- 1School of Life and Environmental Sciences, The University of Sydney, Sydney, NSW, Australia
- 2WaterTechnology Pty, Ltd., Melbourne, VIC, Australia
- 3ARC ITTC Data Analytics for Resources and the Environment, The University of Sydney, Sydney, NSW, Australia
Drought is the most expensive natural hazard and one of the deadliest. While drought propagation through standardised indices has been extensively studied at the regional scale, global scale drought propagation, and particularly quantifying the space and time variability, is still a challenging task. Quantifying the space time variability is crucial to understand how droughts have changed globally in order to cope with their impacts. In particular, better understanding of the propagation of drought through the climate, vegetation and hydrological subsystems can improve decision making and preparedness. This study maps spatial temporal drought propagation through different subsystems at the global scale over the last decades. The standardised precipitation index (SPI) based on the gamma distribution, the standardised precipitation evapotranspiration index (SPEI) based on the log-logistic distribution, the standardised vegetation index (SVI) based on z-scores, and the standardised runoff index (SRI) based on empirical runoff probabilities were quantified. Additionally, drought characteristics, including duration, severity and intensity were estimated. Propagation combined the delay in response in the subsystems using drought characteristics, and trends in time were analysed. All these were calculated at 0.05 to 0.25 arc degree pixels. In general, drought propagates rapidly to the response in runoff and streamflow, and a with longer delay in the vegetation. However, this response varies spatially across the globe and depending on the observation scale, and amplifies progressively in duration and severity across large regions from the meteorological to the agricultural/ecological and hydrologic subsystems, while attenuating in intensity. Significant differences exist between major Köppen climate groups in drought characteristics and propagation. Patterns show intensification of drought severity and propagation affecting vegetation and hydrology in regions of southern South America, Australia, and South West Africa.
1 Introduction
Drought corresponds to a sequence of climate events triggered by ocean or atmospheric circulation conditions which results in rainfall deficits (Zargar et al., 2011; Yuan et al., 2017), leading to a landscape imbalance between water supply and demand (Ault, 2020). Drought conditions can extend in time having large environmental and socio-economical consequences (Apurv et al., 2017). Droughts have been identified as one of the most costly and deadly natural hazards (Ault, 2020), and could be one of the reasons for the collapse of ancient civilizations (Kerr, 1998; Gill et al., 2007). In recent decades, large drought episodes have occurred in different regions and have received different names, such as the millennium drought that occurred in Australia between 2001 and 2009 (Van Dijk et al., 2013) or the megadrought that has affected Chile since 2010 (Garreaud et al., 2020).
Drought can occur due multiple different atmospheric drivers. Atmospheric/oceanic circulation cycles strongly impact the development of dry/wet conditions, leading to interannual/interdecadal climate variability (Vicente-Serrano et al., 2011). Some examples of this are the El Niño Southern Oscillation (ENSO), the Pacific Decadal Oscillation (PDO), or the Indian Ocean Dipole (IOD), which all cause an oscillation in surface ocean temperatures (Mantua and Hare, 2002; Xiao et al., 2015). Other processes, like the Subtropical Ridge (STR) or the North Atlantic Oscillation (NAO), are mainly driven by atmospheric conditions that affect the atmospheric pressure at sea level (Hurrell et al., 2003; Grose et al., 2015). All these cycles strongly impact weather conditions across different time scales. However, anthropogenic impacts on the interannual climate variability as part of “climate change” are still difficult to quantify. Despite this, He and Li (2019) identified an overall increase in the interannual variability of rainfall associated with climate change across all longitudes between latitudes 20°S–50°N, while Zhu (2013) estimated an overall increase in rainfall intensities across the United States. In both cases, estimates were spatially variable. Additionally, climate change has decreased rainfall in Mediterranean regions, and an increase in temperatures is expected to increase evaporative demand, reduce snowfall and increase the ablation of glaciers, the soil water deficit and runoff, leading to an increase in drought risk and severity (Cook et al., 2018).
Drought conditions, triggered by oceanic/atmospheric circulation processes, propagate from the meteorologic subsystem, which is evident through a deficit in precipitation, to the hydrologic and agricultural subsystems (Wang et al., 2016), and therefore, referred to as a multi-scalar phenomenon (McKee, 1995). In terms of hydrology, drought may cause reduced discharge in streams, lower groundwater levels, and a reduction in reservoir storage (Maity et al., 2013). However in the hydrologic subsystem alone, drought may have different propagation rates. For example, in the surface drainage network, propagation may be relatively fast compared to the groundwater system, which depending on variables such as recharge rate and aquifer transmissivity, can have a substantial lagged response, which can also vary significantly across sites (Bloomfield and Marchant, 2013; Lorenzo-Lacruz et al., 2017; Han et al., 2019). Focusing on the surface drainage system, propagation rates can further depend on climate and catchment characteristics (Barker et al., 2016), and may be additionally modulated by water consumption in different reaches and water management (Yuan et al., 2017). Reservoirs, in particular, may play an important role in delaying drought impacts (Lorenzo-Lacruz et al., 2013). Water deficit conditions also lead to a propagation of drought in the agricultural/ecological subsystem, which results in less water in soils and consequently, vegetation stress conditions due to the water deficit (Son et al., 2012). These may additionally translate into economic deficits due to reductions in crop yields, and subsequently into societal distress and starvation, which may cause political instability (Sternberg, 2012). However, in each subsystem the propagation is modulated by different factors that exacerbate/attenuate their impacts.
One of the difficulties in evaluating drought, is related to the methods to characterise and quantify drought, given limitations in the length of historic data and the spatial coverage (Ault, 2020). Water deficits can be evaluated through water budget anomalies, and several standardised indices have been developed to characterize the magnitude of drought (Zargar et al., 2011; Ault, 2020). For instance, meteorologic drought can be quantified through the standardised precipitation index (SPI) (McKee et al., 1993), which has been further modified to include evapotranspiration (standardised precipitation evapotranspiration index; SPEI) (Vicente-Serrano et al., 2010; Guenang and Kamga, 2014). From a hydrologic perspective, indices may include runoff (standardised runoff index, SRI) (Shukla and Wood, 2008), the streamflow in channels (standardised streamflow index, SSI) (Vicente-Serrano et al., 2012), groundwater levels, or storage volumes in reservoirs (Bhuiyan et al., 2006; Nalbantis and Tsakiris, 2009). Likewise, drought has been monitored in agricultural/ecological systems by studying the impacts on vegetation trough the standardised vegetation index (SVI) (Peters et al., 2002), which uses the normalised difference vegetation index (NDVI) as a proxy for vegetation health, or through the standardised soil moisture index (SSMI) (Sohrabi et al., 2015).
The main advantage of these indices is that standardisation is based on simple methodologies which can be used to draw conclusions on the drought severity from the observed anomalies and allows comparison across different sites and scales. This may facilitate the communication of drought research results between institutions (Zargar et al., 2011). However, to have a better understanding of drought effects at different time and space scales, diverse data sources for drought monitoring involving different variables are needed, as well as a critical evaluation of how these indices are estimated and how they may relate with each other (Wanders et al., 2017; Trnka et al., 2018). In this regard, Lorenzo-Lacruz et al. (2010) found changes in the hydrologic response to droughts in regulated systems of the Tangus catchment, in Spain, due to the external demand after the implementation of a water transfer system to other basins. Van Loon et al. (2012) found drought events to become fewer and longer as these propagate through different subsystems, and also concluded that the drought propagation processes were reasonably well reproduced in some European catchments using an ensemble mean of large-scale hydrological models. Vicente-Serrano et al. (2013), studying the vegetation response to drought, found that different biomes vary in the time-scale response to drought due to vegetation adaptation characteristics. Barker et al. (2016) studied the variability in the drought propagation from the meteorological to the hydrological subsystem using standardised indices across different catchments in the United Kingdom, while Barella-Ortiz and Quintana-Seguí (2019) found uncertainties in the characterisation and propagation of drought in the hydrologic and soil moisture subsystems when evaluating regional climate models using standardised indices including SPI, SRI, SSI, and SSMI across Spain. Similarly, Peña-Gallardo et al. (2019) studied the relationships between meteorological and hydrological droughts in the conterminous United States and found a response of SSI to SPEI at short time scales, suggesting that elevation and vegetation play an important role in modulating this response. The authors also found a higher correlation between both indices in natural systems compared to regulated systems.
Given the importance of drought for society, the study of drought has led to an entire sub-discipline where different fields of science converge (Stahl et al., 2020). As a result of this, several unanswered questions for drought research have been identified (Trnka et al., 2018; Ault, 2020). This reinforces that there is still a need to better understand drought propagation, particularly in relation to time lags of propagation across the different hydroclimatic subsystems, and the factors that determine drought propagation (Wang et al., 2016; Trnka et al., 2018). Finally, changes in drought intensity and duration as drought transitions from one subsystem to another need to be studied.
While drought propagation analysis using standardised indices has been widely used at both local and regional scales (Bhuiyan et al., 2006; Barker et al., 2016; Loon et al., 2017; Xu et al., 2019; Zhou et al., 2019; Chen et al., 2020), few studies have addressed this at the global scale, and particularly in reference to the vegetation response to drought (Vicente-Serrano et al., 2013). Investigating the global scale is important, as the shifts and similarities in patterns at the global scale can deliver insights that are not visible at the local and regional scale. This study therefore aims to: 1) Characterise global drought through standardised indices using common average drought metrics (duration-severity-intensity), which evaluates how drought amplifies or diminishes across different subsystems. These include the meteorological subsystem through the evaluation of rainfall-evapotranspiration, the agricultural/ecological subsystem by assessing the vegetation, and the hydrological subsystem by studying modelled runoff and observed discharge. The groundwater subsystem was not included in this study because of the lack of information from global datasets that allows to differentiate monitoring wells dug in shallow alluvial aquifers from deeper aquifers, and the effect that active pumping from surrounding wells may have on the piezometric level of monitoring sites. Therefore, we prioritised surface water in the hydrological subsystem; 2) Quantify the global propagation of drought, i.e., the delay in response to drought across the different subsystems (meteorological, agricultural/ecological, and hydrological), and identify the spatial variation in this delay; 3) Discuss the implications of spatio-temporal changes of the drought characteristics across the last decades (1982–2019) for drought management and future global drought preparedness. It is clear that the 37 years selected for this study may constitute a limitation for the characterisation of drought variability on multidecadal time scales (Ault, 2020). However, this period might shed some light on average drought characteristics among subsystems and on drought propagation.
2 Materials and Methods
2.1 Datasets Used
Because this study has a global extent, several data sets at that scale are used for the analysis. Climate variables and vegetation were based on remote sensing products. Rainfall daily data was based on the Climate Hazards Group InfraRed Precipitation with Station Data (CHIRPS version 2.0) (Funk et al., 2015). CHIRPS daily data was monthly aggregated, with a spatial resolution of 0.05°, covering 1981 to the present. However, this coverage excludes the Northern Arctic regions. CHIRPS data has been validated in many regional studies, which in general indicate a good agreement between estimates and observed data (average r of 0.94 and 0.85 in Northeast Brazil and Cyprus, respectively) (Katsanos et al., 2016; Paredes-Trejo et al., 2017). However the data can have under- and over-predictions in extreme wet and dry events and the data can have a lower performance in mountainous regions (Dinku et al., 2018). Given possible discrepancies beyond those discussed in Funk et al. (2015), monthly observations from 3,301 weather stations across the world were obtained from the World Meteorological Organization (https://climexp.knmi.nl/). These were filtered to the CHIRPS coverage period and extent, which reduced their number to 2,978, and compared against CHIRPS predictions (Figure 1).
