- 1Department of Soil, Water, and Ecosystem Sciences, Everglades Research and Education Center, University of Florida, Belle Glade, FL, United States
- 2School of Forest, Fisheries, and Geomatics Sciences, University of Florida, Gainesville, FL, United States
- 3American Farmland Trust, Washington, DC, United States
- 4Florida Sea Grant, University of Florida/IFAS, Gainesville, FL, United States
- 5Department of Microbiology and Cell Science, Fort Lauderdale Research and Education Center, Institute of Food and Agricultural Sciences, University of Florida, Davie, FL, United States
Soil Organic Matter (SOM) decomposition, vital to the carbon cycle, is influenced by land cover, hydrological conditions, and soil properties. However, understanding of how hydrolytic enzymes involved in SOM turnover vary under these factors remains limited. To address this, a study was conducted in a sub-tropical preserve in South Florida to assess hydrolytic enzyme activities across 23 diverse land covers (Categorized into five ecosystems: A-Upland Forests, B-Wetland ecosystems, C-Shrub ecosystems, D-Range Areas, and E-Barren ecosystems) during wet and dry seasons. The assessed enzymes were β-1,4 glucosidase (βG), β-1,4-N-acetyl glucosaminidase (β-NAG), Acid Phosphatase (AP), and Aryl Sulfatase (AS). A weighted index termed the Hydrolytic Enzyme Decomposition Indicator (HEDI) was derived using principal component analysis to summarize overall enzymatic activity as an indicator of decomposition. The results showed that among the land covers, βG, β-NAG, AP, and AS activities during the dry season ranged from 18.40 to 327.20, 14.71–351.90, 302.89–10,185.80, and 26.51–1,745.75 μg PNP/g soil/hr, respectively, while in the wet season, the activities for all enzymes except AS were higher, ranging from 4.08 to 398.66, 21.72–1,118.97, 372.38–11,960.36, and 28.26–1,475.09 μg PNP/g soil/hr. Among ecosystems, βG and β-NAG showed seasonal variability, with β-NAG consistently higher in A-Upland Forests, B-Range Areas, and C-Shrub. AP and AS showed minimal variation, with all enzymes showing lower activity in D-Barren ecosystems. HEDI values in the dry season A-Upland Forests exhibited the widest range (−0.962–1.613), indicating diverse decomposition rates, while Barren ecosystems showed consistently low activity (−0.928 to −0.916), suggesting lower decomposition. Correlation analysis revealed positive relationships between enzymatic activities and soil properties such as SOM (0.51–0.59), active carbon (0.46–0.58), soil protein (0.27–0.40), and cation exchange capacity (0.28–0.40), while bulk density showed negative correlations (−0.31 to −0.50). Overall, this study highlights the necessity of considering the complex interactions between soil properties, vegetation, moisture, and enzymatic activity in understanding SOM decomposition.
1 Introduction
Soil Organic Matter (SOM) is an essential component of soil (Osman and Osman, 2013; Körschens, 2002) undergoing several transformative processes, including mineralization, immobilization, and decomposition (Filipović et al., 2024; Paul, 2016). Each of these processes plays a crucial role in the functioning of soil ecosystems, directly influencing soil fertility, structure, and overall soil health (Murphy, 2015; Fageria, 2012). Mineralization is the process through which soil microbes convert SOM releasing inorganic forms of nutrients and making them available to plants. Immobilization on the other hand is the process, where inorganic forms of nutrients are converted back into organic forms, making them temporarily unavailable to plants (Mohammadi et al., 2011; Nieder and Benbi, 2008; Rice, 2002; Haynes, 1986). Decomposition which involves the breakdown of SOM by soil microorganisms is considered the most crucial process within the soil because it directly influences both mineralization and immobilization (Carter and Tibbett, 2008). During decomposition, the SOM can either undergo mineralization by soil microorganisms or be reincorporated into microbial biomass through immobilization (Findlay, 2021; Robertson and Paul, 2000; Haynes, 1986). Decomposition of SOM is a fundamental ecological process, vital for the recycling of nutrients, maintenance of soil structure and regulation of the carbon cycle (Raza et al., 2023; Nair et al., 2021; Angst et al., 2021; Paul and Collins, 2020). The rate at which SOM is decomposed also determines the balance between the carbon sequestered in soils and the carbon released into the atmosphere (Prescott, 2010; Swift, 2001), a balance with direct implications for climate change mitigation and the sustainability of diverse ecosystems (Goebel et al., 2011; Anderson, 1991).
The decomposition of SOM is a complex phenomenon influenced by several factors, including land cover, hydrological conditions, soil properties, climate, microbial community composition, nutrient availability, and human activities (Adl, 2003; Guo and Gifford, 2002; Robertson and Paul, 2000). Each factor contributes to the complex dynamics of SOM breakdown, affecting the rate and efficiency with which organic materials are decomposed (Cotrufo and Lavallee, 2022; Paul, 2016). For example, the nature of the land cover plays a significant role, with different vegetation and land uses providing varying SOM inputs and thus influencing the microbial consortia specialized for decomposition in these microhabitats (Ramesh et al., 2019; Moghimian et al., 2017; Deng et al., 2016; Muñoz-Rojas et al., 2015; Guo and Gifford, 2002). Soil type also directly influences the rate of SOM decomposition, affecting factors such as aeration, moisture retention, and nutrient cycling. These variations in soil properties create distinct microenvironments that impact microbial activity, enzyme production, and ultimately the efficiency of SOM decomposition and carbon turnover (Rittl, et al., 2020; Zheng et al., 2019; Frøseth and Bleken, 2015; Tumer et al., 2013; Dijkstra and Cheng, 2007; Scott et al., 1996). Biological properties, including microbial biomass and enzymatic activity, play a crucial role in driving decomposition processes (Wang and Allison, 2019; Glassman et al., 2018; Don et al., 2017 Condron, et al., 2010; Van Veen and Kuikman, 1990). Moreover, hydrological conditions, encompassing wet and dry seasons, particularly in humid sub-tropical climates like that of Central Florida (Lascody and Melbourne, 2002; Black, 1993) also play a crucial role in the decomposition of SOM (Harrison-Kirk et al., 2014; Zhu and Cheng, 2013; Lohse et al., 2009 Denef et al., 2001). For example, during wet periods, increased moisture levels can enhance microbial activity by improving the mobility of enzymes and substrates, thus potentially accelerating decomposition rates (Harrison-Kirk et al., 2014; Xiang et al., 2008; Williams and Rice, 2007). Conversely, dry periods may lead to a reduction in microbial activity due to moisture stress, which can slow down the decomposition process (Schimel, 2018; Ouyang and Li, 2013; Boddy, 1986).
Understanding the role of discussed factors in SOM decomposition is essential. However, to understand the mechanisms of SOM decomposition more comprehensively it is important to consider the effects of these factors on indicative soil parameters that can define SOM decomposition. Central to this understanding are a variety of enzymes that work synergistically to break down complex organic compounds. These include both hydrolytic enzymes such as β-1,4-glucosidase (βG), β-1,4-N-acetyl glucosaminidase (β-NAG), Acid Phosphatase (AP), and Aryl Sulfatase (AS) whichserve as critical mediators in the decomposition process (Bautista-Cruz and Ortiz-Hernández, 2015) and oxidative enzymes, like peroxidases and phenol oxidases, which are essential for degrading more recalcitrant components such as lignin (Hassan et al., 2013; Beckett et al., 2013). In this study, we specifically focus on hydrolytic enzymes due to their well-established role in catalyzing the breakdown of labile SOM fractions and their rapid responsiveness to environmental changes (Bautista-Cruz and Ortiz-Hernández, 2015). These enzymes are directly involved in the mineralization of key nutrients and are therefore considered sensitive indicators of microbial nutrient transformations under varying ecological conditions (Shi, 2010; Sinsabaugh et al., 2008). These enzymes, crucial for microbial nutrient transformations, significantly influence the rate-limiting steps of SOM decomposition (Koch et al., 2007; Speir et al., 2002) as theytarget specific substrates within the soil organic complex, facilitating the breakdown of SOM. βG plays a pivotal role in the carbon cycle by catalyzing the final step in cellulose compound breakdown and enabling the release of simple glucose to microorganisms (Tischer et al., 2015; Piotrowska and Koper, 2010). β-NAG, on the other hand, is vital for hydrolyzing N-acetylglucosamine from fungal chitin and bacterial murein, linking it to microbial turnover of carbon and nitrogen (Tischer et al., 2015). AP and AS contribute to the cycling of phosphorus and sulfur, respectively (Wang et al., 2019; Kang and Freeman, 1999). Despite the significant role of hydrolytic enzymes in SOM decomposition, more research is needed to understand how enzyme activities vary across different hydrological conditions and land cover types, as well as their implications for SOM decomposition. Current studies have primarily focused on controlled environments, leaving a gap in understanding enzyme activities under natural conditions with varying, soil types, and land covers (Burns et al., 2013). Moreover, there is a limited understanding of enzyme activity fluctuations across different hydrological periods, such as wet and dry seasons, which can significantly impact microbial activity and enzyme activity (Henry, 2013). These research gaps hinder our ability to understand SOM decomposition dynamics accurately and develop effective land management strategies for carbon sequestration and soil health improvement. Therefore, investigating these enzyme activities across different hydrological periods, soil types and land covers can provide insights into the temporal and spatial aspects of SOM decomposition. Furthermore, exploring how soil properties influence hydrolytic enzyme activity will enhance our understanding of the factors affecting SOM decomposition, supporting conservation efforts and carbon sequestration in less managed ecosystems.
The Deluca preserve, with its diverse ecosystems and well-defined wet and dry seasons, offers a prime setting for the investigation of all these research gaps. The preserve’s location within a humid subtropical climate zone in central Florida, characterized by distinct hydrological patterns, and its diverse array of ecosystems, from woodlands to wetlands offer a microcosm through which the complex relationship between hydrolytic enzyme activity and SOM decomposition can be observed. This study explores how hydrolytic enzyme activities differ across various land covers and between wet and dry seasons within the DeLuca Preserve, aiming to uncover patterns in enzyme activity that may influence SOM decomposition. Additionally, it investigates the associations between hydrolytic enzyme activities and soil physicochemical properties, seeking to understand how these relationships contribute to SOM dynamics in different environmental contexts within the preserve. The study was conducted with the following objectives. (i) To investigate the variations in hydrolytic enzyme activities in different land covers in dry and wet seasons and their implications on SOM decomposition and (ii) to examine the relationship between soil physiochemical properties and hydrolytic enzyme activities in dry and wet season, and how these interactions affect SOM decomposition.
2 Materials and methods
2.1 Study location
This study was conducted on the Deluca preserve located in Central Florida (South of SR 60, West of FL Turnpike) about 11 km east of the Kissimmee River, geographically located between 27°38′ 20′′ to 27°44′ 10′′ N, and 80°51′ 40′′ to 81°1′ 40′′ W (Figure 1). The site is located in a tropical climate zone with an average annual high and low temperature of 28.89°C, and 17.22°C, and a mean yearly rainfall of approximately 1,204 mm. The preserve is characterized by cyclical patterns of a wet rainy season from June to September and a dry, cool winter season beginning from December to February, which are referred to as the “wet” and “dry” seasons, respectively (Figure 1). This natural hydroperiod of seasonally flooded and desiccated conditions on an annual basis is the major driver creating a favorable condition for the diverse land covers on the preserve (Exum, 2020).
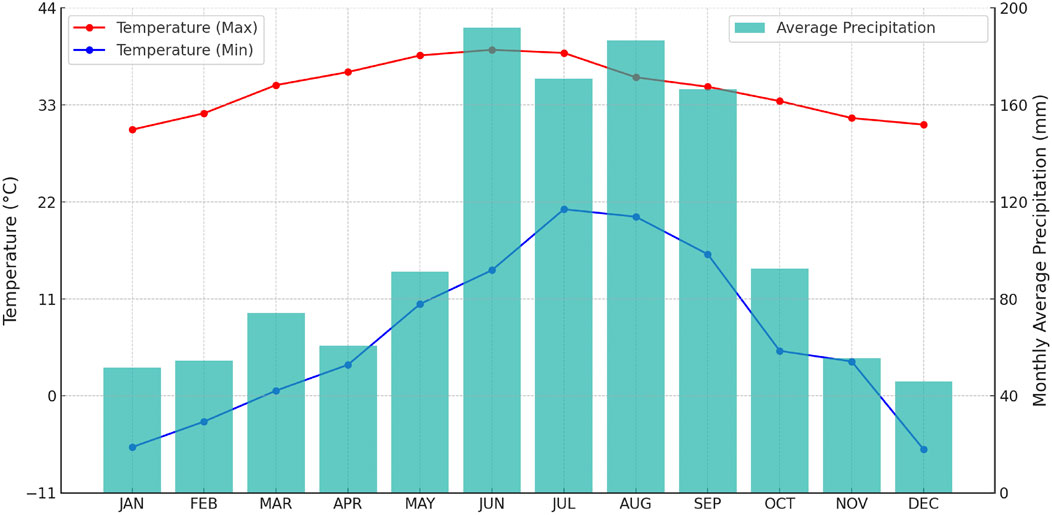
Figure 1. Monthly Temperature and Precipitation Averages for DeLuca preserve (1981–2023) Source: NASA/POWER CERES/MERRA2 Climatology Data 2024.
2.2 Soil sampling and processing
Based on the major vegetation categories in the Deluca preserve, 23 unique land covers were selected for the study (Figure 2; Table 1). Topsoil samples (0–15 cm) were collected from these land covers during two seasons: the dry season (January 2021) and the wet season (August 2022). To capture spatial variability and minimize the impact of sampling point selection on soil properties, composite samples were taken from three locations within each land cover type. At each location, soil was collected within a 1-m diameter and mixed to form a single composite sample, ensuring representativeness for each sample (Supplementary Table S1 in the Supplementary Material for geographic coordinates of sampling locations).
Considering the overall ecological setting, including dominant vegetation type, hydrological characteristics, land management and land-use history, these 23 land covers were further classified into five distinct ecosystems (Table 1; Supplementary Figure S1 in the Supplementary Material for more detailed views of land cover and ecosystems). This classification aimed to facilitate a more structured analysis of regional ecological patterns and to minimize the impact of sampling point selection on observed soil properties. The collected soil samples were oven-dried at 50°C for chemical analysis and air-dried for enzymatic analysis. All samples were then sieved to 2 mm prior to laboratory analysis.
2.3 Ecological setting
The Deluca preserve, across 109.05 square kilometers (km2), is a mix of diverse ecosystems and land uses (Figure 3). The area distribution includes several diverse ecosystems as described below:
i. Wetland, Watercourse, and Waterbody Areas (27.02 km2): Encompassing herbaceous prairies, marshes, and forested wetlands, these areas are essential for water purification, groundwater recharge, and providing habitats for species such as the eastern indigo snake and Florida sandhill crane.
ii. Upland Forests (21.61 km2): Consisting of mesic hammocks, Florida scrub, mesic flatwoods, and scrubby flatwoods, these forests support a wide range of flora and fauna, including rare habitats for the red-cockaded woodpecker and gopher tortoise.
iii. Native Range Areas (30.02 km2): Dominated by dry prairie habitats with indigenous vegetation, these areas are vital for the conservation of species like the Florida grasshopper sparrow, maintained through light grazing and regular fires.
iv. Cultivated Agricultural Areas (3.28 km2): Including active citrus groves and lands previously utilized for row crops, these areas are now mainly used for cattle grazing on bahiagrass, reflecting the agricultural heritage of the preserve.
v. Improved Pasture Areas (26.68 km2): Primarily used for cattle grazing, these pastures have been enhanced for forage with bahiagrass, aiding in the conservation and agricultural management of the landscape.
vi. Intensive Use Areas (0.44 km2): These areas are allocated for more concentrated activities, likely including the infrastructure and facilities essential for the management and operations of the preserve.
