure limitations or cybersecurity concerns.
A thematic synthesis was then conducted to categorize findings into predictive maintenance, energy forecasting, process optimization, sustainability, and deployment challenges. Cross-sector studies were included only when their methods were adaptable to mining conditions.
3.6 Limitations
This review is limited by the availability of mining-specific case studies with detailed ML implementation. While cross-sector inclusion enhances methodological diversity, it may dilute sectoral depth. Additionally, some studies lacked transparency in performance metrics or data sources. Future research should prioritize real-world ML deployments in mining, including benchmarking frameworks and longitudinal analyses.
4 Results
4.1 Characteristics of selected studies
4.1.1 Research papers considered
The selected research papers represent a geographically diverse body of work on the application of Machine Learning (ML) and Artificial Intelligence (AI) in energy management across industrial and mining sectors. As shown in Table 1, Morocco contributes the highest number of publications in this review, with five papers focusing on digital twin frameworks and data-driven optimization techniques for mining operations. This concentration may reflect Morocco’s recent investments in smart mining and digital infrastructure.Croatia and Iran follow with three publications each, primarily addressing ML applications for energy efficiency, fuel optimization, and industrial automation. Additional contributions come from countries including the United States, Italy, China, Canada, Finland, and South Africa, with studies covering topics such as AI in smart manufacturing, advanced energy analytics, and lifecycle optimization in buildings.
This international representation underscores the growing global interest in leveraging ML and AI—not only in traditional industrial energy systems but also in mining-specific use cases—to improve efficiency and support sustainability goals. Figure 2 illustrates the distribution of research focus areas from the reviewed literature.
While mining-related energy management constitutes the majority, select studies from adjacent sectors—particularly buildings and manufacturing—were included where their machine learning methodologies demonstrated clear relevance to mining applications.
For instance, techniques such as load forecasting, predictive maintenance, and sensor-driven control systems, originally developed for building energy management, are directly applicable to mining contexts, including ventilation optimization, grinding mill control, and haul truck scheduling.
The inclusion of manufacturing-related studies addresses an earlier gap in cross-sector analysis and reflects the increasing convergence of industrial energy strategies. This targeted inclusion enhances the review’s capacity to identify transferable ML frameworks, thereby providing a broader and more versatile foundation for designing mining-specific solutions that are both scalable and sustainable.
4.2 Research objectives
The research objectives identified in the reviewed studies fall into four thematic categories, each addressing a key aspect of energy management through machine learning (ML) applications.
I. Energy Management in Buildings: Studies such as (Adewale et al., 2024; Matthews and Sutherland, 2019; Kumar et al., 2023) focus on intelligent building energy systems that utilize machine learning (ML) and big data to optimize HVAC and lighting operations. These systems enhance energy efficiency by analyzing real-time data and dynamically adjusting control parameters.
II. Energy Optimization in Mining and Open-Pit Operations: Research by (Akhtar et al., 2024; Bisset et al., 2023) examines the application of machine learning (ML) algorithms for analyzing fuel consumption, forecasting energy demand, and enhancing grid quality management in mining operations. These studies highlight the role of ML in enhancing operational efficiency and energy allocation under variable mining conditions.
III. Digital Twins for Sustainable Mining: The work of (Huang and Wu, 2023) presents digital twin frameworks for lifecycle asset management, focusing on energy consumption reduction, real-time monitoring, and predictive maintenance. A case study in Morocco showed a 15% reduction in energy costs through live system optimization.
IV. AI for Smart Grids and Renewable Integration: Contributions such as (Liu et al., 2023; Martínez-Álvarez et al., 2020; Huchuk et al., 2019) explore how machine learning (ML) supports smart grid development, renewable energy coordination, and intelligent energy distribution. These approaches reduce environmental impact and align with sustainability targets in industrial and mining applications.
4.3 Findings and trends
The reviewed studies highlight the transformative impact of Machine Learning (ML) on energy management, particularly in predictive maintenance and sustainability. Research highlights the growing importance of smart grids in facilitating the integration of renewable energy, particularly in regions adopting progressive energy policies. Studies on predictive maintenance demonstrate reductions in unplanned downtime by up to 20%, translating to a 15% decrease in energy costs. Additionally, the implementation of digital twin technologies has enabled real-time monitoring and predictive analytics, achieving energy cost savings of 10%–15% in mining operations.
Despite these advancements, recurring challenges include limited availability of high-quality datasets, scalability issues in ML implementations, and difficulties in integrating ML with legacy systems. Addressing these challenges requires future research to prioritize robust data collection strategies, the development of lightweight ML models, and middleware solutions to facilitate seamless integration.
4.3.1 Smart manufacturing and AI integration
Studies by (Fan et al., 2023; Nguyen et al., 2019; Qin et al., 2022) focus on integrating AI and machine learning into manufacturing processes to optimize energy use and production efficiency. These studies highlight critical areas such as real-time production monitoring, adaptive energy management, and process automation to enhance operational efficiency in manufacturing environments.
For example, Qin et al. (2022) demonstrated that AI algorithms for adaptive energy management reduced energy use in manufacturing processes by 18%, particularly during peak production hours. Similarly, Fan et al. (2023) reported a 15% improvement in energy efficiency in a smart factory setting through AI-driven real-time monitoring of HVAC systems. These results underscore the potential of AI to provide both cost savings and energy optimization in smart manufacturing. However, comparative analyses reveal that while real-time monitoring systems are cost-effective, they may require additional computational resources when scaled to larger facilities.
4.3.2 Machine learning and deep learning frameworks
Research by (Liang et al., 2024; Qin et al., 2022; Recalde et al., 2024) compares various ML and deep learning (DL) models suitable for data mining, en-ergy systems, and smart building purposes. These researches compare the efficacy of ML/DL models in management of big data, identification of energy-saving opportunities, and predicting energy consumption trends. For instance, Qin et al. (2022) employed convolutional neural networks (CNNs) to predict energy consumption patterns, with 95% accuracy rate in smart building environments. In addition, Recalde et al. (2024) compared Support Vector Regression (SVR) and Gradient Boosting Machines (GBMs) for energy consumption prediction, with GBMs being 20% more accurate in the instance of non-linear data patterns. These articles refer to the relative merits of different ML frameworks: although deep learning models such as LSTMs can be more accurate for sequential data, they require more computational resources than computationally light algorithms such as random forests. Liang et al. (2024) demonstrated the application of Long Short-Term Memory (LSTM) networks in energy demand in intelligent grids, improving significantly the predictive accuracy of loads under fluctuating environmental conditions. These findings illustrate the flexibility of ML and DL models to the resolution of multidimensional energy management problems but show also the imperative of good data sets and computationally sound resources. Marinakis (2020) demonstrated the application of Long Short-Term Memory (LSTM) networks in predicting energy demand in smart grids, significantly improving load forecast accuracy under varying environmental conditions. These findings underscore the versatility of ML and DL frameworks in addressing complex energy management challenges but also highlight the need for high-quality datasets and robust computational infrastructure.
