- 1Department of Archaeology and Anthropology, Bristol University, Bristol, UK
- 2Department of Geography, Durham University, Durham, UK
- 3Institute of Hazard, Risk and Resilience, Durham University, Durham, UK
- 4School of Biological and Biomedical Sciences, Durham University, Durham, UK
- 5Department of Mathematical Sciences, Durham University, Durham, UK
- 6Durham University Business School, Durham, UK
- 7Department of English Studies, Durham University, UK
- 8Palatine Centre, Durham Law School, Durham University, Durham, UK
- 9Department of History, Durham University, Durham, UK
- 10The York Management School, University of York, York, UK
Recently, Early Warning Signals (EWS) have been developed to predict tipping points in Earth Systems. This discussion highlights the potential to apply EWS to human social and economic systems, which may also undergo similar critical transitions. Social tipping points are particularly difficult to predict, however, and the current formulation of EWS, based on a physical system analogy, may be insufficient. As an alternative set of EWS for social systems, we join with other authors encouraging a focus on heterogeneity, connectivity through social networks and individual thresholds to change.
1. Introduction
Do human social and economic systems undergo critical transitions similar to those observed in ecological systems? The question has potential to advance a discussion that tends to treat environmental change as an exogenous force for societal change; e.g., the “collapse” of ancient civilizations (Shimkin, 1973; Cullen et al., 2000; Kuper and Kröpelin, 2006; Rull et al., 2013; Tierney and DeMenocal, 2013) or future change in food supply, population dynamics, labor capacity or likelihood of conflict in modern societies (White et al., 2006; Battisti and Naylor, 2009; Schlenker and Roberts, 2009; Welch et al., 2010; Hsiang et al., 2013; Dunne et al., 2013).
Recent discussion in ecology and evolutionary studies has focussed specifically on “tipping points” in Earth systems (Hughes et al., 2013; Lenton and Williams, 2013). Enough is now known about the climate and Earth systems to evoke serious concern, though not certainty (Brook et al., 2013), that parameters for humanity's environmentally safe and sustainable activity may be exceeded in this century (Lenton et al., 2008; Rockström et al., 2009; Barnosky et al., 2012; Ehrlich and Ehrlich, 2013; Hughes et al., 2013; Lenton and Williams, 2013). These perspectives are associated with the observation that Earth systems can behave non-linearly and switch relatively abruptly between different modes of operation, potentially within a human lifetime. As these natural tipping points become more starkly recognized —as in a recent Intergovernmental Panel on Climate Change report (IPCC, 2013) or accounts of inevitability of West Antarctic Ice Sheet collapse (Joughin et al., 2014) —the challenge turns to understanding how societies may respond to their anticipation of environmental change.
Because language and socio-political activity are closely correlated, how we collectively define and discuss planetary tipping points is important (O'Neill and Nicholson-Cole, 2009; Lenton and Williams, 2013). In physical and mathematical sciences, precise expressions are used to represent transitions between phases of matter, waves and networks (Bissell and Straughan, in press). Studies of Earth Systems, however, often apply a more general definition of a tipping point as one where “a small perturbation can cause a qualitative change in the future state of a system,” (Lenton and Williams, 2013), e.g., “the flip of a complex dynamical system from one state to another” (May et al., 2008) or simply the point where the system “response (to changing conditions) becomes nonlinear or the rate of change alters steeply” (Brook et al., 2013).
Recent discussion of tipping points in Earth Systems has developed the tools for detecting Early Warning Signals (EWS) in observational environmental variables (time series data) and identifying the causal drivers of abrupt change, including connectivity and homogeneity/heterogeneity of systems, associated cascade effects, and feedback (Barnosky et al., 2012; Scheffer et al., 2012; Brook et al., 2013; Hughes et al., 2013; Lenton and Williams, 2013). The tempo of “abrupt” change in Earth Systems has a vast range, from momentary to human lifetimes to millennia and geological time scales, operating on global, local or microscale individual components of systems (Brook et al., 2013; Hughes et al., 2013).
In the spirit of finding “common ground” between social systems and ecosystems (May et al., 2008), we are optimistic about EWS for understanding and anticipating societal responses to environmental change, but we also caution, however, against applying EWS from natural systems directly to societal systems.
1.1. Early Warning Signals (EWS) for Natural Tipping Points
Research on EWS for tipping points in Earth Systems has developed toolkits to forecast change. These data-driven approaches seek to define EWS in time series data for critical transitions (Scheffer et al., 2012). The set of EWS criteria includes (Scheffer et al., 2009):
• A “critical slowing down,” where the response time to an external perturbation takes longer and longer to return to the steady state, the tipping point being when it ultimately never returns
• Increasing autocorrelation within the time series, as a correlation between subsequent states
• A skewing of the distribution of data when the time axis is collapsed
• A “flickering” between discrete values of the system variable as the system approaches a bifurcation
In the early 1990s—when the term “flickering” was first prominently applied to describe past climate change (Taylor et al., 1993)—the then-new ice core evidence revealed a surprising abruptness of Quaternary climate transitions, on scales as short as decadal and not all with obvious external forcing events (Alley et al., 1993; Cuffey et al., 1995). Since then, EWS have become more frequently and consistently applied to ecological and climatological data (Dakos et al., 2008; Drake and Griffen, 2010; Scheffer et al., 2012; Wang et al., 2012). In our own new lake sediment cores from Greenland and Finnmark (Huntley et al., 2013), for example, we find possible flickering in two different climate proxies pollen and chironomid (non-biting midge) remains that may qualify as EWS for the pronounced Neoglacial cooling event approximately 4000 years ago in the North Atlantic region.
