- 1School of Tourism and Historical Culture, Southwest Minzu University, Chengdu, China
- 2School of Architecture, Southwest Minzu University, Chengdu, China
- 3Faculty of International Tourism and Management, City University of Macau, Macau, China
- 4Business and Tourism School, Sichuan Agricultural University, Dujiangyan, China
- 5Department of Management, Marketing and Entrepreneurship, University of Canterbury, Canterbury, New Zealand
- 6School of Management, Chengdu University of Information Technology, Chengdu, Sichuan, China
It is increasingly urgent to constantly monitor and analyze the carbon emission of international tourism activities. This paper focuses on countries and regions along the Belt and Road (B&R), utilizes the MRIO model to approximate international tourism carbon footprint (ITCT), and analyzes the patterns of carbon transfer. Panel model is further applied to discuss the effects of various influencing factors. The results show that: 1) in 2015, ITCT along the B&R accounts for approximately 40.74% of global carbon emission of the year; 2) ITCT along the B&R experienced continuous growth from 2005 to 2015, with an annual growth rate of 3.22%; 3) individual income level, urbanization, and air passenger volume in the countries along the B&R has positive impact on ITCT, which varies according to the income level of the country. The results are of significance for countries and regions along the B&R to formulate future policies for tourism development.
1 Introduction
In September and October 2013, Chinese President Xi Jinping put forward the cooperation initiative of the New Silk Road Economic Belt and the 21st Century Maritime Silk Road respectively, which is called the Belt and Road (B&R) for short (Zhang, 2019). The Belt and Road Initiative (BRI) aims to achieve win-win cooperations and common prosperities between China and other Asian, African and European countries (Li et al., 2020; Zhai, 2018). Against the backdrop of the current global economic downturn, BRI stimulates demand and employment, encourages investment and consumption, explores the development potential of regions along the B&R, and ultimately provides markets and impetus for global economic development by enhancing the flow of economic factors, promoting connectivity among countries and integrating markets (Irshad, 2015; O’Trakoun, 2018; Pacheco Pardo, 2018). To date, China has signed visa waiver agreements with 57 countries along the B&R, jointly celebrated the “Year of Tourism” with several countries, and established tourism cooperation initiatives such as the Silk Road Tourism Marketing Alliance, the Maritime Silk Road Tourism Promotion Alliance, and the Great Tea Road International Tourism Alliance. This corresponds to the fact that almost all countries along the B&R see tourism as a pillar sector to their economies (Li et al., 2020; Torres-Delgado et al., 2024).
According to the China Tourism Academy, the number of tourists from China alone to other countries along the B&R has been increasing steadily year by year, from 15.49 million in 2013 to 27.41 million in 2017. Clearly, as a driving factor for regional economic expansion within the region (Zhai, 2018), tourism activities on this scale have a significant impact on the environment (Gössling, 2002) as increase energy demand (Selvanathan et al., 2021) and thus are responsible for the increasing CO2 emission (Dogan et al., 2020; Inglesi-Lotz and Dogan, 2018; Moutinho et al., 2018; Wen and Shao, 2019; Yamano and Ahmad, 2006; Zameer and Wang, 2018). Tourism’s global carbon footprint, which are mainly contributed by transport, shopping and food, accounts for around 8% of global greenhouse gas emissions (2009-2013) (Lenzen et al., 2018). Given the commitment of countries associated with the United Nations Framework Convention on Climate Change (UNFCCC) to achieve a net zero increase in carbon emissions, and the distinct Sustainable Development Goal of combating climate change ratified by the UN (2017) and future projections of an unabated tourism growth, continuous monitoring and analysis of tourism-related carbon emissions is becoming increasingly urgent (Lenzen et al., 2018).
Research on tourism carbon footprint has increased significantly focuses on the urban tourism carbon footprint (Yang and Guo, 2024), the impact of tourism on CO 2 emission (Gössling et al., 2011; Le and Nguyen, 2021), namely, the causality between tourism, economic growth and CO 2 emissions (Adedoyin and Bekun, 2020; Selvanathan et al., 2021), the inter-relationship between tourist arrivals, energy consumption and pollutant emissions (Nepal et al., 2019), as well as energy consumption and carbon emission for tourism transport (Tang et al., 2015; Xiao et al., 2022; Zaman et al., 2017; Q; Zhang, 2022). With the concern of environmental protection and low carbon, the concept of low-carbon tourism is beginning to take hold (Changbo and Jingjing, 2011; Zha et al., 2019). Some studies began to use econometric models such as linear model, life cycle model (LCA) and input-output model to estimate tourism carbon footprint. In which, input-output model overcomes the limitations of linear model and the practical difficulties of LCA model and becomes the mainstream of research (Zhang, 2017).
In summary, current studies on international tourism carbon footprint mostly take countries (Le and Nguyen, 2021), regions (Liu et al., 2011; Selvanathan et al., 2021; Zhao et al., 2019), destinations (Cheng et al., 2013; Tang et al., 2015) as the subjects to discuss the legal development regarding tourism carbon emissions (TCEs). Although Lenzen et al. (2018) discussed global TCEs from 2009 to 2013, there has been a lack of discussion on the transfer rules of TCEs from the global or large regional scale in the past decade. However, there is relatively little research in this area at present, especially in the aspects of quantitative analysis, case studies and policy response. To sum up, there are many gaps and challenges in the research field of carbon footprint of international tourism and carbon transfer along the Belt and Road. To fill these gaps, this paper takes countries along the B&R as the research contexts and highlights the importance of tourism carbon footprint issues with respect to multiple influencing factors at multi-region scales. There are two innovations in this paper. Firstly, this paper introduces MRIO model to evaluate the carbon footprint of international tourism along the B&R, which enriches the literature on international tourism carbon footprint research. Secondly, this paper discusses the transfer mode of international tourism carbon footprint, analyzes the influencing factors of international tourism carbon footprint, and clarifies the transfer mechanism and influence mechanism of international tourism carbon footprint. It specifically adopts the input-output model, and uses multi-regional trade data from Eora global supply chain database (Lenzen et al., 2013), which contains the environmental and social Satellite accounts of 190 countries, to assess the international tourism carbon footprint and explore the tourism carbon footprint transfer. The following session reviews the existing research studies on carbon emissions and TCEs in the B&R regions, and followed by the methods and data collection details. The next section illustrates the results and analysis, and concludes with implications and policy recommendations.
