- 1State Key Laboratory of Regional Environment and Sustainability, School of Environment, Beijing Normal University, Beijing, China
- 2School of Biological and Chemical Engineering, Guangxi University of Science and Technology, Liuzhou, Guangxi, China
- 3School of Civil Engineering, Yantai University, Yantai, Shandong, China
- 4Ministry of Education Key Laboratory of Water and Sediment Science, School of Environment, Beijing Normal University, Beijing, China
Introduction: Cascade hydropower development significantly alters the structure and function of river ecosystems. Phytoplankton, as primary producers, are highly sensitive to environmental changes, and their diversity and community structure reflect the state of the water environment. While there is extensive research on how changes in river environments affect phytoplankton community structure, studies on the spatial distribution patterns and community assembly mechanisms of phytoplankton in relation to hydraulic residence time (HRT)—a key characteristic of reservoirs—are limited.
Methods: Linear regression analysis was used to examine the relationship between phytoplankton communities and HRT. Additionally, the Neutral Community Model (NCM) combined with the Modified Stochasticity Ratio (MST) was employed to reveal the types of phytoplankton community assembly in these cascade reservoirs. Finally, Mantel tests and stepwise regression analysis assessed the specific impacts of environmental factors on phytoplankton communities.
Results: In the ecosystem of the Yellow River cascade reservoirs, HRT is positively correlated with phytoplankton abundance and diversity across different seasons. In annual regulation hydropower stations (LJX and LYX), phytoplankton species richness, abundance, and diversity indices are higher compared to those in runoff hydropower stations.
Discussion: The phytoplankton community assembly is primarily driven by deterministic processes, with annual regulation hydropower station being more influenced by these processes than runoff hydropower station. HRT, water temperature (WT), and total nitrogen (TN) are the most significant environmental factors affecting the structural differences of phytoplankton in cascade reservoirs. There is a significant positive correlation between phytoplankton and water temperature, especially in short HRT runoff hydropower station where phytoplankton abundance is more significantly impacted by water temperature.
Highlights
• Regression analysis reveals that hydraulic residence time (HRT) is one of the key driving factors influencing phytoplankton community structure changes.
• HRT positively correlates with phytoplankton abundance and diversity in the upper Yellow River cascade reservoirs.
• Deterministic processes play a major role in the assembly of phytoplankton communities in the upper cascade reservoirs of the Yellow River.
• Water temperature positively correlates with phytoplankton abundance, with shorter HRT reservoirs being more influenced by water temperature.
1 Introduction
To meet multiple demands such as hydropower production, flood control, ice jam prevention, transportation, irrigation, and water supply for both industrial and domestic use, 61,988 large reservoirs exceeding 15 m in height have been constructed on rivers worldwide (ICOLD, 2023). Reservoir impoundment alters natural hydrological rhythms (Datry et al., 2023), affects hydrodynamic conditions (Grill et al., 2019; Belletti et al., 2020), and disrupts suspended sediment deposition processes (Maavara et al., 2020), which has a significant nutrient transport and overall health of river ecosystems impacts. Particularly in reservoir areas, there is an increased risk of eutrophication and excessive algal blooms due to slower flow rates and longer water exchange times, all of which pose a threat to the health and stability of river ecosystems.
Phytoplankton are the main primary producers in aquatic ecosystems, playing crucial roles in material cycling, energy flow, and maintaining of aquatic ecosystem balance (Li et al., 2013; Mor et al., 2018; Yi et al., 2023). They are extremely sensitive to environmental changes, and changes in the aquatic environment can cause changes in the diversity and structure of phytoplankton communities (Chen et al., 2020; Salmaso and Tolotti, 2021; Shen et al., 2024). Environmental factors such as nutrient salts and water temperature have important effects on phytoplankton growth (Salmaso and Tolotti, 2021), while hydrodynamic conditions significantly influence phytoplankton community composition (Liu et al., 2021; Ruan et al., 2024), and cascade reservoirs further add to the complexity of the pattern of this effect. Previous studies on the effect of reservoirs on phytoplankton community composition have focused on the effects of changing nutrient concentrations (Zhang et al., 2024), seasonal fluctuations (Ruan et al., 2024), and hydrodynamic conditions (Liu et al., 2021) in single reservoirs on phytoplankton community structure response patterns. In contrast, the impacts of cascade reservoirs on phytoplankton communities are more complex and multidimensional due to their unique cumulative effects (Liu et al., 2021). In the context of climate change, the impacts of terraced development on river ecosystems have become more complex (He et al., 2024). Therefore, clarifying the effects of environmental factors on phytoplankton community composition is crucial to understanding the ecological impacts of cascade reservoirs.
Hydraulic residence time (HRT) is a crucial factor influencing the biogeochemical cycling and physical transformation of nutrients in rivers (Maavara et al., 2020; Zhao et al., 2024). Reservoirs of different scales show significant variations in water quality factors, which are closely linked to their scale and HRT (Wang et al., 2022). The duration of hydraulic residence time significantly affects the structure of phytoplankton communities (Zeng et al., 2006; Yin et al., 2024). HRT affects the retention of nutrients such as nitrogen and phosphorus, altering their composition (Zhao et al., 2024), and ultimately significantly impacts the structure of phytoplankton communities (Li et al., 2013; Znachor et al., 2020). Rivers at high-altitudes are receiving increasing attention due to the impacts of rapid climate change (Xu et al., 2024). These rivers have driven intensive hydropower development activities due to their large slope drop and abundant hydroelectric resources (Li et al., 2018). However, hydropower development may lead to longer or altered HRT in rivers, which in turn pose potential adverse effects on the health and sustainability of river ecosystems. Nevertheless, there is still a lack of clear understanding on the specific effects of hydraulic residence time on phytoplankton community structure and the role it plays in the community assembly process in the context of cascade hydropower development in high-altitude rivers.
In studies analyzing the response of phytoplankton to environmental conditions, ordination analyses such as principal component analysis (PCA) (Xu et al., 2022) and correlation analyses (e.g., Mantel test, Ruan et al., 2024) are often used to compare the effects of different environmental variables on differences in community structure. To gain a deeper understanding of the multifactor-driven mechanisms of phytoplankton community assembly from an ecological perspective, the neutral community model (NCM) was introduced, which distinguishes between deterministic processes (e.g., environmental filtering and species interactions) and stochastic processes (including births, deaths, migrations, dispersal, etc.) (Sloan et al., 2006). In recent years, the model has been widely applied in aquatic ecosystems such as rivers, reservoirs and lakes (Isabwe et al., 2022; Xiao et al., 2022; Zhang et al., 2024). However, regarding the type of dominant drivers of phytoplankton community construction, the results of studies in different water body types are controversial (Xiao et al., 2022; Zhang et al., 2024). Deterministic processes dominate community construction in plain river ecosystems (Isabwe et al., 2022), while stochastic processes are more critical in estuarine and lake ecosystems (Sun et al., 2023; Xiao et al., 2022). Although the above studies have provided insights into the mechanisms of phytoplankton community assembly, the mechanisms and driving factors of phytoplankton community assembly in the cascade ecosystems formed by high-altitude rivers and reservoirs remain unclear.
The Yellow River is the second-longest river in China and the fifth-longest river in the world. The upper reaches of the Yellow River, from Longyangxia to Liujiaxia, are located in the transition zone between the Tibetan Plateau and the Loess Plateau, and belong to the alpine river region, with a unique plateau-alpine climate, which plays an important role in the earth’s climate, biodiversity and water cycle (Yang et al., 2023). This section of the river is rich in hydropower resources, and it is the most densely populated area of hydropower stations on the main stream of the Yellow River. Currently, studies on the ecological impacts of hydropower development on the Yellow River gradient on the river mainly focus on reservoir hydrological regulation (Xu et al., 2022), water temperature change (Ren et al., 2020), carbon emission (Wang et al., 2023), nutrient salts dynamics (Wu et al., 2021), species diversity (Jia et al., 2020) and biological evaluation of water quality (Ding et al., 2021). However, studies on the types of drivers of phytoplankton community aggregation in alpine river terrace reservoirs are still very limited. Given the importance of this reach in exploring the impacts of alpine river terrace development on aquatic ecosystems, this study targeted six reservoirs with different HRT in the upper reaches of the Yellow River, aiming to address the questions of (1) how does the structure of phytoplankton communities change under different HRT conditions? (2) How do environmental factors affect phytoplankton community structure? (3) What are the differences between the contributions of deterministic and stochastic processes in phytoplankton community structure? We hypothesized that terraced hydropower development interferes with the natural process of phytoplankton community construction in alpine rivers, in which HRT plays a key role. This research aims to improve the understanding of the mechanisms of riverine phytoplankton community construction and its drivers.