A common model of underlying causality for EWS implies the metaphor of a complex system as a physical entity moving into a potential energy well (Scheffer et al., 2009; Lenton, 2011; Scheffer et al., 2012). As conveyed, for example, by the cover of Trends in Ecology and Evolution in July 2013 (Figure 1A), this analogy to a “potential well” has been shown to be useful to model the observed behavior of natural systems under precisely definable conditions (Figure 1B).
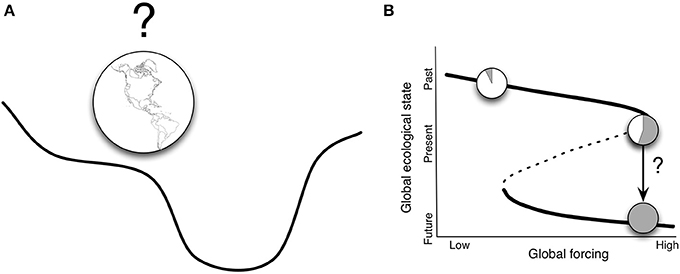
Figure 1. Two representations of tipping points in Earth systems. (A) An adaptation of the cover image of Trends in Ecology and Evolution, July 2013. (B) Adapted representation of a tipping point in Earth system ecological capacity, as a fold bifurcation with hysteresis (Barnosky et al., 2012), with pie charts representing world human presence as fraction of global capacity. The question marks suggest the uncertainty of an impending tipping point for Earth Systems.
In simplified terms, the EWS toolkit can be illustrated in terms of the time t for a perturbed variable x to return to equilibrium (Scheffer et al., 2009):
This has two equilibria of opposing stability, one at x = a and another at x = b (here γ is a positive factor). The potentials are smooth, so the characteristic rate-of-return to equilibrium “−” (or departure “+”) is λ± = ±γ(a − b). As depicted in Figure 1A, this “potential well” analogy implies that a system approaching a tipping point may spend more time in the direction of the alternative attractor it is going to switch to than in the opposite direction, where the potential is steeper (Scheffer et al., 2009; Lenton, 2011). This yields in skewing of the statistical distribution of the data, and also critical slowing down as the system approaches a bifurcation a = b, i.e., as λ → 0 and the return-time diverges (1/λ → ∞) (Scheffer et al., 2009). “Flickering” between multiple attractors can occur where variability is high (either internally, or through the noise in the amplitude of the forcing mechanism), which may be why variance can perform better than the first order autocorrelation coefficient as an EWS (Carpenter and Brock, 2006; Brock and Carpenter, 2010, 2012).
1.2. EWS for Societal Tipping Points?
Although yet not as widely used as in natural systems, efforts to apply EWS to social systems are increasing (Scheffer, 2009; Haldane and May, 2011; Neuman et al., 2011; Saavedra et al., 2011; Kuehn et al., 2013; Moat et al., 2013; Barrett and Dannenberg, 2014; Kallus, 2014, ch.12) As the “potential well” analogy in Figure 1A becomes a common visual metaphor, however, we caution that this could prove misleading in efforts to identify EWS for social transitions, especially through post-hoc reasoning (Roberts and Winters, 2013). To take an example, time series of Twitter activity are claimed to exhibit EWS (including rising variance and autocorrelation) that predict highly anticipated holidays like Halloween and Christmas (Kuehn et al., 2013). Arguably, however, it is not the EWS in Twitter time series data that is predictive, but simply the public knowledge of holidays that drives up the number of relevant tweets by orders of magnitude and correspondingly increases the variance (scaling proportional to frequency squared) and lag-1 autocorrelation. A more direct, alternative EWS may be generated by harvesting the “collective wisdom” of current social media content, rather than examining time trends (Lampos and Cristianini, 2012; Kallus, 2014).
Furthermore, many societal tipping points register with neither EWS nor current content. For an historical example, consider the history of banks, where we find a rather remarkable, abrupt change in the year 1810, with seemingly no EWS at all (Garnett et al., in press). In the late 18th century, hundreds of small private banks operated independently in England and, prior to 1810, their numbers had increased by about 2.7% annually for over 150 years (Figure 2A). After 1810, the population of British banks suddenly went into consistent decline of approximately 1.2% per year for the subsequent two centuries (Figure 2A). There are possible post-hoc historical explanations, but the fact remains that, in the early 1800s, no observers recorded anything remarkable that would have predicted either this sharp peak in bank number or its subsequent steady decline for two centuries.
