- 1Institute of Information of Agricultural Science and Technology, Shanghai Academy of Agricultural Sciences, Shanghai, China
- 2Nakatsugawa Works, Mitsubishi Electric Corporation, Gifu, Japan
- 3Kirin Central Research Institute, Kirin Holdings Company, Ltd., Fujisawa-shi, Kanagawa, Japan
China’s central government introduced the low-carbon agricultural pilot (LCAP) policy to curb carbon emissions and foster sustainable growth. While most research has centered on macro-level impacts (provinces and cities), this study uniquely examines the LCAP policy’s influence on agricultural companies’ environmental expenditures and farmers’ net income. Given the LCAP’s weak-constraining nature, its effectiveness at the company and farmer level remains intriguing. We apply the Propensity Score Matching–Difference in Differences (PSM-DID) method, which excels in mitigating sample selection bias, focusing on the 2011–2020 LCAP phase involving 34 listed companies in China’s agriculture and food sectors. Further, we analyzed data from 410 rice farmers in Hubei, Zhejiang, and Shanghai, assessing the LCAP’s effects on their income. Results reveal that companies in LCAP cities decrease their environmental spending by 0.91 points (1% significance). On the farming front, non-participation leads to a potential 28-thousand-yuan income reduction. Organic fertilizers, compost, and recycling cultivation waste prove impactful, promoting income and ecological sustainability. However, the effectiveness of high-cost, long-payback energy-saving machinery subsidies appears limited under current implementation conditions. These findings suggest a potential misalignment between policy design and implementation outcomes, highlighting the challenges associated with non-mandatory environmental policies such as the LCAP in achieving their intended objectives.
1 Introduction and literature review
The increasing importance of addressing GHG emissions has become more evident in light of changing climate patterns. Recent data indicates that in 2015, the global food system contributed approximately 18 Gt CO2-equivalent, representing 34% of total greenhouse gas emissions (Caliendo and Kopeinig, 2008). In the Chinese context, achieving a balance between advancing eco-friendly agricultural practices and addressing farmers’ economic considerations presents a significant challenge. The transition towards sustainable agriculture in China has been gradual, influenced by the substantial costs associated with implementing green farming methods. Research suggests that financial limitations, stemming from variable income streams and restricted capital access, present substantial barriers to the adoption of environmentally sustainable practices among farmers in developing nations (Giné and Yang, 2009; Karlan et al., 2014).
China’s agricultural development over recent decades has played a pivotal role in meeting the food requirements of its growing population. However, this agricultural expansion has been accompanied by notable environmental consequences, particularly in terms of GHG emissions. Primary contributors to this environmental impact include rice cultivation practices, livestock production systems, and the application of synthetic fertilizers (Liu et al., 2013; Yan et al., 2005; Huang et al., 2012). Specific areas of concern include methane emissions from rice paddies and the environmental implications of the expanding livestock industry.
The development of an integrated approach to mitigate GHG emissions across agricultural, forestry, and fisheries sectors has been identified as essential, as illustrated in Figure 1 (Ministry of Ecology and Environment and The People’s Republic of China, 2022). In response to these challenges, the Chinese government implemented the Low-Carbon Agricultural Policy (LCAP) in 2015. While policy formulation occurs at the national level, the execution and implementation primarily rest with local government entities. The Ministry of Agriculture’s focus on energy efficiency and emission reduction initiatives highlights the significance of adopting low-carbon agricultural technologies.
Contemporary sustainable farming practices, including organic waste management, agricultural byproduct utilization, and advanced irrigation technologies, are increasingly being adopted (Liang et al., 2022). However, the effectiveness of these national policies at the local level may be influenced by fiscal decentralization mechanisms, potentially affecting the implementation outcomes of the LCAP initiative (Zhang et al., 2011). Additionally, the shift towards sustainable agricultural methods may result in increased production expenses and labor requirements. Research indicates that without adequate policy support mechanisms, maintaining stable income growth for agricultural producers may present challenges.
The adoption of sustainable farming practices faces several challenges, primarily related to increased labor demands and associated costs. Policy interventions play a crucial role in addressing these barriers. The Ministry of Agriculture (MOA) has implemented various initiatives to support the adoption of low-carbon agricultural techniques, including financial incentives and skill development programs. Initially launched in Zhejiang Province in 2015, these initiatives were subsequently extended to other regions by 2020, as illustrated in Figure 2.
Research related to farming GHG emissions and associated fiscal policies can be categorized into:
Agricultural GHG Emissions: GHG emissions in China’s agricultural sector primarily arise from rice cultivation, livestock activities, and synthetic fertilizer use (Liu et al., 2013; Yan et al., 2005; Huang et al., 2022). Following the introduction of the LCAP policy, there has been an increased focus on low-carbon agricultural practices.
Environmental Expenditure by Agricultural Companies: Existing research predominantly examines the LCAP policy’s macro effects, with limited exploration of its micro-level impact, particularly its influence on the environmental expenditure of agricultural firms (Du et al., 2023; Li C. et al., 2023; Su et al., 2023).
Impact of Subsidies: Subsidies, as a key fiscal tool, significantly influence the financial behaviors of agricultural firms. These financial supports can reduce the costs associated with sustainable practices, but they may also lead to unintended distortions in investments, potentially resulting in increased pollution (Wang et al., 2020). Studies show that farmers with access to relevant information and subsidies are more likely to adopt low-carbon farming practices. These practices can enhance crop value and alter the agricultural structure, thereby influencing farmers’ production behaviors (Clarke et al., 2012; Breustedt and Glauben, 2007; Duan and Xu, 2022).
Most studies on the LCAP policy adopt a macro perspective, focusing on carbon reduction, efficiency, and productivity (Du et al., 2023; Su et al., 2023; Li L. et al., 2023). However, micro-level research, particularly on the environmental expenses of agricultural companies and the direct effects of these policies on farmers’ incomes, remains limited.
This study aims to address these gaps by utilizing data from Chinese publicly traded agricultural firms between 2016 and 2022. The primary objective is to evaluate the LCAP policy’s impact on environmental expenses using the PSM-DID method. Additionally, data from 410 valid survey responses will be used to assess the policy’s influence on household income in the Yangtze River Basin.
2 Background
2.1 Policy background
Over the past four decades since China’s reform and opening up, its agricultural sector has undergone significant growth, highlighting the ongoing importance of agricultural development. However, this growth has led to increased energy consumption and a corresponding rise in GHG emissions. At the same time, the environmental consequences have been substantial, presenting challenges such as land pollution, soil degradation, and the effects of chemical fertilizer use (Shi et al., 2022).
