- 1Laboratory of Environmental Modelling, Department of Chemical and Environmental Engineering, Escuela Técnica Superior de Ingenieros Industriales, Technical University of Madrid, Madrid, Spain
- 2Air and Environment Quality, Ricardo Energy and Environment, London, UK
- 3Department of Geosciences, Institute of Environmental Assessment and Water Research (IDAEA-CSIC), Barcelona, Spain
- 4Cancer and Environmental Epidemiology Unit, National Centre for Epidemiology, Carlos III Institute of Health, Madrid, Spain
An evaluation of the concentration levels of Particulate Matter (PM) was carried out in Madrid (Spain) by introducing the emissions from road dust resuspension. Road dust resuspension emission factors (EF) for different types of vehicles were calculated from EPA-AP42, a global resuspension factor of 0.097 g veh−1 km−1 as described in Amato et al. (2010) and a rain-dependent correction factor. With these resuspension EFs, a simulation at street canyon level was performed with the OSPM model without rainfall. Subsequently, a simulation using the CMAQ model was implemented adding resuspension emissions affected by the rain. These data were compared with monitored data obtained from air quality stations. OSPM model simulations with resuspension EFs but without the effect of rainfall improve the PM estimates in about 20 μg m−3 compared to the simulation with default EFs. Total emissions were calculated by adding the emissions estimated with resuspension EFs to the default PM emissions to be used by CMAQ. For the study in the Madrid Area, resuspension emissions are approximately of the same order of magnitude as inventoried emissions. On a monthly scale, rain effects are negligible for resuspension emissions due to the dry weather conditions of Spain. With the exception of April and May, the decrease in resuspension emissions is not >3%. The predicted PM10 concentration increases up to 9 μg m−3 on annual average for each station compared to the same scenario without resuspension. However, in both cases, PM10 estimates with resuspension are still underestimating observations. It should be noted that although that accounting for resuspension improves the quality of model predictions, other PM sources (e.g., Saharan dust) were not considered in this study.
Introduction
Numerous pollutants that have an impact on public health and ecosystems influence environmental quality in the world. Public exposure to particulate matter (PM) increases the risk of respiratory and cardiovascular problems in urban areas with high PM concentrations and population densities (Samet et al., 2000; Tsai et al., 2000; Chiaverini, 2002). PM10 emissions are primarily the amount of PM emitted directly from traffic and other particles emitted due to wear of tires and brakes, the road wear and road dust resuspension (Thorpe and Harrison, 2008). Direct emissions are quantified in emissions inventories although resuspension is difficult to estimate because the emission phenomenon is not known in precision. Nevertheless, several studies show the evidence of the influence of non-exhaust emissions from traffic on the amount of urban PM10 (Gehrig et al., 2004; Bukowiecki et al., 2010) resulting to be of the same order of magnitude as the exhaust emissions (Abu-Allaban et al., 2003; Kristensson et al., 2004; Amato et al., 2009a), hence the importance of estimating non-exhaust emissions correctly.
There is a large underestimation in emission inventories and regional air quality models on PM levels (Vautard et al., 2005). Emissions from road dust resuspension are relevant in many parts of the world. In the Scandinavian countries this is due to the accumulation of PM by the contribution of studded tires and road sanding (Kupiainen et al., 2003; Denby et al., 2011; Kupiainen and Pirjola, 2011) and in the Mediterranean countries due to lack of rainfall which increases the contribution of resuspension to the amount of PM (Amato et al., 2009b; Karanasiou et al., 2011). Other events that may affect resuspension in Southern Europe are intrusions of Saharan dust (Artiñano et al., 2003; Borge et al., 2007; Querol et al., 2008). It is essential, depending on the location, to determine the factors involved and the importance of quantifying each of them in order to integrate them in a modeling system that produces representative PM estimates.
Previously published studies estimated specific emission factors (EFs) for resuspension worldwide depending on the country (Abu-Allaban et al., 2003; Gehrig et al., 2004; Omstedt et al., 2005; Thorpe et al., 2007; Amato et al., 2010; Bukowiecki et al., 2010). However, there are few examples in which the resuspension calculations are implemented in an air quality model to study its influence on ambient PM concentrations. In the case of Spain, the CALIOPE model has included a module for the calculation of resuspension at a national scale using a 4-km resolution (Baldasano et al., 2011; Pay et al., 2011). Therefore, the aim of this paper is to evaluate the performance variation in an urban-scale air quality model due to the inclusion of PM resuspension in order to inform local decision-making. The assessment of improvements in PM concentrations due to considering resuspension required was made through the combination of air quality models focusing on two different scales, namely road scale and mesoscale. The models used in this study were Operational Street Pollution Model (OSPM) (Hertel and Berkowicz, 1989; Berkowicz, 2000) for road scale (street canyon) and a mesoscale-modeling system composed by three models: the Weather Research and Forecasting (WRF) model (Skamarock and Klemp, 2008), the Sparse Matrix Operator Kernel Emissions (SMOKE) model (UNC Carolina Environmental Program, 2005), and the Community Multiscale Air Quality (CMAQ) model (Byun and Ching, 1999; Byun and Schere, 2006).
Modified traffic emissions of PM including resuspension and rainfall correction were considered for the Madrid urban area. The procedure is based on the estimation of empirical EFs developed by Amato et al. (2010). For each vehicle type, a ratio is estimated by calculating the average of the different weight classes. The EF for each vehicle type is calculated by adjusting the EF with the fleet composition of the Madrid urban area. The EFs for road dust resuspension were introduced in the two models. The performance of the OSPM model considering resuspension was validated against the results of a sampling campaign carried out in Madrid in 2009 and described in Karanasiou et al. (2011). Subsequently, the outputs of the OSPM model were implemented in an integrated mesoscale air quality model tailored to the conditions of the Madrid urban area as described in Borge et al. (2010, 2014).