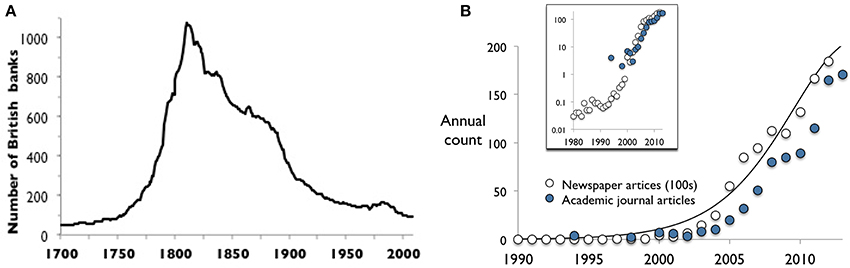
Figure 2. Time scales of social tipping points. (A) The changing population of British banks from 1700 to 2009. Prior to 1810 the bank population increased by 2.7% annually. Post 1810 the population went into a systematic decline of approximately 1.2% per year for the next 200 years (Garnett et al., in press). (B) The popularity of “tipping points.” The number of English-language newspaper (LexisNexis Database) articles (in hundreds) which use the term “tipping point” from 1990 to 2013 (white circles), compared to the number of academic journal articles (ISI Web of Science) using “tipping point” or its plural (blue filled circles). The black curve is a classic social diffusion model (e.g., Bentley et al., 2012) fitted to the newspaper data. The inset shows the data with logarithmic y-axis.
EWS in time series may be unhelpful for predicting societal tipping points partly because the physical system analogy of Figure 1A is inappropriate. It was not part of the original research on tipping points in sociology of the 1950s. That work originated among a community of specialists on the dynamics of racial segregation in urban housing and planning (Grodzins, 1957), focused on neighbor interactions in space and individual, threshold responses to perceived conditions. In the famous segregation model of Schelling (1969), the crucial parameter was the individual threshold that agents had for being the minority among their immediate neighbors. Contemporaneous work on the instability of complex systems with feedbacks (May, 1972) inspired greater study, over the decades, of how seemingly straightforward social interventions may cascade into unanticipated consequences (e.g., Gibson and Gurmu, 2012).
2. Communicating Tipping Points
The metaphor of the “potential well” may be useful for understanding societal system change, but in different ways than for environmental systems. For example, public perception of iconic imagery like Figure 1A, and the emotive term “tipping point,” might influence public behaviors in ways that affect the dynamics of the whole system (e.g., ideas applied to climate change might provoke a social movement toward more sustainable human activity). Thus, “tipping point” models may be beneficial if environmental modeling assumptions are well stated and system-state bifurcation curves are clearly justified. They have the potential, however, to obscure public understanding when more speculative. Furthermore, social reaction to such knowledge may not display smooth potentials, so “tipping behavior” in social systems can be much harder to predict (Abrahamson and Eisenman, 2008; Hastings and Wysham, 2010).
The ecological debate over tipping points has implications, then, not just for communicating science, but in feedback from the public domain concerning future environmental change (Risbey, 2008; Carpenter et al., 2012). Should we be more cognisant that the tipping point concept has acquired different definitions among communities with vested interests in future migration, economic investment and politics? With respect to financial crises, for example, knowledge about the tipping point itself may affect how the crisis plays out, but it does not necessarily make these crises more predictable (Diamond and Dybvig, 1983; Scheffer et al., 2009; Akinbami, 2013; Hollow, 2014).
The use of tipping point has grown exponentially since about 2000 (Figure 2B), the year when The Tipping Point by Gladwell (2000) became a bestseller. The diffusion pattern in newspapers and in academic journal articles is quite similar, and we can soon expect “tipping point” to decline in popularity as it progresses along the fashion cycle (Clark, 2004; Acerbi et al., 2012; Bentley et al., 2012). Given the timing of the diffusion in public media, slightly leading the growth in academic usage (by a longer gap than just academic review process), it seems the interest in tipping points in academic science, or at least the use of the term, was partly driven by media usage. Consider that on 10 Dec 1998, an Atlanta Journal and Constitution newspaper editorial reported:
Even confirmation that the Earth's warming this century is unprecedented for at least the previous 11 centuries does not prove that global warming has become a reality. But … scientists warn that if global warming is truly occurring, … complex systems such as climate tend to stay relatively stable for a long time, absorbing pressure for change until it reaches a tipping point. … Such rapid and abrupt climate change would make it difficult for plant and animal species to adapt.
Note how this media report first reassured readers that there was no proof that a critical transition had begun, before conveying a more alarming message including the term “tipping point.” This tipping point metaphor might be intended to convey “an image of crisis useful for registering public concern and opening avenues for response” (Russill, 2008). Simultaneously, however, an understated, conditional tone seems to be increasingly adopted by climate scientists in what has been called “erring on the side of least drama” (Brysse et al., 2013). The concern is that starker language about planetary boundaries (Risbey, 2008; Rockström et al., 2009; Ehrlich and Ehrlich, 2013) may increase fatalism and resignation (Gardiner, 2009). In a prominent climatologists opinion, for example, “If we say we passed thresholds and tipping points today, this will be an excuse for inaction tomorrow” (Revkin, 2009).
