- Department of Chemical Engineering, Taibah University, Madinah, Saudi Arabia
Greenhouse gas (GHG) emissions, and in particular CO2, are critical drivers of climate change, necessitating coordinated global efforts to mitigate their impact. This has led the Kingdom of Saudi Arabia (KSA) to launch several ambitious initiatives to curb its own emissions. However, there remain significant challenges when it comes to measuring outcomes, due to uncertainties in the existing methods and data. This current study therefore employs Production-Based (PB) and Consumption-Based (CB) emission accounting methods for KSA, emphasizing strategies to minimize the uncertainty inherent in these models. The results reveal that KSA is a net carbon exporter, with PB emissions (523 million tonnes CO2 equivalent (CO2e)) exceeding CB emissions (471 million tonnes CO2e). In addition, the validity of the applied methodology is supported by the literature. This research finds that private households form the largest contributors, along with sectors including electricity, land transport, water, and construction, as well as chemical, rubber, and plastic. The findings also confirm the potential for targeted policies to reduce emissions, i.e., the promotion of energy-efficient appliances, enhanced home insulation, public transportation, and recycling. Furthermore, this study highlights the importance of national investment in the accurate measurement of emissions and detailed energy statistics.
1 Introduction
Greenhouse gas (GHG) emissions exert a profound impact on the environment and pose a threat to human health (Calvin et al., 2023). CO2 emissions are responsible for two-thirds of GHG emissions, and currently demonstrate an unprecedented global atmospheric level, surpassing 420 parts per million (ppm) (Miao and Nduneseokwu, 2024; Clavier et al., 2024), representing a 51% increase compared to pre-industrial levels (Chen and Lee, 2024; Lugo-Morin, 2025). This therefore demands a coordinated global effort to reduce emissions and mitigate the risk of climate change. In November 2024, the UN Climate Change Conference (COP29) in Baku (Azerbaijan) agreed on a new finance target to minimize the impact of climate change on every country (This New Finance Goal is an Insurance Policy for Humanity, 2024; COP29, 2024). In addition, it implemented the Paris Climate Agreement to finalize the technical rules for international carbon markets, in which countries voluntarily pledged to curb their emissions in a collective effort to prevent the global average temperature from reaching 2°C above pre-industrial levels (UNFCCC, 2021). The Kingdom of Saudi Arabia (KSA) has therefore committed to combating climate change, launching a wide range of initiatives and programs, including to generate 50% of its electricity using renewable energy by 2030, and implement the circular carbon economy, which targets achievement of the 4 Rs: Reduce, Reuse, Recycle, and Remove CO2 emissions. KSA now aims to become a global leader in the production and export of clean hydrogen. Another key goal is to enhance energy efficiency across its industries, transportation, buildings, and household appliances. In addition, it is removing CO2 emissions by developing carbon capture and storage technology and planting 10 billion trees across its regions (Leadership Vision, 2024; Saudi and Middle East Green Initiatives, 2024; Saudi and Middle East Green Initiatives, 2022; Saudi Energy Efficiency Center, 2023).
The concept that “if you cannot measure it, you cannot improve it” (Albrecht and Brummett, 2021) highlights the fundamental role played by accurate measurement in driving meaningful change. This aligns with the idea that “you are what you measure”, suggesting that the metric adopted by an organization or country shapes both its decisions and actions (Hauser and Katz, 1998). In the context of GHG emissions, these principles emphasize the necessity of more precise accounting methods, i.e., vital to consider other emissions embedded in the supply chain when transitioning from coal to renewable energy sources. Furthermore, it is crucial to ensure accurate measurements for tracking progress, in order to confirm that reductions are real, rather than assumed. However, countries and organizations tend to rely on readily available tools and methods, which often use averaged data that may not reflect actual national or sector-specific emissions. This reliance on simplified calculations can lead to misinformed policies and ineffective mitigation strategies, so reinforcing the need for more precise GHG accounting. Additionally, imperfect methods can lead to ‘carbon leakage’, in which accounting from a certain angle causes a shift of emissions between regions. Carbon leakage is broadly defined in two different concepts: firstly, weak carbon leakage (also named the consumption-induced carbon leakage), and secondly, strong carbon leakage (i.e., policy-induced carbon leakage) (Peters, 2010; Steininger et al., 2014). The first can be indicated by comparing territorial- and consumption-based emissions, with weak carbon leakage implied when the latter are found to be higher. The second can be studied by using computable general equilibrium models. (Peters, 2010).
The challenge of addressing climate change can only be undertaken through a reduction in the main driver, i.e., carbon emissions. Countries generally announce their targets, which requires a reliable accounting method to measure the existing level of emissions, so assuring transparency and future replication. The Intergovernmental Panel on Climate Change (IPCC) has developed a GHG emissions inventory standard for countries that estimates GHGs solely on domestic production, i.e., only those emissions released within the spatial-political borders. The IPCC Production-Based (PB) (also named territorial-based) inventory standard has been ratified by many countries, and subsequently used to submit their calculated emissions to the United Nations Framework Convention on Climate Change (UNFCCC). An alternative method is the Consumption-Based (CB) inventory, in which emissions are calculated based on domestically consumed goods and services, regardless of where they were produced. Thus, PB and CB models differ when it comes to the treatment of the emissions of traded goods (exports and imports), including that PB models do not fully include emissions related to trade. In addition, it is notable that the World Resource Institute (WRI) supports the use of the CB accounting method for estimating the emissions of cities, with carbon emissions scopes defined as follows: Scope 1 includes emissions sourced within the region’s boundary; Scope 2 includes emissions from using electricity, steam, heating/cooling within the region’s boundary; and Scope 3 includes emissions caused by domestic consumption and sourced outside the region’s boundary. The definitions of these scopes indicate that the first two can be considered PB emissions, and all three can be seen as CB emissions.