2 Materials and methods
2.1 Study area
The Yellow River, China’s second-longest river spanning 5,464 km, with an annual runoff of 58 billion m3 and a drainage area of 752,443 km2, is revered as the “Mother River” of the Chinese nation. The upper Yellow River section from Longyangxia to Liujiaxia (hereafter referred to as the Long-Liu section), is situated on the northeastern fringe of the Qinghai-Tibet Plateau. This region comprises plateaus, wetlands, and valleys, ranging in elevation from 1,800 to 4,800 m. The Long-Liu section experiences a typical plateau continental climate marked by low rainfall, high evaporation, dry air, and cool temperatures. The average annual temperature is 5°C–9°C, with annual precipitation ranging from 194 to 357 mm and evaporation from 1,500 to 2,131 mm. The river section has distinct dry and wet season. The water has high oxygenated, while water temperature and nutrient content remain relatively low. In order to meet the needs of flood control, bulging prevention, irrigation, production and living as well as clean energy supply, 27 reservoirs have been built in the main stream of the Yellow River. Among them, the Long-Liu section is the most concentrated area of reservoirs, with a total of 13. Longyangxia, Laxiwa, Lijiaxia, Gongboxia, Jishixia, and Liujiaxia all have a dam height of more than 100 m, with an annual power generation of more than 1,000 MW (Table 1).
Two surveys were conducted on the cascade reservoirs in the Long–Liu section during the dry season (May 11–26, 2023) and the wet season (September 15–30, 2023). A total of 29 monitoring cross sections were setted, encompassing upstream river reaches, within the reservoirs, in front of the dams and downstream river reaches (Figure 1), to comprehensively characterize the spatial distribution patterns of phytoplankton within the reservoir system. S1-S6 are, in order, the upstream channel, the middle of the reservoir (3 sample sites), the front of the reservoir and the downstream channel of the dam in Longyangxia (LYX) reservoir; S7-S10 are, in order, the upstream channel, the middle of the reservoir, the front of the reservoir and the downstream channel of the dam in Laxiwa (LXW) reservoir; S11-15 are, in order, the upstream channel, the middle of the reservoir (2 sample sites), the front of the reservoir and the downstream channel of the dam in Lijiaxia (LiJX) reservoir; S16- S18 are, in order, the middle, front and downstream channels of Gongboxia (GBX); S19-S22 are, in order, the upstream channel, middle, front and downstream channels of the dam of Jishixia (JSX) reservoir; S23 -S27 are, in order, the upstream channel, middle (2 sample sites), the front of the reservoir and the downstream channel of the dam in Liujiaxia (LYX) reservoir. S28 is a sampling sites in the middle reaches of the Tao River, a tributary, and S29 is where the Tao River tributary joins the Liujiaxia Reservoir area.
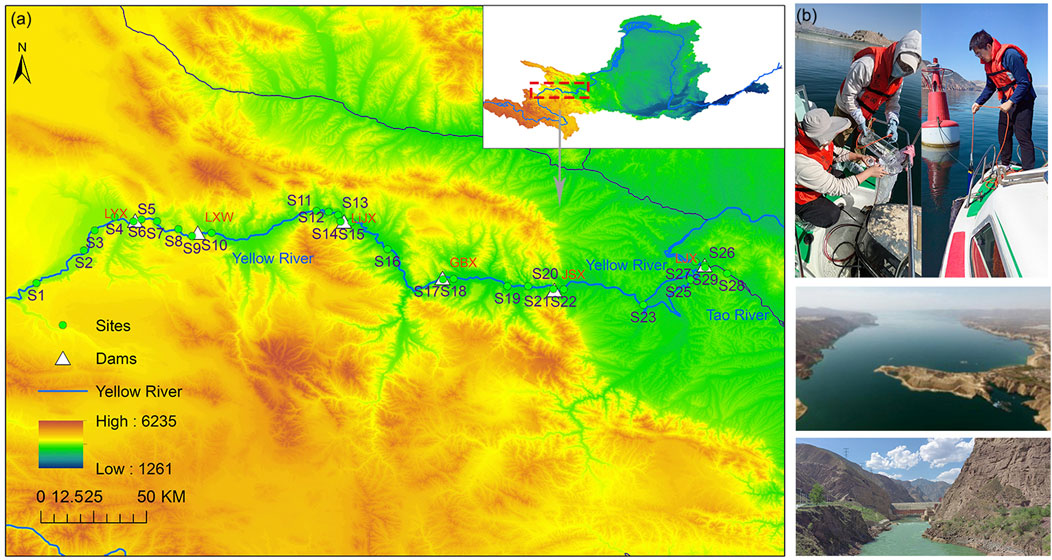
Figure 1. Location of cascade reservoirs and sampling sites in the upper Yellow River. (a) Study area; (b) Photos of field sampling.
2.2 Sample collection and laboratory analyses
Field water quality parameters were measured using the Multiparameter Water Quality Sonde (YSI EXO2, Xylem Inc., United States), including water temperature (WT, °C), pH, electrical conductivity (EC, μS cm−1), salinity (Salt, ng L−1), dissolved oxygen (DO, mg L−1), oxidation-reduction potential (ORP, mV), and chlorophyll-a (Chl-a, μg mL−1). At each sampling site, 2 L of surface water was collected using an organic glass water sampler, sealed, and transported to the laboratory under low-temperature conditions. Following the Chinese Environmental Quality Standards for Surface Water (SEPA, 2002), laboratory analyses were conducted to determine total nitrogen (TN, mg L−1), total phosphorus (TP, mg L−1), nitrate nitrogen (NO3−-N, mg L−1), nitrite nitrogen (NO2−-N, mg L−1), ammonium nitrogen (NH4+-N, mg L−1), and chemical oxygen demand (COD, mg L−1). Total organic carbon (TOC, mg L−1) was measured using a total organic carbon analyzer (TOC-L, Shimadzu Corporation, Japan). Additionally, 5 L of surface water was collected and filtered through a 0.45 μm mixed cellulose membrane (Merck Millipore, United States), and the total suspended solids (TSS, mg L−1) concentration was determined by the gravimetric method. Geographic variables, including longitude, latitude, and altitude, were recorded using the OWI interactive mapping software (V10.1.3).
At each sampling site, qualitative and quantitative phytoplankton samples were collected to analyze phytoplankton community diversity and composition. Qualitative samples were obtained using a No. 25 plankton net (mesh size: 0.064 mm) by towing it in an “∞” pattern at a depth of 50 cm for 1–3 min. The collected samples were transferred into 100 mL sample bottles and fixed with 1%–1.5% (v/v) Lugol’s solution for microscopic examination. Quantitative phytoplankton samples were collected by obtaining 1 L of surface water in high-density polyethylene bottles and fixing them with 1%–1.5% (v/v) Lugol’s solution. After sedimentation for 24 h, the supernatant was removed using a siphon, and the remaining 20–25 mL of concentrated sediment was transferred into 50 mL sample bottles for further microscopic analysis. For cell counting, the sample was thoroughly mixed, and 0.1 mL was placed into a phytoplankton counting chamber. Species identification and cell enumeration were conducted using a microscope (Olympus BX53, Olympus Corporation, Japan). Each sample was counted twice, and if the mean difference between the two counts was within 15%, the data were considered valid (Zhang et al., 2021). Species identification was based on Atlas of Common Freshwater Planktonic Algae in China (Weng and Xu, 2010). Phytoplankton biomass was estimated following the method described by Hillebrand et al. (1999).
2.3 Data statistics and analysis
2.3.1 Types of reservoirs
According to the HRT, reservoirs with an HRT >0.20a are classified as annual regulation hydropower stations, while others are categorized as runoff reservoirs (Yin et al., 2024). LYX and LJX are annual regulation hydropower stations, while LiJX, LXW, GBX, and JSX operate as runoff hydropower stations (Table 1).
where V is the reservoir capacity (m3) and Q is the annual runoff volume (m3).
2.3.2 Biological indices
Alpha diversity analysis: The Shannon-Wiener diversity index (Shannon, 1948) and the Simpson diversity index (Simpson, 1949) were used to analyse the alpha diversity of the phytoplankton. The alpha diversity indices of phytoplankton communities were calculated using the vegan package in R software (version 4.3.2).
