- 1School of Accounting, Dongbei University of Finance and Economics, Dalian, China
- 2School of Environment, Tsinghua University, Beijing, China
Firms’ green R&D and innovation directly impact the national economy’s green and high-quality development. However, data resources play a significant role in altering traditional development models and promoting sustainable economic growth. Government data openness (GDO) offers a vital opportunity for the widespread transmission of data value. Existing literature has not yet examined whether GDO can intrinsically affect the green R&D and innovation of micro-level firms. In this study, we examine the impact of GDO on corporate green innovation using data from A-share industrial listed companies in China spanning 2007–2022 and employing the difference-in-differences (DID) method. The results indicate that GDO significantly promotes corporate green innovation. Mechanism tests reveal that GDO fosters corporate green innovation through channels such as enhancing corporate risk-taking, alleviating financing constraints, and optimizing the business environment. Further examination shows that improvements in the quality of GDO significantly enhance corporate green innovation. Moreover, the green effect of GDO is comprehensive and does not vary with the classification of green innovation types. According to the heterogeneity analysis, when management’s green cognition is higher, the firm is younger, environmental regulations are more stringent, and intellectual property is less protected, the green effect of GDO is more noticeable. This paper provides insights for promoting high-quality GDO, advancing green innovation and development in emerging market countries.
1 Introduction
Following 45 years of rapid growth, China’s social productivity has significantly increased. In 1978, China’s GDP was less than 370 billion RMB, whereas by 2023, it has surpassed 120 trillion RMB, growing at an average annual rate close to double digits. However, it is worth noting that China’s progress has historically been accompanied by an extensive development model. Throughout this process, China has experienced considerable environmental degradation as a trade-off for development (Wang et al., 2022). Therefore, this development model is difficult to sustain in the long term in China and has significant drawbacks. In response to the challenges posed by environmental pollution on various aspects of China, China’s government has moved quickly to realign the economy in a sustainable and green direction (Lin and Ma, 2022; Feng et al., 2023). Specifically, the Chinese government has not only made solemn commitments to achieve dual carbon targets—carbon peaking and carbon neutrality—but has also repeatedly emphasized that development should adhere to the theories of “innovation, coordination, green, openness, and sharing” in numerous major domestic meetings and reports. Additionally, the government has called for the establishment of a modern environmental governance system, the promotion of green consumption, the coordination of industrial structure adjustments, and the acceleration of green transformation in development methods. In prior studies, green innovation, noted for its dual beneficial externalities of technology diffusion and sustainable development (Feng et al., 2023), has been widely regarded as an effective path to achieving green economic development (Lin and Ma, 2022). The adoption of green innovation can integrate environmental technologies into corporate production processes, product design, and organizational operations, reducing unnecessary production inputs and optimizing emissions, thereby ensuring that companies align with the green concept in their operations (Xie et al., 2024). Thus, in China’s green development path, how to encourage the quick and efficient growth of green innovation is a crucial strategic concern.
Meanwhile, with continuous technological advancements, the digital economy has gained significant development opportunities worldwide (Ma and Zhu, 2022). The Chinese government places great importance on the long-term development and planning of the digital economy and regards it as a key driver for transforming the internal structure of the economy. As a new type of production factor, data is an indispensable strategic resource for driving the sustained advancement of the digital economy. The government of China, in the “Opinions of the Central Committee of the Communist Party of China and the State Council on Improving the Market Allocation Mechanism of Production Factors,” has, for the first time, equated data elements with traditional factors such as land, labor, capital, and technology. Subsequently, follow-up policy documents emphasize leveraging the advantage of massive data scales, unlocking the potential of data elements, optimizing and expanding the digital economy, and strengthening the development of new drivers of economic growth. However, the government is the largest holder and creator of data in society (Dawes, 2012). Government data encompasses a broad range of areas, including production data such as geographical, energy, and resource exploration; market data such as population, consumption, and industry; and administrative service data such as fiscal, judicial, and credit services (Tan et al., 2022; Peng, 2023). These data resources span numerous fields and, with the endorsement of the government, possess high quality (Attard et al., 2015). This high-quality data can accelerate the formation of new production relations compatible with data elements across society, thereby creating new development prospects for the digital economy. Consequently, a significant body of literature in previous studies has discussed the potential impacts of releasing vast amounts of internal government data. Some research has pointed out that government data openness (GDO) increases government transparency, assists public supervision of government operations and decisions, and strengthens accountability (Kassen, 2013; Yan et al., 2023). GDO stimulates social entities’ enthusiasm, encouraging them to integrate, evaluate, and leverage government data for their own development. Ultimately, this promotes innovation, supports the emergence of novel goods and services, and produces significant economic and social benefits (Yan et al., 2023; Zhou et al., 2023). It is evident that GDO has a significant value creation effect. Against the backdrop of China’s commitment to green and sustainable development, can GDO also impart positive value to green innovation? If this impact is present, through what mechanisms does GDO influence corporate green innovation? Discussing the aforementioned issues can not only expand our understanding of the green effect of GDO but also provide valuable insights for the long-term advancement of the green economy in China and other emerging markets.
Shanghai took the lead in 2012 by launching a GDO platform at the provincial level. In the following years, the government released the “Action Plan for Promoting Big Data Development,” which called for accelerating government data openness and sharing, promoting resource integration, fostering industrial innovation and development, and supporting economic transformation. This marked the beginning of the nationwide launch of local GDO platforms. It is noteworthy that the launch of these platforms across various regions in China did not occur simultaneously but rather in different years. In this setting, the economic consequences of the openness of government data could be explored in a quasi-natural experiment. According to statistics, by 2022, 25 provincial-level GDO platforms (including provinces, autonomous regions, and municipalities) have been launched in China.
We examine the potential green effect of GDO by analyzing local governments launching open data platforms. According to the results, GDO significantly encourages green innovation in corporations. According to mechanism tests, GDO fosters corporate green innovation through channels such as enhancing corporate risk-taking, alleviating financing constraints, and optimizing the business environment. Further tests indicate that improvements in the quality of GDO significantly enhance corporate green innovation. Moreover, the green effect of GDO is comprehensive and does not vary with the classification of green innovation types. Based on the heterogeneity analysis, the green effect of GDO is more pronounced when management’s green cognition is higher, firm age is lower, environmental regulations are stricter, and intellectual property protection is weaker. The logic of this paper is illustrated below (Figure 1):
Our research makes marginal contributions in the four aspects:
First, this study broadens the scope of the discussion on the economic consequences of GDO. Previous research has primarily examined the value creation of GDO in areas such as public participation in decision-making and corporate performance (Kassen, 2013; Jetzek et al., 2014; Zhou et al., 2023). However, in the macro context of green and sustainable development, no research has concentrated on the green effect of GDO, especially at the level of corporate green innovation. By integrating GDO and corporate green innovation into a unified framework, this study addresses the limitations of previous research and provides the first empirical evidence that government data elements can effectively promote the greening of micro-level entities. At the same time, unlike previous studies based on market big data, this paper highlights the green value of government data elements with a public nature. It also enhances the understanding of the green effect of GDO among stakeholders such as governments, corporate management, and investors, providing empirical support for broader data openness initiatives. In addition, this paper employs the fixed-effects Poisson model, as recommended by existing studies (Campbell and Mau, 2021; Wang et al., 2023a; Gao et al., 2024), which is more suitable for count variables such as patent data. This approach enhances the precision of evaluating the green effects of GDO and strengthens the robustness of the study’s conclusions.
Second, we further investigate the mechanism through which GDO influences corporate green innovation. The findings indicate that enhancing corporate risk-taking, alleviating financing constraints, and optimizing the business environment are key channels through which GDO exerts its green effect. This conclusion unpacks the “black box” of how GDO’s green effect is realized, revealing the specific transmission mechanism by which government data elements drive the greening of micro-level entities. It also expands the theoretical framework of the existing literature. Furthermore, it provides guidance for policymakers to refine data openness policies, maximizing the spread of GDO’s green effect. Finally, in the discussion of mechanisms, this paper incorporates Schumpeter’s Entrepreneurship Theory, Resource Dependence Theory, and Transaction Cost Theory as key theoretical foundations. The findings of this study further validate the explanatory power of these theories within the context of discussing the green effect of GDO. Moreover, the results expand their theoretical applicability and enrich our understanding of their practical application scenarios.