2 Literature review
2.1 Carbon emissions in the B&R regions
China and the countries along the B&R are richly endowed with proven reserves of energy and resources, among which their carbon reserves accounted for 52.27% of the world’s standard coal in 2005 (Zhao et al., 2019). Rich energy reserves contribute to regional and even global prosperity by providing energy security for infrastructure construction which require substantial energy consumption (Zhang et al., 2017). However, many B&R countries lack renewable energy facilities, energy demands are largely met by fossil fuels. As one of the most carbon-intensive economies worldwide, China accounts for about 25% of global greenhouse gas emissions (GHGEs) (Dargusch, 2017) and the B&R regions account for 95% of the world’s net carbon exports (Han et al., 2018). In recent years, the geography of Global Supply Chains are shifting from China to successor developing countries in South Asia, Africa and Latin America by streamlining exports (Jiang and Green, 2017), which also indicates that the GHGEs generated in the production process are also shifting to less developing economies (Lambin et al., 2018; Mi et al., 2017; Pendrill et al., 2019). While the current embodied carbon flows are virtually all from BR regions to developed economies (Han et al., 2018). Thus, the operation and maintenance of the infrastructure in the B&R countries, the growth of energy-intensive industries under spatial shift of industrial capacity finally contribute to the considerable global carbon emissions (Zhang et al., 2017).
Some studies have evaluated the impact of BRI infrastructure projects on CO2 emissions, and specifically relevant studies show that investment in infrastructure in the B&R regions is beneficial to reduce transportation time and cost, and reduce CO2 emissions (De Soyres et al., 2019). 36 renewable energy projects in the B&R regions may reduce CO2 emissions by 240 Mt by 2030 (Gu and Zhou, 2020). For every 1% increase in Chinese investment in the region, CO2 emissions along the B&R regions will decrease by 0.04% in high-income countries and 0.02% in low-income countries (Su et al., 2022). In general, existing studies have evaluated the impact of infrastructure on CO2 emissions along the B&R countries and regions (De Soyres et al., 2020; Wang et al., 2020), in terms of CO2 emissions from power generation (Gu and Zhou, 2020), industrial carbon efficiency (Lu and Li, 2020), and the dynamic process of logistics efficiency (Zheng et al., 2020). Additionally, the changing trends of consumption and embodied emissions are also calculated in terms of consumption (Han et al., 2020; Hou et al., 2020). Methodologically speaking, majority studies estimated carbon emissions with the production-based accounting (PBA) analysis and consumption-based accounting (CBA) analysis (Chen et al., 2019; Chen et al., 2018; Kanemoto et al., 2016; Xu et al., 2020).
2.2 Tourism carbon emissions assessment
Tourism carbon emissions are usually studied in terms of tourism carbon footprint (TCF), and most of these studies focus on the carbon directly emitted from tourism activities and the carbon contained in the commodities purchased by tourists (Lenzen et al., 2018; Rosselló et al., 2024). The three most widely applied models to evaluate the carbon emissions of tourism industry are linear model (Kannan et al., 2012; Sun, 2016), life cycle model (LCA) (Cederberg et al., 2011; Jones and Kammen, 2011; Tang and Ge, 2018), and input-output model (Lenzen et al., 2018).
Linear model refers to a statistical model that connects all links related to the tourism industry through a certain process to calculate the carbon emissions of the tourism industry (Cooper and McCullough, 2021). Cooper and McCullough (2021) proposed a statistical model through stadium operation and travel, food, waste, and lodging of fans and teams, and believed that travel accounts for nearly 80% of the total carbon footprint. Zhiyong et al. (2021) discussed the carbon footprint of China’s inbound tourism, and concluded that the carbon footprint of inbound tourism in China increased from 5.623 million tons in 2007 to 10.8809 million tons in 2017, among which transportation, post, and telecommunications are the most significant contributors. Huang and Tang (2021) estimated the TCF of Heilongjiang Province from 2009 to 2018 by using TCF and the tourism carbon capacity model, and believed that the per capita TCF increased from 53.9 kg in 2009 to 116.0 kg in 2018.
Life cycle model uses LCA as a tool to evaluate energy consumption, which runs through the whole life cycle process of goods and services, that is, the emissions are allocated to the production of capital goods required in an industry (Cadarso et al., 2016). Tang et al. (2018) proposed a measurement model for energy and carbon emission efficiency of the destination tourism industry by combining the life cycle assessment theory and material flow analysis, and measured the energy efficiency and carbon emission efficiency of the tourism industry in Wulingyuan Scenic area from 1979 to 2015. They believed that with the evolution of the tourism life cycle stage, energy and carbon efficiency is improving in the tourism sector.