2.4 Soils geology and hydrology
Based on the NRCS soil survey for Osceola County, a comprehensive analysis has identified a total of 38 distinct soil types and 6 soil orders within the DeLuca Preserve (Exum, 2020), as detailed in Figure 4 and summarized in Table 2. Table 3, derived from the Soil Survey Geographic Database (National Resources Conservation Service, 2024), provides key soil hydrological properties across various land cover types, including parameters such as available water storage, water table depth, saturated hydraulic conductivity, and moisture content. These parameters remain relatively stable and serve as a comprehensive source of information, offering a detailed description of hydrological attributes for different land cover and ecosystems. Wetland and poorly drained soils have higher water retention capacities and hydric soil presence, while xeric and sandy soils, such as those in hammocks and abandoned citrus groves, exhibit lower available water capacities and higher drainage. Additional details on specific soil types and their distributions can be found in Supplementary Materials S2, S3.
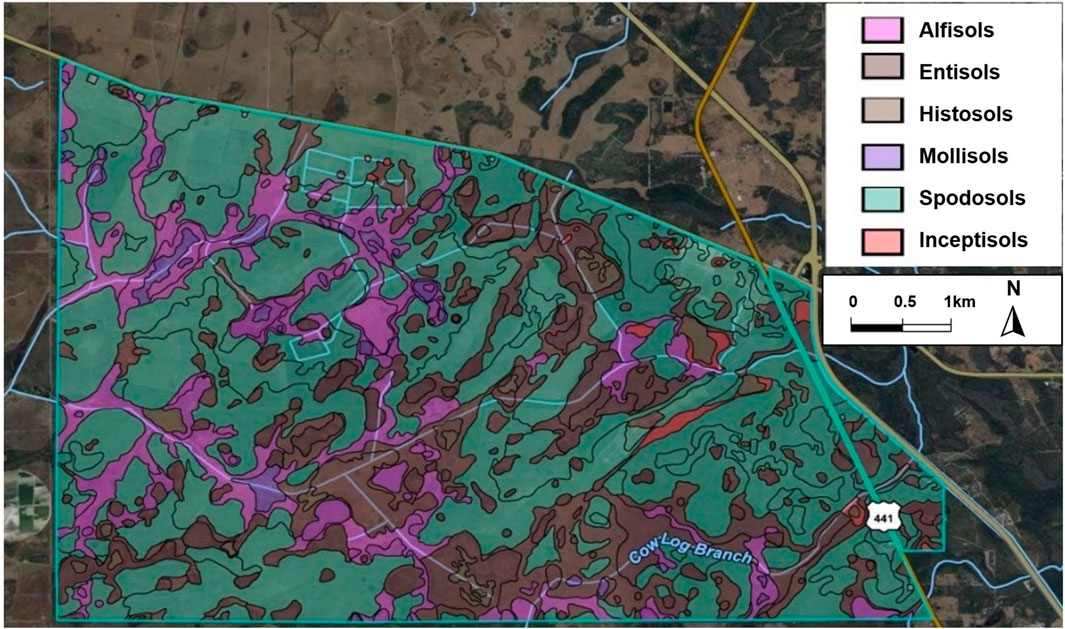
Figure 4. Soil taxonomy map of soil orders across the preserve. Source: (U.S. Department of Agriculture, [USDA]-NRCS, 2024).
2.5 Laboratory analysis
All soil samples were subjected to physicochemical analyses following standard procedures. For Enzymatic analysis, air-dried soil samples were sieved through a 2 mm sieve for analysis of βG and β-NAG, AP, and AS by the method described by Tabatabai (1994). Enzyme activities were expressed in µg PNP/g dry soil/h, representing the micrograms of p-nitrophenol released per gram of dry soil per hour. To examine the relationship between soil physiochemical properties and hydrolytic enzyme activities, ten soil properties were analysed: pH, bulk density (BD), maximum water holding capacity (MWHC), cation exchange capacity (CEC), SOM, active carbon (AC), soil protein (SP), total phosphorus (TP), total potassium (TK), and total Kjeldahl nitrogen (TKN). Soil pH (soil/water suspension of 1.5:15) was determined using a pH meter (Amgain, Martens-Habbena, and Bhadha, 2022). BD was measured by filling a 25 mL graduated cylinder with oven-dry soil and dividing the soil mass by the cylinder volume (25 cm3) (Xu et al., 2022). MWHC was measured following a modified protocol by Jenkinson and Polson (1976). SOM was determined using the loss on ignition method at 550°C. CEC was determined using the ammonium acetate method (Sumner, Miller, and Sparks, 1996). AC was measured using the Potassium Permanganate (KMnO4) method (Schindelbeck et al., 2016). SP was measured using the sodium citrate extraction method under autoclaving at high temperature and pressure (Schindelbeck et al., 2016). TP and TK were measured by ashing samples at 550°C in a muffle furnace for at least 5 h (not exceeding 16 h), followed by extraction with 6 M HCl and analysis using ICP-OES. TKN was analyzed using the Kjeldahl method (Bremner and Mulvaney, 1982).
2.6 Statistical analysis
All statistical analyses were performed using statistical software R-4.4.0 (R Development Core Team, 2024). Data were tested for normality, and transformations were applied as necessary to meet assumptions. For the first objective, a two-way ANOVA was conducted with land cover and season as factors to examine their interaction and main effects on the response variables. Mean comparisons were conducted using Bonferroni adjustments at a 5% significance level, separately between land covers and between seasons. Similarly a two-way ANOVA was also performed with the five ecosystems and years as factors to provide an overall understanding of SOM decomposition across a broader scale, reflecting the aggregated ecosystem groups from the 23 distinct land covers. Mean comparisons were conducted using Bonferroni adjustments at a 5% significance level, separately between ecosystems and between seasons.
Principal Component Analysis (PCA) was performed on all four hydrolytic enzymes to determine their combined effect on SOM decomposition. Using the results from the PCA, an index termed the PCA-based hydrolytic enzymes decomposition indicator (HEDI) was calculated to represent the overall enzymatic activity which can act as an indicator of decomposition. This index incorporates the weighted methodology that considers the principal components (PC) scores. The weight of each PCs score is given by the proportion of variance it explains. HEDI was calculated with the following equation:
Where PCn is the score for principal component n, and Vn is the variance explained by that component. Similar approaches of using weighted PCA scores has been employed to assess soil quality and variability, where PCA scores, weighted by explained variance, serve as critical indicators (Martin-Sanz et al., 2022; Karaca et al., 2021; Kumar et al., 2012). This study adapts and modifies these established methodologies to develop HEDI. Seasonal comparisons using the HEDI are not feasible because the index is calculated based on distinct principal components derived from the dry and wet seasons, which prevents a direct comparison of enzymatic activity between these periods. However, to facilitate relative comparisons of enzymatic activity across seasons, the activities in each season were classified into quartiles. PCA scores for the dry and wet seasons are provided in Supplementary Tables S2A, B, and the PCA Biplot is illustrated in Supplementary Figure S4.
For second objective, Spearman correlation analysis was conducted to explore the interactions between hydrolytic enzyme activities and the soil parameters across different land covers. This method was directly applied due to its suitability for non-normal, non-transformed data and its ability to capture both monotonic and non-linear relationships. Multiple regression analyses were performed for each of the four enzymes, using all soil physicochemical properties and other enzyme activities as predictors. Standardized coefficients were calculated for each equation to identify significant predictors and compare their relative effects on soil hydrolytic enzymes.
3 Results
3.1 Variations in hydrolytic enzyme activities in different land covers in dry and wet seasons and their implications on SOM decomposition
3.1.1 Hydrolytic enzyme activities across land covers
Distinct patterns in hydrolytic enzyme activities emerged from seasonal comparisons across various land covers, with a significant interaction (p < 0.05) observed between land cover and season for all enzymes (Figures 5–8).
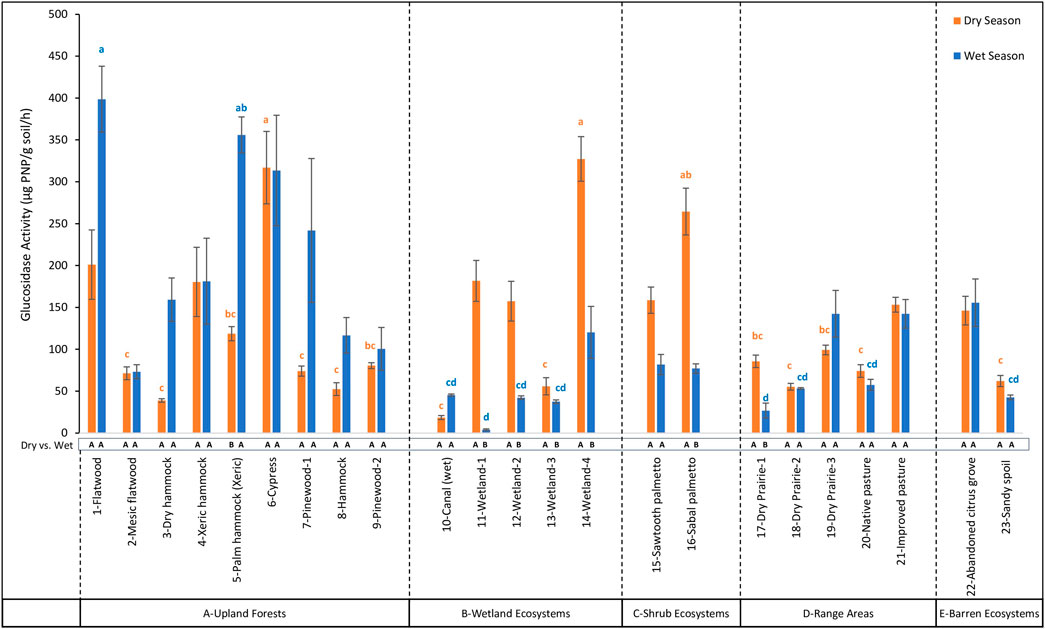
Figure 5. Comparison of β-1,4 glucosidase (βG) activity across 23 land cover types at the DeLuca Preserve in wet and dry seasons (Error bars represent ±standard error of the mean. Lowercase letters above bars indicate significant differences among land covers within each season (Bonferroni-adjusted, p < 0.05), with blue letters denoting differences in the wet season and orange letters in the dry season. Capital letters below bars denote significant seasonal differences (dry vs wet) within each land cover type (Bonferroni-adjusted, p < 0.05). To enhance clarity, only the extreme significance labels are displayed; detailed comparisons are provided in the Supplementary Table S3. Land cover types are grouped by ecosystem categories (A–E), separated by dashed lines).
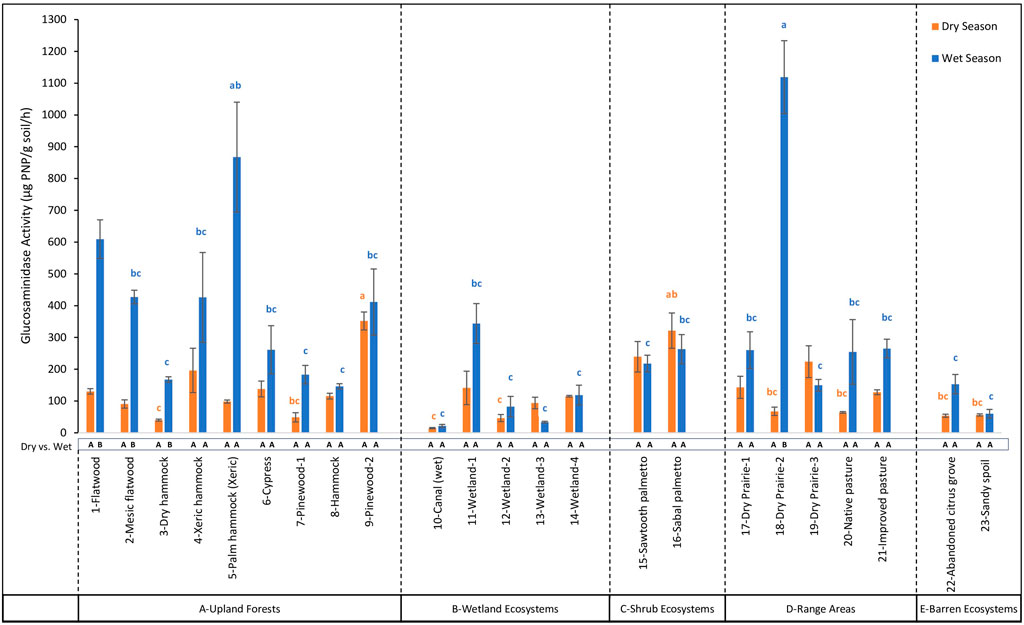
Figure 6. Comparison of β-1,4-N-acetyl glucosaminidase (β-NAG) activity across 23 land cover types at the DeLuca Preserve in wet and dry seasons (Error bars represent ±standard error of the mean. Lowercase letters above bars indicate significant differences among land covers within each season (Bonferroni-adjusted, p < 0.05), with blue letters denoting differences in the wet season and orange letters in the dry season. Capital letters below bars denote significant seasonal differences (dry vs wet) within each land cover type (Bonferroni-adjusted, p < 0.05). To enhance clarity, only the extreme significance labels are displayed; detailed comparisons are provided in Supplementary Table S3. Land cover types are grouped by ecosystem categories (A–E), separated by dashed lines).
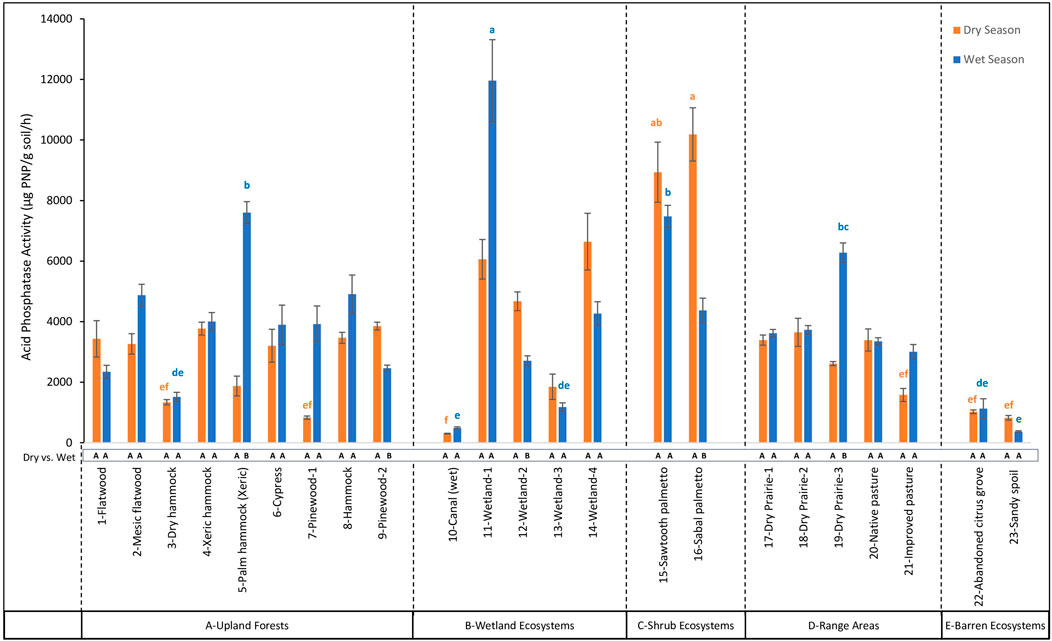
Figure 7. Comparison of Acid Phosphatase (AP) activity across 23 land cover types at the DeLuca Preserve in wet and dry seasons (Error bars represent ±standard error of the mean. Lowercase letters above bars indicate significant differences among land covers within each season (Bonferroni-adjusted, p < 0.05), with blue letters denoting differences in the wet season and orange letters in the dry season. Capital letters below bars denote significant seasonal differences (dry vs wet) within each land cover type (Bonferroni-adjusted, p < 0.05). To enhance clarity, only the extreme significance labels are displayed; detailed comparisons are provided in Supplementary Table S3. Land cover types are grouped by ecosystem categories (A–E), separated by dashed lines).