Third, this paper offers a fresh viewpoint on how to promote corporate green innovation. Prior studies have found that various factors at the corporate level, such as executives’ green experiences and corporate digital transformation, as well as external factors like environmental taxes, influence corporate green innovation (Zhang and Zhang, 2023; Liu et al., 2023; Wang et al., 2023b). Nevertheless, there is no research investigating the potential impact of government data resource supplementation on corporate green innovation from the perspective of GDO. Therefore, our research not only fills the gap in the literature regarding the influence of GDO on corporate green innovation but also provides new perspectives and approaches to promoting the greening of micro-level entities. Furthermore, it offers important insights for advancing green development strategies in China and other emerging market economies.
Fourth, this paper examines the dynamic variations in the green impact of GDO across diverse governance scenarios. We investigate whether firm age, managerial green cognition, environmental regulations, and intellectual property protection influence the green impact of GDO. This analysis helps systematically explore the contextual conditions that influence GDO’s green effect and encourages both firms and governments to actively adjust their decision-making. This includes preventing corporate path dependence, emphasizing the cultivation of green awareness among management, and continuing to strengthen external environmental regulations to enhance the green enabling role of GDO.
2 Literature research and development of hypotheses
2.1 Literature review
2.1.1 Research on open government data
Many scholars believe that GDO is crucial to a country’s social and economic development (Zhao and Fan, 2021). As a result, this topic has attracted considerable attention and discussion in the academic community. Current research on GDO mainly focuses on its influencing factors and the resultant effects.
From the perspective of influencing factors, first, GDO is not simply a matter of relevant government departments releasing public data. It involves data characteristics, data standards, data storage, data usage, and the protection of personal privacy, all of which directly affect the smooth implementation of GDO (Martin et al., 2013; Conradie and Choenni, 2014; Yang et al., 2015). These requirements necessitate strong technical capabilities from the government. Second, the openness of administrative culture, as well as the consideration of innovation and risk within government culture, also influence the execution of GDO projects (Zhao and Fan, 2021). Next, financial resources are also crucial when considering government data openness. Insufficient or non-prioritized funding arrangements can directly impede the effective progress of government data openness initiatives (Weerakkody et al., 2017). Additionally, a robust legal framework is essential to ensure the steady implementation of GDO (Zhao and Fan, 2021). In addition to the aforementioned factors, external environmental factors such as competition among local governments, various coercive pressures, and normative pressures can also affect the implementation of GDO (Grimmelikhuijsen and Feeney, 2017).
From an effectiveness perspective, numerous studies have found that GDO has extensive positive effects. Specifically, at the government level, data openness can enhance the transparency of government departments by releasing public information, thereby increasing public participation in the process of government decision-making (Kassen, 2013; Yan et al., 2023). At the corporate level, GDO provides various types of public information, such as commercial and educational data, which can be collected and utilized by companies to clarify their development directions and enhance their economic value (Magalhaes and Roseira, 2020; Zhou et al., 2023). For instance, Finnish companies have leveraged publicly available government information to boost their annual revenue by hundreds of millions of euros (Leviäkangas and Molarius, 2020). Additionally, GDO has been proven to reduce information asymmetry in the market, thereby lowering corporate debt costs (Xing et al., 2024). Moreover, GDO can spur corporate innovation (Jetzek et al., 2014) and enhance total factor productivity (Peng, 2023).
2.1.2 Research on corporate green innovation
Corporate green innovation is an effective method for promoting a green development path for companies and addressing environmental pollution issues (Liu et al., 2023). Consequently, the development of corporate green innovation has long garnered sustained attention from the academic community. In exploring the factors influencing green innovation, extensive studies have focused on both internal and external perspectives of firms.
From an internal perspective, scholars have focused on various factors such as executive characteristics, information disclosure, and operational strategies. Specifically, research has shown that CEOs with green experience can enhance a company’s green management practices and reduce its debt ratio, thereby promoting green technological innovation (Zhang and Zhang, 2023). Additionally, overconfident executives are more likely to embrace challenges, which boosts corporate green innovation levels (Zhou et al., 2022). From the viewpoint of information disclosure, environmental information disclosure and corporate social responsibility reports can influence firms through signaling, thereby promoting green R&D activities and increasing green output (Ren et al., 2023; Lu and Li, 2023). Additionally, digital transformation in enterprises will continuously enhance green innovation output by reducing resource input and costs (Liu et al., 2023).
From an external perspective, existing research primarily based on the Porter Hypothesis explores the impact of heterogeneous environmental regulations on firms’ green R&D and output. Some studies have pointed out that environmental taxes restrict corporate green innovation by suppressing cash flow and financing levels (Wang et al., 2023b). Other researchers have investigated the effects of carbon emission trading and found that it significantly inhibits corporate green innovation through channels such as rising carbon trading prices and decreased R&D investment (Zhang et al., 2022). Additionally, some researchers have discovered that command-and-control environmental regulations significantly promote corporate green innovation (Zhang et al., 2020). Liu et al. (2021) focused on environmental protection laws and found that their implementation effectively promotes corporate green innovation, with a more pronounced effect on state-owned enterprises. From the perspective of voluntary environmental regulations, some studies have found that corporate environmental management system certification significantly promotes corporate green innovation (Su et al., 2022).
Overall, despite extensive prior research, no studies have examined whether GDO influences corporate green R&D activities and subsequently affects their green innovation outputs. Similarly, existing literature on GDO has discussed its influencing factors and economic consequences, but a large portion of this research remains qualitative in nature. In practice, questions such as whether companies effectively utilize open data in their green innovation and development, whether data openness has fully alleviated information asymmetry among market participants, and whether the value creation of GDO has a green effect all await empirical verification. We seek to offer a marginal contribution by discussing the potential effects of GDO on corporate green decision-making.
2.2 Research hypothesis development
In the digital era, data elements have become crucial strategic resources for enhancing the current level of socio-economic development. Effectively improving the quality of data elements, accelerating their flow, and fully leveraging their value creation effects in economic development are pressing issues that need to be addressed. The government is often viewed as the largest holder of data resources in the socio-economic domain (Dawes, 2012). Consequently, the openness of government data will provide a substantial increase in data elements, accelerating their flow across various sectors of society. During this process, the public, businesses, and intermediaries can collect, organize, analyze, and utilize the open data, extracting potential economic and social value. It is foreseeable that the openness and circulation of government data will not only help alleviate friction among market participants and improve corporate operations and development but also reduce public institutional friction, strengthen public oversight and accountability, and thereby enhance administrative efficiency, judicial protection, and resource allocation (Peng, 2023; Kassen, 2013). Thus, the theoretical logic of how GDO influences corporate green innovation is as follows.
First, according to Schumpeter’s Entrepreneurship Theory, firms inevitably face various risks and uncertainties during the innovation process, such as slower-than-expected R&D progress internally and external market risks (Schumpeter, 2013). Compared to general innovation, however, green innovation activities are inherently more complex. These activities require firms not only to create and integrate knowledge across multiple dimensions—such as green product design, circular production, and pollution treatment—within their organizations but also to acquire cross-disciplinary knowledge and achieve technological integration (Wang et al., 2023a; Strambach, 2017). Therefore, when firms engage in green R&D and other innovation activities, they must possess the capability to bear risks. Corporate risk-taking requires firms to have sufficient reserves of resources to manage risks (Fazzari et al., 1987; Almeida and Campello, 2007). The resources required for risk-taking include funding, technology, product sales, and operations. Consequently, if a company has low operational and managerial capabilities and weak technological levels, its risk resources supply will naturally be affected, leading to the failure of risky projects such as green innovation. GDO can effectively enhance a company’s risk-taking ability and level, thereby promoting corporate green innovation. With GDO, companies can conveniently access local public data through API interfaces. The types of open data include more than ten categories, such as credit services, market regulation, economic development, fiscal and financial affairs, trade and commerce, and other datasets. By analyzing and utilizing extensive public big data, firms can better grasp national policy orientations, track trends in raw material and product markets, and understand consumer preferences, thereby significantly enhancing their demand forecasting, product design, pricing, inventory management, and supply chain management (Chen et al., 2020). In addition, integrating and analyzing open data in areas such as credit services, trade and commerce, and geospatial information can help firms identify potential business partners, select optimal locations for factories and warehouses, and optimize business objectives (Peng, 2023; Ghasemaghaei and Calic, 2019; 2020), thereby improving overall business management capabilities. From a research and development (R&D) perspective, GDO has further dismantled “information silos” and “information barriers,” allowing diverse and rich open data to flow into firms as new production factors. This influx facilitates the recombination of internal production factors, breaking traditional knowledge innovation models and supplementing firms with high-quality innovation resources, thereby enhancing their intrinsic R&D capabilities (Schumpeter, 2013; Yan et al., 2023). Furthermore, collecting and processing large volumes of public information—such as data on technological innovation, fiscal and financial affairs, and resource and energy sectors—can assist firms in obtaining price information and optimization strategies related to green innovation investments, thereby improving their ability to control green innovation costs. On this basis, incorporating additional data related to economic development and trade and commerce can help firms identify and predict potential market risks in green innovation and R&D processes, allowing them to prepare risk mitigation plans in advance, thereby enhancing their risk-taking capacity and level. Overall, in the context of the country’s strong promotion of corporate green transformation, GDO can enhance a company’s risk-taking ability and level in multiple ways, thereby continuously improving corporate green innovation.