Input-output model (IOA) was proposed and refined by economist Leontief (1986), and has been widely used in resources and environment research since the 1970s (Kitzes, 2013). The essence of IOA method is to interconnect resource flows and environmental impacts to categories of intermediate use and final demand (Chen and Chen, 2011a; Chen and Chen, 2011b). As a well-established macro-level statistical method of TCF, it has been widely used in the study of TCF. For example, Sun (2016) proposed an analytical framework for national TCF and discussed its dynamic relationship with economic growth. Lenzen et al. (2018) used the input-output model to measure TCF of 160 countries in the world, and explored its transfer rule. They believed that, from 2009 to 2013, tourism global carbon footprint has increased from 3.9 to 4.5 GtCO2e. Tang and Ge, (2018) estimated that TCF in Shanghai accounted for 20.45% of the total carbon emission in 2012, indicating that tourism industry in Shanghai is not a low-carbon industry. There are also some derived input-output model, such as the Economic Input-Output Life Cycle Assessment (EIO-LCA) model (Suh, 2009), which uses a top-down economy-wide approach (Jones and Kammen, 2011), the life cycle assessment input-output (LCA-IO) model (Cadarso et al., 2016), the input-output life cycle model (Jones and Kammen, 2011), and the multi-regional input-output (MRIO) model (Davis and Caldeira, 2010; Han et al., 2018; Lin and Sun, 2010). The MRIO model has been employed to compute the spatial distribution of carbon intensities (Han et al., 2018) and the embodied regional carbon emission flows (Chen and Han, 2015), including embodied carbon flows along the B&R regions (Han et al., 2018). In general, input-output model has become a new macro method to compile TCF inventory. Relative to traditional bottom-up approaches, input-output models can improve the consistency of applications with the Tourism Satellite Accounts (TSAs) and the treatment of embedded emissions from imports, and contribute to the implementation of the Sustainable Development Goals (Sun et al., 2020). Given the vision of a community with a shared future for mankind and the complexity of regional tourism economies, MRIO analysis is implied to determine ITCF of countries in the B&R regions.
3 Methods and data
3.1 Multi-regional input–output model
Based on the MRIO model, detailed TSAs and CO2 emission data, this paper calculated the carbon footprint of international tourism along the B&R regions. The MRIO model use the multi-regional trade transfer matrix to calculate the CO2 emitted by tourists in the process of transportation and consumption of goods. The key to the MRIO model is the input-output table and major databases (Kitzes, 2013; Minx et al., 2009; Tukker and Dietzenbacher, 2013), including OECD (19 countries) (Yamano and Ahmad, 2006), WIOD (43 countries) (Dietzenbacher et al., 2013), EORA (190 countries) (Lenzen et al., 2012a; Lenzen et al., 2012b), GTAP (140 countries) (Narayanan, 2008), and EXIOPOL (43 countries) (Tukker et al., 2009). EORA database which features continuous time series and data reliability (Lenzen et al., 2013) is selected for this study. The input-output model can be expressed by the following Equation 1 (Chen and Han, 2015):
Where
Matrix T represents the absolute value of supply and demand between different sectors in each region. Since CO2 emission intensity between countries and sectors cannot be accurately compared, it is necessary to introduce direct consumption coefficient A, which is also known as direct consumption coefficient. Subsequently, the above equation can be converted Equation 2:
Here
Where I is the identity matrix,
Where
Therefore, the ITCF can be calculated by the following Equation 5:
Where,
3.2 Data collection
By March 2022, 149 countries had signed agreements with China on B&R Cooperation. A number of 65 countries are selected in this research, with total population of 4.4 billion, land area of about 50.7 million km2, and economy accounting for nearly 30% of global GDP.
4 Results and analysis
4.1 International tourism carbon footprint along the belt and road
The carbon footprint of international tourism in countries and regions along the B&R increased from 295.65 Mt in 2005 (Figure 1), namely, 37.82% of the world’s total, to 390.89 Mt in 2015, accounting for 40.74% of the world’s total, with an average annual growth rate of 3.22% (Table 1). In 2015, the top five countries (regions) along the B&R were China, Turkey, Russia, Thailand, and Hong Kong, China, were with their ITCF of 35.67, 32.79, 26.93, 24.74, and 21.98 Mt respectively (Figure 2). Ukraine and Malaysia were among the top five countries and regions along the B&R in terms of ITCF in 2005, and were replaced by Hong Kong, China and Thailand in 2015. The five countries and regions along the B&R with the smallest ITCF are Bhutan, Bangladesh, Moldova, Turkmenistan, and Tajikistan (Figure 2).
From 2005 to 2015, Bhutan, Kyrgyzstan, Myanmar, Vietnam, and Laos experienced the fastest growth in ITCF, with an annual growth rate of 85.88%, 70.42%, 49.67%, 42.22%, and 34.32%, respectively. In 2005, ITCF of China (43.52 Mt), Russia (20.62 Mt), and Turkey (20.01 Mt) were greater than 20 Mt. In 2015, ITCF of China (35.67 Mt), Turkey (32.79 Mt), Russia (26.93 Mt), Thailand (24.74 Mt), Hong Kong, China (21.98 Mt), and Malaysia (21.21 Mt) were greater than 20 Mt. Results show that the BRI has changed the tourism development distribution of the countries along the B&R regions (Table 2).
4.2 The inflow and outflow of international tourism carbon footprint along the B&R regions
From 2005 to 2015, the net outflow of ITCF along the B&R decreased from 0 to −0.08 Mt, and tourism carbon footprint of countries along the B&R was transferred to other countries and regions in the world. In 2005, the top five countries and regions with net inflow were Poland, Macao, Malaysia, Hong Kong, China, and Turkey respectively, which changed to Macao, Malaysia, Thailand, Hong Kong, China, and Turkey in 2015. These countries and regions received travel carbon transfers from the rest of the world (Table 2).
In 2005, China, Russia, Moldova, Belarus, India, and Iran were the top five countries in terms of ITCF outflow under the BRI, with the outflow volume of 21.57, 19.53, 11.83, 9.53, 6.07, and 5.88 Mt, respectively, accounting for 53.55% of the total outflow volume from countries and regions along the B&R. By 2015, the carbon footprint outflow of international tourism from China, Russia, India, Saudi Arabia and Belarus was 92.6, 17.52, 11.56, 11.13, and 8.97 Mt, respectively, accounting for 64.88% of the total outflow from countries along the B&R (Table 3). In general, the outflow of ITCF along the B&R regions was highly concentrated.
