- 1Chongqing Ecological and Environmental Monitoring Center, Chongqing, China
- 2China Merchants Ecological Environmental Protection Technology Co., Ltd., Chongqing, China
- 3Institute of Agricultural Quality Standard and Testing Technology, Chongqing Academy of Agricultural Sciences, Chongqing, China
- 4China National Environmental Monitoring Center, Beijing, China
In China, coal remains the dominant energy source, leading to substantial production of coal gangue. This study established comparative zones—a study area and a control area—and applied the Geo-Detector model (GDM), Absolute Principal Component Score-Multiple Linear Regression (APCS-MLR) model, and cadmium isotope fingerprinting to delineate the origins of heavy metal(loid)s (HMs) in soil proximate to accumulations of coal gangue. The contents of cadmium (Cd), mercury (Hg), arsenic (As), and lead (Pb) were significantly elevated in the study area relative to the control, whereas the levels of chromium (Cr), copper (Cu), nickel (Ni), and zinc (Zn) displayed no marked differences between the zones, underscoring an intensified pollution level in the study region. The integration of GDM, APCS-MLR, and Cd isotope fingerprinting methods significantly enhanced the precision and dependability in identifying pollution sources. Soil contamination in the study area was predominantly due to persistent coal gangue accumulation, vehicular emissions, and agricultural chemicals, contributing 40.1%, 33.7%, and 26.2% respectively, while in the control area, vehicular emissions and agricultural activities were the main contributors. Surface runoff from coal gangue accumulation emerged as the dominant pathway for soil contamination in the study area. The study underscores the urgency of implementing strategic coal gangue management and mitigation of ecological hazards, particularly those associated with elevated Cd levels.
1 Introduction
Coal has long been a crucial energy source, extensively utilized across multiple industries (Wang et al., 2024). China is the world’s largest coal producer and consumer, contributing ∼50% of global coal production (Liu and Song, 2024), and coal consumption escalated from 60 million tons of oil equivalent (Mtoe) in 1971 to 1907 Mtoe in 2018, this trajectory, projected to reach 2,410 Mtoe by 2030 (Shahbaz et al., 2015; Tiwari et al., 2023). This significant increase in consumption has prompted widespread mining operations. Generated primarily through these processes, coal gangue constitutes 10%–15% of overall coal output and has amassed to over a billion tons, with a yearly rise of 150–200 million tons. This has become a significant byproduct of industrial processes (Zhang et al., 2015; Gao et al., 2021). A multitude of studies has identified the accumulation of coal gangue as a significant contributor to heavy metal(loid)s (HMs) pollution, which disperses to surrounding soils through surface runoff and atmospheric deposition (Song et al., 2023; Zhang et al., 2024; Sun et al., 2021; Wang and Wang, 2018; Tokatlı et al., 2025). Importantly, HMs such as Cd, As, and Pb are designated as priority control pollutants by the United States Environmental Protection Agency (USEPA) because of their persistent toxicity (Arora and Chauhan, 2021; Ma et al., 2024a; Din et al., 2024). The presence of these HMs can adversely affect a wide range of plant species at increased contents, while also presenting significant health hazards to humans (Yüksel and Ustaoğlu, 2025; Din et al., 2023; Ali and Muhammad, 2023).