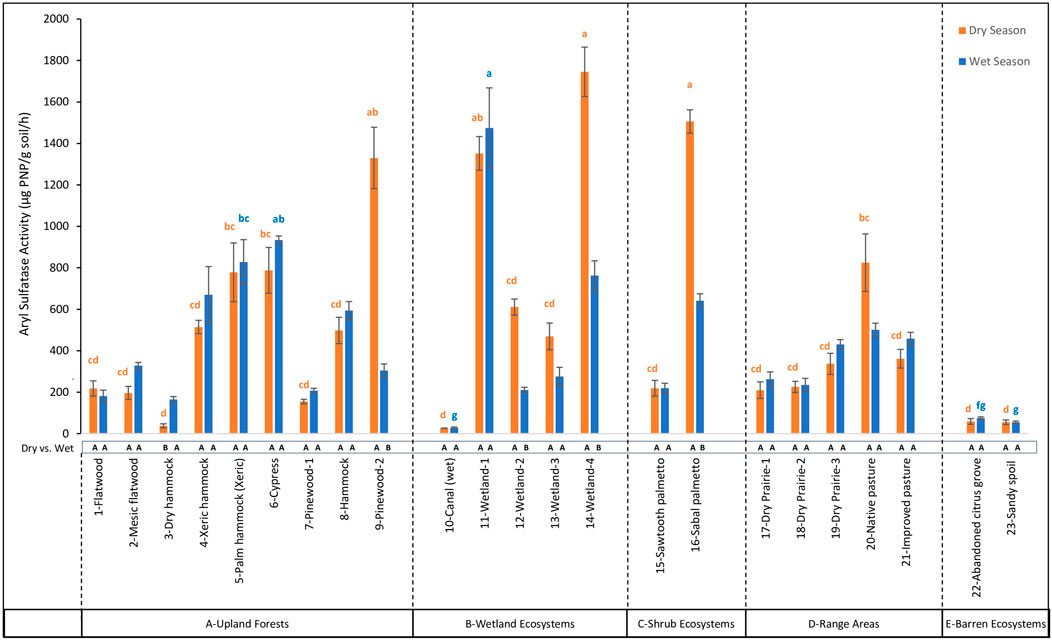
Figure 8. Comparison of Aryl Sulfatase (AS) activity across 23 land cover types at the DeLuca Preserve in wet and dry seasons. (Error bars represent ± standard error of the mean. Lowercase letters above bars indicate significant differences among land covers within each season (Bonferroni-adjusted, p < 0.05), with blue letters denoting differences in the wet season and orange letters in the dry season. Capital letters below bars denote significant seasonal differences (dry vs. wet) within each land cover type (Bonferroni-adjusted, p < 0.05). To enhance clarity, only the extreme significance labels are displayed; detailed comparisons are provided in Supplementary Table S3. Land cover types are grouped by ecosystem categories (A–E), separated by dashed lines).
Among different land covers during the dry season, βG activity ranged from 18.40 to 327.20 μg PNP/g soil/hr (Figure 5) with highest values observed in 14-Wetland-4, which was not significantly different (p > 0.05) from nine other land cover types, reflecting consistent enzymatic activity across multiple ecosystems. On the other hand, in the wet season, βG activity showed a wider range, from 4.02 to 398.66 μg PNP/g soil/hr, with the highest value in 1-Flatwood which was not significantly different (p > 0.05) from ten other land cover types. For β-NAG activity, values during the dry season ranged from 14.71 to 351.90 μg PNP/g soil/hr (Figure 6), with the highest levels observed in 9-Pinewood-2, which were not significantly different (p > 0.05) from 16 other land covers. In the wet season, however, β-NAG activity showed a broader range of 21.72 to 1,118.97 μg PNP/g soil/hr, with the highest value observed in 17-Dry Prairie-1. These values were not significantly different (p > 0.05) from 1-Flatwood and 5-Palm Hammock (Xeric) but were statistically higher than other land covers, indicating more variability among land covers compared to the dry season. AP activity showed the largest range of activity among all enzymes. During the dry season, AP ranged from 302.89 to 10,185.80 μg PNP/g soil/hr (Figure 7), with the highest levels observed in 16-Sabal Palmetto. These values were not significantly different (p > 0.05) from those in 11-Wetland-1, 14-Wetland-4, and 15-Sawtooth Palmetto. In the wet season, AP activity displayed a broader range, from 372.38 to 11,960.36 μg PNP/g soil/hr, with 11-Wetland-1 recording statistically higher value (p < 0.05) than all other land covers. In contrast to βG, β-NAG and AP, AS activity showed opposite trends from the dry to wet season. In the dry season, AS activity ranged from 26.51 to 1,745.75 μg PNP/g soil/hr (Figure 8), with the highest levels observed in 14-Wetland-4 not significantly different (p > 0.05) from 16-Sabal Palmetto, 9-Pinewood-2 and 10-Canal (wet). In the wet season, AS activity exhibited a narrower range, from 28.26 to 1,475.09 μg PNP/g soil/hr, with the highest levels observed in 11-Wetland-1 not significantly different (p > 0.05) from 6-Cypress. Overall, the highest enzymatic activity exhibited variable trends between the dry and wet seasons, while the lowest enzymatic activity remained consistently lowest in 10-Canal (wet) across all enzymes during the dry season. This trend was similar in the wet season with minimum values of β-NAG and AS in 10-Canal (wet).
3.1.2 Hydrolytic enzyme activities across seasons
For pairwise seasonal comparisons for each land cover, hydrolytic enzyme activities displayed variable patterns for different enzymes. βG activity increased significantly in 5-Palm hammock (Xeric) and 10-Canal (wet), while decreased significantly in 11-Wetland-1, 12-Wetland-2, 14-Wetland-4, 16-Sabal palmetto, and 17-Dry Prairie-1. For β-NAG, only a significant increase was recorded in 1-Flatwood, 2-Mesic flatwood, 3-Dry hammock, and 18-Dry Prairie-2. AP activity exhibited significant increases in 5-Palm hammock (Xeric), 7-Pinewood-1, 10-Canal (wet), and 19-Dry Prairie-3, while decreased significantly in 9-Pinewood-2, 12-Wetland-2, 16-Sabal palmetto, and 23-Sandy spoil. Additionally, AS activity showed a significant increase in 3-Dry hammock and decreased significantly in 9-Pinewood-2, 12-Wetland-2, and 16-Sabal palmetto. Other land covers such as 6-Cypress, 8-Hammock, 15-Sawtooth Palmetto, 20-Native Pasture, 21-Improved Pasture, and 22-Abandoned Citrus Grove, exhibited no significant seasonal differences in enzymatic activity.
3.1.3 Ecosystems comparison
Across different ecosystems, a visible pattern emerged with the transition from dry to wet conditions (Figure 9). Overall, the C-Shrub ecosystems demonstrated the highest range of enzymatic activity, spanning from 79.42 to 9,561.07 μg PNP/g soil/hr, while the D-Barren ecosystems exhibited the lowest range, from 54.94 to 106.58 μg PNP/g soil/hr.
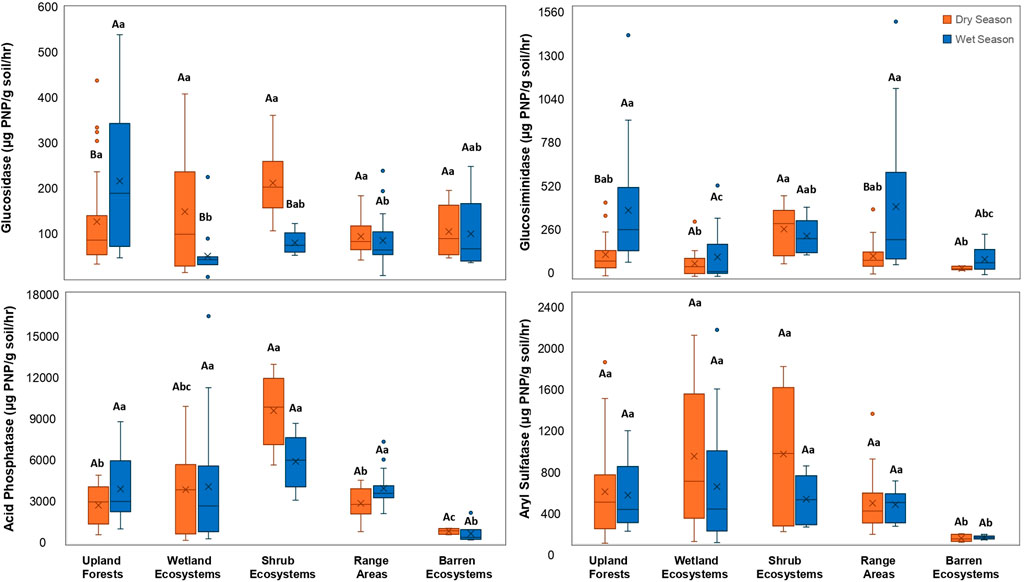
Figure 9. Comparison of hydrolytic enzyme activities- β-glucosidase, β-1,4-N-acetylglucosaminidase (β-NAG), acid phosphatase (AP), and arylsulfatase (AS) across five ecosystem categories at the DeLuca Preserve in wet and dry seasons. (Error bars represent ±standard error of the mean. Each ecosystem comprises multiple land cover types (Table 1). Capital letters above each box indicate significant seasonal differences within an ecosystem (dry vs. wet), while lowercase letters denote significant differences among ecosystems within each season (Bonferroni-adjusted, p < 0.05).
Among different ecosystems in the dry season, βG activity showed no significant differences; however, it varied more in the wet season, with βG being highest in A-Flatwoods, not statistically different (p > 0.05) to the C-Shrub and D-Barren ecosystems. For β-NAG, the highest activity in the dry season was observed in the C-Shrub ecosystems, not statistically different (p > 0.05) to the B-Range Areas and A-Upland Forests. Similarly, in the wet season, β-NAG was highest in the B-Range Areas not statistically different (p > 0.05) to A-Flatwoods C-Shrub ecosystems. The results indicated that β-NAG activity was consistently higher in these three ecosystems (A-Upland Forests, B-Range Areas, and C-Shrub ecosystems) across both seasons. AP activity was significantly higher in the C-Shrub ecosystems during the dry season. However, in the wet season, AP activity showed no significant differences, except for the D-Barren ecosystems, which showed significantly lower values. AS showed minimal variation among ecosystems in both seasons, with no statistical difference (p > 0.05) among all ecosystems except for the D-Barren ecosystems which showed significantly lower activity in both seasons. Overall, the results indicate that both AP and AS activities remained relatively stable across most ecosystems, highlighting the distinct differences in enzymatic responses based on seasonal changes.
From dry to wet seasons, βG increased significantly in A-Flatwoods, while it decreased significantly in both B-Wetlands and C-Shrub ecosystems. β-NAG showed significant increases in A-Flatwoods and B-Range Areas, with no significant decreases observed in any ecosystem. Both AP and AS demonstrated no significant changes from dry to wet season across all ecosystems.
3.1.4 HEDI across different land covers and ecosystems
The HEDI values demonstrated significant variability across different land covers and seasons (Table 4). Among different land covers during the dry season, HEDI values ranged from a minimum of −1.217 in 10-Canal (wet) to a maximum of 2.281 in 16-Sabal palmetto, indicating a spectrum of enzymatic activity. In the wet season however, the HEDI values exhibited a narrower range, with the lowest value at −0.943 again in 10-Canal (wet) and the highest at 1.623 in 5-Palm Hammock (Xeric). Land covers such as 1-Flatwood, 6-Cypress, 11-Wetland-1, 15-Sabal palmetto, and 16-Sawtooth palmetto consistently showed higher HEDI values, classifying in the highest quartile classes (I or II) in both seasons, which reflects overall higher enzymatic activity. In contrast, several land covers, including 3-Dry hammock, 10-Canal (wet), 12-Wetland-2, 13-Wetland-3, 21-Improved pasture, 22-Abandoned citrus grove, and 23-Sandy spoil, remained in the lower quartile classes (III and IV) for HEDI values, indicating minimal enzymatic activity.
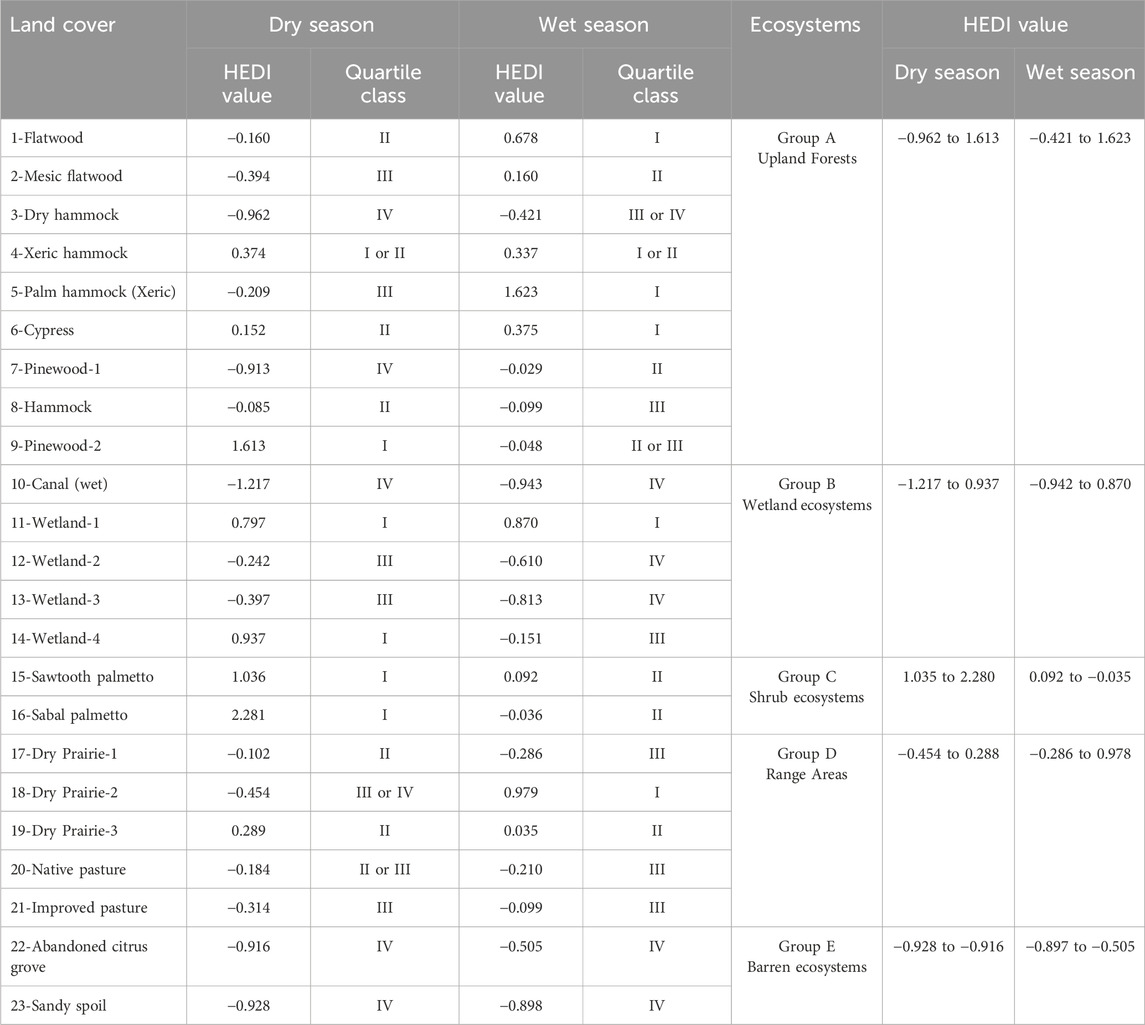
Table 4. HEDI with corresponding Quartile class among different land covers and ecosystems in dry and wet season.
Among different ecosystems in the dry season, A-Upland Forests showed the widest range of HEDI values, ranging from −0.962 to 1.613, indicating a wide variation of enzymatic activity. C-Shrub ecosystems demonstrated the highest values of HEDI ranging from 1.035 to 2.280, suggesting high hydrolytic enzyme activity. In contrast, E-Barren ecosystems showed lowest HEDI values between −0.928 and −0.916, emphasizing limited enzymatic activity. In the wet season, A-Upland Forests again showed a wide range of HEDI values, from −0.421 to 1.623, along with B-Wetland ecosystems ranging from −0.942 to 0.870, indicating a diverse enzymatic activity across these ecosystems. Similarly, E-Barren ecosystems remained consistent with low HEDI of −0.897 to −0.505 in the wet season as well, indicating persistently limited enzymatic activity across both seasons.