In 2005, China, Turkey, Russia, Ukraine, and Malaysia were the top five countries along the B&R regions in terms of ITCF inflow, which were 16.8, 8.95, 8.25, 7.51, and 7.15 Mt, respectively, accounting for 38.01% of the total inflow of countries along the B&R regions. By 2015, Turkey, China, Russia, Thailand, and Hong Kong, China ranked among top five in terms of ITCF inflows, which were 18.92, 14.89, 14.89, 14.21, and 12.65 Mt, respectively, accounting for 34.57% of the total outflow of countries along the B&R regions (Table 3). In general, the inflow of ITCF along the B&R regions was relatively dispersed.
4.3 Factors influencing international tourism carbon footprint along the B&R regions
To further examine the influencing factors of ITCF along the B&R regions, a panel model is adopted in this paper. According to the report of the World Bank’s national income data, the samples are divided into the overall sample, the sample of low- and middle-income countries, high- and middle-income countries, and high-income countries. Low-income samples were not included in the analysis due to lack of data.
The results show that ITCF along the B&R regions is affected by per capita income. Despite the heterogeneity of per capita income among countries, the influence coefficient of per capita income of countries and regions along the B&R regions on the ITCF is maintained between 0.307 and 0.387. The influence coefficient of urbanization rate on the ITCF is 0.439, and the impact on low- and middle-income countries is the largest (0.820). For high-income countries, there is a negative effect, with an influence coefficient of 0.401. The influence coefficient of the number of tourists on the ITCF is maintained between 0.373 and 0.606, and the impact on high-income countries is the largest (0.606). The influence coefficient of air passenger volume on the ITCF is 0.118, among which the influence of air passenger volume on high- and middle-income countries is not significant, and its influence on low-income countries is the largest, with an impact coefficient of 0.318 (Table 4).
4.4 The transfer of international tourism carbon footprint along the B&R regions
From 2005 to 2015, the carbon emission structure of China’s international tourism became more diversified. In 2005, China’s ITCF was mainly transferred from Russia, accounting for 33.56% of the top six countries in China’s international tourism carbon emissions. In 2015, the carbon footprint of China’s international tourism was mainly transferred from Russia, India, Iran, Saudi Arabia and Albania, accounting for 12.06%–27.01%. Russia accounted for 27.01%, with a decrease of 6.55%. This suggests China’s international tourism carbon emissions more diversified among countries along the B&R (Figure 3).
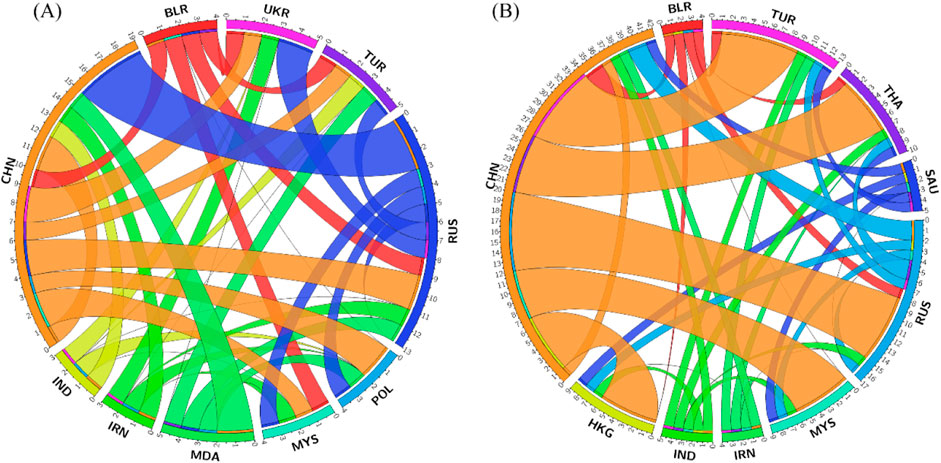
Figure 3. Carbon footprint transfer of international tourism in countries and regions along the B&R (2015). (A) for 2005 and (B) for 2015.
5 Discussion
The results of the study show that the BRI has significantly increased the number of inbound tourists from countries along the route, which has been confirmed by Li et al. (2020). Results also show that the tourism carbon footprint has shifted to the rest of the world, which can be attributed to three reasons.
First, tourism cooperation between countries has increased. After the BRI was put forward, countries adopted visa-free (Han et al., 2018), jointly held tourism years, established tourism promotion alliances and other measures (Rosselló et al., 2024; Wang et al., 2020), which enhanced the visibility and accessibility of countries along the B&R, attracted more international tourists, and promoted the transfer of international tourism carbon footprint to other parts of the world. For example, China has reached visa-free agreements with 57 countries to jointly build the B&R, jointly host the Tourism Year, and established cooperative alliance with many other B&R countries, such as the New Silk Road Economic Belt and the 21st Century Maritime Silk Road and the Silk Road Tourism Marketing Alliance, the Maritime Silk Road Tourism Promotion Alliance, and the Great Tea Road International Tourism Alliance.
Second, infrastructure connectivity is a top priority for the BRI. A series of economic corridors have been built along the route, such as New Eurasian Land Bridge, China-Mongolia-Russia, China-Central Asia-West Asia, China-Indochina Peninsula, China-Pakistan, and Bangladesh-China-India-Myanmar economic corridors (Li et al., 2020). These economic corridors have boosted cultural exchanges and incomes, stimulating trade and tourism between countries (Irshad, 2015).
Third, the BRI has increased investment and reduced poverty in countries along the route. According to a World Bank study, B&R investments could help lift more than 34 million people out of moderate poverty, 29.4 million of whom live in countries and regions along the routes. Driven by the BRI, foreign economic and trade cooperation among countries along the B&R has continued to grow, and the scale of Chinese investment has increased year by year, employing hundreds of thousands of local workers (Li et al., 2020). With the support of Chinese capital and technology, the industrial structure of the countries along the route has become more complete, and the advantageous production capacity has been shown. At the same time, the digital B&R cooperation has also blossomed in the countries along the route, and the digital trade dominated by e-commerce has been booming, promoting the development of business tourism in various countries (De Soyres et al., 2019).