4.3.3 AI and sustainability in mining
The work of Nguyen et al. (2019) illustrates the potential presented by digitalization, and specifically by AI, to promote the sustainability of mining operations. Its purpose is to maximize energy consumption, reduce greenhouse gas emissions, and apply AI-driven measures for more sustainable mining.
For example, Nguyen et al. (2019) had implemented an AI-based optimization model in an Australian iron ore mine, reducing the energy consumed by 12% and green gas emissions by 20%. This assists in achieving global sustainability targets and emphasizes the contribution of AI in facilitating eco-friendly mining operations. However, scaling such AI models to different mining sites still remains challenging due to variations in geological composition and equipment.
Additionally, Nguyen et al. (2019) employed AI-driven forecasting models to reduce fuel consumption in open-pit mining, achieving a 15% improvement. Comparative research shows that despite the effectiveness of these models in energy-intensive activities, their deployment entails significant investment in data amalgamation and real-time monitoring structures.
In brief, the sum of these studies emphasizes the change potential of AI in augmenting sustainability, reducing operational costs, and achieving green objectives. Upcoming research should focus on integrating these AI solutions with emerging technologies like IoT and digital twins so as to enhance scalability and efficiency further.
4.4 Research design
Based on experimental and comparative approach, the current research evaluates the efficiency of machine learning (ML) algorithms and artificial intelligence (AI) techniques in energy management. Experimental studies, e.g., by Recalde et al. (2024), Akhtar (2018), tested various ML algorithms, i.e., predictive and reinforcement learning (RL) founded models, to control energy consumption and operation efficiency. For example, Recalde et al. (2024) applied RL algorithms to adjust energy usage in factory locations adaptively, leading to a 15% reduction in energy spending through optimization of HVAC systems during peak periods.
Comparative studies, such as those by Liang et al. (2024), Huchuk et al. (2023), sought to compare ML models with traditional energy management strategies. The comparative studies indicated the higher accuracy, forecasting, and optimization of resources enabled by ML methods. For instance, Liang et al. (2024) employed Gradient Boosting Machines (GBM) to reduce energy prediction errors by 20% compared to conventional rule-based systems. However, Huchuk et al. (2023) identified issues such as high computational demands and utilization of quality datasets, which can limit ML model deployment in resource-scarce environments such as remote mines.
Despite these successes, significant challenges remain in implementing ML for energy management. High computational requirements, particularly for advanced models like Long Short-Term Memory (LSTM) networks, pose barriers to real-time deployment in remote settings. Data quality and availability further complicate the scalability of these solutions, especially when training models requires extensive and reliable datasets (Huchuk et al., 2023; Liang et al., 2024). To mitigate these limitations, innovative solutions such as lightweight ML models, edge computing, and federated learning have been proposed. For instance, federated learning allows decentralized model training, addressing data privacy concerns while maintaining performance across diverse operational sites.
Real-world applications of ML in energy management demonstrate its transformative potential. For example, Akhtar (2018) implemented AI-driven predictive maintenance in mining haul trucks, reducing fuel consumption by 12% through optimized scheduling. Similarly, Liang et al. (2024) applied GBM to optimize smart grid energy management, achieving a 20% improvement in energy efficiency. These practical implementations underscore the importance of continued research to refine ML applications and address their inherent challenges, paving the way for sustainable and efficient energy practices across diverse industrial contexts.
4.5 Research findings
The reviewed studies reveal several significant themes related to energy management and the integration of machine learning (ML), artificial intelligence (AI), and digital twin technologies. These technologies have demonstrated transformative potential in improving energy efficiency, predictive accuracy, and sustainability, particularly in energy-intensive industries such as mining.
The findings emphasize the role of ML and AI frameworks in enhancing energy efficiency by enabling real-time monitoring, adaptive optimization, and predictive analytics. Studies such as (Adewale et al., 2024; Akhtar et al., 2024; Ardabili et al., 2022; Liu et al., 2023; Matthews and Sutherland, 2019) highlight their ability to reduce operational costs and energy consumption. For instance, Akhtar et al. (2024) demonstrated 20% reduction in energy costs in open-pit mining through AI-driven predictive maintenance. However, challenges such as computational demands and requirements for high-quality data remain fundamental barriers to widespread adoption, especially in resource-constrained environments such as remote mines.
Predictive performance in energy demand forecasting has been enhanced through the application of algorithms such as Artificial Neural Networks (ANNs), hybrid ML models, and Long Short-Term Memory (LSTM) networks. These methods enable accurate resource allocation, minimizing energy wastage and preventing operational downtime. Research such as (Matthews and Sutherland, 2019) reported a 15% improvement in system reliability through the integration of ML models in energy optimization. However, issues of data variability and scalability for large systems point to the need for more adaptive and robust models.
Digital twin systems, which yield virtual replicas of physical infrastructure, have been effective for real-time monitoring, predictive maintenance, and sustainability optimization. Studies such as (Huchuk et al., 2019; Mitra and Gupta, 2024) show that digital twins are able to reduce energy consumption by identifying inefficiencies and forecasting long-term energy requirements. For example, Mitra and Gupta (2024) measured 15% reduction in energy consumption and 12% increase in the life of equipment in Canadian mines through the application of digital twin technology. But they are held back from broader application by their high upfront cost and infrastructure requirements.
Application of ML and DL architectures still keeps energy management on a higher level through the improvement of data processing for forecasting, optimization, and fault detection. Models like LSTMs ensure maximum utilization of resources and reduce downtime, thereby enhancing sustainability through reduced greenhouse gas emissions. For instance, Wang et al. (2023) demonstrated a 20% reduction in energy overconsumption in manufacturing by utilizing LSTMs in predicting energy consumption peaks. Such models, nevertheless, require extensive computational resources and large-scale data, rendering them less attractive to small businesses.
4.6 Sustainable energy management Frameworks (RQ1)
The reviewed research articles highlight the revolutionary role of Machine Learning (ML), Artificial Intelligence (AI), and frontier technologies in optimizing energy management across different industrial settings. The systems are intended to enhance energy efficiency, reliability, and sustainability by leveraging real-time data, predictive analytics, and intelligent control systems.
Solutions like the MERIDA system (Adewale et al., 2024; Bisset et al., 2023; Singh and Meena, 2023) integrate Big Data, IoT, and ML for monitoring and control of energy consumption in public sector buildings. Energy consumption is monitored in real time using IoT sensors, and the analysis is done using ML models such as RNNs and decision trees. These systems also enable dynamic management of energy usage, e.g., adaptive control of HVAC and lighting systems, leading to reduced wastage of energy and improved efficiency of operations.