Materials and Methods
PM resuspension was implemented into the selected models to estimate its corresponding proportion to non-exhaust emissions. EFs of road dust resuspension were obtained from a sampling campaign in the city of Barcelona detailed in Amato et al. (2010). The results of this study allowed considering as an initial approximation an average PM resuspension EF of 0.082 g veh−1 km−1, which was directly applied to the Madrid urban area due to the similar climatic conditions that affect resuspension. As a result, it was subject to subsequent changes due to improvements in the estimation procedures of EFs. As previously stated, two air quality simulations were configured using PM resuspension. A first simulation was carried out with the OSPM model to determine the influence of resuspension at the street canyon level. The time frame of this simulation was set to coincide with the period of the sampling campaign described in Karanasiou et al. (2011) which ran for 1 month between the 19 of June and the 19 of July 2009 for model validation purposes. Once the OSPM results were checked, an annual simulation for 2007 with 8760 hourly-data was conducted with the CMAQ model considering the resuspension and the effect of rain to determine the effects of resuspension without meteorological limitations inherent to a specific period of time [e.g., a given month in the year and to account for the importance of specific meteorological episodes and moisture content on the results, as compared to Gromaire et al. (2000)].
Air Quality Modeling with Dust Resuspension
The modeling domain is centered in the region of Madrid and comprises 40 rows and 44 columns of 1 × 1 km2 resolution (Figure 1). It has a vertical structure that covers the troposphere but the impact of resuspension on emissions is quantified only in the layers of the atmosphere that are closer to the surface. The meteorological model (WRF) was configured with the optimal settings determined for 2007 as discussed in Borge et al. (2008a). Emissions for the Madrid urban area were based on the Spanish National Emissions Inventory (SNEI), adapted following the findings of a comparison exercise between COPERT 4 and Handbook Emission Factors for Road Transport (HBEFA) (Borge et al., 2012) and processed with the SMOKE model as described in Borge et al. (2008b). The chemical speciation of volatile organic compounds (VOCs) and PM was made to match the requirements of the Carbon Bond mechanism CB05 (Yarwood et al., 2005) with information from the EMEP/CORINEAIR guidebook [European Environment Agency (EEA), 2007], COPERT 4 (Gkatzoflias et al., 2007) and the U.S. EPA SPECIATE database (Hsu et al., 2006).
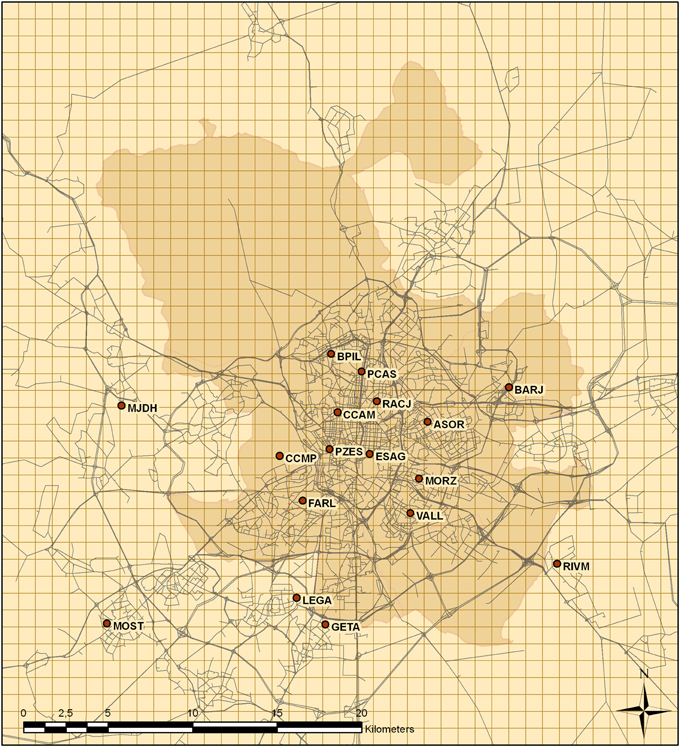
Figure 1. CMAQ modeling domain for Madrid area and air quality monitoring stations selected to evaluate the resuspension analysis.
The calculation of the contribution of PM resuspension and rain to the ambient levels of PM concentrations (PM10) was performed and implemented as a scaling factor inside the emission model. On one hand, emissions from resuspension were calculated by vehicle type and rain using mobility and traffic data for the Madrid urban area. On the other hand, total emissions were estimated as indicated in the National Inventory for all pollution sources. Then, emissions were grouped and integrated with the total emissions in the emissions model for their use in CMAQ. Finally, the results of the air quality model were compared against observations aided by statistical indicators of performance.
Traffic Correction of Resuspension
It has been shown by some studies that traffic flows directly affect PM resuspension (Cao et al., 2006). As a consequence, the resuspension EF needs to be adapted depending on the different types of vehicle that are present in the fleet. In this study, these adaptation was made by weighting the different EFs by the hourly traffic intensities provided at street level according to the recommendations of the EPA fugitive dust emissions for paved roads (US-EPA, 2011).
The first adjustment was carried out using the EPA version of the emissions model. As the model representation exhibits limitations according to some studies (APEG, 1999; Venkatram, 2000), it has only been used as a correction factor for calculating emission ratios based on the weight classes of each vehicle type.
Where k is the particle size multiplier for a given particle size range in g/VKT (VKT stands for Vehicle Kilometer Traveled), sL is the silt load in g m−2, W is the average weight of a vehicle of the fleet in tons and E is the PM10 emission in g VKT−1. Ratios have been calculated using a passenger car as reference and are shown in Table 1.
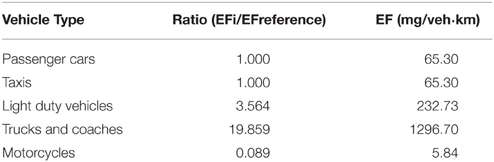
Table 1. Emission factors and ratios calculated according to EPA-AP42 and EF defined in Amato et al. (2010).
Furthermore, values of traveled vehicle-kilometers for every vehicle class were obtained from the Traffic Department of the Municipality of Madrid in order to calculate specific EFs as in Equation 2.
Individual EF by vehicle type can be calculated from the global resuspension EF through relating the ratios obtained with the vehicle-kilometers for each vehicle type (Equation 3).
Thus, individual EFs by vehicle type for resuspension can be introduced in the models as an element of calculation and to study variations in air quality.
The calculation of resuspension is performed on an hourly basis at street level so it can be consistently applied to both models. For the OSPM model, EFs are modified to ensure that total emissions are the sum of direct emissions and resuspension emissions. For the CMAQ model, resuspension emissions are calculated offline and are added to the direct emissions before carrying out the simulations.