6 Conclusion
In this study, the MRIO model (Chen and Han, 2015) is used to estimate the ITCF along the B&R, its inflow and outflow rule and transfer rule are also analyzed, and the panel model is used to discuss the influencing factors of the ITCF. Results show that the ITCF along the B&R region accounted for 37.82%–40.74% of the global total between 2005 and 2015.
Results show that the BRI has changed the ITCF along the B&R regions and the ITCF increased continuously from 2005 to 2015, with an average annual growth rate of 3.22%, indicating the pressure of tourism development on climate change mitigation. Tourism development distribution of the countries along the B&R region has also been changed with China (35.67 Mt), Turkey (32.79 Mt), Russia (26.93 Mt), Thailand (24.74 Mt) and Hong Kong, China (21.98 Mt) being the top five countries (regions) along the B&R in terms of ITCF in 2015. In general, the inflow of ITCF along the B&R regions was relatively dispersed, the outflow of ITCF along the B&R regions was highly concentrated. Macao, Malaysia, Thailand, Hong Kong, China and Turkey were the countries/regions with net ITCF inflows in 2015.
There is little difference in the impact of income level on ITCF among countries/regions along the B&R, ranging from 0.307 to 0.387. Urbanization rate is an important factor affecting the ITCF (0.439), especially in low-income countries, which is as high as 0.820, indicating that the higher the urbanization rate of countries, the larger the ITCF and the higher the carbon emissions of tourism industry. The influence coefficient of air passenger volume on ITCF is 0.118.
The research reveals that countries and regions along the B&R should consider the carbon burden brought by the development of the tourism industry when formulating tourism industry development strategies. In order to meet the challenge of climate change mitigation brought by carbon emissions from the tourism industry, tourists should be encouraged to choose more environmentally friendly ways to travel. Tourist destinations are advised to increase green public transport systems. In addition, referring to some international deals, such as the European Green Deal (Rosselló et al., 2024), relevant countries and regions are encouraged to take an active role in the tourism carbon footprint transfer in the B&R region, and jointly promote the development of green and low-carbon tourism by formulating policies, providing financial support, strengthening publicity and education, developing international tourism carbon tax and tourism carbon trading systems and promoting international cooperation to limit the future growth of tourism-related carbon emissions in the B&R region.
Generally speaking, most of these regions are developing countries, the analysis of the carbon emission rules of tourism industry in this region is of great significance for understanding the mitigation of climate change in the tourism industry. However, due to the time-lag of input-output table, this paper only focused on the carbon footprint of international tourism along the B&R regions from 2005 to 2015. How to simulate the future carbon footprint of international tourism through historical rules becomes the focus of future research. The MRIO model in this study also has some limitations. First, there is a time lag of EORA database generated by MRIO model; Second, the MRIO model is still a statistical model that complies with accounting standards, and is mostly used for macro-scale research. In the future, mixed experiments should be adopted to explore the scientific issues of the carbon footprint of international tourism. In addition, this paper mainly focuses on the carbon footprint of international tourists, and the carbon footprint of domestic tourists has not been studied due to the missing data. Relevant scientific issues and rules of domestic tourism carbon footprint should be explored from the perspective of domestic tourists.
Data availability statement
The original contributions presented in the study are included in the article/supplementary material, further inquiries can be directed to the corresponding author.
Author contributions
HS: Conceptualization, Data curation, Funding acquisition, Methodology, Supervision, Writing–original draft, Writing–review and editing. QL: Methodology, Formal analysis, Resources, Writing–review and editing. RY: Data curation, Formal Analysis, Software, Writing–original draft. TL: Formal Analysis, Supervision, Visualization, Writing–review and editing. NC: Conceptualization, Project administration, Supervision, Writing–review and editing. YR: Supervision, Visualization, Writing–review and editing.
Funding
The author(s) declare that financial support was received for the research, authorship, and/or publication of this article. This work was supported by the Southwest Minzu University Research Startup Funds (Grant No. RQD2021038).
Conflict of interest
The authors declare that the research was conducted in the absence of any commercial or financial relationships that could be construed as a potential conflict of interest.
Publisher’s note
All claims expressed in this article are solely those of the authors and do not necessarily represent those of their affiliated organizations, or those of the publisher, the editors and the reviewers. Any product that may be evaluated in this article, or claim that may be made by its manufacturer, is not guaranteed or endorsed by the publisher.
References
Adedoyin, F. F., and Bekun, F. V. (2020). RETRACTED ARTICLE: modelling the interaction between tourism, energy consumption, pollutant emissions and urbanization: renewed evidence from panel VAR. Environ. Sci. Pollut. Res. 27 (31), 38881–38900. doi:10.1007/s11356-020-09869-9
Cadarso, M. Á., Gomez, N., López, L. A., and Tobarra, M. Á. (2016). Calculating tourism's carbon footprint: measuring the impact of investments. J. Clean. Prod. 111, 529–537. doi:10.1016/j.jclepro.2014.09.019
Cederberg, C., Persson, U. M., Neovius, K., Molander, S., and Clift, R. (2011). Including carbon emissions from deforestation in the carbon footprint of Brazilian beef. New York, NY: ACS Publications.