The Middle and Lower Reaches of the Yangtze River Basin, encompassing provinces such as Hubei, Hunan, Zhejiang, and Jiangsu, constitute a major grain-producing region, playing a critical role in China’s food security. This region is also the largest source of the country’s agricultural GHG emissions. Environmental issues, including intensive use of agricultural resources, declining soil quality, and water pollution, have become increasingly significant in this area, highlighting the need for targeted mitigation efforts (Yang et al., 2020).
In response, the Ministry of Agriculture, in 2013, introduced the blueprint of an agricultural GHG reduction strategy, subsequently designating Zhejiang as the first low-carbon agriculture pilot province in 2015. This initiative expanded post-2020 to cover the entire basin. Zhejiang, as the initial pilot province, implemented measures such as reducing chemical fertilizer use, improving the efficiency of chemical inputs, promoting low-emission farming machinery, and optimizing livestock and poultry waste management. These measures were designed to reduce agricultural GHG emissions and promote a low-carbon, sustainable agricultural model.
2.2 Institutional background
Post-1978, marking the beginning of China’s economic liberalization and reform period, there has been a significant transfer of decision-making powers between the central and local governments (Tang et al., 2018). The key fiscal reform, known as the tax-sharing system, was introduced in 1993, defining three central elements: the separation of administrative and financial responsibilities, the division of revenue streams, and the creation of a fiscal transfer payment mechanism between the two levels of government. This reform provided local governments with increased autonomy in economic governance, enabling them to plan urban development, manage local public services, and develop policies to support regional business sectors.
Nevertheless, these powers are counterbalanced by increased financial accountability. With the central government receiving the majority of tax revenue, local governments consistently face fiscal constraints, requiring a focus on economic growth to meet local expenditure needs—a challenge that is often difficult to address (Tang et al., 2018). Within such a fiscal context, local authorities may demonstrate limited enthusiasm for the implementation of environmental regulations, potentially affecting the effectiveness of such policies at the local level.
2.3 LCAP policy and its knowledge gap
Low-carbon agricultural policies have become significant in guiding sustainable economic development in developed nations. The growing academic interest in LCAP policy primarily focuses on its effectiveness (Ganda, 2023; Laborde et al., 2021; Mittenzwei et al., 2017). While carbon footprint reduction remains a key indicator of policy success, definitive conclusions have yet to be reached. Notably, the majority of research is concentrated on developed countries, with limited attention given to developing nations. Additionally, the difficulty of accurately measuring carbon emissions, due to methodological limitations, has led to a shift from macroscopic to microscopic analytical paradigms.
Macro-level investigations primarily focus on the procedural and outcome dynamics of LCAP across different regions (Liang et al., 2021; Zhang et al., 2023). In contrast, micro-level studies, particularly empirical analyses, remain relatively limited. Macro perspectives may not fully capture the differentiated impacts on smaller entities, often providing a generalized overview. As companies and farmers constitute the foundation of the agricultural sector, it is essential to examine the LCAP’s effects on agribusiness operations and farmer income. An important aspect of micro-level analysis involves resident behaviour and lifestyle patterns. For example, factors influencing urban bicycle usage in Baoding were assessed (Li et al., 2017), emphasizing the role of variables such as gender, age, income, transportation accessibility, and awareness of policies like LCCP (Low-carbon City Policy).
LCCP represents a component of China’s broader strategy to reduce emissions within industrial sectors and urban areas. However, some studies suggest potential challenges associated with the policy’s implementation. Scholars (Cheng et al., 2019) analyzed the initial phase of LCCP at the provincial level, identifying gaps in support from other policy frameworks and institutional mechanisms. Additionally, a misalignment between environmental policy design and its execution has been noted (Lo, 2014; Ran, 2013; Chen et al., 2021). Factors contributing to this issue include limited involvement from local governments and potential coordination between these entities and corporate enterprises.
The implementation of policy, particularly at the grassroots level, depends significantly on local governments, whose priorities can influence the policy’s effectiveness. Lo (Lo, 2014) highlights the case of low-carbon urban innovations in Changchun, where implementation was less effective, primarily due to local governments prioritizing economic growth over environmental policy compliance. At the same time, the alignment of interests between local authorities and industrial firms poses a challenge to the enforcement of environmental regulations.
Liang and Gao (Liang and Gao, 2014) provide empirical evidence linking the tenure of local officials with the robustness of legal frameworks, suggesting that longer leadership tenures may foster closer relationships between government officials and polluting enterprises, potentially reducing regulatory oversight. In summary, the limited achievement of LCCP’s goals can be attributed to China’s unique institutional context and the prevailing focus on local economic development.
Furthermore, it is important to note that similar initiatives, such as the LCCP, have been associated with unintended outcomes in multiple studies. Therefore, investigating the effects of the LCAP, as a policy similar to the LCCP, on the financial performance of agricultural firms and farmers’ income is a key objective of this research. Our analysis highlights a gap in the literature: the lack of studies examining the LCAP’s impact on agricultural firms’ environmental expenditures and farmers’ net income. This paper aims to address this gap by providing empirical analyses that could contribute to the LCAP discourse and clarify its effects at the grassroots level—both for companies and farmers. Given the LCAP’s nature as a weakly enforced central directive, its effectiveness at these micro-levels merits further investigation.
2.4 Theoretical mechanism and research hypotheses
2.4.1 Multi-level governance and enforcement
From a multi-level governance perspective, central government policy directives are delegated to local authorities with varying degrees of financial autonomy and administrative capacity (Tang et al., 2018; Lo, 2014). Although the LCAP seeks to reduce agricultural GHG emissions via techniques such as chemical input reduction or recycling, it is non-mandatory in nature, meaning its success largely depends on local enforcement enthusiasm. If a local government prioritizes economic growth or lacks adequate fiscal resources, environmental programs may receive lower enforcement or be implemented superficially (Lo, 2014; Ran, 2013). This governance dynamic implies that, under a weak enforcement context, policy targets may be attained only formally—on paper—while actual environmental investments at the firm level remain modest.
2.4.2 Corporate environmental expenditures under weak constraints
For agribusinesses, engaging in environmentally friendly processes often entails upfront costs (e.g., purchasing low-carbon machinery or investing in new waste management systems). In a setting where LCAP enforcement is lenient, firms may opt to reduce or strategically reallocate their environmental budgets to maintain short-term competitiveness. By contrast, in jurisdictions that rigorously promote low-carbon farming (e.g., through stricter oversight, aligned incentives, or penalty mechanisms), firms might be compelled or encouraged to sustain or even increase their environmental spending. The nature of local government support thus becomes a deciding factor in how agribusinesses balance the tension between short-term cost savings and long-term environmental responsibilities (Shi et al., 2022; Yang et al., 2020; Cheng et al., 2019).
