- Institute of Finance and Economics, Shanghai University of Finance and Economics, Shanghai, China
As a convenient means of transportation, high-speed rail (HSR) plays an important role in green development. In the context of the rapid development of China’s HSR, this study selects the SBM-DDF-SML model to construct the green total factor productivity (GTFP) index to measure urban green development; moreover, it empirically tests the impact of the opening of the HSR on GTFP using the spatial difference-in-differences (SDID) model. The results show that the opening of the HSR could significantly promote GTFP for HSR-served cities. In addition, the opening of HSR has a positive effect on the GTFP for neighboring HSR-served cities but a negative impact on that for neighboring non-HSR-served cities. The mechanism test shows that HSR can influence GTFP by promoting urban green innovation and entrepreneurial vitality. This study is a supplement to the research on the impact of HSR on the GTFP, in order to provide corresponding policy advice. The government should optimize the layout of HSR and help cities achieve green and sustainable development.
1 Introduction
Since the Industrial Revolution, accompanied by the prosperity of the world economy, environmental pollution has become increasingly severe (Chen and You, 2021). Environmental pollution has seriously impaired human physical and mental health (Chen et al., 2013; Calderón-Garcidueñas et al., 2014). In particular, the accumulation of greenhouse gases, which leads to the frequent occurrence of all kinds of weather and climate events, has brought severe threats to human society (Han et al., 2020). Governments and environmental protection organizations around the world have made great efforts to deal with the issues of environmental pollution and climate change (Rehman et al., 2022). However, the problem of environmental degradation has not been effectively curbed. According to a report on global climate change released by the World Meteorological Organization in 2020, in the first half of the 21st century, global greenhouse gases reached the highest level they had attained in three million years (Guo et al., 2021). In coping with environmental pollution, green growth has increasingly become one of the most favored development models in modern society.
China is the largest developing country, and its economic development and environmental pollution issues have a high profile around the world (Zhang et al., 2020). After the reform and opening up, China quickly became the second-largest economy, growing at a three times higher rate than the global average annual growth rate. However, it has also paid a heavy environmental price for economic growth (Xia and Xu, 2020), in terms of air pollution (Zeng et al., 2019), soil pollution, and deteriorating water quality (He et al., 2020). The environmental pollution issues in China were already quite severe (Yang et al., 2021), restricting the country’s economic development. Over time, the contradiction between China’s economic development and environmental protection has become increasingly prominent (Li T. et al., 2020). Transforming the mode of economic development to improve the quality of economic growth has become an important means of resolving the contradiction between economic development and environmental protection (Yang et al., 2021).
Green development is a development model that essentially involves balancing economic development and environmental protection. In this sense, GTFP is a reasonable quantification of green development (Liu G. et al., 2020). Hence, improving GTFP has become an important means of coordinating the relationship between the environment and economy (Zhao et al., 2020) and an inevitable choice for achieving economic quality improvement (Gao et al., 2021). The impact factors of GTFP have been extensively studied, including environmental regulations (Zhao et al., 2020), industrial structures (Peng et al., 2020), factor market misallocations (Zhang et al., 2019), and development of the internet (Li T. et al., 2020). In addition, public infrastructure is regularly treated as the key to promoting economic efficiency by reducing production costs and transaction costs (Chen and Vickerman, 2016; Diao, 2018). The role of rail transit in reducing air pollution (Zheng S. et al., 2019; Guo and Chen, 2019) and improving environmental quality (Fan et al., 2020; Guo et al., 2020) has also been widely confirmed. This raised the question of what impact HSR, which integrates clean, speed, convenience, and safety (Guo et al., 2020), has on the GTFP? However, despite the importance of the topic, few scholars have discussed this issue in detail at present. Thus, this study is a supplement to the research on the impact of traffic infrastructure on the GTFP.
After the opening of the world’s first HSR, Japan’s Shinkansen Line in 1964, Western countries such as Germany, France, and Italy successively started a wave of HSR construction (Guo et al., 2020). Since China’s first HSR, the Beijing–Tianjin Intercity Railway, opened in 2008, the construction of HSR in China has been increasing at an alarming rate. In addition, the “8 + 8” corridor HSR framework has gradually come into being in China (Yu et al., 2019). By 2020, the operating mileage of HSR in China reached 35,000 km, accounting for more than half of the global HSR operating mileage and ranking first in the world (Huang and Zong, 2021). Now, more and more travelers take HSR as their preferred mode for traveling (Guo et al., 2020). Meanwhile, as a novel transport tool with the characteristics of safety, comfort, and efficiency (Cheng, 2010), HSR shortens the travel time between cities (Jiao et al., 2017) and changes individual travel patterns (Li et al., 2016). Moreover, the role of HSR has become increasingly prominent in promoting economic restructuring (Chen, 2012) and optimizing resource allocation (Zhu et al., 2020). Hence, the positive impact of HSR on socioeconomic booms has been widely accepted by scholars (Ke et al., 2017). In addition, the literature also talks about the positive relationship between HSR and pollution reduction effects (Dalkic et al., 2017), although there is another view to the effect that HSR has a negative impact on the ecological environment (Kaewunruen et al., 2019). Moreover, there is spatial unevenness in the improvement of regional accessibility by the opening of HSR, which leads to uncertainty about the positive economic effect of HSR. On occasions, some scholars have found that HSR will only promote economic growth in HSR-served cities, causing economic recession in non–HSR-served cities (Martin, 1997). Therefore, the impacts of HSR on the economy and environment are still much debated (Chen, 2012; Chen et al., 2016). This has caused us to rethink the relationship between HSR and socioeconomic development and ask the following question: can the opening of HSR improve GTFP?
In China, HSR projects are mainly planned by the state based on economic benefits and security issues. However, the economic benefits brought by HSR have led cities to confront in competition for HSR stops. In 2009, in Shaoyang, Hunan Province, 10,000 citizens took part in a campaign lobbying for an HSR to pass through their city. Following this, a fierce competition for HSR stations between neighboring cities nationwide, such as Shiyan and Xiangyang, Jingmen and Jingzhou, often arose. What caused the fierce HSR competition? How can this be explained from an economic perspective? Is it just because HSR can boost local economic growth? So far, few scholars have conducted an in-depth analysis of this problem. However, this research will give a more reasonable explanation for the fierce competition between neighboring cities.
Existing studies on HSR projects mainly assume the opening of HSR stations as a quasi-natural experiment, using a difference-in-difference (DID) model to evaluate the impact of this project on local socioeconomic development (Guo et al., 2020; Zhu et al., 2020). However, the DID model cannot effectively assess the socioeconomic impact of HSR on neighboring areas, in particular, on those neighboring areas without HSR stations. Thus, this study adopts a spatial difference-in-difference (SDID) model to identify the impact of HSR on neighboring HSR-served and non–HSR-served cities. This method for evaluating the impact of HSR on GTFP is more complete than the traditional DID model (Chagas et al., 2016).
The main contributions of this study to the existing literature are as follows: First, from the perspective of research, we tested the impact of HSR on GTFP, which enriched the current research on HSR and GTFP projects. Second, in terms of research methods, we adopted the SDID model, which is a frontier approach in econometrics to evaluate the effects of HSR on both HSR-served and non–HSR-served cities. This method can not only measure the effects of HSR on treatment cities but also the spatial spillover benefits to neighboring non–HSR-served cities. This research is conducive to our comprehensive assessment of the social impact of HSR. Moreover, existing research focused on the economic and environmental impacts of HSR, but this study investigates whether the opening of HSR can promote both economic and sustainable development. It provides a systematic empirical analysis framework for future-related research. In addition, our research conclusions can better explain the realistic issues, such as why there is fierce competition for HSR stations between neighboring cities.
2 Literature Review and Hypotheses
2.1 Direct Effects of High-Speed Rail
In the context of green and sustainable development, scholars have included energy consumption and environmental capacity as new input factors in the production function in traditional total factor productivity (TFP) analysis (Feng and Serletis, 2014). Based on these studies, some scholars define input–output efficiency as GTFP, which incorporates energy consumption and pollutant emissions (Chen and Golley, 2014; Zhu et al., 2018). Since then, GTFP has been used to study the input–output efficiency of economic systems (Fare et al., 2007; Chen and Golley, 2014) and assess the sustainability of economic development (Liu G. et al., 2020; Xia and Xu, 2020; Yan et al., 2020). As a phenomenon, the growth of GTFP is considered to achieve both economic growth and ecological development of cities. Environmental degradation has become one of the most important issues because of the increase in CO2 emissions (Fareed et al., 2022). Ullah et al. (2022) examined the relationship between tourism, economic growth, and CO2 emissions in Brazil from 1995 to 2018; they suggested that sustainable and eco-friendly economic growth and tourism policies significantly preserve environmental quality. Rehman et al. (2021) also concluded that export diversification, renewable energy, and regulatory quality are negatively associated with GHG emissions. They proposed mechanisms and pathways to avoid environmental degradation. As mentioned in the previous section, scholars have conducted a series of discussions around the impact factors of GTFP; among these, the role of transportation infrastructure on GTFP has been extensively studied (Zhang et al., 2019; Li Y. et al., 2020; Peng et al., 2020). However, little literature has focused on the relationship between HSR, which represents an essential component of transportation infrastructure and the GTFP.