Rain Correction of Resuspension
Rain is another factor to take into account for the limitations of the calculation. In Europe, there have been studies where a reduction in the available amount of dust for resuspension has been found (Bris et al., 1999; Gromaire et al., 2000; Amato et al., 2009b). Other studies which evaluated the influence of road cleaning on air quality observed reductions of up to 2 μg m−3 or 6% in the PM concentrations (Düring et al., 2004, 2005; Norman and Johansson, 2006). The effects of rain events on PM according to Gromaire et al. (2000) may resemble to the effects that are generated by street cleaning using water flushing. The influence of rain tends to reduce resuspension emissions generated by traffic but there are uncertainties that are difficult to quantify. The rainfall decreases the amount of deposited dust available for resuspension by aggregating particles and runoff into the sewer. However, the amount of road dust when the effect of the rain is over is considered sufficient to assume that it does not limit emissions for new resuspension.
In the light of the above, there are two fundamental assumptions that need to be considered: (i) the amount of dust available on streets is generally not known but due to the contribution of resuspension to PM that has been quantified before and after washing the street (Yu et al., 2006), it can be said that the available dust on the street is greater than its contribution to the PM concentration; (ii) once it is known that the existing dust on the road does not limit the maximum amount provided by traffic on the resuspension, it is necessary to estimate the limitation that the rain has on the amount of dust that can be resuspended.
The precipitation threshold is set at 0.254 mm (0.01 in) in hourly basis according to US-EPA (2011). The duration of the impact of rain on the amount of particles may start affecting resuspension and decreasing the PM concentration of PM after between 2 and 4 h, depending on the type of particulates, Total Solid Particles (TSP) or PM10 (Chang et al., 2005; Chou et al., 2007). This impact is difficult to quantify so it was assumed that the influence of rain decreases linearly with time due to the limited availability of better information to implement a more refined parameterization.
Composition of the Resuspended Dust
In order to adequately simulate the PM concentration is important to know the particle composition. It is expected that the composition of dust high in the resuspension is similar to the composition of PM present in ambient air. A specific study in which the elemental composition of resuspended dust was carried out in Madrid using Positive Matrix Factorisation and is shown in Table 2 (Karanasiou et al., 2011). The compositions of the resuspended dust were reflected in the emission model.
Monitoring Stations
The PM concentrations resulting from the simulations are compared with observed data to evaluate the impact of the incorporation of resuspension in the model. These observed data were chosen to represent the study area and reflect all the different conditions that exist within it. For the OSPM results, only kerbside stations were selected due to the fact that the effects of resuspension are more noticeable in these locations as they are dependent only on traffic. For the CMAQ results, predictions were evaluated against observations from suburban locations that are deemed representative of the average concentration at the cell level.
Moreover, for each model monitoring stations were selected depending on the simulated event, the study area and the scale used in the simulations. OSPM simulations were validated for two locations that corresponded to where mobile stations in the PM sampling campaign were placed during the summer of 2009 (19 June–19 July 2009). These locations were in the center of the Madrid urban area along an extremely busy road (Velázquez Road) and placed 1.5 km apart at the junctions with Alcalá Road and Maldonado Road as described in Karanasiou et al. (2011). However, the results from CMAQ were evaluated using a larger number of air quality stations with annual observations for 2007. The stations used for validation are presented in Table 3 and Figure 1.
Evaluation Procedure
The assessment seeks to determine the ability of the model to represent road dust resuspension by comparing the predicted PM concentrations against observations from a series of monitoring stations within the studied domain. A set of statistical indicators were chosen to characterize the performance of the model, as well as suitable goals and criteria for assessment. In this study, the benchmarks proposed by Boylan and Russell (2006) are considered as sensible reference to assess model performance for the prediction of PM ambient air concentration. These indicators have been complemented with other generally-recognized statistical indicators that complement the evaluation of representativeness. The statistical analysis was complemented with the use of Taylor diagrams, which simultaneously present the centered root-mean square error (RMSE), the correlation coefficient (r) and the standard deviation (Taylor, 2001). These diagrams were drawn with the aid of the FAIRMODE Delta Tool (Thunis, 2011).
The main analysis is focused on determining the differences in modeling considering emissions with or without resuspension and the influence these have in the air quality estimates. In addition, OSPM and CMAQ model predictions were re contrasted against observations in a different way. The values obtained from the OSPM model include emissions from resuspension without taking into account the mitigation added by the impact of rain at the street canyon level while CMAQ predictions consider resuspension by traffic and rain effects on resuspension emissions at the mesoscale level.
Results
An analysis of resuspension was carried out for different scales. EF for the OSPM model have been modified to add the effects of resuspension at street canyon for the locations of the sampling mobile stations. For this simulation, the effects of rain are not taken into consideration due to the difficulty of implementation in the model. Another assessment was performed of simulated values from CMAQ against observations at the mesoscale for the selected stations in order to evaluate the ability of the model to represent resuspension in the Madrid urban area.
Street-scale Simulation
The OSPM model simulations were taken to confirm the improvement in modeling PM through the implementation of road dust resuspension. The validation of the resuspension module was made against the results of the sampling campaign for Velázquez Road in 2009 because there are reliable traffic data to feed OSPM model and also there are in situ measurements of PM for comparison with model results. To perform the simulations taking into account the resuspension, the emission factor was modified to introduce in the simulation the sum of direct emissions and indirect emissions which are resuspension emissions.
Two simulations have been carried out for the two locations described above. In the first simulation, representative exhaust PM EF have been used in the OSPM model without considering the effect of resuspension while in the second simulation the resuspension EF described in Section Traffic Correction of Resuspension was added. The traffic intensity that was obtained from measured data is very similar for the two sites, ~1200 vehicles/day (Figure 2). Besides data composition of the fleet of vehicles, other parameters such as speed were taken from the traffic model of the city of Madrid as previously indicated.
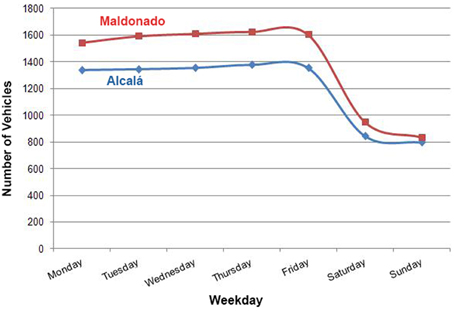
Figure 2. Daily average total number of vehicles for OSPM simulations in Alcalá and Maldonado sites.