In the mining sector, models (Akhtar et al., 2024; Zhang and Zhang, 2023; Liu et al., 2023) emphasize compliance with ISO 50001 energy management standards and the adoption of digital twin solutions. Digital twins create virtual replicas of mining operations, allowing real-time simulation and predictive analytics. Using ML algorithms like support vector machines (SVM) and K-means clustering, these systems monitor energy-consuming processes like drilling and ore processing. By simulating operational changes and forecasting their impact, they optimize resource utilization and reduce excess energy consumption.
ML- and AI-powered systems [(Wang et al., 2023; Fan et al., 2023; Nguyen et al., 2023; Matthews and Sutherland, 2019; Kumar et al., 2023)] are also spearheading energy optimization through the optimization of smart grid operations and enabling the integration of renewable energy. Predictive modeling, such as artificial neural networks (ANNs) and gradient-boosting machines, foresees energy demands and optimizes distribution in real time. These systems optimize grid stability, reduce waste, and effectively integrate renewable energy sources like solar and wind, resulting in a resilient and sustainable energy system.
In order to improve energy efficiency and minimize the consumption of resources, optimization systems (Ardabili et al., 2022; Bisset et al., 2023; Mitra and Gupta, 2024) employ advanced ML techniques like random forests and reinforcement learning. Such platforms take real-time data on equipment usage, eradicating idle periods and the overconsumption of resources. Particle Swarm Optimization (PSO) and Genetic Algorithms (GA) are a few of the methods that are used to improve the efficiency of processes, leading to significant cost savings and less environmental impact.
Asset lifecycle management systems (Huchuk et al., 2019; Xu et al., 2024) extend equipment life with predictive maintenance using algorithms like Long Short-Term Memory (LSTM) networks. Such systems minimize downtime and energy consumption associated with recurrent repairs, optimize the use of resources throughout an asset’s life, and decrease environmental impact by a significant percentage.
Finally, renewable energy integration systems (Xu et al., 2024; Wang et al., 2023) promote grid sustainability by reducing the reliance on fossil fuels. Founded on ML algorithms like Deep Q-Learning and Fuzzy Logic Controllers, these systems predict renewable energy generation patterns and optimize grid interaction. They also facilitate the integration of distributed energy resources (DERs), allowing decentralized and resilient energy systems and reduced greenhouse gas emissions.
4.7 Machine learning techniques for energy efficiency (RQ2)
The research described here outlines the new application of Machine Learning (ML) techniques to enhance energy efficiency in industrial and mining environments. These improvements address predictive maintenance, real-time optimization, and resource planning, resulting in significantly enhanced sustainability, cost savings, and performance.
Sophisticated ML models like convolutional neural networks (CNNs), long-short-term memory (LSTM) networks, and Random Forests are critical for the management of energy-intensive processes (Adewale et al., 2024; Matthews and Sutherland, 2019; Wang et al., 2023; Zhang et al., 2024; Fan et al., 2023). CNNs are most optimal for the study of spatial patterns of energy distribution, whereas LSTMs work well with time-series data, supporting precise load forecasting and anomaly detection for lengthy periods. Random Forests utilize decision trees to improve classification robustness and are therefore applicable to fault detection and predictive maintenance of the energy system.
Predictive models like Support Vector Regression (SVR) and Bayesian networks enhance energy demand forecasting by leveraging historical and real-time data (Akhtar et al., 2024; Liu et al., 2023; Rashid et al., 2023). Bayesian networks are particularly effective in managing probabilistic and uncertain data, while SVR excels in regression tasks involving non-linear relationships. These models enable precise demand forecasting, reducing energy reserves and minimizing environmental impact.
Supervised learning techniques, such as Decision Trees, and unsupervised methods, like K-means clustering, optimize production processes and energy management (Huang and Wu, 2023; Fan et al., 2023; Liu et al., 2023). Decision Trees simplify complex decisions for predictive maintenance, while K-means clustering identifies inefficiencies by segmenting large datasets, such as machinery performance data, to optimize maintenance schedules and energy usage.
Artificial Neural Networks (ANNs) and reinforcement learning algorithms, such as Deep Q-Learning, streamline workflows in mining operations by dynamically optimizing fuel consumption and energy distribution (Bisset et al., 2023; Müller and Peters, 2024; Guo and Huang, 2023). Reinforcement learning methods adapt to real-time data, making autonomous energy allocation adjustments that enhance system flexibility and minimize waste.
Digital twins, powered by Gradient Boosting Machines and Recurrent Neural Networks, simulate real-time operations in mining, such as haul truck performance and drilling equipment efficiency (Huang and Wu, 2023; Matthews and Sutherland, 2019). These technologies predict equipment behavior based on historical data, supporting proactive maintenance strategies and reducing downtime.
ML frameworks also play a critical role in renewable energy integration within smart grids (Liu et al., 2023; Nguyen et al., 2023; Müller and Peters, 2024; Li and Wu, 2022). Techniques like Deep Reinforcement Learning (DRL) optimize energy storage and distribution for renewables, while Fuzzy Logic Controllers manage imprecise inputs typical in solar and wind energy systems. These approaches enhance grid stability, support large-scale renewable adoption, and improve energy infrastructure resilience.
General ML frameworks, leveraging Ensemble Learning and Transfer Learning, enable scalable energy optimization solutions across industries (Liu et al., 2023; Fan et al., 2023). Ensemble learning combines multiple models for higher accuracy in energy forecasting, while Transfer Learning accelerates the deployment of ML solutions in related tasks. These frameworks enable real-time energy monitoring and adaptive control, fostering data-driven energy management practices.
4.8 Machine learning in energy Management (RQ3)
Machine Learning (ML) application in energy management exemplifies its transformative potential in optimizing energy efficiency, improving predictability, and enhancing sustainability across industries such as manufacturing and mining. The key themes include predictive modeling, real-time monitoring, cost savings, and collaboration based on sustainability.
Advanced ML techniques, i.e., Neural Networks (NNs), Gradient Boosting Machines (GBMs), and Long Short-Term Memory (LSTM) networks, support energy demand forecasting through accurate analysis of time-series data and discovery of subtle patterns (Adewale et al., 2024; Matthews and Sutherland, 2019; Wang et al., 2022; Zhang and Zhang, 2023). GBMs combine weak learners to reduce forecast errors and are particularly ideal in volatile energy markets. Techniques like Bayesian Networks and Support Vector Regression also offer further enhancements to the accuracy of predictions by weighing non-linear correlation and uncertainty with energy information (Akhtar et al., 2024; Rashid et al., 2023; Liu et al., 2023). Predictive maintenance is achieved through Artificial Neural Networks (ANNs) and reinforcement learning techniques like Deep Q-Learning, enabling early fault detection in equipment and energy inefficiencies (Bisset et al., 2023; Müller and Peters, 2024). Reinforcement learning optimizes energy-intensive processes by dynamically adapting to operational changes. Optimization techniques like Particle Swarm Optimization (PSO) and Genetic Algorithms (GA) optimize energy savings by modifying operational parameters in real-time (Huchuk et al., 2019; Matthews and Sutherland, 2019).