Shannon-Wiener diversity index,
Simpson diversity index, D:
In the formula, Pi = Ni/N, Pi represents the ratio of the number of individuals of species i to the total number of individuals in the sample, Ni denotes the number of individuals of species i, and N represents the total number of individuals in the sample; S represents the total number of species in the sample.
Dominance is an index used to assess the advantageous or disadvantageous status of species within a phytoplankton community. It is determined based on both the occurrence frequency and the individual abundance of each species.
Where fi represents the occurrence frequency of species i across all sampling sites, Ni is the individual count of species i, and N is the total number of individuals in the sample. A species is classified as dominant when Y > 0.02 (Lampitt et al., 1993).
2.3.3 Data analysis
This study employed ArcGIS 10.8 for mapping sampling sites. Microsoft Excel 2021 was used for initial data processing. Origin 2023 was utilized for generating chord diagrams, conducting linear regression analysis, and creating corresponding graphs. The NCM, assessed using the Hmisc package, was used to evaluate the relative importance of deterministic and stochastic processes in shaping phytoplankton community structure (Sloan et al., 2006). The NTS package computed the Modified Stochasticity Ratio (MST) to quantitatively assess ecological randomness (Ning et al., 2019). Forward stepwise regression analysis of environmental factors impacting phytoplankton communities was performed using the MASS package (Jiao et al., 2020). To assess the significance of different factors on phytoplankton, Mantel tests were conducted, and the plspm package was employed for partial least squares path modeling (PLS-PM) to explore key driving factors influencing phytoplankton community structure (Liu et al., 2024). Phytoplankton data were transformed using Hellinger transformation during computations, while environmental factors (excluding pH) were log-transformed as log (x+1).
3 Results
3.1 Characteristics of the phytoplankton community composition
A total of 38 species (genera) of phytoplankton were identified across two sampling events, belonging to 7 phyla, 11 classes, 18 orders, and 24 families, with Bacillariophyta, Chlorophyta, and Cyanobacteria being the dominant groups (Figure 2; Table 2). Among them, Bacillariophyta had the highest species richness (14 species), followed by Chlorophyta (13 species) and Cyanophyta (4 species), while Cryptophyta and Chrysophyta had fewer species. The number of phytoplankton species was slightly lower during the dry season (28 species) compared to the wet season (32 species). Annual regulation hydropower station (LJX and LYX), the species richness of phytoplankton was higher compared to other reservoirs. The sequence of phytoplankton richness was LJX > LYX > LiJX > JSX > LXW > GBX.
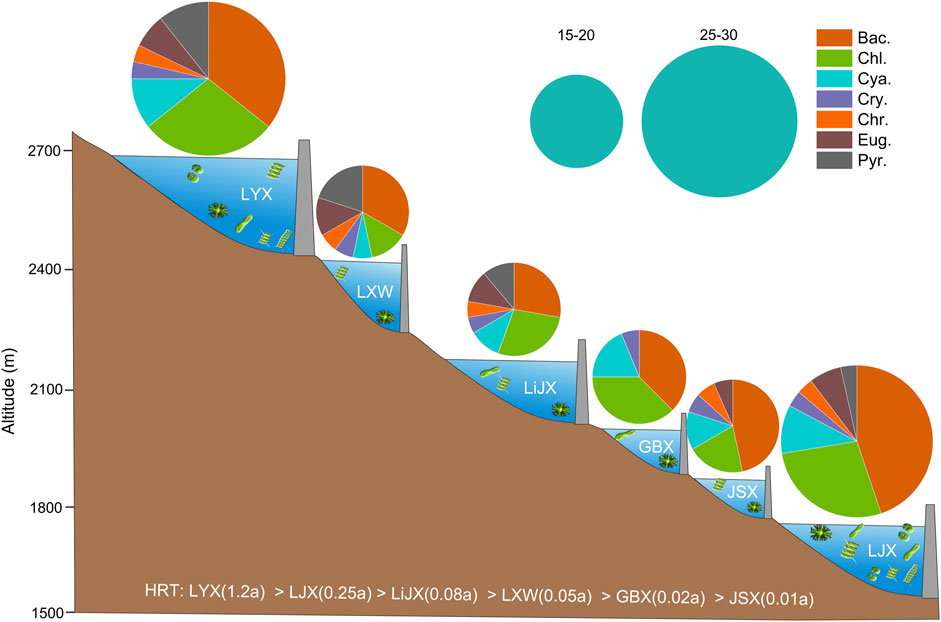
Figure 2. Illustrates the distribution of phytoplankton species in the upper Yellow River. The size of the circles represents the number of species, while the pie charts indicate the proportion of each species. Bac: Bacillariophyta; Chl, Chlorothyta; Cya, Cyanobacteria; Cry, Cryptophyta; Chr, Chrysophyta; Eug, Euglenophyta; Pyr, Pyrrothyta.
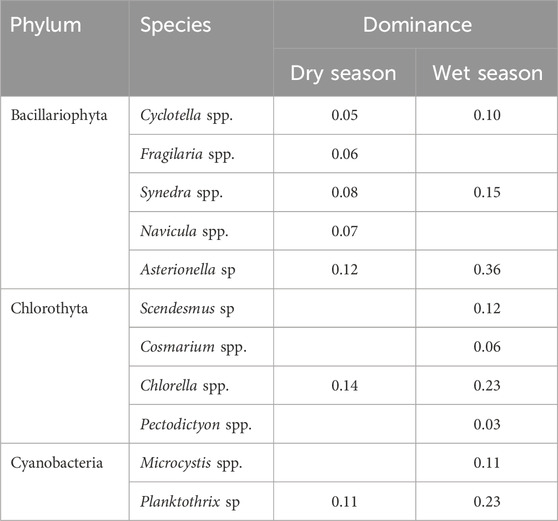
Table 2. Dominant species and dominance of phytoplankton in the cascade reservoirs of the upper Yellow River.
The chord diagram (Figure 3) illustrates that Bacillariophyta, Chlorophyta, and Cyanobacteria dominate the phytoplankton in the upper Yellow River reservoirs, accounting for over 85% of the total abundance. The seasonal variation in phytoplankton community structure in these reservoirs is pronounced and varies with different HRT levels. Specifically, from the dry to the wet season, Bacillariophyta’s relative abundance decreases significantly, while Cyanobacteria and Chlorophyta increase substantially. As HRT increases, especially during the wet season, Bacillariophyta’s relative abundance shows a clear downward trend, while Cyanobacteria and Chlorophyta show an upward trend. Particularly in the two reservoirs with longer hydraulic residence times (LJX and LYX), during the wet season, Bacillariophyta’s relative abundance decreased by 70.01% and 77.62%, respectively, while Cyanobacteria in LJX and Chlorophyta in LYX increased by 2.16 and 4.31 times, respectively (Figure 3).
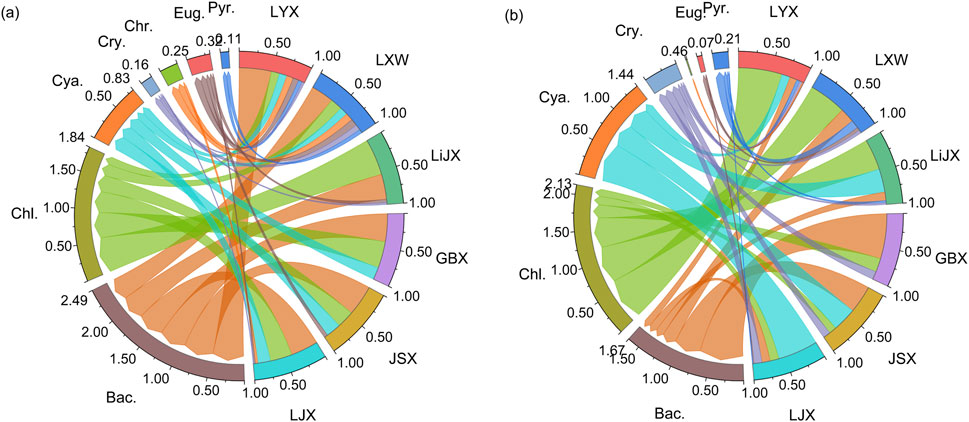
Figure 3. Differences in phytoplankton community abundance in the upper Yellow River. (a) Dry season (b) Wet season. Bac, Bacillariophyta; Chl, Chlorothyta; Cya, Cyanobacteria; Cry, Cryptophyta; Chr, Chrysophyta; Eug: Euglenophyta; Pyr, Pyrrothyta.