PM10 concentrations were evaluated and the results are shown in the Table 4. Mean predicted values for the campaign using default EF underestimate the mean observed values. In Alcalá Road site, the mean predicted concentration has been 21.4 μg m−3 against an average of 49.7 μg m−3 from observations while, in Maldonado Road, the mean predicted concentration is 20.4 μg m−3, against an average observed concentration of 43.6 μg m−3. In Figure 3, the daily average PM10 concentration modeled for both sites is presented. From the comparison of Figures 2, 3 a clear relationship between traffic and PM10 concentration can be inferred. According to the results in Figure 3 it is clear that the inclusion of resuspension in the model improves PM10 predictions, being the modeled and observed series very similar for the two locations. In Figure 4, hourly modeled values against the observed values are compared for Maldonado Road as an illustrative example. There is a trend of increasing PM10 concentrations when resuspension emissions factors are added generating an increase in variability. Therefore, the model predicts values close to 20 μg m−3 but the observations are closer to 40 μg m−3. However, mean predicted values for the simulations with resuspension emission factor are 43.7 μg m−3 and 41.6 μg m−3 for Alcalá Road and Maldonado Road being much closer to the average of the observed values.

Table 4. Aggregated statistics by Emission Factor for PM10 concentration in OSPM model for Alcala and Maldonado sites.
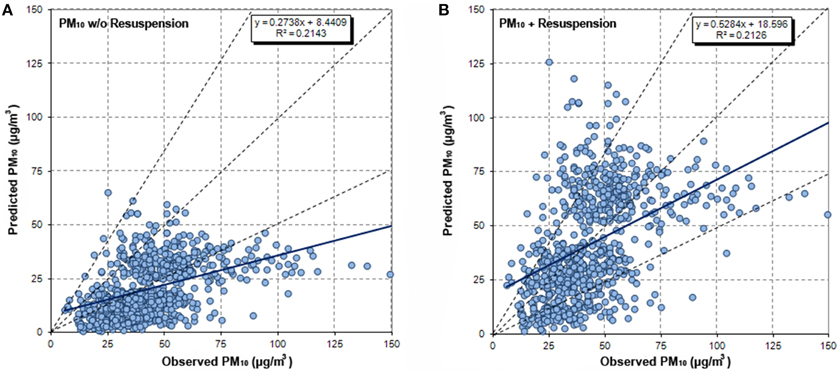
Figure 4. Comparison between hourly OSPM PM10 predictions and observations in Maldonado site: (A) without resuspension (B) with resuspension.
Regarding the values of the correlation coefficient (r), these are virtually identical in the default and resuspension simulations. This can be attributed to the good representation of the model tendency in both simulations, with an almost identical r coefficient of 0.48 and 0.46 respectively for Alcalá and Maldonado. It can be appreciated that in an hourly basis, the predicted values from the default simulation underestimate systematically the observed values whereas for the resuspension simulation, predicted values were almost equal to the observed values in both sites.
To validate this modeling approach, the values proposed in the study of Boylan and Russell (2006) have been considered. The recommended values for measuring the accuracy of the model were the Mean Fractional Bias (MFB) and the Mean Fractional Error (MFE). The goal is defined for MFE and MFB as less or equal than +50 and ±30% and for criteria as less or equal than +75 and ±60%, respectively. For the resuspension simulations in Alcalá Road and Maldonado Road, MFB values are lower than the values set as a goal being −13.4 y −15.1%. For MFE values, the goal is also achieved by obtaining in 49.9% in Alcala and 48.7% in Maldonado.
In summary and according to the selected statistical indicators, the OSPM model with the representation of resuspension seems to improve the overall model performance than the without it.
Urban-scale Simulation
Emissions considering the effect of resuspension and the effect of rain on it have been used in CMAQ simulations. These emissions have been calculated according to the guidelines described above. These values represent 53% of the total emissions for PM10 emitted by adding of the emissions of resuspended PM to the emissions from SNEI for the whole domain (Figure 5). The influence of rain on resuspension appeared to be practically negligible due to dry weather conditions, except for the months of April and May which correspond to the rainiest months in Madrid (Figure 6). For the other months, the changes in the resuspension emissions due to the rain accounted for < 3% of emissions from resuspension without rain.
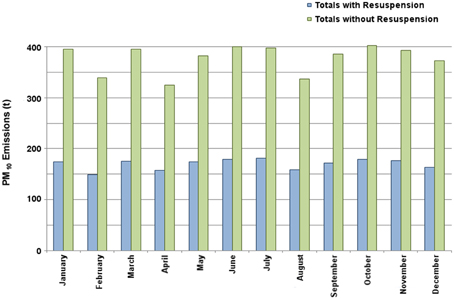
Figure 5. Monthly comparison of PM10 emissions for the Madrid Region with and without traffic-induced resuspension.
In this part of the study, two annual simulations have been carried out. In the first simulation, the emissions used to feed the model were those estimated by the SNEI without modification due to resuspension while for the second simulation, emissions from resuspension modified by the effect of rain were combined with the SNEI emissions The resulting PM emissions indicate that resuspension appears to have an important contribution to the final PM10 concentration. Domain cells affected by the resuspension are those containing roads defined in the traffic model. The remaining cells do not significantly modify the PM10 concentrations (Figure 7).