2.1. Communicating Uncertainty and Risk
On both sides of the public debate concerning climate change, knowledge of actual scientific consensus is far from perfect (Lewandowsky et al., 2013; Kahan, 2014). Even if public comprehension of climate science were excellent, however, the inherent scientific uncertainty of prediction still allows for cultural variations in how that risk is perceived (Kahan, 2014).
In other words, concern over climate change is at least as much a social network phenomenon as it is a rational decision based upon the existing evidence. For this reason, a way forward may be through mapping the parameter space of potential social cascades, rather than attempting to predict them. This “ecological” approach focuses on the connectivity and heterogeneity among systems of discrete, interacting agents, each with their own individual thresholds for conditions at which they are likely to change (May et al., 2008; Caccioli et al., 2009; Haldane and May, 2011; Garnett et al., in press; Picoli et al., 2014). In the application to a financial system, for example, banks are modeled as the agents, with inter-bank loans as their interconnections, and cash reserves as the threshold to each bank's failure (Brock et al., 2009; Haldane and May, 2011). The heterogeneity among the agents in how they learn and respond to information is key to the stability of the financial system (Brock et al., 2009).
In the abstract, this is similar to a model of information cascades across a social network, based on a classic experiment on social conformity (Asch, 1955), in which each agent has a threshold defined by the fraction of neighboring agents that would need to adopt an idea before she would adopt it Watts (2002). Each time an agent switches, this changes the friend circle for its surrounding agents and might cause one of them to switch, and so on, percolating through the “vulnerable cluster” of agents (Watts, 2002).
Rather than EWS in time series data, these approaches focus on interaction networks and individual thresholds for change (Barrett and Dannenberg, 2014), which can guide practical intervention efforts. This network approach has been applied for example in efforts to reduce the spread of street violence through the work of neighborhood volunteers who try to interrupt violent events before they happen (Skogan et al., 2008).
Concerning climate change, we might identify specific psychological thresholds for individuals and their thinking about the Earth's tipping points —as opposed to “aggregates” in the language of Individual-based Models (IBMs) (Grimm, 1999). As an illustration, when uncertainty about climate change (e.g., “By 2120, sea levels will rise between 60 and 170 cm”) is reframed as certainty but at an uncertain time (e.g., “Sea levels will rise by 100 cm, somewhere between 2090 and 2160”), people perceive a greater risk and alter their preferred management of that risk (Lewandowsky et al., 2013). In psychological experiments, people cooperate and avoid crossing a tipping point (2°C increase in temperatures) if it is known, but if there is uncertainty about it, then people are less inclined to cooperate, even if they understand the consequential “tragedy of the commons” (Barrett and Dannenberg, 2012; Lewandowsky et al., 2013). Another individual psychological threshold can be knowledge that others are cooperating (e.g., through democratic vote), which favors sustainable behavior (Hauser et al., 2014).
How scientists discuss tipping points seems crucial to social diffusion of public knowledge and response behaviors (O'Neill and Nicholson-Cole, 2009; Bentley et al., 2012). Analysis of group dynamics reveals that uninformed (Couzin et al., 2011), or informed but passive (Banerjee et al., 2013) members can crucially affect the diffusion of ideas, depending on network structures and prior conditions (Lieberman et al., 2005; Gross et al., 2009; Del Genio et al., 2011). Social decision-making can be sensitive to prior conditions (path dependent) and to the information passing through various connections across the whole network (Salganik et al., 2006; Couzin et al., 2011). Uninformed individuals may propagate “noise” that overwhelms the beneficial information offered by informed individuals. Thus the online data deluge may be so overwhelming that it prevents most of the population from picking up the beneficial information and making well informed decisions (Sparrow et al., 2011; Ben-Akiva et al., 2012; Giguère and Love, 2013; Bentley et al., 2014).
3. Conclusions
We have discussed to what extent the recent advances in predicting tipping points in natural (ecologic) systems can be replicated in social and economic systems. Whilst EWS provide testable predictions for natural or mechanistic systems approaching a bifurcation, we lack similar prediction methods for social systems. Given the inherent difficulties in applying a natural-systems model such as the “potential well” to social systems, it may be better to focus attention more toward probabilistic insights from research on collective social dynamics. These approaches identify measurable qualities of social systems or networks, such as heterogeneity, connectivity and individual-based thresholds, that make social tipping points more likely.
Although we have critiqued various uses of the tipping point metaphor in social systems, we maintain that the metaphor is crucial to framing, communication and group identity. For example, the IPCC (2013) reports that: “debate as to whether human influence could… even force parts of the climate system across critical thresholds or tipping points, causing irreversible change,” and that “there is little agreement on how likely they are in the 21st century or what the human consequences would be.”