From an economic perspective, HSR is the “buster” of natural barriers and space-time distance in the new era; it can speed up the flow of labor factors and promote the economic growth of cities along the line (Banerjee et al., 2020). HSR’s social effects have been recorded in many studies, and they include economic growth (Li Y. et al., 2020; Jin et al., 2020), industrial agglomeration (Matas et al., 2020), and city accessibility (Xu et al., 2018). Compared with traditional railroad investment, HSR construction will bring higher economic input (Diao, 2018), and accordingly, higher economic output. Once a government chooses to build an HSR station in a city, the economic indicators of the area tend to show a growth trend (Sun et al., 2020). In addition, investment in HSR drives the development of related industries (Shao et al., 2017; De Rus, 2021). For instance, Bernard et al. (2019) scrutinized the significant impact of firm production chains and supplier selection for Shinkansen in Japan. The study results showed that HSR has a positive influence on firm productivity. Thus, HSR can promote regional economic growth by improving the economic efficiency of enterprises (Pontarollo and Ricciuti, 2020). Second, the continuous improvement of HSR will break the market segmentation between cities (Vickerman, 2018). HSR speeds up the flow of resources, such as technical factors, information factors, and time-sensitive senior talent factors (Wang et al., 2019). It also prompts the redistribution of resource factors that bring new vitality to different cities (Shao et al., 2017). Third, HSR is efficient and convenient, allowing it to compress the time cost between regions (Chen and Haynes, 2015). The construction of HSR enhances accessibility and communication between cities, and it creates good external conditions for urban economic development (Israel and Cohen-Blankshtain, 2010).
From an environmental perspective, the railway network is closely related to energy consumption and environmental pollution (Ibanez and McCalley, 2011). Some scholars have advocated that the government shift road investment to the construction of HSR (Song et al., 2016). The construction of HSR is a practical manifestation of China’s green and sustainable development policy in transportation construction (Song et al., 2016). The positive and negative externalities of HSR have complex effects on environmental efficiency (Sun et al., 2020). It is well known that HSR has high cleanliness and low energy consumption characteristics, which can directly reduce environmental pollution in actual operation (Chester and Horvath, 2012). Car emissions represent the main cause of air pollution (Li and Yu, 2019). HSR reduces traffic congestion and gaseous waste emissions, as well as environmental pollution, by replacing traditional transportation (Anderson, 2014). According to the study of Chen et al. (2016), HSR has a significant and positive effect on environmental degradation. In addition, Wang et al. (2018) found that HSR has a significant impact on enterprise energy saving and emission reduction, and it helps enterprises achieve clean production and reduce pollution emissions. Looking specifically at carbon reduction, Dalkic et al. (2017) noted that HSR consumes fewer fossil fuels for passenger and freight transport, helping reduce carbon emissions. Chen et al. (2016) confirmed the reducing role of HSR on CO2 emissions but pointed out that this effect would be offset by the scale effect of passenger traffic brought by HSR. Chang et al. (2019) used the Beijing–Shijiazhuang HSR line as a research object and found that HSR can significantly reduce greenhouse gas emissions. Compared with road transport and airlines, HSR carries the same number of passengers but consumes half the energy and produces half the CO2 emissions (Åkerman, 2011; Yang et al., 2021). As a result of transportation substitution and technological innovation, the increase in HSR service intensity has significantly reduced carbon dioxide emissions and curbed carbon dioxide emissions in neighboring cities (Jia et al., 2021).
Thus, based on the abovementioned analysis, this study puts forward the following hypothesis:
H1: HSR promotes GTFP for HSR-served cities.
2.2 Spatial Effects of High-Speed Rail
Improvements in transportation infrastructure can enhance the spillover effect between economies (Chen and Wei, 2018). As a transportation link between different regions, railways can effectively allocate natural resources, human resources, or commodities. Existing studies have focused on the spillover effects and siphon effects generated by the opening of HSR (Hernández and Luis Jiménez, 2014; Liu S. et al., 2020; Moyano and Dobruszkes, 2017; Zheng L. et al., 2019). Local governments tend to use the spatial spillover effects of HSR to promote development in urban areas (Zheng L. et al., 2019), while enhancing the land and property values of surrounding cities (Huang and Du, 2021). Thus, HSR can serve as an important channel for infrastructure financing and government revenue sources (Li Y. et al., 2020), achieving economic growth through spatial spillover effects (Huang and Xu, 2021). In the United Kingdom, HSR has had spillover effects on the surrounding areas because of knowledge diffusion, increased accessibility, and lower transaction costs (Ahlfeldt and Feddersen, 2018). It has been argued that the spatial spillover effects of HSR on neighboring cities are homogeneous and undifferentiated (Banister and Berechman, 2001). However, cities are complex network structures with increasingly weak geographic distance limits, so different spillover effects of HSR on surrounding cities may be found (Zheng L. et al., 2019).
After the opening of HSR, there is also a siphon effect between cities, and the gap between HSR-served cities and non–HSR-served cities is gradually increasing (Qin, 2016). Cascetta et al. (2020) studied Italy’s economic growth and social effects after 10 years of HSR service, finding that the HSR not only reduces resident travel time by 11% but also creates inequality between HSR-served and non–HSR-served cities. The same problem was found in a study based on data from Japan’s high-speed railroad (Li and Xu, 2018). Furthermore, while Lille (the largest city in northern France) is connected to Paris by HSR, the surrounding villages without HSR stations have suffered as a result (Ollivier et al., 2014). In China, HSR-served cities have higher economic benefits than non-HSR cities because of the significant increase in fixed-asset investment after the opening of HSR (Li X. et al., 2020). Moreover, HSR makes more commercial activities migrate to urban centers. This is extremely disadvantageous for non–HSR-served cities because it means population loss (Yang et al., 2020). In addition, HSR-served cities can provide more jobs than non-HSR cities can (Cheng et al., 2015). To make matters worse, the opening of HSRs leads to lower gross domestic product (GDP) growth in non–HSR-served cities, resulting in inequality between them (Faber, 2014; Meng et al., 2018).
According to the abovementioned analysis, we put forward the following hypotheses:
H2a: The opening of HSR can promote GTFP in neighboring HSR-served cities.
H2b: The opening of HSR has a negative effect on GTFP for neighboring non–HSR-served cities.
2.3 The Mechanism of High-Speed Rail on Green Total Factor Productivity
The improvement of GTFP cannot be achieved without technological progress (James et al., 2013). Technological progress is key to GTFP enhancement, especially in terms of green technology innovation (Aldieri et al., 2021). Green innovation is not just about reducing environmental burdens but also involves the aim of generating significant environmental benefits (Schiederig et al., 2012; Driessen et al., 2013). Innovation activity can significantly moderate the relationship between financial inclusion and environmental degradation (Fareed et al., 2022). Moreover, as Kassouri (2022) indicated, the public budget for energy research, development, and demonstration (RD & D) can effectively promote green technology innovation through the fiscal decentralization of government. Existing research concludes that green innovation makes growth sustainable (Rennings, 2000), which has a positive impact on productivity in the medium and long term (Aldieri et al., 2021). It is also a key catalyst for sustainable development (Porter and Van Der Linde, 1995).
Innovative talent and academic exchanges are the basic conditions for green innovation in cities (Dong et al., 2020). HSR can promote population clustering (Debrezion et al., 2010) and reduce commuting costs. High-level innovative talents always prefer faster HSR as a travel mode for cross-regional exchanges to save time. Dong et al. (2020) also found that HSR had a positive effect on researcher communication, showing that HSR significantly facilitated academic communication activities. In addition, the opening of HSR has facilitated factor mobility and stimulated the innovation output of firms and society. Faced with fierce competition, firms carry out green innovation activities to improve their core competitiveness (Oum and Fu, 2008). These activities can reduce pollution prevention costs (Leonidou et al., 2015) and receive financial subsidies from the government in terms of green innovation (Aldieri et al., 2021). Moreover, HSR reduces trade and search costs, increasing real profits of firms and encouraging them to invest more in research and development (R & D) for green innovation (Bernard et al., 2019; Kuang et al., 2021).
Based on the abovementioned analysis, this study proposes the following hypothesis:
H3: HSR promotes GTFP by increasing green innovation.