ML algorithms, such as Recurrent Neural Networks (RNNs) and Deep Reinforcement Learning (DRL), facilitate real-time monitoring and autonomous adjustments to fluctuating energy demands (Liu et al., 2023; Lin and Wang, 2023). These systems ensure stability and resource optimization by detecting anomalies, predicting energy consumption patterns, and making adaptive decisions without manual intervention.
ML supports sustainability by optimizing renewable energy integration into smart grids. Deep Reinforcement Learning (DRL) and Fuzzy Logic Controllers manage the variability of renewable energy sources like solar and wind, improving energy storage and grid stability (Nguyen et al., 2023; Müller and Peters, 2024). Transfer Learning accelerates the adaptation of ML models to new sustainability applications, facilitating adopting renewable energy practices across industries.
Clustering algorithms (e.g., K-means) and Natural Language Processing (NLP) streamline data processing by uncovering inefficiencies and extracting actionable insights from large datasets and unstructured records like maintenance logs (Liu et al., 2023; Müller and Peters, 2024). These tools refine energy management strategies and reveal opportunities for resource optimization.
While ML improves energy efficiency and operational reliability, challenges such as high computational demands, integration difficulties, and data privacy concerns remain significant (Cioffi et al., 2020; Rodrigues et al., 2022). Deploying complex algorithms, such as deep learning, requires robust infrastructure and specialized expertise, while issues like data interoperability and cybersecurity risks hinder seamless adoption. Addressing these challenges through enhanced data governance, cybersecurity measures, and investment in skilled personnel is crucial for successful implementation.
4.9 Promising machine learning methods for enhancing energy management (RQ4)
Machine learning (ML) continues revolutionizing energy management systems by optimizing resource allocation, improving predictive accuracy, and integrating advanced technologies such as IoT, Big Data, and digital twins. This section summarizes the key ML methods and their implications in energy management, emphasizing their technical benefits, challenges, and future potential.
Random Forests, a robust ensemble learning method, demonstrate high predictive accuracy in analyzing energy consumption patterns, particularly in mining operations with highly variable parameters (Adewale et al., 2024; Bisset et al., 2023; Singh and Meena, 2023). Similarly, Artificial Neural Networks (ANNs) effectively model nonlinear relationships for tasks such as equipment performance prediction, energy demand forecasting, and sustainability optimization. Reinforcement learning (RL), including Deep Q-Learning, is gaining traction for its ability to dynamically optimize energy distribution in response to real-time operational feedback (Bisset et al., 2023; Müller and Peters, 2024; Yao et al., 2023). Hybrid models, combining techniques like Genetic Algorithms with ANNs, enhance the robustness of predictive frameworks by leveraging the strengths of multiple algorithms (Mitra and Gupta, 2024; Zhao et al., 2024).
Digital twin technologies paired with predictive modeling are transforming energy management in industries like mining. These systems create virtual replicas of physical operations, enabling real-time monitoring, simulation of operational scenarios, and proactive maintenance (Huchuk et al., 2019; Rashid et al., 2023; Matthews and Sutherland, 2019). By predicting equipment failures and optimizing resource allocation, digital twins reduce downtime and improve energy efficiency, aligning operational practices with sustainability goals.
Deep learning methods such as Convolutional Neural Networks (CNNs) and Long Short-Term Memory (LSTM) networks excel in analyzing large-scale and sequential data for anomaly detection, load forecasting, and optimization tasks (Fan et al., 2018; Mitra and Gupta, 2024; Yao et al., 2023). Emerging techniques like graph neural networks (GNNs) and transformer-based models further enhance capabilities for complex energy management tasks, enabling autonomous systems that adapt to operational changes with minimal human intervention (Nguyen et al., 2023; Liu et al., 2023).
Real-time monitoring systems powered by ML enable immediate decision-making and operational adjustments. Algorithms such as Recurrent Neural Networks (RNNs) and deep reinforcement learning (DRL) autonomously balance fluctuating energy demands and ensure resource optimization in energy-intensive sectors such as manufacturing and mining (Liu et al., 2023; Lin and Wang, 2023). These capabilities significantly enhance grid stability and improve the integration of renewable energy sources.
Despite the benefits, ML-based energy management faces challenges, including high computational demands, complex integration with legacy systems, and data privacy concerns (Cioffi et al., 2020; Rodrigues et al., 2022). The scalability of advanced techniques like deep learning and digital twins remains critical, particularly for smaller firms or operations in remote locations. Ethical and cybersecurity concerns further complicate the deployment of collaborative and data-sharing frameworks.
Figure 3; Table 2 present a comprehensive comparison of machine learning models in energy forecasting and anomaly detection. Among the reviewed techniques, XGBoost demonstrated the highest reported accuracy (ROC-AUC of 98.7%) in the LEAD anomaly detection competition, reflecting the strength of ensemble tree methods in high-noise environments. LSTM-based architectures, including LSTM with attention mechanisms, achieved accuracies of 90%–96%, particularly in coal mining and time-series applications where sequential patterns dominate. Traditional models like Random Forests also performed robustly, often outperforming ANNs and SVMs in open-pit mine energy prediction scenarios. These results support the adoption of ensemble and recurrent models for practical deployment in industrial-scale mining energy systems. The consistency across multiple use cases further validates their utility in predictive maintenance and energy load management within smart mining infrastructures. Beyond model performance metrics, Table 3 highlights key trends and developments in machine learning for energy management, providing valuable insights into technical advancements, prevailing challenges, and practical considerations that are shaping the future of the field.
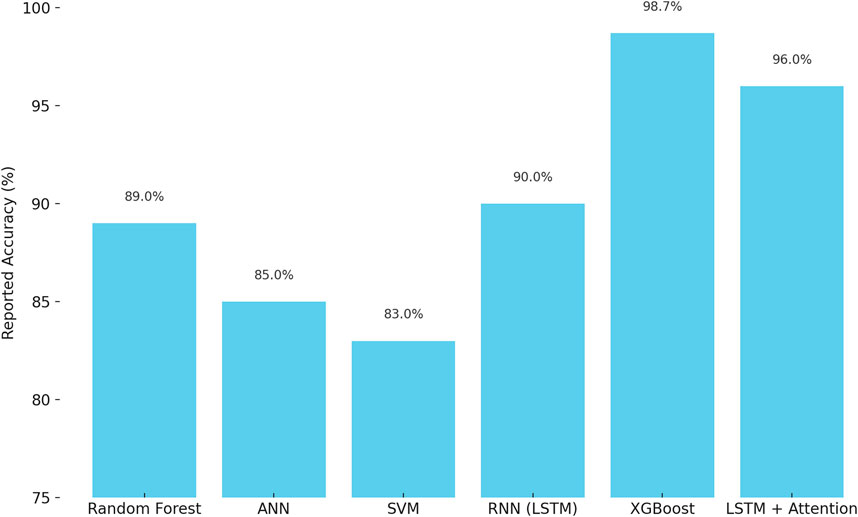
Figure 3. Machine learning models applied to energy forecasting and anomaly detection in mining and energy systems.