In response to these challenges, this study addresses the following questions. Firstly, is it possible to develop an efficient tool that requires less effort while producing more reliable results, enabling countries to achieve ambitious sustainability goals? Secondly, how much discrepancy will the models show between the global average emissions and the emission estimations from KSA? Thirdly, is KSA a net emissions exporter or importer?
To answer these questions, the researcher used a comprehensive model called Environmentally Extended Input-Output Life Cycle Assessment (EIO-LCA) (Hendrickson et al., 2016) to trace the journey of products from raw materials to the end of their lifecycle (i.e., the whole economy). EIO-LCA is an integrated model that quantifies environmental impacts by combining Economic Input-Output (EIO) analysis with Life Cycle Assessment (LCA). The EIO framework represents a nation’s economy through a table capturing the interdependences between economic sectors (industries) and their relationship with both domestic and international consumers. By integrating LCA, EIO-LCA extends this analysis to assess the environmental impacts associated with products and services throughout their lifecycle, from raw material extraction to disposal, thus facilitating the calculation of GHG emissions from the perspective of both consumers and producers. Hence, this study also aims to identify the sectors and types of consumers responsible for the majority of emissions. Furthermore, EIO-LCA can quantify environmental impacts beyond GHG emissions, including water scarcity (Ridoutt et al., 2018), land use (Schmidt and De Rosa, 2018), biodiversity loss (Marques et al., 2017), air pollution (Roca and Serrano, 2007), and metal use (Nakamura et al., 2008).
Several previous studies have analyzed GHG emission accounting at national and sectoral levels, including Rahman et al. (2022), who applied the IPCC 2006 Guidelines to assess emissions from KSA’s industrial processes, and Hamieh et al. (Quantification and analysis of CO2, 2023), who examined sectoral CO2 footprints using conventional emissions estimation methods. Additionally, multiple reports from organizations listed in Table 1 have provided emissions estimates that cover only specific sectors of the economy, rather than a full-economy perspective.
While these studies have contributed valuable understanding of GHG accounting, they primarily focus on direct emissions or use methodologies that fail to comprehensively capture the impact of supply chain and trade-related emissions. This current study is thus unique in employing an enhanced EIO-LCA approach, while also improving its precision by calculating sector-specific emission intensities for key KSA industries, in order to overcome a common limitation of traditional EIO-LCA, which often lacks precision due to reliance on sector-wide averages. To the best of our knowledge, existing studies of regions like KSA do not inquire beyond average emission intensities, while this current research provides a more precise investigation into industries, technologies, and types of fuel. Furthermore, unlike studies focusing on individual industrial sectors, this research estimates total national emissions, accounting for both direct and indirect emissions while also considering those relate to trade. This comprehensive approach offers a more holistic and policy-relevant perspective that facilitates better-informed decisions for emissions mitigation and economic planning.
This study therefore lays the groundwork for the development of an online tool to enable producers and consumers to measure their emissions quickly and in a cost-effective manner, to make informed decisions concerning their management. The model thus serves as a valuable screening tool, allowing policymakers, businesses, and sustainability analysts to identify key processes (or sectors) responsible for emissions. By highlighting these high-impact areas, the study provides a foundation for more detailed investigations using methods such as process-based LCA (Hendrickson et al., 2016), so ultimately supporting more effective emissions reduction strategies.
The current objectives are to introduce the applied method of GHG estimations, explain the data employed, present the results and compare the GHG estimations with those in the literature.
2 Methods
This study applied and evaluated PB and CB approaches to calculating a region’s carbon footprint. Firstly, the mathematical basis of the economic IO (Leontief) (Leontief, 1986) is as in Equation 1:
where X is the total monetary required production vector whose elements represent the total output of each economic sector, I is the identity matrix, and A is the technology matrix that shows the required outputs of the other economic sectors to produce US$1 output of each sector. Thus, the multiplicative matrix inverse
The lower levels of time and expense associated with the EIO-LCA model often result in uncertainty. This study therefore prioritizes the identification and mitigation of these uncertainties, which stem from both the economic IO model and the environmental LCA component. One major limitation arises from the aggregation of industries into broad sectors, assuming homogeneity in production processes—an approach known as the ‘average production’ assumption. To address this, the emission intensity values (R) were calculated specifically for the most emission-intensive sectors in Saudi Arabia, based on the dominant industries within each sector (see Appendix S1). Furthermore, while IO models traditionally reflect production prices and exclude downstream life cycle stages (i.e., cradle to gate), this study adopted a database with a purchase price basis (cradle to consumer), following recommendations from a well-established textbook on LCA (Hendrickson et al., 2016). The analysis also improves on the common assumption that imported and domestic goods share identical production processes. Instead, the technology matrix A was differentiated for domestic and imported flows, and foreign emission intensities were incorporated based on global averages—an innovative aspect that reduces uncertainty related to trade. Additionally, the use of the IO model’s matrix inversion inherently accounts for circular economic flows, enhancing system completeness. Finally, to quantify the remaining uncertainty, particularly in sectoral CO2e intensities, a Monte Carlo simulation was conducted to assess its impact on total national emissions and test the robustness of the results.
2.1 The EIO-LCA models for production based (PB) and consumption based (CB) emissions accounting
The PB accounting method is officially recognized by an international environmental agreement (e.g., the Paris Agreement), requiring its use by countries when submitting updated National Determined Contribution (NDC) to UNFCCC (Tukker et al., 2020; Re-examining the realization of provincial, 2024). This method includes the emissions occurring within the borders of the country from the producers’ side (the supply side). This uses Equation 3 that considers the notable features noted above.
where R is the carbon intensity calculated for KSA,
The CB accounting method includes emissions caused by Saudi consumers, regardless of provider. The method exists as an opposition for the alleged unfairness of the PB method, which allows for rich countries to leak their emissions abroad. In this work, Equation 4 is used to calculate the CB emissions.
where the first part of the left side of the equation is the PB accounting equation, the second part of the left side of the equation accounts for the emissions coming from the imports using an average Rest of the World (ROW) carbon intensity and technology matrix. Finally, the third part of the left side of the equation represents the emissions caused by the KSA exports.