The arguments presented above suggest that, hypothetically, we can understand the processes driving “human influence” of our environment better through research on public knowledge of, and reaction to evidence from “objective” observations of the ways that environmental systems can “tip” into an altered state. The existence of EWS provided by the scientific community may impact on human behavior in ways that scientists do not as yet fully understand.
Conflict of Interest Statement
The authors declare that the research was conducted in the absence of any commercial or financial relationships that could be construed as a potential conflict of interest.
Acknowledgment
The research underlying this review was funded by the Leverhulme Trust (UK) “Tipping Points” programme grant at Durham University.
References
Abrahamson, E., and Eisenman, M. (2008). Employee-management techniques: transient fads or trending fashions? Admin. Sci. Q. 53, 719–744. doi: 10.2189/asqu.53.4.719
Acerbi, A., Ghirlanda, S., and Enquist, M. (2012). The logic of fashion cycles. PLoS ONE 7:e32541. doi: 10.1371/journal.pone.0032541
Akinbami, F. (2013). Is meta-regulation all it's cracked up to be? The case of UK financial regulation. J. Bank Regul. 14, 16–32.
Alley, R. B., Meese, D. A., Shuman, C. A., Gow, A. J., Taylor, K. C., Grootes, P. M., et al. (1993). Abrupt increase in Greenland snow accumulation at the end of the Younger Dryas event. Nature 362, 527–529. doi: 10.1038/362527a0
Asch, S. E. (1955). Opinions and social pressure. Sci. Am. 193, 31–35. doi: 10.1038/scientificamerican1155-31
Banerjee, A., Chandrasekhar, A. G., Duflo, E., and Jackson, M. O. (2013). The diffusion of microfinance. Science 341:1236498. doi: 10.1126/science.1236498
Barnosky, A. D., Hadly, E. A., Bascompte, J., Berlow, E. L., Brown, J. H., Fortelius, M., et al. (2012). Approaching a state shift in Earth's biosphere. Nature 486, 52–58. doi: 10.1038/nature11018
Barrett, S., and Dannenberg, A. (2012). Climate negotiations under scientific uncertainty. Proc. Natl. Acad. Sci. U.S.A. 109, 17372–17376. doi: 10.1073/pnas.1208417109
Barrett, S., and Dannenberg, A. (2014). Sensitivity of collective action to uncertainty about climate tipping points. Nat. Climate Change 4, 36–39. doi: 10.1038/nclimate2059
Battisti, D. S., and Naylor, R. L. (2009). Historical warnings of future food insecurity with unprecedented seasonal heat. Science 323, 240–244. doi: 10.1126/science.1164363
Ben-Akiva, M., Palma, A., McFadden, D., Abou-Zeid, M., Chiappori, P.-A., Lapparent, M., et al. (2012). Process and context in choice models. Market. Lett. 23, 439–456. doi: 10.1007/s11002-012-9180-7
Bentley, R. A., Acerbi, A., Ormerod, P., and Lampos, V. (2014). Books average previous decade of economic misery. PLoS ONE 9:e83147. doi: 10.1371/journal.pone.0083147
Bentley, R. A., Garnett, P., O'Brien, M. J., and Brock, W. A. (2012). Word diffusion and climate science. PLoS ONE 7:e47966. doi: 10.1371/journal.pone.0047966
Bissell, J., and Straughan, B. (in press). Discontinuity waves as tipping points: Applications to biological and sociological systems. Discr. Contin. Dyn. Syst. B 19.