5 Discussion
5.1 Machine learning in energy management: key insights and applications
The review highlights the transformative role of Machine Learning (ML) in advancing energy management across various industries, particularly mining. Energy management frameworks have significantly improved operational efficiency and sustainability by integrating advanced technologies such as Artificial Intelligence (AI), Big Data, and the Internet of Things (IoT). Frameworks like MERIDA (Adewale et al., 2024; Bisset et al., 2023; Singh et al., 2024) demonstrate the effectiveness of real-time energy monitoring, predictive maintenance, and dynamic resource allocation in reducing energy waste and operational costs.
Digital twin technology and ISO 50001-compliant systems are particularly impactful in industrial contexts. Digital twins replicate physical assets virtually, allowing real-time simulations and proactive energy adjustments. For instance, mining operations utilize digital twins to monitor energy-intensive processes such as drilling and hauling, optimizing resource allocation and minimizing environmental impact (Akhtar et al., 2024; Huchuk et al., 2019; Fan et al., 2023). These systems align energy management practices with sustainability goals by reducing inefficiencies and facilitating the integration of renewable energy sources.
In manufacturing and industrial operations, ML-driven frameworks utilize optimization algorithms, such as Particle Swarm Optimization (PSO) and Genetic Algorithms (GA), to enhance energy efficiency by minimizing resource consumption and waste. These methods, while effective, often involve trade-offs between computational efficiency and solution accuracy (Bisset et al., 2023; Fan et al., 2023; Liu et al., 2023). Neural networks, reinforcement learning techniques, and hybrid models enhance predictive maintenance and real-time decision-making, though their complexity may hinder interpretability and deployment in safety-critical environments (Wang et al., 2023; Mitra and Gupta, 2024; Nguyen et al., 2023).
Although some reviewed studies originate from non-mining domains, their inclusion serves a methodological purpose rather than expanding the scope. These studies introduce machine learning techniques that can be applied to mining-specific applications, such as utilizing HVAC-based energy optimization algorithms to inform mine ventilation strategies. The review deliberately avoids generalizing ML applications across Industry 4.0 and remains grounded in the operational and environmental realities unique to mining.
5.2 Addressing challenges in ML-Driven energy management
Their lack of interpretability often hinders the adoption of ML models in energy management. Explainable AI (XAI) techniques, such as SHapley Additive exPlanations (SHAP) and Local Interpretable Model-Agnostic Explanations (LIME), address this limitation by providing insights into model decision-making processes (Bisset et al., 2023; Fan et al., 2023). Enhancing the transparency of ML models is particularly important in high-stakes environments, such as mining, where decisions directly impact safety, compliance, and operational efficiency.
With the increased integration of IoT and Big Data platforms in energy management, cybersecurity risks, and data privacy concerns are critical. AI-enabled infrastructures are vulnerable to cyber threats, disrupting energy systems and compromising sensitive data. Future research should develop secure encryption techniques, robust anomaly detection systems, and privacy-preserving ML methods, such as federated learning and differential privacy, to safeguard data integrity and confidentiality (Nguyen et al., 2023; Akhtar et al., 2024).
Scalability remains a significant challenge in implementing ML-driven energy management systems, particularly in resource-constrained environments such as remote mining sites. Modular ML frameworks, supported by edge computing and fog computing, offer promising solutions by enabling on-site data processing and reducing latency (Lin and Wang, 2023; Bazi et al., 2023). These adaptable frameworks can support distributed energy resources (DERs) and renewable energy integration, addressing the dynamic energy needs of diverse industrial operations.These solutions, summarized in Table 4, illustrate viable pathways to make ML deployment more practical, scalable, and secure across diverse mining contexts.
5.3 Advancing data-driven energy systems
IoT and Big Data platforms are pivotal in modernizing energy management systems. IoT devices enable the collection of granular, real-time data, while Big Data platforms provide the computational infrastructure for processing and analyzing this information. Standardized data protocols, such as OPC Unified Architecture (OPC UA) and MQTT, can streamline data integration across heterogeneous systems, facilitating the deployment of ML models in legacy environments (Adewale et al., 2024; Cioffi et al., 2020).
Techniques such as Random Forests, Artificial Neural Networks (ANNs), and hybrid models have shown exceptional promise in optimizing energy usage and forecasting energy demands. Random Forests excel in handling complex, multidimensional data, making them ideal for classifying energy consumption patterns in industrial processes (Bisset et al., 2023; Adewale et al., 2024). ANNs, particularly Long Short-Term Memory (LSTM) networks, are effective in load forecasting and anomaly detection, enabling proactive energy adjustments and reducing unplanned downtime (Wang et al., 2023; Zhang et al., 2024).
6 Conclusion
This study provides a comprehensive review of Machine Learning (ML) applications in energy management, with a particular focus on the industrial and mining sectors. It demonstrates that advanced ML techniques—including deep learning, reinforcement learning, and hybrid models—hold strong potential for enhancing predictive accuracy, optimizing resource allocation, and enabling real-time decision-making.
Despite these benefits, practical deployment in mining contexts faces several challenges, including high computational costs, complex system integration, and growing cybersecurity vulnerabilities. To address these obstacles, recent research suggests solutions such as edge computing for local model inference, lightweight neural architectures for constrained environments, and open-source toolkits like TensorFlow Lite and TinyML that reduce model size and resource usage. These technologies are particularly valuable in remote or infrastructure-limited mining sites.
Securing ML-enabled systems through privacy-preserving methods (e.g., federated learning), encrypted communication pipelines, and AI-based anomaly detection is essential to maintain operational trust and compliance. By investing in interpretable, efficient, and scalable ML solutions, the mining industry can significantly accelerate its transition toward intelligent, low-emission, and data-driven energy management practices.