Entrepreneurship is a social activity in which entrepreneurs obtain higher economic value by integrating their various resources and using an effective combination of their resources and social resources. Entrepreneurial vitality is a measure of entrepreneurial activity. Previous literature has pointed out that improvements in transportation infrastructure have a positive impact on entrepreneurial activity (Fritsch and Storey, 2014; Berger and Kuckertz, 2016). Similarly, transportation infrastructure is crucial for maintaining business relationships, information exchange, and the establishment of new business opportunities (Ma et al., 2021). Thus, railroads and highways have a significant impact on regional entrepreneurial vitality (Audretsch et al., 2014). As the most popular mode of travel nowadays, HSR helps stimulate entrepreneurial vitality in cities and drive regional entrepreneurial enthusiasm (Ma et al., 2021). First, the opening of HSR can accelerate the urbanization process and improve the internal and external communication of cities (Petersen and Rajan, 2002). In this way, it can attract the inflow of entrepreneurs and provide strong support for regional entrepreneurial activities and the entrepreneurial behavior of talents. Second, HSR can promote the clustering and allocation of factors, alleviate entrepreneur information asymmetry, and create a good resource network and social environment for entrepreneurs. With the help of smooth, high-speed, and efficient communication channels, entrepreneurs can improve the operational efficiency and transaction costs of their businesses and the success rate of their ventures (Shao et al., 2017). Third, the opening of HSR will bring spatial spillover of economic growth and knowledge spillover of human capital. From this foundation, it can stimulate forward-looking entrepreneurial thinking and promote individual entrepreneurial behavior.
Existing studies have explored the positive impacts of entrepreneurial vitality on the GTFP (Liu S., et al., 2020; Xia and Xu, 2020; Zhang et al., 2021). First, entrepreneurial activity can accelerate the R & D of new technological achievements, which may force green technological innovation and product development. Therefore, entrepreneurial vitality can promote the improvement of GTFP. Second, entrepreneurial activities can accelerate the generation of new enterprises and industries and promote market competition and resource integration (Noseleit, 2012). This results in the achievement of economic growth and effective resource conservation. Third, entrepreneurial activities can generate new employment opportunities, improve human capital levels, and provide important support for economic growth and environmental quality improvement (Glaeser et al., 2010). In addition, entrepreneurial activities can attract and drive entrepreneurial subjects to exert agglomeration effects, releasing the potential for high-quality economic development (Glaeser et al., 2010). On the one hand, the clustering of similar entrepreneurial enterprises can realize the intensive use of infrastructure, reduce operating costs through resource-sharing and knowledge spillover effects, and effectively reduce pollution pressure (Duan et al., 2020). On the other hand, the agglomeration of complementary entrepreneurial enterprises can realize complementary advantages and win–win collaboration and effectively improve the efficiency of regional economic development (Acs and Armington, 2004).
In summary, we put forward the following hypothesis:
H4: HSR promotes GTFP by increasing entrepreneurial vitality.
3 China’s High-Speed Rail Development
In January 2004, the National Development and Reform Commission (NDRC) approved the implementation of the Medium- and Long-Term Railway Plan (MLTRP). After that, China’s railway system has been developing rapidly. In August 2008, China’s first intercity railway with a maximum speed of 350 kph was opened for operation, marking China's entry into the era of high-speed rail. The 12th Five-Year Plan is a golden period for China’s high-speed rail development. During this period, the “four vertical and four horizontal” corridors were completed, connecting major urban agglomerations. In June 2016, NDRC revised the MLTRP again, expanding the network structure from the original “4 + 4” corridors to “8 + 8” corridors 1 (Figure 1A). The 2025 target is for the HSR network to reach 38,000 km. By that time, travel times will be within 4 h between the large- and medium-sized cities and within 2 h around the regional centers.
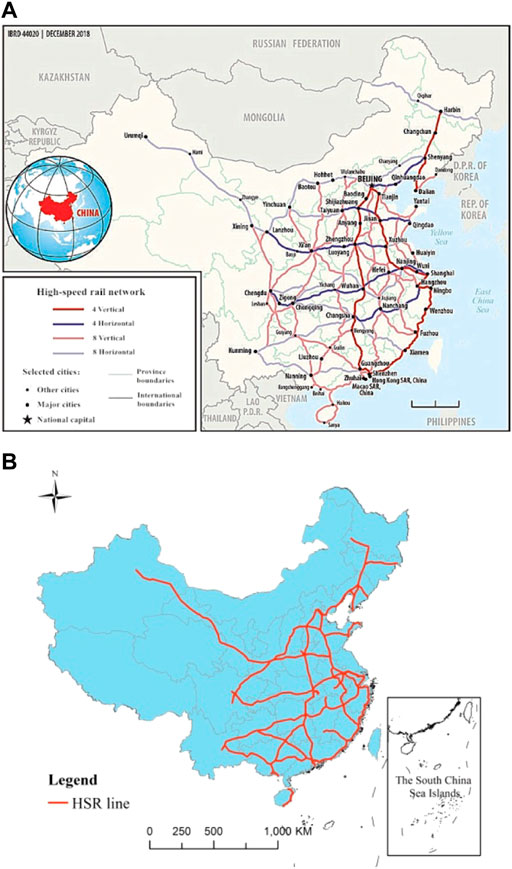
FIGURE 1. (A) Planned high-speed rail corridors. Source: from Growth of High-Speed Rail in China Published by World Bank Group. http://www.worldbank.org. (B) Completed HSR network in China, 2017. Source: Drawing by authors based on data from http://cn.gaotie.cn/.
Since 2008, HSR passenger volume has grown at 81 percent per year. In addition, the total number of HSR passenger traffic reached from 7.34 million in 2008 to 1.75 billion in 20172. By the end of 2017, 85 percent of prefecture-level cities in China have connected to HSR. However, there is spatial uneven distribution for China’s HSR. That is, the HSR lines and stations are densely distributed in eastern China and sparsely distributed in western China (Figure 1B).
4 Green Total Factor Productivity in China
4.1 Measure of Green Total Factor Productivity
Data Envelopment Analysis (DEA) first proposed by Farrell (1957) is one of the commonly used methods to measure the classic total factor productivity (TFP) with multi-input and single-output. However, in the classic measurement method, GDP is regarded as a single “good” output; conversely, “bad” outputs at the expense of the environment are not included in the measurement system. Therefore, GTFP is the production efficiency that achieves maximum economic output while minimizing pollutant emissions under the premise of given input factors. Drawing on the research of Fare et al. (2007), we assume a city as a decision-making unit (DMU) with N inputs denoted by
Then, we introduce the directional slacks-based measure (SBM) proposed by Fukuyama and Weber (2009):
where
On this basis, we use the Luenberger productivity indicator proposed by Chambers et al. (1996) as GTFP:
4.2 Variables
To measure GTFP, we need to select three important indicators: inputs, desirable outputs, and undesirable outputs. For inputs, in this study, we use labor force, capital, and energy, measured by the population of employment, total energy consumption, and capital stock calculated by the perpetual inventory method, respectively. For desirable output, we used real GDP at constant prices in 2000. Limited by statistical data, we adopt carbon dioxide emissions calculated by the fossil energy consumption of each city and industrial wastewater and waste solids as undesirable outputs. The data for all the aforementioned indicators are taken from the China City Statistical Yearbook (2007–2018).
4.3 Green Total Factor Productivity Calculation Results
According to models (1–3), we calculated the GTFP of 284 prefecture-level cities in China from 2006 to 2017. As Figure 2 shows, from 2006 to 2017, there was an overall growth trend in GTFP in HSR-served and non–HSR-served cities. Specifically, before the opening of China’s first HSR (in 2008), there was no significant difference in GTFP between the two groups. However, after 2008, there was a larger increase in GTFP in HSR-served cities than in non-HSR-served cities. Thus, the gap between the two groups is getting wider.
Figures 3A,B depicts the spatial distribution of GTFP for 284 cities in 2006 and 2017, respectively. Figure 3A shows in 2006 nearly half of the cities with GTFP less than 1, widely distributed in central China, while cities with GFTP greater than 1 are mainly concentrated in the Northeast regions, coastal regions, and the Western regions (e.g., Chongqing, Chengdu, and Guiyang, etc.). Yet, as Figure 3B shows, the number of cities with GTFP greater than 1 in 2017 has increased significantly from 2006. At the same time, GTFP also presents the following characteristics. 1) Spatial agglomeration. Cities with GTFP lower than 0.7 are mainly concentrated in northern China’s provinces such as Ningxia, while cities with GTFP greater than 1.6 are mainly concentrated in the Northeast China, Shandong Peninsula, Yangtze River Delta, and Pearl River Delta. 2) GTFP in HSR-served cities is greater than that in others. From 2006 to 2017, GTFP showed an overall upward trend. However, the gap between different cities is also increasing. Although GTFP presents a certain degree of geographical concentration, it also shows the characteristics that HSR-served cities are greater than other cities.