Changbo, S., and Jingjing, P. (2011). Construction of low-carbon tourist attractions based on low-carbon economy. Energy Procedia 5, 759–762. doi:10.1016/j.egypro.2011.03.133
Chen, G., and Han, M. (2015). Global supply chain of arable land use: production-based and consumption-based trade imbalance. Land Use Policy 49, 118–130. doi:10.1016/j.landusepol.2015.07.023
Chen, Y., Li, X., Liu, X., Zhang, Y., and Huang, M. (2019). Quantifying the teleconnections between local consumption and domestic land uses in China. Landsc. Urban Plan. 187, 60–69. doi:10.1016/j.landurbplan.2019.03.011
Chen, Z., and Chen, G. (2011a). Embodied carbon dioxide emission at supra-national scale: a coalition analysis for G7, BRIC, and the rest of the world. Energy Policy 39 (5), 2899–2909. doi:10.1016/j.enpol.2011.02.068
Chen, Z., and Chen, G. (2011b). An overview of energy consumption of the globalized world economy. Energy Policy 39 (10), 5920–5928. doi:10.1016/j.enpol.2011.06.046
Chen, Z., Ohshita, S., Lenzen, M., Wiedmann, T., Jiborn, M., Chen, B., et al. (2018). Consumption-based greenhouse gas emissions accounting with capital stock change highlights dynamics of fast-developing countries. Nat. Commun. 9 (1), 3581–3589. doi:10.1038/s41467-018-05905-y
Cheng, Q., Su, B., and Tan, J. (2013). Developing an evaluation index system for low-carbon tourist attractions in China–A case study examining the Xixi wetland. Tour. Manag. 36, 314–320. doi:10.1016/j.tourman.2012.10.019
Cooper, J., and McCullough, B. P. (2021). Bracketing sustainability: carbon footprinting March Madness to rethink sustainable tourism approaches and measurements. J. Clean. Prod. 318, 128475. doi:10.1016/j.jclepro.2021.128475
Dargusch, P. (2017). China must lead on emissions trading. Science 357 (6356), 1106–1107. doi:10.1126/science.aap7960
Davis, S. J., and Caldeira, K. (2010). Consumption-based accounting of CO2 emissions. Proc. Natl. Acad. Sci. 107 (12), 5687–5692. doi:10.1073/pnas.0906974107
De Soyres, F., Mulabdic, A., Murray, S., Rocha, N., and Ruta, M. (2019). How much will the Belt and Road Initiative reduce trade costs? Int. Econ. 159, 151–164. doi:10.1016/j.inteco.2019.07.003
De Soyres, F., Mulabdic, A., and Ruta, M. (2020). Common transport infrastructure: a quantitative model and estimates from the Belt and Road Initiative. J. Dev. Econ. 143, 102415. doi:10.1016/j.jdeveco.2019.102415
Dietzenbacher, E., Los, B., Stehrer, R., Timmer, M., and De Vries, G. (2013). The construction of world input–output tables in the WIOD project. Econ. Syst. Res. 25 (1), 71–98. doi:10.1080/09535314.2012.761180
Dogan, E., Tzeremes, P., and Altinoz, B. (2020). Revisiting the nexus among carbon emissions, energy consumption and total factor productivity in African countries: new evidence from nonparametric quantile causality approach. Heliyon 6 (3), e03566. doi:10.1016/j.heliyon.2020.e03566
Gössling, S. (2002). Global environmental consequences of tourism. Glob. Environ. change 12 (4), 283–302. doi:10.1016/s0959-3780(02)00044-4
Gössling, S., Garrod, B., Aall, C., Hille, J., and Peeters, P. (2011). Food management in tourism: reducing tourism’s carbon ‘foodprint. Tour. Manag. 32 (3), 534–543. doi:10.1016/j.tourman.2010.04.006
Gu, A., and Zhou, X. (2020). Emission reduction effects of the green energy investment projects of China in belt and road initiative countries. Ecosyst. Health Sustain. 6 (1), 1747947. doi:10.1080/20964129.2020.1747947
Han, M., Lao, J., Yao, Q., Zhang, B., and Meng, J. (2020). Carbon inequality and economic development across the Belt and Road regions. J. Environ. Manag. 262, 110250. doi:10.1016/j.jenvman.2020.110250
Han, M., Yao, Q., Liu, W., and Dunford, M. (2018). Tracking embodied carbon flows in the Belt and Road regions. J. Geogr. Sci. 28 (9), 1263–1274. doi:10.1007/s11442-018-1524-7
Hou, J., Deng, X., Springer, C. H., and Teng, F. (2020). A global analysis of CO2 and non-CO2 GHG emissions embodied in trade with Belt and Road Initiative countries. Ecosyst. Health Sustain. 6 (1), 1761888. doi:10.1080/20964129.2020.1761888
Huang, T., and Tang, Z. (2021). Estimation of tourism carbon footprint and carbon capacity. Int. J. Low-Carbon Technol. 16 (3), 1040–1046. doi:10.1093/ijlct/ctab026
Inglesi-Lotz, R., and Dogan, E. (2018). The role of renewable versus non-renewable energy to the level of CO2 emissions a panel analysis of sub-Saharan Africa’s Βig 10 electricity generators. Renew. Energy 123, 36–43. doi:10.1016/j.renene.2018.02.041
Irshad, M. S. (2015). One belt and one road: dose China-Pakistan economic corridor benefit for Pakistan's economy? J. Econ. Sustain. Dev. 6 (24).