The origins of soil HM pollution are varied and intricate. Beyond coal gangue, significant sources include soil parent material weathering, agricultural irrigation, fertilizer and pesticide usage, and emissions from industrial and road traffic (Ma et al., 2024a; Wang et al., 2019; Xiao et al., 2019; Muhammad et al., 2024). Thus, precise identification and quantification of HMs sources in soils near coal gangue heap are crucial for effectively managing and reducing regional soil HM pollution. Presently employed receptor models such as the APCS-MLR and Positive Matrix Factorization (PMF) are preferred for their ease, speed, and efficiency (Shi et al., 2024; Ma J. et al., 2023; Yao et al., 2024). The former identifies sources via PCA, quantifying their contributions through MLR between HMs content and APCS. The latter uses uncertainty-based weighting, decomposing the data matrix into factor contributions and profiles through iterative least-squares refinement (Ma et al., 2024b). Nevertheless, these models present limitations: outliers may skew the distribution of HM source apportionment results (Huang et al., 2018; Lv, 2019), and these models frequently overlook additional environmental factors (Ma J. et al., 2023; Yang et al., 2021), resulting in source identifications that rely predominantly on subjective assumptions by the user, which are challenging to substantiate (Ma J. et al., 2023; Shi et al., 2023). Recent advancements have demonstrated that combining receptor models with the Geo-Detector Model (GDM) can decrease user subjectivity and enhance the precision and dependability of source apportionment (Ma et al., 2024b; Guo et al., 2022). This enhancement benefits from the GDM’s capability to discern principal factors influencing soil HMs and to assess the nonlinear relationships between these factors and soil HMs, making it especially suited for analyzing spatial variations (Guo et al., 2022; Shi et al., 2018; Zeng et al., 2023).
However, robust evidence directly connecting receptors to specific contaminants is still limited. Isotopic ratios serve as distinctive markers in environmental studies, allowing researchers to identify possible origins of HMs and track their movement (Yao et al., 2023; Zhong et al., 2022; Wang et al., 2021). Such as elevated-temperature operations, including smelting, coal combustion, and the production of glass, induce notable isotopic fractionation in cadmium. In these processes, lighter isotopes preferentially ascend into the gaseous state, whereas heavier isotopes accumulate in residual materials (Xia et al., 2025; Zhong et al., 2020). Moreover, isotopic ratios of Cd in terrestrial minerals and rocks generally show scant variance (Liang et al., 2024). Extensive research has demonstrated that the cadmium isotope ratio (δ114/110Cd) is a robust tracer for pinpointing the origins of cadmium within environmental settings, elucidating isotopic discrepancies across varying contents (Cloquet et al., 2006). We believe that the combined application of multiple source analysis methods can make the analytical results more reliable, accurate and scientific.
This study are designed to achieve primary objectives: (i) ascertain the levels, spatial arrangement, and stratigraphic profiles of HMs adjacent to a coal gangue heap by designating investigatory and control zones; (ii) combined application of multiple source analysis methods, discern the sources of these HMs and quantitative assess their effects on the soil near the coal gangue heap.
2 Materials and methods
2.1 Study area
The southern Qijiang District of Chongqing Municipality in southwest China is where the specified study and control regions are located (Figure 1a). The area experiences four separate seasons of pleasant, humid weather with an annual rainfall of around 1,100 mm, and it is characterized by a subtropical monsoon climate.
The study area encompasses approximately 30 hm2, positioned at elevations between 700 and 800 m (Figure 1b), nestled among valleys and encircled by steep mountainous terrain. It includes two residential clusters spanning about 2 hm2, interconnected by primary roads. An abandoned gangue heap, occupying about 7 hm2 and standing 30 m tall with a 40-year storage history, lies to the east. Currently inactive, the site no longer accepts coal gangue.
Conversely, the control area is situated 2 km south of the study zone, covering an area of about 30 hm2 with elevations ranging from 430 to 700 m (Figure 1b). It abuts a major road on the east, bordered by dispersed settlements. A mountain barrier distinctly separates the study and control zones, shielding the latter from any surface runoff from the coal gangue heap and devoid of any nearby industrial or mining activities.
In both the study and control areas, the land use type was predominantly cultivated land, primarily planted with corn and vegetables. Nitrogen and organic fertilizers were the main inputs applied. Furthermore, there were no industrial or mining enterprises operating within a 3 km radius of either area.