4.4 Spatial Attribute Analysis of Green Total Factor Productivity
Figures 3A,B illustrates that there is a spatial agglomeration for GTFP. Thus, there may be a spatial correlation in GTFP. Therefore, we adopt the global Moran’s Index to analyze the spatial correlation relationship from 2006 to 2017. The specific expression form is
where
Based on Formula 3, we test the spatial autocorrelation of GTFPs of 284 cities from 2006 to 2017. The results are shown in Table 1. The results show that all of Moran’s indexes are positive and pass the significance test of 1%, suggesting GTFP has significant spatial autocorrelation. In other words, the GTFP will be affected by green development in neighboring cities. To show the spatial autocorrelation of GTFP visually, we draw the Moran scatter plot. However, due to space limitations, we chose the beginning and ending years of the sample for presentation (Figure 4). From Figure 4, we can see that most of the values in the first and third quadrants further imply that GTFP has positive spatial autocorrelation.
5 Research Design
5.1 Method
Existing studies have taken the opening of HSR as a quasi-natural experiment and used the DID model to deal with the endogeneity problems between transportation infrastructure and economic factors (Ke et al., 2017; Guo et al., 2020). However, there is a spatial correlation for GTFP between treatments and the other units, and this leads to a violation of Stable Unit Treatment Value Assumption (SUTVA). Thus, the classical DID model will fail to obtain consistent estimators for HSR and GTFP. Therefore, to accurately assess the impact of HSR on GTFP, we follow Chagas et al. (2016) and construct an SDID model. Depending on the spatial term, the spatial econometric model can be set in various forms. The spatial Durbin model (SDM) is more general because it contains spatial lag terms for both dependent and independent variables. Thus, this study adopts the SDM model to construct the SDID model to evaluate the effects of HSR on GTFP:
where
5.2 Variable Specification
In this study, the dependent variable is GTFP, which we calculated in Section 4. The core-independent variable we focus on is
To avoid estimation errors caused by missing variables, we also control some variables that could affect the urban GTFP. Urban openness (
5.3 Data
We used prefecture cities as the research sample in this study. Two main sources of data were used for empirical analysis. HSR data were manually collected from the China Railway Customer Service Website (www.12306.cn), China Railway’s official operation service website. The other data were collected from China City Statistical Yearbook (2007–2018). It should be noted that to ensure the completeness of the sample information of the core variables and robustness of estimation results, cities with missing mass core variables were excluded. We finally selected 284 cities as the research sample. To test the parallel trend, we chose the first two years of the opening time of the first HSR as the starting year. At the same time, to balance the sample size between the experimental group and the control group, the time span of this study was 2006–2017.
6 Results
6.1 Model Test
Although the spatial autocorrelation analysis of GTFP in Section 4.4 shows that GTFP has a significant spatial correlation, this is not enough to justify the use of a spatial econometric model to measure the effects of HSR on GTFP is effective. Thus, following Elhorst et al. (2012) and Anselin et al. (1996), this study adopts Lagrange Multiplier (LM) to test whether our data fit the spatial econometric model (results in Table 3). The test results of LM in Table 3 show that the spatial econometric model used in this study is suitable. However, further tests are still needed to verify whether the SDM model is suitable for this study.
In this study, we use the Wald test and LR test to establish the specific model (results in Table 4). The results of the Wald and LR test in Table 4 pass the significance test of 1%, rejecting the null hypothesis that the SDM model can be simplified to the SAR or SEM model. So, the SDM model is the best choice for this study. Hence, we finally chose the SDM-DID model with fixed effect to assess the effect of HSR on GTFP.
6.2 Benchmark Regression Results
Table 4 reports the treatment effect of HSR on GTFP from Formula 5. The results in column 1) are based on the classical DID model with city and year fixed effects, representing a baseline for comparing our results. The classical DID model indicates that the opening of HSR increases GTFP by 0.04, which is significant at 1%. This conclusion also suggests that HSR can promote green development. The results of the regression are consistent with Hypothesis 1. Next, we report the results of the SDM-DID in column 4). The coefficient of
Based on SDM-DID and SAR-DID, the direct effect, indirect effect, and total effect are shown in Table 5. The direct effect of
6.3 Robustness Check
To verify the reliability of our regression results, several robustness tests are also conducted.
6.3.1 Alternative Model or Spatial Weight Matrix
First, we changed the setting form of the SDID model. Although we have confirmed that these models are not the most effective setting form, as a robustness test, they can still provide a reference for our results. The SAR-DID model, SEM-DID model, and SLX-DID3 model were used to examine the relationship between HSR and GTFP. The results of the SAR-DID model and SEM-DID model are shown in Columns 2) and 3) of Table 4, respectively. The results of the SLX-DID model are shown in Column 1) of Table 6.
Second, this study uses a geographic distance weight matrix to replace the queen matrix. Affected by the cost of transportation, when the distance between the two cities is reduced, the frequency of interaction between them will be higher. Therefore, the economic activities between cities will decrease as the geographical distance increases. We used the reciprocal of the distance between two cities to reflect this spatial relationship. We set the geographic distance weight matrix as follows:
where
6.3.2 Endogeneity
In this research, we added as many control variables as possible to reduce the bias of the estimated results. However, omitting the unobserved factors that affect both the opening of HSR and GTFP leads to serious endogeneity problems. Moreover, the economically developed eastern regions have denser HSR networks. Thus, there may be a bidirectional causal relationship between HSR and GTFP. We adopted an exogenous instrumental variable (IV) model to eliminate endogenous problems and estimate the effects of HSR on GTFP.
The cost of HSR construction is related to terrain factors. Generally, the construction costs in plain areas are lower than those in mountainous and hilly areas. Thus, we used the urban geographic slope as an instrumental variable for HSR. For econometric models with endogenous variables, the two-stage least square (2SLS) method is most commonly applied for estimating the coefficient of endogenous variables (Wooldridge, 2009). However, in this study,
As shown in Column 3) of Table 6, the regression results of the first stage suggest that the coefficient of
6.3.3 Parallel Trend Test
HSR-served cities had a parallel trend with non–HSR-served cities before the opening of HSR, which is the basic condition for ensuring the effectiveness of the DID model. In our study, a spatial weight matrix has been introduced to comprehensively evaluate the impact of HSR on GTFP; however, if there is no parallel trend for GTFP, we still cannot draw robust conclusions. Therefore, parallel trend testing is necessary for this study. The opening of HSR is a multi-stage experiment. For GTFP, we need to test whether there is a systematic difference between HSR-served and non–HSR-served cities. When there is no systematic difference, or even if there is a difference and the difference is fixed, the GTFP in HSR-served and non–HSR-served cities has a parallel trend. In this article, the event analysis method will be used to test whether there is a parallel trend. The following model, proposed by Beck et al. (2010), provides us with a way to test this issue:
where
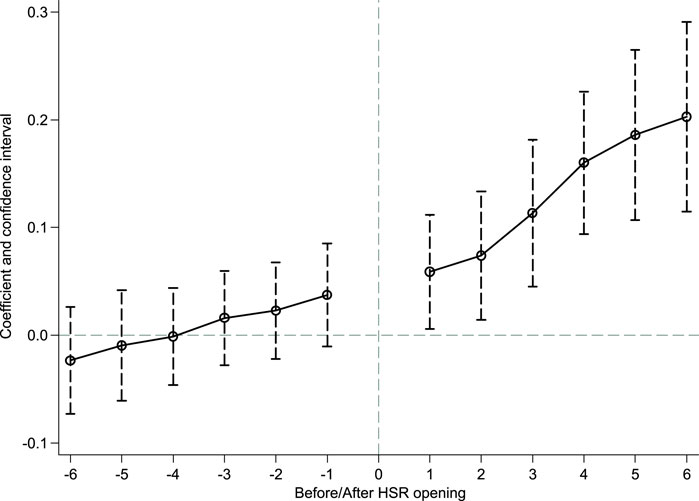
FIGURE 5. Results of the parallel trend test. Note: The dashed lines represent 95% confidence intervals.
6.4 Mechanism Test
The opening of HSR has a significant positive effect on GTFP, but how does HSR act on GTFP? We try to explore the mechanism of HSR on GTFP from the perspective of urban innovation and urban entrepreneurial vitality.
6.4.1 High-Speed Rail and Urban Innovation
The improvement of scientific and technological levels is an important means to realize energy saving and emission reduction. Green patents refer to inventions, utility models, and design patents with the theme of green technologies that are conducive to saving resources, improving energy efficiency, and preventing and controlling pollution. In this study, we use the green invention patent as a measure of urban innovation (
Table 7 reports the results. The coefficient of
6.4.2 High-Speed Rail and Urban Entrepreneurial Vitality
A city with entrepreneurial vitality tends to have more entrepreneurial activities. Entrepreneurial activities can accelerate the R & D of new technology; accelerate the generation of new enterprises and new industries, especially low-pollution industries with competitive advantages; promote market competition and resource integration; and thus improve GTFP. Therefore, we use urban innovation vitality (
7 Further Analysis
In Formula 5, the coefficient
where
Table 9 reports the results. The coefficient of
In terms of the coefficient of
In summary, the opening of HSR can promote GTFP. Meanwhile, HSR can affect the green development of neighboring cities via spatial spillover effects. Specifically, HSR promotes GTFP in neighboring HSR-served cities, whereas the impact on neighboring non–HSR-served cities is just the opposite. This can reasonably explain why cities go head-to-head in competition for HSR stops, as mentioned in the introduction. When a city becomes the winner in the HSR competition, it not only means that it can get convenient transportation, but it also means that through HSR, this city can achieve green development. However, the losers in the HSR competition may face more serious consequences; that is, the GTFP will not continue the previous growth trend or even face the risk of recession.