3.2 Relationship between soil physiochemical properties and hydrolytic enzyme activities in wet and dry seasons
The analysis of the relationship between soil biogeochemical properties and hydrolytic enzyme activities revealed significant insights, particularly in understanding the differences between wet and dry seasons (Figure 10). SOM, SP, AC, CEC, and MWHC showed positive correlations with all enzymes in both seasons, while BD showed consistently negative correlations, and pH showed no significant correlations. Other parameters, such as TP, TKN, and TK, showed more variable correlation values. During the dry season, SOM and AC showed significant positive correlations at p < 0.001 with all enzymes. The correlations for SOM were 0.59, 0.54, 0.51, and 0.55, and for AC, were 0.58, 0.58, 0.46, and 0.50, with βG, β-NAG, AP, and AS, respectively. In the wet season, although SOM and AC retained positive correlations with enzymatic activities, they showed greater variability, with significant correlations at p < 0.001 observed only with AS (0.51 with SOM and 0.50 with AC). A general trend of decreasing correlation strength was observed from the dry to wet season for most relationships, with exceptions such as SP with AP (Dry: 0.27, p < 0.05; Wet: 0.32, p < 0.01) and AS (Dry: 0.30, p < 0.05; Wet: 0.40, p < 0.001), MWHC with AS (Dry: 0.28, p < 0.05; Wet: 0.61, p < 0.001), and CEC with AS (Dry: 0.28, p < 0.05; Wet: 0.28, p < 0.01). Other important correlations included SP, TKN and TP, which exhibited positive relationships during dry seasons which was more variable during wet season. The correlations between enzymes were all positive and generally exhibited significant reduction from the dry to wet season, with exceptions for AP and AS (Dry: 0.60, p < 0.001; Wet: 0.63, p < 0.001) and β-NAG and AP (Dry: 0.50, p < 0.001; Wet: 0.39, p < 0.001), with no significant reduction.
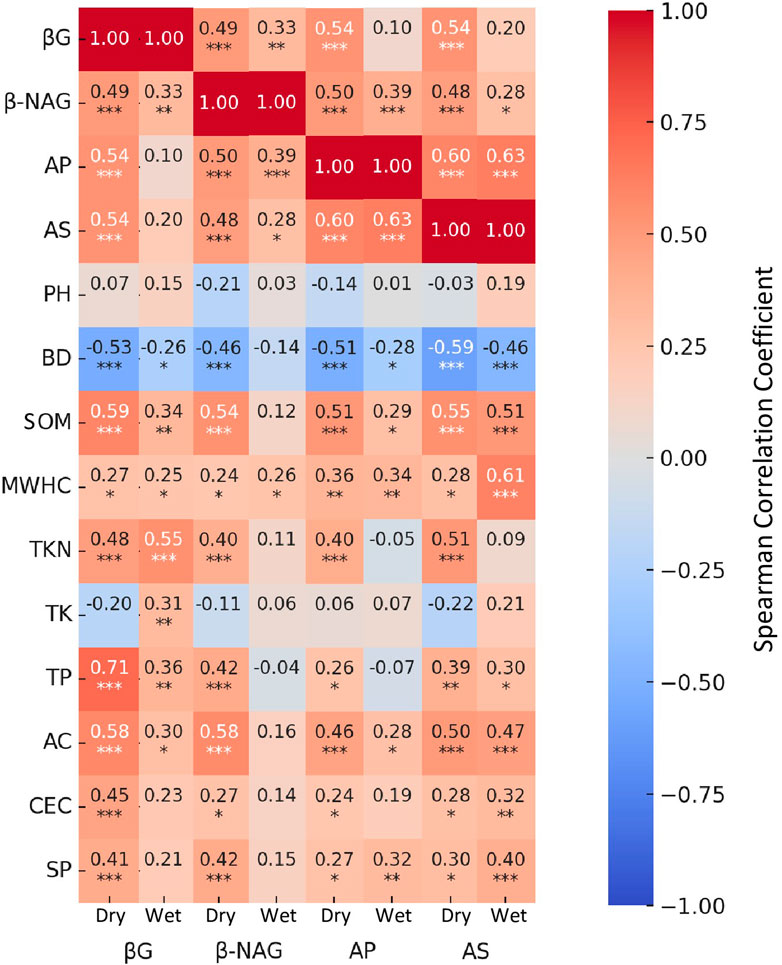
Figure 10. Spearman correlation heatmaps showing the relationships between hydrolytic enzyme activities- β-glucosidase, β-1,4-N-acetylglucosaminidase (β-NAG), acid phosphatase (AP), and arylsulfatase (AS) and soil physicochemical parameters under dry and wet season conditions at the DeLuca Preserve. Each cell displays the Spearman correlation coefficient (ρ) between a given enzyme and soil property. Statistical significance is denoted by asterisks: ***p < 0.001, **p < 0.01, *p < 0.05. Non-significant correlations are unmarked. Abbreviated soil variables: bulk density (BD), soil organic matter (SOM), maximum water holding capacity (MWHC), total nitrogen (TKN), total potassium (TK), total phosphorus (TP), active carbon (AC), cation exchange capacity (CEC), and soil protein (SP).
Based on the Multiple Linear regression analysis (Table 5), the predictors influencing enzyme activities varied between the dry and wet seasons, reflecting dynamic soil-enzyme interactions. For βG and β-NAG, the R2 values decreased between seasons, with βG dropping from 0.71 in the dry season to 0.41 in the wet season and β-NAG declining from 0.48 to 0.31 (p < 0.001). Conversely, for AP and AS, the R2 values increased between seasons. AP rose from 0.51 in the dry season to 0.74 in the wet season, while AS increased from 0.56 to 0.71 (p < 0.001). HEDI, representing overall enzymatic activity, had a high R2 of 0.80 in the dry season and was predicted by a broader range of soil properties, including AP, β-NAG, AS, MWHC, CEC, and AC. In the wet season, HEDI had a slightly lower R2 of 0.72, with fewer significant predictors, including β-NAG, AP, and pH.
4 Discussion
4.1 Variations in hydrolytic enzyme activities in different land covers in wet and dry seasons and their implications on SOM decomposition
4.1.1 Hydrolytic enzyme activities across land covers
Among different enzymes the observed activities for βG in both seasons and β-NAG in the dry season demonstrate consistently high activity across several land covers, likely driven by the combined effects of vegetation type, hydrological, and soil properties regulating microbial activity and enzyme production (Wang et al., 2020; Simpson et al., 2019; Salazar et al., 2011). This suggests that βG and β-NAG enzymes play a key role in stabilizing decomposition processes across these land covers (Sherene, 2017), facilitating consistent SOM turnover under varying environmental conditions. Additionally, environmental factors such as rainfall and temperature (Mariscal-Sancho et al., 2018; Rastin et al., 1988), along with soil characteristics like substrate availability (Mir et al., 2023), likely influenced the βG and β-NAG activities, contributing to the observed results. β-NAG activity exhibits greater variability among land covers during the wet season compared to the dry season, suggesting that increased moisture availability may contribute to the broader range of β-NAG activity and decomposition processes (Borowik and Wyszkowska, 2016; Zhang et al., 2011). AP and AS exhibited different trends compared to βG and β-NAG during the dry season, with their activities showing greater variability across land covers, possibly because these enzymes are more influenced by factors such as land cover characteristics (Mayor et al., 2016; Salam et al., 2001) and soil type (Nedyalkova et al., 2020) indicating varying levels of SOC decomposition. Overall, all enzymes exhibited increased activity ranges from the dry to wet season, indicating enhanced SOC decomposition, except for AS, which showed an opposite trend. This divergence may result from AS’s regulation by sulfate availability and its sensitivity to wetting and drying cycles, which can suppress activity (Kunito et al., 2022; Cooper, 1972). In contrast, βG, β-NAG, and AP activities were positively influenced by increased moisture, consistent with studies showing enhanced enzyme activities during wet seasons or after rewetting (Hammerl et al., 2019; Kapila et al., 2017; Liao et al., 2016). These results emphasize the critical role of hydrology in regulating enzyme-mediated SOC decomposition.
Among land covers in the dry season, 11-Wetland-1, 14-Wetland-4, 15-Sawtooth palmetto, and 16-Sabal palmetto generally showed higher enzymatic activity. This could be attributed to specific characteristics of these land covers, such as very poorly drained loamy soils, which are known to enhance water retention and support microbial activity (Kim, 2015). Additionally, these soils are typically associated with a high moisture class and high available water storage capacity, creating favourable conditions for microbial processes and enzyme production (Kim, 2015; Wu et al., 2021). These conditions may have maintained sufficient soil moisture even during dry periods, supporting microbial activity and enzyme production (Sinsabaugh et al., 2008). Whereas the lowest enzymatic activities during the dry season in the 10-Canal (wet) ecosystem for all enzymes could have resulted from over-saturated conditions, which may hinder oxygen diffusion and microbial activity necessary for enzyme production (Furtak et al., 2020; Borowik and Wyszkowska, 2016). The persistence of minimum values for β-NAG and AS in 10-Canal (wet) across both seasons, again suggests that prolonged wetland conditions might consistently inhibit these enzymes. This inhibition could be due to factors such as reduced oxygen availability or changes in substrate accessibility under saturated conditions (Veres et al., 2015; Daunoras et al., 2024). Moreover, the 23-Sandy spoil land cover also showed consistently lower enzymatic activity, which could be attributed to lower substrate availability in this type of soil (Allison et al., 2011; Babcock and Esen, 1994).
4.1.2 Hydrolytic enzyme activities across seasons
Insignificant seasonal differences in the enzymatic activity across several land covers like 6-Cypress, 8-Hammock, 15-Sawtooth Palmetto, 20-Native Pasture, 21-Improved Pasture, and 22-Abandoned Citrus Grove could be a result of the interactions of several soil and environmental factors with a diverse range of vegetation (Shao et al., 2015; Ullah et al., 2013; Wallenstein et al., 2009; Boerner et al., 2005; Kang and Freeman, 1999). This interaction might have resulted in insignificant changes in enzyme activity from dry to wet season enzymatic activity, minimally influencing SOM decomposition rates and the turnover of SOM components. The presence of aeric moisture subclass in most of these ecosystems is characterized by a well-aerated soil environment (Soil Survey Staff, 2003; Soil Survey Staff, 1999) potentially could also cause these results. The sufficient aeration could have led to consistent enzymatic activity, making the transition to wetter conditions less impactful on enzymatic processes and SOM decomposition (Wang et al., 2022; Ge et al., 2020). Moreover, the presence of Spodosols soil order with sandy texture (McKeague et al., 1983) and poor drainage capacity in most of these ecosystems may have promoted consistent hydrological conditions resulting in insignificant changes in enzyme activity.
The increase in some specific enzymatic activity in land covers like 1-Flatwood, 2-Mesic flatwood, 3-Dry hammock, 5-Palm hammock (Xeric) and 7-Pinewood-1 A- Upland ecosystems, during the transition from dry to wet season can be attributed to the inherent attributes of trees and their environmental interactions, enhancing SOM decomposition (Błońska et al., 2021). Trees such as oaks and pines contribute rich SOM through leaf litter, enhancing substrate availability for microbes and facilitating the decomposition of both labile and stable SOM components thereby promoting enzymatic activity (Prescott and Vesterdal, 2021; Prescott and Grayston, 2013; Hättenschwiler, 2005). Similarly diverse root exudates from species like sabal palm can stimulate microbial communities to produce enzymes further enhancing SOM decomposition (Zhang, et al., 2019; Grayston et al., 1997). The presence of adequate moisture in this resource-rich environment in wet season could also have increased enzymatic activity (Brockett, et al., 2012). On the other hand, the nonsignificant differences among the majority of land covers within this ecosystem demonstrate that the forest canopy creates a stable microclimate, maintaining consistent conditions that protect microbial enzymatic activity from seasonal extremes ensuring stable SOM decomposition rates (De Frenne, et al., 2021; Breshears et al., 1998). Contrarily to this, 9-Pinewood-2 land cover despite having similar vegetative and soil conditions as other upland forests, showed a significant reduction in AP and AS. This reduction may stem from unique interactions between pine root exudates, microbial communities, and other soil and environmental factors under wet conditions (Prescott and Grayston, 2013; Shi et al., 2011). The composition of root exudates, particularly organic acids, significantly influences soil bacterial community structure and diversity (Shi et al., 2011), and some pine root exudates may reduce soil enzyme activity (Zhang et al., 2015). For instance, certain exudates may favor microbes that produce fewer extracellular enzymes, thereby decreasing the breakdown of organic matter (Kawasaki et al., 2021; Ferreira et al., 2024). These factors, along with some other soil and environmental factors, could have affected AP and AS activity uniquely in wet conditions (Huang et al., 2011; Baligar et al., 2005). The decline in enzymatic activities among land covers like 11-Wetland-1, 12-Wetland-2, and 14-Wetland-4 within Group B Aquatic and Wetland ecosystems could be attributed to the saturated conditions of these ecosystems, which may hinder oxygen diffusion and microbial activity necessary for enzyme production thus detrimentally affecting decomposition of SOM (Borowik and Wyszkowska, 2016; de Macedo et al., 2002; Eswaran, 1982). Aquatic plants typically thrive in water-saturated soils, which may experience enzymatic dilution or washout due to excessive wetness leading to a significant reduction in βG activity in the wet season also indicating a decline in the decomposition rates (Długosz et al., 2023; Xue et al., 2018). However, the significant increase in βG and AP activities in the 10-canal (wet) land cover, with similar conditions highlights the fact that wetland plants like Cattails and Pickerelweed growing in land cover might have enhanced the microbial substrate (Mallison et al., 2001; Sistani et al., 1999) and conditions conducive to enzymatic reactions during wet seasons (Rejmánková and Sirová, 2007). These land covers, along with some others exhibiting decreased enzymatic activity, was observed to fall under Alfisols or Entisols, suggesting soil orders may play a role in enzymatic responses to moisture changes. Alfisols, with their finer textures and higher nutrient contents, tend to retain moisture but may become waterlogged in wet conditions, inhibiting oxygen flow and reducing the activity of aerobic microbes responsible for enzymatic production (de Maced eta al., 2002; Russell, 1978; Rust, 1983). Entisols, conversely, are often sandy and well-drained, leading to rapid moisture percolation. In wet seasons, this could have resulted in the leaching of nutrients necessary for microbial activity, thus reducing enzymatic activity (Grossman, 1983). These all observations emphasize the critical roles of vegetation, soil type, and hydrological conditions in regulating enzymatic activities, reflecting the complex ecological processes that govern SOM decomposition. Vegetation influences enzyme activity through the type and quality of organic inputs, including litter and root exudates, which act as substrates for microbial metabolism. For instance, flatwood ecosystems dominated by pine, oak and palms, wetlands with aquatic plants, prairie grasses, and shrublands each contribute distinct litter chemistry and root exudates, thereby shaping microbial communities and enzyme production (Zhang et al., 2022; Schroeter et al., 2022; Meena and Rao, 2020). These plant-soil-microbe interactions directly influence the expression of enzymes involved in nutrient cycling. Soil type further modulates enzymatic activity through its inherent physical and chemical properties. Soils classified as Entisols and Alfisols, which dominate large portions of the study area, differ in their development, organic matter content, and nutrient-holding capacity. In particular, loamy textured soils typically provide higher water retention and improved aeration (Zega, 2024). Compared to sandy soils, supporting more stable microbial habitats and enhancing enzyme activity (Kim, 2015; Schnecker et al., 2014; Sinsabaugh et al., 2008). Hydrological conditions, especially the contrast between wet and dry seasons, strongly influence soil enzyme dynamics. In dry conditions, moderate moisture and aerobic environments enhance microbial respiration and promote the activity of hydrolytic enzymes. In contrast, wet conditions, especially in poorly drained wetland systems can lead to waterlogging, reduce oxygen availability, and lower redox potential, thereby suppressing enzyme production and microbial function (Hammerl et al., 2019; Kapila et al., 2017; Liao et al., 2016). Together, these interrelated factors along with other environmental variables shape the biochemical and microbial environment, ultimately driving the decomposition of SOM across diverse ecosystems.