Jiang, X., and Green, C. (2017). The impact on global greenhouse gas emissions of geographic shifts in global supply chains. Ecol. Econ. 139, 102–114. doi:10.1016/j.ecolecon.2017.04.027
Jones, C. M., and Kammen, D. M. (2011). Quantifying carbon footprint reduction opportunities for US households and communities. Environ. Sci. & Technol. 45 (9), 4088–4095. doi:10.1021/es102221h
Kanemoto, K., Moran, D., and Hertwich, E. G. (2016). Mapping the carbon footprint of nations. Environ. Sci. & Technol. 50 (19), 10512–10517. doi:10.1021/acs.est.6b03227
Kannan, D., Diabat, A., Alrefaei, M., Govindan, K., and Yong, G. (2012). A carbon footprint based reverse logistics network design model. Resour. conservation Recycl. 67, 75–79. doi:10.1016/j.resconrec.2012.03.005
Kitzes, J. (2013). An introduction to environmentally-extended input-output analysis. Resources 2 (4), 489–503. doi:10.3390/resources2040489
Lambin, E. F., Gibbs, H. K., Heilmayr, R., Carlson, K. M., Fleck, L. C., Garrett, R. D., et al. (2018). The role of supply-chain initiatives in reducing deforestation. Nat. Clim. change 8 (2), 109–116. doi:10.1038/s41558-017-0061-1
Le, T.-H., and Nguyen, C. P. (2021). The impact of tourism on carbon dioxide emissions: insights from 95 countries. Appl. Econ. 53 (2), 235–261. doi:10.1080/00036846.2020.1804051
Lenzen, M., Kanemoto, K., Moran, D., and Geschke, A. (2012a). Mapping the structure of the world economy. Environ. Sci. & Technol. 46 (15), 8374–8381. doi:10.1021/es300171x
Lenzen, M., Moran, D., Kanemoto, K., Foran, B., Lobefaro, L., and Geschke, A. (2012b). International trade drives biodiversity threats in developing nations. Nature 486 (7401), 109–112. doi:10.1038/nature11145
Lenzen, M., Moran, D., Kanemoto, K., and Geschke, A. (2013). Building Eora: a global multi-region input–output database at high country and sector resolution. Econ. Syst. Res. 25 (1), 20–49. doi:10.1080/09535314.2013.769938
Lenzen, M., Sun, Y.-Y., Faturay, F., Ting, Y.-P., Geschke, A., and Malik, A. (2018). The carbon footprint of global tourism. Nat. Clim. change 8 (6), 522–528. doi:10.1038/s41558-018-0141-x
Li, T., Shi, H., Yang, Z., and Ren, Y. (2020). Does the belt and road initiative boost tourism economy? Asia Pac. J. Tour. Res. 25 (3), 311–322. doi:10.1080/10941665.2019.1708758
Lin, B., and Sun, C. (2010). Evaluating carbon dioxide emissions in international trade of China. Energy Policy 38 (1), 613–621. doi:10.1016/j.enpol.2009.10.014
Liu, J., Feng, T., and Yang, X. (2011). The energy requirements and carbon dioxide emissions of tourism industry of Western China: a case of Chengdu city. Renew. Sustain. Energy Rev. 15 (6), 2887–2894. doi:10.1016/j.rser.2011.02.029
Lu, Y., and Li, M. (2020). Industrial carbon emission efficiency in the yangtze river economic belt and its influencing factors. Int. J. Des. & Nat. Ecodynamics 15 (1), 25–32. doi:10.18280/ijdne.150104
Mi, Z., Meng, J., Guan, D., Shan, Y., Song, M., Wei, Y.-M., et al. (2017). Chinese CO2 emission flows have reversed since the global financial crisis. Nat. Commun. 8 (1), 1712. doi:10.1038/s41467-017-01820-w
Minx, J. C., Wiedmann, T., Wood, R., Peters, G. P., Lenzen, M., Owen, A., et al. (2009). Input–output analysis and carbon footprinting: an overview of applications. Econ. Syst. Res. 21 (3), 187–216. doi:10.1080/09535310903541298
Moutinho, V., Madaleno, M., Inglesi-Lotz, R., and Dogan, E. (2018). Factors affecting CO2 emissions in top countries on renewable energies: a LMDI decomposition application. Renew. Sustain. Energy Rev. 90, 605–622. doi:10.1016/j.rser.2018.02.009
Narayanan, b. G. (2008). Global trade, assistance, and production: the GTAP 7 data base: center for global trade analysis.
Nepal, R., Al Irsyad, M. I., and Nepal, S. K. (2019). Tourist arrivals, energy consumption and pollutant emissions in a developing economy–implications for sustainable tourism. Tour. Manag. 72, 145–154. doi:10.1016/j.tourman.2018.08.025
O’Trakoun, J. (2018). China’s belt and road initiative and regional perceptions of China. Bus. Econ. 53 (1), 17–24. doi:10.1057/s11369-017-0062-0
Pacheco Pardo, R. (2018). Europe’s financial security and Chinese economic statecraft: the case of the Belt and Road Initiative. Asia Eur. J. 16 (3), 237–250. doi:10.1007/s10308-018-0511-z
Pendrill, F., Persson, U. M., Godar, J., and Kastner, T. (2019). Deforestation displaced: trade in forest-risk commodities and the prospects for a global forest transition. Environ. Res. Lett. 14 (5), 055003. doi:10.1088/1748-9326/ab0d41
Rosselló, L. B. F., Bestard, A. B., and Font, A. R. (2024). Is carbon footprint reduction always preferred over offsetting? An analysis of tourists' preferences in the Mallorca region. Econ. Analysis Policy 81, 1371–1381. doi:10.1016/j.eap.2024.02.018
Selvanathan, E. A., Jayasinghe, M., and Selvanathan, S. (2021). Dynamic modelling of inter-relationship between tourism, energy consumption, 2 emissions and economic growth in South Asia. Int. J. Tour. Res. 23 (4), 597–610. doi:10.1002/jtr.2429
Su, X., Li, Y., Fang, K., and Long, Y. (2022). Does China's direct investment in “Belt and Road Initiative” countries decrease their carbon dioxide emissions? J. Clean. Prod. 339, 130543. doi:10.1016/j.jclepro.2022.130543
Suh, S. (2009). “Developing the sectoral environmental database for input-output analysis: comprehensive environmental data archive of the US,” in Handbook of input-output economics in industrial ecology (Springer), 689–712.
Sun, Y.-Y. (2016). Decomposition of tourism greenhouse gas emissions: revealing the dynamics between tourism economic growth, technological efficiency, and carbon emissions. Tour. Manag. 55, 326–336. doi:10.1016/j.tourman.2016.02.014
Sun, Y.-Y., Cadarso, M. A., and Driml, S. (2020). Tourism carbon footprint inventories: a review of the environmentally extended input-output approach. Ann. Tour. Res. 82, 102928. doi:10.1016/j.annals.2020.102928
Tang, C., Zhong, L., Fan, W., and Cheng, S. (2015) “Energy consumption and carbon emission for tourism transport in W orld H eritage S ites: a case of the W ulingyuan area in C hina,” in Paper presented at the natural resources forum.