Second, according to the Resource Dependence Theory, a firm’s survival and development depend not only on its internal resources and capabilities but also on its ability to acquire critical resources from the external environment (Pfeffer and Salancik, 2015). The exploration of eco-friendly technologies is a challenging and relatively complex process (Nambisan et al., 2017), involving the optimization of various stages such as production, technology application, and management processes (Kuratko et al., 2014). Therefore, sufficient funding is crucial for the orderly implementation of green R&D activities. GDO can help firms secure additional external funding, ease financing constraints, and thereby promote their green innovation and development. On one hand, data elements serve as the underlying foundation of digital finance. The GDO leads to the influx of significant incremental data resources into the market. This influx helps guide traditional financial institutions to combine new-generation information technologies, extracting economic value from these high-quality, government-endorsed data resources. For instance, leveraging data related to economic development, trade and commerce, and credit services allows these institutions to deliver intelligent and efficient online financial services. This process not only promotes the growth of the digital finance industry but also enhances the convenience of corporate financing. On the other hand, the opening up of large volumes of data helps alleviate information asymmetry between enterprises and financial institutions. According to the theory of information asymmetry, there is often an information gap between borrowers and financial institutions. Financial institutions frequently face difficulties in adequately assessing borrowers’ creditworthiness and business development levels, which can lead to moral hazard (Stiglitz and Weiss, 1981). The incremental data released by the government across categories such as credit services, enterprise services, and fiscal and financial matters provides existing financial institutions with high-quality Supplementary Data on the corporate background. This enriched data enables financial institutions to comprehensively understand a company’s operational status, creditworthiness, and developmental trajectory. This reduces information asymmetry between companies and financial institutions, lowering risk expectations (Xing et al., 2024), thereby allowing for more targeted and diversified corporate financing solutions. Taking Shanghai as an example, as of June 2022, 11 financial institutions had accessed nearly 680,000 records of government procurement data through the Shanghai Government Data Open Platform. These records were used to verify contract transaction data related to corporate clients’ participation in government procurement activities, thereby facilitating the development of “government procurement loan” financial services. Additionally, the incremental data elements also enhance the identification capabilities and efficiency of green credit allocation, ensuring that financial institutions’ green funds genuinely flow to green industries and substantial green innovation projects. Next, institutional investors typically possess strong data analysis capabilities and have their own advanced algorithms and models (Beraja et al., 2023). By integrating the rich data made available by the government, they can effectively identify the operations, strategies, and future investment potential of firms. Consequently, institutional investors can facilitate funding for firms that truly align with the national green development strategy, exhibit strong green innovation capabilities, and hold significant growth potential by increasing their stock holdings in the capital market, while requiring only a low risk premium.
Third, according to transaction cost theory, firms incur certain costs to complete transactions or activities in market economies (Coase, 2012). In this study, institutional transaction costs arise during interactions between firms and governments. These costs may include complex administrative regulations, lengthy approval processes, and opaque policies, all of which can hinder firms’ business operations or R&D activities. Such institutional burdens may impose resource constraints and, in turn, affect firms’ willingness, cost management, and innovation efficiency when engaging in green R&D activities. Accordingly, previous research has found that a high-quality business environment not only directly influences local economic growth but also significantly impacts corporate innovation performance (Chen et al., 2024). A transparent and stable business environment helps eliminate institutional barriers, reduce transaction costs, and enhance resource allocation efficiency, thereby directly affecting the efficiency and mode of R&D activities, such as green technological innovation. GDO can improve the business environment, thereby enhancing corporate green innovation. First, GDO provides various sectors of society with a wealth of data and information about government activities, which to some extent enhances the transparency of government daily activities and operational processes (Park and Gil-Garcia, 2022). This helps enterprises fully understand the administrative logic, approval processes, and policy directions of the government and encourages them to align their own business processes and green innovation models with the governmental institutional framework, thereby reducing transaction costs and boosting their own development and green innovation efficiency. Moreover, increased government transparency means that the management and decision-making processes of the government are subject to widespread public oversight. This helps to strengthen the accountability mechanisms of the public toward the government (Yan et al., 2023), encouraging the introduction of policies that support green innovation projects, reduce unfair competition, optimize resource allocation, and promote green and low-carbon development. Additionally, it fosters government initiatives to streamline administration, reform the approval system, and enhance administrative efficiency. Not only does the opening of government data facilitate one-way public oversight, but it also creates a scenario of bidirectional use (Peng, 2023). On the one hand, the public uses the data for production and operations; on the other hand, the government requires feedback on the utilization results from the public. This leads to governance innovation and enhances policy efficiency and stability. For example, the United States released the Federal Data Strategy 2020 Action Plan with the aim of effectively leveraging the dual role of the federal government as both a data provider and user.
Consequently, this paper proposes the hypothesis:
Hypothesis 1. GDO will promote corporate green innovation.
Hypothesis 1a. GDO will enhance corporate risk-taking levels, thereby promoting corporate green innovation.
Hypothesis 1b. GDO will ease financing constraints, thereby promoting corporate green innovation.
Hypothesis 1c. GDO will improve the business environment, thereby promoting corporate green innovation.
3 Research design
3.1 Sample and data
Considering that environmental pollution mainly originates from the industrial sector and that Shanghai launched the first government data open platform in 2012, this paper limits the sample period to 2007 to 2022, using Chinese publicly listed industrial companies as the research subjects. Based on previous research, we screened the sample by excluding financial companies and companies with special statuses, and we removed companies with missing data for relevant variables. Ultimately, this study includes 30,030 company-year observations. The green patent data used in this study were manually classified and organized from the public system of the China National Intellectual Property Administration and the “IPC Green Inventory” of the World Intellectual Property Organization. Additionally, the remaining data were sourced from the CSMAR and CNRDS databases.
3.2 Selection and definition of variables
3.2.1 Measuring green innovation
Following the method of Liu et al. (2023), we used the number of green patent applications collected to reflect the level of corporate green innovation (GI). Specifically, we matched the stock codes of sample companies with data from the national patent system and then identified whether the patents were green patents based on the World Intellectual Property Organization’s “IPC Green Inventory.” Through this process, we manually collected the annual green patent data for each target company.
3.2.2 Measuring GDO
The successive launch of data open platforms by local governments provided an ideal quasi-natural experimental environment for this study. Therefore, we set GDO as a dummy variable (OPEN). If the GDO platform is launched in the province, autonomous region, or municipality where the sample company is located, OPEN is given a value of 1; otherwise, it is set to 0. As of 2022, 25 GDO platforms have been launched (see Table 1).
3.2.3 Control variables
According to prior studies (Ren et al., 2021; Liu et al., 2023), we controlled for a set of influencing factors: firm size (SIZE), financial leverage (LEV), return on assets (ROA), sales growth rate (SGR), cash ratio (CASH), firm age (AGE), CEO duality (DUAL), institutional ownership (INST), independent director ratio (IDR), board size (BSIZE), economic level (GDP), education level (EDU), population size (POP). For detailed definitions of these variables, see Supplementary Appendix A.