2.2 Data sources
The EIO-LCA model combines two types of data. The first is the economic IO data, which gives the monetary interdependencies between industries, either domestically or overseas. In addition, IO data classifies and records the consumers (final demand in this work) of these industries. The second type of data is environmental, which in this work is limited to GHG emissions. As noted above, the reference year for both the IO and environmental data is 2011, selected for the availability of rigorous data for both the IO and detailed GHG emissions data for KSA, in addition to the rich literature allowing the validation and comparison of the current results.
Firstly, the economic data, based on our models in Equations 3, 4, consist of the technology matrices for both KSA and foreign industries, and the final demand Ys for domestic, imports, and exports. These were obtained, or calculated, using the Global Trade Analysis Project (GTAP 9) database, which covers detailed information concerning domestic IO tables and their consumers for 57 economic sectors in 140 regions. Moreover, in addition to production prices, the GTAP database gives purchase prices benefitting this analysis, as recommended by Hendrickson et al. (2016), by accounting for the emissions starting from raw materials to consumers. In the GTAP nomenclature, the IO tables utilized the value flows at market prices for domestic and imported commodities, and at market prices for domestic and imported traded commodities purchased by private households, government, and capital (investment) used for the final demands.
When it came to the GHG data, this study used several resources to calculate or retrieve (in the absence of specific KSA data) the GHG intensity R values for the 57 domestic and imported GTAP sector. The researcher used the R values to serve as the emission factors, representing the amount of CO2-equivalent emitted per unit of economic output (kg CO2e/US$) for each sector. These values were calculated specifically for KSA, rather than adopted from IPCC defaults, by collecting total GHG emissions for each sector in the year 2011, then dividing them by the corresponding monetary output from the GTAP IO table for the same year (Hendrickson et al., 2016). The GHG emission data used to calculate R values were based on sources following the Tier 3 methodology recommended by IPCC (Zhu et al., 2023; Eggleston, 2006). Tier 3 provided the most detailed and accurate estimates, using technology-specific, country-specific, and fuel-specific emission factors. These used sources to estimate total GHG emissions for each sector from the Third National Communication and the First Biennial Update Report submitted by KSA to the UNFCCC (National Inventory, 2024; UNFCCC, 2024), which corresponds to 2010 and 2012, respectively. In addition, the average of the 2010 and 2012 emission values was calculated for each available sector in order to align with the 2011 reference year of the GTAP IO data used in this study, which allowed for a more representative and time-consistent estimation of sectoral carbon intensities. Another vital source was prepared by Hamieh et al. (Quantification and analysis of CO2, 2023) and follows the 2006 IPCC Guidelines, while OECD Inter-Country GHG footprint indicators were used for sectors not included in the previous three important resources (OECD, 2024). The OECD dataset provided both emission intensities (direct emissions per dollar of output) and emission multipliers (including indirect supply-chain emissions) (CO2 Emissions, 2022). It should be noted that only the emission intensity values were utilized, due to this study focusing on estimating direct emission factors.
For the purposes of transparency, Appendix S1 includes the complete list of calculated R values (i.e., sectoral emission factors), along with the associated GTAP sector names, data sources, and notes. The researcher recognizes that the assumptions and data transformations made in this study introduce inherent uncertainty, and that it is vital to quantify this in order to understand its impact on results. Therefore, to assess the uncertainty associated with sectoral emission intensities, a Monte Carlo simulation was conducted, for the PB accounting method, by applying a ±10% uniform variation to the R values (CO2e/US$) across all sectors. In each of 10,000 iterations, the R values were randomly perturbed and then multiplied by the corresponding final demand to recalculate total national emissions. This probabilistic approach follows established uncertainty analysis practices in EIO-LCA models (Lenzen et al., 2010; Wiedmann, 2009).
3 Results and discussion
The current research applied the two methods in relation to KSA for the year 2011, revealing that the PB emissions were 5.23 E+11 kg CO2e (523 million tonnes CO2e), higher than CB at 4.71 E+11 kg CO2e (471 million tonnes CO2e) (Figure 1a). This reveals that KSA was a net emissions exporter, as the emissions resulting from its exports were higher than those from its imports, as shown in Figures 1b, c. Moreover, these two graphs show that private households (regular consumers) were responsible for the majority of the emissions (41.76% in PB and 46.35% in CB). In both methods, the second highest emitter was investment (also called gross fixed capital formation (Aguiar et al., 2022)), while government proved the lowest.
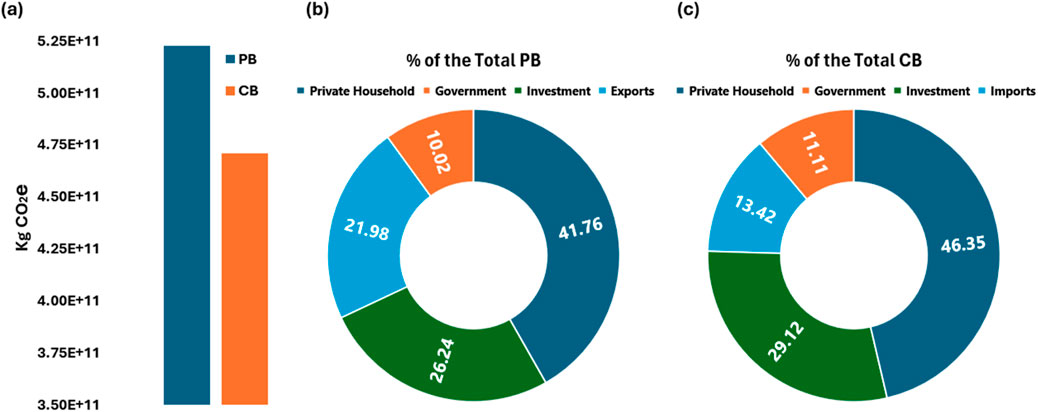
Figure 1. (a) Comparison between total PB and CB emissions; (b) composition of PB emissions by final demand components; (c) composition of CB emissions by final demand components.