2.4.3 Farmer household participation and income
At the micro level, households’ willingness to embrace low-carbon practices (e.g., composting, waste recycling, or reduced chemical fertilizer usage) depends on financial feasibility, awareness, and policy-induced incentives (Ganda, 2023; Laborde et al., 2021; Mittenzwei et al., 2017). When local authorities effectively channel LCAP subsidies—particularly for organic fertilization or new technology adoption—farmers stand to reduce operating costs and potentially increase net income. However, if such incentives are scarce, or if the administrative process is burdensome, farmers may be reluctant to deviate from traditional practices. This aligns with evidence suggesting that weakly structured programs can yield uneven benefits, wherein only certain regions or demographics capitalize on policy provisions while others fall behind (Liang et al., 2021; Zhang et al., 2023; Li et al., 2017). The initial capital outlay (e.g., energy-saving machinery) and the speed of returns thus become critical considerations for farmers deciding whether to engage in LCAP initiatives (Lo, 2014; Ran, 2013; Chen et al., 2021).
2.4.4 Research hypotheses
In light of the above theoretical reasoning, two core hypotheses guide our empirical investigation.
H1 (Corporate Sphere): In localities with limited enforcement of LCAP directives, agribusinesses will exhibit lower or reduced environmental spending, reflecting the diminished regulatory pressure and the potential reallocation of financial resources toward other priorities.
H2 (Farmer Tier): Households that actively participate in LCAP-driven programs—either through direct subsidies, training, or technology adoption—are more likely to enhance their net incomes compared to non-participating households, given the policy’s focus on cost-sharing and improved resource efficiency.
These hypotheses rest on the premise that a non-mandatory environmental policy can yield diverse outcomes depending on how effectively local governments implement it and how motivated firms and households are to comply. By specifying these two pathways, we accommodate the possibility of counterintuitive corporate behavior (such as reduced environmental expenditures) alongside positive income effects for farmers. The following chapters will detail the methodological design and empirical strategies used to test these hypotheses, offering insight into the actual impact of LCAP at both the corporate and grassroots levels.
3 Methods and data
3.1 Company level analysis
3.1.1 Propensity score matching method (PSM)
Given the non-random nature of LCAP policy implementation (with some cities voluntarily declaring themselves LCAP cities), there’s a potential for selection bias in the initial sample. To counteract this, we use the Propensity Score Matching (PSM) method. Rooted in the counterfactual inference model, PSM manages non-random datasets by statistically determining the propensity score for each observation based on covariates, subsequently matching these scores based on their proximity.
Considering 2015 as the benchmark year for policy implementation, the treatment variable is dichotomous:
3.1.2 Difference-in-differences method (DID)
The DID method is pivotal for evaluating the impact of exogenous shocks and discerning causal relations between economic entities. We utilize a quasi-naturalistic trial with data sourced from Chinese-listed companies between 2011 and 2020 to analyze the LCAP policy’s influence on their environmental expenditures. Here, the control group encompasses companies outside LCAP jurisdictions. By juxtaposing changes in environmental expenditures between LCAP and non-LCAP cities, we estimate the policy’s average treatment effect (ATT). The foundational model is:
Where
3.1.3 Data source and study area
The dependent variable,
Post-reform, China’s expanding economy has observed the agriculture and food sectors contributing notably to its carbon emissions. This is attributed to the substantial fossil fuel utilization associated with agricultural production and the transportation involved in processing agricultural products, leading to significant environmental impacts.
Data for this study is primarily sourced from the China Stock Market and Accounting Research (CSMAR) Database, supplemented with financial statements from the listed companies. The sample includes 34 companies from the agriculture and food sectors. The classification of a company under an LCAP city was determined by the location of its main office. A descriptive statistical breakdown of the study variables is provided in Table 2.
Although the sample includes 34 companies, this selection reflects a meaningful cross-section of companies in the agriculture and food sectors from both LCAP and non-LCAP cities in the middle and lower Yangtze River Basin. The companies were selected based on industry classification and data availability from authoritative sources, and they include state-owned and private entities with diverse financial and governance profiles. The 10-year longitudinal span enhances the analytical depth and helps mitigate concerns about temporal limitations. While the sample size is relatively modest, the combination of a well-targeted industry focus, geographic concentration, and robust empirical methods (DID, PSM, and PSM-DID) ensures that the findings have meaningful internal validity and policy relevance.
3.2 Farmer level analysis
3.2.1 Conceptual framework
Participation in support policies can help smallholder farmers adopt advanced agricultural methods, thereby reducing greenhouse gas emissions. A farmer’s decision to participate in these policies is framed within a utility choice problem (McFadden, 1974). Essentially, a farmer will participate if the anticipated net utility from doing so surpasses non-participation. This utility,
Here,
This study pivots on net farm income as the dependent variable. Four key support policies underpin the explanatory variables: organic fertilizer or compost subsidy (SP1); cultivation waste recycling subsidy (SP2); energy-saving agricultural machinery subsidy (SP3); and eco-friendly farming support (SP4). A farmer’s engagement in any of these policies influences their income.
3.2.2 Propensity score matching method (PSM)
Evaluating the causal effects of participation in support policies, such as net farm income, presents challenges due to potential endogeneity biases. Voluntary and non-random participation may lead to systematic differences between participants and non-participants based on socioeconomic factors, which could influence net farm income. Direct comparisons of net farm incomes may yield biased results.
Accurate evaluation of impacts requires consideration of both observable and unobservable farmer characteristics. In an ideal scenario, farmers would be randomly assigned to groups to minimize selection bias. However, the absence of random assignment implies that certain farmer characteristics might influence their decision to utilize support policies, thereby affecting outcomes such as net farm income.
The relationship between support policies and net farm income can be expressed as:
Where
Here, D is the treatment variable, which is 1 if a farmer participates and 0 otherwise.
Utilizing the PSM approach offers a robust estimation of the effects of agricultural policies on farmer incomes, guiding policymakers and stakeholders. Participants are then matched with non-participants using their propensity scores, ensuring similar observable characteristics.
This research uses three primary matching methods:
Nearest-neighbor matching (k = 4): Pairs each treated farmer with the nearest untreated counterpart based on propensity scores.
Radius matching (caliper = 0.05): Matches treated farmers to untreated ones within a specific propensity score range.