Tang, C., Zhong, L., and Jiang, Q. o. (2018). Energy efficiency and carbon efficiency of tourism industry in destination. Energy Effic. 11 (3), 539–558. doi:10.1007/s12053-017-9598-0
Tang, M., and Ge, S. (2018). Accounting for carbon emissions associated with tourism-related consumption. Tour. Econ. 24 (5), 510–525. doi:10.1177/1354816618754691
Torres-Delgado, A., Font, X., and Oliver-Solà, J. (2024). Self-defence against carbon footprint evidence: how employees of destination management and marketing organisations cope with conflicting environmental and economic data. Ann. Tour. Res. 104, 103722. doi:10.1016/j.annals.2023.103722
Tukker, A., and Dietzenbacher, E. (2013). Global multiregional input–output frameworks: an introduction and outlook. Econ. Syst. Res. 25 (1), 1–19. doi:10.1080/09535314.2012.761179
Tukker, A., Poliakov, E., Heijungs, R., Hawkins, T., Neuwahl, F., Rueda-Cantuche, J. M., et al. (2009). Towards a global multi-regional environmentally extended input–output database. Ecol. Econ. 68 (7), 1928–1937. doi:10.1016/j.ecolecon.2008.11.010
Wang, C., Lim, M. K., Zhang, X., Zhao, L., and Lee, P. T.-W. (2020). Railway and road infrastructure in the Belt and Road Initiative countries: estimating the impact of transport infrastructure on economic growth. Transp. Res. Part A Policy Pract. 134, 288–307. doi:10.1016/j.tra.2020.02.009
Wen, L., and Shao, H. (2019). Analysis of influencing factors of the CO2 emissions in China: nonparametric additive regression approach. Sci. Total Environ. 694, 133724. doi:10.1016/j.scitotenv.2019.133724
Xiao, F., Pang, Z., Yan, D., Kong, Y., and Yang, F. (2022). How does transportation infrastructure affect urban carbon emissions? an empirical study based on 286 cities in China. Environ. Sci. Pollut. Res. 30, 10624–10642. doi:10.1007/s11356-022-22866-4
Xu, X., Zhang, Y., and Chen, Y. (2020). Projecting China's future water footprint under the shared socio-economic pathways. J. Environ. Manag. 260, 110102. doi:10.1016/j.jenvman.2020.110102
Yang, Y., and Guo, X. (2024). Characteristics and drivers of changes in the carbon footprint of urban tourism in the post-COVID-19 era: a case study of Xi'an. J. Clean. Prod. 434, 140015. doi:10.1016/j.jclepro.2023.140015
Zaman, K., Moemen, M. A.-e., and Islam, T. (2017). Dynamic linkages between tourism transportation expenditures, carbon dioxide emission, energy consumption and growth factors: evidence from the transition economies. Curr. Issues Tour. 20 (16), 1720–1735. doi:10.1080/13683500.2015.1135107
Zameer, H., and Wang, Y. (2018). Energy production system optimization: evidence from Pakistan. Renew. Sustain. Energy Rev. 82, 886–893. doi:10.1016/j.rser.2017.09.089
Zha, J., He, L., Liu, Y., and Shao, Y. (2019). Evaluation on development efficiency of low-carbon tourism economy: a case study of Hubei Province, China. Socio-Economic Plan. Sci. 66, 47–57. doi:10.1016/j.seps.2018.07.003
Zhai, F. (2018). China’s belt and road initiative: a preliminary quantitative assessment. J. Asian Econ. 55, 84–92. doi:10.1016/j.asieco.2017.12.006
Zhang, J. (2017). Evaluating regional low-carbon tourism strategies using the fuzzy Delphi-analytic network process approach. J. Clean. Prod. 141, 409–419. doi:10.1016/j.jclepro.2016.09.122
Zhang, J. (2019). Oil and gas trade between China and countries and regions along the ‘Belt and Road’: a panoramic perspective. Energy Policy 129, 1111–1120. doi:10.1016/j.enpol.2019.03.020
Zhang, N., Liu, Z., Zheng, X., and Xue, J. (2017). Carbon footprint of China's belt and road. Science 357 (6356), 1107. doi:10.1126/science.aao6621
Zhang, Q. (2022). Investigating the impact of transportation infrastructure and tourism on carbon dioxide emissions in China. J. Environ. & Public Health 2022, 8421756. doi:10.1155/2022/8421756
Zhao, Y., Liu, X., Wang, S., and Ge, Y. (2019). Energy relations between China and the countries along the Belt and Road: an analysis of the distribution of energy resources and interdependence relationships. Renew. Sustain. Energy Rev. 107, 133–144. doi:10.1016/j.rser.2019.03.007
Zheng, W., Xu, X., and Wang, H. (2020). Regional logistics efficiency and performance in China along the Belt and Road Initiative: the analysis of integrated DEA and hierarchical regression with carbon constraint. J. Clean. Prod. 276, 123649. doi:10.1016/j.jclepro.2020.123649
Keywords: the belt and road, MRIO model, international tourism carbon footprint, tourism development, global carbon emission
Citation: Shi H, Liu Q, Yan R, Li T, Chen NC and Ren Y (2024) International tourism carbon footprint and carbon transfer along the belt and road regions. Front. Environ. Sci. 12:1440510. doi: 10.3389/fenvs.2024.1440510
Received: 29 May 2024; Accepted: 13 November 2024;
Published: 28 November 2024.
Edited by:
Zhen Wang, Huazhong Agricultural University, ChinaReviewed by:
Elżbieta Ryńska, Warsaw University of Technology, PolandDongmei Tang, East China Normal University, China
Copyright © 2024 Shi, Liu, Yan, Li, Chen and Ren. This is an open-access article distributed under the terms of the Creative Commons Attribution License (CC BY). The use, distribution or reproduction in other forums is permitted, provided the original author(s) and the copyright owner(s) are credited and that the original publication in this journal is cited, in accordance with accepted academic practice. No use, distribution or reproduction is permitted which does not comply with these terms.
*Correspondence: Taohong Li, NDE1MDZAc2ljYXUuZWR1LmNu