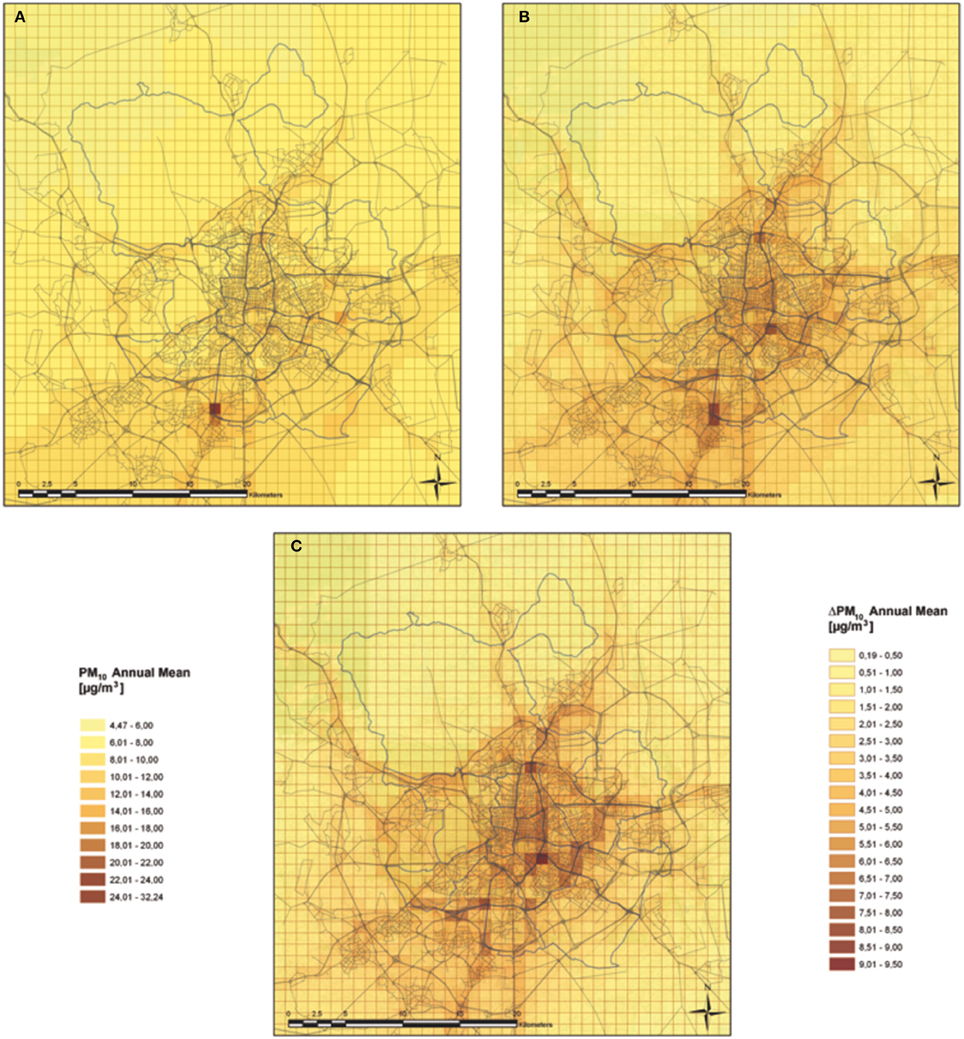
Figure 7. Annual average PM10 concentrations for CMAQ simulations considering: (A) emissions with resuspension and the effect of rain (B) emissions without resuspension (C) differences between (A) and (B).
The CMAQ model simulations generally underestimate PM10 concentrations between 15 and 25 μg m−3 with respect to the observations for both simulations in average (Table 5). PM10 concentrations are closer to the observations for the simulation with resuspension that for the simulation without it up to 9 μg m−3 on annual average for all stations. These results are similar to those of previous air quality modeling studies in Spain where the contribution of resuspension to PM ambient concentration were estimated between 3 and 7 μg m−3 (Pay et al., 2011). Moreover, the correlation coefficients were slightly higher for all stations considered when the values of resuspension are implemented on the model (Figure 8). The global mean bias improved 4.2 μg m−3 on average when resuspension was considered. The MFB and MFE were reduced a 27.2 and a 17.1%, respectively. Although the addition of resuspension emissions to the model reduced the average bias in the concentration of PM10 with respect to the simulation without resuspension, the predicted concentrations do not have a full correspondence with the observed PM10 concentrations.
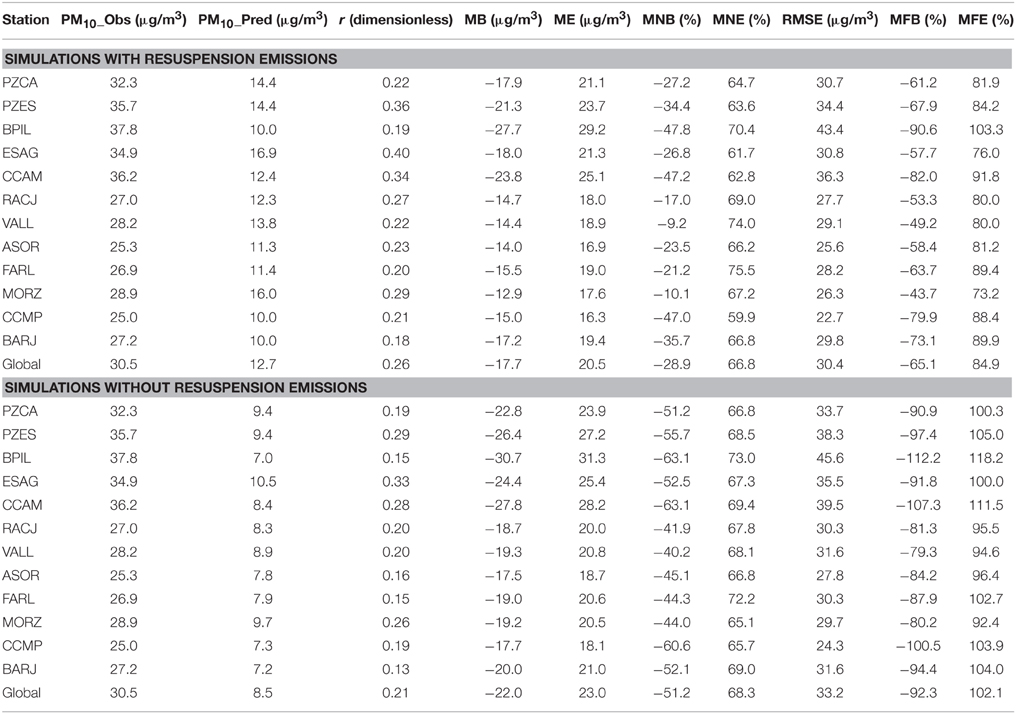
Table 5. Statistics of PM10 for CMAQ simulations with resuspension emissions and without resuspension emissions.
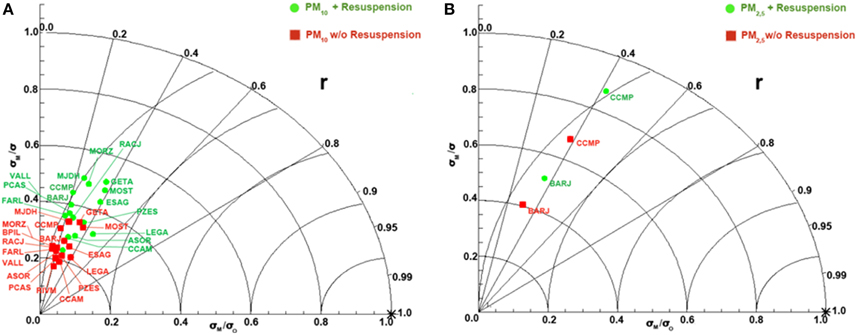
Figure 8. Taylor diagrams showing CMAQ model performance for particulate matter prediction with and without implementation of resuspension: (A) results for PM10 (B) results for PM2.5.