The researcher undertook a comparison between the results of these models and the literature (Table 1). As discussed previously, all values presented in Table 1 correspond to 2011, which was selected as the reference year due to the availability of consistent economic and emissions data, as well as to facilitate comparison with existing literature. Two observations were expected in this comparison. Firstly, there were few CB estimations, as official environmental agencies require nations to submit their estimates using the PB model. Secondly, differences between estimations are expected for number of complex reasons, one being their boundaries, with some including only fossil fuel combustion emissions, while others include the cement industry and the whole economy. In addition, there remain variable data when it comes to fossil fuel statistics, as the variety of emission factors used between studies accounting for country-specific emission factors is available for some, while others employ average emission factors. This uncertainty can explain the small range of differences between estimations for developed countries with higher-quality data (e.g., 3%–5% for the United States (Gregg et al., 2008)), which increases for less developed countries (e.g., 15%–20% for China (Gregg et al., 2008)), and can exceed 50% for countries with a poorly maintained statistical infrastructure (Andres et al., 2012; Marland et al., 1999).
Liu et al. (2015) re-evaluated the carbon emissions from China using updated energy statistics and the measured carbon factor for the specific coal used in the country, identifying these as 14% lower in 2013 than other international emissions inventories. Table 1 shows the comparison between the results of this study and other prominent CO2 inventories, highlighting a number of interesting observations. Firstly, the results of this analysis are expected to be higher than other estimations, due to its coverage of the whole economy. Secondly, despite covering broader emissions, some of the compared estimates in this analysis show slightly lower numbers, as they only include narrower boundaries as in Global Carbon Budget and Emissions Database for Global Atmospheric Research (EDGAR). Thirdly, the emissions of the PB model are higher in this analysis than those of CB and Our World Data, apart from those from the China Economic Information Center (CEIC), where the CB model shows higher emissions than the PB, even though CEIC obtained their data from (CEIC, 2022b).
It is significant that the CEIC platform reports significantly lower estimates of emissions for KSA, despite referencing Our World Data as its source and applying similar system boundaries. This discrepancy highlights the risk of relying on secondary data sources without any transparency of methodology. The comparison presented in this study emphasizes the importance of countries developing and investing in their own emissions accounting systems, in order to ensure accuracy and credibility. Finally, considering the complexity of the comparison, the results of these analysis models were found to be logical, and the comparison could therefore be used to validate the methodology for both models. The per capita emissions were calculated according to the PB model (Table 1), and a similar population denominator was applied for all inventories. The population size (25.09 million) for that year was obtained from the official General Authority for Statistics (GASTAT) (GASTAT, 2024). The per capita CO2 emissions for KSA in relation to PB and CB were 20.84 tonnes and 18.77 tonnes, respectively, and the per capita CO2 emissions for the KSA for all inventories were higher than the global average of 4.88 tonnes that year (Statista, 2023).
As the primary distinction between PB and CB accounting lies in the treatment of trade, Figure 2 effectively illustrates how sectoral contributions vary across the two models (sector names and descriptions are provided in the second column of Appendix S1). PB emissions are notably higher in the petroleum products (p_c) and chemical, rubber, and plastic products (crp) sectors, reflecting KSA’s substantial export activity in these industries. Conversely, the CB model attributes greater emissions to the machinery and equipment (ome) sector, due to the country’s significant reliance on imports in this category. These contrasts highlight the critical role of trade flows in shaping national emissions profiles and emphasize the need to consider both production- and consumption-based perspectives in mitigation planning.
Figure 3 reveals how different economic components drive emissions across distinct sectors. Private household emissions are dominated by electricity and transport, highlighting energy-intensive domestic consumption. These results match with findings in the literature regarding the main sources of emissions in KSA (Quantification and analysis of CO2, 2023; Al-Sinan et al., 2023). The government category emissions were dominated by public administration, defense, education, and health, with government spending being the highest in these services (Alshahrani and Alsadiq, 2014; Haque and Khan, 2019). Investment-related emissions show substantial contributions from water, construction, and electricity—reflecting infrastructure development priorities. The dominance of the water sector can be explained by KSA being the largest global producer of desalinated water, with investment in this sector being actively continued (Technologies, 2024; Alobireed, 2021). Export emissions are heavily skewed toward petroleum and chemical products, aligning with Saudi Arabia’s export structure, while import emissions are most concentrated in machinery and manufactured goods, indicating reliance on foreign capital and high-tech equipment. Two notifications require further discussion. First, the oil sector’s exports represent a significant portion of KSA’s exports, but the GHG intensity of extracting and producing oil is significantly lower than burning (OECD, 2024), which explains the small contribution of the oil mining sector. Second, it might be surprising that electricity is one of the exporting sectors. However, the exports/imports data shows that in 2011 KSA exported electricity to neighboring countries like Qatar, Jordan, and others (not specified) (Our World in Data, 2025; Saudi Arabia Electricity supply, 2011; GWH, 2025). These variations underscore the importance of disaggregating emissions by economic function to better inform policy interventions and trade-adjusted mitigation strategies.