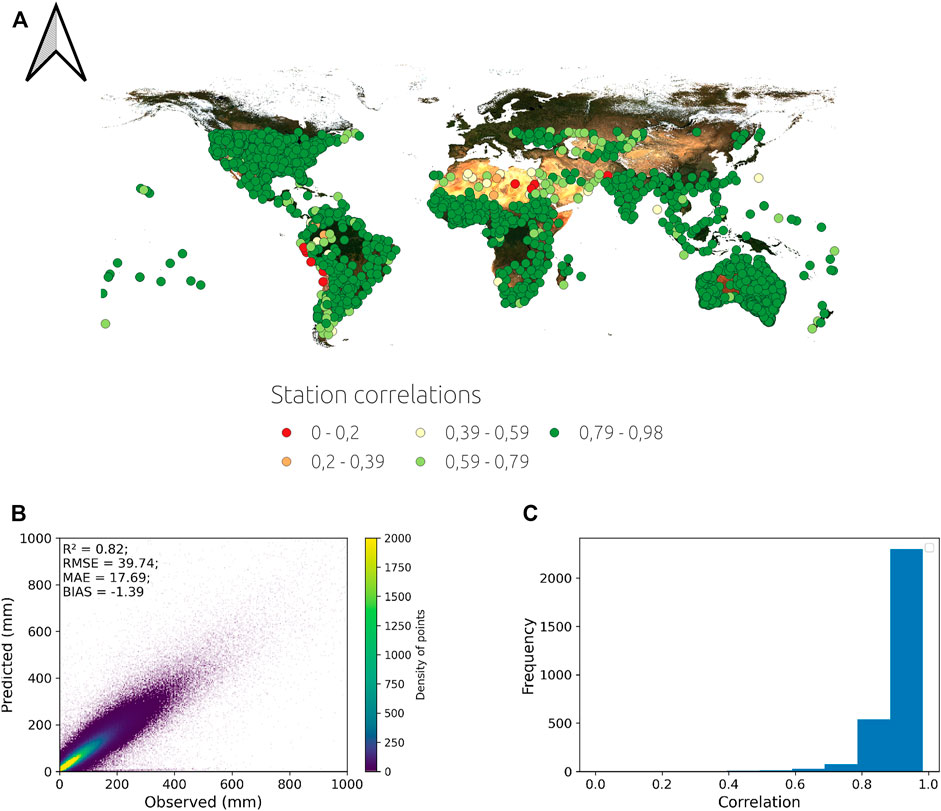
FIGURE 1. CHIRPS global validation against meteorological stations. The (A) presents a map with the correlation between meteorological stations and CHIRPS. The (B) presents a density scatter plot with monthly observations and predictions for all stations, while the (C) shows the histogram of the correlations.
At the global scale CHIRPS rainfall estimates are good, presenting globally on average an R2 of 0.82 (r
Monthly temperature, wind and surface pressure were based on the 0.25° European Centre for Medium-Range Weather Forecasts (ECMWF) Reanalysis v5 (ERA5) dataset, which is associated with the fifth generation ECMWF atmospheric reanalysis of the global climate (Hoffmann et al., 2019). Several of the ERA5 variables have been compared to other datasets, and have been described as equivalent to using observational data for large areas in North America (Tarek et al., 2020). Compared with the Modern-Era Retrospective analysis for Research and Applications, Version 2 (MERRA-2 reanalysis data), it outperforms that data set in all aspects related to wind (Olauson, 2018). Monthly incoming shortwave radiation from the Famine Early Warning Systems Network (FEWS NET) Land Data Assimilation System (FLDAS) was obtained at a 0.1° resolution (McNally et al., 2017).
The third generation Global Inventory Modeling and Mapping Studies (GIMMS) normalized difference vegetation index (NDVI) from the Advanced Very High Resolution Radiometer (AVHRR) sensors at five arc minute resolution was aggregated to monthly data for 1981 to 2014 (Pinzon and Tucker, 2014). We added the Moderate Resolution Imaging Spectroradiometer (MODIS) NDVI from Terra instruments (MOD13A2), which is available from 2001 to the present, but at a much higher resolution (1,000 m) (Didan, 2015).
Modelled mean monthly runoff rasters from the ERA5 collection were also included in the analysis to account for hydrological variables. Runoff corresponds to the fraction of water that flows through the surface or subsurface, which does not stay stored in the soils. Additionally, to evaluate hydrologic droughts using point measurements, level 4 basins from the HydroSHEDS dataset (Lehner and Grill, 2013) were combined with reference stations from the Global Runoff Data Centre (GRDC; https://www.bafg.de/GRDC/). These stations (and basins) were filtered based on drained areas of at least 10,000 km2, and discharge data was filtered from 1981. From the combined basins and gauging stations, stations located furthest downstream within each basin were selected for further analysis. Additionally, the degree of regulation attribute of the basins contained in the HydroATLAS version 1.0 dataset (Lehner et al., 2011) was appended to the stations to differentiate between regulated and natural systems.
The raster datasets were all accessed, pre-processed, and analysed using Google Earth Engine (Gorelick et al., 2017), while observational data was processed using Python libraries.
2.2 Pre-processing and Drought Indices
As discussed, there are several drought indices. In this study, we used the Standardised Precipitation Index (SPI), the Standardised Precipitation Evapotranspiration Index (SPEI), the Standardised Vegetation Index (SVI), and we also standardised the runoff to get a Standardised Runoff Index (SRI). These indices can be calculated for different time scales based on the sum of observations in a selected time window (Zhang and Li, 2020):
where
2.2.1 Standardised Precipitation Index
The SPI was standardised assuming a gamma distribution, as this distribution has been used as the benchmark for studying drought using positive hydroclimatic variables and is recommended for use at large scales (Stagge et al., 2015), which was implemented in Google Earth Engine (Guenang and Kamga, 2014):
where α and β are the shape and scale parameters.
2.2.2 Standardised Precipitation Evapotranspiration Index
Since SPEI requires the subtraction of rainfall and evapotranspiration, this last was calculated in Google Earth Engine by combining the ERA5 dataset with the FLDAS forcing radiation using the Food and Agriculture Organization (FAO) Penman Monteith equation (Pereira et al., 2015):
where ETr is the monthly reference evapotranspiration, Rn is the net radiation, G is the soil heat flux, Δ is the slope vapour pressure, T the mean temperature, es the saturation vapour pressure, ea the actual vapour pressure, γ the psychrometric constant, and u2 the wind speed at 2 m height.
The subtraction of precipitation and ETr can result in negative values. As a result, and based on earlier comparisons (Vicente-Serrano and Beguería, 2016), SPEI was standardised using a log-logistic distribution with three parameters (Beguería et al., 2010) in Google Earth Engine. The probability distribution function for the log-logistic distribution is:
where α, β, and γ are the parameters of the function that can be estimated from a probability weighted moments calculation. From this, P, i.e., the probability of exceeding a value of D (D stands for difference between rain and evapotranspiration), is calculated as 1−F(x). Finally, SPEI is calculated as:
where
2.2.3 Standardised Vegetation Index
Given that the GIMMS NDVI from the AVHRR sensors is discontinued after 2013, MODIS NDVI was used to generate a monthly continuous series from 1981 to the present. However, MODIS data had to be re-projected and re-scaled to the GIMMS projection and resolution. Additionally, since some artifacts arise from the difference between sensors, which are evident even when re-projecting and re-scaling MODIS, a correction using ordinary least squares (OLS) between sensors was used. Thus, GIMMS NDVI was modelled from MODIS NDVI based on a modification of the Mao et al. (2012) methodology, which is needed because of the seasonal NDVI variability. The modification consisted of using OLS on both datasets for each month using 10 years (2001–2010) of observations. A validation was carried out on 1,000 randomly distributed points sampled from 14 polygons drawn in different continents, comparing the predictions against the monthly GIMMS NDVI observations from 2011 to 2013 (36 months), which results in a total of 36,000 observations.
Validation results of merging AVHRR and MODIS NDVI are in Figure 2. The validation of the modelled NDVI has an error of around 5%, with a small bias of 0.003, and a determination coefficient of 0.96, which was considered acceptable for subsequent analysis.
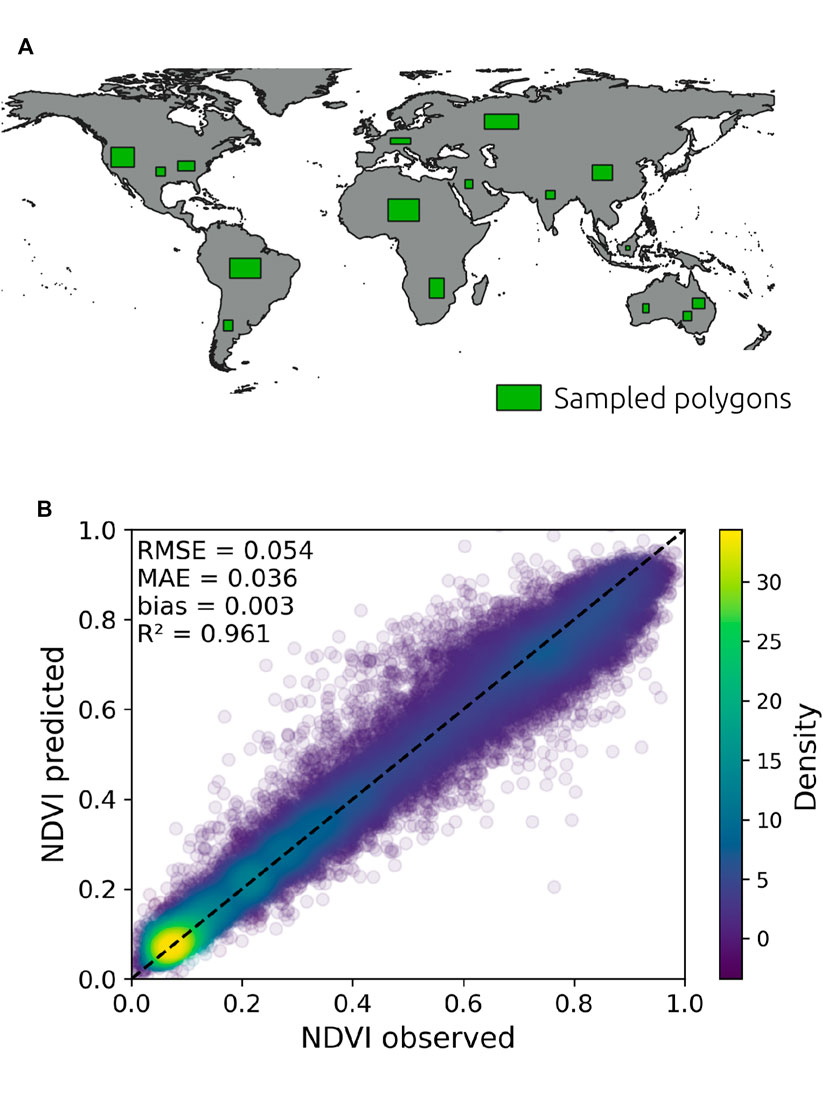
FIGURE 2. Validation of the combination of the GIMMS AVHRR NDVI and MODIS NDVI using monthly observations of GIMMS NDVI from 2011 to 2013. Polygons where random samples were taken for validation are presented in the (A), while the (B) shows a density scatter plot of the validation and associated statistics.
The SVI was then calculated following the original calculation described in Peters et al. (2002) based on a z-scores estimation, which is the deviation from mean values in standard deviation units:
where NDVIi,k is the NDVI for observation i at the time period k,
2.2.4 Standardised Runoff Index
Lastly, SRI was calculated using empirical probabilities that lead to non-parametric standardised indices based on the Gringorten plotting position (Farahmand and AghaKouchak, 2015; Wu et al., 2018):
being n the length of observations and i the rank of event x in the collection. Then, the inverse normal function needs to be applied to standardise the range of values:
where erfinv corresponds to the inverse error function. SRI was estimated for the ERA5 runoff rasters and the GRDC gauging stations. In this case, SRI was used because it allows to relax the assumption of representative parametric distribution types assigned to the data (Farahmand and AghaKouchak, 2015).
2.3 Calculations of Drought Characteristics
Drought indices describe wet and dry conditions based on time series records of different variables, and can be separated in dry/wet classes (Table 1) (Jain et al., 2015; Potopová et al., 2015).
While drought propagation can be defined as the delay in the drought response in the different subsystems, a characterisation of drought in each subsystem was calculated in Google Earth Engine to evaluate the amplification or diminishing of drought effects during drought conditions through subsystems.
Thus, based on a threshold defined at −1 (Van Loon and Van Lanen, 2013; Li et al., 2020), a drought period was assumed to be any period where the drought index goes below −1, corresponding to moderately to extremely dry conditions (Potopová et al., 2015). Drought duration has been defined in different ways (Halwatura et al., 2015; Cavus and Aksoy, 2020). For simplicity, drought duration was defined as the consecutive months of drought indices below −1, which are preceded and followed by values above −1. Severity, considered as the strength of droughts, refers to the cumulative effects of drought and is calculated as:
where Se corresponds to the drought severity for the e drought event, with D the drought duration (in months) and SI the estimate of the respective standardised drought index. The intensity of the drought was also estimated as the ratio of the drought severity and the drought duration.
2.4 Spatial and Temporal Analysis of Drought Characteristics
To study the lag response between meteorological, agricultural, and hydrological drought, the lag at the maximum cross correlation between the meteorological and the agricultural, and between the meteorological and hydrological drought indices was estimated at each pixel. Additionally, this analysis was evaluated at the basin scale by combining the hydrologic drought calculated from GRDC stations and mean meteorologic—agricultural/ecological droughts in the filtered basins. This was done because pixel values refer to surface estimations at a particular scale, but point observations such as terminal gauges in a catchment or pixels aggregated within the catchment refer to hydrologic/vegetational values representative of the catchment scale, and different study scales may lead to different conclusions (Joao, 2002). In addition, runoff corresponds to a modelled variable, while GRDC stations correspond to observational data, which might be useful as a source of comparison to evaluate the drought propagation using modelled data in the hydrologic subsystem.