3.3 Model setting
To test our hypotheses, we will use Equation 1, which is shown as follows:
The outcome variable GI indicates the level of green innovation, while the predictor variable OPEN signifies the degree of GDO (OPEN equals Treati × Postt, representing the interaction between whether a firm is located in a platform rollout region and the timing of the GDO platform’s launch). CONTROLS encompasses all selected control variables, with u and v representing time and individual fixed effects, respectively, and ε denoting the random disturbance term. Given that the number of green patent applications used in this study is a non-negative discrete random variable, using a linear model estimation approach might lead to estimation bias (Campbell and Mau, 2021). Therefore, following other studies (Wang et al., 2023a; Gao et al., 2024), we adopt the fixed-effects Poisson model as the baseline model1.
4 Empirical results
4.1 Descriptive statistics
As shown in Table 2, the mean value of green innovation (GI) is 2.898, indicating that there is still significant room for growth in firms’ green technology R&D capabilities. The standard deviation (sd) of 23.16 indicates substantial differences among firms. The mean value of government data openness (OPEN) is 0.482, suggesting that 48.2% of the sample firms are located in provinces that have established GDO platforms. The descriptive statistics for other variables align with real-world conditions and will not be elaborated further here.
4.2 Baseline results
Table 3 presents the regression results of the green effect of GDO. In the table, we display results using both fixed effects Poisson regression and conventional linear regression. Comparison shows that regardless of the regression model used or whether control variables are included, the coefficient of OPEN remains significant at the 1% level, indicating that GDO has a green effect and can significantly promote corporate green innovation activities and output. These results support Hypothesis 1, indicating that GDO significantly enhances firms’ green innovation levels.
4.3 Robustness checks
To ensure that the conclusions of this study are not influenced by other factors, we employed the following methods: parallel trends test, a placebo test, consideration of lagged effects, replacement of the sample period, changes in clustering methods, and exclusion of policy interference.
4.3.1 Parallel trend test
Since this study utilizes exogenous shocks, it must also satisfy the parallel trend assumption. Therefore, we further examine whether the trends in green innovation levels were consistent between regions that implemented GDO platforms and those that did not, prior to the implementation. Based on model (1) and drawing on the event study methodology, we use a dynamic DID approach to analyze the changes in green innovation trends among firms before the introduction of GDO. We construct the following variables: Before3, Before2, and Before1 represent the 3 years, 2 years, and 1 year before the launch of GDO platforms, respectively, in the regions that implemented them. Current, After1, After2, After3, and After4 represent the year of launch, 1 year after, 2 years after, 3 years after, and four or more years after the launch, respectively. To avoid multicollinearity issues, this paper uses variables representing the 4 years and beyond before the official launch of government data open platforms in the respective regions (Before4) as a reference baseline and removes it. The final results, as shown in Table 4, indicate that before the launch of the data open platforms by local governments, there were no significant differences in green R&D output among firms in different regions. However, after the launch, the levels of green innovation significantly increased.
4.3.2 Placebo test
To ensure that the green effect of GDO is not random, this paper also conducts a placebo test. First, we randomly generate new treatment and control groups and repeat this process in 1,000 regressions. Then, we plot the kernel density of the regression coefficients from all these regressions.
The graph depicted in Figure 2, based on 1,000 regressions, shows that the coefficients are primarily concentrated around zero and approximate a normal distribution. These results indicate that the randomly assigned placebo GDO variables do not significantly affect firms’ green innovation.
4.3.3 Considering the hysteresis of the effect
Considering that the green effect of GDO may have some lag, we re-estimated the model by setting the dependent variable to t+1. In column (1) of Table 5, we can see that the coefficient of OPEN is 0.164, which remains positive and passes the significance test, confirming the robustness of our conclusion.
4.3.4 Replacement sample interval
We re-estimate the model using sample data from 2009 to 2019. This approach is intended to avoid potential interference from major events such as global economic fluctuations and the pandemic. The regression outcome in column (2) of Table 5 indicate that our findings are not affected by these events and are reliable.
4.3.5 Alternative clustering method
We re-estimate the model using various clustering methods: at the industry level, province level, time-industry level, time-province level, industry-province level, firm-industry level, firm-province level, and firm-time level. The findings presented in columns (1) through (8) of Table 6 indicate that the green effect of GDO remains consistent across these different clustering methods.
4.3.6 Eliminating policy interference
To account for the potential impact of various policies during the sample period, we conduct additional analyses excluding the effects of these policies. First, China released the “Pilot Program for the Openness of Public Information Resources” in January 2018, designating Beijing, Shanghai, Zhejiang, Fujian, and Guizhou as pilot areas. To exclude the potential impact of this policy, we remove samples from 2018 onwards. Second, China issued the “Interim Measures for the Management of National Smart City Pilot Projects” in 2012, which promoted the construction of smart cities and the flow of data elements, with Shanghai achieving the best pilot results. Therefore, we exclude samples from firms located in Shanghai. Third, to implement the “Action Outline for Promoting the Development of Big Data” issued by the State Council, China established the first national-level big data comprehensive pilot area in Guizhou Province, significantly promoting the integration and flow of data resources in Guizhou. Thus, we exclude samples from firms located in Guizhou. The findings, presented in Table 7, indicate that after excluding the interference from these policies, our conclusions remain robust.
4.3.7 Addressing omitted variable bias
This study may still face concerns regarding omitted variable bias. For instance, to promote firms’ green transition, the government has implemented various measures, such as levying pollution discharge fees, introducing environmental taxes, and offering environmental subsidies. Additionally, firms’ R&D investment decisions may also influence their level of green innovation. Therefore, to rule out potential confounding effects, we proceed to further control for the aforementioned factors. First, in 2018, China transitioned from collecting pollution discharge fees to implementing an environmental protection tax. Following the methodology of Li et al. (2024), we construct the variable PDEF to measure the green fees paid by firms. Before 2018, green fees refer to pollution discharge fees, while for 2018 and subsequent years, they refer to environmental protection taxes. Second, drawing on the approach of Han et al. (2024), we identify firms’ receipt of environmental subsidies from the notes to their annual reports and construct the variable GES. Finally, we construct the variable RD to reflect firms’ R&D investment. All these variables are standardized by firms’ operating revenue. The results in Column (4) of Table 7 indicate that after accounting for these factors, the conclusions of this paper remain robust.
5 Potential mechanism analysis
In discussing Hypothesis 1, we propose that GDO enhances firms’ green innovation levels through pathways such as increasing firms’ risk-taking, alleviating financing constraints, and optimizing the regional business environment. The commonly used method for testing mediation mechanisms is the stepwise regression method, which, despite being well-developed, still faces considerable debate regarding its endogeneity issues. To address this problem and verify whether the proposed mechanisms hold, we follow the method of Jiang and Luo (2022) for the mediation mechanism test, focusing only on examining the impact of the explanatory variable on the mechanism variables.
5.1 Enterprise risk-taking mechanism
To verify the existence of the corporate risk-taking mechanism, we use the volatility of corporate earnings to reflect the firm’s risk-taking (RISK) (Boubakri et al., 2013). Specifically, we use 5-year observation periods to calculate the risk levels of firms over different periods. Next, we test the firm risk-taking mechanism, with the results shown in Table 8. In column (1), we see that the coefficient of the variable OPEN is 0.002 and passes the significance test. This finding suggests that GDO increases firms’ risk-taking levels. Therefore, Hypothesis 1a, which posits the existence of a firm risk-taking mechanism, is supported.
5.2 Enterprise financing constraint mechanism
To analyze whether the financing constraint mechanism exists, we use COD and ICC to reflect the cost of capital for firms and indicate whether firms have sufficient funding. First, following the approach of previous scholars (Shi et al., 2022; Chen et al., 2023), we constructed indicators for the cost of debt financing (COD) and the cost of equity financing (ICC). Next, we used these indicators to test for the existence of a financing constraint mechanism. Columns (2) and (3) of Table 8 present the test outcomes, revealing that the coefficients of OPEN are −0.001 and −0.004, both markedly negative. This indicates that GDO indeed reduces information asymmetry, enhances the efficiency of green capital flow, and facilitates firms’ access to funding, thereby supporting green R&D activities and increasing green R&D output. Therefore, Hypothesis 1b, which posits the existence of a financing constraint mechanism, is supported.