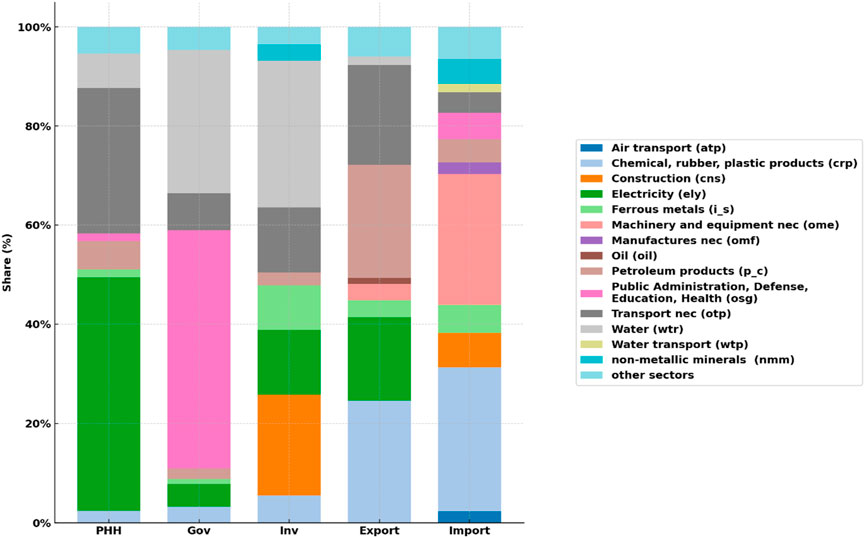
Figure 3. The sectoral distribution of greenhouse gas emissions associated with five economic components in KSA: private household consumption (PHH), government consumption (Gov), investment (Inv), exports, and imports. Sectoral shares are expressed as percentages of each component’s total emissions.
The Monte Carlo simulation for the PB accounting produced a distribution of total CO2e emissions centered around the baseline value of 5.23E+11 kg CO2e (Figure 4). The results revealed a 95% confidence interval of approximately ±20 billion kg CO2e, demonstrating that small variations in emission intensity assumptions can result in meaningful changes at the national scale. This range, which spans several percentage points of total emissions, underscores the potential for misestimating national targets or mitigation outcomes if uncertainty is not explicitly considered. These findings not only highlight the importance of incorporating uncertainty analysis in EIO-LCA studies, but also emphasize the need for higher-quality sectoral data to improve the robustness and credibility of national emission inventories.
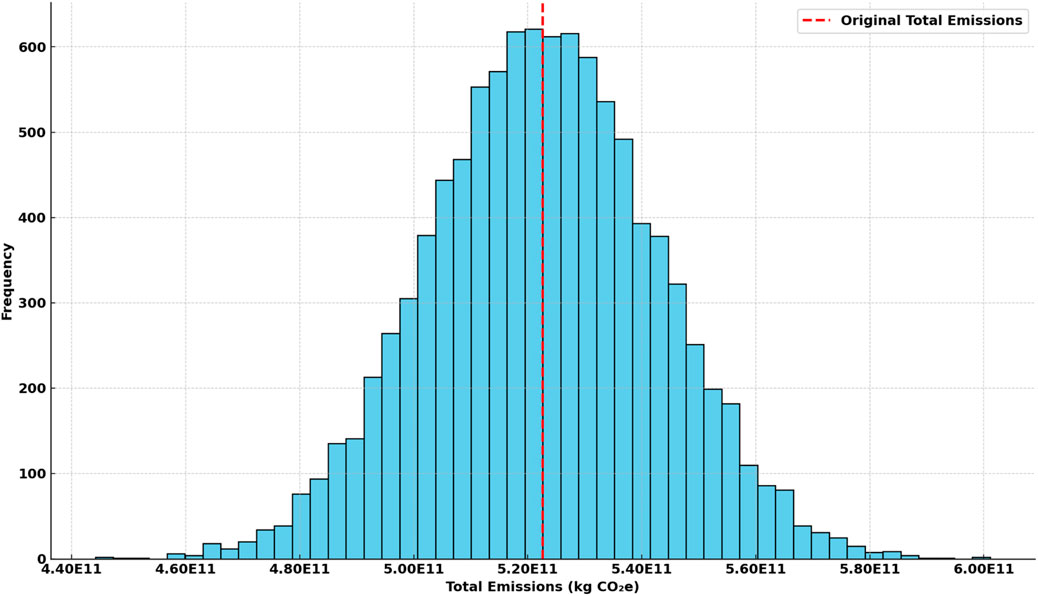
Figure 4. Monte Carlo simulation of the total PB CO2e emissions (±10% random variation in R values).
4 Conclusion and implications
This study demonstrates the value of using both production-based (PB) and consumption-based (CB) models to comprehensively assess greenhouse gas (GHG) emissions in KSA. Applying EIO-LCA with the GTAP database for 2011, the analysis found that Saudi Arabia is a net carbon exporter, with PB emissions exceeding CB emissions (523 vs 471 Mt CO2e). The fact that its PB emissions were higher than its CB emissions indicates that KSA does not experience significant consumption-induced (weak) carbon leakage. However, the researcher recommends the benefits of evaluating the potential presence of policy-induced (strong) carbon leakage by means of further investigation through detailed structural decomposition analysis or modeling of trade patterns and policy dynamics.
The researcher validated the methods used by comparing the results with literature as an indication of reasonable results. Moreover, the comparison reveals that some estimates, even with narrower boundaries, result in higher figures, which may stem from uncertainties in the emission factors employed or inaccuracies in energy statistics. These findings highlight the importance of encouraging countries to invest in accurately measuring their emission factors, as well as estimating emissions using fully accessible energy data. In addition, this suggests the need for the role of international environmental agencies and consultants to shift towards focusing on auditing and verification.
Sectoral analysis identified electricity, transport, water, construction, and energy-intensive industries as dominant emission sources. Private households were found to be key contributors, emphasizing the need for demand-side interventions such as energy-efficient appliances, improved building insulation, and sustainable transport policies. Similarly, reducing emissions in trade-intensive sectors will require aligning mitigation efforts with Saudi Arabia’s export and import profiles.