3.2 The relationship between phytoplankton community and HRT
The relationship between phytoplankton communities and HRT in six cascade reservoirs in the upper Yellow River is highlighted in the findings (Figure 4). It is evident that HRT significantly influences the structure of phytoplankton communities. Both during the dry and wet seasons, phytoplankton abundance, Chl-a concentration, Shannon-Wiener index, and Simpson index increase with longer HRT. Except for the Simpson index, this increasing trend shows consistent seasonal differences, with a higher rate of increase observed during the wet season compared to the dry season. This difference is particularly notable in phytoplankton abundance. Furthermore, the results indicate that HRT does not alter the characteristic where phytoplankton community structure, Chl-a concentration, and diversity indices are consistently higher during the wet season compared to the dry season in these reservoirs.
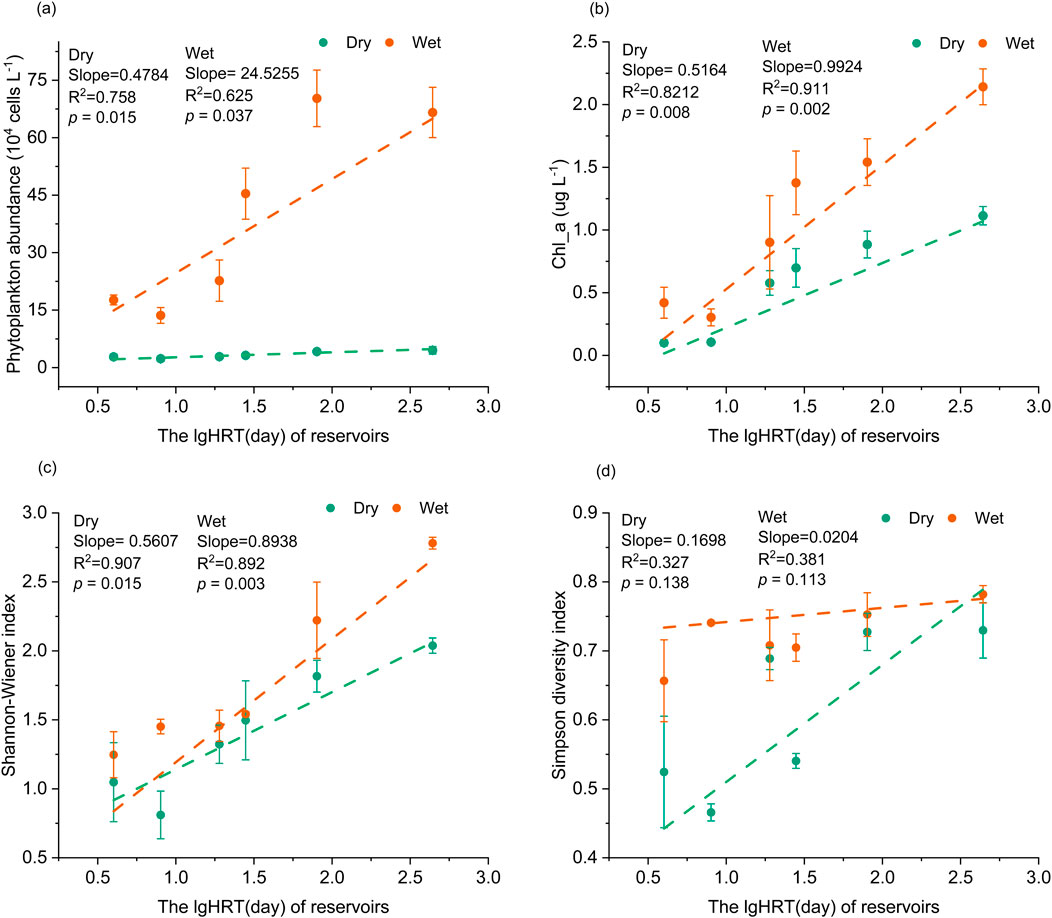
Figure 4. The impact of HRT on the phytoplankton community in the upper Yellow River. (a) The relationship between HRT and phytoplankton abundance; (b) The relationship between HRT and chlorophyll a (Chl-a) concentration (c–d) The relationship between HRT and Shannon and Simpson diversity index.
3.3 Phytoplankton community assembly
Based on the aforementioned results, it is evident that the richness and diversity of phytoplankton communities in the two annual regulation hydropower station with longer hydraulic residence times (LJX and LYX) are significantly higher compared to the runoff hydropower station. Therefore, this study utilized the NCM and MST to quantitatively the relative importance of deterministic and stochastic processes in the assembly of phytoplankton communities in these two types of reservoirs. The average Modified Stochasticity Ratio is 36.41% in annual regulation hydropower station and 45.18% in runoff hydropower station (Figure 5), both of which are below the 50% threshold. This indicates that deterministic processes predominantly govern phytoplankton community assembly in the upper Yellow River reservoirs. Moreover, as HRT increases, the influence of deterministic processes on phytoplankton community assembly also intensifies. In line with these findings, the study employed the NCM to corroborate the MST model results. The migration rate (m) in the NCM reflects the dispersal ability of species. As depicted in the NCM results (Figure 5), the migration rates (m) in annual regulation hydropower station and runoff hydropower station are 0.0010 and 0.0120, respectively, highlighting minimal migration rates for phytoplankton. This suggests that stochastic processes associated with migration and dispersal make negligible contributions to phytoplankton community assembly. Furthermore, the Neutral Community Model explains 20.1% and 46.3% of phytoplankton community variability in annual regulation hydropower station and runoff hydropower station, respectively. This underscores that with increasing HRT, deterministic processes exert a more pronounced influence on phytoplankton community assembly, consistent with the analysis of MST.
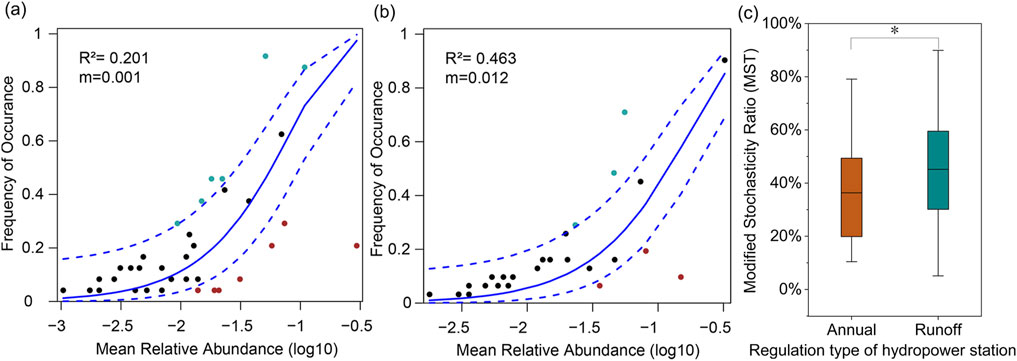
Figure 5. Illustrates the Neutral Community Model of phytoplankton communities in the Long-Liu section of the upper Yellow River cascade reservoirs. Species with frequencies higher than the model-predicted values are depicted in green, those with lower frequencies in red, and species falling within the predicted range in black. The solid blue line represents the optimal fit of the Neutral Community Model, while the dashed blue lines indicate the model’s 95% confidence intervals. R2 indicates the overall goodness of fit of the Neutral Community Model, and m denotes the migration rate of phytoplankton communities. (a) Annual regulation hydropower station; (b) Runoff hydropower station; (c) MST for annual regulation hydropower station and runoff hydropower station.
3.4 The relationship between phytoplankton and environmental factors
The Mantel test was employed to analyze the significant correlations between phytoplankton communities and environmental factors in different types of reservoirs (Figures 6a, b). In annual regulation hydropower station, the phytoplankton community structure showed a highly significant correlation with WT, TN, COD, and pH (Supplementary Table S3, P < 0.001), and a significant correlation with NO3−-N, EC, salt, and HRT (P < 0.05). In runoff hydropower station, changes in the phytoplankton community structure were highly significantly correlated with WT and salt (P < 0.001), and significantly correlated with NO3−-N, COD, DO, and HRT (P < 0.05). Employing stepwise multiple regression analysis, this study discerned that phytoplankton abundance within the cascade reservoirs of the upper Yellow River is significantly swayed by HRT, WT and TN than by other environmental factors (Abundance = 2.8919×TN+1.2504×WT+0.1614×HRT, R2 = 0.6121, 3.52 × 10−11). Specifically, as HRT, WT and TN increase, the abundance of phytoplankton significantly rises.