4.1.3 Comparison across ecosystems
Among different ecosystems, the broad range of enzymatic activity in C-Shrub ecosystems in dry season can be attributed to dense and diverse shrub vegetation, such as Saw palmetto and Gal berry, which contribute significant plant debris to the soil. This debris serves as a rich source of SOM, enhancing a variety of enzymes necessary for decomposing plant material (Charlebois, et al., 2010; Archibold and Archibold, 1995). On the other hand, the absence of vegetation in barren ecosystems might also contribute to the lowest enzymatic activities observed (Minick et al., 2022).
Across different ecosystems, higher βG activities in the wet season under A-Flatwoods and C-Shrub ecosystems can be attributed to the combined influence of higher available water storage capacity and sufficient substrate availability (Diédhiou et al., 2020; Bracho et al., 2008), which are favorable conditions indicative of high SOM decomposition (Blonska et al., 2020; Weintraub et al., 2013). For β-NAG, higher activities in A-Upland Forests, B-Range Areas, and C-Shrub ecosystems during both seasons can be attributed to optimal moisture levels (Borowik and Wyszkowska, 2016) combined with sufficient substrate availability (Song et al., 2021; Yao et al., 2019), conditions that promote SOM decomposition (Blonska et al., 2020; Blonska et al., 2020; Weintraub et al., 2013). Moreover, higher AP activity in C-Shrub ecosystems during the dry season could be attributed to the role of shrubs in maintaining soil moisture and stimulating microbial activity under dry conditions (Diédhiou et al., 2020; Bach and Hofmockel, 2016), which likely enhances enzymatic activity in these ecosystems. AS showing minimal variation in both seasons indicates its relatively insensitive nature (Moreira et al., 2017; Wyszkowska et al., 2010). Several studies suggest that while some enzymes are sensitive to environmental changes, AS often exhibits stability under varying conditions (Moreira et al., 2017; Wyszkowska et al., 2010; Ekenler and Tabatabai, 2003; Bergstrom et al., 1998).
From dry to wet seasons the increase in βG and β-NAG activity in A-Upland Forests possibly due to the presence of Spodosols. These soils, with their sandy texture, can retain moisture without becoming waterlogged (New York State College of Agriculture and United States Soil Management Support Services, 1985). This moist yet aerated environment, enriched with organic material from varied vegetation (Prescott and Vesterdal, 2021; Prescott and Grayston, 2013; Hättenschwiler, 2005), likely encouraged microbial proliferation and enzymatic activity during the wet season. The decline in βG activity from the dry to the wet season within the B-Wetlands and C-Shrub ecosystems may be attributed to reduced microbial activity during the wet season, as during the dry season the presence of aeric and typic moisture subclasses likely promote greater microbial activity due to higher oxygen availability (Soil Survey Staff, 2003; Dekker et al., 1984). Conversely, in the wet season, the loamy soils with very poorly drained textures might lead to anaerobic, water-logged conditions, diminishing microbial activity and, consequently, enzymatic activities (Bogati et al., 2023; Steinweg et al., 2012).
4.1.4 HEDI across different land covers and ecosystems
The observed variability in the HEDI across different land covers and seasons shows the complex interplay of ecological factors influencing soil enzymatic activity and subsequent SOM decomposition (Wang, et al., 2020; Simpson et al., 2019). Low HEDI values, particularly in Canal (wet), indicate environments where conditions might be limiting for enzymatic processes, potentially due to factors like water saturation which could impede oxygen diffusion and thus microbial activity (Borowik and Wyszkowska, 2016).
The variation within ecosystems, particularly the contrast between the broad variability in Upland Forests and the consistently low activity in Barren ecosystems, highlights the role of vegetation (Li et al., 2015; Yin et al., 2014; Caldwell, et al., 1999) and soil characteristics (Błońska et al., 2017) in shaping enzymatic activity. Upland Forests, with a mix of vegetation supplying sufficient SOM, create conditions that can either foster or inhibit microbial activity depending on seasonal changes (Prescott and Vesterdal, 2021; Prescott and Grayston, 2013). For example, in favorable conditions, such as during moderate moisture and temperature, this vegetation-derived SOM provides abundant substrates that stimulate microbial growth and enzyme production (Osman, 2013; Baldrian and Štursová, 2010). However, in wet conditions, excess organic matter can lead to reduced oxygen availability or the accumulation of inhibitory compounds (e.g., polyphenols), which may suppress microbial processes (Zak et al., 2019; Zak et al., 2015; Myers et al., 2001). In Barren ecosystems, the minimal fluctuation in HEDI values suggests that the lack of vegetation and potentially poor soil conditions provide limited organic substrates for microbial decomposition, leading to consistently low enzymatic activity regardless of season (De Varennes and Torres, 2011; Badiane et al., 2001).
The observed shift towards higher HEDI values in A-Upland Forests, D-Range Areas and E-Barren ecosystems and lowering down in B-Wetland ecosystems and C-Shrub ecosystems during the wet season, underlines the complexity of SOM decomposition dynamics (Lehmann and Kleber, 2015; Schnitzer and Khan, 1975). While moisture is generally favorable for decomposition but only in optimum amount, it can also lead to conditions that may not always benefit enzymatic activity, as seen in the Range Areas where excessive moisture may not yield increased enzymatic action (Brockett et al., 2012; Hinojosa et al., 2004).
4.2 Relationship between soil physiochemical properties and hydrolytic enzyme activities in wet and dry seasons
The study revealed significant seasonal variations in soil enzyme-property relationships, emphasizing the dynamic nature of soil enzymes. Enzyme activities, strongly associated with microbial activity, showed positive correlations with OM (Kouchou et al., 2017; Madejón et al., 2001), SP (Theng, 2012; Ladd, 1985), and AC (Bhaduri et al., 2016; Zhongmei and Changchun, 2008), highlighting their roles in substrate availability and SOC decomposition (Smith et al., 2018; Kumar and Sharma, 2019). Additionally, enzyme activities are influenced by nutrient concentration and cycling (Ndlovu et al., 2023), as nutrients regulate microbial metabolism and enzyme synthesis (Liu et al., 2022; Chettri et al., 2020), aligning with the positive correlation observed with CEC. MWHC, meanwhile, improves water retention and provides essential nutrients for soil microorganisms (Mohammadi et al., 2011). The negative correlation between bulk density and enzyme activities across seasons supports Wang et al.'s (2019) conclusions on the impact of soil compaction on microbial processes. Moreover, BD is negatively related to SOM (Tanveera et al., 2016; Ahad et al., 2015; Chaudhari et al., 2013; Angers and Simard, 1986), a key driver of enzymatic activity, further emphasizing the negative correlation between BD and enzyme activity. Seasonal shifts in enzyme correlations with reduction from the dry to wet season suggest changes in microbial resource allocation strategies, consistent with observations by Merino et al. (2016) regarding microbial adaptations to environmental fluctuations and nutrient demands in wet conditions.
The multiple linear regression analysis reveals dynamic seasonal shifts in soil-enzyme interactions, highlighting the complex nature of these relationships (Lee et al., 2021; Weintraub et al., 2013). The decreased R2 values for βG and β-NAG in the wet season suggest that these carbon and nitrogen-cycling enzymes may be more sensitive to changes in soil moisture and associated environmental conditions. This aligns with the findings by Steinweg et al. (2012), who observed that soil moisture can significantly affect hydrolytic enzyme activities. Conversely, the increased R2 values AP and AS in the wet season indicate that phosphorus and sulfur-cycling enzymes may be more strongly regulated by moisture-related factors. This could be due to increased substrate availability or changes in microbial community composition under wetter conditions, as suggested by Burns et al. (2013). The HEDI values showed strong associations with a broader range of soil properties in the dry season, where substrate availability and microbial activity were key drivers (Sistla and Schimel, 2013) but became more streamlined to fewer predictors in the wet season, where soil hydrology played a dominant role (Sistla and Schimel, 2013; Weintraub et al., 2013). This shift indicates that dominant controlling factors for overall enzyme activity may change between seasons, reflecting alterations in substrate availability, microbial community dynamics, or physicochemical soil properties (Wallenstein and Burns, 2011).
5 Conclusion
The investigation into the variations of hydrolytic enzyme activities between dry and wet conditions across different land covers has provided significant insights into the underlying mechanisms driving SOM decomposition in various land covers during wet and dry season. The study underlines the complex interaction between soil biogeochemical properties, vegetation types, moisture levels, and enzymatic activity, highlighting their collective impact on the dynamics of SOM decomposition. The higher βG activities observed during the wet season in A-Flatwoods and C-Shrub ecosystems emphasize the importance of adequate water storage and substrate availability in facilitating SOM decomposition under hydrologically favourable conditions. Similarly, the consistently elevated β-NAG activities across A-Upland Forests, B-Range Areas, and C-Shrub ecosystems indicate that optimal moisture levels and sufficient substrates are critical drivers of microbial activity and SOM decomposition. The increased AP activity in C-Shrub ecosystems during the dry season reflects the adaptive role of shrubs in maintaining soil moisture and promoting microbial processes under arid conditions. Conversely, the minimal seasonal variation in AS activity suggests its relative insensitivity to environmental fluctuations, highlighting its potential as a stable indicator of soil enzymatic functionality. Moreover, among ecosystems, B-Wetlands demonstrated consistently low enzymatic activity in wet season due to water saturation hindering oxygen diffusion and microbial activity. In contrast, upland forests and shrub ecosystems showed higher enzyme activities due to favourable conditions such as adequate substrate availability and well-aerated soils. The HEDI further highlighted these patterns, with variability across ecosystems reflecting the interplay of vegetation, soil type, and moisture conditions.
The correlations and regression analyses provide a deeper understanding of how soil properties such as OM, SP, and AC consistently correlate positively with enzyme activity, reflecting their central roles in enhancing substrate supply and promoting microbial growth. Similarly, CEC and MWHC foster an environment conducive to enzymatic functions by improving nutrient retention and water availability. On the other hand, high BD and waterlogging in wetland or canal ecosystems restrict oxygen diffusion and reduce enzymatic activity, inhibiting SOM turnover. This variability indicates the adaptive strategies of microbial communities in response to changing environmental conditions, particularly in maintaining the balance between the decomposition of labile and stable SOM. The variability in HEDI values, serving as a proxy for soil enzymatic processes governing SOM decomposition, across land covers and seasons provides critical insights into ecological functioning within this subtropical preserve. These findings emphasize the necessity of tailored conservation strategies to enhance ecosystem resilience and functionality. For instance, in wetland systems, managing hydroperiods to reduce waterlogging can improve oxygen diffusion and enzymatic activity. In shrub ecosystems, preserving native vegetation supports adaptive processes during dry seasons by maintaining soil moisture. Similarly, in upland forests, optimizing substrate availability through organic matter inputs sustains βG and β-NAG driven decomposition. HEDI offers a novel, integrative index that combines the activity of key hydrolytic enzymes involved in SOM breakdown, enabling a more holistic understanding of microbial decomposition potential. Unlike traditional approaches that assess enzymes individually, HEDI provides a composite measure that reflects microbial functioning across diverse ecosystems and seasons. Moreover, integrating HEDI into a monitoring framework and accounting for the complex interactions among soil properties, vegetation, and moisture can help track early shifts in soil functionality and enable the design of conservation practices that can maintain the crucial balance between labile and stable carbon turnover in SOM.
Moreover, the land covers in this study were not evenly distributed across the five major ecosystems; however, the sampling design still allowed us to capture representative variability within and across land cover types. While the current sampling density revealed meaningful patterns in enzyme activity and SOM dynamics, future studies with more balanced representation and increased sampling intensity alongside consideration of additional environmental could further strengthen these insights. Importantly, the findings of this study offer a transferable framework for understanding SOM decomposition and soil functioning in other subtropical or ecologically similar landscapes, supporting informed soil management and conservation strategies beyond the DeLuca Preserve.
Data availability statement
The original contributions presented in the study are included in the article/Supplementary Material, further inquiries can be directed to the corresponding author.
Author contributions
SM: Conceptualization, Data curation, Formal Analysis, Investigation, Methodology, Validation, Visualization, Writing – original draft. NM: Conceptualization, Data curation, Formal Analysis, Investigation, Methodology, Writing – review and editing. AR: Formal Analysis, Investigation, Methodology, Writing – review and editing. NM-I: Conceptualization, Investigation, Methodology, Writing – review and editing. SS: Conceptualization, Funding acquisition, Investigation, Methodology, Project administration, Supervision, Writing – review and editing. AB: Conceptualization, Funding acquisition, Investigation, Methodology, Project administration, Supervision, Writing – review and editing. WM-H: Conceptualization, Funding acquisition, Investigation, Methodology, Project administration, Supervision, Writing – review and editing. JB: Conceptualization, Funding acquisition, Investigation, Methodology, Project administration, Supervision, Validation, Visualization, Writing – original draft.
Funding
The author(s) declare that financial support was received for the research and/or publication of this article. This work was supported by the University of Florida/Institute of Food and Agricultural Sciences DeLuca Preserve/Forest Systems Jumpstart Funding.
Acknowledgments
Additional support was provided by Bai Xue, Md. Anik Mahmud for their valuable discussions, technical assistance, and help with data interpretation. Special thanks to Salvador and Carolina for their help with lab analysis and ensuring the accuracy of experimental procedures.
Conflict of interest
The authors declare that the research was conducted in the absence of any commercial or financial relationships that could be construed as a potential conflict of interest.
The author(s) declared that they were an editorial board member of Frontiers, at the time of submission. This had no impact on the peer review process and the final decision.
Generative AI statement
The authors declare that no Generative AI was used in the creation of this manuscript.
Publisher’s note
All claims expressed in this article are solely those of the authors and do not necessarily represent those of their affiliated organizations, or those of the publisher, the editors and the reviewers. Any product that may be evaluated in this article, or claim that may be made by its manufacturer, is not guaranteed or endorsed by the publisher.
Supplementary material
The Supplementary Material for this article can be found online at: https://www.frontiersin.org/articles/10.3389/fenvs.2025.1564047/full#supplementary-material
References
Adl, S. M. (2003). The ecology of soil decomposition. Wallingford, Cambridge: CABI. doi:10.1079/9780851996615.0000
Ahad, T., Kanth, T. A., and Nabi, S. (2015). Soil bulk density as related to texture, organic matter content and porosity in kandi soils of district Kupwara (Kashmir Valley), India. Int. J. Sci. Res. 4 (1), 198–200.