Kernel matching (bwidth = 0.06): Uses a weighted average of all untreated farmers, based on the proximity of propensity scores.
After matching, the Average Treatment effect on the Treated (ATT) quantifies the net farm income difference attributed to participating in support policies:
The ATT sheds light on income disparities between participants and their matched non-participant counterparts. To address potential biases arising from observable characteristics, PSM may still be susceptible to unobserved biases influencing the outcome. To evaluate the robustness of the findings against such unobserved biases, a Rosenbaum bounds sensitivity test is employed. If significant variations in unobserved biases do not alter the outcomes, this strengthens the reliability of the results. Acknowledging possible caveats and limitations is essential for accurately interpreting the findings and informing the design of future research.
3.2.3 Data source and study area
Data was collected from Hubei, Zhejiang, and Shanghai—three provinces located in the middle and lower reaches of the Yangtze River basin—during May and June 2022. This basin, which also includes provinces such as Hunan and Jiangsu, is a major grain production area in China, playing a significant role in the nation’s food security. Geographically, the region is characterized by a basin-like structure, with flat terrains in the central area featuring surface runoff and lakes. In contrast, the surrounding areas are predominantly mountainous.
This diverse region encompasses various land uses, including arable land, woodland, grassland, water bodies, construction sites, and unutilized areas. It is also important to note that this region is a major source of China’s agricultural GHG emissions, as indicated in Figure 3.
Our survey primarily targeted rice farmers, with the questionnaire focusing on household-specific attributes and their participation in low-carbon agricultural support policies. Households were selected randomly, resulting in an initial 453 samples across the three provinces and cities. Out of these, 410 were considered valid.
The sample size was sufficient, ensuring that the 410 farming households represented the region’s demographic characteristics. Using the lists compiled by local village officers, households were selected randomly. For the survey, the primary decision-makers, household heads, or those responsible for farming activities provided responses to the questions.
From the data presented in Tables 3, 4, it can be observed that households that participate exhibit higher income levels compared to those that do not. The uptake rate for support policies is above 48%. Statistically significant differences are observed in attributes such as age, gender, education level, farming tenure, and the presence of a family member in village officialdom between participating and non-participating households. Participating households tend to be younger and have higher levels of educational attainment. In contrast, non-participating households are predominantly male, have longer farming tenures, operate smaller farms, and are more likely to engage in traditional farming practice.
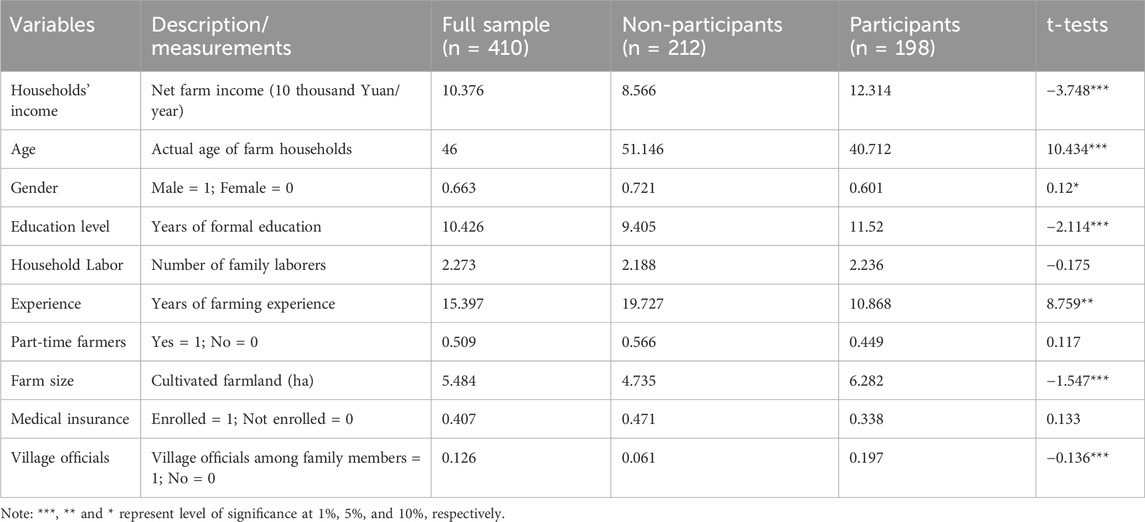
Table 4. Description, measurement and average of variables according to participation status in support policies (SP).
Although the final valid sample comprises 410 farming households, several steps were taken to ensure its representativeness. First, the sample was drawn from three core agricultural provinces (Hubei, Zhejiang, and Shanghai) located in the policy-priority Yangtze River Basin. Second, the survey followed a random sampling strategy, covering a broad spectrum of age, education, and farm size categories. Furthermore, the data were collected recently (May–June 2022), capturing up-to-date policy effects. Thus, the dataset is both timely and regionally representative, despite not being nationwide in scale.
4 Econometric analysis
4.1 Company level analysis
4.1.1 DID analysis
Using DID regression, we evaluate the LCAP policy’s influence on the environmental expenditures of listed companies (refer to Table 5). In the basic DID regression model, the environmental expenditure ln (
4.1.2 PSM analysis
For matched company characteristic variables, we considered the asset-liability ratio (lev), return on equity (ROE), company size (size), company nature (SOA), and board size (board size). The nearest neighbor match (k = 4) serves as the chosen matching method. Post matching, a balance test gauges the efficiency of the match. Its foundational hypothesis posits no systematic variance between control and treated groups. The PSM balance test outcomes in Table 6, with p-values exceeding 5% across all covariates, indicate non-rejection of this hypothesis. Thus, the matched sample meets prerequisites for subsequent regression examinations.
4.1.3 PSM-DID analysis
Our regression analysis, as shown in Table 7, indicates a statistically significant reduction in corporate environmental spending associated with the LCAP, with a decrease of 0.91 points at the 1% significance level. A key limitation of this study should be noted: the unavailability of proprietary corporate cost data, which restricts a thorough examination of the financial activities driving these outcomes.
Consequently, the interpretation regarding the LCAP’s impact on environmental expenditure is necessarily based on a theoretical framework as outlined by existing scholarly literature, rather than on direct empirical cost analyses. Theoretical perspectives suggest that regulatory interventions, such as the LCAP, may lead to a strategic reorientation of corporate environmental strategies, potentially enabling a more efficient allocation of resources. This could result in a reduction in reported environmental spending, reflecting a shift towards more cost-effective practices without necessarily compromising environmental stewardship.