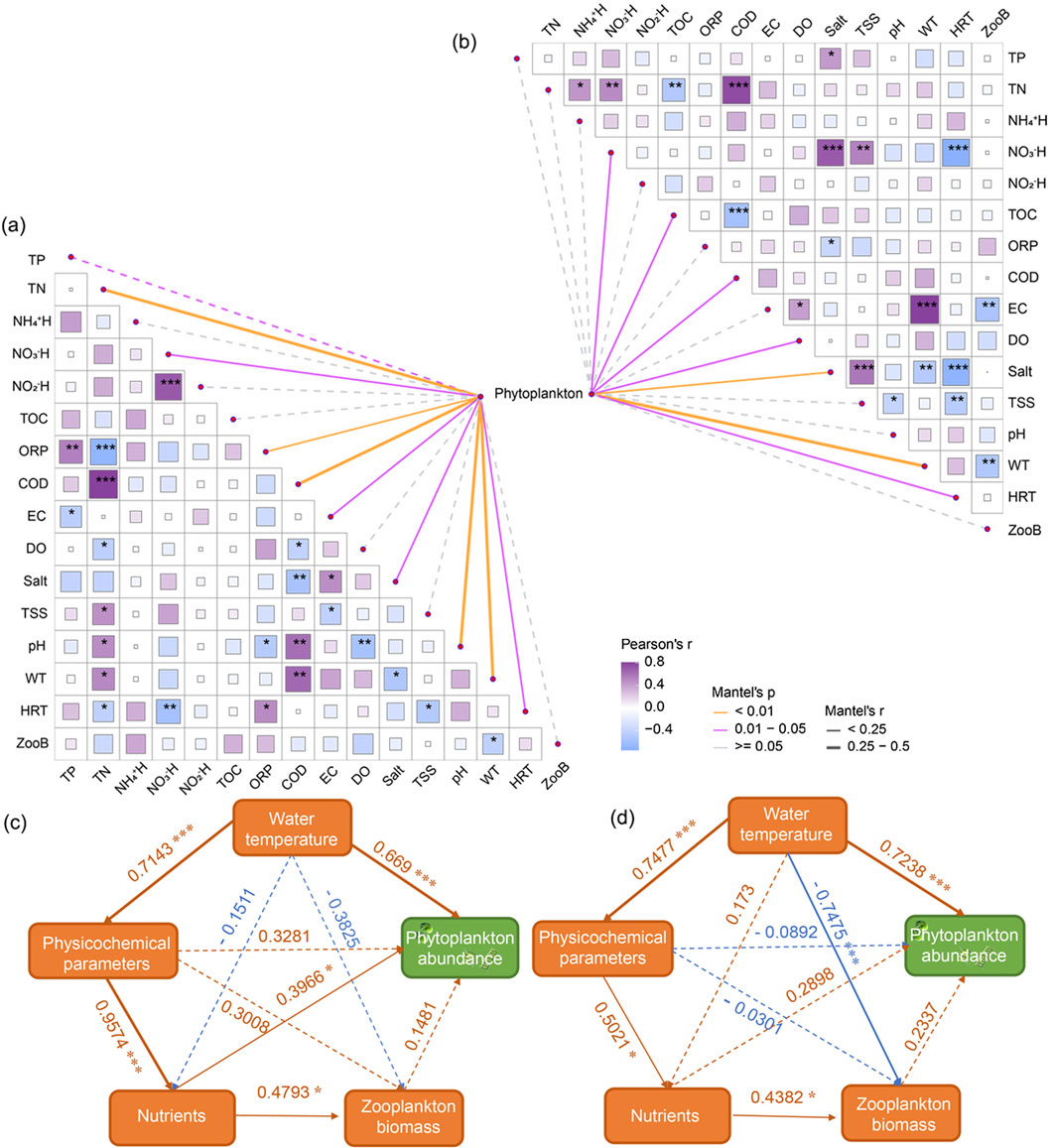
Figure 6. Analysis of the correlation between the phytoplankton community and environmental factors. (a) The relationship between phytoplankton and environmental factors in annual regulation hydropower station; (b) The relationship between phytoplankton and environmental factors in runoff hydropower stations; (c) Partial Least Squares Path Modeling (PLS-PM) analysis results for annual regulation hydropower station; (d) PLS-PM analysis results for runoff hydropower stations. Parameters included: WT, phytoplankton abundance, zooplankton biomass, nutrient parameters, and physicochemical parameters. Nutrient parameters included: TN, NO2−-N, NO3−-N, NH4+-N, TP and TOC. Physicochemical parameters included: pH, ORP, DO, EC, Salt, TSS, and COD. Solid lines in (a, b) indicate significant correlations (P < 0.05), while dashed lines indicate non-significant correlations (P > 0.05).
Based on the results of the Mantel test and multiple regression analysis, WT and nutrients are key factors influencing the structure of phytoplankton. Consequently, we established a partial least squares path model (PLS-PM) that includes phytoplankton abundance, zooplankton biomass, and environmental factors to analyze the effects of upstream influences (environmental factors) and downstream influences (zooplankton predation) on phytoplankton abundance (Figure 6). In both annual regulation hydropower station or runoff hydropower station, phytoplankton abundance is significantly positively correlated with water temperature, with this influence being stronger in runoff hydropower station. Additionally, zooplankton and nutrients are positively correlated with phytoplankton abundance. In runoff hydropower station, the predation effect of zooplankton on phytoplankton abundance is more pronounced. However, compared to annual regulation hydropower station, the upstream effect of nutrients on phytoplankton abundance is less significant in runoff hydropower station. Further analysis indicates that under two different HRT conditions, the roles of physicochemical parameters on phytoplankton show significant differences. In annual regulation hydropower station, these parameters exhibit a strong positive influence, whereas in runoff hydropower station, they show a weaker negative influence.
4 Discussion
4.1 The impact of HRT on phytoplankton communities in cascade reservoirs
HRT is one of the key hydrological characteristics of reservoirs and has been shown to play a significant role in shaping phytoplankton communities (Ruan et al., 2024; Yin et al., 2024). This study demonstrates that there are significant differences in the phytoplankton community structures in the cascade reservoirs of the upper Yellow River, with reservoirs having longer HRT exhibiting higher species richness and abundance. The mechanisms underlying this relationship can be attributed to several factors. Firstly, reservoirs with longer HRT have larger water surface areas and greater light penetration, creating favorable conditions for phytoplankton photosynthesis. Secondly, extended HRT increases the interaction time between phytoplankton and available nutrients, thereby enhancing primary productivity and nutrient cycling within the reservoir (Chen et al., 2020; Znachor et al., 2020; Zhao et al., 2024). Thirdly, prolonged HRT promotes nutrient deposition, facilitating nutrient retention in reservoirs and lakes (Maavara et al., 2020; Wu et al., 2022). Notably, when HRT exceeds 5.3 days, the nutrient retention capacity of reservoirs significantly increases (Zhao et al., 2024), further supporting phytoplankton growth. In this study, except for the JSX reservoir, all other reservoirs had HRT exceeding 5.3 days. Among them, the annual regulation reservoirs, LJX and LYX, exhibited exceptionally long HRTs of 80 days and 440 days, respectively—substantially higher than the critical threshold identified by Zhao et al. (2024). The prolonged water retention in these reservoirs allows for greater nutrient accumulation, providing ample resources for phytoplankton development and reproduction. This may explain the higher species richness observed in LYX and LJX compared to other reservoirs.
Beyond its direct impact on nutrient retention, HRT also indirectly shapes phytoplankton community dynamics by influencing water quality. This study found a significant positive correlation between HRT and phytoplankton community structure (Figure 4), as well as with TN, NO3−-N, salt, TSS, and ORP (Figure 6). This suggests that HRT indirectly affects phytoplankton nutrient uptake by regulating complex physical, chemical, and biological processes. Longer HRT enhances the retention of nutrients and particulates (Zhao et al., 2024), improves water transparency, prolongs the interaction time between phytoplankton and nutrients, and strengthens internal nutrient cycling. Furthermore, extended HRT increases the interaction time between phytoplankton and key environmental factors such as nutrient availability and water temperature, thereby influencing their growth patterns and competitive relationships (Yin et al., 2024). Generally, longer HRT is associated with higher phytoplankton abundance and biomass, leading to significant shifts in community structure (Li et al., 2013; Znachor et al., 2020; Liu et al., 2021). These combined factors enable phytoplankton to proliferate rapidly during wet seasons, increasing the risk of eutrophication in reservoirs. Therefore, optimizing reservoir operation to reduce HRT and enhance water exchange can effectively curb excessive algal growth, while releasing ecological flows and flood pulses to lower HRT can increase downstream flow velocity, disrupting algal aggregation and inhibiting their growth. Based on these findings, proper HRT management could be a crucial strategy for controlling phytoplankton overgrowth and maintaining water quality in reservoir ecosystems.