While EIO-LCA offers a rapid and cost-effective method for hotspot detection, its limitations—including data aggregation, linearity, and static IO structures—should be acknowledged. This study addressed uncertainty through careful database selection and sectoral disaggregation, yet highlights the need for updated and granular IO datasets, improved emission factor precision, and hybrid modeling. Future work should focus on refining sector-specific emission intensities and developing dynamic tools to support forward-looking policy scenarios. Ultimately, this research underscores the importance of transparent, trade-adjusted accounting for national emissions planning and highlights the potential of EIO-LCA as a practical screening tool to guide mitigation strategies in data-constrained contexts.
Data availability statement
The original contributions presented in the study are included in the article/Supplementary Material, further inquiries can be directed to the corresponding author.
Author contributions
KA: Conceptualization, Data curation, Formal Analysis, Funding acquisition, Investigation, Methodology, Project administration, Resources, Software, Supervision, Validation, Visualization, Writing – original draft, Writing – review and editing.
Funding
The author(s) declare that no financial support was received for the research and/or publication of this article.
Conflict of interest
The author declares that the research was conducted in the absence of any commercial or financial relationships that could be construed as a potential conflict of interest.
Generative AI statement
The author(s) declare that no Generative AI was used in the creation of this manuscript.
Publisher’s note
All claims expressed in this article are solely those of the authors and do not necessarily represent those of their affiliated organizations, or those of the publisher, the editors and the reviewers. Any product that may be evaluated in this article, or claim that may be made by its manufacturer, is not guaranteed or endorsed by the publisher.
Supplementary material
The Supplementary Material for this article can be found online at: https://www.frontiersin.org/articles/10.3389/fenvs.2025.1569523/full#supplementary-material
References
Aguiar, A., Chepeliev, M., Corong, E., and van der Mensbrugghe, D. (2022). The global trade analysis project (GTAP) data base: version 11. J. Glob. Econ. Analysis 7 (2), 1–37. doi:10.21642/jgea.070201af
Albrecht, E., and Brummett, C. M. (2021). If you cannot measure it, you cannot improve it. Anaesthesia 76 (10), 1304–1307. doi:10.1111/anae.15480
Alobireed, A. (2021). Global water desalination: a comparison between Saudi Arabia and the United States of America. Doctoral dissertation, University of Pittsburgh. Available online at: http://d-scholarship.pitt.edu/40982/.
Alshahrani, M. S. A., and Alsadiq, M. A. J. (2014). Economic growth and government spending in Saudi Arabia: an empirical investigation. Washington, DC: International Monetary Fund. Available online at: https://books.google.com/books?hl=en&lr=&id=fLEZEAAAQBAJ&oi=fnd&pg=PA4&dq=saudi+government+spending+on+education+health+defense&ots=no0Zysa48q&sig=Gav0_iX3n5jmJW5yHBPUn68cyp8.
Al-Sinan, M. A., Bubshait, A. A., and Alamri, F. (2023). Saudi Arabia’s journey toward net-zero emissions: progress and challenges. Energies 16 (2), 978. doi:10.3390/en16020978
Andres, R. J., Boden, T. A., Bréon, F. M., Ciais, P., Davis, S., Erickson, D., et al. (2012). A synthesis of carbon dioxide emissions from fossil-fuel combustion. Biogeosciences 9 (5), 1845–1871. doi:10.5194/bg-9-1845-2012
Calvin, K., Dasgupta, D., Krinner, G., Mukherji, A., Thorne, P. W., Trisos, C., et al. (2023). “IPCC, 2023: climate change 2023: synthesis report,” in Contribution of working groups I, II and III to the sixth assessment report of the intergovernmental Panel on climate change core writing team. IPCC. Editors H. Lee, and J. Romero (Geneva, Switzerland: First. Intergovernmental Panel on Climate Change IPCC). Available online at: https://www.ipcc.ch/report/ar6/syr/.
CEIC (2022a). Saudi Arabia consumption based emissions of CO2 | economic indicators | CEIC. Available online at: https://www.ceicdata.com/en/saudi-arabia/environmental-co2-and-greenhouse-gas-emissions-annual/consumption-based-emissions-of-co2.
CEIC (2022b). Saudi Arabia | environmental: CO2 and greenhouse gas emissions: annual | CEIC. Available online at: https://www.ceicdata.com/en/saudi-arabia/environmental-co2-and-greenhouse-gas-emissions-annual.
Chen, W. S., and Lee, C. H. (2024). Advancements in carbon capture technologies. CRC Press. Available online at: https://books.google.com/books?hl=en&lr=&id=Gt0SEQAAQBAJ&oi=fnd&pg=PA33&dq=20+parts+per+million+(ppm).+This+significant+rise+marks+a+51+%25+increase+compared+to+pre-industrial+levels.&ots=eUsLHLc3AF&sig=IafLBBE7oW1hs5pA2oFoN1wMovs.
Clavier, C., Meftah, M., Sarkissian, A., Romand, F., Hembise Fanton d’Andon, O., Mangin, A., et al. (2024). Assessing greenhouse gas monitoring capabilities using solatmos end-to-end simulator: application to the uvsq-sat ng mission. Remote Sens. 16 (8), 1442. doi:10.3390/rs16081442
CO2 Emissions (2022). Note on CO2 emissions, intensities, and multipliers - june 2022. Available online at: https://climatedata.imf.org/documents/dde2fa06078943a8a0c098fb7cc70e10.
CO2 Emissions from fuel Combustion (2024). World statistics on CO2 updated | enerdata. Available online at: https://yearbook.enerdata.net/co2/emissions-co2-data-from-fuel-combustion.html.
COP29 (2024). COP29 UN climate conference agrees to triple finance to developing countries. Prot. Lives Livelihoods. Available online at: https://unfccc.int/news/cop29-un-climate-conference-agrees-to-triple-finance-to-developing-countries-protecting-lives-and.