Additionally, it is hypothesized that the LCAP’s incentive system may alter the financial frameworks governing environmental investments, potentially leading to a strategic reallocation of capital towards more sustainable practices with potential long-term financial benefits. These hypotheses, however, remain speculative in the absence of detailed cost data, highlighting the need for further empirical research to clarify the complex financial decisions influenced by policy.
The data highlight potential discrepancies in policy implementation between the central and local governments in China, a divergence that may lead to central policies such as the LCAP producing unexpected outcomes at the corporate level. Several factors may explain the empirical observations regarding the LCAP’s implementation and its subsequent effects on corporate environmental spending. The introduction of the LCAP in various municipalities may be hindered by a lack of motivation and consistent enforcement. Furthermore, differences in policy enforcement between central and local governments are particularly notable in the area of environmental regulation and tend to become more pronounced when directives are communicated to the corporate sector (Lo, 2014; Ran, 2013). Additionally, the tenure length of local officials in China is often associated with the economic growth patterns of their regions, sometimes leading to close relationships between government agencies and businesses. As tenure durations increase, they may facilitate the development of entrenched networks, potentially weakening the oversight of corporate compliance with environmental regulations (Huo et al., 2022). A further factor contributing to delayed adoption is evident in the local implementation of centrally mandated policies, with entities occasionally adopting a cautious approach towards central directives (Göbel, 2011; Chung, 2000). Given the priority of economic development on local agendas, environmental regulations may be given lower priority when perceived as less urgent than immediate economic goals.
At the enterprise level, the LCAP requires firms to adopt business practices with lower carbon emissions. At the same time, enterprises within LCAP jurisdictions are subject to strict environmental requirements, necessitating significant operational changes and potentially reducing short-term profitability (Duan and Xu, 2022). In a competitive environment, firms may reduce overall environmental spending as a way to offset the additional operational costs incurred. This strategic reallocation of resources may explain the observed reduction in environmental expenditures among the firms studied.
4.2 Farmer level analysis
4.2.1 PSM analysis
Table 8 presents the results from the probit model on the determinants influencing farmers’ participation in support policies. These determinants are not merely linked to participation but also play a pivotal role in computing the propensity scores for assessing the impact of the policies.
The estimated coefficients suggest that factors such as age, gender, and being a part-time farmer negatively influence the likelihood of participating in the support policies, and these findings are statistically significant. Research indicates that younger individuals may be more open to adopting new technologies due to their higher adaptability and optimism towards innovative practices (Li W. et al., 2021). Similarly, the gender gap in technology adoption, particularly in agriculture, can be attributed to a variety of socio-economic factors, where female farmers may be more proactive in adopting sustainable practices due to their greater concern for environmental stewardship (Schmidt et al., 2021). The lower participation rates among part-time farmers could be linked to the divided attention between farming and other employment responsibilities, which may limit their engagement with agricultural innovations (Li Z. et al., 2021).
Conversely, as anticipated, higher education levels, larger farm sizes, and having family members who hold official village positions correlate positively with a greater probability of participating in the support policies. A plausible rationale is that better-educated farmers possess a heightened ability to assimilate and interpret information, making them more inclined to adopt innovative agricultural practices (Huang et al., 2022). This is supported by evidence suggesting that education plays a crucial role in the adoption of agricultural technology, including low-carbon practices (Razzante et al., 2021). Additionally, farmers managing larger land areas may exhibit a greater need for information, particularly regarding innovative agricultural techniques, as larger-scale operations often require more efficient and sustainable practices (Lo, 2014). Moreover, farmers with familial ties to local government officials may have enhanced access to policy-related information, which could increase their likelihood of participating in support programs (Li et al., 2017; Ran, 2013; Li Z. et al., 2021; Jiang et al., 2022).
These insights are corroborated by empirical evidence from studies on rice farmers in Hubei province, which found that cognitive factors such as value perception and self-efficacy, often influenced by educational background and socio-economic status, significantly affect the adoption of low-carbon technologies (Zhao et al., 2021; Guo et al., 2021).
4.2.2 Impact of the support policies participation on net household’s income
Utilizing the Propensity Score Matching (PSM) methodology, we assessed the influence of participation in support policies on net household income. The results of this examination are detailed in Table 9. To guarantee the robustness of the outcomes, three distinct matching estimators - nearest-neighbor matching, radius matching, and kernel matching were employed.
As delineated in Table 9, irrespective of the matching estimator employed, the findings consistently reveal that participation in support policies exerts a positive and statistically meaningful impact on household income. Specifically, the evidence indicates an average increase in household income by approximately 28 thousand yuan if farmers choose to participate in these support policies. It is important to note that the minor variations in estimated values across the three different matching techniques support the reliability and robustness of these findings. This consistency suggests that our analytical results are not significantly dependent on the choice of a specific matching algorithm, thereby reinforcing the validity of the study’s conclusions.
In addition to our main analysis, we conducted robustness tests to validate the reliability of our results using various methods detailed by Caliendo and Kopeinig (Caliendo and Kopeinig, 2008). We employed three distinct approaches: Nearest-neighbor Matching, Radius Matching, and Kernel Matching. Post-matching, our findings confirmed significant balance between treated and control groups. Additionally, sensitivity analysis, based on the Rosenbaum boundary test further vouches for the robustness of our estimates against potential hidden biases. Overall, these assessments corroborate the genuine and impactful influence of support policies on farm income without exaggeration.
4.2.3 Impact of different support policies on farm household income
While Table 4 highlights that participation in low-carbon agricultural support policies can increase farmers’ income, the effectiveness of each individual policy varies. Therefore, it is essential to examine the specific impacts of each policy type.
In Table 10, a detailed analysis shows that the Average Treatment Effect on the Treated (ATT) for SP1 to SP3 is significantly positive, substantially influencing household income. In contrast, SP4 does not achieve statistical significance, indicating it does not have a notable impact on household income.
SP1 and SP2: The ATT results related to these policies, which involve subsidies for organic fertilizers and waste recycling, clearly indicate that they can contribute to increasing household income. It is worth noting that both SP1 and SP2 require minimal time and financial investment from farmers. Their implementation quickly improves the farmland environment and enhances soil quality. Additionally, the provision of subsidies reduces the financial costs associated with these policies, thereby boosting household income.
SP3: The ATT result for SP3 is somewhat more moderate compared to SP1 and SP2. While the adoption of energy-conserving machinery can enhance production efficiency, the relatively high initial investment limits its short-term income-boosting effects, even with subsidies considered. However, the long-term potential of this machinery is significant. It can substantially reduce greenhouse gas emissions and is expected to deliver considerable economic and environmental benefits over time.