8 Conclusion
China’s first HSR opened in 2008, marking the country’s entry into the HSR era. HSR projects have achieved rapid development in China, and their status in social and economic development has become increasingly outstanding. HSR has also become the main resource for competition among local governments. However, the environmental costs brought about by the development of HSR have also become a focus of attention. Existing research on HSR has achieved substantial achievements in the fields of labor market, technological innovation, and housing prices. In contrast, the impact on GTFP has received little scholastic attention. In light of the lack of research on GTFP, this study considered the opening of HSR at different times and in different cities as a quasi-natural experiment while considering spatial spillover effects of HSR on economic development; moreover, it introduced a frontier approach to the SDID model to measure the net effects of HSR on GTFP.
First, we used the SBM-DDF-SML index to calculate the GTFPs of 284 cities in China. We further tested the spatial autocorrelation of urban GTFP year by year. The results show that the GTFP in China has a strong spatial autocorrelation. In other words, green development will be affected by neighboring cities. Second, this study used the SDM-DID model to estimate the effects of HSR on GTFP. Specifically, the results suggest that the opening of HSR could significantly promote GTFP. In addition, there is a statistically insignificant spatial spillover effect on neighboring cities. In light of this result, we conducted further analysis and found that there was a spatially heterogeneous effect of HSR on GTFP. It was found that the opening of HSR could promote neighboring HSR-served cities’ GTFP, which means there is a positive spillover effect between cities connected by HSR. However, HSR has a negative effect on neighboring non–HSR-served cities, mainly because the siphoning effects of HSR-served cities on non–HSR-served cities are greater than their spillover effects. The mechanism test suggested that HSR can influence GTFP by promoting urban green innovation and entrepreneurial vitality. Third, the government needs to invest a lot of labor and financial resources to construct HSR and other infrastructure. However, most HSRs in China are operating at a loss. As a novel public good provided by the government, the main goal of HSR is to maximize social welfare; it does not necessarily need to be profitable. Therefore, effective evaluation of the welfare effects of HSR has important practical significance. However, the welfare effects of HSR include not only economic but also public welfare effects, such as environment and health. From the perspective of environmental welfare, this article provided evidence that the opening of HSR brings additional benefits. On the whole, HSR can promote the improvement of GTFP; however, HSR seems to be unfair to non–HSR-served cities because it will hinder the green development of these cities.
Based on these findings outlined above, the study holds that as one of the most important infrastructures in the new era, HSR has accelerated the cross-regional flow of labor, logistics, and information, and it has played a positive role in improving energy efficiency and optimizing industrial structure. In this context, China may roll out various policies to support the construction of HSR as follows: 1) HSR could promote GTFP for HSR-served cities; therefore, the government may promote the construction of HSR and continuously increase the density of HSR networks. Moreover, the government can accelerate the construction of supporting infrastructure around HSR stations to truly build a green transportation system with zero-distance interchanges and seamless connections. 2) The opening of HSR can promote GTFP in neighboring HSR-served cities. Therefore, the government may strengthen the construction of urban agglomerations and implement prevention and control mechanisms for environmental pollution. Meanwhile, HSR-served cities can consider their positioning and industrial division of labor from the perspective of urban agglomeration. On the one hand, HSR-served cities can strengthen their efforts to undertake international high-end industries. On the other hand, HSR-served cities can support neighboring cities through industrial transfer, improving the competitiveness of urban agglomeration. 3) The opening of HSR has a negative effect on GTFP for neighboring non–HSR-served cities. Thus, non–HSR-served cities may take the initiative to undertake the quality transfer industries of regional central cities and make maximum use of the “spillover effect” by HSR. Then, non–HSR-served cities can form a differentiated development pattern from central cities. 4) Finally, HSR promotes GTFP by increasing green innovation and entrepreneurial vitality. The government can encourage enterprises to carry out green innovation through policy subsidies. In addition, the public sector may create an innovative and entrepreneurial urban environment to stimulate the innovative vitality of human capital. Ultimately, the implementation of these strategies can optimize the layout of HSR and help build an integrated HSR network in China.
Though, the study is not without limitations as it only focuses on a single country. In addition, it took a lot of time to collect the data, and the available sample size allows for a good experimental study, though it only covers the period 2006–2017. It will be interesting to see if other important and innovative variables such as the internet of things (IoT) and digital transformation can better explain this relationship. In addition, it would be interesting to analyze the impact of different transportation infrastructures, such as highways, urban rail, and air transportation, on GTFP. In the future, researchers should consider the long-term impact of HSR and the logistics and transportation of China’s HSR. They can also study the different ways of developed and developing countries’ implementation to achieve sustainable development.
Data Availability Statement
The raw data supporting the conclusion of this article will be made available by the authors, without undue reservation.
Author Contributions
SL: empirical research and analysis. YZ: literature review and mechanism analysis. JC: design and literature collection.
Funding
We are grateful for the financial support of the Social Science Planning Annual Project of Shanghai Province (No. 2021BJB003); Graduate Innovation Fund of Shanghai University of Finance and Economics (No. CXJJ-2021-441).
Conflict of Interest
The authors declare that the research was conducted in the absence of any commercial or financial relationships that could be construed as a potential conflict of interest.
Publisher’s Note
All claims expressed in this article are solely those of the authors and do not necessarily represent those of their affiliated organizations, or those of the publisher, the editors, and the reviewers. Any product that may be evaluated in this article, or claim that may be made by its manufacturer, is not guaranteed or endorsed by the publisher.
Acknowledgments
Author gratitude is extended to the prospective editor(s) and reviewers who will/have spared time to guide toward a successful publication.
Footnotes
1“4 + 4” indicates four lines running north–south (verticals) and four lines running east–west (horizontals), while “8 + 8” indicates eight lines running north–south (verticals) and eight lines running east–west (horizontals).
2Data from: China Statistical Yearbook.
3In this study, the SLX-DID model is set as
4To decompose the spatial effect of HSR on GTFP, we need to decompose the spatial weight matrix. Thus,
References
Acs, Z., and Armington, C. (2004). Employment Growth and Entrepreneurial Activity in Cities. Reg. Stud. 38 (8), 911–927. doi:10.1080/0034340042000280938
Ahlfeldt, G. M., and Feddersen, A. (2018). From Periphery to Core: Measuring Agglomeration Effects Using High-Speed Rail. J. Econ. Geogr. 18 (2), 355–390. doi:10.1093/jeg/lbx005