Brock, W. A., and Carpenter, S. R. (2010). Interacting regime shifts in ecosytems: implication for early warnings. Ecol. Monogr. 80, 353–367. doi: 10.1890/09-1824.1
Brock, W. A., and Carpenter, S. R. (2012). Early warnings of regime shifts when the ecosystem structure is unknown. PLoS ONE 7:e45586. doi: 10.1371/journal.pone.0045586
Brock, W. A., Hommes, C., and F, W. (2009). More hedging instruments may destabilize markets. J. Econ. Dyn. Control 33, 1912–1928. doi: 10.1016/j.jedc.2009.05.004
Brook, B. W., Ellis, E. C., Perring, M. P., Mackay, A. W., and Blomqvist, L. (2013). Does the terrestrial biosphere have planetary tipping points? Trends Ecol. Evol. 28, 396–401. doi: 10.1016/j.tree.2013.01.016
Brysse, K., Oreskes, N., O'Reilly, J., and Oppenheimer, M. (2013). Climate change prediction: erring on the side of least drama? Global Environ. Change 23, 327–337. doi: 10.1016/j.gloenvcha.2012.10.008
Caccioli, F., Marsili, M., and Vivo, P. (2009). Eroding market stability by proliferation of financial instruments. Eur. Phys. J. B 71, 467–479. doi: 10.1140/epjb/e2009-00316-y
Carpenter, S. R., Arrow, K. J., Barrett, S., Biggs, Reinette Brock, W. A., Crépin, A.-S., et al. (2012). General resilience to cope with extreme events. Sustainability 4, 3248–3259. doi: 10.3390/su4123248
Carpenter, S. R., and Brock, W. A. (2006). Rising variance: a Leading indicator of ecological transition. Ecol. Lett. 9, 311–318. doi: 10.1111/j.1461-0248.2005.00877.x
Clark, T. (2004). The fashion of management fashion: a surge too far? Organization 11, 297–306. doi: 10.1177/1350508404030659
Couzin, I. D., Ioannou, C. C., Demirel, G., Gross, T., Torney, C. J., Hartnett, A., et al. (2011). Uninformed individuals promote democratic consensus in animal groups. Science 334, 1578–1580. doi: 10.1126/science.1210280
Cuffey, K., Clow, G., and Alley, R. (1995). Large arctic temperature change at the Wisconsin-Holocene glacial transition. Science 270, 455–458. doi: 10.1126/science.270.5235.455
Cullen, H. M., DeMenocal, P. B., Hemming, S., Hemming, G., Brown, F. H., Guilderson, T., et al. (2000). Climate change and the collapse of the Akkadian empire: evidence from the deep sea. Geology 28, 379–382. doi: 10.1130/0091-7613(2000)28<379:CCATCO>2.0.CO;2
Dakos, V., Scheffer, M., van Nes, E. H., Brovkin, V., Petoukhov, V., and Held, H. (2008). Slowing down as an early warning signal for abrupt climate change. Proc. Natl. Acad. Sci. U.S.A. 105, 14308–14312. doi: 10.1073/pnas.0802430105
Del Genio, C. I., Gross, T., and Bassler, K. E. (2011). All scale-free networks are sparse. Phys. Rev. Lett. 107:178701. doi: 10.1103/PhysRevLett.107.178701
Diamond, D. W., and Dybvig, P. H. (1983). Bank runs, deposit insurance, and liquidity. J. Polit. Econ. 91, 401–419. doi: 10.1086/261155
Drake, J. M., and Griffen, B. D. (2010). Early warning signals of extinction in deteriorating environments. Nature 467, 456–459. doi: 10.1038/nature09389
Dunne, J. P., Stouffer, R. J., and John, J. G. (2013). Reductions in labour capacity from heat stress under climate warming. Nat. Climate Change 3, 1–4. doi: 10.1038/nclimate1827
Ehrlich, P. R., and Ehrlich, A. H. (2013). Can a collapse of global civilization be avoided? Proceedings of the Royal Society B 280:20122845. doi: 10.1098/rspb.2012.2845
Gardiner, S. M. (2009). Saved by disaster? Abrupt climate change, political inertia, and the possibility of an intergenerational arms race. J. Soc. Philos. 40, 140–162. doi: 10.1111/j.1467-9833.2009.01444.x
Garnett, P., Mollan, S., and Bentley, R. (in press). Complexity in history: modelling the organizational demography of the British banking sector. Bus. Hist. 56.
Gibson, M. A., and Gurmu, E. (2012). Rural to urban migration is an unforeseen impact of development intervention in Ethiopia. PLoS ONE 7:e48708. doi: 10.1371/journal.pone.0048708
Giguère, G., and Love, B. C. (2013). Limits in decision making arise from limits in memory retrieval. Proc. Natl. Acad. Sci. U.S.A. 110, 7613–7618. doi: 10.1073/pnas.1219674110
Gladwell, M. (2000). The Tipping Point: How Little Things Can Make a Big Difference. New York, NY: Little Brown.
Grimm, V. (1999). Ten years of individual-based modeling in ecology: what have we learned and what could we learn in the future? Ecol. Modell. 115, 129–148. doi: 10.1016/S0304-3800(98)00188-4
Grodzins, M. (1957). Metropolitan segregation. Sci. Am. 197, 33–41. doi: 10.1038/scientificamerican1057-33
Gross, T., Rudolf, L., Levin, S. A., and Dieckmann, U. (2009). Generalized models reveal stabilizing factors in food webs. Science 325, 747–750. doi: 10.1126/science.1173536
Haldane, A. G., and May, R. M. (2011). Systemic risk in banking ecosystems. Nature 469, 351–355. doi: 10.1038/nature09659
Hastings, A., and Wysham, D. B. (2010). Regime shifts in ecological systems can occur with no warning. Ecol. Lett. 13, 464–472. doi: 10.1111/j.1461-0248.2010.01439.x
Hauser, O. P., Rand, D. G., Peysakhovich, A., and Nowak, M. A. (2014). Cooperating with the future. Nature 511, 220–223. doi: 10.1038/nature13530
Hollow, M. (2014). Strategic inertia, financial fragility and organisational failure: the case of the Birkbeck Bank, 1870–1911. Bus. Hist. 56, 746–764, doi: 10.1080/00076791.2013.839660
Hsiang, S. M., Burke, M., and Miguel, E. (2013). Quantifying the influence of climate on human conflict. Science 341:1235367. doi: 10.1126/science.1235367
Hughes, T. P., Carpenter, S., Rockström, J., Scheffer, M., and Walker, B. (2013). Multiscale regime shifts and planetary boundaries. Trends Ecol. Evol. 28, 389–395. doi: 10.1016/j.tree.2013.05.019
Huntley, B., Long, A. J., and Allen, J. (2013). Spatio-temporal patterns in late-glacial and holocene vegetation and climate of finnmark, northernmost europe. Q. Sci. Rev. 70, 158–175. doi: 10.1016/j.quascirev.2013.03.006
IPCC. (2013). Climate Change: Action, Trends and Implications for Business. Intergovernmental Panel on Climate Change, Fifth Assessment Report. Cambridge University Press.