4.2 Environmental drivers of phytoplankton communities in cascade reservoirs
The Long–Liu section is located at the edge of the Qinghai-Tibet Plateau and represents a typical plateau alpine river. Due to the influence of high altitude, this region experiences persistently low temperatures, which significantly inhibit phytoplankton growth, resulting in a strong correlation between altitude and species richness (Ding et al., 2021; Song et al., 2020). Studies have shown that the community characteristics in this area are similar to those of other cold alpine rivers and reservoirs, with diatoms as the dominant group (Liu et al., 2021). The phytoplankton community composition is relatively simple, with low species richness. A total of 38 species were identified, which is significantly lower than those found in downstream river channels and reservoirs at lower altitudes with higher water temperatures (Song et al., 2020; Ding et al., 2021). During the dry season, diatoms were the dominant group, reflecting their adaptation to low-temperature environments (Liu et al., 2021), with Cyclotella spp., Fragilaria spp., Synedra spp., Navicula spp., and Asterionella sp. as the dominant species. In the wet season, the dominant species shifted to Scendesmus sp., Cosmarium spp., Chlorella spp., and Pectodictyon spp. from the Chlorophyta, as well as Microcystis spp. and Chlorella spp. from the Cyanophyta. Compared to Bacillariophyta, Chlorothyta and Cyanobacteria are more adapted to high-temperature environments (Chen, 2015; Baker and Geider, 2021). During the wet season, the highest recorded water temperature reached 19.35°C, significantly higher than in the dry season (Supplementary Table S1, S2). This temperature increase created favorable conditions for the proliferation of cyanobacteria and green algae, accelerating their growth and enhancing their competitive advantage over other phytoplankton groups. Consequently, the species richness, abundance, and dominance of cyanobacteria and green algae were significantly higher in the wet season than in the dry season.
The stepwise multiple regression analysis showed that as WT, TN, and HRT increased, the abundance of phytoplankton significantly increased. WT not only directly influences the growth, metabolism, and life cycle of phytoplankton but also indirectly affects their development and reproduction by regulating nutrient decomposition (Ruan et al., 2024). The PLS-PM results further confirmed a stable and significant positive correlation between phytoplankton abundance and WT. However, in annually regulated hydropower stations (LYX and LJX), the influence of WT on phytoplankton communities was weaker compared to runoff-type hydropower stations (LXW, LiJX, GBX, and JSX). This may be attributed to the longer HRT and larger water surface areas of LYX and LJX, which enhance light-heat exchange and result in relatively higher water temperatures. Consequently, the water temperatures in Longyangxia and Liujiaxia reservoirs were notably higher than in other reservoirs, creating more favorable conditions for phytoplankton growth. Additionally, elevated WT may accelerate nutrient mineralization, increasing the availability of essential nutrients for phytoplankton uptake (Zhang et al., 2024), leading to significantly higher algal density and biomass in Longyangxia and Liujiaxia reservoirs compared to other reservoirs. As the largest annually regulated reservoir in the upper Yellow River, the outflow from Longyangxia Reservoir has substantially altered the interannual variation in downstream water temperatures (Ren et al., 2020). Meanwhile, the cumulative effects of cascade reservoirs have exacerbated the negative impacts of temperature fluctuations on phytoplankton survival and reproduction (Liu et al., 2021). Therefore, optimizing reservoir operation strategies to minimize WT fluctuations and mitigate their adverse effects on downstream aquatic ecosystems is crucial for maintaining ecological balance.
Nitrogen is an essential nutrient for phytoplankton growth and a key environmental factor influencing phytoplankton community structure (Reynolds et al., 2006). In the cascade reservoirs of the upper Yellow River, phytoplankton communities exhibit a significant positive correlation with total nitrogen (TN) concentration, likely because higher TN levels promote the proliferation of diatoms and green algae. These groups have high nutrient utilization efficiency, allowing them to gain a competitive advantage in resource competition, dominate ecological niches, and suppress the growth of other algae (Zhang et al., 2024; Ruan et al., 2024). TN is significantly correlated with NO3−-N, NH4+-N, COD, ORP, TSS, pH, WT, and HRT. These environmental factors regulate TN transformation through physical and chemical processes such as sedimentation, nitrification, and denitrification, directly or indirectly altering its bioavailability and influencing phytoplankton nitrogen uptake efficiency (Reynolds et al., 2006). Consequently, this affects their growth and reproduction. Given that phytoplankton are a fundamental resource in the food web, their unhealthy growth may ultimately disrupt the structure and function of the entire ecosystem. Therefore, it is crucial to appropriately adjust hydraulic residence time and adequately regulate nutrient loads and water temperature to ensure the healthy growth of aquatic organisms in reservoirs and downstream rivers.
4.3 The importance of HRT in the construction of phytoplankton communities
The NCM, grounded in neutral theory, is used to infer the roles of stochastic and deterministic processes in community assembly. It has been widely applied in microbial community studies (Dini-Andreote et al., 2015; Yang et al., 2023). In recent years, NCM has also been employed in phytoplankton community research (Liu et al., 2024; Zhang et al., 2024). However, whether the assembly of phytoplankton communities in cascade reservoirs of alpine rivers is primarily driven by deterministic or stochastic processes remains unclear (Yang et al., 2023; Sun et al., 2023). The results of this study indicate that deterministic processes dominate the assembly of phytoplankton communities in the upper Yellow River cascade reservoirs (Figure 5), with a particularly strong influence observed in annually regulated hydropower stations.
Specifically, the results of the NCM show that the explanatory power of the model (R2 = 0.463) and migration rate (m = 0.012) for phytoplankton communities in runoff hydropower stations are higher than those in annually regulated hydropower stations (R2 = 0.201, m = 0.0010). Additionally, the MST for phytoplankton in runoff hydropower station is 45.18%, higher than the 36.41% observed in annually regulated hydropower stations. These findings suggest that phytoplankton community assembly in annually regulated hydropower stations is more influenced by deterministic processes compared to runoff hydropower stations. This conclusion aligns with previous research in stepped reservoirs in plains, where phytoplankton community assembly is also primarily shaped by deterministic processes (Isabwe et al., 2022).
The differences in phytoplankton community assembly between annually regulated and runoff-type hydropower stations may be attributed to their respective hydrological characteristics. Runoff-type hydropower stations have a higher water exchange frequency and shorter hydraulic residence time, which enhance connectivity between reservoirs and reduce isolation effects. These hydrological conditions favor increased migration and dispersal of phytoplankton (Liu et al., 2024; Isabwe et al., 2022), potentially explaining why deterministic processes play a lesser role in runoff hydropower stations and a more prominent role in annually regulated hydropower stations.
Similarly, in large lakes with good connectivity, such as Dongting Lake, stochastic processes dominate phytoplankton community assembly (Wu et al., 2022). In contrast, the spatial isolation and limited connectivity of the annually regulated hydropower station at the head and tail of the study area make phytoplankton dispersal more challenging (Zhang et al., 2024). In single-stage reservoirs, the assembly of phytoplankton communities is also dominated by stochastic processes (Liu et al., 2024). The large storage capacity and weak hydrodynamic conditions of annual regulation hydropower stations (Zhao et al., 2024) reduce the ability of phytoplankton to migrate and disperse with water flow. Furthermore, the longer water exchange period and hydraulic residence time increase the interaction time between phytoplankton and environmental factors, thereby strengthening the influence of deterministic processes. While stochastic processes may dominate in lakes (such as Lake Dongting) (Wu et al., 2021) or single-stage reservoirs (Liu et al., 2024), in the upper Yellow River, phytoplankton community assembly is mainly governed by deterministic processes, particularly in annual regulation hydropower stations, where deterministic processes have a greater impact on community assembly than in runoff hydropower stations.