5.3 Business environment mechanism
To verify whether the business environment mechanism exists, this paper adopts the approach by Xin et al. (2023) and uses the overall index of the provincial-level marketization index of China to measure the business environment (BE)2 in which firms operate. This index fully considers the legal environment, market maturity, government-business relations, and the development of intermediary organizations within the region, providing a comprehensive reflection of the regional business environment. We then test the business environment mechanism. The findings are presented in Table 8. In column (4), OPEN is 0.271 and passes the significance test, indicating that GDO significantly improves the regional business environment. Thus, Hypothesis 1c, which posits the existence of the business environment mechanism, is supported. Specifically, GDO enhances the business environment, thereby promoting firms’ green innovation.
6 Further analysis
6.1 Quality inspection of GDO
In the previous section, we used a multi-period DID approach to investigate the green effect of GDO. However, we did not explore whether the specific quality of GDO affects firms’ green innovation. Therefore, following the methodology of Peng (2023), we replace the original explanatory variable with the comprehensive index from the China Open Data Index. This index, evaluated at the provincial level, professionally assesses the data openness of local governments in China, effectively reflecting the richness and quality of the data released by these governments. It is the first high-quality assessment index in the field of data openness in China. As demonstrated in column (1) of Table 9, after changing the measurement method, the GDO indicator remains significantly positive, suggesting that higher quality GDO also brings a stronger green effect to enterprises.
6.2 Enterprise green innovation classification test
Green innovation can be categorized into different types. One type involves greater R&D challenges and requires more resources. The other type has lower technological content, easier R&D processes, and requires fewer resources. Their primary function is to meet government and market demands. Thus, we categorize patents into green inventions and utility models. The results are shown in Table 9, columns (2) and (3). When the dependent variable is Gpatent_invest, the GDO indicator is significantly positive. When the dependent variable is Gpatent_unity, the significance and sign of the GDO indicator remain unchanged. These results indicate that the green effect of GDO does not disappear with changes in the type of green innovation. In other words, the green effect of GDO is comprehensive and effectively promote the green and sustainable development of enterprises.
6.3 Heterogeneity analysis
6.3.1 Heterogeneity of management’s green cognition
Managerial green cognition refers to managers’ knowledge and understanding of how green development strategies can provide long-term competitiveness to firms. Given the high-risk and long-cycle characteristics of green R&D activities, managers lacking green cognition often focus on short-term benefits, such as job stability and personal reputation, while avoiding risky activities (Hoskisson et al., 2002). Therefore, under the context of GDO, the heterogeneity in managerial green cognition may directly influence their recognition and grasp of strategic opportunities, subsequently affecting the utilization of rich public data, including production materials, product markets, government services, and socio-economic statistics, in green innovation activities. We use text analysis to measure managerial cognition. Specifically, we analyze the annual reports of listed companies, extracting the frequency of green and environmental-related keywords to measure managerial green cognition (EEC)3. We then perform a grouped regression based on the industry-year median frequency of these keywords. As shown in columns (1) and (2) of Table 10, in the group with higher managerial green cognition, the coefficient of OPEN is 0.260 and passes the significance test. In the group with lower green cognition, the coefficient of OPEN is 0.102, which is not statistically significant. These findings suggest that managerial green cognition significantly affects their recognition and utilization of the strategic opportunity presented by GDO. Managers with higher green cognition are better able to leverage this opportunity to enhance firms’ green innovation levels.
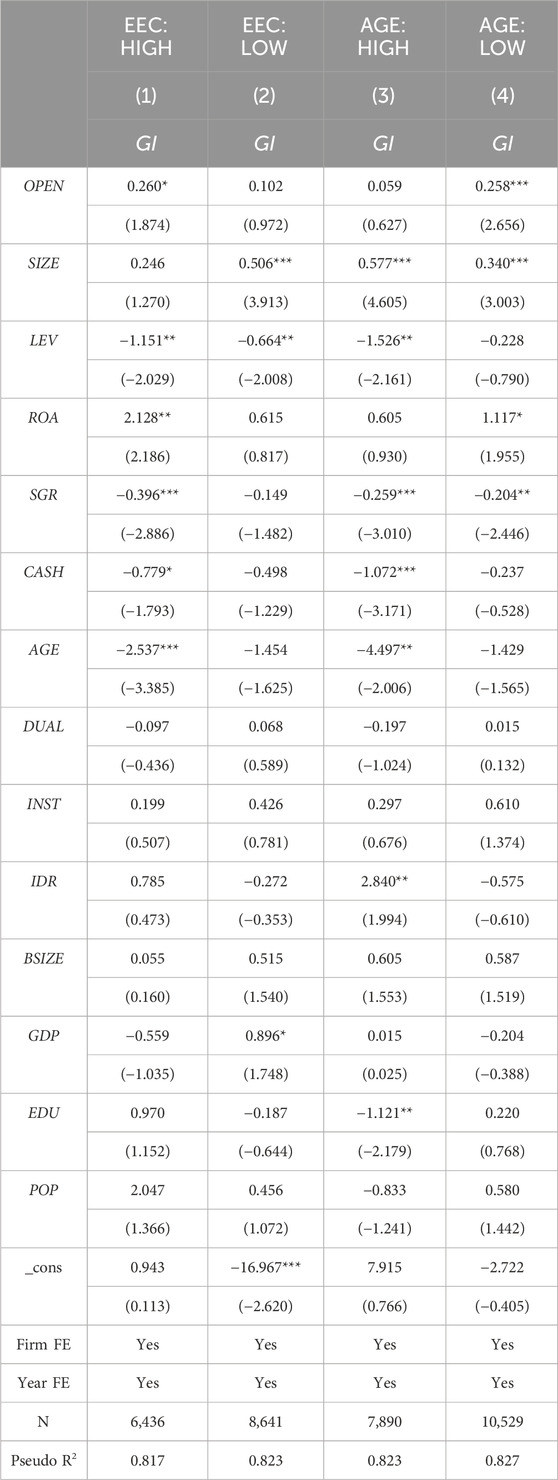
Table 10. Regression results of management green cognition heterogeneity and enterprise age heterogeneity.
6.3.2 Heterogeneity of firm age
The age of a firm often reflects its stage of development, exhibiting heterogeneous operational and management characteristics. Compared to mature firms, younger firms typically have fewer resources, such as capital and information, and their business models and systems are less developed. Conversely, younger firms tend to have lower path dependence, are more open to new ideas, and exhibit greater flexibility in management and decision-making (Shimizu and Hitt, 2005; Naldi and Davidsson, 2014). Therefore, younger firms may be more proactive in seizing the strategic opportunity presented by GDO. Consequently, the extent of the green effect of GDO may vary depending on the age of the firm.
To explore this, we first grouped companies by the median age within the same province, as shown in columns (3) and (4) of Table 10. When the firms are younger, the coefficient of OPEN is 0.258 and passes the significance test. For older firms, the coefficient of OPEN is 0.059 and does not pass the significance test. This indicates that the green effect of GDO is more pronounced in younger companies. This indicates, on one hand, that younger firms may place greater emphasis on this important opportunity provided by GDO, and on the other hand, due to their limited resources and capabilities, the value creation effect of GDO is more apparent for these younger firms.
6.3.3 Heterogeneity of environmental regulation
Due to its non-excludability, non-rivalry, and the negative externalities of pollution, environmental protection faces market failure. Environmental regulation effectively internalizes environmental costs, encouraging firms to assume environmental protection responsibilities. Leiter et al. (2011) argue that increased government environmental regulation significantly influences firms’ investment decisions, promoting green development. Consequently, varying intensities of environmental regulation may directly affect firms’ environmental pressures, leading to differentiated responses and performance when faced with GDO. Referring to previous research, we measure environmental regulation intensity (ER) by the ratio of regional industrial pollution control investment to the value-added of the secondary industry. We then use the annual median of this indicator for grouping. The findings are presented in columns (1) and (2) of Table 11. The coefficients of OPEN are 0.207 and 0.223, significant in the first group but not in the second group. These findings suggest that firms under greater environmental pressure are more likely to prioritize green transformation. They are more inclined to leverage advantageous factors, including GDO, to accelerate green R&D and innovation, thereby promoting green transformation.