However, since the time series are in general serially correlated, these were first prewhitened to convert at least one of them into white noise (Shumway and Stoffer, 2017). Therefore, multiband raster grids were downloaded and converted into arrays. The prewhitening used the auto-ARIMA function from the pmdarima library (Smith 2017) along the 0 axis (time) of the independent array (x-variable in the cross correlation analysis) and setting minimum and maximum autoregressive (p) and moving average (q) terms to 0 and 5 respectively, and a minimum and maximum differencing (d) of 0 and 2. The auto-ARIMA function fits an ARIMA model to the time series:
where ϕ are the constants associated with the autoregressive behaviour, θ corresponds to the parameters associated with the moving average in the model, and ϵ corresponds to the error terms, being:
The auto-ARIMA function identifies the (p, d, q) model parameters of ARIMA optimizing (minimizing) the Akaike Information Criterion (AIC). The x-variable to use in the cross correlation corresponds then to the residuals of the ARIMA model. Stationarity in the series is evaluated based on the d parameter from the ARIMA model. Subsequently, the selected ARIMA model is also fitted to the cross correlation y-variable and the residuals are used for the cross correlation. This makes the series stationary and removes serial correlation before the cross correlation analysis. The significance of the cross correlation was based on:
being r the correlation coefficient at lag k and n the length of the series.
A further hypothesis is that continued climate change would introduce a trend in the drought characteristics. Therefore a trend analysis using Ordinary Least Squares (OLS) was applied to the drought characteristics, rather than directly to the standardised indices since drought events are considered independent. The significance of the trend (p-value) was estimated using Google Earth Engine.
2.5 Major Köppen Climate Groups and Drought Propagation
Different climate characteristics might result in variations in drought, especially if droughts are calculated globally in a standardised way. Vectorial information of major Köppen climate groups from Rubel and Kottek (2010) was used to aggregate drought characteristics from the SPEI, SRI, and SVI and propagation characteristics (Figure 3). Thus, drought rasters were sampled using all groups and were compared using analysis of variance or the non-parametric Kruskal Wallis test to evaluate if major climate groups indicate drought differences and drought propagation differences. Additionally, a multiple comparison to evaluate differences between groups was carried out through the non-parametric Dunn’s multiple comparison test, setting a step-down method that uses Bonferroni to adjust the p-values (Glantz, 2002). For all these tests, a p-value
3 Results
3.1 Meteorological Drought
Since drought is associated with atmospheric and oceanic circulation patterns, and these are dynamic in time and space around the world, it is possible to observe large spatial differences in dry/wet conditions on any specific date. This is what can be observed in the Figure 4A for SPI and SPEI maps in July 2019. The upper and middle panels correspond to a graphical representation of a single SPI/SPEI record. The lower panel indicates the Pearson correlation between both series for 1981–2019 at each pixel, which shows high correlation in South America and the tropics, and more generally in high rainfall areas.
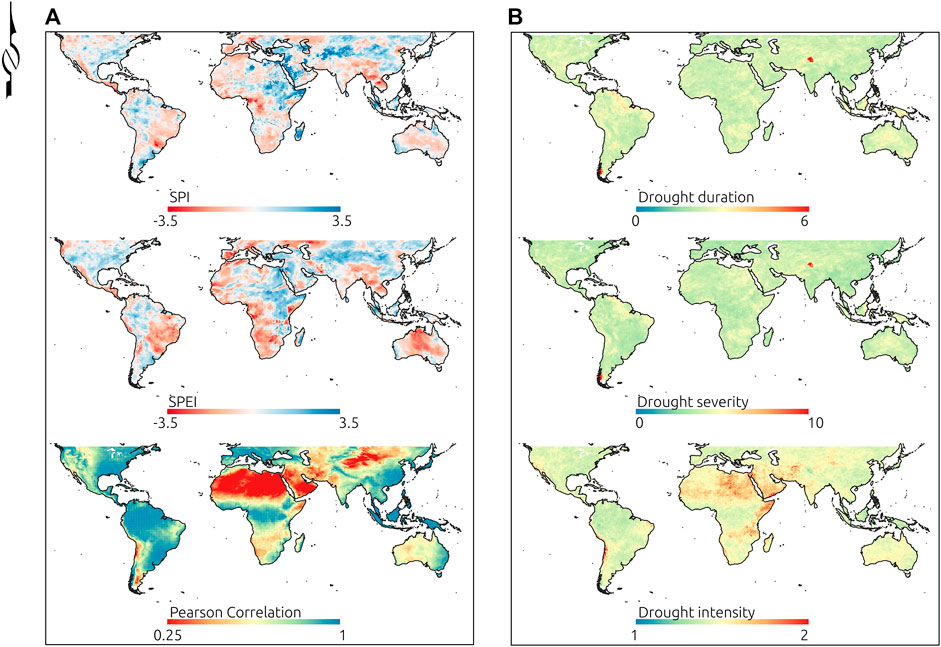
FIGURE 4. SPI—SPEI example maps for July 2019 and correlation between the entire time series of both indices (A) and 3-month SPEI drought characteristics (B).
Average meteorological drought characteristics estimated from the 3-month SPEI series are in Figure 4B. While the lowest drought duration regions are in northern latitudes (western Europe and Asiatic Russia), low duration can also be found in southern and eastern Asia. On the other hand, regions showing the largest drought duration seem to be constrained, in some cases, to areas with high annual rainfall, including some countries of Oceania such as Indonesia, Philippines, and Papua New Guinea, which can reach in average to more than 5 months of continuous drought (SPEI
In terms of drought intensity, northern Africa and western Asia have on average the largest values. In contrast, on average, lower intensities are found in the Amazon, Micronesia, Polynesia, and Melanesia regions. However, the lowest drought intensity also occurs around the Kashmir region, but neighbour areas of very high intensity are also visible.
3.2 Agricultural/Ecological and Hydrologic Drought
An example map of SVI for July 2019 is in the Supplementary materials (Supplementary Figure S1), while average agricultural drought characteristics are in the Figure 5A calculated using the entire series of 3-month SVI. Agricultural drought duration and severity increases in most regions compared to the meteorological drought using SPEI (Figure 4), except in Melanesia. For instance, while the mean meteorological drought duration in southern Africa is 2.1 months, its mean agricultural drought duration increases up to 2.4 months using SVI. In central Asia the mean drought duration increases from 2.4 to 3.1 months, in North America from 2.3 to 3 months, and in Australia and New Zealand from 2.5 to 3.7 months. Additionally, there is a general increase in spatial variability of drought duration in different regions using SVI, except in South America. The increase in variability is stronger in western Europe and Polynesia. The intensity of agricultural drought using SVI is in the lower Figure 5A. Higher intensities can be found in northern regions which can be associated with large severity of short duration events, since the intensity refers to the ratio between severity and duration.
An example map of the SRI index calculated for July 2019 and its correlation with SPEI are in the Supplementary materials (Supplementary Figure S2). Higher correlations between both indices occur in large areas of the United States, eastern sectors of Australia and Southern Africa when considering the entire time series. Similar to the other indices, average SRI characteristics are in the Figure 5B. Low duration and severity of drought occur in the Saharan and Middle East regions, where dry climates occur, but this is not extensive for Australian deserts, and low duration and severity also occur in central Asia and southern Africa. On the other hand, large values can be found in central Africa, to the east of the Andes mountain range in South America, in north western Australia and in western and eastern Europe. Intensities, in general, indicate an opposite behaviour relative to duration and severity.
3.3 Drought Propagation
Apart from the drought characteristics, which may describe how the drought affects the different subsystems in terms of duration, severity and intensity, the propagation was identified as the lag in the peak of correlation between drought time series in different subsystems.
Theoretically, drought propagation progresses from the meteorological subsystem (SPEI) to the hydrologic subsystem (SRI), passing through the agricultural subsystem (SVI) (Wang et al., 2016). Figure 6 shows the time series of SPEI (red and blue colours) and the SVI propagation (grey colour) at different global locations. Different patterns are visible at each of these locations. For instance, Australia (New South Wales) (E) shows a pattern with clear drought events after 2012, characterised by low SPEI, which amplifies the duration in the SVI series. A similar pattern can be observed in California, United States (A), and in central Chile (B), especially after 2008, while an opposite behaviour can be detected in Greece (C).
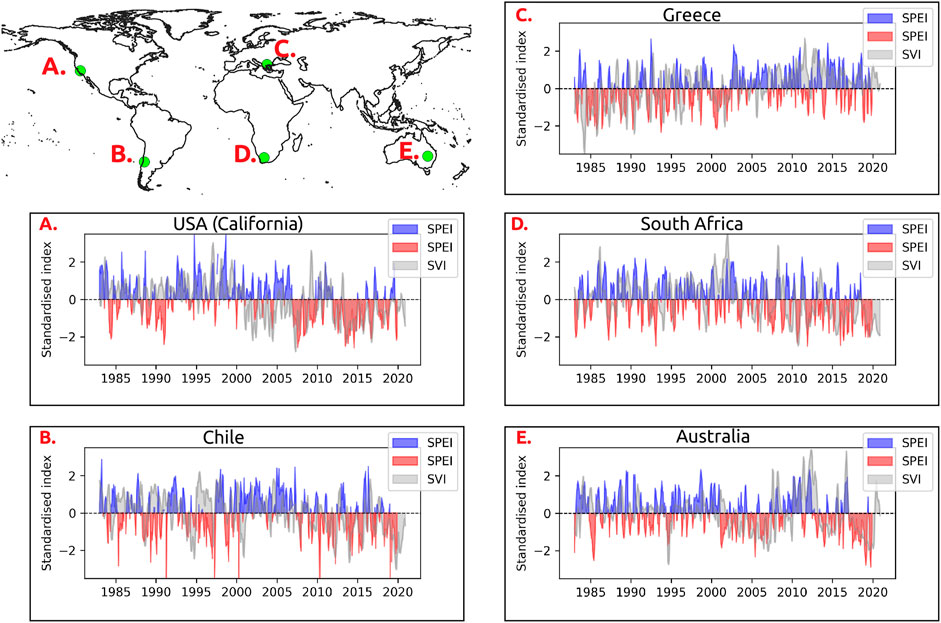
FIGURE 6. SPEI and SVI time series in different locations. Blue and red colours in plots correspond to wet and dry conditions in the SPEI time series, respectively, while grey colours correspond to the SVI series.
The lagged responses from the meteorological (SPEI) to the other subsystems (Figures 7A,C) indicate how these vary depending on the location and the subsystem being evaluated. The vegetation subsystem response (SVI) can be direct with maximum cross correlation with SPEI at lag 0. This seems to occur especially in Temperate and Dry climates such as in the eastern part of Australia, the pampas area (Argentina) in South America, southern and eastern Africa, and south central United States. These regions also tend to present larger maximum cross correlation values between both indices (Figures 7B,D). However, in most cases the response is delayed several months. In equatorial forest areas like the Amazon and central Africa, a large lag can be observed, but this also occurs in mid latitude regions, such as northern North America. In contrast to what is generally described in the literature (Zargar et al., 2011; Wang et al., 2016), the response in the hydrologic subsystem, through runoff, is mainly direct and minima and maxima are at the same time as the SPEI, but this occurs on a pixel basis. However, there are also some patches where large lags occur between SPEI and SRI, for instance in the Mountainous western region of United States, or surrounding the Great lakes in the United States and Canada, to the west of the Andes mountain range in South America, and spread out in several regions of Europe, and across eastern and central Asia.