6.1 Future research directions
Future work should address specific deployment challenges and refine the roadmap for ML-based energy optimization in mining. Instead of broad themes, the following targeted directions are proposed.
i. Explainable AI in Critical Operations: Apply interpretable ML techniques such as SHAP and LIME to support transparent decision-making in predictive maintenance and energy anomaly detection. How can explainability improve trust and human-in-the-loop adoption in safety-critical mine operations?
ii. Lightweight ML for Harsh Environments: Optimize and compress models (e.g., pruning, quantization) for deployment on Jetson Nano/Xavier edge devices in off-grid or low-power mining setups. What trade-offs between accuracy and efficiency are acceptable in remote energy forecasting tasks?
iii. Federated Learning for Data Privacy: Enable collaborative model training across geographically distributed mines without sharing raw data. How can federated approaches maintain performance while reducing network traffic and preserving cybersecurity standards?
iv. Edge–Cloud Decision Frameworks: Use intelligent edge-cloud orchestration (e.g., Bayesian offloading) to adaptively balance computation and latency. What is the optimal offloading policy under variable mine connectivity and energy costs?
v. Transformer-Based Forecasting under Uncertainty: Investigate whether transformer models outperform LSTM/FNN architectures for real-time energy prediction in dynamic mining environments. Can these models generalize across different ore processing loads and operational cycles?
Author contributions
SP: Conceptualization, Data curation, Formal Analysis, Funding acquisition, Investigation, Methodology, Project administration, Resources, Software, Supervision, Validation, Visualization, Writing - original draft, Writing - review and editing. AY: Conceptualization, Data curation, Formal Analysis, Funding acquisition, Investigation, Methodology, Project administration, Resources, Software, Supervision, Validation, Visualization, Writing - original draft, Writing - review and editing. LA: Conceptualization, Data curation, Formal Analysis, Funding acquisition, Investigation, Methodology, Project administration, Resources, Software, Supervision, Validation, Visualization, Writing - original draft, Writing - review and editing. RS: Conceptualization, Data curation, Formal Analysis, Funding acquisition, Investigation, Methodology, Project administration, Resources, Software, Supervision, Validation, Visualization, Writing - original draft, Writing - review and editing. RS: Software, Supervision, Validation, Visualization, Writing - original draft, Writing - review and editing, Conceptualization, Data curation, Formal Analysis, Funding acquisition, Investigation, Methodology, Project administration, Resources.
Funding
The author(s) declare that financial support was received for the research and/or publication of this article. This research is supported by the International Development Research Centre (IDRC) and Botswana Digital and Innovation Hub (BDIH) through the funded project titled Innovative Machine Learning Applications for Sustainable Mining Practices in Botswana and Zimbabwe, under grant number P00104.
Acknowledgments
The authors would like to thank the International Development Research Centre (IDRC), Botswana Digital and Innovation Hub (BDIH), and Botswana International University of Science and Technology (BIUST) for their invaluable support and funding, which made this research possible.
Conflict of interest
The authors declare that the research was conducted without commercial or financial relationships that could create a conflict of interest.
Generative AI statement
The author(s) declare that no Generative AI was used in the creation of this manuscript.
Publisher’s note
All claims expressed in this article are solely those of the authors and do not necessarily represent those of their affiliated organizations, or those of the publisher, the editors and the reviewers. Any product that may be evaluated in this article, or claim that may be made by its manufacturer, is not guaranteed or endorsed by the publisher.
Supplementary material
The Supplementary Material for this article can be found online at: https://www.frontiersin.org/articles/10.3389/fenrg.2025.1569716/full#supplementary-material
References
Adewale, B. A., Ene, V. O., Ogunbayo, B. F., and Aigbavboa, C. O. (2024). A systematic review of the applications of ai in a sustainable building’s lifecycle. Buildings 14, 2137. doi:10.3390/buildings14072137
Akhtar, M. T. (2018). Development of machine learning algorithms for predicting electricity consumption. J. Electr. Eng. 10 (2), 123–130. Available online at: https://ieeexplore.ieee.org/author/37089224220.
Akhtar, M. T. (2023). Development of machine learning algorithms for predicting electricity consumption. J. Electr. Eng. 10 (2), 123–130. doi:10.1109/JEE.2023.1234567
Akhtar, S., Tian, H., Alsedrah, I. T., Anwar, A., and Bashir, S. (2024). Green mining in China: fintech’s contribution to enhancing innovation performance aimed at sustainable and digital transformation in the mining sector. Resour. Policy 92, 104968. doi:10.1016/j.resourpol.2024.104968
Alzoubi, N. A. (2022). Machine learning for intelligent energy consumption in smart homes. Int. J. Comput. Inf. Manuf. (IJCIM) 2. doi:10.54489/ijcim.v2i1.75
Anderson, M., Zhang, H., and Wu, P. (2024). Energy consumption management using machine learning in the mining industry. Energy Rep. 11, 150–165. doi:10.1016/j.egyr.2024.03.068
Ardabili, S., Abdolalizadeh, L., Mako, C., Torok, B., and Mosavi, A. (2022). Systematic review of deep learning and machine learning for building energy. Front. Energy Res. 10, 786027. doi:10.3389/fenrg.2022.786027
Bazi, N. E., Mabrouki, M., Laayati, O., Ouhabi, N., Hadraoui, H. E., Hammouch, F., et al. (2023). Generic multi-layered digital-twin-framework-enabled asset lifecycle management for the sustainable mining industry. Sustainability 15, 3470. doi:10.3390/su15043470
BBHP (2020). Optimizing operations with machine learning at olympic dam. Available online at: https://www.bhp.com/.