Allison, S. D., Weintraub, M. N., Gartner, T. B., and Waldrop, M. P. (2011). Evolutionary-economic principles as regulators of soil enzyme production and ecosystem function. Soil Enzym., 229–243. doi:10.1007/978-3-642-14225-3_12
Amgain, N. R., Martens-Habbena, W., and Bhadha, J. H. (2022). Effect of dry and flooded rice as cover crops on soil health and microbial community on histosols. Agric. Res. 11 (4), 40. doi:10.5539/sar.v11n4p40
Anderson, J. M. (1991). The effects of climate change on decomposition processes in grassland and coniferous forests. Ecol. Appl. 1 (3), 326–347. doi:10.2307/1941761
Angers, D. A., and Simard, R. R. (1986). Relations entre la teneur en matière organique et la masse volumique apparente du sol. Can. J. soil Sci. 66 (4), 743–746. doi:10.4141/cjss86-074
Angst, G., Pokorný, J., Mueller, C. W., Prater, I., Preusser, S., Kandeler, E., et al. (2021). Soil texture affects the coupling of litter decomposition and SOM formation. Soil Biol. Biochem. 159, 108302. doi:10.1016/j.soilbio.2021.108302
Archibold, O. W., and Archibold, O. W. (1995). Terrestrial wetlands. Ecol. World Veg., 319–353. doi:10.1007/978-94-011-0009-0_10
Babcock, G. D., and Esen, A. (1994). Substrate specificity of maize β-glucosidase. Plant Sci. 101 (1), 31–39. doi:10.1016/0168-9452(94)90162-7
Bach, E. M., and Hofmockel, K. S. (2016). A time for every season: soil aggregate turnover stimulates decomposition and reduces carbon loss in grasslands managed for bioenergy. Gcb Bioenergy 8 (3), 588–599. doi:10.1111/gcbb.12267
Badiane, N. N. Y., Chotte, J. L., Pate, E., Masse, D., and Rouland, C. (2001). Use of soil enzyme activities to monitor soil quality in natural and improved fallows in semi-arid tropical regions. Appl. soil Ecol. 18 (3), 229–238. doi:10.1016/s0929-1393(01)00159-7
Baldrian, P., and Štursová, M. (2010). Enzymes in forest soils. Berlin, Heidelberg: Springer, 61–73. doi:10.1007/978-3-642-14225-3_4
Baligar, V. C., Wright, R. J., and Hern, J. L. (2005). Enzyme activities in soil influenced by levels of applied sulfur and phosphorus. Commun. soil Sci. plant analysis 36 (13-14), 1727–1735. doi:10.1081/CSS-200062431
Bautista-Cruz, A., and Ortiz-Hernández, Y. D. (2015). Hydrolytic soil enzymes and their response to fertilization: a short review. Comun. Sci. Hortic. J. 6 (3), 255–262. doi:10.14295/CS.V6I3.962
Beckett, R. P., Zavarzina, A. G., and Liers, C. (2013). Oxidoreductases and cellulases in lichens: possible roles in lichen biology and soil organic matter turnover. Fungal Biol. 117 (6), 431–438. doi:10.1016/j.funbio.2013.04.007
Berg, B. (2014). Decomposition patterns for foliar litter–a theory for influencing factors. Soil Biol. Biochem. 78, 222–232. doi:10.1016/j.soilbio.2014.08.005
Bergstrom, D. W., Monreal, C. M., and King, D. J. (1998). Sensitivity of soil enzyme activities to conservation practices. Soil Sci. Soc. Am. J. 62 (5), 1286–1295. doi:10.2136/sssaj1998.03615995006200050020x
Bhaduri, D., Saha, A., Desai, D., and Meena, H. N. (2016). Restoration of carbon and microbial activity in salt-induced soil by application of peanut shell biochar during short-term incubation study. Chemosphere 148, 86–98. doi:10.1016/j.chemosphere.2015.12.130
Black, R. J. (1993). Florida climate data. Univ. Fla. Coop. Ext. Serv. Inst. Food Agric. Sci., 1–4. EDIS. doi:10.32473/edis-ep108-2002
Blonska, E., Lasota, J., da Silva, G. R. V., Vanguelova, E., Ashwood, F., Tibbett, M., et al. (2020). SOM stabilization and carbon-cycling enzyme activity are affected by land management. Ann. For. Res. 63 (1), 71–86. doi:10.1016/j.foreco.2019.05.040
Błońska, E., Lasota, J., and Zwydak, M. (2017). The relationship between soil properties, enzyme activity and land use. doi:10.1515/frp-2017-0004
Błońska, E., Piaszczyk, W., Staszel, K., and Lasota, J. (2021). Enzymatic activity of soils and soil organic matter stabilization as an effect of components released from the decomposition of litter. Appl. Soil Ecol. 157, 103723. doi:10.1016/j.apsoil.2020.103723
Boddy, L. Y. N. N. E. (1986). “Water and decomposition processes in terrestrial ecosystems,” in Water, fungi and plants (Cambridge: Cambridge University Press), 375–398. doi:10.1525/9780520407114-003
Boerner, R. E. J., Brinkman, J. A., and Smith, A. (2005). Seasonal variations in enzyme activity and organic carbon in soil of a burned and unburned hardwood forest. Soil Biol. Biochem. 37 (8), 1419–1426. doi:10.1016/j.soilbio.2004.12.012
Bogati, K., Sewerniak, P., and Walczak, M. (2023). Effect of changes in soil moisture on agriculture soils: response of microbial community, enzymatic and physiological diversity. Ecol. Quest. 34 (4), 1–33. doi:10.12775/eq.2023.043
Borowik, A., and Wyszkowska, J. (2016). Soil moisture as a factor affecting the microbiological and biochemical activity of soil. Plant Soil Environ. 62 (6), 250–255. doi:10.17221/158/2016-PSE
Bracho, R., Powell, T. L., Dore, S., Li, J., Hinkle, C. R., and Drake, B. G. (2008). Environmental and biological controls on water and energy exchange in Florida scrub oak and pine flatwoods ecosystems. J. Geophys. Res. Biogeosciences 113 (G2). doi:10.1029/2007JG000469
Bremner, J. M., and Mulvaney, C. S. (1982). “Nitrogen-total,” in Methods of soil analysis. Part 2. Chemical and microbiological properties. Editor A. L. Page (New York: American Society of Agronomy, Soil Science Society of America), 595–624. doi:10.2134/agronmonogr9.2.2ed.c31
Breshears, D. D., Nyhan, J. W., Heil, C. E., and Wilcox, B. P. (1998). Effects of woody plants on microclimate in a semiarid woodland: soil temperature and evaporation in canopy and intercanopy patches. Int. J. Plant Sci. 159 (6), 1010–1017. doi:10.1086/297622
Brockett, B. F., Prescott, C. E., and Grayston, S. J. (2012). Soil moisture is the major factor influencing microbial community structure and enzyme activities across seven biogeoclimatic zones in western Canada. Soil Biol. Biochem. 44 (1), 9–20. doi:10.1016/j.soilbio.2011.09.003
Burns, R. G., DeForest, J. L., Marxsen, J., Sinsabaugh, R. L., Stromberger, M. E., Wallenstein, M. D., et al. (2013). Soil enzymes in a changing environment: current knowledge and future directions. Soil Biol. Biochem. 58, 216–234. doi:10.1016/j.soilbio.2012.11.009
Caldwell, B. A., Griffiths, R. P., and Sollins, P. (1999). Soil enzyme response to vegetation disturbance in two lowland Costa Rican soils. Soil Biol. Biochem. 31 (12), 1603–1608. doi:10.1016/s0038-0717(99)00067-x
Carter, D. O., and Tibbett, M. (2008). “Cadaver decomposition and soil: processes,” in Soil analysis in forensic taphonomy. Boca Raton, USA: (CRC Press), 41–64. doi:10.1201/9781420069921.ch2
Charlebois, D., Byers, P. L., Finn, C. E., and Thomas, A. L. (2010). Elderberry: botany, horticulture, potential. Hortic. Rev. 37 (37), 213–280. doi:10.1002/9780470543672.ch4
Chaudhari, P. R., Ahire, D. V., Ahire, V. D., Chkravarty, M., and Maity, S. (2013). Soil bulk density as related to soil texture, organic matter content and available total nutrients of Coimbatore soil. Int. J. Sci. Res. Publ. 3 (2), 1–8.
Chettri, B., Jang, W., and Seo, T. (2020). Adhaeribacter rhizoryzae sp. nov., a fibrillar matrix-producing bacterium isolated from the rhizosphere of rice plant. Int. J. Syst. and Evolu. Micro. 70 (10), 5382–5388. doi:10.1099/ijsem.0.004422
Condron, L., Stark, C., O’Callaghan, M., Clinton, P., and Huang, Z. (2010). The role of microbial communities in the formation and decomposition of SOM. Soil Microbiol. Sustain. crop Prod., 81–118. doi:10.1007/978-90-481-9479-7_4
Cooper, P. J. M. (1972). Aryl sulphatase activity in northern Nigerian soils. Soil Biol. Biochem. 4 (3), 333–337. doi:10.1016/0038-0717(72)90029-6
Cotrufo, M. F., and Lavallee, J. M. (2022). Soil organic matter formation, persistence, and functioning: a synthesis of current understanding to inform its conservation and regeneration. Adv. Agron. 172, 1–66. doi:10.1016/bs.agron.2021.11.002
Daunoras, J., Kačergius, A., and Gudiukaitė, R. (2024). Role of soil microbiota enzymes in soil health and activity changes depending on climate change and the type of soil ecosystem. Biology 13 (2), 85. doi:10.3390/biology13020085
De Frenne, P., Lenoir, J., Luoto, M., Scheffers, B. R., Zellweger, F., Aalto, J., et al. (2021). Forest microclimates and climate change: importance, drivers and future research agenda. Glob. Change Biol. 27 (11), 2279–2297. doi:10.1111/gcb.15569
Dekker, L. W., Wösten, J. H. M., and Bouma, J. (1984). Characterizing the soil moisture regime of a Typic Haplohumod. Geoderma 34 (1), 37–42. doi:10.1016/0016-7061(84)90004-1
de Macedo, J. R., Meneguelli, N. D. A., Ottoni Filho, T. B., and de Sousa Lima, J. A. (2002). Estimation of field capacity and moisture retention based on regression analysis involving chemical and physical properties in Alfisols and Ultisols of the state of Rio de Janeiro. Commun. soil Sci. plant analysis 33 (13-14), 2037–2055. doi:10.1081/css-120005747
Denef, K., Six, J., Bossuyt, H., Frey, S. D., Elliott, E. T., Merckx, R., et al. (2001). Influence of dry–wet cycles on the interrelationship between aggregate, particulate organic matter, and microbial community dynamics. Soil Biol. Biochem. 33 (12-13), 1599–1611. doi:10.1016/s0038-0717(01)00076-1
Deng, L., Zhu, G. Y., Tang, Z. S., and Shangguan, Z. P. (2016). Global patterns of the effects of land-use changes on soil carbon stocks. Glob. Ecol. Conservation 5, 127–138. doi:10.1016/j.gecco.2015.12.004
De Varennes, A., and Torres, M. O. (2011). Post-fallow tillage and crop effects on soil enzymes and other indicators. Soil use Manag. 27 (1), 18–27. doi:10.1111/j.1475-2743.2010.00307.x
Diedhiou, S., Assigbetsee, K. B., Goudiaby, A. O. K., Diedhiou, I., Badiane, A. N., Sène, M., et al. (2020). Arid agroecosystem shrubs enhance enzyme activities during the dry season. doi:10.4236/ajps.2020.112014
Dijkstra, F. A., and Cheng, W. (2007). Moisture modulates rhizosphere effects on C decomposition in two different soil types. Soil Biol. Biochem. 39 (9), 2264–2274. doi:10.1016/j.soilbio.2007.03.026
Długosz, J., Piotrowska-Długosz, A., and Breza-Boruta, B. (2023). The effect of differences in soil water content on microbial and enzymatic properties across the soil profiles. Ecohydrol. Hydrobiology. doi:10.1016/j.ecohyd.2023.06.010
Don, A., Böhme, I. H., Dohrmann, A. B., Poeplau, C., and Tebbe, C. C. (2017). Microbial community composition affects soil organic carbon turnover in mineral soils. Biol. Fertil. soils 53 (4), 445–456. doi:10.1007/s00374-017-1198-9
Ekenler, M., and Tabatabai, M. A. (2003). Responses of phosphatases and arylsulfatase in soils to liming and tillage systems. J. Plant Nutr. Soil Sci. 166 (3), 281–290. doi:10.1002/jpln.200390045
Eswaran, H. (1982). “Alfisols and ultisols,” in Proceedings of South pacific regional forum on soil taxonomy, suva, Fiji, 2-13 november 1981. Editors R. J. Morrison,, and D. M. Leslie (Suva, Fiji: Institute of Natural Resources, University of the South Pacific), 1982. doi:10.25082/ree.2020.02.001
Exum, J. H. (2020). Conservation easement baseline documentation report DeLuca preserve Osceola county. Florida: Exum Associated Inc. doi:10.3133/ofr791595
Fageria, N. K. (2012). Role of soil organic matter in maintaining sustainability of cropping systems. Commun. soil Sci. plant analysis 43 (16), 2063–2113. doi:10.1080/00103624.2012.697234
Ferreira, M. I., Marais, A., Botha, A., Reinhardt, C. F., and Rijst, M. (2024). Root exudates from weedy ryegrass hybrid type and selected crop plants affect soil microbial communities in two soil types of the Western Cape, South Africa. West. Cape, S. Afr. 4, 2378. doi:10.54517/ama.v4i2.2378
Filipović, A., Perčin, A., Hadžiabulić, A., and Mandić, A. (2024). “Transformation of SOM and impact on the ecosystem,” in Agroforestry for carbon and ecosystem management (Academic Press), 311–329. doi:10.1016/b978-0-323-95393-1.00018-x
Findlay, S. E. (2021). “SOM decomposition,” in Fundamentals of ecosystem science (Academic Press), 81–102. doi:10.1016/b978-0-12-812762-9.00004-6
Frøseth, R. B., and Bleken, M. A. (2015). Effect of low temperature and soil type on the decomposition rate of soil organic carbon and clover leaves, and related priming effect. Soil Biol. Biochem. 80, 156–166. doi:10.1016/j.soilbio.2014.10.004
Furtak, K., Gałązka, A., and Niedźwiecki, J. (2020). Changes in soil enzymatic activity caused by hydric stress. Pol. J. Environ. Stud. 29 (4), 2653–2660. doi:10.15244/pjoes/112896
Ge, M., Zhou, H., Shen, Y., Meng, H., Li, R., Zhou, J., et al. (2020). Effect of aeration rates on enzymatic activity and bacterial community succession during cattle manure composting. Bioresour. Technol. 304, 122928. doi:10.1016/j.biortech.2020.122928
Glassman, S. I., Weihe, C., Li, J., Albright, M. B., Looby, C. I., Martiny, A. C., et al. (2018). Decomposition responses to climate depend on microbial community composition. Proc. Natl. Acad. Sci. 115 (47), 11994–11999. doi:10.1073/pnas.1811269115
Goebel, M. O., Bachmann, J., Reichstein, M., Janssens, I. A., and Guggenberger, G. (2011). Soil water repellency and its implications for SOM decomposition–is there a link to extreme climatic events? Glob. Change Biol. 17 (8), 2640–2656. doi:10.1111/j.1365-2486.2011.02414.x
Grayston, S. J., Vaughan, D., and Jones, D. (1997). Rhizosphere carbon flow in trees, in comparison with annual plants: the importance of root exudation and its impact on microbial activity and nutrient availability. Appl. soil Ecol. 5 (1), 29–56. doi:10.1016/s0929-1393(96)00126-6
Grossman, R. B. (1983). Chapter 2 Entisols. Dev. soil Sci. 11, 55–90. doi:10.1016/s0166-2481(08)70613-5
Guo, L. B., and Gifford, R. M. (2002). Soil carbon stocks and land use change: a meta analysis. Glob. change Biol. 8 (4), 345–360. doi:10.1046/j.1354-1013.2002.00486.x
Hammerl, V. B., Grant, K., Pritsch, K., Jentsch, A., Schloter, M., Beierkuhnlein, C., et al. (2019). Seasonal effects of extreme weather events on potential extracellular enzyme activities in a temperate grassland soil. Front. Environ. Sci. 6, 157. doi:10.3389/fenvs.2018.00157
Harrison-Kirk, T., Beare, M. H., Meenken, E. D., and Condron, L. M. (2014). Soil organic matter and texture affect responses to dry/wet cycles: changes in soil organic matter fractions and relationships with C and N mineralisation. Soil Biol. Biochem. 74, 50–60. doi:10.1016/j.soilbio.2014.02.021
Hassan, W., Chen, W., Cai, P., and Huang, Q. (2013). Oxidative enzymes, the ultimate regulator: implications for factors affecting their efficiency. J. Environ. Qual. 42 (6), 1779–1790. doi:10.2134/jeq2013.05.0204
Hättenschwiler, S. (2005). “Effects of tree species diversity on litter quality and decomposition,” in Forest diversity and function: temperate and boreal systems (Berlin, Heidelberg: Springer Berlin Heidelberg), 149–164. doi:10.1007/3-540-26599-6_8
Haynes, R. J. (1986). The decomposition process: mineralization, immobilization, humus formation, and degradation. Mineral nitrogen plant-soil Syst., 52–126. doi:10.1016/b978-0-12-334910-1.50006-6
Henry, H. A. (2013). Reprint of “Soil extracellular enzyme dynamics in a changing climate”. Soil Biol. Biochem. 56, 53–59. doi:10.1016/j.soilbio.2012.10.022
Hinojosa, M. B., Carreira, J. A., García-Ruíz, R., and Dick, R. P. (2004). Soil moisture pre-treatment effects on enzyme activities as indicators of heavy metal-contaminated and reclaimed soils. Soil Biol. Biochem. 36 (10), 1559–1568. doi:10.1016/j.soilbio.2004.07.003