5 Conclusion
This study systematically investigates the spatial distribution patterns and community assembly types of phytoplankton communities in reservoirs with varying HRT. Significant seasonal differences in phytoplankton composition were observed, with these variations differing under different HRT levels. Notably, the richness and diversity of phytoplankton communities in annual regulation hydropower station were much higher than in runoff hydropower stations. From the dry season to the wet season, the relative abundance of diatoms significantly decreased, while the relative abundance of cyanobacteria and green algae greatly increased. In the wet season, the dominant species shifted to Scendesmus sp., Cosmarium spp., Chlorella spp., and Pectodictyon spp. from the Chlorophyta, as well as Microcystis spp. and Chlorella spp. from the Cyanophyta. The neutral community model results indicate that phytoplankton community assembly in upstream cascade reservoirs is primarily governed by deterministic processes. Furthermore, as HRT increases, the influence of these deterministic processes becomes more pronounced, suggesting that in annually regulated hydropower stations, deterministic processes play a more dominant role in community assembly compared to runoff hydropower stations. Stepwise multiple regression analysis further reveals that HRT, WT, and TN are the primary environmental factors driving variations in phytoplankton community structure. Phytoplankton abundance is significantly positively correlated with water temperature, particularly in runoff hydropower stations, where its influence is more pronounced. This study reveals the structure, aggregation characteristics, and influencing factors of phytoplankton communities in the upstream cascade reservoirs of the Yellow River under different HRT conditions, thereby deepening our understanding of the assembly processes of river phytoplankton communities.
Data availability statement
The original contributions presented in the study are included in the article/Supplementary Material, further inquiries can be directed to the corresponding author.
Author contributions
QL: Conceptualization, Data curation, Methodology, Software, Visualization, Writing – original draft. LZ: Investigation, Methodology, Validation, Writing – review and editing. DL: Validation, Writing – review and editing. ZZ: Investigation, Writing – review and editing. KC: Investigation, Writing – review and editing. JW: Investigation, Writing – review and editing. YY: Conceptualization, Methodology, Funding acquisition, Investigation, Project administration, Writing – review and editing.
Funding
The author(s) declare that financial support was received for the research and/or publication of this article. This research was supported by the Joint Funds of the National Natural Science Foundation of China (U2243236), the Innovative Research Group of the National Natural Science Foundation of China (52221003), and National Science Fund for Distinguished Young Scholars (52025092).
Conflict of interest
The authors declare that the research was conducted in the absence of any commercial or financial relationships that could be construed as a potential conflict of interest.
Generative AI statement
The author(s) declare that no Generative AI was used in the creation of this manuscript.
Publisher’s note
All claims expressed in this article are solely those of the authors and do not necessarily represent those of their affiliated organizations, or those of the publisher, the editors and the reviewers. Any product that may be evaluated in this article, or claim that may be made by its manufacturer, is not guaranteed or endorsed by the publisher.
Supplementary material
The Supplementary Material for this article can be found online at: https://www.frontiersin.org/articles/10.3389/fenvs.2025.1551988/full#supplementary-material
References
Baker, K., and Geider, R. (2021). Phytoplankton mortality in a changing thermal seascape. Glob. Change Biol. 27 (20), 5253–5261. doi:10.1111/gcb.15772
Belletti, B., Garcia de Leaniz, C., Jones, J., Bizzi, S., Börger, L., Segura, G., et al. (2020). More than one million barriers fragment Europe’s rivers. Nature 588 (7838), 436–441. doi:10.1038/s41586-020-3005-2
Chen, B. (2015). Patterns of thermal limits of phytoplankton. J. Plankton Res. 37 (2), 285–292. doi:10.1093/plankt/fbv009
Chen, Q., Shi, W., Huisman, J., Maberly, S. C., Zhang, J., Yu, J., et al. (2020). Hydropower reservoirs on the upper Mekong River modify nutrient bioavailability downstream. Natl. Sci. Rev. 7, 1449–1457. doi:10.1093/nsr/nwaa026
Datry, T., Boulton, A., Fritz, K., Stubbington, R., Cid, N., Crabot, J., et al. (2023). Non-perennial segments in river networks. Nat. Rev. Earth and Environ. 4 (12), 815–830. doi:10.1038/s43017-023-00495-w
Ding, Y., Pan, B., Zhao, G., Sun, C., Han, X., and Li, M. (2021). Geo-climatic factors weaken the effectiveness of phytoplankton diversity as a water quality indicator in a large sediment-laden river. Sci. Total Environ. 792, 148346. doi:10.1016/j.scitotenv.2021.148346
Dini-Andreote, F., Stegen, J. C., Van Elsas, J. D., and Salles, J. F. (2015). Disentangling mechanisms that mediate the balance between stochastic and deterministic processes in microbial succession. Proc. Natl. Acad. Sci. 112 (11), E1326–E1332. doi:10.1073/pnas.1414261112
Grill, G., Lehner, B., Thieme, M., Geenen, B., Tickner, D., Antonelli, F., et al. (2019). Mapping the world’s free-flowing rivers. Nature 569 (7755), 215–221. doi:10.1038/s41586-019-1111-9
He, F., Zarfl, C., Tockner, K., Olden, J. D., Campos, Z., Muniz, F., et al. (2024). Hydropower impacts on riverine biodiversity. Nat. Rev. Earth and Environ. 5, 755–772. doi:10.1038/s43017-024-00596-0
Hillebrand, H., Dürselen, C. D., Kirschtel, D., Pollingher, U., and Zohary, T. (1999). Biovolume calculation for pelagic and benthic microalgae. J. Phycol. 35 (2), 403–424. doi:10.1046/j.1529-8817.1999.3520403.x
ICOLD, (2023). World Register of Dams: General Synthesis. Available online at: https://www.icold-cigb.org/GB/world_register/general_synthesis.asp (December, 2023)
Isabwe, A., Yang, J., Wang, Y., Wilkinson, D., Graham, E., Chen, H., et al. (2022). Riverine bacterioplankton and phytoplankton assembly along an environmental gradient induced by urbanization. Limnol. Oceanogr. 67 (9), 1943–1958. doi:10.1002/lno.12179
Jia, Y., Kennard, M. J., Liu, Y., Sui, X., Li, K., Wang, G., et al. (2020). Human disturbance and long-term changes in fish taxonomic, functional and phylogenetic diversity in the Yellow River, China. Hydrobiologia 847 (18), 3711–3725. doi:10.1007/s10750-020-04244-8
Jiao, S., Yang, Y., Xu, Y., Zhang, J., and Lu, Y. (2020). Balance between community assembly processes mediates species coexistence in agricultural soil microbiomes across eastern China. ISME J. 14 (1), 202–216. doi:10.1038/s41396-019-0522-9
Lampitt, R. S., Wishner, K. F., Turley, C. M., and Angel, M. V. (1993). Marine snow studies in the Northeast Atlantic Ocean: distribution, composition and role as a food source for migrating plankton. Mar. Biol. 116 (4), 689–702. doi:10.1007/BF00355486
Li, J., Dong, S., Liu, S., Yang, Z., Peng, M., and Zhao, C. (2013). Effects of cascading hydropower dams on the composition, biomass and biological integrity of phytoplankton assemblages in the middle Lancang-Mekong River. Ecol. Eng. 60, 316–324. doi:10.1016/j.ecoleng.2013.07.029
Li, X., Chen, Z., Fan, X., and Cheng, Z. (2018). Hydropower development situation and prospects in China. Renew. Sustain. Energy Rev. 82, 232–239. doi:10.1016/j.rser.2017.08.090
Liu, X., Song, Y., Ni, T., Yang, Y., Ma, B., Huang, T., et al. (2024). Ecological evolution of algae in connected reservoirs under the influence of water transfer: algal density, community structure, and assembly processes. Sci. Total Environ. 915, 170086. doi:10.1016/j.scitotenv.2024.170086
Liu, Y., Li, C., Jian, S., Miao, S., Li, K., Guan, H., et al. (2021). Hydrodynamics regulate longitudinal plankton community structure in an alpine cascade reservoir system. Front. Microbiol. 12, 749888. doi:10.3389/fmicb.2021.749888
Maavara, T., Chen, Q., Van Meter, K., Brown, L., Zhang, J., Ni, J., et al. (2020). River dam impacts on biogeochemical cycling. Nat. Rev. Earth and Environ. 1 (2), 103–116. doi:10.1038/s43017-019-0019-0
Mor, J. R., Ruhí, A., Tornés, E., Valcárcel, H., Muñoz, I., and Sabater, S. (2018). Dam regulation and riverine food-web structure in a Mediterranean river. Sci. Total Environ. 625, 301–310. doi:10.1016/j.scitotenv.2017.12.296
Ning, D., Deng, Y., Tiedje, J., and Zhou, J. (2019). A general framework for quantitatively assessing ecological stochasticity. Proc. Natl. Acad. Sci. 116 (34), 16892–16898. doi:10.1073/pnas.1904623116
Ren, L., Song, C., Wu, W., Guo, M., and Zhou, X. (2020). Reservoir effects on the variations of the water temperature in the upper Yellow River, China, using principal component analysis. J. Environ. Manag. 262, 110339. doi:10.1016/j.jenvman.2020.110339
Reynolds, C. S. (2006). The ecology of phytoplankton. Camb. Univ. Press. doi:10.1017/CBO9780511542145
Ruan, Q., Liu, H., Dai, Z., Wang, F., and Cao, W. (2024). Damming exacerbates the discontinuities of phytoplankton in a subtropical river in China. J. Environ. Manag. 351, 119832. doi:10.1016/j.jenvman.2023.119832
Salmaso, N., and Tolotti, M. (2021). Phytoplankton and anthropogenic changes in pelagic environments. Hydrobiologia 848 (1), 251–284. doi:10.1007/s10750-020-04323-w
SEPA (2002). Environmental quality standard for surface water (GB3838-2002) state environmental protection administration of China, Beijing of regula (in Chinese). Beijing: China Environmental Science Press, 1–10.