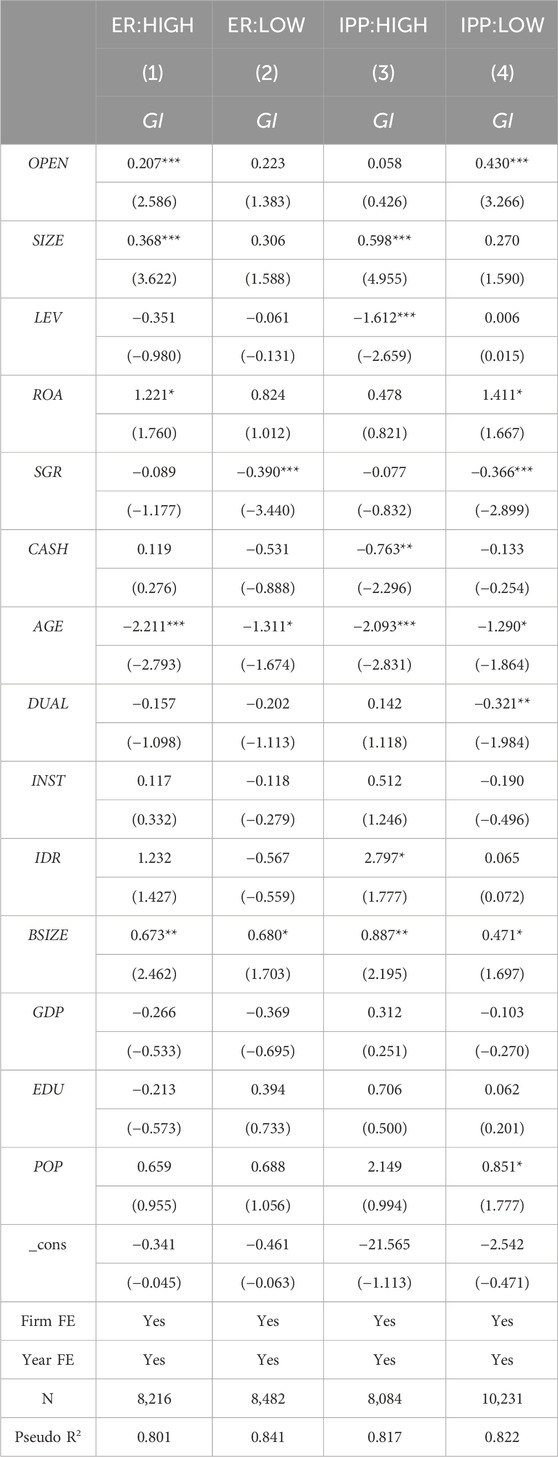
Table 11. Regression results of environmental regulation heterogeneity and intellectual property protection heterogeneity.
6.3.4 Heterogeneity of intellectual property protection
Firm innovation often entails high investment, high risk, positive externalities, and low imitation costs. Thus, the absence of intellectual property protection may lead to a mismatch between the costs and benefits of innovation for innovative firms, dampening their enthusiasm for R&D and subsequently inhibiting sustained innovation (Ang et al., 2014). In other words, strong intellectual property protection, which provides exclusivity, can help innovative firms secure monopoly profits, thereby incentivizing sustained innovation output (Sampat and Williams, 2019). According to the earlier discussion, GDO facilitates the flow of internal government activity data and information to society, enhancing government transparency (Park and Gil-Garcia, 2022). This transparency helps strengthen public and corporate oversight and accountability of the government (Yan et al., 2023), leading to improved administrative efficiency, a better regional business environment, and reduced unfair competition such as innovation imitation.
Therefore, it can be inferred that in regions with lower levels of intellectual property protection, the green effect of GDO is more pronounced. Following Tang and Liu (2024), we use an index that reflects the status of intellectual property protection in the region from the “National Intellectual Property Development Report” published by the National Intellectual Property Administration, divided by 100, as an indicator of regional intellectual property protection levels (IPP). We then perform a grouped regression based on the annual median. The findings are displayed in columns (3) and (4) of Table 11. When local government protection of intellectual property is inadequate, the coefficient of OPEN is 0.430 and is significant. When the local government emphasizes the production and dissemination of knowledge and prioritizes intellectual property protection, the coefficient of OPEN is 0.058 and does not pass the significance test. The aforementioned findings suggest that the green effect of GDO is significant only in regions with weaker intellectual property protection, fully supporting the theoretical expectations.
7 Conclusion and implications
Currently, the Chinese government is attempting to transform the traditional development model, placing high importance on the digital economy and viewing data elements as key to enhancing new drivers of economic growth. Thus, whether GDO, as an important measure, can stimulate the role of data elements and promote a green transition and sustainable development of the economy is a vital issue worth attention.
Based on this, we examine the potential green effect of GDO. The findings reveal that GDO significantly promotes corporate green innovation. Mechanism tests reveal that GDO fosters corporate green innovation through channels such as enhancing corporate risk-taking, alleviating financing constraints, and optimizing the business environment. Further tests indicate that improvements in the quality of GDO significantly enhance corporate green innovation. Moreover, the green effect of GDO is comprehensive and does not vary with the classification of green innovation types. The heterogeneity analysis indicates that the green effect of GDO is more pronounced when management’s green cognition is higher, firm age is lower, environmental regulations are stricter, and intellectual property protection is weaker.
The findings of this study hold significant reference value. First, within the strategic framework of national green transformation and innovative development, the government should continue to uphold and expand GDO. At the same time, the government should further enhance the quality of open data, broaden the scope of data accessibility, and ensure the standardization of data. These efforts would improve the ease of use of open data for various societal actors, thereby maximizing the positive green effect of GDO on firms and promoting sustainable economic growth. Second, in the process of driving firm-level transitions, it is not only essential for the government to consistently promote GDO policies but also to intensify environmental regulations to exert institutional pressure on firms. This dual-policy approach would foster coordinated governance and accelerate the green transformation of firms. Third, firms should focus on the green enabling role of GDO and consider it a vital pathway for advancing their green transformation. At the same time, firms need to further strengthen their digitalization capabilities and enhance their proficiency in big data collection and analysis. These efforts can facilitate the efficient integration of internal and external data, thereby maximizing the empowering effects of GDO on firm development. Furthermore, firms should emphasize candidates’ green cognition during the selection of managerial personnel, as such cognition may directly influence the extent to which firms recognize and leverage the green effect of GDO in their green development. Fourth, established firms should be wary of potential path dependence issues. Therefore, in their green transformation, established firms should pay attention to critical external opportunities, such as the introduction of GDO policies, and boldly break away from traditional development paths. They should actively embrace new pathways of development in the digital era, restructure their business and management processes with an information-driven approach, and ultimately leverage high-quality government data to enhance the efficiency of their transformation. Meanwhile, as younger firms tend to adapt to new developments more rapidly, they should take a leading role in utilizing government data, seize strategic opportunities to accelerate the empowering effects of GDO, and drive their intelligent and green transformation.
Data availability statement
The original contributions presented in the study are included in the article/Supplementary Material, further inquiries can be directed to the corresponding author.
Author contributions
JW: Conceptualization, Formal Analysis, Investigation, Supervision, Writing–original draft. YC: Conceptualization, Funding acquisition, Investigation, Supervision, Writing–review and editing. QL: Conceptualization, Data curation, Methodology, Supervision, Writing–review and editing.
Funding
The author(s) declare that financial support was received for the research, authorship, and/or publication of this article. This work was supported by the Humanities and Social Science Fund of Educational of China [number 23YJA630014].
Conflict of interest
The authors declare that the research was conducted in the absence of any commercial or financial relationships that could be construed as a potential conflict of interest.
Generative AI statement
The author(s) declare that no Generative AI was used in the creation of this manuscript.
Publisher’s note
All claims expressed in this article are solely those of the authors and do not necessarily represent those of their affiliated organizations, or those of the publisher, the editors and the reviewers. Any product that may be evaluated in this article, or claim that may be made by its manufacturer, is not guaranteed or endorsed by the publisher.