Crippa, M., Guizzardi, D., Banja, M., Solazzo, E., Muntean, M., Schaaf, E., et al. (2022). CO2 emissions of all world countries: JRC/IEA/PBL 2022 report. Luxembourg: Publications Office of the European Union. Available online at: https://data.europa.eu/doi/10.2760/07904.
Eggleston, H. (2006). Estimation of emissions from CO2 capture and storage: the 2006 IPCC Guidelines for national greenhouse gas inventories.
Friedlingstein, P., O’Sullivan, M., Jones, M. W., Andrew, R. M., Gregor, L., Hauck, J., et al. (2022). Global carbon Budget 2022. Earth Syst. Sci. Data 14 (11), 4811–4900. doi:10.5194/essd-14-4811-2022
GASTAT (2024). The general authority for statistics (GASTAT). Available online at: https://www.stats.gov.sa.
Gregg, J. S., Andres, R. J., and Marland, G. (2008). China: emissions pattern of the world leader in CO2 emissions from fossil fuel consumption and cement production. Geophys. Res. Lett. 35 (8). doi:10.1029/2007GL032887
GTAP (2025). Data bases: GTAP 9 data base sectors. Available online at: https://www.gtap.agecon.purdue.edu/databases/v9/v9_sectors.asp.
GWH (2025). The power import in Saudi Arabia (2017 - 2020, GWh). Available online at: https://www.linkedin.com/company/globaldataplc/.
Haque, M. I., and Khan, M. R. (2019). Role of oil production and government expenditure in improving human development index: evidence from Saudi Arabia. Int. J. Energy Econ. Policy 9 (2), 251–256.
Hauser, J., and Katz, G. (1998). Metrics: you are what you measure. Eur. Manag. J. 16 (5), 517–528. doi:10.1016/s0263-2373(98)00029-2
Hendrickson, C. T., Matthews, H. S., and Matthews, D. (2016). Life cycle assessment: quantitative approaches for decisions that matter.
IEA (2024). Saudi Arabia - countries and regions. Available online at: https://www.iea.org/countries/saudi-arabia/emissions.
Leadership Vision (2024). Leadership vision. Available online at: https://www.pif.gov.sa/en/private-sector-hub/leadership-vision/.
Lenzen, M., Wood, R., and Wiedmann, T. (2010). Uncertainty analysis for multi-region input-output models - a case study of the UK’S carbon footprint. Econ. Syst. Res. 22 (1), 43–63. doi:10.1080/09535311003661226
Leontief, W. (1986). Input-output economics. Oxford University Press. Available online at: https://books.google.com/books?hl=en&lr=&id=HMnQCwAAQBAJ&oi=fnd&pg=PR11&dq=Leontief&ots=LJt7LHTlol&sig=zRbdKzNet4Tp7eFujVCVjxBR1tc.
Liu, Z., Guan, D., Wei, W., Davis, S. J., Ciais, P., Bai, J., et al. (2015). Reduced carbon emission estimates from fossil fuel combustion and cement production in China. Nature 524 (7565), 335–338. doi:10.1038/nature14677
Lugo-Morin, D. R. (2025). Anthropocene futures: regeneration as a decarbonization strategy. Sustain. Soc. Dev. 3 (1), 3153. doi:10.54517/ssd3153
Marland, G., Brenkert, A., and Olivier, J. (1999). CO2 from fossil fuel burning: a comparison of ORNL and EDGAR estimates of national emissions. Environ. Sci. and Policy 2 (3), 265–273. doi:10.1016/s1462-9011(99)00018-0
Marques, A., Verones, F., Kok, M. T., Huijbregts, M. A., and Pereira, H. M. (2017). How to quantify biodiversity footprints of consumption? A review of multi-regional input–output analysis and life cycle assessment. Curr. Opin. Environ. Sustain. 29, 75–81. doi:10.1016/j.cosust.2018.01.005
Miao, Q., and Nduneseokwu, C. (2024). “Global environmental leadership: addressing the triple planetary crisis,” in Environmental leadership in a VUCA era (Singapore: Springer Nature Singapore), 37–119. Available online at: https://link.springer.com/10.1007/978-981-96-0324-4_2.
Nakamura, S., Murakami, S., Nakajima, K., and Nagasaka, T. (2008). Hybrid Input−Output approach to metal production and its application to the introduction of lead-free solders. Environ. Sci. Technol. 42 (10), 3843–3848. doi:10.1021/es702647b
National Inventory (2024). National inventory of anthropogenic emissions by sources and removals by sinks of greenhouse gases. Available online at: https://datasource.kapsarc.org/explore/dataset/gas-emission-by-major-source-category/.
OECD (2024). Greenhouse gas footprint indicators. Available online at: https://www.oecd.org/en/data/datasets/greenhouse-gas-footprint-indicators.html.
Our World in Data (2025). Net electricity imports. Available online at: https://ourworldindata.org/grapher/net-electricity-imports.
Peters, G. P. (2010). Policy update: managing carbon leakage. Carbon Manag. 1 (1), 35–37. doi:10.4155/cmt.10.1
Quantification and analysis of CO2 (2023). Quantification and analysis of CO2 footprint from industrial facilities in Saudi Arabia - ScienceDirect. Available online at: https://www.sciencedirect.com/science/article/pii/S2590174522001222.
Rahman, M. M., Rahman, M. S., Chowdhury, S. R., Elhaj, A., Razzak, S. A., Abu, S. S., et al. (2022). Greenhouse gas emissions in the industrial processes and product use sector of Saudi arabia—an emerging challenge. Sustainability 14 (12), 7388. doi:10.3390/su14127388
Re-examining the realization of provincial (2024). “Re-examining the realization of provincial carbon dioxide emission intensity reduction targets,” in China from a consumption-based accounting - ScienceDirect. Available online at: https://www.sciencedirect.com/science/article/abs/pii/S095965261933358X.