Huang, W., Liu, J., Zhou, G., Zhang, D., and Deng, Q. (2011). Effects of precipitation on soil acid phosphatase activity in three successional forests in southern China. Biogeosciences 8 (7), 1901–1910. doi:10.5194/bg-8-1901-2011
Jenkinson, D. S., and Polson, D. S. (1976). The effects of biocidal treatments on metabolism in soil-I. Fumigation with chloroform. Soil Biol. Biochem. 8 (3), 167–177. doi:10.1016/0038-0717(76)90001-8
Kang, H., and Freeman, C. (1999). Phosphatase and arylsulphatase activities in wetland soils: annual variation and controlling factors. Soil Biol. Biochem. 31 (3), 449–454. doi:10.1016/s0038-0717(98)00150-3
Kapila, S., Devi, K., Thakur, S., and Rao, A. (2017). Acid phosphatase activity in some Indian Liverworts. Cryptogam. Bryol. 38 (3), 325–331. doi:10.7872/cryb/v38.iss3.2017.325
Karaca, S., Dengiz, O., Turan, İ. D., Özkan, B., Dedeoğlu, M., Gülser, F., et al. (2021). An assessment of pasture soils quality based on multi-indicator weighting approaches in semi-arid ecosystem. Ecol. Indic. 121, 107001. doi:10.1016/j.ecolind.2020.107001
Kawasaki, A., Dennis, P. G., Forstner, C., Raghavendra, A. K. H., Mathesius, U., Richardson, A., et al. (2021). Manipulating exudate composition from root apices shapes the microbiome throughout the root system. Plant Physiol. 187, 2279–2295. doi:10.1093/plphys/kiab337
Kim, H. (2015). A review of factors that regulate extracellular enzyme activity in wetland soils. Korean J. Microbiol. 51 (2), 97–107. doi:10.7845/kjm.2015.4087
Koch, O., Tscherko, D., and Kandeler, E. (2007). Temperature sensitivity of microbial respiration, nitrogen mineralization, and potential soil enzyme activities in organic alpine soils. Glob. Biogeochem. Cycles 21 (4). doi:10.1029/2007gb002983
Körschens, M. (2002). Importance of soil organic matter (SOM) for biomass production and environment (a review). Archives Agron. Soil Sci. 48 (2), 89–94. doi:10.1080/03650340214162
Kouchou, A., Rais, N., Thoisy, J. C., Duplay, J., Ghazi, M., Elsass, F., et al. (2017). Behavior of enzyme activities exposed to contamination by heavy metals and dissolved organic carbon in calcareous agricultural soils. Soil Sediment Contam. Int. J. 26 (3), 259–276. doi:10.1080/15320383.2017.1289499
Kumar, A., and Sharma, S. (2019). Microbes and enzymes in soil health and bioremediation. Singapore: Springer, 353–366. doi:10.1007/978-981-13-9117-0
Kumar, S., Lal, R., and Lloyd, C. D. (2012). Assessing spatial variability in soil characteristics with geographically weighted principal components analysis. Comput. Geosci. 16, 827–835. doi:10.1007/s10596-012-9290-6
Kunito, T., Kurita, H., Kumori, M., Sakaguchi, K., Nishizawa, S., Fujita, K., et al. (2022). Microbial synthesis of arylsulfatase depends on the soluble and adsorbed sulfate concentration in soils. Eur. J. Soil Biol. 111, 103418. doi:10.1016/j.ejsobi.2022.103418
Ladd, J. N. (1985). “Soil enzymes,” in Soil organic matter and biological activity. Dordrecht: Springer Netherlands, 175–221. doi:10.1007/978-94-009-5105-1_6
Lascody, R., and Melbourne, N. (2002). The onset of the wet and dry seasons in East Central Florida, a subtropical wet-dry climate. Melbourne, USA: National Weather Service Weather Forecast Office Melbourne, FL. doi:10.3334/ornldaac/723
Lee, J., Kim, H. S., Jo, H. Y., and Kwon, M. J. (2021). Revisiting soil bacterial counting methods: Optimal soil storage and pretreatment methods and comparison of culture-dependent and -independent methods. PLOS ONE 16 (2), e0246142. doi:10.1371/journal.pone.0246142
Lehmann, J., and Kleber, M. (2015). The contentious nature of soil organic matter. Nature 528 (7580), 60–68. doi:10.1038/nature16069
Li, J., Zhou, X., Yan, J., Li, H., and He, J. (2015). Effects of regenerating vegetation on soil enzyme activity and microbial structure in reclaimed soils on a surface coal mine site. Appl. Soil Ecol. 87, 56–62. doi:10.1016/j.apsoil.2014.11.010
Liao, X., Inglett, P. W., and Inglett, K. S. (2016). Seasonal patterns of nitrogen cycling in subtropical short-hydroperiod wetlands: effects of precipitation and restoration. Sci. Total Environ. 556, 136–145. doi:10.1016/j.scitotenv.2016.02.203
Liu, C., Ma, J., Qu, T., Xue, Z., Li, X., Chen, Q., et al. (2022). Extracellular enzyme activity and stoichiometry reveal nutrient dynamics during microbially-mediated plant residue transformation. Forests 14 (1), 34. doi:10.3390/f14010034
Lohse, K. A., Brooks, P. D., McIntosh, J. C., Meixner, T., and Huxman, T. E. (2009). Interactions between biogeochemistry and hydrologic systems. Annu. Rev. Environ. Resour. 34, 65–96. doi:10.1146/annurev.environ.33.031207.111141
Madejón, E., Burgos, P., Murillo, J. M., and Cabrera, F. (2001). Phytotoxicity of organic amendments on activities of select soil enzymes. Commun. soil Sci. plant analysis 32 (13-14), 2227–2239. doi:10.1081/CSS-120000279
Mallison, C. T., Stocker, R. K., and Cichra, C. E. (2001). Physical and vegetative characteristics of floating islands. J. Aquatic Plant Manag. 39, 107–111. doi:10.1577/m03-043.1
Mariscal-Sancho, I., Ball, B., and McKenzie, B. (2018). Influence of tillage practices, organic manures and extrinsic factors on β-glucosidase activity: the final step of cellulose hydrolysis. Soil Syst. 2 (2), 21. doi:10.3390/soilsystems2020021
Martin-Sanz, J. P., de Santiago-Martin, A., Valverde-Asenjo, I., Quintana-Nieto, J. R., Gonzalez-Huecas, C., and Lopez-Lafuente, A. L. (2022). Comparison of soil quality indexes calculated by network and principal component analysis for carbonated soils under different uses. Ecol. Indic. 143, 109374. doi:10.1016/j.ecolind.2022.109374
Mayor, A. G. , Goirán, S. B., Vallejo, V. R., and Bautista, S. (2016). Variation in soil enzyme activity as a function of vegetation amount, type, and spatial structure in fire-prone Mediterranean shrublands. Sci. Total Environ. 573, 1209–1216. doi:10.1016/j.scitotenv.2016.03.139
McKeague, J. A., DeConinck, F., and Franzmeier, D. P. (1983). “Chapter 6 Spodosols,” in Developments in soil science (Elsevier), 11, 217–252. doi:10.1016/s0166-2481(08)70617-2
Meena, A., and Rao, K. S. (2020). Assessment of soil microbial and enzyme activity in the rhizosphere zone under different land use/cover of a semi-arid ecosystem. India. doi:10.21203/rs.3.rs-42033/v1
Merino, C., Godoy, R., and Matus, F. (2016). Soil enzymes and biological activity at different levels of SOM stability. J. Soil Sci. plant Nutr. 16 (1), 14–30. doi:10.4067/s0718-95162016005000002
Minick, K. J., Aguilos, M., Li, X., Mitra, B., Prajapati, P., and King, J. S. (2022). Effects of spatial variability and drainage on extracellular enzyme activity in coastal freshwater forested wetlands of Eastern North Carolina, USA. Forests 13 (6), 861. doi:10.3390/f13060861
Mir, Y. H., Ganie, M. A., Shah, T. I., Bangroo, S. A., Mir, S. A., Shah, A. M., et al. (2023). Soil microbial and enzyme activities in different land use systems of the Northwestern Himalayas. PeerJ 11, e15993. doi:10.7717/peerj.15993
Moghimian, N., Hosseini, S. M., Kooch, Y., and Darki, B. Z. (2017). Impacts of changes in land use/cover on soil microbial and enzyme activities. Catena 157, 407–414. doi:10.1016/j.catena.2017.06.003
Mohammadi, K., Heidari, G., Khalesro, S., and Sohrabi, Y. (2011). Soil management, microorganisms and SOM interactions: a review. Afr. J. Biotechnol. 10 (86), 19840. doi:10.5897/ajbx11.006
Moreira, R. S., Chiba, M. K., Nunes, S. B., and Maria, I. D. (2017). Air-drying pretreatment effect on soil enzymatic activity. Plant Soil Environ. 63, 29–33. doi:10.17221/656/2016-PSE
Muñoz-Rojas, M., Jordán, A., Zavala, L. M., De la Rosa, D., Abd-Elmabod, S. K., and Anaya-Romero, M. (2015). Impact of land use and land cover changes on organic carbon stocks in Mediterranean soils (1956–2007). Land Degrad. Dev. 26 (2), 168–179. doi:10.1002/ldr.2194
Murphy, B. W. (2015). Impact of soil organic matter on soil properties—a review with emphasis on Australian soils. Soil Res. 53 (6), 605–635. doi:10.1071/sr14246
Myers, R. T., Zak, D. R., White, D. C., and Peacock, A. D. (2001). Landscape-level patterns of microbial community composition and substrate use in upland forest ecosystems. Soil Sci. Soc. Am. J. 65 (2), 359–367. doi:10.2136/SSSAJ2001.652359X
Nair, P. R., Kumar, B. M., Nair, V. D., Nair, P. R., Kumar, B. M., and Nair, V. D. (2021). Soil organic matter (SOM) and nutrient cycling. Introd. Agrofor. Four Decades Sci. Dev., 383–411. doi:10.1007/978-3-030-75358-0_16
National Resources Conservation Service (2024). Soil survey geographic Database (SSURGO). United States Department of Agriculture. Available online at: https://www.nrcs.usda.gov.
Ndlovu, S., Suinyuy, T. N., Pérez-Fernández, M. A., and Magadlela, A. (2023). Encephalartos natalensis, their nutrient-cycling microbes and enzymes: a story of successful trade-offs. Plants 12 (5), 1034. doi:10.3390/plants12051034
Nedyalkova, K., Donkova, R., and Malinov, I. (2020). Acid phosphatase activity under the impact of erosion level in agricultural soils of different type and land use. Bulg. J. Agric. Sci. 26 (6).
New York State College of Agriculture, Life Sciences. Department of Agronomy, and United States. Soil Management Support Services (1985). Keys to soil taxonomy: Alfisols, aridisols, Entisols, histosols, inceptisols, mollisols, oxisols, Spodosols, ultisols, vertisols, soil family (No. 6). Soil Manag. Support Serv. doi:10.21474/ijar01/12414
Nieder, R., and Benbi, D. K. (2008). Carbon and nitrogen transformations in soils. Carbon nitrogen Terr. Environ., 137–159. doi:10.1007/978-1-4020-8433-1_5
Osman, K. T. (2013). Organic matter of forest soils. Cham: Springer, 63–76. doi:10.1007/978-3-319-02541-4_4
Osman, K. T., and Osman, K. T. (2013). Soil organic matter (SOM). In Soils: principles. Dordrecht, Netherlands: Properties and Management, 89–96. doi:10.1007/978-94-007-5663-2_7
Ouyang, Y., and Li, X. (2013). Recent research progress on soil microbial responses to drying–rewetting cycles. Acta Ecol. Sin. 33 (1), 1–6. doi:10.1016/j.chnaes.2012.12.001
Paul, E. A. (2016). The nature and dynamics of soil organic matter: plant inputs, microbial transformations, and organic matter stabilization. Soil Biol. Biochem. 98, 109–126. doi:10.1016/j.soilbio.2016.04.001
Paul, E. A., and Collins, H. P. (2020). “The characteristics of SOM relative to nutrient cycling,” in Methods for assessment of soil degradation Boca Raton, USA: (CRC Press), 181–197. doi:10.1201/9781003068716-9
Piotrowska, A., and Koper, J. (2010). Soil beta-βG activity under winter wheat cultivated in crop rotation systems depleting and enriching the soil in SOM. J. Elem. 15 (3), 593–600. doi:10.5601/jelem.2010.15.3.593-600
Prescott, C. E. (2010). Litter decomposition: what controls it and how can we alter it to sequester more carbon in forest soils? Biogeochemistry 101, 133–149. doi:10.1007/s10533-010-9439-0
Prescott, C. E., and Grayston, S. J. (2013). Tree species influence on microbial communities in litter and soil: current knowledge and research needs. For. Ecol. Manag. 309, 19–27. doi:10.1016/j.foreco.2013.02.034
Prescott, C. E., and Vesterdal, L. (2021). Decomposition and transformations along the continuum from litter to soil organic matter in forest soils. For. Ecol. Manag. 498, 119522. doi:10.1016/j.foreco.2021.119522
Ramesh, T., Bolan, N. S., Kirkham, M. B., Wijesekara, H., Kanchikerimath, M., Rao, C. S., et al. (2019). Soil organic carbon dynamics: impact of land use changes and management practices: a review. Adv. Agron. 156, 1–107. doi:10.1016/bs.agron.2019.02.001
Rastin, N., Rosenplänter, K., and Hüttermann, A. (1988). Seasonal variation of enzyme activity and their dependence on certain soil factors in a beech forest soil. Soil Biol. Biochem. 20 (5), 637–642. doi:10.1016/0038-0717(88)90147-2
Raza, T., Qadir, M. F., Khan, K. S., Eash, N. S., Yousuf, M., Chatterjee, S., et al. (2023). Unraveling the potential of microbes in decomposition of organic matter and release of carbon in the ecosystem. J. Environ. Manag. 344, 118529. doi:10.1016/j.jenvman.2023.118529
R Development Core Team (2023). R: a language and environment for statistical computing (Version 4.3.2). Vienna, Austria: R Foundation for Statistical Computing. [Computer software]. doi:10.1201/9780203738672-4
Rejmánková, E., and Sirová, D. (2007). Wetland macrophyte decomposition under different nutrient conditions: relationships between decomposition rate, enzyme activities and microbial biomass. Soil Biol. Biochem. 39 (2), 526–538. doi:10.1016/j.soilbio.2006.08.022
Rice, C. W. (2002). SOM and nutrient dynamics. Encycl. soil Sci. 2, 1180–1183. doi:10.1081/e-ess3-120002258
Rittl, T. F., Canisares, L., Sagrilo, E., Butterbach-Bahl, K., Dannenmann, M., and Cerri, C. E. (2020). Temperature sensitivity of soil organic matter decomposition varies with biochar application and soil type. Pedosphere 30 (3), 336–342. doi:10.1016/s1002-0160(20)60013-3
Robertson, G. P., and Paul, E. A. (2000). “Decomposition and SOM dynamics,” in Methods in ecosystem science (New York, NY: Springer New York), 104–116. doi:10.1007/978-1-4612-1224-9_8
Russell, M. B. (1978). “Profile moisture dynamics of soil in Vertisols and Alfisols,” in Proceedings, international workshop on the agroclimatological research needs of the semi-arid tropics, 22–24. doi:10.2134/asaspecpub34.c6
Rust, R. H. (1983). Chapter 7 Alfisols. Dev. Soil Sci. 11, 253–281. doi:10.1016/s0166-2481(08)70618-4
Salam, A. K., Afandi, , Sriyani, N., and Kimura, M. (2001). Soil enzymatic activities in a hilly coffee plantation in Lampung Province, South Sumatra, Indonesia, under plant cover management. J. Soil Sci. Plant Nutr. 47 (4), 695–702. doi:10.1080/00380768.2001.10408434
Salazar, S., Sánchez, L. E., Alvarez, J., Valverde, A., Galindo, P., Igual, J. M., et al. (2011). Correlation among soil enzyme activities under different forest system management practices. Ecol. Eng. 37 (8), 1123–1131. doi:10.1016/j.ecoleng.2011.02.007
Schimel, J. P. (2018). Life in dry soils: effects of drought on soil microbial communities and processes. Annu. Rev. Ecol. Evol. Syst. 49, 409–432. doi:10.1146/annurev-ecolsys-110617-062614
Schindelbeck, R. R., Moebius-Clune, B. N., Moebius-Clune, D. J., Kurtz, K. S., and van Es, H. M. (2016). Cornell University comprehensive assessment of soil health laboratory standard operating procedures. Ithaca, NY: Cornell Univ. doi:10.2134/jeq2016.05.0182
Schnecker, J., Wild, B., Hofhansl, F., Eloy Alves, R. J., Bárta, J., Čapek, P., et al. (2014). Effects of soil organic matter properties and microbial community composition on enzyme activities in cryoturbated arctic soils. PLoS One 9 (4), e94076. doi:10.1371/journal.pone.0094076
Schroeter, S. A., Eveillard, D., Chaffron, S., Zoppi, J., Kampe, B., Lohmann, P., et al. (2022). Microbial community functioning during plant litter decomposition. Dent. Sci. Rep. 12 (1), 7451. doi:10.1038/s41598-022-11485-1
Scott, N. A., Cole, C. V., Elliott, E. T., and Huffman, S. A. (1996). Soil textural control on decomposition and soil organic matter dynamics. Soil Sci. Soc. Am. J. 60 (4), 1102–1109. doi:10.2136/sssaj1996.03615995006000040020x
Shao, X., Yang, W., and Wu, M. (2015). Seasonal dynamics of soil labile organic carbon and enzyme activities in relation to vegetation types in Hangzhou Bay tidal flat wetland. PLoS One 10 (11), e0142677. doi:10.1371/journal.pone.0142677
Sherene, T. (2017). Role of soil enzymes in nutrient transformation: a review. Bio Bull. 3 (1), 109–131.