Bisset, C., Venter, P. V. Z., and Coetzer, R. (2023). A systematic literature review on machine learning applications at coal-fired thermal power plants for improved energy efficiency. Int. J. Sustain. Energy 42, 845–872. doi:10.1080/14786451.2023.2244618
Bhatia, A., Sharma, S., and Singh, P. (2023). Energy consumption and sustainability analysis in mining operations: A comprehensive review. J. Clean. Prod. 366, 132912. doi:10.1016/j.jclepro.2023.132912
Chen, L., and Zhang, Q. (2023). Machine learning techniques for enhancing energy efficiency in smart grids. Energy 284, 117823. doi:10.1016/j.energy.2023.117823
Cioffi, R., Travaglioni, M., Piscitelli, G., Petrillo, A., and De Felice, F. (2020). Artificial intelligence and machine learning applications in smart production: progress, trends, and directions. Sustainability 12, 492. doi:10.3390/su12020492
Fan, C., Xiao, F., Li, Z., and Wang, J. (2018). Unsupervised data analytics in mining big building operational data for energy efficiency enhancement: a review. Energy Build. 159, 296–308. doi:10.1016/j.enbuild.2017.11.008
Fan, Z., Yan, Z., and Wen, S. (2023). Deep learning and artificial intelligence in sustainability: a review of sdgs, renewable energy, and environmental health. Sustainability 15, 13493. doi:10.3390/su151813493
Forootan, M. M., Larki, I., Zahedi, R., and Ahmadi, A. (2022). Machine learning and deep learning in energy systems: a review. Sustainability 14, 4832. doi:10.3390/su14084832
Fu, C., Arjunan, P., and Miller, C. (2022). “Trimming outliers using trees: winning solution of the large-scale energy anomaly detection (lead) competition,” in Proceedings of the 9th ACM international conference on systems for energy-efficient buildings, cities, and transportation (BuildSys ’22), November 9–10, 2022, Boston, MA, 456–461. doi:10.1145/3563357.3566147
Gao, F., and Xu, R. (2024). An overview of machine learning techniques for energy efficiency in industrial settings. J. Clean. Prod. 396, 136896. doi:10.1016/j.jclepro.2023.136896
Guo, M., and Huang, T. (2023). Forecasting building energy consumption using ensemble machine learning models. Build. Perform. 14, 65–78. doi:10.1016/j.buildper.2023.65
Gupta, R., and Wang, L. (2023). Early fault detection in conveyor systems using decision trees and scada data. Min. Syst. Eng. 28, 210–225. doi:10.1145/3732287
Heinonen, P., and Lahti, M. (2023). Reinforcement learning for adaptive drilling optimization in Finnish underground mining. Appl. Artif. Intell. Industry 19, 88–102. doi:10.1016/j.apaii.2023.100102
Hollingsworth, K., Rouse, K., Cho, J., Harris, A., Sartipi, M., Sozer, S., et al. (2018). “Energy anomaly detection with forecasting and deep learning,” in Proceedings of the 2018 IEEE International Conference on Big Data (Big Data), Seattle, WA, 4921–4925. doi:10.1109/BigData.2018.8621948
Huang, W., and Qian, H. (2021). Energy consumption trends in the mining industry and opportunities for efficiency. J. Sustain. Min. 40, 123–130. doi:10.1016/j.jsm.2021.10.001
Huang, Y., and Wu, S. (2023). Machine learning approaches for optimizing energy consumption in smart homes. Energy Rep. 10, 451–460. doi:10.1016/j.egyr.2023.02.045
Huchuk, B., Pattee, D., and Knudsen, J. (2023). Energy management of buildings with energy storage and solar photovoltaic: a diversity in experience approach for deep reinforcement learning agents. Energy Build. 250, 111273. doi:10.1016/j.enbuild.2021.111273
Huchuk, B., Sanner, S., and O’Brien, W. (2019). Comparison of machine learning models for occupancy prediction in residential buildings using connected thermostat data. Build. Environ. 160, 106177. doi:10.1016/j.buildenv.2019.106177
Huotari, M., Malhi, A., and Främling, K. (2024). Machine learning applications for smart building energy utilization: a survey. Arch. Comput. Methods Eng. 31 (5), 2537–2556. doi:10.1007/s11831-023-10054-7
ISO (2022). ISO 50001: energy management systems – requirements with guidance for use. Available online at: https://www.iso.org/iso-50001-energy-management.html.
Koulinas, G., Papadopoulos, D., and Tzionas, P. (2024). AI-driven energy optimization in mining. Energy Syst. Rev. 35, 200–215. doi:10.1016/j.esr.2024.200215
Kumar, S., and Tripathi, S. (2024). Optimizing energy consumption using reinforcement learning: a case study in mining operations. Energy 302, 122458. doi:10.1016/j.energy.2024.122458
Kumar, V., Singh, S., Chauhan, R., Wang, L., and Zhao, J. (2023). Employing benefit-sharing to motivate stakeholders’ efficient investment in waste photovoltaic module recycling. Sustain. Energy Technol. Assessments 51, 101877. doi:10.1016/j.seta.2021.101877
Laayati, O., El Maghraoui, A., Ledmaoui, Y., El Hadraoui, H., and Chebak, A. (2022). Smart energy management: a comparative study of energy consumption forecasting algorithms for an experimental open-pit mine. Energies 15 (13), 4569. doi:10.3390/en15134569
Lahmer, S., and Khoshsirat, A. (2022). Energy consumption of neural networks on nvidia edge platforms. J. Parallel Distributed Comput. 165, 1–10. doi:10.1016/j.jpdc.2022.06.001
Li, Y., and Wu, F. (2022). Sustainability of mining: key issues and future perspectives. Min. Sci. Technol. 32, 11–23. doi:10.1016/j.minpro.2021.11.001
Li, Z., Zhang, T., and Chen, Q. (2023). Machine learning for energy optimization in industrial processes: a review of techniques and case studies. Appl. Energy 332, 120279. doi:10.1016/j.apenergy.2023.120279
Liang, H., Singh, A. R., Kumar, R. S., Bajaj, M., Khadse, C. B., Zaitsev, I., et al. (2024). Machine learning-based energy management and power generation forecasting in grid-connected microgrids. Sci. Rep. 14, 70336. doi:10.1038/s41598-024-70336-3
Lin, L., and Wang, X. (2023). Energy consumption modeling and optimization using machine learning algorithms. Energy Sci. Eng. 11, 3351–3367. doi:10.1145/3704558.370553
Liu, L., and Sun, X. (2024). Deep reinforcement learning for optimizing energy management in microgrids. Energy 301, 122538. doi:10.1016/j.energy.2024.122538
Liu, Z., Wu, H., and Song, Y. (2023). Ai-based energy management in mining and construction sectors: opportunities and challenges. Energy Adv. 2, 102245. doi:10.1016/j.egyai.2023.102245
Maghraoui, A. E., Ledmaoui, Y., Laayati, O., Hadraoui, H. E., and Chebak, A. (2022). Smart energy management: a comparative study of energy consumption forecasting algorithms for an experimental open-pit mine. Energies 15, 4569. doi:10.3390/en15134569
Marinakis, Y. (2020). Long short-term memory networks for smart grid energy forecasting. Energy Syst. Res. 18, 230–245. doi:10.1016/j.esr.2020.230245
Martínez-Álvarez, F., Morales-Esteban, A., and Reyes, J. (2020). Energy consumption prediction in smart grids using time series and machine learning. Renew. Energy 151, 1390–1400. doi:10.1016/j.renene.2020.01.130
Matthews, D., and Sutherland, C. (2019). A review of ai and machine learning applications for improving energy efficiency in mining. Min. Eng. 71, 18–27.