Åkerman, J. (2011). The Role of High-Speed Rail in Mitigating Climate Change - the Swedish Case Europabanan from a Life Cycle Perspective. Transp. Res. Part D Transp. Environ. 16 (3), 208–217. doi:10.1016/j.trd.2010.12.004
Aldieri, L., Kotsemir, M., and Paolo Vinci, C. (2021). Environmental Innovations and Productivity: Empirical Evidence from Russian Regions. Resour. Policy 74, 101444. doi:10.1016/j.resourpol.2019.101444
Anderson, M. L. (2014). Subways, Strikes, and Slowdowns: The Impacts of Public Transit on Traffic Congestion. Am. Econ. Rev. 104 (9), 2763–2796. doi:10.1257/aer.104.9.2763
Anselin, L., Bera, A. K., Florax, R., and Yoon, M. J. (1996). Simple Diagnostic Tests for Spatial Dependence. Regional Sci. Urban Econ. 26 (1), 77–104. doi:10.1016/0166-0462(95)02111-6
Audretsch, D. B., Heger, D., and Veith, T. (2014). Infrastructure and Entrepreneurship. Small Bus. Econ. 44 (2), 219–230. doi:10.1007/s11187-014-9600-6
Banerjee, A., Duflo, E., and Qian, N. (2020). On the Road: Access to Transportation Infrastructure and Economic Growth in China. J. Dev. Econ. 145, 102442. doi:10.1016/j.jdeveco.2020.102442
Banister, D., and Berechman, Y. (2001). Transport Investment and the Promotion of Economic Growth. J. Transp. Geogr. 9 (3), 209–218. doi:10.1016/s0966-6923(01)00013-8
Beck, T., Levine, R., and Levkov, A. (2010). Big Bad Banks? the Winners and Losers from Bank Deregulation in the United States. J. Finance 65 (5), 1637–1667. doi:10.1111/j.1540-6261.2010.01589.x
Berger, E. S. C., and Kuckertz., A. (2016). Female Entrepreneurship in Startup Ecosystems Worldwide. J. Bus. Res. 69 (11), 5163–5168. doi:10.1016/j.jbusres.2016.04.098
Bernard, A. B., Moxnes, A., and Saito, Y. U. (2019). Production Networks, Geography, and Firm Performance. J. Political Econ. 127 (2), 639–688. doi:10.1086/700764
Calderón-Garcidueñas, L., Calderón-Garcidueñas, A., Torres-Jardón, R., Avila-Ramírez, J., Kulesza, R. J., and Angiulli, A. D. (2014). Air Pollution and Your Brain: What Do You Need to Know Right Now. Prim. Health Care Res. Dev. 16 (04), 329–345. doi:10.1017/S146342361400036X
Cascetta, E., Cartenì, A., Henke, I., and Pagliara, F. (2020). Economic Growth, Transport Accessibility and Regional Equity Impacts of High-Speed Railways in Italy: Ten Years Ex Post Evaluation and Future Perspectives. Transp. Res. Part A Policy Pract. 139, 412–428. doi:10.1016/j.tra.2020.07.008
Chagas, A. L. S., Azzoni, C. R., and Almeida, A. N. (2016). A Spatial Difference-In-Differences Analysis of the Impact of Sugarcane Production on Respiratory Diseases. Regional Sci. Urban Econ. 59, 24–36. doi:10.1016/j.regsciurbeco.2016.04.002
Chambers, R. G., Chung, Y., and Färe, R. (1996). Benefit and Distance Functions. J. Econ. Theory 70 (2), 407–419. doi:10.1006/jeth.1996.0096
Chang, Y., Lei, S., Teng, J., Zhang, J., Zhang, L., and Xu, X. (2019). The Energy Use and Environmental Emissions of High-Speed Rail Transportation in China: A Bottom-Up Modelling. Energy 182, 1193–1201. doi:10.1016/j.energy.2019.06.120
Chen, C.-L. (2012). Reshaping Chinese Space-Economy through High-Speed Trains: Opportunities and Challenges. J. Transp. Geogr. 22, 312–316. doi:10.1016/j.jtrangeo.2012.01.028
Chen, C.-L., and Vickerman, R. (2016). Can Transport Infrastructure Change Regions' Economic Fortunes? Some Evidence from Europe and China. Reg. Stud. 51 (1), 144–160. doi:10.1080/00343404.2016.1262017
Chen, L., and You, Y. (2021). How Does Environmental Pollution Erode Political Trust in China? A Multilevel Analysis. Environ. Impact Assess. Rev. 88, 106553. doi:10.1016/j.eiar.2021.106553
Chen, S., and Golley, J. (2014). 'Green' Productivity Growth in China's Industrial Economy. Energy Econ. 44, 89–98. doi:10.1016/j.eneco.2014.04.002
Chen, Y., Ebenstein, A., Greenstone, M., and Li, H. (2013). Evidence on the Impact of Sustained Exposure to Air Pollution on Life Expectancy from China's Huai River Policy. Proc. Natl. Acad. Sci. U.S.A. 110 (32), 12936–12941. doi:10.1073/pnas.1300018110
Chen, Y., and Wei, L. (2018). Railroad Development, Temporal-Spatial Externalities, and Growth Spillover: Theory and Empirical Evidence. J. Reg. Sci. 58 (5), 980–1002. doi:10.1111/jors.12407
Chen, Z., and Haynes, K. E. (2015). Impact of High-Speed Rail on Housing Values: An Observation from the Beijing-Shanghai Line. J. Transp. Geogr. 43, 91–100. doi:10.1016/j.jtrangeo.2015.01.012
Chen, Z., Xue, J., Rose, A. Z., and Haynes, K. E. (2016). The Impact of High-Speed Rail Investment on Economic and Environmental Change in China: A Dynamic CGE Analysis. Transp. Res. Part A Policy Pract. 92, 232–245. doi:10.1016/j.tra.2016.08.006
Cheng, Y.-H. (2010). High-Speed Rail in Taiwan: New Experience and Issues for Future Development. Transp. Policy 17 (2), 51–63. doi:10.1016/j.tranpol.2009.10.009
Cheng, Y.-s., Loo, B. P. Y., and Vickerman, R. (2015). High-Speed Rail Networks, Economic Integration and Regional Specialisation in China and Europe. Travel Behav. Soc. 2 (1), 1–14. doi:10.1016/j.tbs.2014.07.002
Chester, M., and Horvath, A. (2012). High-speed Rail with Emerging Automobiles and Aircraft Can Reduce Environmental Impacts in California's Future. Environ. Res. Lett. 7 (3), 034012. doi:10.1088/1748-9326/7/3/034012
Dalkic, G., Balaban, O., Tuydes-Yaman, H., and Celikkol-Kocak, T. (2017). An Assessment of the CO 2 Emissions Reduction in High-Speed Rail Lines: Two Case Studies from Turkey. J. Clean. Prod. 165, 746–761. doi:10.1016/j.jclepro.2017.07.045
De Rus, G. (2021). The Bca of Hsr: Should the Government Invest in High-Speed Rail Infrastructure? J. Benefit Cost. Anal. 2 (1), 1–28. doi:10.2202/2152-2812.1058
Debrezion, G., Pels, E., and Rietveld, P. (2010). The Impact of Rail Transport on Real Estate Prices. Urban Stud. 48 (5), 997–1015. doi:10.1177/0042098010371395
Diao, M. (2018). Does Growth Follow the Rail? the Potential Impact of High-Speed Rail on the Economic Geography of China. Transp. Res. Part A Policy Pract. 113, 279–290. doi:10.1016/j.tra.2018.04.024
Dong, X., and Zheng, S., and Kahn, M. E. (2020). The Role of Transportation Speed in Facilitating High Skilled Teamwork across Cities. J. Urban Econ. 115, 103212. doi:10.1016/j.jue.2019.103212
Driessen, P. H., Hillebrand, B., Kok, R. A. W., and Verhallen, T. M. M. (2013). Green New Product Development: The Pivotal Role of Product Greenness. IEEE Trans. Eng. Manage. 60 (2), 315–326. doi:10.1109/tem.2013.2246792
Duan, L., Sun, W., and Zheng, S. (2020). Transportation Network and Venture Capital Mobility: An Analysis of Air Travel and High-Speed Rail in China. J. Transp. Geogr. 88, 102852. doi:10.1016/j.jtrangeo.2020.102852
Elhorst, J. P., Lacombe, D. J., and Piras, G. (2012). On Model Specification and Parameter Space Definitions in Higher Order Spatial Econometric Models. Regional Sci. Urban Econ. 42 (1-2), 211–220. doi:10.1016/j.regsciurbeco.2011.09.003
Faber, B. (2014). Trade Integration, Market Size, and Industrialization: Evidence from China's National Trunk Highway System. Rev. Econ. Stud. 81 (3), 1046–1070. doi:10.1093/restud/rdu010
Fan, X., Xu, Y., Nan, Y., Li, B., and Cai, H. (2020). Impacts of High-Speed Railway on the Industrial Pollution Emissions in China. Kybernetes 49 (11), 2713–2735. doi:10.1108/k-07-2019-0499
Fare, R., Grosskopf, S., and Pasurkajr, C. (2007). Environmental Production Functions and Environmental Directional Distance Functions. Energy 32 (7), 1055–1066. doi:10.1016/j.energy.2006.09.005
Fareed, Z., Rehman, M. A., Adebayo, T. S., Wang, Y., Ahmad, M., and Shahzad, F. (2022). Financial Inclusion and the Environmental Deterioration in Eurozone: The Moderating Role of Innovation Activity. Technol. Soc. 69, 101961. doi:10.1016/j.techsoc.2022.101961
Farrell, M. J. (1957). The Measurement of Productive Efficiency. J. R. Stat. Soc. Ser. A General. 120 (3), 253–281. doi:10.2307/2343100
Feng, G., and Serletis, A. (2014). Undesirable Outputs and a Primal Divisia Productivity Index Based on the Directional Output Distance Function. J. Econ. 183 (1), 135–146. doi:10.1016/j.jeconom.2014.06.014
Fritsch, M., and Storey, D. J. (2014). Entrepreneurship in a Regional Context: Historical Roots, Recent Developments and Future Challenges. Reg. Stud. 48 (6), 939–954. doi:10.1080/00343404.2014.892574
Fukuyama, H., and Weber, W. L. (2009). A Directional Slacks-Based Measure of Technical Inefficiency. Socio-Economic Plan. Sci. 43 (4), 274–287. doi:10.1016/j.seps.2008.12.001