Supplementary material
The Supplementary Material for this article can be found online at: https://www.frontiersin.org/articles/10.3389/fenvs.2025.1530892/full#supplementary-material
Footnotes
1Similar to the study by Gao et al. (2024), we employ the ppmlhdfe command in Stata for estimating the fixed-effects Poisson model. This command eliminates observations that might prevent the maximum likelihood estimation from converging due to issues such as singletons and separation. As a result, the sample size reported in the estimation results for different equations may vary.
2Since the index is only updated until 2019, we follow the methodology of previous studies (Xin et al., 2023) by using the average annual growth rates of the total marketization index for each region to estimate the growth rates for 2019–2020, 2020–2021, and 2021–2022. This allows us to calculate the marketization index for 2020–2022 for each region.
3A summary table of green and environmental protection keywords is shown in the Supplementary Appendix B.
References
Almeida, H., and Campello, M. (2007). Financial constraints, asset tangibility, and corporate investment. Rev. Financial Stud. 20 (5), 1429–1460. doi:10.1093/rfs/hhm019
Ang, J. S., Cheng, Y., and Wu, C. (2014). Does enforcement of intellectual property rights matter in China? Evidence from financing and investment choices in the high-tech industry. Rev. Econ. Statistics 96 (2), 332–348. doi:10.1162/REST_a_00372
Attard, J., Orlandi, F., Scerri, S., and Auer, S. (2015). A systematic review of open government data initiatives. Gov. Inf. Q. 32 (4), 399–418. doi:10.1016/j.giq.2015.07.006
Beraja, M., Yang, D. Y., and Yuchtman, N. (2023). Data-intensive innovation and the State: evidence from AI firms in China. Rev. Econ. Stud. 90 (4), 1701–1723. doi:10.1093/restud/rdac056
Boubakri, N., Cosset, J. C., and Saffar, W. (2013). The role of state and foreign owners in corporate risk-taking: evidence from privatization. J. financial Econ. 108 (3), 641–658. doi:10.1016/j.jfineco.2012.12.007
Campbell, D. L., and Mau, K. (2021). On “Trade induced technical change: the impact of Chinese imports on innovation, IT, and productivity”. Rev. Econ. Stud. 88 (5), 2555–2559. doi:10.1093/restud/rdab037
Chen, J., Huang, S., and Liu, Y. (2020). Operations management in the digitization era: from empowering to enabling. Manag. World 36 (02), 117–128+222. (In Chinese). doi:10.19744/j.cnki.11-1235/f.2020.0025
Chen, X., Chen, Y., Luo, Z., and Jiang, J. (2024). Business environment, R&D subsidies, and substantive innovation in state-owned enterprises. Sci. Res. Manag. 45 (02), 155–164. (In Chinese). doi:10.19571/j.cnki.1000-2995.2024.02.016
Chen, Y., Li, T., Zeng, Q., and Zhu, B. (2023). Effect of ESG performance on the cost of equity capital: evidence from China. Int. Rev. Econ. and Finance 83, 348–364. doi:10.1016/j.iref.2022.09.001
Coase, R. H. (2012). “The nature of the firm,” in The roots of logistics (Berlin, Heidelberg: Springer), 317–333. doi:10.1007/978-3-642-27922-5_22
Conradie, P., and Choenni, S. (2014). On the barriers for local government releasing open data. Gov. Inf. Q. 31, S10–S17. doi:10.1016/j.giq.2014.01.003
Dawes, S. S. (2012). A realistic look at open data. Albany, NY: Center for Technology in Government, University at Albany/SUNY. Available at: http://www.w3.org/2012/06/pmod/pmod2012_submission_38.pdf.
Fazzari, S., Hubbard, R. G., and Petersen, B. C. (1987). Financing constraints and corporate investment.
Feng, Y., Chen, Z., and Nie, C. (2023). The effect of broadband infrastructure construction on urban green innovation: evidence from a quasi-natural experiment in China. Econ. Analysis Policy 77, 581–598. doi:10.1016/j.eap.2022.12.020
Gao, Y., Li, X., and Li, J. (2024). Fintech and the path of technological innovation: from the perspective of green transformation. China Ind. Econ. (02), 80–98. (In Chinese). doi:10.19581/j.cnki.ciejournal.2024.02.006
Ghasemaghaei, M., and Calic, G. (2019). Does big data enhance firm innovation competency? The mediating role of data-driven insights. J. Bus. Res. 104, 69–84. doi:10.1016/j.jbusres.2019.07.006
Ghasemaghaei, M., and Calic, G. (2020). Assessing the impact of big data on firm innovation performance: big data is not always better data. J. Bus. Res. 108, 147–162. doi:10.1016/j.jbusres.2019.09.062
Grimmelikhuijsen, S. G., and Feeney, M. K. (2017). Developing and testing an integrative framework for open government adoption in local governments. Public Adm. Rev. 77 (4), 579–590. doi:10.1111/puar.12689
Han, F., Mao, X., Yu, X., and Yang, L. (2024). Government environmental protection subsidies and corporate green innovation: evidence from Chinese microenterprises. J. Innovation and Knowl. 9 (1), 100458. doi:10.1016/j.jik.2023.100458
Hoskisson, R. E., Hitt, M. A., Johnson, R. A., and Grossman, W. (2002). Conflicting voices: the effects of institutional ownership heterogeneity and internal governance on corporate innovation strategies. Acad. Manag. J. 45 (4), 697–716. doi:10.5465/3069305
Jetzek, T., Avital, M., and Bjorn-Andersen, N. (2014). Data-driven innovation through open government data. J. Theor. Appl. Electron. Commer. Res. 9 (2), 15–16. doi:10.4067/S0718-18762014000200008
Jiang, T., and Luo, Z. B. (2022). LOC102724163 promotes breast cancer cell proliferation and invasion by stimulating MUC19 expression. China Ind. Econ. 23 (05), 100–120. (In Chinese). doi:10.3892/ol.2022.13220
Kassen, M. (2013). A promising phenomenon of open data: a case study of the Chicago open data project. Gov. Inf. Q. 30 (4), 508–513. doi:10.1016/j.giq.2013.05.012
Kuratko, D. F., Covin, J. G., and Hornsby, J. S. (2014). Why implementing corporate innovation is so difficult. Bus. Horizons 57 (5), 647–655. doi:10.1016/j.bushor.2014.05.007
Leiter, A. M., Parolini, A., and Winner, H. (2011). Environmental regulation and investment: evidence from European industry data. Ecol. Econ. 70 (4), 759–770. doi:10.1016/j.ecolecon.2010.11.013
Leviäkangas, P., and Molarius, R. (2020). Open government data policy and value added-Evidence on transport safety agency case. Technol. Soc. 63, 101389. doi:10.1016/j.techsoc.2020.101389
Li, F., Na, P., Wang, X., and Li, X. (2024). Environmental protection taxes, audit fees and corporate ESG performance. Finance Res. Lett. 69, 106058. doi:10.1016/j.frl.2024.106058
Lin, B., and Ma, R. (2022). How does digital finance influence green technology innovation in China? Evidence from the financing constraints perspective. J. Environ. Manag. 320, 115833. doi:10.1016/j.jenvman.2022.115833
Liu, X., Liu, F., and Ren, X. (2023). Firms' digitalization in manufacturing and the structure and direction of green innovation. J. Environ. Manag. 335, 117525. doi:10.1016/j.jenvman.2023.117525
Liu, Y., Wang, A., and Wu, Y. (2021). Environmental regulation and green innovation: evidence from China’s new environmental protection law. J. Clean. Prod. 297, 126698. doi:10.1016/j.jclepro.2021.126698
Lu, Z., and Li, H. (2023). Does environmental information disclosure affect green innovation? Econ. Analysis Policy 80, 47–59. doi:10.1016/j.eap.2023.07.011
Ma, D., and Zhu, Q. (2022). Innovation in emerging economies: research on the digital economy driving high-quality green development. J. Bus. Res. 145, 801–813. doi:10.1016/j.jbusres.2022.03.041
Magalhaes, G., and Roseira, C. (2020). Open government data and the private sector: an empirical view on business models and value creation. Gov. Inf. Q. 37 (3), 101248. doi:10.1016/j.giq.2017.08.004
Martin, S., Foulonneau, M., Turki, S., and Ihadjadene, M. (2013). Risks analysis to overcome barriers to open data. Electron. J. e-Government 11 (2), 348–359. Available at: https://academic-publishing.org/index.php/ejeg/article/view/576.