SP4: This policy, which focuses on skills training or lectures, shows a weak ATT. Such non-monetary support policies do not have a direct or immediate impact on farmers’ income. This muted result can be attributed to the variability in knowledge absorption from these trainings. Additionally, there is often a gap between farmers’ stated intentions to adopt low-carbon agricultural practices and their actual implementation.
In summary, while low-carbon agricultural support policies collectively contribute to increasing farmers’ income, the extent of this increase varies significantly depending on the specific policy. Monetary policies, especially those requiring minimal investment, tend to produce quicker financial returns, while non-monetary policies or those requiring substantial investments may realize their primary benefits over a longer period.
4.2.4 Impact of heterogeneity among support policies beneficiaries
The propensity score matching (PSM) method typically assumes that treatment effects are uniform across all beneficiaries of a specific policy. While this assumption simplifies the analytical process, it may overlook the varied impacts resulting from the diverse socioeconomic characteristics of the participant population.
To explore this inherent heterogeneity, the sample was divided based on the mean values of two key determinants: education levels and farm size. These factors have consistently been identified as critical in influencing the effectiveness of agricultural support interventions. As a result, the analysis compared the “above the mean value” group with the “below the mean value” group.
Educational Attainment: The findings presented in Table 11 highlight a notable pattern. The benefits of support policy participation are more pronounced for farmers with educational attainment above the mean. A potential explanation is that education equips farmers with the ability to assimilate, process, and apply new knowledge, particularly in the context of low-carbon agricultural practices. This advantage enables more efficient implementation of low-carbon techniques, leading to better income outcomes compared to farmers with lower educational attainment.
Farm Size Considerations: Farm size also plays a significant role in determining the extent of the policy impact. The analysis indicates that farmers with farm sizes exceeding the mean value derive greater benefits from the support policies. This outcome can be attributed to two main factors.
First, larger farms benefit from economies of scale, enhancing the tangible effects of financial incentives and input subsidies. Second, the per-unit transaction costs, whether in terms of time or resources, decrease as farm size increases. This efficiency, combined with targeted information on low-carbon agricultural practices, maximizes the gains for farmers operating larger farms.
These findings emphasize the importance of acknowledging the differential responses of farmers to agricultural support policies. Such heterogeneity, driven by variations in educational backgrounds and operational scales, should be a central consideration in the design and refinement of policy interventions. A more nuanced approach will help ensure a more equitable and effective distribution of resources and benefits.
5 Results and discussion
5.1 Discussion and implications at the corporate Sphere
In the field of corporate environmental economics, the LCAP initiative requires a fundamental shift toward sustainable operational practices, measured against historical carbon footprints. Entities subject to LCAP regulations are navigating a stricter regulatory environment, marked by mandatory eco-focused corporate practices and increased financial penalties for environmental violations. This regulatory shift demands operational adjustments, likely raising short-term operational costs and affecting profit margins. Within competitive markets, firms may exercise strategic discretion in allocating environmental budgets, potentially explaining the LCAP’s moderating effect on such expenditures.
This study expands the understanding of the implications of China’s LCAP directive. Employing an advanced PSM-DID regression approach, which addresses sample selection biases more effectively than traditional DID analysis, we identify a statistically significant reduction in environmental expenditures among public enterprises due to the LCAP, supported at a 1% significance level. This decrease of 0.91 points in environmental spending, while statistically robust, requires careful interpretation within the context of an unexamined cost structure. It raises questions about whether this reduction reflects strategic resource reallocation or a genuine decline in environmental stewardship.
This research explores previously unexamined areas, offering quantitative insights into the LCAP’s influence on corporate environmental spending. It highlights the divergence between policy objectives and corporate actions, potentially revealing unintended economic consequences at the corporate level.
Moving forward, the balance between environmental responsibility and economic performance warrants further attention at the microeconomic level. Local governance structures, with their nuanced understanding of economic and social priorities, play a critical role in ensuring balanced policy implementation. Strengthening their involvement in policy design could help address disparities in policy execution.
However, the conclusions drawn here must be considered in light of the study’s limitations. The lack of comprehensive corporate cost data restricts the depth of economic analysis regarding the LCAP’s impacts. Additionally, the focus on listed companies may not fully represent the broader corporate landscape.
Despite these limitations, this study provides a distinct perspective on the microeconomic implications of the LCAP, calling for more extensive and methodologically rigorous future research. Subsequent studies should incorporate a broader range of financial data to develop a more detailed and accurate understanding of the LCAP’s effects on corporate economic and environmental strategies.
5.2 Reflections and conclusions at the farmer tier
The empirical findings of this study highlight the complex factors influencing farmers’ adoption of low-carbon practices. Educational attainment, farm size, and established local leadership connections are identified as significant facilitators, while personal demographics and external responsibilities may act as barriers. Within this intricate socio-institutional context, policy measures must be flexible and culturally sensitive to effectively encourage farmer participation.
Our rigorous PSM analysis demonstrates a notable increase in farmer income, with the average opportunity cost of non-participation estimated at 28 thousand yuan in terms of net income. Subsidies for organic fertilization and agricultural residue recycling are particularly effective, offering both economic benefits and ecological advantages. In contrast, the adoption of energy-efficient machinery, while environmentally beneficial, faces challenges due to high initial costs and delayed returns on investment.
Based on these findings, the study emphasizes the dual advantages of organic composting and waste recycling initiatives, advocating for their central role in China’s agricultural environmental strategy. These initiatives are supported not only for their direct financial incentives but also for their alignment with ecological sustainability. The transformative potential of these low-carbon incentives is confirmed, with the understanding that improved farmer education and community engagement are essential to sustaining this green transition.
While the analysis suggests that the LCAP policy contributes to increasing farmer income, it is important to note the absence of comprehensive cost data. This limitation calls for cautious interpretation of the policy’s net income effects. Nonetheless, given the LCAP’s emphasis on subsidies, a reduction in the costs associated with adopting low-carbon agricultural practices is expected. Despite the lack of detailed cost analysis, the policy’s potential to lower production costs and enhance farmer income remains plausible.
In summary, the insights provided by this study offer policymakers a solid foundation for designing and implementing comprehensive, farmer-focused, and sustainable agricultural policies.
5.3 Policy recommendations
The findings across corporate and farmer tiers point to the need for more integrated and adaptive policy design. Although the LCAP policy offers clear economic incentives, its fragmented implementation and limited alignment with other environmental frameworks may reduce its overall effectiveness.
First, LCAP should be better integrated with ecological compensation programs, carbon markets, and green finance instruments. For example, providing carbon credits or preferential loans for firms and farmers engaging in verified low-carbon practices could enhance motivation and long-term sustainability.