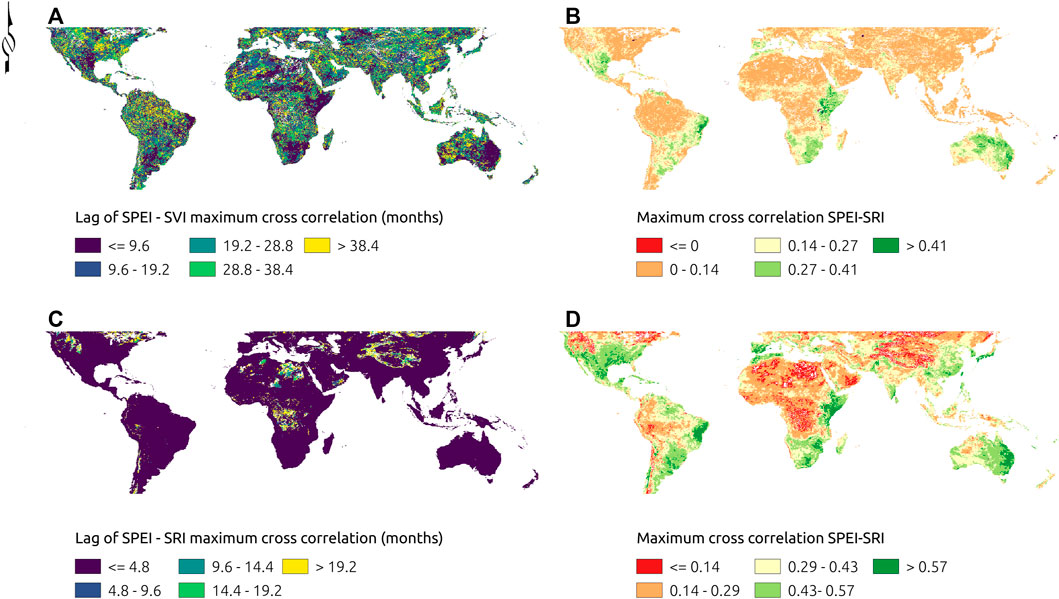
FIGURE 7. SPEI-SVI (A,B), and SPEI-SRI (A,C) response lag (A,C) corresponding to the maximum cross correlation (B,D) between variables. White pixels correspond to locations where the maximum cross correlations were non-significant.
Basin aggregated lagged responses in the agricultural/ecologic and hydrological subsystems are in Figure 8 and tend to confirm the results found on a pixel basis. Again, it is evident that the hydrological response tends to be faster than the vegetation response, except in some particular basins, such as the Great Plains in North America, to the east of the Andes in South America, or downstream of the Tsimlyanskoya reservoir in Russia and the Guanting reservoir in China. These are most likely all snow/glacier driven systems. From the 403 basins filtered in the preprocessing steps, 302 and 233 had significant SVI-SPEI and SRI-SPEI cross correlation values, respectively. For the maximum SRI-SPEI cross correlation at lag (month) 0 there were 101 basins, while 121 basins indicate lags between 1 and 3, and 11 basins indicate lags greater than 3. On the other hand, 49 basins indicate a maximum SVI-SPEI cross correlation at lag 0, while 48 basins were between 1 and 3 months, 39 basins between 3 months and 1 year, and 166 longer than 1 year.
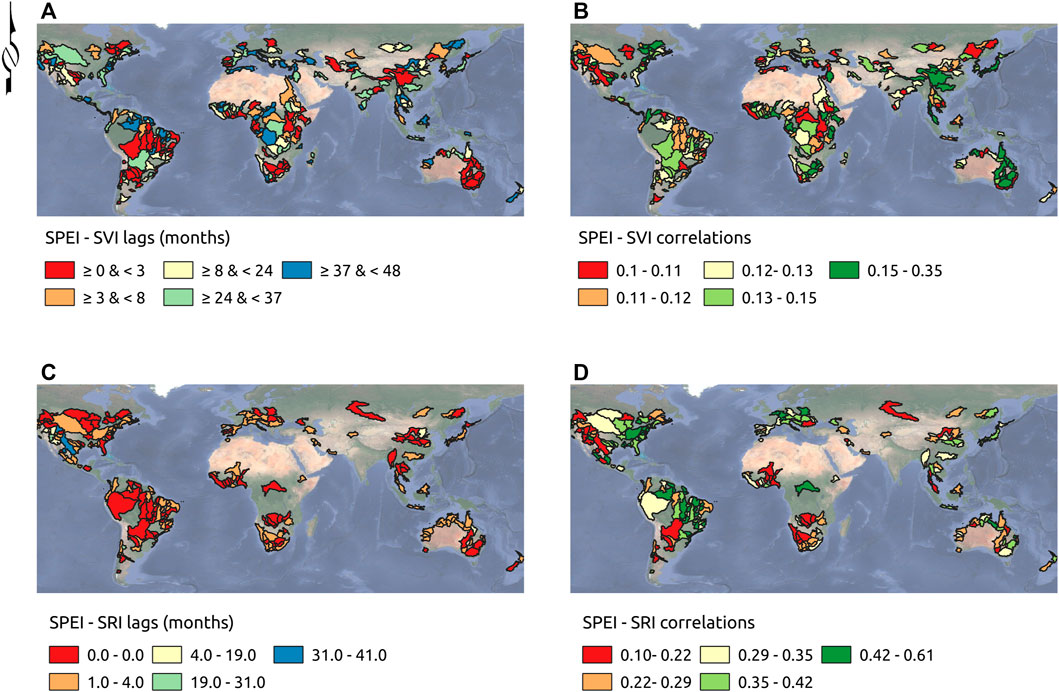
FIGURE 8. Basin aggregated SPEI-SVI (A,B), and SPEI-SRI (A,C) response lags (A,C) corresponding to the maximum cross correlation (B,D) between prewhitened average aggregated SPEI/SVI and SRI calculated from gauging stations. Basins with non-significant cross correlations were masked out.
Differences between regulated and natural basins are in Figure 9. Natural drainage systems indicate higher and more significant correlations between SPEI and SRI, which was confirmed using the Kruskal Wallis test (p-value
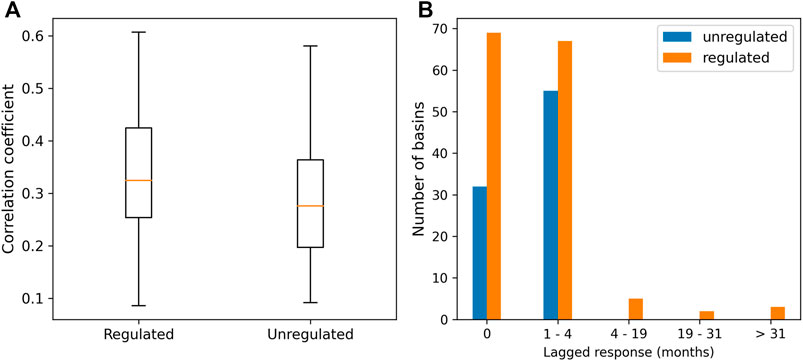
FIGURE 9. Maximum cross correlation coefficient distributions (A) and significant lagged response categories [months; (B)] between 3-month SPEI and 3-month SRI for regulated and unregulated basins.
Additionally, by subtracting the average drought characteristics in each subsystem, the change in duration and intensity can be evaluated (amplification or attenuation of drought) in the different subsystems. Figure 10 shows the difference between SVI and SPEI in terms of duration (months), severity and intensity (left panel).
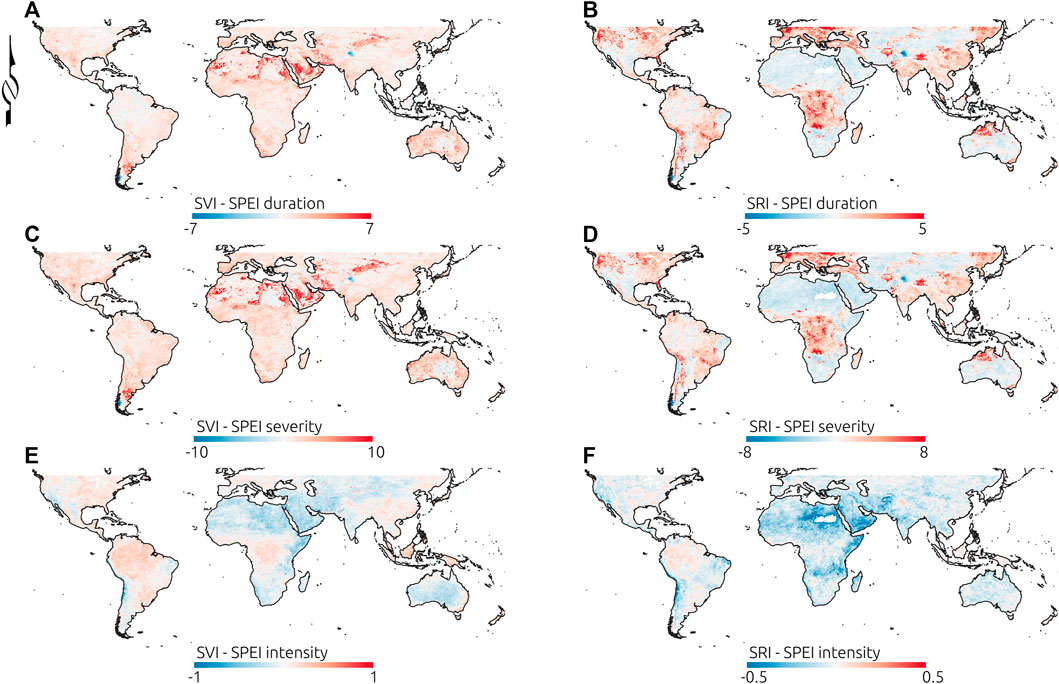
FIGURE 10. Duration (A,B), severity (C,D) and intensity (E,F) mean differences between SVI and SPEI (A,C,E) and between SRI and SPEI (B,D,F).
Drought duration and severity amplify in large regions of the mid-latitudes. In contrast, smaller patches of attenuation can be observed in between those areas, probably associated with vegetation patches. Small areas in the northern South America, around the Great Lakes in North America, in the Malay Archipelago, and in the Kashmir region indicate drought duration/severity attenuation in the agricultural/ecological subsystem. Drought intensity, on the other hand, attenuates in several regions. It also shows an opposite behaviour compared to duration and severity in dry regions where these strongly amplify, such as in the Argentinian Patagonia, western Australia, some regions in northern Africa and middle East. In these regions agricultural/ecological drought intensity reduces to about half of the magnitude of meteorological drought intensity.
The difference of drought characteristics between SRI and SPEI are in the right panel of Figure 10. In general, duration and severity indicate a larger heterogeneity compared to the difference between SVI and SPEI. Drought duration/severity attenuation in the hydrological subsystem can be seen in the Saharan and sub-Saharan regions, Middle East, central Australia, southern Africa, and the Patagonia, while amplification can be found to the west of Los Andes and in large extents of Brazil, in eastern North America, Europe, central Africa, and eastern Asia, among others. Similar heterogeneity between drought intensity amplification/attenuation regions can be observed across the world.
3.4 Spatial and Temporal Drought Patterns
The trends based on the OLS analysis and associated levels of significance for the 3-month SPEI drought characteristics are in Figure 11. Trends in the duration, severity and intensity of meteorological drought are quite variable across the globe. Concerning are increases in drought characteristic trends which can be observed in western Europe (Portugal and Spain), western regions of North America, Central America, Australia, southern South America, south west Africa, and eastern Russia. In all those regions droughts are increasing, either in duration, severity, or intensity, or in all of these.
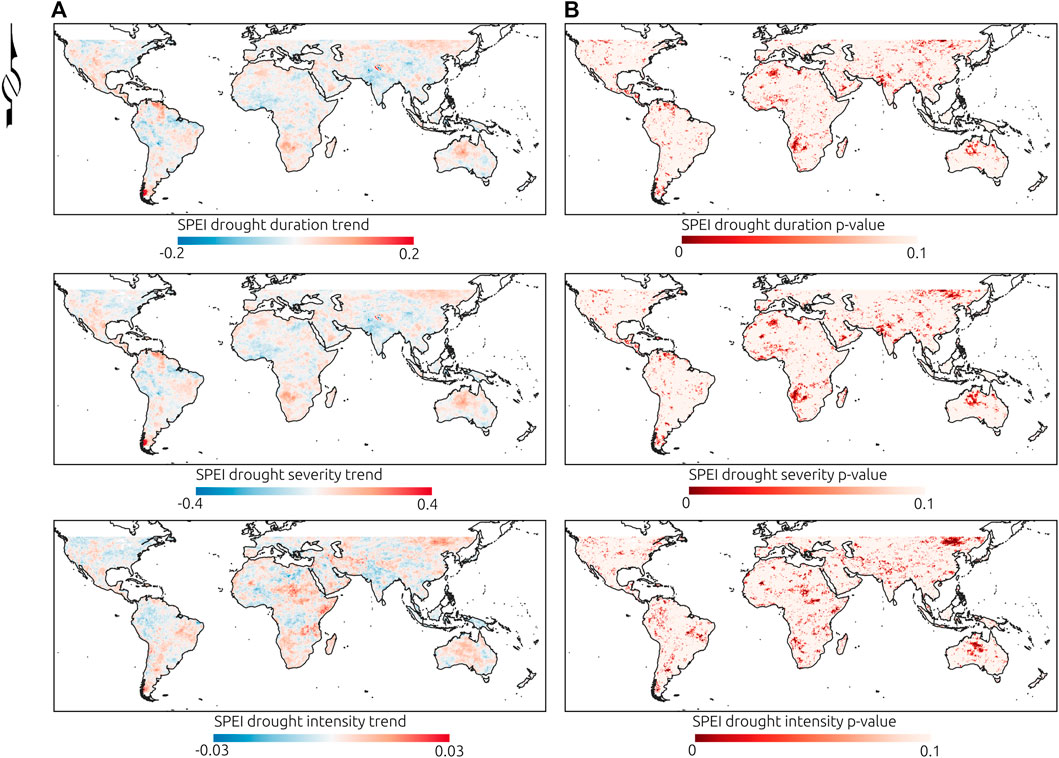
FIGURE 11. Annual trends in the meteorological characteristics (A) and the significance of the trends from the OLS analysis (B) obtained using 3-month SPEI.