Naldi, L., and Davidsson, P. (2014). Entrepreneurial growth: the role of international knowledge acquisition as moderated by firm age. J. Bus. Ventur. 29 (5), 687–703. doi:10.1016/j.jbusvent.2013.08.003
Nambisan, S., Lyytinen, K., Majchrzak, A., and Song, M. (2017). Digital innovation management: reinventing innovation management research in a digital world. MIS Q. 41 (1), 223–238. doi:10.25300/misq/2017/41:1.03
Park, S., and Gil-Garcia, J. R. (2022). Open data innovation: visualizations and process redesign as a way to bridge the transparency-accountability gap. Gov. Inf. Q. 39 (1), 101456. doi:10.1016/j.giq.2020.101456
Peng, Y. (2023). The value creation role of open government data: the perspective of firm’s total factor productivity. J. Quantitative and Tech. Econ. 40 (09), 50–70. (In Chinese). doi:10.13653/j.cnki.jqte.20230725.001
Pfeffer, J., and Salancik, G. (2015). “External control of organizations—resource dependence perspective,” in Organizational behavior 2 (London: Routledge), 355–370. doi:10.4324/9781315702001-24
Ren, S., Huang, M., Liu, D., and Yan, J. (2023). Understanding the impact of mandatory CSR disclosure on green innovation: evidence from Chinese listed firms. Br. J. Manag. 34 (2), 576–594. doi:10.1111/1467-8551.12609
Ren, S., Wang, Y., Hu, Y., and Yan, J. (2021). CEO hometown identity and firm green innovation. Bus. Strategy Environ. 30 (2), 756–774. doi:10.1002/bse.2652
Sampat, B., and Williams, H. L. (2019). How do patents affect follow-on innovation? Evidence from the human genome. Am. Econ. Rev. 109 (1), 203–236. doi:10.1257/aer.20151398
Schumpeter, J. A. (2013). Capitalism, socialism and democracy. London: Routledge. doi:10.4324/9780203202050
Shi, J., Yu, C., Li, Y., and Wang, T. (2022). Does green financial policy affect debt-financing cost of heavy-polluting enterprises? An empirical evidence based on Chinese pilot zones for green finance reform and innovations. Technol. Forecast. Soc. Change 179, 121678. doi:10.1016/j.techfore.2022.121678
Shimizu, K., and Hitt, M. A. (2005). What constrains or facilitates divestitures of formerly acquired firms? The effects of organizational inertia. J. Manag. 31 (1), 50–72. doi:10.1177/0149206304271381
Stiglitz, J. E., and Weiss, A. (1981). Credit rationing in markets with imperfect information. Am. Econ. Rev. 71 (3), 393–410. Available at: https://www.jstor.org/stable/1802787.
Strambach, S. (2017). Combining knowledge bases in transnational sustainability innovation: microdynamics and institutional change. Econ. Geogr. 93 (5), 500–526. doi:10.1080/00130095.2017.1366268
Su, W., Lei, G., Guo, S., and Dan, H. (2022). Study on the influence mechanism of environmental management system certification on enterprise green innovation. Int. J. Environ. Res. Public Health 19 (19), 12379. doi:10.3390/ijerph191912379
Tan, J., Chan, K. C., and Chen, Y. (2022). The impact of air pollution on the cost of debt financing: evidence from the bond market. Bus. Strategy Environ. 31 (1), 464–482. doi:10.1002/bse.2904
Tang, J., and Liu, Q. (2024). R&D tax incentive policy, intellectual property right protection, and corporate innovation in an emerging market. Res. Int. Bus. Finance 69, 102244. doi:10.1016/j.ribaf.2024.102244
Wang, F., He, J., and Chen, L. (2023a). Will interlocking directors with green experience promote quantity increase and quality improvement of enterprise green innovation. China Ind. Econ. (10), 155–173. (In Chinese). doi:10.19581/j.cnki.ciejournal.2023.10.006
Wang, L., Long, Y., and Li, C. (2022). Research on the impact mechanism of heterogeneous environmental regulation on enterprise green technology innovation. J. Environ. Manag. 322, 116127. doi:10.1016/j.jenvman.2022.116127
Wang, Y., Xu, S., and Meng, X. (2023b). Environmental protection tax and green innovation. Environ. Sci. Pollut. Res. 30 (19), 56670–56686. doi:10.1007/s11356-023-26194-z
Weerakkody, V., Irani, Z., Kapoor, K., Sivarajah, U., and Dwivedi, Y. K. (2017). Open data and its usability: an empirical view from the Citizen’s perspective. Inf. Syst. Front. 19, 285–300. doi:10.1007/s10796-016-9679-1
Xie, C., Ye, L., Zhong, N., and Wan, W. (2024). Impact of digital finance on corporate green innovation: exploring role of land resource misallocation in China. Resour. Policy 91, 104920. doi:10.1016/j.resourpol.2024.104920
Xin, J., Cai, J., Yang, C., and Guan, X. (2023). Business environment, financing structures, and investment in innovation of family enterprises. Sci. Res. Manag. 44 (01), 56–65. (In Chinese). doi:10.19571/j.cnki.1000-2995.2023.01.007
Xing, Q., Xu, G., and Wang, Y. (2024). Open government data and the cost of debt. Int. Rev. Financial Analysis 95 (Part A), 103384. doi:10.1016/j.irfa.2024.103384
Yan, R., Xing, C., Chen, X., and Zhao, Y. (2023). Is it real or illusory? An empirical examination of the impact of open government data on innovation capability in the case of China. Technol. Soc. 75, 102396. doi:10.1016/j.techsoc.2023.102396
Yang, T. M., Lo, J., and Shiang, J. (2015). To open or not to open? Determinants of open government data. J. Inf. Sci. 41 (5), 596–612. doi:10.1177/0165551515586715
Zhang, J., Liang, G., Feng, T., Yuan, C., and Jiang, W. (2020). Green innovation to respond to environmental regulation: how external knowledge adoption and green absorptive capacity matter? Bus. Strategy Environ. 29 (1), 39–53. doi:10.1002/bse.2349
Zhang, J., and Zhang, K. (2023). How does CEO green experience affect green innovation of energy firms? Evidence from China. Energy and Environ., 0958305X231207057. doi:10.1177/0958305X231207057
Zhang, W., Li, G., and Guo, F. (2022). Does carbon emissions trading promote green technology innovation in China? Appl. Energy 315, 119012. doi:10.1016/j.apenergy.2022.119012
Zhao, Y., and Fan, B. (2021). Effect of an agency’s resources on the implementation of open government data. Inf. and Manag. 58 (4), 103465. doi:10.1016/j.im.2021.103465
Zhou, M., Wang, Y., Huang, X., and Li, G. (2023). Can open government data policy improve firm performance? Evidence from listed firms in China. Manag. Decis. Econ. 44 (5), 2593–2603. doi:10.1002/mde.3835
Keywords: government data openness, green effect, data element, sustainability development, corporate risk-taking, financing constraints, business environment
Citation: Wang J, Chen Y and Luo Q (2025) Does government data openness have a green effect? an examination from the perspective of green innovation. Front. Environ. Sci. 13:1530892. doi: 10.3389/fenvs.2025.1530892
Received: 19 November 2024; Accepted: 27 January 2025;
Published: 14 February 2025.
Edited by:
Xiaolei Sun, Chinese Academy of Sciences (CAS), ChinaReviewed by:
Lu Liu, Southwestern University of Finance and Economics, ChinaJinyu Chen, Capital University of Economics and Business, China
Copyright © 2025 Wang, Chen and Luo. This is an open-access article distributed under the terms of the Creative Commons Attribution License (CC BY). The use, distribution or reproduction in other forums is permitted, provided the original author(s) and the copyright owner(s) are credited and that the original publication in this journal is cited, in accordance with accepted academic practice. No use, distribution or reproduction is permitted which does not comply with these terms.
*Correspondence: Qingfeng Luo, cWluZ2ZlbmdsbHVvQGZveG1haWwuY29t