Second, localized governance must be strengthened. Local governments and cooperatives should be granted flexibility in tailoring LCAP programs to regional conditions, supported by standardized national guidelines and performance benchmarks.
Third, enhancing capacity building and stakeholder engagement is essential. Targeted training, digital information platforms, and participatory planning can lower adoption barriers, especially for smallholders and resource-constrained enterprises.
Data availability statement
The raw data supporting the conclusions of this article will be made available by the authors, without undue reservation.
Author contributions
JL: Investigation, Resources, Supervision, Writing – original draft, Writing – review and editing. MY: Data curation, Investigation, Methodology, Software, Writing – original draft. LH: Data curation, Formal Analysis, Resources, Software, Writing – original draft, Writing – review and editing.
Funding
The author(s) declare that no financial support was received for the research and/or publication of this article.
Acknowledgments
The authors want to acknowledge the advice of experts and the farmers who cooperated with the questionnaire.
Conflict of interest
Author YM was employed by Mitsubishi Electric Corporation. Author HL was employed by Kirin Holdings Company, Ltd.
The remaining author declares that the research was conducted in the absence of any commercial or financial relationships that could be construed as a potential conflict of interest.
The handling editor JP declared a past co-authorship with the author JL.
Generative AI statement
The author(s) declare that no Generative AI was used in the creation of this manuscript.
Publisher’s note
All claims expressed in this article are solely those of the authors and do not necessarily represent those of their affiliated organizations, or those of the publisher, the editors and the reviewers. Any product that may be evaluated in this article, or claim that may be made by its manufacturer, is not guaranteed or endorsed by the publisher.
References
Breustedt, G., and Glauben, T. (2007). Driving forces behind exiting from farming in Western Europe. J. Agric. Econ. 58, 115–127. doi:10.1111/j.1477-9552.2007.00082.x
Caliendo, M., and Kopeinig, S. (2008). Some practical guidance for the implementation of propensity score matching. J. Econ. Surv. 22 (1), 31–72. doi:10.1111/j.1467-6419.2007.00527.x
Chen, H., Guo, W., Feng, X., Wei, W., Liu, H., Feng, Y., et al. (2021). The impact of low-carbon city pilot policy on the total factor productivity of listed enterprises in China. Resour. Conserv. Recycl. 169, 105457. doi:10.1016/j.resconrec.2021.105457
Cheng, J., Yi, J., Dai, S., and Xiong, Y. (2019). Can low-carbon city construction facilitate green growth? Evidence from China's pilot low-carbon city initiative. J. Clean. Prod. 231, 1158–1170. doi:10.1016/j.jclepro.2019.05.327
Chung, J. H. (2000). Central control and local discretion in China: leadership and implementation during post-mao decollectivization. USA, OUP Catalogue.
Clarke, C. L., Shackleton, S. E., and Powell, M. (2012). Climate change perceptions, drought responses and views on carbon farming amongst commercial livestock and game farmers in the semiarid great fish river valley, eastern cape province, South Africa. Afr. J. Range Forage Sci. 29 (1), 13–23. doi:10.2989/10220119.2012.687041
Du, Y., Liu, H., and Huang, Li X. (2023). The carbon emission reduction effect of agricultural policy - evidence from China. J. Clean. Prod. 294, 137005. doi:10.1016/j.jclepro.2023.137005
Duan, M., and Xu, R. (2022). Does low-carbon city pilot affect the enterprise competitiveness in China? Based on a staggered difference-in-difference model. Environ. Sci. Pollut. Control Ser. 30, 37233–37247. doi:10.1007/s11356-022-24667-1
Ganda, F. (2023). The influence of agricultural policy on carbon emissions in selected OECD countries. Heliyon 9 (9), 19881. doi:10.1016/j.heliyon.2023.e19881
Giné, X., and Yang, D. (2009). Insurance, credit, and technology adoption: field experimental evidence from Malawi. J. Dev. Econ. 89 (1), 1–11. doi:10.1016/j.jdeveco.2008.09.007
Göbel, C. (2011). Uneven policy implementation in rural China. China J. 65, 53–76. doi:10.1086/tcj.65.25790557
Guo, L., Li, H., Cao, X., Cao, A., and Huang, M. (2021). Effect of agricultural subsidies on the use of chemical fertilizer. J. Environ. Manag. 299, 113621. doi:10.1016/j.jenvman.2021.113621
Huang, B., Kong, H., Yu, J., and Zhang, X. (2022). A study on the impact of low-carbon technology application in agriculture on the returns of large-scale farmers. Int. J. Environ. Res. Public Health 19 (16), 10177. doi:10.3390/ijerph191610177
Huang, Y., Sun, W., Zhang, W., Yu, Y., and Su, Y. (2012). Net primary production of Chinese croplands from 1950 to 1999. Ecol. Model. 227, 27–37. Available online at: https://www.jstor.org/stable/40061833.