Similarly, 3-month trends in SVI drought characteristics follow slightly different patterns, but again increasing trends occur in southern South America, the Northern Territory and Western Australia, south west Africa, and regions in central and eastern Asia (Supplementary Figure S3). On the other hand, strong and significant increasing trends in drought effects for runoff can be detected in central Africa, central Australia, to the east of the Andes mountain range in South America and in central and eastern Europe (Supplementary Figure S4).
Heat maps of the severity of drought based on the 3-month SPEI for latitude and longitude are in Figure 12. About four severe global drought events can be observed surrounding the equator (mean SPEI severity
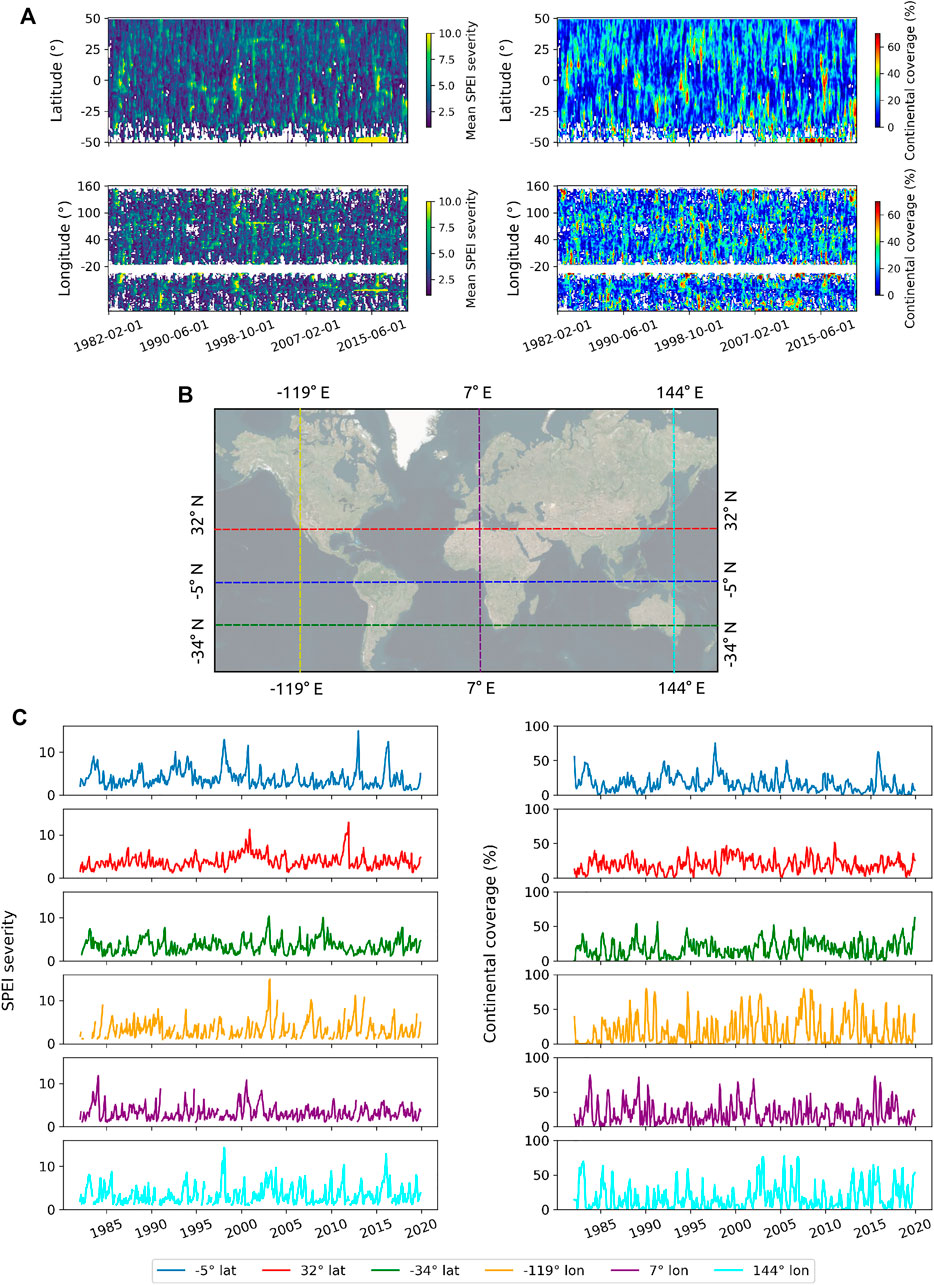
FIGURE 12. Heat maps (A) of mean 3-month SPEI severity by latitude and longitude (B) with the corresponding latitudinal and longitudinal continental coverage [percentage of latitudinal/longitudinal continental extent affected by drought; (C)]. Examples of three parallel and meridian mean SPEI severity time series and their corresponding continental extents (%) are in the middle and lower panels.
The heat map for SVI (Figure 13A) indicates quite a different behaviour. The drought observed in 1983 through SPEI record can also be detected in the SVI heat map. During the 1994–1995 a large drought is evident in the vegetation based index, covering a large extent of continental surface, but with the largest severity close to the equator. Then, after a long gap (1995–2000), some drought events are observed through the vegetation index which increase in severity and propagate longer in time, especially after 2005. Another obvious drought event occurs around the 25°parallel, which propagates over 3–4 years. The same can be observed surrounding the -25°parallel, which would be consistent with the millennium drought described in Australia (Van Dijk et al., 2013).
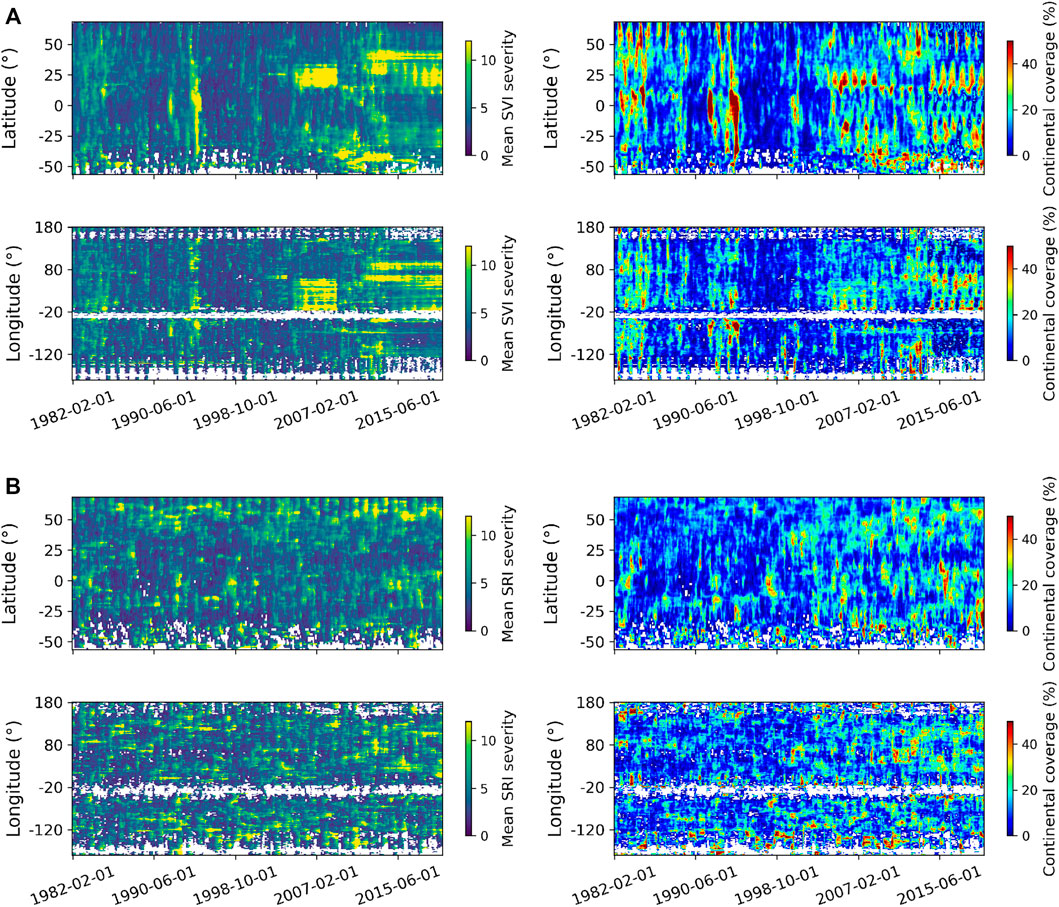
FIGURE 13. Heat maps of mean 3-month SVI severity (A) and 3-month SRI severity (B) by latitude and longitude with the corresponding latitudinal and longitudinal continental coverage (right panels).
The largest severity in SRI occurs above the 50°parallel (Figure 13B). However, severe hydrological drought events can also be seen in equatorial and southern latitudes. Again, the drought events detected surrounding the equator (1983, 1993, 1998, 2016) can be also detected in the SRI severity. But one thing that stands out is the increase over time in SRI severity and its propagation period, especially after 2008, in northern latitudes, and the increase and spread in the continental coverage with time, which means that droughts are extending in space in the hydrologic subsystem.
Spatial patterns in the difference of drought impacts between subsystems are in the Supplementary materials (Supplementary Figure S5). While in the meteorological subsystem drought severity tends to follow some sort of interannual variability, which is especially evident surrounding the equator, with a limited propagation period, such events extend to northern and southern parallels in the vegetation subsystem, but also increasing in severity and with longer propagation from 2005. The propagation also occurs in the hydrological subsystem, but not as severe as in vegetation, and tend to increase in continental coverage in low and high latitudes, while diminishing in extent surrounding the −25 and 25°parallels.
3.5 Major Köppen Climate Groups and Drought
The distribution of pixels sampled from the mean SPEI, SVI, and SRI drought characteristics based on the major Köppen climate groups are in the Supplementary materials (Supplementary Figure S6). In general, greater homogeneity can be observed through more compact violins and smaller y-scale range of values. Even though distributions appear quite homogeneous in some cases, the Kruskal Wallis test indicated significant differences (p value
Additionally, Figure 14 highlights the difference between SVI and SPEI (left panel), and between the SRI and SPEI mean characteristics (right panel). The Polar climate group has the lowest drought duration difference between SVI and SPEI, while the largest differences occur in Dry climates, which show larger variations, but also the lowest intensity differences. Significant differences using Kruskal Wallis were found between Köppen climate groups, and in most cases, these differences were significant comparing between groups (Supplementary Tables S10–S15).
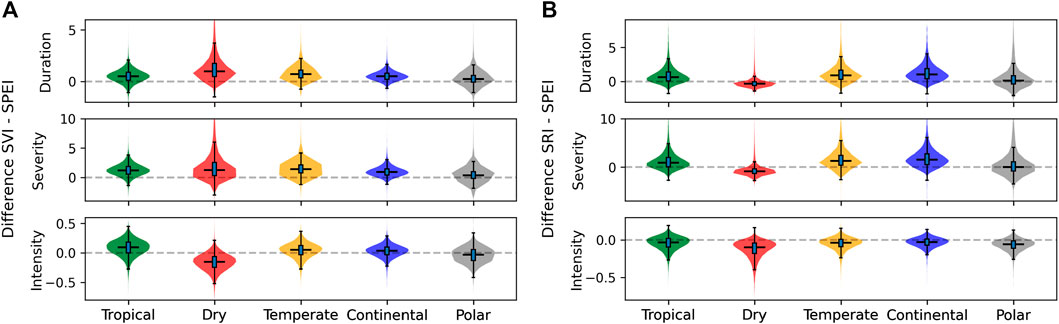
FIGURE 14. Differences of drought characteristics between SVI and SPEI (A) and between SRI and SPEI (B) for major Köppen climate groups.
The largest differences between SRI and SPEI occur in Continental climates, while the Polar and Dry climate groups contain the smallest differences. Differences between SRI and SPEI differ in magnitude relative to SVI—SPEI differences, especially conditional on climate groups. Thus, for instance, the median difference in duration between SVI and SPEI is 0.5 for the Tropical, 1.0 for the Dry, 0.7 for the Temperate, 0.5 for the Continental, and 0.2 months for the Polar climates. However, these differences change to 0.6, −0.3, 0.9, 1.0, and 0.1 months for the difference between SRI and SPEI.