There are studies that show for Spain that the composition of PM10 concentration at high levels is basically influenced by wind-blown dust as Saharan dust intrusions (Rodríguez et al., 2001; de la Paz et al., 2013; Pandolfi et al., 2014). Other processes such as road gritting which generate natural erosion are known to contribute to the total PM10 concentration but its magnitude has not been determined in this study. In the case of Spain, the contribution of these sources might be substantial due to the generally dry weather conditions.
Discussion
In this study, the influence of traffic-induced road dust resuspension was studied. For this purpose, simulations were performed to test the resuspension approach using the OSPM model for two specific locations in the city of Madrid without assessing the changes introduced by the rain. Then the calculation of resuspension affected by rain was implemented to determine PM10 concentrations using the CMAQ model for the Madrid urban area.
For the OSPM simulations, the predicted PM10 concentrations with resuspension were much closer to observations than PM10 predictions without resuspension. The bias values from the observed values were 5 and 20 μg m−3, respectively. This is due to the fact that the model greatly underestimates the concentration of particles without considering the emissions from resuspension. However, the linear correlations for both simulations were similar (r = 0.5) due to the fact that the trend in the changes in concentrations was properly captured. This underlies the important contribution of road traffic in the PM10 concentrations.
For the CMAQ simulations, the amount of emissions from resuspension was of the same order of magnitude than the PM emissions reported in the SNEI, as pointed out in other studies (Abu-Allaban et al., 2003; Amato et al., 2009a).
Rain did not prove to have a substantial effect in the resuspension emissions due to the generally dry weather conditions in the Madrid urban area. For these simulations, the model underestimated the PM10 concentration in the two cases with and without resuspension. However, predictions were closer to the observations in the case with resuspension, which exhibited higher correlation coefficients and lower errors.
In summary both simulations using the OSPM and CMAQ models with resuspension emissions increase the statistical correspondence between predictions and observations from monitoring stations, compared with the same simulations without resuspension. In this study, the influence of rain has been confirmed as negligible due to the dry weather conditions in the Madrid urban area. Other differences between predictions and observations may be attributed to potential underestimations in the emissions of PM in the SNEI, as shown in other studies (e.g., Vautard et al., 2005).
Conflict of Interest Statement
The authors declare that the research was conducted in the absence of any commercial or financial relationships that could be construed as a potential conflict of interest.
Acknowledgments
This project was partially financed by the Spanish Ministry of the Environment (SERCA-058/PC08/3-18.1).
References
Abu-Allaban, M., Gillies, J. A., Gertler, A. W., Clayton, R., and Tailpipe, P. D. (2003). Resuspended road dust, and brake-wear emission factors from on-road vehicles. Atmos. Environ. 37, 5283–5293. doi: 10.1016/j.atmosenv.2003.05.005
Amato, F., Nava, S., Lucarelli, F., Querol, X., Alastuey, A., Baldasano, J. M., et al. (2010). A comprehensive assessment of PM emissions from paved roads: real-world emission factors and intense street cleaning trials. Sci. Total Environ. 408, 4309–4318. doi: 10.1016/j.scitotenv.2010.06.008
Amato, F., Pandolfi, M., Escrig, A., Querol, X., Alastuey, A., Pey, J., et al. (2009a). Quantifying road dust resuspension in urban environment by multilinear engine: a comparison with PMF2. Atmos. Environ. 43, 2770–2780. doi: 10.1016/j.atmosenv.2009.02.039
Amato, F., Querol, X., Alastuey, A., Pandolfi, M., Moreno, T., Gracia, J., et al. (2009b). Evaluating urban PM10 pollution benefit induced by street cleaning activities. Atmos. Environ. 43, 4472–4480. doi: 10.1016/j.atmosenv.2009.06.037
APEG (1999). Report of the Airborne Particle Expert Group: Source Apportionment of Airborne Particulate Matter in the United Kingdom. Department of Environment, Transport and the Regions UK, London.
Artiñano, B., Salvador, P., Alonso, D. G., Querol, X., and Alastuey, A. (2003). Anthropogenic and natural influence on the PM10 and PM2.5 aerosol in Madrid (Spain). Analysis of high concentration episodes. Environ. Pollut. 125, 453–465. doi: 10.1016/S0269-7491(03)00078-2
Baldasano, J. M., Pay, M. T., Jorba, O., Gassó, S., and Jiménez-Guerrero, P. (2011). An annual assessment of air quality with the CALIOPE modeling system over Spain. Sci. Total Environ. 409, 2163–2178. doi: 10.1016/j.scitotenv.2011.01.041
Berkowicz, R. (2000). OSPM - A parameterised street pollution model. Environ. Monit. Assess. 65, 323–331. doi: 10.1023/A:1006448321977
Borge, R., Alexandrov, V., del Vas, J. J., Lumbreras, J., and Rodríguez, M. E. (2008a). A comprehensive sensitivity analysis of the WRF model for air quality applications over the Iberian Peninsula. Atmos. Environ. 42, 8560–8574. doi: 10.1016/j.atmosenv.2008.08.032
Borge, R., López, J., Lumbreras, J., Narros, A., and Rodríguez, E. (2010). Influence of boundary conditions on CMAQ simulations over the Iberian Peninsula. Atmos. Environ. 44, 2681–2695. doi: 10.1016/j.atmosenv.2010.04.044
Borge, R., Lumbreras, J., and Rodríguez, M. E. (2008b). Development of a high-resolution emission inventory for Spain using the SMOKE modelling system: a case study for the years 2000 and 2010. Environ. Model. Softw. 23, 1026–1044. doi: 10.1016/j.envsoft.2007.11.002
Borge, R., Lumbreras, J., Pérez, J., de la Paz, D., Vedrenne, M., de Andrés, J. M., et al. (2014). Emission inventories and modeling requirements for the development of air quality plans. Application to Madrid (Spain). Sci. Total Environ. 466–467, 809–819. doi: 10.1016/j.scitotenv.2013.07.093
Borge, R., Lumbreras, J., Vardoulakis, S., Kassomenos, P., and Rodriguez, M. E. (2007). Analysis of long-range transport influences on urban PM10 using two-stage atmospheric trajectory clusters. Atmos. Environ. 41, 4434–4450. doi: 10.1016/j.atmosenv.2007.01.053
Borge, R., Miguel, I., Paz, D., Lumbreras, J., Pérez, J., and Rodríguez, E. (2012). Comparison of road traffic emission models in Madrid (Spain). Atmos. Environ. 62, 461–471. doi: 10.1016/j.atmosenv.2012.08.073
Boylan, J. W., and Russell, A. G. (2006). PM and light extinction model performance metrics, goals, and criteria for three-dimensional air quality models. Atmos. Environ. 40, 4946–4959. doi: 10.1016/j.atmosenv.2005.09.087
Bris, F. J., Garnuad, S., Apperry, N., Gonzalez, A., Mouchel, J. M., and Chebbo, G. (1999). A street deposit sampling method for metal and hydrocarbon contamination assessment. Sci. Total Environ. 235, 211–220. doi: 10.1016/S0048-9697(99)00192-8
Bukowiecki, N., Lienemann, P., Hill, M., Furger, M., Richard, A., Amato, F., et al. (2010). PM10 emission factors for non-exhaust particles generated by road traffic in an urban street canyon and along a freeway in Switzerland. Atmos. Environ. 44, 2330–2340. doi: 10.1016/j.atmosenv.2010.03.039
Byun, D. W., and Ching, J. K. S. (1999). Science Algorithms of the EPA Models-3 Community Multi-scale Air Quality (CMAQ) Modeling System. EPA/600/R-99/030. US EPA National Exposure Research Laboratory, Research Triangle Park, NC, Washington DC.