Huo, W., Yang, Q., Liu, J., Liu, M., and Zhou, Z. (2022). Effects of China's pilot low-carbon city policy on carbon emission reduction: a quasi-natural experiment based on satellite data. Technol. Forecast. Soc. Change 175, 121422. doi:10.1016/j.techfore.2021.121422
Jiang, L., Huang, H., He, S., Huang, H., and Luo, Y. (2022). What motivates farmers to adopt low-carbon agricultural technologies? Empirical evidence from thousands of rice farmers in Hubei province, central China. Front. Psychol. 13 (18), 983597. doi:10.3389/fpsyg.2022.983597
Karlan, D., Osei, R., Osei-Akoto, I., and Udry, C. (2014). Agricultural decisions after relaxing credit and risk constraints. Q. J. Econ. 129 (2), 597–652. doi:10.1093/qje/qju002
Laborde, D., Mamun, A., Martin, W., Piñeiro, V., and Vos, R. (2021). Agricultural subsidies and global greenhouse gas emissions. Nat. Commun. 12 (1), 2601–2609. doi:10.1038/s41467-021-22703-1
Li, C., Li, X., and Jia, W. (2023a). Non-farm employment experience, risk preferences, and low-carbon agricultural technology adoption: evidence from 1843 grain farmers in 14 provinces in China. Agriculture 13 (1), 24. doi:10.3390/agriculture13010024
Li, L., Han, J., and Zhu, Y. (2023b). Does environmental regulation in the form of resource agglomeration decrease agricultural carbon emissions? Quasi-natural experimental on high-standard farmland construction policy. J. Clean. Prod. 420, 138342. doi:10.1016/j.jclepro.2023.138342
Li, P., Jiang, S., and Cao, X. (2017). A study on the influencing factors of bicycle travel for residents in low-carbon pilot cities--Baoding city as an example. Surv. World 12, 23–27. doi:10.13778/j.cnki.11-3705/c.2017.12.004
Li, W., Menjivar, J., Zhang, L., and Zhang, J. (2021a). Climate change perceptions and the adoption of low-carbon agricultural technologies: evidence from rice production systems in the Yangtze River Basin. Sci. Total Environ. 759, 143554. doi:10.1016/j.scitotenv.2020.143554
Li, Z., Pan, Y., Yang, W., Ma, J., and Zhou, M. (2021b). Effects of government subsidies on green technology investment and green marketing coordination of supply chain under the cap-and-trade mechanism. Energy Econ. 101, 105426. doi:10.1016/j.eneco.2021.105426
Liang, D., Lu, X., Zhuang, M., Shi, G., Hu, C., Wang, S., et al. (2021). China’s greenhouse gas emissions for cropping systems from 1978–2016. Sci. Data 8 (1), 171–210. doi:10.1038/s41597-021-00960-5
Liang, H., Meng, Y., and Ishii, K. (2022). The effect of agricultural greenhouse gas emissions reduction policies: evidence from the middle and lower basin of Yangtze River, China. Discov. Sustain. 3 (43), 43–17. doi:10.1007/s43621-022-00107-5
Liang, P., and Gao, N. (2014). Personnel change, legal environment and local environmental pollution. Manag. World 2014, 65–78. doi:10.19744/j.cnki.11-1235/f.2014.06.007
Liu, J., You, L., Amini, M., Obersteiner, M., Herrero, M., Zehnder, A. J., et al. (2013). A high-resolution assessment on global nitrogen flows in cropland. Proc. Natl. Acad. Sci. 110 (21), 8035–8040. doi:10.1073/pnas.0913658107
Lo, K. (2014). China's low-carbon city initiatives: the implementation gap and the limits of the target responsibility system. Habitat Int. 42, 236–244. doi:10.1016/j.habitatint.2014.01.007
McFadden, D. (1974). The measurement of urban travel demand. J. Publ. Econ. 3 (4), 303–328. doi:10.1016/0047-2727(74)90003-6
Ministry of Ecology and Environment, The People’s Republic of China (2022). China’s policies and actions for addressing climate change. Available online at: https://english.mee.gov.cn/Resources/Reports/reports/(Accessed December 4, 2022).
Mittenzwei, K., Persson, T., Höglind, M., and Kværnø, S. (2017). Combined effects of climate change and policy uncertainty on the agricultural sector in Norway. Agric. Sys 153, 118–126. doi:10.1016/j.agsy.2017.01.016
Ran, R. (2013). Perverse incentive structure and policy implementation gap in China's local environmental politics. J. Environ. Pol. Plann. 15 (1), 17–39. doi:10.1080/1523908x.2012.752186
Razzante, S., Labarta, R., and Bilton, A. (2021). Adoption of agricultural technology in the developing world: a meta-analysis of the empirical literature. World Dev. 146, 105599. doi:10.1016/j.worlddev.2021.105599
Schmidt, C., Goetz, S. J., and Tian, Z. (2021). Female farmers in the United States: research needs and policy questions. Food Policy 101, 102039. doi:10.1016/j.foodpol.2021.102039
Shi, H., Xu, H., Gao, W., Zhang, J., and Chang, M. (2022). The impact of energy poverty on agricultural productivity: the case of China. Energy Polic. 167, 113020. doi:10.1016/j.enpol.2022.113020
Su, L., Wang, Y., and Yu, F. (2023). Analysis of regional differences and spatial spillover effects of agricultural carbon emissions in China. Heliyon 9 (6), 16752. doi:10.1016/j.heliyon.2023.e16752
Tang, P., Yang, S., Shen, S., and Fu, S. (2018). Does China's low-carbon pilot programme really take off? Evidence from land transfer of energy-intensive industry. Energy Pol. 114, 482–491. doi:10.1016/j.enpol.2017.12.032
Wang, C., Deng, M., and Deng, J. (2020). Factor reallocation and structural transformation implications of grain subsidies in China. J. Asian Econ. 71, 101248. doi:10.1016/j.asieco.2020.101248
Yan, X., Akiyama, H., Yagi, K., and Akimoto, H. (2005). Direct N2O emissions from rice paddy fields: summary of available data. Glob. Biogeochem. Cycles 19 (2). doi:10.1029/2004GB002378
Yang, B., Chen, X., Wang, Z., Li, W., Zhang, C., and Yao, X. (2020). Analyzing land use structure efficiency with carbon emissions: a case study in the Middle Reaches of the Yangtze River, China. China J. Clean. Prod. 274, 123076. doi:10.1016/j.jclepro.2020.123076
Zhang, K., Wang, J., and Cui, S. (2011). Fiscal decentralization and environmental pollution: a carbon emission perspective. China Ind. Econ. 10, 65–75. doi:10.19581/j.cnki.ciejournal.2011.10.007
Zhang, Z., Tian, Y., and Chen, Y. (2023). Can agricultural credit subsidies affect county-level carbon intensity in China? Sustain. Prod. Consum. 38, 80–89. doi:10.1016/j.spc.2023.03.028
Keywords: low-carbon agricultural pilot policy, environmental expenditures, farmers’ net income, propensity score matching -difference in differences, China’s agriculture and food industries, policy-implementation gap
Citation: Jia L, Meng Y and Liang H (2025) Efficacy of China’s low-carbon agricultural pilot policy: a company-farmer analysis from the middle and lower yangtze river basin, China. Front. Environ. Sci. 13:1581752. doi: 10.3389/fenvs.2025.1581752
Received: 23 February 2025; Accepted: 28 April 2025;
Published: 09 May 2025.
Edited by:
Jiachao Peng, Wuhan Institute of Technology, ChinaReviewed by:
Wang Zhang, Northwest University, ChinaZhengwei Cao, Shanghai Jiao Tong University, China
Copyright © 2025 Jia, Meng and Liang. This is an open-access article distributed under the terms of the Creative Commons Attribution License (CC BY). The use, distribution or reproduction in other forums is permitted, provided the original author(s) and the copyright owner(s) are credited and that the original publication in this journal is cited, in accordance with accepted academic practice. No use, distribution or reproduction is permitted which does not comply with these terms.
*Correspondence: Hanzhong Liang, SGFuemhvbmdfTGlhbmdAa2lyaW4uY28uanA=