Joughin, I., Smith, B. E., and Medley, B. (2014). Marine ice sheet collapse potentially under way for the Thwaites Glacier Basin, West Antarctica. Science 344, 735–738. doi: 10.1126/science.1249055
Kahan, D. M. (2014). “Making climate-science communication evidence-based all the way down,” in Culture, Politics and Climate Change, eds D. A. Crow and M. T. Boykoff (New York, NY: Routledge Press), 203–220.
Kuehn, C., Martens, E., and Romero, D. (2013). Critical transitions in social network activity. arXiv preprint arXiv:1307.8250.
Kuper, R., and Kröpelin, S. (2006). Climate-controlled Holocene occupation in the Sahara. Science 313, 803–807. doi: 10.1126/science.1130989
Lampos, V., and Cristianini, N. (2012). Nowcasting events from the social web with statistical learning. ACM Trans. Intel. Syst. Technol. 3, 72. doi: 10.1145/2337542.2337557
Lenton, T. M. (2011). Early warning of climate tipping points. Nat. Climate Change 1, 201–209. doi: 10.1038/nclimate1143
Lenton, T. M., Held, H., Kriegler, E., Hall, J. W., Lucht, W., Rahmstorf, S., et al. (2008). Tipping elements in the earth's climate system. Proc. Natl. Acad. Sci. U.S.A. 105, 1786–1793. doi: 10.1073/pnas.0705414105
Lenton, T. M., and Williams, H. T. P. (2013). On the origin of planetary-scale tipping points. Trends Ecol. Evol. 28, 380–382. doi: 10.1016/j.tree.2013.06.001
Lewandowsky, S., Gignac, G. E., and Vaughan, S. (2013). The pivotal role of perceived scientific consensus in acceptance of science. Nat. Climate Change 3, 399–404. doi: 10.1038/nclimate1720
Lieberman, E., Hauert, C., and Nowak, M. A. (2005). Evolutionary dynamics on graphs. Nature 433, 312–316. doi: 10.1038/nature03204
May, R. M. (1972). Will a large complex system be stable? Nature 238, 413–414. doi: 10.1038/238413a0
May, R. M., Levin, S. A., and Sugihara, G. (2008). Complex systems: Ecology for bankers. Nature 451, 893–895. doi: 10.1038/451893a
Moat, H. S., Curme, C., Avakian, A., Kenett, D. Y., Stanley, H. E., and Preis, T. (2013). Quantifying Wikipedia usage patterns before stock market moves. Sci. Rep. 3:1801. doi: 10.1038/srep01801
Neuman, Y., Nave, O., and Dolev, E. (2011). Buzzwords on their way to a tipping-point: a view from the blogosphere. Complexity 16, 58–68. doi: 10.1002/cplx.20347
O'Neill, S., and Nicholson-Cole, S. (2009). “fear won't do it”: Promoting positive engagement with climate change through visual and iconic representations. Sci. Commun. 30, 355–379. doi: 10.1177/1075547008329201
Picoli, S., Castillo-Mussot, M. D., Ribeiro, H. V., Lenzi, E. K., and Mendes, R. S. (2014). Universal bursty behaviour in human violent conflicts. Sci. Reports 4:4773. doi: 10.1038/srep04773
Revkin, A. C. (2009, March 28). Among climate scientists, a dispute over ‘tipping points’. New York Times, p. 28. Available online at: www.nytimes.com/2009/03/29/weekinreview/29revkin.html.
Risbey, J. S. (2008). The new climate discourse: alarmist or alarming? Glob. Environ. Change 18, 26–37. doi: 10.1016/j.gloenvcha.2007.06.003
Roberts, S., and Winters, J. (2013). Linguistic diversity and traffic accidents: Lessons from statistical studies of cultural traits. PLoS ONE 8:e70902. doi: 10.1371/journal.pone.0070902
Rockström, J., Steffen, W., Noone, K., Persson, A., Chapin, F. S., Lambin, E. F., et al. (2009). A safe operating space for humanity. Nature 461, 472–475. doi: 10.1038/461472a
Rull, V., Cañellas Boltà, N., Sáez, A., Margalef, O., Bao, R., Pla-Rabes, S., et al. (2013). Challenging Easter Island's collapse: the need for interdisciplinary synergies. Front. Ecol. Evol. 1:3. doi: 10.3389/fevo.2013.00003
Russill, C. (2008). Tipping point forewarnings in climate change communication. Environ. Commun. 2, 133–153. doi: 10.1016/j.gloenvcha.2009.04.001
Saavedra, S., Duch, J., and Uzzi, B. (2011). Tracking traders' understanding of the market using e-communication data. PLoS ONE 6:e26705. doi: 10.1371/journal.pone.0026705
Salganik, M. J., Dodds, P. S., and Watts, D. J. (2006). Experimental study of inequality and unpredictability in an artificial cultural market. Science 311, 854–856. doi: 10.1126/science.1121066
Scheffer, M. (2009). Critical Transitions in Nature and Society. Princeton, NJ: Princeton University Press.