Byun, D. W., and Schere, K. L. (2006). Review of the governing equations, computational algorithms, and other components of the Models-3 community Multiscale Air Quality (CMAQ) modeling system. Appl. Mech. Rev. 59, 51–77. doi: 10.1115/1.2128636
Cao, J. J., Lee, S. C., Ho, K. F., Fung, K., Chow, J. C., and Waston, J. G. (2006). Characterization of roadside fine particulate carbon and its eight fractions in Hong Kong. Aerosol Air Qual. Res. 6, 106–122.
Chang, Y. M., Chou, C. M., Su, K. T., and Tseng, C. H. (2005). Effectiveness of street sweeping and washing for controlling ambient TSP. Atmos. Environ. 39, 1891–1902. doi: 10.1016/j.atmosenv.2004.12.010
Chiaverini, L. (2002). Asthma, particulates, and diesel exhaust Medicine and health. Rhode Island 85, 140–142.
Chou, C., Chang, Y., Lin, W., and Tseng, C. (2007). Evaluation of street sweeping and washing to reduce ambient PM10. Int. J. Environ. Pollut. 31, 431–448. doi: 10.1504/IJEP.2007.016507
de la Paz, D., Vedrenne, M., Borge, R., Lumbreras, J., de Andrés, J. M., Pérez, J., et al. (2013). Modelling Saharan dust transport into the Mediterranean basin with CMAQ. Atmos. Environ. 70, 337–350. doi: 10.1016/j.atmosenv.2013.01.013
Denby, B., Sundvor, I., Johansson, C., Kauhaniemi, M., Härkönen, J., Omstedt, G., et al. (2011). “Progress towards traffic induced road dust and salt emission modelling: model development within NORTRIP,” in Harmonisation Conference on Dispersion Modeling for Regulatory Purposes (Kos).
Düring, I., Zippack, L., Bächlin, W., and Lohmeyer, A. (2004). Auswertung der Messungen des BLUME Während der Abspülmaßnahme am Abschnitt Frankfurter Allee 86. Ingenieurbüro Lohmeyer GmbH & Co. KG. Immissionsschutz, Klima, Aerodynamik, Umweltsoftware.
Düring, I., Zippack, L., Bächlin, W., and Lohmeyer, A. (2005). Auswertung der Messungen des BLUES während der Abspülmassnahme im Bereich der Messstation Neuenlander Strasse in Bremen. Ingenieurbüro Lohmeyer GmbH & Co. KG. Immissionsschutz, Klima, Aerodynamik, Umweltsoftware.
European Environment Agency (EEA) (2007). EMEP/CORINAIR Emission Inventory Guidebook – 2007. European Union, EEA Technical Report no. 16/2007.
Gehrig, R., Hill, M., Buchmann, B., Imhof, D., Weingartner, E., and Baltensperger, U. (2004). Separate determination of PM10 emission factors of road traffic for tailpipe emissions and emissions from abrasion and resuspension processes. Int. J. Environ. Pollut. 22, 312–325. doi: 10.1504/IJEP.2004.005549
Gkatzoflias, D., Kouridis, C., Ntziachristos, L., and Samaras, Z. (2007). COPERT 4. Computer Programme to Calculate Emissions from Road Transport. User manual (version 5.0). Available online at: http://www.emisia.com/copert/
Gromaire, M. C., Garnaud, S., Ahyerre, M., and Chebbo, G. (2000). The quality of street cleaning waters: comparison with dry and wet weather flows in a Parisian combined sewer system. Urban Water 2, 39–46. doi: 10.1016/S1462-0758(00)00038-8
Hertel, O., and Berkowicz, R. (1989). Modelling Pollution from Traffic in a Street Canyon. Evaluation of Data and Model Development. Roskilde: NERI.
Hsu, Y., Strait, R., Roe, S., and Holoman, D. (2006). SPECIATE 4.0. Speciation Database Development Documentation. U.S. Federal Government, Final Report. EPA contract. Nos EP-D-06.001, work assignment Numbers 0-03 and 68-D-02-063, WA 4-04 and WA 5-05. EPA/600/R-06/161. 2006.