Gao, Y., Zhang, M., and Zheng, J. (2021). Accounting and Determinants Analysis of China's Provincial Total Factor Productivity Considering Carbon Emissions. China Econ. Rev. 65, 101576. doi:10.1016/j.chieco.2020.101576
Glaeser, E. L., Rosenthal, S. S., and Strange, W. C. (2010). Urban Economics and Entrepreneurship. J. Urban Econ. 67 (1), 1–14. doi:10.1016/j.jue.2009.10.005
Guo, S., and Chen, L. (2019). Can Urban Rail Transit Systems Alleviate Air Pollution? Empirical Evidence from Beijing. Growth Change 50 (1), 130–144. doi:10.1111/grow.12266
Guo, S., Tang, X., Meng, T., Chu, J., Tang, H., and Zhang, Wei. (2021). Industrial Structure, R&D Staff, and Green Total Factor Productivity of China: Evidence from the Low-Carbon Pilot Cities. Complexity 2021, 1–13. doi:10.1155/2021/6690152
Guo, X., Sun, W., Yao, S., and Zheng, S. (2020). Does High-Speed Railway Reduce Air Pollution along Highways? - Evidence from China. Transp. Res. Part D Transp. Environ. 89, 102607. doi:10.1016/j.trd.2020.102607
Han, D., Li, T., Feng, S., and Shi, Z. (2020). Application of Threshold Regression Analysis to Study the Impact of Clean Energy Development on China's Carbon Productivity. Ijerph 17 (3), 1060. doi:10.3390/ijerph17031060
He, G., Wang, S., and Zhang, B. (2020). Watering Down Environmental Regulation in China*. Q. J. Econ. 135 (4), 2135–2185. doi:10.1093/qje/qjaa024
Hernández, A., and Luis Jiménez, J. (2014). Does High-Speed Rail Generate Spillovers on Local Budgets? Transp. Policy 35, 211–219. doi:10.1016/j.tranpol.2014.06.003
Huang, Y., and Xu, W. (2021). Spatial and Temporal Heterogeneity of the Impact of High-Speed Railway on Urban Economy: Empirical Study of Chinese Cities. J. Transp. Geogr. 91, 102972. doi:10.1016/j.jtrangeo.2021.102972
Huang, Y., and Zong, H. (2021). Has High-Speed Railway Promoted Spatial Equity at Different Levels? A Case Study of Inland Mountainous Area of China. Cities 110, 103076. doi:10.1016/j.cities.2020.103076
Huang, Z., and Du, X. (2021). How Does High-Speed Rail Affect Land Value? Evidence from China. Land Use Policy 101, 105068. doi:10.1016/j.landusepol.2020.105068
Ibanez, E., and McCalley, J. D. (2011). Multiobjective Evolutionary Algorithm for Long-Term Planning of the National Energy and Transportation Systems. Energy Syst. 2 (2), 151–169. doi:10.1007/s12667-011-0031-z
Israel, E., and Cohen-Blankshtain, G. (2010). Testing the Decentralization Effects of Rail Systems: Empirical Findings from Israel. Transp. Res. Part A Policy Pract. 44 (7), 523–536. doi:10.1016/j.tra.2010.03.021
James, D. E., Jansen, H. M. A., and Opschoor, J. B. (2013). Economic Approaches to Environmental Problems: Techniques and Results of Empirical Analysis. Elsevier.
Jia, R., Shao, S., and Yang, L. (2021). High-Speed Rail and Co2 Emissions in Urban China: A Spatial Difference-In-Differences Approach. Energy Econ. 99, 105271. doi:10.1016/j.eneco.2021.105271
Jiao, J., Wang, J., and Jin, F. (2017). Impacts of High-Speed Rail Lines on the City Network in China. J. Transp. Geogr. 60, 257–266. doi:10.1016/j.jtrangeo.2017.03.010
Jin, M., Lin, K.-C., Shi, W., Lee, P. T. W., and Li, K. X. (2020). Impacts of High-Speed Railways on Economic Growth and Disparity in China. Transp. Res. Part A Policy Pract. 138, 158–171. doi:10.1016/j.tra.2020.05.013
Kaewunruen, S., Sresakoolchai, J., Peng, J., and Peng, J. (2019). Life Cycle Cost, Energy and Carbon Assessments of Beijing-Shanghai High-Speed Railway. Sustainability 12 (1), 206. doi:10.3390/su12010206
Kassouri, Y. (2022). Fiscal Decentralization and Public Budgets for Energy RD&D: A Race to the Bottom? Energy Policy 161, 112761. doi:10.1016/j.enpol.2021.112761
Ke, X., Chen, H., Hong, Y., and Hsiao, C. (2017). Do China's High-Speed-Rail Projects Promote Local Economy?-New Evidence from a Panel Data Approach. China Econ. Rev. 44, 203–226. doi:10.1016/j.chieco.2017.02.008
Kuang, C., Liu, Z., and Zhu, W. (2021). Need for Speed: High-Speed Rail and Firm Performance. J. Corp. Finance 66, 101830. doi:10.1016/j.jcorpfin.2020.101830
Leonidou, L. C., Christodoulides, P., Kyrgidou, L. P., and Palihawadana, D. (2015). Internal Drivers and Performance Consequences of Small Firm Green Business Strategy: The Moderating Role of External Forces. J. Bus. Ethics 140 (3), 585–606. doi:10.1007/s10551-015-2670-9
LeSage, J. P., and Pace, R. K. (2010). “Spatial Econometric Models,” in Handbook of Applied Spatial Analysis: Software Tools, Methods and Applications. Editors M. M. Fischer, and A. Getis (Berlin, Heidelberg: Springer Berlin Heidelberg), 355–376. doi:10.1007/978-3-642-03647-7_18
Li, T., Han, D., Ding, Y., and Shi, Z. (2020). How Does the Development of the Internet Affect Green Total Factor Productivity? Evidence from China. IEEE Access 8, 216477–216490. doi:10.1109/access.2020.3041511
Li, X., Huang, B., Li, R., and Zhang, Y. (2016). Exploring the Impact of High-Speed Railways on the Spatial Redistribution of Economic Activities - Yangtze River Delta Urban Agglomeration as a Case Study. J. Transp. Geogr. 57, 194–206. doi:10.1016/j.jtrangeo.2016.10.011
Li, X., Wu, Z., and Zhao, X. (2020). Economic Effect and its Disparity of High-Speed Rail in China: A Study of Mechanism Based on Synthesis Control Method. Transp. Policy 99, 262–274. doi:10.1016/j.tranpol.2020.09.003
Li, X., and Yu, B. (2019). Peaking Co2 Emissions for China's Urban Passenger Transport Sector. Energy Policy 133, 110913. doi:10.1016/j.enpol.2019.110913
Li, Y., Chen, Z., and Wang, P. (2020). Impact of High-Speed Rail on Urban Economic Efficiency in China. Transp. Policy 97, 220–231. doi:10.1016/j.tranpol.2020.08.001
Li, Z., and Xu, H. (2018). High-Speed Railroads and Economic Geography: Evidence from Japan. J. Reg. Sci. 58 (4), 705–727. doi:10.1111/jors.12384
Liu, G., Wang, B., Cheng, Z., and Zhang, N. (2020). The Drivers of China's Regional Green Productivity, 1999-2013. Resour. Conservation Recycle. 153, 104561. doi:10.1016/j.resconrec.2019.104561
Liu, S., Wan, Y., and Zhang, A. (2020). Does China's High-Speed Rail Development Lead to Regional Disparities? A Network Perspective. Transp. Res. Part A Policy Pract. 138, 299–321. doi:10.1016/j.tra.2020.06.010
Ma, L., Niu, D., and Sun, W. (2021). Transportation Infrastructure and Entrepreneurship: Evidence from High-Speed Railway in China. China Econ. Rev. 65, 101577. doi:10.1016/j.chieco.2020.101577
Martin, F. (1997). Justifying a High-Speed Rail Project: Social Value vs. Regional Growth. Ann. regional Sci. 31 (2), 155–174. doi:10.1007/s001680050043
Matas, A., Raymond, J. L., and Roig, J. L. (2020). Evaluating the Impacts of Hsr Stations on the Creation of Firms. Transp. Policy 99, 396–404. doi:10.1016/j.tranpol.2020.09.010
Meng, X., Lin, S., and Zhu, X. (2018). The Resource Redistribution Effect of High-Speed Rail Stations on the Economic Growth of Neighbouring Regions: Evidence from China. Transp. Policy 68, 178–191. doi:10.1016/j.tranpol.2018.05.006
Moyano, A., and Dobruszkes, F. (2017). Mind the Services! High-Speed Rail Cities Bypassed by High-Speed Trains. Case Stud. Transp. Policy 5 (4), 537–548. doi:10.1016/j.cstp.2017.07.005
Noseleit, F. (2012). Entrepreneurship, Structural Change, and Economic Growth. J. Evol. Econ. 23 (4), 735–766. doi:10.1007/s00191-012-0291-3
Oum, T. H., and Fu, X. (2008). Impacts of Airports on Airline Competition: Focus on Airport Performance and Airport-Airline Vertical Relations. OECD/ITF Joint Transport Research Centre Discussion Paper, No.2008-17. doi:10.1787/235140743836
Peng, Y., Chen, Z., Xu, J., and Lee, J. (2020). Analysis of Green Total Factor Productivity Trend and its Determinants for the Countries along Silk Roads. Growth Change 51 (4), 1711–1726. doi:10.1111/grow.12435
Petersen, M. A., and Rajan, R. G. (2002). Does Distance Still Matter? the Information Revolution in Small Business Lending. J. Finance 57 (6), 2533–2570. doi:10.1111/1540-6261.00505
Pontarollo, N., and Ricciuti, R. (2020). Railways and Manufacturing Productivity in Italy after Unification. J. Reg. Sci. 60 (4), 775–800. doi:10.1111/jors.12484
Porter, M. E., and Van der Linde, C. (1995). Green and Competitive: Ending the Stalemate. Harv. Bus. Rev. 73 (5), 120–134.