Ridoutt, B. G., Hadjikakou, M., Nolan, M., and Bryan, B. A. (2018). From water-use to water-scarcity footprinting in environmentally extended input–output analysis. Environ. Sci. Technol. 52 (12), 6761–6770. doi:10.1021/acs.est.8b00416
Ritchie, H., and Roser, M. (2022). Our world in data. Available online at: https://ourworldindata.org/co2/country/saudi-arabia.
Roca, J., and Serrano, M. (2007). Income growth and atmospheric pollution in Spain: an input–output approach. Ecol. Econ. 63 (1), 230–242. doi:10.1016/j.ecolecon.2006.11.012
Saudi Arabia Electricity supply (2011). Saudi Arabia Electricity supply, production and calibrating exports by country | 2011 | Data. Available online at: https://wits.worldbank.org/trade/comtrade/en/country/SAU/year/2011/tradeflow/Exports/partner/ALL/product/902830.
Saudi Energy Efficiency Center (2023). Saudi energy efficiency center Available online at: https://www.seec.gov.sa/en
Saudi and Middle East Green Initiatives (2022). Greening Saudi | Saudi green initiative target. Available online at: https://10.50.50.79/about-sgi/sgi-targets/greening-saudi.
Saudi and Middle East Green Initiatives (2024). Reducing carbon emissions | Saudi green initiative target. Available online at: https://10.50.50.79/about-sgi/sgi-targets/reduce-carbon-emissions.
Schmidt, J., and De Rosa, M. (2018). “Enhancing land use change modelling with IO data,” in Extended abstract presented at the SETAC europe 28th annual meeting, rome, 13–17. Available online at: https://lca-net.com/files/SETAC_Rome_Schmidt_DeRosa_Extended-abstract_20180515.pdf.
Statista (2023). Global per capita CO2 emissions 1960-2023. Available online at: https://www.statista.com/statistics/268753/co2-emissions-per-capita-worldwide-since-1990/.
Steininger, K., Lininger, C., Droege, S., Roser, D., Tomlinson, L., and Meyer, L. (2014). Justice and cost effectiveness of consumption-based versus production-based approaches in the case of unilateral climate policies. Glob. Environ. Change 24, 75–87. doi:10.1016/j.gloenvcha.2013.10.005
Technologies, A. (2024). SWCC chronicle - SWCC. Available online at: https://www.swcc.gov.sa/en/WhoAreWe/∼/en/WhoAreWe/SWCCChronicle.
This New Finance Goal is an Insurance Policy for Humanity (2024). “This new finance goal is an insurance policy for humanity”: simon stiell at close of COP29 | UNFCCC Available online at: https://unfccc.int/news/this-new-finance-goal-is-an-insurance-policy-for-humanity-simon-stiell-at-close-of-cop29?_gl=1%2A3zbt2u%2A_gcl_aw%2AR0NMLjE3MjkyNDgwMzcuQ2p3S0NBandqc2k0QmhCNUVpd0FGQUwwWUEyVncxT2JsbDhybVluaFRsQzhCMG9TT3NKa0ZBb2hjWXZhMjBCSkpfalVfbWxTT3V3V0FCb0M3QzhRQXZEX0J3RQ.%2A_ga%2AMjEzNTM4MTI0LjE2ODU2MzM4ODk.%2A_ga_7ZZWT14N79%2AMTczMjQwNjA5OC40ODUuMS4xNzMyNDEwNTcwLjAuMC4w
Tukker, A., Pollitt, H., and Henkemans, M. (2020). Consumption-based carbon accounting: sense and sensibility. Clim. Policy 20 (Suppl. 1), S1–S13. doi:10.1080/14693062.2020.1728208
UNFCCC (2021). The Paris Agreement | UNFCCC. Available online at: https://unfccc.int/process-and-meetings/the-paris-agreement.
UNFCCC (2024). Documents | UNFCCC. Available online at: https://unfccc.int/documents?f%5B0%5D=country%3A1236.
Wiedmann, T. (2009). A review of recent multi-region input–output models used for consumption-based emission and resource accounting. Ecol. Econ. 69 (2), 211–222. doi:10.1016/j.ecolecon.2009.08.026
Zhu, S., Cai, B., Fang, S., Zhu, J., and Gao, Q. (2023). “The development and influence of IPCC Guidelines for national greenhouse gas inventories,” in Annual report on actions to address climate change (2019): climate risk prevention. Editors G. Zhuang, Q. Chao, G. Hu, and J. Pan (Singapore: Springer Nature), 233–246. doi:10.1007/978-981-19-7738-1_16
Keywords: production-based (PB) emissions, consumption-based (CB) emissions, sustainability, EIO-LCA, GHG emissions, Saudi Arabia
Citation: Almutairi K (2025) Assessing production-based and consumption-based greenhouse gas emissions in the Kingdom of Saudi Arabia: insights for mitigation and policy. Front. Environ. Sci. 13:1569523. doi: 10.3389/fenvs.2025.1569523
Received: 10 February 2025; Accepted: 21 April 2025;
Published: 29 April 2025.
Edited by:
Isidro A. Pérez, University of Valladolid, SpainReviewed by:
Muhammad Farhan Bashir, Chengdu University of Technology, ChinaMithun Kumar Biswas, The University of Melbourne, Australia
Copyright © 2025 Almutairi. This is an open-access article distributed under the terms of the Creative Commons Attribution License (CC BY). The use, distribution or reproduction in other forums is permitted, provided the original author(s) and the copyright owner(s) are credited and that the original publication in this journal is cited, in accordance with accepted academic practice. No use, distribution or reproduction is permitted which does not comply with these terms.
*Correspondence: Kamel Almutairi, YWxtdXRhaXJpa2FtZWxAZ21haWwuY29t, a211dGFpcmlAdGFpYmFodS5lZHUuc2E=