Shannon, C. E., and Tolotti, M. (1948). A mathematical theory of communication. Bell Syst. Tech. J. 27 (3), 379–423. doi:10.1002/j.1538-7305.1948.tb01338.x
Shen, Y., Zhang, Y., Zhou, X., Li, Q., Zhang, J., Cheng, R., et al. (2024). Environmental DNA metabarcoding revealing the distinct responses of phytoplankton and zooplankton to cascade dams along a river-way. Ecol. Indic. 166, 112545. doi:10.1016/j.ecolind.2024.112545
Sloan, W., Lunn, M., Woodcock, S., Head, I., Nee, S., and Curtis, T. (2006). Quantifying the roles of immigration and chance in shaping prokaryote community structure. Environ. Microbiol. 8 (4), 732–740. doi:10.1111/j.1462-2920.2005.00956.x
Song, J., Hou, C., Liu, Q., Wu, X., Wang, Y., and Yi, Y. (2020). Spatial and temporal variations in the plankton community because of water and sediment regulation in the lower reaches of Yellow River. J. Clean. Prod. 261, 120972. doi:10.1016/j.jclepro.2020.120972
Sun, Y., Li, H., Wang, X., Jin, Y., Nagai, S., and Lin, S. (2023). Phytoplankton and microzooplankton community structure and assembly mechanisms in northwestern pacific ocean estuaries with environmental heterogeneity and geographic segregation. Microbiol. Spectr. 11 (2), e0492622–22. doi:10.1128/spectrum.04926-22
Wang, J., Wu, W., Zhou, X., and Li, J. (2023). Carbon dioxide (CO2) partial pressure and emission from the river-reservoir system in the upper Yellow River, northwest China. Environ. Sci. Pollut. Res. 30 (7), 19410–19426. doi:10.1007/s11356-022-23489-5
Wang, X., Chen, Y., Yuan, Q., Xing, X., Hu, B., Gan, J., et al. (2022). Effect of river damming on nutrient transport and transformation and its countermeasures. Front. Mar. Sci. 9, 1078216. doi:10.3389/fmars.2022.1078216
Weng, J., and Xu, H. (2010). Atlas of Common freshwater planktonic algae in China. Shanghai, China: Shanghai Scientific and Technical Publishers. (in Chinese).
Wu, N., Liu, S. M., Zhang, G. L., and Zhang, H. M. (2021). Anthropogenic impacts on nutrient variability in the lower Yellow River. Sci. Total Environ. 755, 142488. doi:10.1016/j.scitotenv.2020.142488
Wu, Z., Li, J., Sun, Y., Peñuelas, J., Huang, J., Sardans, J., et al. (2022). Imbalance of global nutrient cycles exacerbated by the greater retention of phosphorus over nitrogen in lakes. Nat. Geosci. 15 (6), 464–468. doi:10.1038/s41561-022-00958-7
Xiao, Z., Li, H., Li, X. C., Li, R. H., Huo, S. L., Yu, G. L., et al. (2022). Geographic pattern of phytoplankton community and their drivers in lakes of middle and lower reaches of Yangtze River floodplain, China. Environ. Sci. Pollut. Res. 29 (55), 83993–84005. doi:10.1007/s11356-022-21657-1
Xu, M., Wang, G., Wang, Z., Hu, H., Singh, D. K., and Tian, S. (2022). Temporal and spatial hydrological variations of the Yellow River in the past 60 years. J. Hydrology 609, 127750. doi:10.1016/j.jhydrol.2022.127750
Xu, S., Li, S. L., Bufe, A., Klaus, M., Zhong, J., Wen, H., et al. (2024). Escalating carbon export from high-elevation rivers in a warming climate. Environ. Sci. and Technol. 58 (16), 7032–7044. doi:10.1021/acs.est.3c06777
Yang, Q., Zhang, P., Li, X., Yang, S., Chao, X., Liu, H., et al. (2023). Distribution patterns and community assembly processes of eukaryotic microorganisms along an altitudinal gradient in the middle reaches of the Yarlung Zangbo River. Water Res. 239, 120047. doi:10.1016/j.watres.2023.120047
Yi, Y., Zhao, F., Liu, Q., and Song, J. (2023). Runoff from upstream changes the structure and energy flow of food web in estuary. Front. Mar. Sci. 10, 1103502. doi:10.3389/fmars.2023.1103502
Yin, Q., Lin, F., Xu, B., Jiang, X., Li, H., Xian, H., et al. (2024). Unveiling the dominant influence of reservoir characteristics over water quality on algal concentrations revealed by qPCR detection. ACS ES&T Water 4, 4299–4311. doi:10.1021/acsestwater.3c00831
Zeng, H., Song, L., Yu, Z., and Chen, H. (2006). Distribution of phytoplankton in the Three-Gorge Reservoir during rainy and dry seasons. Sci. Total Environ. 367 (2-3), 999–1009. doi:10.1016/j.scitotenv.2006.03.001
Zhang, H., Xu, Y., Liu, X., Ma, B., Huang, T., Kosolapov, D. B., et al. (2024). Different seasonal dynamics, ecological drivers, and assembly mechanisms of algae in southern and northern drinking water reservoirs. Sci. Total Environ. 922, 171285. doi:10.1016/j.scitotenv.2024.171285
Zhang, H., Zong, R., He, H., Liu, K., Yan, M., Miao, Y., et al. (2021). Biogeographic distribution patterns of algal community in different urban lakes in China: insights into the dynamics and co-existence. J. Environ. Sci. 100, 216–227. doi:10.1016/j.jes.2020.07.024
Zhao, B., Zeng, Q., Wang, J., Jiang, Y., Liu, H., Yan, L., et al. (2024). Impact of cascade reservoirs on nutrients transported downstream and regulation method based on hydraulic retention time. Water Res. 252, 121187. doi:10.1016/j.watres.2024.121187
Keywords: phytoplankton, community assembly, hydraulic residence time, cascade reservoir, the upper yellow river
Citation: Luo Q, Zhu L, Li D, Zu Z, Chen K, Wang J and Yi Y (2025) Role of hydraulic residence time in shaping phytoplankton community assembly in the upper yellow river cascade reservoirs. Front. Environ. Sci. 13:1551988. doi: 10.3389/fenvs.2025.1551988
Received: 31 December 2024; Accepted: 24 March 2025;
Published: 09 April 2025.
Edited by:
Qinghua Cai, Chinese Academy of Sciences (CAS), ChinaReviewed by:
Yuying Li, Nanyang Normal University, ChinaFangyan Cheng, Zhejiang Agriculture and Forestry University, China
Copyright © 2025 Luo, Zhu, Li, Zu, Chen, Wang and Yi. This is an open-access article distributed under the terms of the Creative Commons Attribution License (CC BY). The use, distribution or reproduction in other forums is permitted, provided the original author(s) and the copyright owner(s) are credited and that the original publication in this journal is cited, in accordance with accepted academic practice. No use, distribution or reproduction is permitted which does not comply with these terms.
*Correspondence: Yujun Yi, eWl5dWp1bkBibnUuZWR1LmNu