4 Discussion
Drought propagation and temporal changes are spatially variable across the globe and linked to climate groups. Correlations between meteorological indices (SPI and SPEI) depend on the precipitation abundance or the dominance of precipitation relative to evapotranspiration. For example, lower correlations can be observed in mid latitudes associated with areas with arid and desert climates, characterised by low rainfall and elevated evapotranspiration. Generally speaking, drought progresses through the subsystems and, as in Peña-Gallardo et al. (2019), this varies by location. The propagation lag increases from the runoff to the vegetation subsystems. Similar to other studies (Lorenzo-Lacruz et al., 2013; Sattar et al., 2019), runoff or streamflow, in most cases, have a maximum response to meteorological droughts at short time lags, e.g., in the same month on a pixel basis, or during the next few months if aggregated to a larger scale. Additionally, the severity and duration of drought tend to amplify across large regions moving from meteorological to the agricultural and the hydrological subsystems, but attenuating in intensity on dry and polar climates. However, the dominant idea that drought progresses following the meteorological-agricultural-hydrologic order (Zargar et al., 2011; Wang et al., 2016) does not necessarily apply to all regions, at least in terms of the time lag of response among subsystems (start of drought event in subsystems). In some places, the hydrological response to rainfall through runoff is almost immediate and may precede the vegetation response to drought. Differences in this lagged response would depend on catchment characteristics, land cover, vegetation, climate, and water management (Lorenzo-Lacruz et al., 2010; Vicente-Serrano et al., 2013; Barker et al., 2016; Yuan et al., 2017; Ding et al., 2021). For example, runoff/discharge may proceed faster in areas with large rainfall and with a large slope gradient, while vegetation in semiarid and subhumid biomes may take several months to respond to meteorological droughts (Vicente-Serrano et al., 2013). Yet the streamflow response to meteorological droughts may be delayed/affected by geologic/geomorphic features, the location of the gauging station relative to the basin, water storage, and water usage (Lorenzo-Lacruz et al., 2013), which may explain the drop in stations that had significant cross correlation values between SRI and SPEI, clearly distinguished among regulated river systems as in Peña-Gallardo et al. (2019).
Vegetation, on the other hand, may have drought adaptation strategies (Vicente-Serrano et al., 2013), and can strongly rely on groundwater either by irrigation (Siebert et al., 2010) or due to root growth exploring the vadose zone and even reaching aquifers (Miller et al., 2010). In this case, groundwater has been reported to have a delayed response to meteorological droughts of on average 20.1 months in the United States (Schreiner-McGraw and Ajami, 2021), which might translate into a delayed response of vegetation in areas that rely extensively on groundwater resources. Additionally, different indices can lead to different results in the lagged response of subsystems. For instance, if soil moisture is used as an indicator of agricultural drought as proposed by (Zargar et al., 2011) instead of vegetation, it might lead to a faster response than the hydrological subsystem (runoff and streamflow).
While the characterisation of meteorological drought through SPEI and SPI is widely accepted (Hayes et al., 2011; Zargar et al., 2011; Wang et al., 2016; Ault, 2020), agricultural drought indices based on NDVI are less well accepted (Hayes et al., 2011). In this case, SVI, as any vegetation standardised drought index, can be questionable, since land cover changes affect the continuity of the NDVI series, which may translate into abrupt changes, causing non-stationary behaviour (Karnieli et al., 2010; De Keersmaecker et al., 2017). Additionally, NDVI can also be affected by natural hazards, such as fires and floods (Zargar et al., 2011) and agricultural practices (harvesting). These changes can obscure the real drought response and might affect time series analysis as well as trend detection (Peters et al., 2002). However, while at the local scale these are considered relevant, at a global scale these effects may attenuate. Other indices, such as those using soil moisture, have a shorter data length, which limits their applicability (Ault, 2020).
The global drought characteristic and propagation analysis presented here can be also extrapolated to include other hydrological and economic subsystems (Wang et al., 2016; Jehanzaib and Kim, 2020). However, a clear identification of the targets for drought evaluation need to be defined. Additionally, the spatial representation of the relevant data needs to be addressed. For instance, gauging stations, monitoring wells and reservoirs refer to point estimates that, in some limited cases, can be representative of catchment conditions. This means boundaries of the spatial units for the properties being evaluated and the aggregation of meteorological or agricultural/ecological data to be used needs to be considered. Additionally, while raster data is commonly available at the global scale, hydrological streamflow data cannot really be represented well at the global scale. More general hydrological variables, such as GRACE records, have disadvantages, including low spatial resolution and relatively short length of records (Li et al., 2019). In the present study, we choose to use runoff, which is frequently available as a modelled variable in a gridded format. However, using other variables, such as streamflow or groundwater, may lead to a different propagation behaviour given the lagged response of these variables (Kuss and Gurdak, 2014; Lorenzo-Lacruz et al., 2017), which was observed in a slight increase in the lagged response using catchment discharge.
Additionally, a multi-scale analysis should be considered when studying drought and the potential effect of water usage (McKee, 1995; Lorenzo-Lacruz et al., 2010), because it requires of a regional/local component for water withdrawals (Loon et al., 2019; Rangecroft et al., 2019). As stated by Barker et al. (2016), different catchment characteristics may be responsible for the drought propagation, but also different reaches within the same river might vary in the drought propagation and response given water withdrawals (Yuan et al., 2017). This means quantifying drought may require a nested scale analysis given the complexity of processes.
Mitigation plans for drought require consideration of local/regional climate characteristics and drought impacts in different subsystems by policy makers and planners (Wilhite, 2016) given the clear spatial variation in drought propagation observed here. Drought monitoring and knowledge of drought propagation characteristics and trends give governments further tools to develop national scale drought preparedness plans. This means resources can be prioritised to cope with drought based on the likelihood drought will amplify in the agricultural/hydrological subsystems and in time at certain locations. Additionally, the lagged drought response between subsystems can be used as an early warning by decision makers to trigger drought mitigation actions and reduce socioeconomic impacts. Similarly, at the global scale, the study of drought propagation can help to identify spatially connected regions prone to drought impacts. Overall this study maps the global temporal changes in drought and the regional extent, likely partly associated with climate change. This can help global aid and development organizations to delineate strategies for drought impact mitigation and water and food security improvement. For instance, the definition of drought resistant crops and promotion in regions/countries where drought strongly amplifies in the agricultural/ecological subsystem can reduce drought effects (Wang et al., 2014). Groundwater storage is an important subsystem to consider for drought mitigation at regional scales (Wendt et al., 2021). In drought prone regions, replenishing groundwater and soil water stocks in wet periods should be further promoted to increase water security in dry periods (Zhang et al., 2020), as groundwater reduces the large evaporation losses associated with water storage in dams (Zhao and Gao, 2019; Fuentes et al., 2020). On the other hand, a clear global concern is the increasing hydrological drought extent observed in the last years (Figure 13B) and its severity increase in northern latitudes. Drought effects in the hydrological and agricultural/ecological subsystems are clearly increasing in some regions, which can also be observed in trend analysis. This increase in the drought effects in these subsystems may arise as a response to a loss in catchment memory caused by a reduction in storage capacity due to climate change, which needs to be addressed.
Different drivers can lead to spatially variable dry/wet conditions (Schubert et al., 2016) such as those evaluated here. Here we observed that major climate groups lead to differences in the drought characteristics and in the propagation of drought. However, no evaluation of changes in atmospheric/oceanic circulation patterns was carried out such as in Van Dijk et al. (2013) at a smaller scale. Additionally, there is no clarity on where different drivers have preeminence at the global scale, nor the interaction between these and their hierarchical importance. Since differences in drought propagation arise, it is necessary to understand why such differences occur. These are questions that we will address in future research.
5 Conclusion
Drought differs in average characteristics based on different standardised drought indices. Its effects vary spatially and lead to a propagation from the meteorological subsystem towards the agricultural/ecological and hydrologic subsystems. However, the lag in the response from the meteorologic subsystem to other subsystems is also spatially variable but in general faster towards the hydrological subsystem, while this propagation is much slower reflected in the vegetation subsystem. Drought can both amplify and attenuate from one subsystem to the other, driven by differences in major Köppen climate groups. However, drought duration and severity tend to amplify progressively into the agricultural/ecologic subsystem, especially under Dry and Temperate climates, and into the hydrological subsystem, especially under Continental and Temperate climates, while attenuating in intensity in Dry and Polar climates. Drought characteristics have intensified in the last decades in several regions of the world, including areas in southern South America, central Australia, south west Africa, and central and eastern Asia, and these changes are much more evident in the vegetation and hydrological subsystems, which may be explained by a decrease in catchment memory as a consequence of climate change. The results of this study highlight the need for policy and decision makers to consider the global space and time relationships to prioritise resources for drought mitigation plans.
Data Availability Statement
The datasets presented in this study can be found in online repositories. The names of the repository/repositories and accession number(s) can be found below: https://github.com/IFuentesSR/FES_drought/.
Author Contributions
The original idea was developed by IF, and discussed with RV and JP; IF carried out the analysis and wrote the original draft, which was reviewed by RV and JP; IF wrote the final manuscript based on RV and JP suggested changes.
Funding
European Union’s Horizon 2020 Research and Innovation Programme (H2020-SPACE-2018-2020); Grant Agreement No. 870344.
Conflict of Interest
IF was employed by the company WaterTechnology Pty, Ltd.
The remaining authors declare that the research was conducted in the absence of any commercial or financial relationships that could be construed as a potential conflict of interest.
Publisher’s Note
All claims expressed in this article are solely those of the authors and do not necessarily represent those of their affiliated organizations, or those of the publisher, the editors and the reviewers. Any product that may be evaluated in this article, or claim that may be made by its manufacturer, is not guaranteed or endorsed by the publisher.
Supplementary Material
The Supplementary Material for this article can be found online at: https://www.frontiersin.org/articles/10.3389/fenvs.2022.788248/full#supplementary-material
References
Abramowitz, M., and Stegun, I. A. (1964). Handbook of Mathematical Functions with Formulas, Graphs, and Mathematical Tables, 55. Washington: US Government printing office.