Karanasiou, A., Moreno, T., Amato, F., Lumbreras, J., Narros, A., Borge, R., et al. (2011). Road dust contribution to PM levels - Evaluation of the effectiveness of street washing activities by means of Positive Matrix Factorization. Atmos. Environ. 45, 2193–2201. doi: 10.1016/j.atmosenv.2011.01.067
Kristensson, A., Johansson, C., Westerholm, R., Swietlicki, E., Gidhagen, L., Wideqvist, U., et al. (2004). Real-world traffic emission factors of gases and particles measured in a road tunnel in Stockholm, Sweden. Atmos. Environ. 38, 657–673. doi: 10.1016/j.atmosenv.2003.10.030
Kupiainen, K., and Pirjola, L. (2011). Vehicle non-exhaust emissions from the tyre-road interface-effect of stud properties, traction sanding and resuspension. Atmos. Environ. 45, 4141–4146. doi: 10.1016/j.atmosenv.2011.05.027
Kupiainen, K., Tervahattu, H., and Räisänen, M. (2003). Experimental studies about the impact of traction sand on urban road dust composition. Sci. Total Environ. 308, 175–184. doi: 10.1016/S0048-9697(02)00674-5
Norman, M., and Johansson, C. (2006). Studies of some measures to reduce road dust emissions from paved roads in Scandinavia. Atmos. Environ. 40, 6154–6164. doi: 10.1016/j.atmosenv.2006.05.022
Omstedt, G., Johansson, C., and Bringfelt, B. (2005). A model for vehicle induced non-tailpipe emissions of particles along Swedish roads. Atmos. Environ. 39, 6088–6097. doi: 10.1016/j.atmosenv.2005.06.037
Pandolfi, M., Tobias, A., Alastuey, A., Sunyer, J., Schwartz, J., Lorente, J., et al. (2014). Effect of atmospheric mixing layer depth variations on urban air quality and daily mortality during Saharan dust outbreaks. Sci. Total Environ. 494–495, 283–289. doi: 10.1016/j.scitotenv.2014.07.004
Pay, M. T., Jiménez-Guerrero, P., and Baldasano, J. M. (2011). Implementation of resuspension from paved roads for the improvement of CALIOPE air quality system in Spain. Atmos. Environ. 45, 802–807. doi: 10.1016/j.atmosenv.2010.10.032
Querol, X., Alastuey, A., Moreno, T., and Viana, M. (2008). Spatial and temporal variations in airborne particulate matter (PM10 and PM2.5) across Spain 1999-2005. Atmos. Environ. 42, 3964–3979. doi: 10.1016/j.atmosenv.2006.10.071
Rodríguez, S., Querol, X., Alastuey, A., Kallos, G., and Kakaliagou, O. (2001). Saharan dust contributions to PM10 and TSP levels in Southern and Eastern Spain. Atmos. Environ. 35, 2433–2447. doi: 10.1016/S1352-2310(00)00496-9
Samet, J. M., Dominici, F., Curriero, F. C., Coursac, I., and Zeger, S. L. (2000). Fine particulate air pollution and mortality in 20 U.S. cities, 1987–1994. N. Engl. J. Med. 343, 1742–1749. doi: 10.1056/NEJM200012143432401
Skamarock, W. C., and Klemp, J. B. (2008). A time-split nonhydrostatic atmospheric model. J. Comput. Phys. 227, 3465–3485. doi: 10.1016/j.jcp.2007.01.037
Taylor, K. E. (2001). Summarizing multiple aspects of model performance in a single diagram. J. Geophys. Res. 106, 7183–7192. doi: 10.1029/2000JD900719
Thorpe, A., and Harrison, M. (2008). Sources and properties of non-exhaust particulate matter from road traffic: a review. Sci. Total Environ. 400, 270–282. doi: 10.1016/j.scitotenv.2008.06.007
Thorpe, A., Harrison, R. M., Boulter, P. G., and McCrae, I. S. (2007). Estimation of particle resuspension source strength on a major London Road. Atmos. Environ. 41, 8007–8020. doi: 10.1016/j.atmosenv.2007.07.006
Thunis, P. (2011). “DELTA: a benchmarking software to assess modeling performances related to EU Air Quality Directive applications,” in Harmonisation Conference on Dispersion Modeling for Regulatory Purposes (Kos).
Tsai, F. C., Apte, M. G., and Daisey, J. M. (2000). An exploratory analysis of the relationship between mortality and the chemical composition of airborne particulate matter. Inhal. Toxicol. 12(Suppl. 2), 121–135. doi: 10.1080/08958378.2000.11463204
UNC Carolina Environmental Program (2005). Sparse Matrix Operator Kernel Emissions (SMOKE) Modeling System. North Carolina: Carolina Environmental Programs - CEP, Research Triangle Park.
US-EPA (2011). AP-42, 5th Edn., Vol. 1, Chapter 13, Section 13.2.1. Miscellaneous Sources. Technical Report. U.S. Environmental Protection Agency.
Vautard, R., Bessagnet, B., Chin, M., and Menut, L. (2005). On the contribution of natural Aeolian sources to particulate matter concentration in Europe: testing hypothesis with a modeling approach. Atmos. Environ. 39, 3291–3303. doi: 10.1016/j.atmosenv.2005.01.051
Venkatram, A. (2000). A critique of empirical emission factor models: a case study of the AP-42 model for estimating PM10 emissions from paved roads. Atmos. Environ. 34, 1–11. doi: 10.1016/S1352-2310(99)00330-1
Yarwood, G., Rao, S., Yocke, M., and Whitten, G. (2005). Updates to the Carbon Bond Chemical Mechanism: CB05. Final Report to the US EPA, RT-0400675. Available online at: http://www.camx.com/publ/pdfs/CB05_Final_Report_120805.pdf
Keywords: air quality modelling, resuspension, CMAQ, OSPM, urban PM levels
Citation: de la Paz D, Borge R, Vedrenne M, Lumbreras J, Amato F, Karanasiou A, Boldo E and Moreno T (2015) Implementation of road dust resuspension in air quality simulations of particulate matter in Madrid (Spain). Front. Environ. Sci. 3:72. doi: 10.3389/fenvs.2015.00072
Received: 14 July 2015; Accepted: 19 October 2015;
Published: 04 November 2015.
Edited by:
Leonidas Ntziachristos, Aristotle University of Thessaloniki, GreeceReviewed by:
Eleni Drakaki, National Technical University of Athens, GreeceEduardo Landulfo, Instituto de Pesquisas Energéticas e Nucleares, Brazil
Christos H. Halios, University of Reading, UK
Copyright © 2015 de la Paz, Borge, Vedrenne, Lumbreras, Amato, Karanasiou, Boldo and Moreno. This is an open-access article distributed under the terms of the Creative Commons Attribution License (CC BY). The use, distribution or reproduction in other forums is permitted, provided the original author(s) or licensor are credited and that the original publication in this journal is cited, in accordance with accepted academic practice. No use, distribution or reproduction is permitted which does not comply with these terms.
*Correspondence: Rafael Borge, cmJvcmdlQGV0c2lpLnVwbS5lcw==