Qin, Y. (2016). ‘No County Left behind?’ the Distributional Impact of High-Speed Rail Upgrades in China. J. Econ. Geogr. (3), 1–32. doi:10.1093/JEG/LBW013
Rehman, M. A., Fareed, Z., Salem, S., Kanwal, A., and Pata, U. K. (2021). Do Diversified Export, Agriculture, and Cleaner Energy Consumption Induce Atmospheric Pollution in Asia? Application of Method of Moments Quantile Regression. Front. Environ. Sci. 9, 781097. doi:10.3389/fenvs.2021.781097
Rehman, M. A., Fareed, Z., Shahzad, F., and Shahzad, F. (2022). When Would the Dark Clouds of Financial Inclusion Be over, and the Environment Becomes Clean? the Role of National Governance. Environ. Sci. Pollut. Res. 29 (19), 27651–27663. doi:10.1007/s11356-021-17683-0
Rennings, K. (2000). Redefining Innovation - Eco-Innovation Research and the Contribution from Ecological Economics. Ecol. Econ. 32 (2), 319–332. doi:10.1016/s0921-8009(99)00112-3
Schiederig, T., Tietze, F., and Herstatt, C. (2012). Green Innovation in Technology and Innovation Management - an Exploratory Literature Review. R&D Manage 42 (2), 180–192. doi:10.1111/j.1467-9310.2011.00672.x
Shao, S., Tian, Z., and Yang, L. (2017). High Speed Rail and Urban Service Industry Agglomeration: Evidence from China's Yangtze River Delta Region. J. Transp. Geogr. 64, 174–183. doi:10.1016/j.jtrangeo.2017.08.019
Song, M., Zhang, G., Zeng, W., Liu, J., and Fang, K. (2016). Railway Transportation and Environmental Efficiency in China. Transp. Res. Part D Transp. Environ. 48, 488–498. doi:10.1016/j.trd.2015.07.003
Sun, X., Yan, S., Liu, T., and Wu, J. (2020). High-Speed Rail Development and Urban Environmental Efficiency in China: A City-Level Examination. Transp. Res. Part D Transp. Environ. 86, 102456. doi:10.1016/j.trd.2020.102456
Ullah, I., Rehman, A., Svobodova, L., Akbar, A., Shah, M. H., Zeeshan, M., et al. (2022). Investigating Relationships between Tourism, Economic Growth, and CO2 Emissions in Brazil: An Application of the Nonlinear ARDL Approach. Front. Environ. Sci. 10, 843906. doi:10.3389/fenvs.2022.843906
Vickerman, R. (2018). Can High-Speed Rail Have a Transformative Effect on the Economy? Transp. Policy 62, 31–37. doi:10.1016/j.tranpol.2017.03.008
Wang, F., Wei, X., Liu, J., He, L., and Gao, M. (2019). Impact of High-Speed Rail on Population Mobility and Urbanisation: A Case Study on Yangtze River Delta Urban Agglomeration, China. Transp. Res. Part A Policy Pract. 127, 99–114. doi:10.1016/j.tra.2019.06.018
Wang, X., Xie, Z., Zhang, X., and Huang, Y. (2018). Roads to Innovation: Firm-Level Evidence from People's Republic of China (PRC). China Econ. Rev. 49, 154–170. doi:10.1016/j.chieco.2017.12.012
Wooldridge, J. M. (2009). Introductory Econometrics: A Modern Approach. Mason: South-Western Cengage learning.
Xia, F., and Xu, J. (2020). Green Total Factor Productivity: A Re-examination of Quality of Growth for Provinces in China. China Econ. Rev. 62, 101454. doi:10.1016/j.chieco.2020.101454
Xu, W., Zhou, J., Yang, L., and Li, L. (2018). The Implications of High-Speed Rail for Chinese Cities: Connectivity and Accessibility. Transp. Res. Part A Policy Pract. 116, 308–326. doi:10.1016/j.tra.2018.06.023
Yan, Z., Zou, B., Du, K., and Li, K. (2020). Do Renewable Energy Technology Innovations Promote China's Green Productivity Growth? Fresh Evidence from Partially Linear Functional-Coefficient Models. Energy Econ. 90, 104842. doi:10.1016/j.eneco.2020.104842
Yang, H., Zhang, F., and He, Y. (2021). Exploring the Effect of Producer Services and Manufacturing Industrial Co-agglomeration on the Ecological Environment Pollution Control in China. Environ. Dev. Sustain. 23, 16119–16144. doi:10.1007/s10668-021-01339-7
Yang, Z., Li, C., Jiao, J., Liu, W., and Zhang, F. (2020). On the Joint Impact of High-Speed Rail and Megalopolis Policy on Regional Economic Growth in China. Transp. Policy 99, 20–30. doi:10.1016/j.tranpol.2020.08.007
Yu, F., Lin, F., Tang, Y., and Zhong, C. (2019). High‐speed Railway to Success? the Effects of High‐speed Rail Connection on Regional Economic Development in China. J. Reg. Sci. 59 (4), 723–742. doi:10.1111/jors.12420
Zeng, Y., Cao, Y., Qiao, X., Seyler, B. C., and Tang, Y. (2019). Air Pollution Reduction in China: Recent Success but Great Challenge for the Future. Sci. Total Environ. 663, 329–337. doi:10.1016/j.scitotenv.2019.01.262
Zhang, J., Lu, G., Skitmore, M., and Ballesteros-Pérez, P. (2021). A Critical Review of the Current Research Mainstreams and the Influencing Factors of Green Total Factor Productivity. Environ. Sci. Pollut. Res. 28 (27), 35392–35405. doi:10.1007/s11356-021-14467-4
Zhang, Q., Yan, F., Li, K., and Ai, H. (2019). Impact of Market Misallocations on Green Tfp: Evidence from Countries along the Belt and Road. Environ. Sci. Pollut. Res. 26 (34), 35034–35048. doi:10.1007/s11356-019-06601-0
Zhang, Y., Song, Y., and Zou, H. (2020). Transformation of Pollution Control and Green Development: Evidence from China's Chemical Industry. J. Environ. Manag. 275, 111246. doi:10.1016/j.jenvman.2020.111246
Zhao, M., Liu, F., Sun, W., and Tao, X. (2020). The Relationship between Environmental Regulation and Green Total Factor Productivity in China: An Empirical Study Based on the Panel Data of 177 Cities. Ijerph 17 (15), 5287. doi:10.3390/ijerph17155287
Zheng, L., Long, F., Chang, Z., and Ye, J. (2019). Ghost Town or City of Hope? the Spatial Spillover Effects of High-Speed Railway Stations in China. Transp. Policy 81, 230–241. doi:10.1016/j.tranpol.2019.07.005
Zheng, S., Zhang, X., Sun, W., and Wang, J. (2019). The Effect of a New Subway Line on Local Air Quality: A Case Study in Changsha. Transp. Res. Part D Transp. Environ. 68, 26–38. doi:10.1016/j.trd.2017.10.004
Zhu, X., Chen, Y., and Feng, C. (2018). Green Total Factor Productivity of China's Mining and Quarrying Industry: A Global Data Envelopment Analysis. Resour. Policy 57, 1–9. doi:10.1016/j.resourpol.2017.12.009
Keywords: high-speed rail, green total factor productivity, mechanism testing, spatial difference-in-difference, China
Citation: Liu S, Zhang Y and Cao J (2022) Can High-Speed Rail Help Achieve Urban Green Development? From a Spatial Perspective. Front. Environ. Sci. 10:894697. doi: 10.3389/fenvs.2022.894697
Received: 12 March 2022; Accepted: 13 May 2022;
Published: 23 June 2022.
Edited by:
Zeeshan Fareed, Huzhou University, ChinaReviewed by:
Yacouba Kassouri, Leipzig University, GermanyFei Fan, Wuhan University, China
Mubeen Abdur Rehman, The University of Lahore, Pakistan
Copyright © 2022 Liu, Zhang and Cao. This is an open-access article distributed under the terms of the Creative Commons Attribution License (CC BY). The use, distribution or reproduction in other forums is permitted, provided the original author(s) and the copyright owner(s) are credited and that the original publication in this journal is cited, in accordance with accepted academic practice. No use, distribution or reproduction is permitted which does not comply with these terms.
*Correspondence: Yumeng Zhang, emhhbmd5dW1lbmdAMTYzLnN1ZmUuZWR1LmNu