Apurv, T., Sivapalan, M., and Cai, X. (2017). Understanding the Role of Climate Characteristics in Drought Propagation. Water Resour. Res. 53 (11), 9304–9329. doi:10.1002/2017wr021445
Ault, T. R. (2020). On the Essentials of Drought in a Changing Climate. Science 368 (6488), 256–260. doi:10.1126/science.aaz5492
Barella-Ortiz, A., and Quintana-Seguí, P. (2019). Evaluation of Drought Representation and Propagation in Regional Climate Model Simulations across spain. Hydrol. Earth Syst. Sci. 23 (12), 5111–5131. doi:10.5194/hess-23-5111-2019
Barker, L. J., Hannaford, J., Chiverton, A., and Svensson, C. (2016). From Meteorological to Hydrological Drought Using Standardised Indicators. Hydrol. Earth Syst. Sci. 20 (6), 2483–2505. doi:10.5194/hess-20-2483-2016
Beguería, S., Vicente-Serrano, S. M., and Angulo-Martínez, M. (2010). A Multiscalar Global Drought Dataset: the Speibase: a New Gridded Product for the Analysis of Drought Variability and Impacts. Bull. Amer. Meteorol. Soc. 91 (10), 1351–1356. doi:10.1175/2010bams2988.1
Bhuiyan, C., Singh, R. P., and Kogan, F. N. (2006). Monitoring Drought Dynamics in the Aravalli Region (india) Using Different Indices Based on Ground and Remote Sensing Data. Int. J. Appl. Earth Observation Geoinformation 8 (4), 289–302. doi:10.1016/j.jag.2006.03.002
Bloomfield, J. P., and Marchant, B. P. (2013). Analysis of Groundwater Drought Building on the Standardised Precipitation index Approach. Hydrol. Earth Syst. Sci. 17 (12), 4769–4787. doi:10.5194/hess-17-4769-2013
Cavus, Y., and Aksoy, H. (2020). Critical Drought Severity/intensity-Duration-Frequency Curves Based on Precipitation Deficit. J. Hydrol. 584, 124312. doi:10.1016/j.jhydrol.2019.124312
Chen, N., Li, R., Zhang, X., Yang, C., Wang, X., Zeng, L., et al. (2020). Drought Propagation in Northern china plain: A Comparative Analysis of Gldas and Merra-2 Datasets. J. Hydrol. 588, 125026. doi:10.1016/j.jhydrol.2020.125026
Cook, B. I., Mankin, J. S., and Anchukaitis, K. J. (2018). Climate Change and Drought: From Past to Future. Curr. Clim. Change Rep. 4 (2), 164–179. doi:10.1007/s40641-018-0093-2
De Keersmaecker, W., Lhermitte, S., Hill, M., Tits, L., Coppin, P., and Somers, B. (2017). Assessment of Regional Vegetation Response to Climate Anomalies: A Case Study for australia Using Gimms Ndvi Time Series between 1982 and 2006. Remote Sensing 9 (1), 34. doi:10.3390/rs9010034
Didan, K. (2015). Mod13a2 Modis/terra Vegetation Indices 16-day L3 Global 1km Sin Grid V006. doi:10.5067/MODIS/MOD13A2.006
Ding, Y., Xu, J., Wang, X., Cai, H., Zhou, Z., Sun, Y., et al. (2021). Propagation of Meteorological to Hydrological Drought for Different Climate Regions in china. J. Environ. Manage. 283, 111980. doi:10.1016/j.jenvman.2021.111980
Dinku, T., Funk, C., Peterson, P., Maidment, R., Tadesse, T., Gadain, H., et al. (2018). Validation of the Chirps Satellite Rainfall Estimates over Eastern Africa. Q. J. R. Meteorol. Soc. 144, 292–312. doi:10.1002/qj.3244
Farahmand, A., and AghaKouchak, A. (2015). A Generalized Framework for Deriving Nonparametric Standardized Drought Indicators. Adv. Water Resour. 76, 140–145. doi:10.1016/j.advwatres.2014.11.012
Fuentes, I., van Ogtrop, F., and Vervoort, R. W. (2020). Long-term Surface Water Trends and Relationship with Open Water Evaporation Losses in the Namoi Catchment, australia. J. Hydrol. 584, 124714. doi:10.1016/j.jhydrol.2020.124714
Funk, C., Peterson, P., Landsfeld, M., Pedreros, D., Verdin, J., Shukla, S., et al. (2015). The Climate Hazards Infrared Precipitation with Stations-Aa New Environmental Record for Monitoring Extremes. Sci. Data 2 (1), 1–21. doi:10.1038/sdata.2015.66
Garreaud, R. D., Boisier, J. P., Rondanelli, R., Montecinos, A., Sepúlveda, H. H., and Veloso‐Aguila, D. (2020). The central chile Mega Drought (2010-2018): A Climate Dynamics Perspective. Int. J. Climatol 40 (1), 421–439. doi:10.1002/joc.6219
Gill, R. B., Mayewski, P. A., Nyberg, J., Haug, G. H., and Peterson, L. C. (2007). Drought and the Maya Collapse. Ancient Mesoam 18 (2), 283–302. doi:10.1017/s0956536107000193
Gorelick, N., Hancher, M., Dixon, M., Ilyushchenko, S., Thau, D., and Moore, R. (2017). Google Earth Engine: Planetary-Scale Geospatial Analysis for Everyone. Remote sensing Environ. 202, 18–27. doi:10.1016/j.rse.2017.06.031
Grose, M., Timbal, B., Wilson, L., Bathols, J., and Kent, D. (2015). The Subtropical ridge in Cmip5 Models, and Implications for Projections of Rainfall in Southeast australia. Amoj 65 (1), 90–106. doi:10.22499/2.6501.007
Guenang, G. M., and Kamga, F. M. (2014). Computation of the standardized precipitation index (spi) and its use to assess drought occurrences in cameroon over recent decades. Journal of Applied Meteorology and Climatology 53 (10), 2310–2324. doi:10.1175/jamc-d-14-0032.1
Halwatura, D., Lechner, A. M., and Arnold, S. (2015). Drought Severity-Duration-Frequency Curves: a Foundation for Risk Assessment and Planning Tool for Ecosystem Establishment in post-mining Landscapes. Hydrol. Earth Syst. Sci. 19 (2), 1069–1091. doi:10.5194/hess-19-1069-2015
Han, Z., Huang, S., Huang, Q., Leng, G., Wang, H., Bai, Q., et al. (2019). Propagation Dynamics from Meteorological to Groundwater Drought and Their Possible Influence Factors. J. Hydrol. 578, 124102. doi:10.1016/j.jhydrol.2019.124102
Hayes, M., Svoboda, M., Wall, N., and Widhalm, M. (2011). The lincoln Declaration on Drought Indices: Universal Meteorological Drought index Recommended. Bull. Am. Meteorol. Soc. 92 (4), 485–488. doi:10.1175/2010bams3103.1
He, C., and Li, T. (2019). Does Global Warming Amplify Interannual Climate Variability? Clim. Dyn. 52 (5), 2667–2684. doi:10.1007/s00382-018-4286-0
Hoffmann, L., Günther, G., Li, D., Stein, O., Wu, X., Griessbach, S., et al. (2019). From ERA-Interim to ERA5: the Considerable Impact of ECMWF's Next-Generation Reanalysis on Lagrangian Transport Simulations. Atmos. Chem. Phys. 19 (5), 3097–3124. doi:10.5194/acp-19-3097-2019
Hurrell, J. W., Kushnir, Y., Ottersen, G., and Visbeck, M. (2003). An Overview of the North Atlantic Oscillation. Geophys. Monograph-American Geophys. Union 134, 1–35. doi:10.1029/134gm01
Jain, V. K., Pandey, R. P., Jain, M. K., and Byun, H.-R. (2015). Comparison of Drought Indices for Appraisal of Drought Characteristics in the Ken River basin. Weather Clim. Extremes 8, 1–11. doi:10.1016/j.wace.2015.05.002
Jehanzaib, M., and Kim, T.-W. (2020). Exploring the Influence of Climate Change-Induced Drought Propagation on Wetlands. Ecol. Eng. 149, 105799. doi:10.1016/j.ecoleng.2020.105799
João, E. (2002). How Scale Affects Environmental Impact Assessment. Environ. impact Assess. Rev. 22 (4), 289–310. doi:10.1016/s0195-9255(02)00016-1
Karnieli, A., Agam, N., Pinker, R. T., Anderson, M., Imhoff, M. L., Gutman, G. G., et al. (2010). Use of Ndvi and Land Surface Temperature for Drought Assessment: Merits and Limitations. J. Clim. 23 (3), 618–633. doi:10.1175/2009jcli2900.1
Katsanos, D., Retalis, A., and Michaelides, S. (2016). Validation of a High-Resolution Precipitation Database (Chirps) over cyprus for a 30-year Period. Atmos. Res. 169, 459–464. doi:10.1016/j.atmosres.2015.05.015
Kerr, R. A. (1998). Sea-floor Dust Shows Drought Felled Akkadian empire. Science 279 (5349), 325–326. doi:10.1126/science.279.5349.325
Kuss, A. J. M., and Gurdak, J. J. (2014). Groundwater Level Response in U.S. Principal Aquifers to ENSO, NAO, PDO, and AMO. J. Hydrol. 519, 1939–1952. doi:10.1016/j.jhydrol.2014.09.069
Lehner, B., and Grill, G. (2013). Global River Hydrography and Network Routing: Baseline Data and New Approaches to Study the World's Large River Systems. Hydrol. Process. 27 (15), 2171–2186. doi:10.1002/hyp.9740
Lehner, B., Liermann, C. R., Revenga, C., Vörösmarty, C., Fekete, B., Crouzet, P., et al. (2011). High‐resolution Mapping of the World's Reservoirs and Dams for Sustainable River‐flow Management. Front. Ecol. Environ. 9 (9), 494–502. doi:10.1890/100125
Li, B., Rodell, M., Kumar, S., Beaudoing, H. K., Getirana, A., Zaitchik, B. F., et al. (2019). Global grace Data Assimilation for Groundwater and Drought Monitoring: Advances and Challenges. Water Resour. Res. 55 (9), 7564–7586. doi:10.1029/2018wr024618
Li, L., She, D., Zheng, H., Lin, P., and Yang, Z.-L. (2020). Elucidating Diverse Drought Characteristics from Two Meteorological Drought Indices (Spi and Spei) in china. J. Hydrometeorology 21 (7), 1513–1530. doi:10.1175/jhm-d-19-0290.1
Lorenzo-Lacruz, J., Garcia, C., and Morán-Tejeda, E. (2017). Groundwater Level Responses to Precipitation Variability in Mediterranean Insular Aquifers. J. Hydrol. 552, 516–531. doi:10.1016/j.jhydrol.2017.07.011
Lorenzo-Lacruz, J., Vicente-Serrano, S., González-Hidalgo, J., López-Moreno, J., and Cortesi, N. (2013). Hydrological Drought Response to Meteorological Drought in the Iberian peninsula. Clim. Res. 58 (2), 117–131. doi:10.3354/cr01177
Lorenzo-Lacruz, J., Vicente-Serrano, S. M., López-Moreno, J. I., Beguería, S., García-Ruiz, J. M., and Cuadrat, J. M. (2010). The Impact of Droughts and Water Management on Various Hydrological Systems in the Headwaters of the Tagus River (central spain). J. Hydrol. 386 (1-4), 13–26. doi:10.1016/j.jhydrol.2010.01.001
Maity, R., Ramadas, M., and Govindaraju, R. S. (2013). Identification of Hydrologic Drought Triggers from Hydroclimatic Predictor Variables. Water Resour. Res. 49 (7), 4476–4492. doi:10.1002/wrcr.20346
Mantua, N. J., and Hare, S. R. (2002). The pacific Decadal Oscillation. J. oceanography 58 (1), 35–44. doi:10.1023/a:1015820616384
Mao, D., Wang, Z., Luo, L., and Ren, C. (2012). Integrating Avhrr and Modis Data to Monitor Ndvi Changes and Their Relationships with Climatic Parameters in Northeast china. Int. J. Appl. Earth Observation Geoinformation 18, 528–536. doi:10.1016/j.jag.2011.10.007
McKee, T. B., Doesken, N. J., and Kleist, J. (1993). “The Relationship of Drought Frequency and Duration to Time Scales,” in Proceedings of the 8th Conference on Applied Climatology (Boston, 179–183.17
McKee, T. B. (1995). Drought Monitoring with Multiple Time Scales. Proceedings of 9th Conference on Applied Climatology. Boston.
McNally, A., Arsenault, K., Kumar, S., Shukla, S., Peterson, P., Wang, S., et al. (2017). A Land Data Assimilation System for Sub-saharan Africa Food and Water Security Applications. Sci. Data 4 (1), 170012–170019. doi:10.1038/sdata.2017.12
Miller, G. R., Chen, X., Rubin, Y., Ma, S., and Baldocchi, D. D. (2010). Groundwater Uptake by Woody Vegetation in a Semiarid Oak savanna. Water Resour. Res. 46 (10). doi:10.1029/2009wr008902
Nalbantis, I., and Tsakiris, G. (2009). Assessment of Hydrological Drought Revisited. Water Resour. Manage. 23 (5), 881–897. doi:10.1007/s11269-008-9305-1
Olauson, J. (2018). Era5: The New champion of Wind Power Modelling? Renew. Energ. 126, 322–331. doi:10.1016/j.renene.2018.03.056
Paredes-Trejo, F. J., Barbosa, H. A., and Lakshmi Kumar, T. V. (2017). Validating Chirps-Based Satellite Precipitation Estimates in Northeast brazil. J. arid environments 139, 26–40. doi:10.1016/j.jaridenv.2016.12.009
Peña-Gallardo, M., Vicente-Serrano, S. M., Hannaford, J., Lorenzo-Lacruz, J., Svoboda, M., Domínguez-Castro, F., et al. (2019). Complex Influences of Meteorological Drought Time-Scales on Hydrological Droughts in Natural Basins of the Contiguous Unites States. J. Hydrol. 568, 611–625. doi:10.1016/j.jhydrol.2018.11.026
Pereira, L. S., Allen, R. G., Smith, M., and Raes, D. (2015). Crop Evapotranspiration Estimation with Fao56: Past and Future. Agric. Water Management 147, 4–20. doi:10.1016/j.agwat.2014.07.031
Peters, A. J., Walter-Shea, E. A., Ji, L., Vina, A., Hayes, M., and Svoboda, M. D. (2002). Drought Monitoring with Ndvi-Based Standardized Vegetation index. Photogrammetric Eng. remote sensing 68 (1), 71–75.