Scheffer, M., Bascompte, J., Brock, W. A., Brovkin, V., Carpenter, S. R., Dakos, V., et al. (2009). Early-warning signals for critical transitions. Nature 461, 53–59. doi: 10.1038/nature08227
Scheffer, M., Carpenter, S. R., Lenton, T. M., Bascompte, J., Brock, W., Dakos, V., et al. (2012). Anticipating critical transitions. Science 338, 344–348. doi: 10.1126/science.1225244
Schlenker, W., and Roberts, M. J. (2009). Nonlinear temperature effects indicate severe damages to U.S. crop yields under climate change. Proc. Natl. Acad. Sci. U.S.A. 106, 15594–15598. doi: 10.1073/pnas.0906865106
Shimkin, D. B. (1973). “Models for the downfall: some ecological and culture-historical considerations,” The Classic Maya Collapse, ed T. P. Culbert (Albuquerque, NM: University of New Mexico Press), 269–300.
Skogan, W. G., Hartnett, S. M., Bump, N., and Dubois, J. (2008). Evaluation of CeaseFire-Chicago. Evanston, IL: Center for Policy Research, Northwestern University.
Sparrow, B., Liu, J., and Wegner, D. M. (2011). Google effects on memory. Science 333, 776–778. doi: 10.1126/science.1207745
Taylor, K. C., Lamorey, G. W., Doyle, G. A., Alley, R. B., Grootes, P. M., Mayewski, P. A., et al. (1993). The ‘flickering switch’ of late Pleistocene climate change. Nature 361, 432–436. doi: 10.1038/361432a0
Tierney, J. E., and DeMenocal, P. B. (2013). Abrupt shifts in Horn of Africa hydroclimate since the Last Glacial Maximum. Science 342, 843–846. doi: 10.1126/science.1240411
Wang, R., Dearing, J. A., Langdon, P. G., Zhang, E., Yang, X., Dakos, V., et al. (2012). Flickering gives early warning signals of a critical transition to a eutrophic lake state. Nature 492, 419–422. doi: 10.1038/nature11655
Watts, D. J. (2002). A simple model of global cascades on random networks. Proc. Natl. Acad. Sci. U.S.A. 99, 5766–5771. doi: 10.1073/pnas.082090499
Welch, J. R., Vincent, J. R., Auffhammer, M., Moya, P. F., Dobermann, A., and Dawe, D. (2010). Rice yields in tropical/subtropical Asia exhibit large but opposing sensitivities to minimum and maximum temperatures. Proc. Natl. Acad. Sci. U.S.A. 107, 14562–14567. doi: 10.1073/pnas.1001222107
Keywords: ecological breakdown, social collapse, paleoecology, paleoclimatology, research synergies
Citation: Bentley RA, Maddison EJ, Ranner PH, Bissell J, Caiado CCS, Bhatanacharoen P, Clark T, Botha M, Akinbami F, Hollow M, Michie R, Huntley B, Curtis SE and Garnett P (2014) Social tipping points and Earth systems dynamics. Front. Environ. Sci. 2:35. doi: 10.3389/fenvs.2014.00035
Received: 05 June 2014; Accepted: 28 July 2014;
Published online: 19 August 2014.
Edited by:
Rob Swart, Alterra, NetherlandsReviewed by:
Nathaniel Kenneth Newlands, Federal Government of Canada, CanadaJaroslav Mysiak, Fondazione Eni Enrico Mattei, Italy
Tim Lenton, University of Exeter, UK
Copyright © 2014 Bentley, Maddison, Ranner, Bissell, Caiado, Bhatanacharoen, Clark, Botha, Akinbami, Hollow, Michie, Huntley, Curtis and Garnett. This is an open-access article distributed under the terms of the Creative Commons Attribution License (CC BY). The use, distribution or reproduction in other forums is permitted, provided the original author(s) or licensor are credited and that the original publication in this journal is cited, in accordance with accepted academic practice. No use, distribution or reproduction is permitted which does not comply with these terms.
*Correspondence: R. A. Bentley, Department of Archaeology and Anthropology, Bristol University, 43 Woodland Road, BS8 1UU, Bristol, UK e-mail:ci5hLmJlbnRsZXlAYnJpc3RvbC5hYy51aw==