Shi, S., Richardson, A. E., O'Callaghan, M., DeAngelis, K. M., Jones, E. E., Stewart, A., et al. (2011). Effects of selected root exudate components on soil bacterial communities. FEMS Microbiol. Ecol. 77 (3), 600–610. doi:10.1111/j.1574-6941.2011.01150.x
Shi, W. (2010). Agricultural and ecological significance of soil enzymes: soil carbon sequestration and nutrient cycling. Berlin, Heidelberg: Springer, 43–60. doi:10.1007/978-3-642-14225-3_3
Simpson, R. M., Mason, K., Robertson, K., and Müller, K. (2019). Relationship between soil properties and enzyme activities with soil water repellency. Soil Res. 57 (6), 689–702. doi:10.1071/sr18199
Sinsabaugh, R. L., Lauber, C. L., Weintraub, M. N., Ahmed, B., Allison, S. D., Crenshaw, C., et al. (2008). Stoichiometry of soil enzyme activity at global scale. Ecol. Lett. 11 (11), 1252–1264. doi:10.1111/j.1461-0248.2008.01245.x
Sistani, K. R., Mays, D. A., and Taylor, R. W. (1999). Development of natural conditions in constructed wetlands: biological and chemical changes. Ecol. Eng. 12 (1-2), 125–131. doi:10.1016/s0925-8574(98)00058-5
Sistla, S. A., and Schimel, J. P. (2013). Seasonal patterns of microbial extracellular enzyme activities in an arctic tundra soil: identifying direct and indirect effects of long-term summer warming. Soil Biol. Biochem. 66, 119–129. doi:10.1016/j.soilbio.2013.07.003
Smith, P., Lutfalla, S., Riley, W. J., Torn, M. S., Schmidt, M. W., and Soussana, J. F. (2018). The changing faces of soil organic matter research. European Journal of Soil Science 69 (1), 23–30. doi:10.1111/ejss.12500
Soil Survey Staff (1999). “Soil taxonomy: a basic system of soil classification for making and interpreting soil surveys,” in U.S. Department of agriculture, natural resources conservation Service. 2nd ed. (Agriculture Handbook No. 436). doi:10.1111/j.1475-2743.2001.tb00008.x
Soil Survey Staff (2003). Keys to soil taxonomy. 9th ed. Washington, DC: United States Department of Agriculture, Natural Resources Conservation Service. doi:10.1111/j.1475-2743.2001.tb00008.x
Song, Y., Jiang, L., Song, C., Wang, X., Ma, X., Zhang, H., et al. (2021). Microbial abundance and enzymatic activity from tussock and shrub soil in permafrost peatland after 6-year warming. Ecol. Indic. 126, 107589. doi:10.1016/j.ecolind.2021.107589
Speir, T. W., and Ross, D. J. (2002). Hydrolytic enzyme activities to assess soil degradation and recovery. Enzymes in the environments: activity, ecology and applications, 407–431. doi:10.1201/9780203904039.ch16
Steinweg, J. M., Dukes, J. S., and Wallenstein, M. D. (2012). Modeling the effects of temperature and moisture on soil enzyme activity: linking laboratory assays to continuous field data. Soil Biol. Biochem. 55, 85–92. doi:10.1016/j.soilbio.2012.06.015
Sumner, M. E., Miller, W. P., and Sparks, D. (1996). Methods of soil analysis. Part 3. Chemical methods. Cation exchange capacity, and exchange coefficients. Madison: Soil Science Society of America and American Society of Agronomy, 65–94.
Swift, R. S. (2001). Sequestration of carbon by soil. Soil Sci. 166 (11), 858–871. doi:10.1097/00010694-200111000-00010
Tabatabai, M. A. (1994). Soil enzymes. Methods soil analysis Part 2 Microbiol. Biochem. Prop. 5, 775–833. doi:10.2136/sssabookser5.2.c37
Tanveera, A., Kanth, T. A., Tali, P. A., and Naikoo, M. (2016). Relation of soil bulk density with texture, total organic matter content and porosity in the soils of Kandi Area of Kashmir valley, India. Int. Res. J. Earth Sci. 4 (1), 1–6. doi:10.9734/JGEESI/2016/21344
Theng, B. K. G. (2012). Proteins and enzymes. Dev. Clay Sci. 4, 245–318. doi:10.1016/B978-0-444-53354-8.00008-6
Tischer, A., Blagodatskaya, E., and Hamer, U. (2015). Microbial community structure and resource availability drive the catalytic efficiency of soil enzymes under land-use change conditions. Soil Biol. Biochem. 89, 226–237. doi:10.1016/j.soilbio.2015.07.011
Tumer, A. R., Karacaoglu, E., Namli, A., Keten, A., Farasat, S., Akcan, R., et al. (2013). Effects of different types of soil on decomposition: an experimental study. Leg. Med. 15 (3), 149–156. doi:10.1016/j.legalmed.2012.11.003
Ullah, R., Lone, M. I., Ullah, K. S., Mehdi, S. M., and Qazi, M. A. (2013). Effect of cropping system and seasonal variation on soil microbial biomass and enzymatic activities in arid soils. doi:10.1007/s12665-014-3376-5
U.S. Department of Agriculture, Natural Resources Conservation Service (2024). Web soil survey. Available online at: https://websoilsurvey.sc.egov.usda.gov/.
Van Veen, J. A., and Kuikman, P. J. (1990). Soil structural aspects of decomposition of organic matter by micro-organisms. Biogeochemistry 11, 213–233. doi:10.1007/bf00004497
Veres, Z., Kotroczó, Z., Fekete, I., Tóth, J. A., Lajtha, K., Townsend, K., et al. (2015). Soil extracellular enzyme activities are sensitive indicators of detrital inputs and carbon availability. Appl. Soil Ecol. 92, 18–23. doi:10.1016/j.apsoil.2015.03.006
Wallenstein, M. D., and Burns, R. G. (2011). Ecology of extracellular enzyme activities and organic matter degradation in soil: a complex community-driven process. Methods of soil enzymology 9, 35–55. doi:10.2136/sssabookser9.c2
Wallenstein, M., Steinweg, J. M., and McMahon, S. (2009). Recent advancements in understanding the ecology of soil extracellular enzymes. Nat. Precedings. doi:10.1038/npre.2009.4115
Wang, B., and Allison, S. D. (2019). Emergent properties of organic matter decomposition by soil enzymes. Soil Biol. Biochem. 136, 107522. doi:10.1016/j.soilbio.2019.107522
Wang, C., Dippold, M. A., Blagodatskaya, E., and Dorodnikov, M. (2022a). Oxygen matters: short-and medium-term effects of aeration on hydrolytic enzymes in a paddy soil. Geoderma 407, 115548. doi:10.1016/j.geoderma.2021.115548
Wang, L., Pang, X., Li, N., Qi, K., Huang, J., and Yin, C. (2020). Effects of vegetation type, fine and coarse roots on soil microbial communities and enzyme activities in eastern Tibetan plateau. Catena 194, 104694. doi:10.1016/j.catena.2020.104694
Wang, S., Zhou, K., Mori, T., Mo, J., and Zhang, W. (2019). Effects of phosphorus and nitrogen fertilization on soil aryl sulfatase activity and sulfur availability of two tropical plantations in southern China. For. Ecol. Manag. 453, 117613. doi:10.1016/j.foreco.2019.117613
Weintraub, S. R., Wieder, W. R., Cleveland, C. C., and Townsend, A. R. (2013). Organic matter inputs shift soil enzyme activity and allocation patterns in a wet tropical forest. Biogeochemistry 114, 313–326. doi:10.1007/s10533-012-9812-2
Williams, M. A., and Rice, C. W. (2007). Seven years of enhanced water availability influences the physiological, structural, and functional attributes of a soil microbial community. Appl. Soil Ecol. 35 (3), 535–545. doi:10.1016/j.apsoil.2006.09.014
Wu, D., Chi, Q., Sui, X., Zhang, M., Zhang, M., Jia, H., et al. (2021). Metabolic diversity and seasonal variation of soil microbial communities in natural forested wetlands. J. For. Res. 32 (6), 2619–2631. doi:10.1007/S11676-021-01326-8
Wyszkowska, J., Kucharski, M., and Kucharski, J. (2010). Activity of beta-glucosidase, arylsulfatase and phosphatases in soil contaminated with copper. J. Elem. 15 (1), 213–226. doi:10.5601/jelem.2010.15.1.213-226
Xiang, S. R., Doyle, A., Holden, P. A., and Schimel, J. P. (2008). Drying and rewetting effects on C and N mineralization and microbial activity in surface and subsurface California grassland soils. Soil Biol. Biochem. 40 (9), 2281–2289. doi:10.1016/j.soilbio.2008.05.004
Xu, N., Amgain, N. R., Rabbany, A., Capasso, J., Korus, K., Swanson, S., et al. (2022). Interaction of soil health indicators to different regenerative farming practices on mineral soils. Agrosystems, Geosciences Environ. 5 (1), e20243. doi:10.1002/agg2.20243
Xue, W. L., Pan, W., Lu, Q., Xu, Q. R., Wu, C. N., and Du, S. T. (2018). Aquatic plant debris changes sediment enzymatic activity and microbial community structure. Environ. Sci. Pollut. Res. 25, 21801–21810. doi:10.1007/s11356-018-2310-x
Yao, Y., Shao, M., Fu, X., Wang, X., and Wei, X. (2019). Effects of shrubs on soil nutrients and enzymatic activities over a 0–100 cm soil profile in the desert-loess transition zone. Catena 174, 362–370. doi:10.1016/j.catena.2018.11.031
Yin, R., Deng, H., Wang, H. L., and Zhang, B. (2014). Vegetation type affects soil enzyme activities and microbial functional diversity following re-vegetation of a severely eroded red soil in sub-tropical China. Catena 115, 96–103. doi:10.1016/j.catena.2013.11.015
Zak, D., Roth, C., Gelbrecht, J., Fenner, N., and Reuter, H. (2015). Polyphenols as enzyme inhibitors in different degraded peat soils: implication for microbial metabolism in rewetted peatlands. Available online at: https://ui.adsabs.harvard.edu/abs/2015EGUGA.1714824Z/abstract.
Zak, D., Roth, C., Unger, V., Goldhammer, T., Fenner, N., Freeman, C., et al. (2019). Unraveling the importance of polyphenols for microbial carbon mineralization in rewetted riparian peatlands. Front. Environ. Sci. 7. doi:10.3389/FENVS.2019.00147
Zega, N. D. (2024). Pengaruh tekstur dan struktur tanah terhadap distribusi air dan udara di profil tanah. J. Ilmu Pertan. Dan. Perikan. 1 (2), 1–6. doi:10.70134/penarik.v1i2.52
Zhang, X., Dippold, M. A., Kuzyakov, Y., and Razavi, B. S. (2019). Spatial pattern of enzyme activities depends on root exudate composition. Soil Biol. Biochem. 133, 83–93. doi:10.1016/j.soilbio.2019.02.010
Zhang, Y., Chen, L., Wu, Z., and Sun, C. (2011). Kinetic parameters of soil β-glucosidase response to environmental temperature and moisture regimes. Rev. Bras. Ciência do Solo 35, 1285–1291. doi:10.1590/S0100-06832011000400022
Zhang, Y. Q., Chen, L., Pang, D., He, W., Li, X.-B., Wu, M.-Y., et al. (2022). Responses of soil microbial community structure to litter inputs. Chin. J. Appl. Ecol. 33 (11), 2943–2953. doi:10.13287/j.1001-9332.202211.031
Zhang, Z., Qiao, M., Li, D., Zhao, C., Li, Y., Yin, H., et al. (2015). Effects of two root-secreted phenolic compounds from a subalpine coniferous species on soil enzyme activity and microbial biomass. Chem. Ecol. 31 (7), 636–649. doi:10.1080/02757540.2015.1075515
Zheng, Q., Hu, Y., Zhang, S., Noll, L., Böckle, T., Dietrich, M., et al. (2019). Soil multifunctionality is affected by the soil environment and by microbial community composition and diversity. Soil Biol. Biochem. 136, 107521. doi:10.1016/j.soilbio.2019.107521
Zhongmei, W., and Changchun, S. (2008). Vertical dynamics of soil enzyme activities and its relationship with active organic carbon indicators in Calamagrostis angustifolia wetland. Wetl. Sci. 6 (2), 249–257.
Keywords: soil organic matter, decomposition, hydrolytic enzymes, land covers, wet season, dry season
Citation: Melkani S, Manirakiza N, Rabbany A, Medina-Irizarry N, Smidt S, Braswell A, Martens-Habbena W and Bhadha JH (2025) Understanding the mechanisms of hydrolytic enzyme mediated organic matter decomposition under different land covers within a subtropical preserve. Front. Environ. Sci. 13:1564047. doi: 10.3389/fenvs.2025.1564047
Received: 21 January 2025; Accepted: 07 April 2025;
Published: 24 April 2025.
Edited by:
Jing Liu, University of Birmingham, United KingdomReviewed by:
Vanessa Otero Jimenez, University of Idaho, United StatesYumin Cai, Northeastern University, United States
Copyright © 2025 Melkani, Manirakiza, Rabbany, Medina-Irizarry, Smidt, Braswell, Martens-Habbena and Bhadha. This is an open-access article distributed under the terms of the Creative Commons Attribution License (CC BY). The use, distribution or reproduction in other forums is permitted, provided the original author(s) and the copyright owner(s) are credited and that the original publication in this journal is cited, in accordance with accepted academic practice. No use, distribution or reproduction is permitted which does not comply with these terms.
*Correspondence: Jehangir H. Bhadha, amFuZ29AdWZsLmVkdQ==