Pinzon, J., and Tucker, C. (2014). A Non-stationary 1981-2012 Avhrr Ndvi3g Time Series. Remote sensing 6 (8), 6929–6960. doi:10.3390/rs6086929
Potopová, V., Štěpánek, P., Možný, M., Türkott, L., and Soukup, J. (2015). Performance of the Standardised Precipitation Evapotranspiration index at Various Lags for Agricultural Drought Risk Assessment in the Czech republic. Agric. For. Meteorology 202, 26–38. doi:10.1016/j.agrformet.2014.11.022
Rangecroft, S., Van Loon, A. F., Maureira, H., Verbist, K., and Hannah, D. M. (2019). An Observation-Based Method to Quantify the Human Influence on Hydrological Drought: Upstream-Downstream Comparison. Hydrological Sci. J. 64 (3), 276–287. doi:10.1080/02626667.2019.1581365
Rubel, F., and Kottek, M. (2010). Observed and Projected Climate Shifts 1901-2100 Depicted by World Maps of the Köppen-Geiger Climate Classification. metz 19 (2), 135–141. doi:10.1127/0941-2948/2010/0430
Sattar, M. N., Lee, J.-Y., Shin, J.-Y., and Kim, T.-W. (2019). Probabilistic Characteristics of Drought Propagation from Meteorological to Hydrological Drought in south korea. Water Resour. Manage. 33 (7), 2439–2452. doi:10.1007/s11269-019-02278-9
Schreiner-McGraw, A. P., and Ajami, H. (2021). Delayed Response of Groundwater to Multi-Year Meteorological Droughts in the Absence of Anthropogenic Management. J. Hydrol. 603, 126917. doi:10.1016/j.jhydrol.2021.126917
Schubert, S. D., Stewart, R. E., Wang, H., Barlow, M., Berbery, E. H., Cai, W., et al. (2016). Global Meteorological Drought: A Synthesis of Current Understanding with a Focus on Sst Drivers of Precipitation Deficits. J. Clim. 29 (11), 3989–4019. doi:10.1175/jcli-d-15-0452.1
Shukla, S., and Wood, A. W. (2008). Use of a Standardized Runoff index for Characterizing Hydrologic Drought. Geophys. Res. Lett. 35 (2). doi:10.1029/2007gl032487
Shumway, R. H., and Stoffer, D. S. (2017). Time Series Analysis and its Applications with R Examples. Fourth Edition. Springer.
Siebert, S., Burke, J., Faures, J. M., Frenken, K., Hoogeveen, J., Döll, P., et al. (2010). Groundwater Use for Irrigation - a Global Inventory. Hydrol. Earth Syst. Sci. 14 (10), 1863–1880. doi:10.5194/hess-14-1863-2010
Smith, T. G. (2017), ‘Pmdarima: Arima Estimators for Python’. Availableat: http://www.alkaline-ml.com/pmdarima
Sohrabi, M. M., Ryu, J. H., Abatzoglou, J., and Tracy, J. (2015). Development of Soil Moisture Drought index to Characterize Droughts. J. Hydrol. Eng. 20 (11), 04015025. doi:10.1061/(asce)he.1943-5584.0001213
Son, N. T., Chen, C. F., Chen, C. R., Chang, L. Y., and Minh, V. Q. (2012). Monitoring Agricultural Drought in the Lower Mekong basin Using Modis Ndvi and Land Surface Temperature Data. Int. J. Appl. Earth Observation Geoinformation 18, 417–427. doi:10.1016/j.jag.2012.03.014
Stagge, J. H., Tallaksen, L. M., Gudmundsson, L., Van Loon, A. F., and Stahl, K. (2015). Candidate Distributions for Climatological Drought Indices ( SPI and SPEI ). Int. J. Climatol. 35 (13), 4027–4040. doi:10.1002/joc.4267
Stahl, K., Vidal, J.-P., Hannaford, J., Tijdeman, E., Laaha, G., Gauster, T., et al. (2020). The Challenges of Hydrological Drought Definition, Quantification and Communication: an Interdisciplinary Perspective. Proc. IAHS 383, 291–295. doi:10.5194/piahs-383-291-2020
Sternberg, T. (2012). Chinese Drought, Bread and the arab spring. Appl. Geogr. 34, 519–524. doi:10.1016/j.apgeog.2012.02.004
Tarek, M., Brissette, F. P., and Arsenault, R. (2020). Evaluation of the Era5 Reanalysis as a Potential Reference Dataset for Hydrological Modelling over north america. Hydrol. Earth Syst. Sci. 24 (5), 2527–2544. doi:10.5194/hess-24-2527-2020
Tirivarombo, S., Osupile, D., and Eliasson, P. (2018). Drought Monitoring and Analysis: Standardised Precipitation Evapotranspiration index (Spei) and Standardised Precipitation index (Spi). Phys. Chem. Earth, Parts A/B/C 106, 1–10. doi:10.1016/j.pce.2018.07.001
Trnka, M., Hayes, M., Jurečka, F., Bartošová, L., Anderson, M., Brázdil, R., et al. (2018). Priority Questions in Multidisciplinary Drought Research. Clim. Res. 75 (3), 241–260. doi:10.3354/cr01509
Van Dijk, A. I. J. M., Beck, H. E., Crosbie, R. S., de Jeu, R. A. M., Liu, Y. Y., Podger, G. M., et al. (2013). The Millennium Drought in Southeast australia (2001-2009): Natural and Human Causes and Implications for Water Resources, Ecosystems, Economy, and Society. Water Resour. Res. 49 (2), 1040–1057. doi:10.1002/wrcr.20123
Van Loon, A. F., Kumar, R., and Mishra, V. (2017). Testing the Use of Standardised Indices and grace Satellite Data to Estimate the European 2015 Groundwater Drought in Near-Real Time. Hydrol. Earth Syst. Sci. 21 (4), 1947–1971. doi:10.5194/hess-21-1947-2017
Van Loon, A. F., Rangecroft, S., Coxon, G., Breña Naranjo, J. A., Van Ogtrop, F., and Van Lanen, H. A. J. (2019). Using Paired Catchments to Quantify the Human Influence on Hydrological Droughts. Hydrol. Earth Syst. Sci. 23 (3), 1725–1739. doi:10.5194/hess-23-1725-2019
Van Loon, A. F., Van Huijgevoort, M. H. J., and Van Lanen, H. A. J. (2012). Evaluation of Drought Propagation in an Ensemble Mean of Large-Scale Hydrological Models. Hydrol. Earth Syst. Sci. 16 (11), 4057–4078. doi:10.5194/hess-16-4057-2012
Van Loon, A. F., and Van Lanen, H. A. J. (2013). Making the Distinction between Water Scarcity and Drought Using an Observation-Modeling Framework. Water Resour. Res. 49 (3), 1483–1502. doi:10.1002/wrcr.20147
Vicente-Serrano, S. M., and Beguería, S. (2016). Comment on 'Candidate Distributions for Climatological Drought Indices (SPI and SPEI)' by James H. Staggeet Al. Int. J. Climatol. 36 (4), 2120–2131. doi:10.1002/joc.4474
Vicente-Serrano, S. M., Beguería, S., and López-Moreno, J. I. (2010). A Multiscalar Drought index Sensitive to Global Warming: the Standardized Precipitation Evapotranspiration index. J. Clim. 23 (7), 1696–1718. doi:10.1175/2009jcli2909.1
Vicente-Serrano, S. M., Gouveia, C., Camarero, J. J., Beguería, S., Trigo, R., López-Moreno, J. I., et al. (2013). Response of Vegetation to Drought Time-Scales across Global Land Biomes. Proc. Natl. Acad. Sci. 110 (1), 52–57. doi:10.1073/pnas.1207068110
Vicente-Serrano, S. M., López-Moreno, J. I., Beguería, S., Lorenzo-Lacruz, J., Azorin-Molina, C., and Morán-Tejeda, E. (2012). Accurate Computation of a Streamflow Drought index. J. Hydrol. Eng. 17 (2), 318–332. doi:10.1061/(asce)he.1943-5584.0000433
Vicente-Serrano, S. M., López-Moreno, J. I., Gimeno, L., Nieto, R., Morán-Tejeda, E., Lorenzo-Lacruz, J., et al. (2011). A Multiscalar Global Evaluation of the Impact of Enso on Droughts. J. Geophys. Res. Atmospheres 116 (D20). doi:10.1029/2011jd016039
Wanders, N., Loon, A. F. V., and Van Lanen, H. A. (2017). ‘Frequently Used Drought Indices Reflect Different Drought Conditions on Global Scale’, Hydrology And Earth System Sciences Discussions, 1–16. doi:10.5194/hess-2017-512
Wang, Q., Wu, J., Lei, T., He, B., Wu, Z., Liu, M., et al. (2014). Temporal-spatial Characteristics of Severe Drought Events and Their Impact on Agriculture on a Global Scale. Quat. Int. 349, 10–21. doi:10.1016/j.quaint.2014.06.021
Wang, W., Ertsen, M. W., Svoboda, M. D., and Hafeez, M. (2016). ‘Propagation of Drought: from Meteorological Drought to Agricultural and Hydrological Drought’. Hindawi 2016, 6547209.
Wendt, D. E., Van Loon, A. F., Scanlon, B. R., and Hannah, D. M. (2021). Managed Aquifer Recharge as a Drought Mitigation Strategy in Heavily-Stressed Aquifers. Environ. Res. Lett. 16 (1), 014046. doi:10.1088/1748-9326/abcfe1
Wilhite, D. A. (2016). “Drought-management Policies and Preparedness Plans: Changing the Paradigm from Crisis to Risk Management,” in Land Restoration (Elsevier), 443–462. doi:10.1016/b978-0-12-801231-4.00007-0
Wu, J., Miao, C., Tang, X., Duan, Q., and He, X. (2018). A Nonparametric Standardized Runoff index for Characterizing Hydrological Drought on the Loess Plateau, china. Glob. Planet. Change 161, 53–65. doi:10.1016/j.gloplacha.2017.12.006
Xiao, M., Zhang, Q., and Singh, V. P. (2015). Influences of Enso, Nao, Iod and Pdo on Seasonal Precipitation Regimes in the Yangtze River basin, china. Int. J. Climatol 35 (12), 3556–3567. doi:10.1002/joc.4228
Xu, Y., Zhang, X., Wang, X., Hao, Z., Singh, V. P., and Hao, F. (2019). Propagation from Meteorological Drought to Hydrological Drought under the Impact of Human Activities: A Case Study in Northern china. J. Hydrol. 579, 124147. doi:10.1016/j.jhydrol.2019.124147
Yuan, X., Zhang, M., Wang, L., and Zhou, T. (2017). Understanding and Seasonal Forecasting of Hydrological Drought in the Anthropocene. Hydrol. Earth Syst. Sci. 21 (11), 5477–5492. doi:10.5194/hess-21-5477-2017
Zargar, A., Sadiq, R., Naser, B., and Khan, F. I. (2011). A Review of Drought Indices. Environ. Rev. 19, 333–349. doi:10.1139/a11-013
Zhang, H., Xu, Y., and Kanyerere, T. (2020). A Review of the Managed Aquifer Recharge: Historical Development, Current Situation and Perspectives. Phys. Chem. Earth, Parts A/B/CParts A/B/C 118-119, 102887. doi:10.1016/j.pce.2020.102887
Zhang, Y., and Li, Z. (2020). Uncertainty Analysis of Standardized Precipitation Index Due to the Effects of Probability Distributions and Parameter Errors. Front. Earth Sci. 8, 76. doi:10.3389/feart.2020.00076
Zhao, G., and Gao, H. (2019). Estimating Reservoir Evaporation Losses for the united states: Fusing Remote Sensing and Modeling Approaches. Remote Sensing Environ. 226, 109–124. doi:10.1016/j.rse.2019.03.015
Zhou, J., Li, Q., Wang, L., Lei, L., Huang, M., Xiang, J., et al. (2019). Impact of Climate Change and Land-Use on the Propagation from Meteorological Drought to Hydrological Drought in the Eastern Qilian Mountains. Water 11 (8), 1602. doi:10.3390/w11081602
Keywords: drought propagation, meteorological drought, agricultural drought, hydrologic drought, trend analysis
Citation: Fuentes I, Padarian J and Vervoort RW (2022) Spatial and Temporal Global Patterns of Drought Propagation. Front. Environ. Sci. 10:788248. doi: 10.3389/fenvs.2022.788248
Received: 01 October 2021; Accepted: 07 February 2022;
Published: 01 March 2022.
Edited by:
Sergio M. Vicente-Serrano, Spanish National Research Council (CSIC), SpainReviewed by:
Zengyun Hu, Chinese Academy of Sciences (CAS), ChinaJorge Lorenzo Lacruz, University of La Rioja, Spain
Copyright © 2022 Fuentes, Padarian and Vervoort. This is an open-access article distributed under the terms of the Creative Commons Attribution License (CC BY). The use, distribution or reproduction in other forums is permitted, provided the original author(s) and the copyright owner(s) are credited and that the original publication in this journal is cited, in accordance with accepted academic practice. No use, distribution or reproduction is permitted which does not comply with these terms.
*Correspondence: Ignacio Fuentes, aWduYWNpby5mdWVudGVzQHN5ZG5leS5lZHUuYXU=