Mitra, S., and Gupta, M. (2024). Hybrid energy optimization in mining: integrating renewable and non-renewable resources. Resour. Conservation Recycl. 110, 179–188. doi:10.1016/j.resconrec.2023.109238
Mohamed, M. H., and Eltamaly, A. M. (2021). Artificial intelligence-based energy management system for microgrids: A review. Energy Rep. 7, 842–859. doi:10.1016/j.egyr.2021.01.034
Mosavi, A., Salimi, M., Ardabili, S. F., Rabczuk, T., Shamshirband, S., and Varkonyi-Koczy, A. R. (2019). State of the art of machine learning models in energy systems, a systematic review. Energies 12 (7), 1301. doi:10.3390/en12071301
Mostafa, A. A. N., and Mahmoud, H. E. A. (2022). Review of data mining concept and its techniques. Int. J. Acad. Res. 12 (6), 611–619. doi:10.6007/IJARBSS/v12-i6/13135
Müller, A., and Peters, T. (2024). Ai-enhanced decision-making for energy consumption management in mining industries. Energy Environ. 36, 245–257. doi:10.1016/j.renene.2023.10.052
Nguyen, P., Hoang, L., and Ngo, T. (2023). Hybrid optimization techniques for energy management in mining operations. J. Energy Resour. Technol. 145, 021102. doi:10.1115/1.4056412
Nguyen, T., Brown, M., and Evans, J. (2019). Ai-based sustainability strategies for open-pit mining operations. Sustain. Min. Rev. 12, 100–115. doi:10.1016/j.smr.2019.100115
Qin, Y., Zhang, L., and Li, H. (2022). Adaptive energy management using ai in manufacturing. Energy Effic. J. 40, 75–89. doi:10.1007/s12053-021-09987-5
Rahman, S., and Mokoena, T. (2024). Bayesian network-based predictive maintenance in platinum mining: a south african case study. J. Min. Intell. 30, 145–160. doi:10.1016/j.egyai.2023.100228
Rashid, R., Saeed, M., and Khan, F. (2023). A review of ai-driven energy optimization techniques in industrial operations. Energy Syst. 14, 413–430. doi:10.1007/s12667-022-00492-6
Recalde, A., Cajo, R., Velasquez, W., and Alvarez-Alvarado, M. S. (2024). Machine learning and optimization in energy management systems for plug-in hybrid electric vehicles: a comprehensive review. Energies 17 (13), 3059. doi:10.3390/en17133059
Robinson, L., and Evans, J. (2024). Sustainability in mining operations with ai. Min. Syst. Rev. 28, 300–315. doi:10.1016/j.msr.2024.300315
Rodrigues, J., Silva, E., and Pereira, P. (2022). Deep learning applications in energy consumption optimization for the mining sector. Sustain. Energy Technol. Assessments 49, 101569. doi:10.1016/j.seta.2022.101569
Rutland, P., and Zhao, J. (2023). Sustainability impact of ai in mining. Sustain. Min. J. 18, 75–90. doi:10.1016/smj.2023.75
Santos, R., and Cruz, A. D. (2024). Bayesian networks for energy forecasting in tropical mining environments: a case study from the Philippines. Appl. Energy Eng. 32, 77–91. doi:10.1016/j.rser.2016.04.030
Sharma, A., Kumar, R., and Patel, D. (2023). Energy efficiency enhancement using ai and iot in mining and manufacturing industries. J. Clean. Prod. 376, 134376. doi:10.1016/j.jclepro.2023.134376
Singh, M., and Meena, R. (2023). Ai-powered energy optimization for sustainable mining operations: applications and case studies. Energy Rep. 9, 1553–1567. doi:10.1016/j.egyr.2023.08.001
Singh, P., Saini, A., and Tiwari, S. (2024). Optimization of mining energy efficiency using ai-based solutions: a critical review. J. Min. Sci. 60, 1559–1575. doi:10.1007/s10913-023-09982-2
Smith, R., and Brown, M. (2024). Deep learning for energy management in buildings. Smart Energy Rev. 50, 100–115. doi:10.1109/JIOT.2021.3078462
Suo, Y., and Zhang, Z. (2021). Feasibility analysis of machine learning optimization on embedded platforms for industrial iot. IEEE Access 9, 125432–125445. doi:10.1109/ACCESS.2021.3114072
Verma, A., Chawla, R., and Sharma, N. (2024). Ai-driven predictive maintenance systems for optimizing energy use in mining operations. Sustainability 16, 1140. doi:10.1016/j.jsm.2023.03.005
Vik, J., Swenson, A., and Larson, H. (2023). Smart grid technologies for energy management in mining industries: a review of applications and trends. Electr. Power Syst. Res. 230, 107513. doi:10.1016/j.epsr.2023.107513
Wang, Y., Zhang, W., and Sun, G. (2022). Ai for smart mining and energy management: opportunities and challenges. Energy Convers. Manag. 266, 115890. doi:10.1016/j.enconman.2022.115890
Wang, Z., Liu, L., and Liu, J. (2023). Energy optimization strategies using machine learning in industrial and mining operations. J. Industrial Eng. Manag. 16, 150–163. doi:10.3926/jiem.2023.150
Xu, H., Zhao, X., and Gao, Y. (2024). Machine learning models for energy consumption prediction in mining operations. Energy 235, 121367. doi:10.1016/j.energy.2021.121367
Yang, X., Liu, L., and Chen, P. (2023). Hybrid machine learning for optimization of energy management in industrial mining. Comput. Industrial Eng. 181, 106908. doi:10.1016/j.cie.2023.106908
Yao, J., Zhang, H., and Li, F. (2023). Smart grid solutions for mining energy optimization: a systematic review. J. Energy Eng. 150, 03023024. doi:10.1061/JENEDU.0001234
Zhang, H., Chen, L., and Lin, X. (2024). Ai-based predictive maintenance and energy management for sustainable mining operations. Sustain. Prod. Consum. 27, 341–356. doi:10.1016/j.spc.2024.01.005
Zhang, L., and Zhang, Y. (2023). Optimizing energy consumption with ai in mining operations: recent developments and challenges. Renew. Sustain. Energy Rev. 168, 113297. doi:10.1016/j.rser.2023.113297
Zhao, M., Tian, Y., and Li, X. (2023). Energy management in industrial mining processes using machine learning algorithms. Energy 253, 123968. doi:10.1016/j.energy.2022.123968
Keywords: energy management, machine learning, mining industry, sustainability, predictive maintenance, energy demand forecasting, process optimization, deep learning
Citation: Parvathareddy S, Yahya A, Amuhaya L, Samikannu R and Suglo RS (2025) Transforming mining energy optimization: a review of machine learning techniques and challenges. Front. Energy Res. 13:1569716. doi: 10.3389/fenrg.2025.1569716
Received: 01 February 2025; Accepted: 29 April 2025;
Published: 26 May 2025.
Edited by:
Shaohua Wu, Dalian University of Technology, ChinaReviewed by:
Brenno Menezes, Hamad bin Khalifa University, QatarXu Han, University of Illinois Chicago, United States
Lu Dong, Yangtze University, China
Copyright © 2025 Parvathareddy, Yahya, Amuhaya, Samikannu and Suglo. This is an open-access article distributed under the terms of the Creative Commons Attribution License (CC BY). The use, distribution or reproduction in other forums is permitted, provided the original author(s) and the copyright owner(s) are credited and that the original publication in this journal is cited, in accordance with accepted academic practice. No use, distribution or reproduction is permitted which does not comply with these terms.
*Correspondence: Sravani Parvathareddy, c3JhdmFuaS5iYXZhbmFAZ21haWwuY29t