2.2 Samples and analysis
Since both the study and control areas are situated in hilly regions, the cultivated land plots are small and fragmented, with most field surfaces measuring approximately 150 m in length. Therefore, a 150 m × 150 m grid system was established, with 38 agricultural soil sampling sites (19 in each zone) distributed across the study and control areas (Figure 1b). Surface soil (SS) samples were collected following the standardized five-point sampling protocol, ensuring representative coverage of each grid: one central subsample and four peripheral subsamples taken 10 m from the center in the cardinal directions (Yao et al., 2024). Additionally, to enhance the representativeness of sampling points, the selection of soil profile locations primarily considered the distance from the coal gangue heap. In each area, eight distinct soil profiles (T1, T6, T8, T10, T30, T32, T36, T38) were excavated and segmented into three layers: surface soil (SS, 0–20 cm), subsoil (20–60 cm), and substratum (60–100 cm) (Ma et al., 2024b).
Post a 48-hour air-drying period, all samples were cleared of roots and organic residues using tweezers. The soil’s pH level was measured after grinding and sieving through a 2 mm nylon mesh polyethylene sieve, using a pH conductivity meter (SevenExcellence, CH) with a 1:2.5 soil-to-water ratio (MEE, 2018). For Cd and Hg analysis, the ground samples were further sieved through a 0.150 mm nylon mesh. Cd digestion followed the protocol of HCl-HF-HNO3-HClO4 as specified in GB/T 17141 (MEE, 1997), with detection by graphite furnace atomic absorption spectroscopy (ZEEnit700P, GER). Hg was treated with HNO3-HCl as per GB/T 22105.1 (SAMR and NSA, 2008), analyzed using atomic fluorescence spectrophotometry (AFS-9750, CHN). For other HMs like As and Zn, after sieving through a 0.075 mm sieve, the samples were examined using X-ray fluorescence spectrometry (S8 TIGER, GER) in accordance with HJ 780 (MEE, 2015). The method detection limits for Cd, As, Pb, Cr, Cu, Ni, and Zn were 0.01, 0.002, 0.01, 2.0, 3.0, 1.2, 1.5, and 2.0 mg/kg, respectively. To ensure the accuracy and reliability of the data, Geochemical Soil Standard Reference Samples (GSS-5a and GSS-8) were employed for quality control. The standard deviation for each sample remained below 10%, confirming the high precision and reliability of the experimental results.
In addition, five coal gangue samples (CG1, CG2 at 0–50 cm; CG3, CG4, CG5 at 50–100 cm) along with 24 soil samples from the mentioned profiles were prepared for Cd isotope analysis following the protocols established by Yao et al. (2023) (Yao et al., 2023). Adopting the robust alkaline anion exchange resin AGMP-1M, Cd contents between 50 and 100 ng were reduced. Beginning with 2 mol/L HCl, moving on to 1 mol/L HCl, 0.3 mol/L HCl, 0.06 mol/L HCl, and finally 0.0012 mol/L HCl, the matrix elements were eluted in a graduated fashion after the samples were introduced. Further, an HCl solution with a content of 0.0012 mol/L was used to extract Cd. The separated Cd solutions were dried out by evaporating them on a hotplate set at 130°C for the entire night. For maximal Cd refinement, the air-dried samples were reconstituted in 2 mol/L HCl and were purified cyclically repeated using the same column arrangement. Afterwards, the stock solution for isotope analysis was prepared by resolving the Cd samples in 2% HNO3. Surprisingly, all samples and reference materials consistently achieved recovery rates over 90% during the digestion and purification processes. A ulticollector inductively coupled plasma mass spectrometer (MC-ICP-MS, Neptune II, Thermo Scientific, United States) was applied to assess Cd isotope patterns. The assay was calibrated and quality-controlled using the widely-recognized Cd isotopic standard (NIST SRM 3108). The State Key Laboratory of Environmental Geochemistry conducted all related procedures.
2.3 Research methods
2.3.1 GDM
The GDM, introduced by Wang and Hu (2012), is effective in recognizing inherent correlations and addressing the spatial variability of factors. This tool is instrumental in the systematic determination of significant influencers (Wang and Hu, 2012). and has been broadly applied in uncovering driving elements in soil studies (Dong et al., 2021).
The interaction between the independent variables (X) and the dependent variable (Y) can be quantified by factor analysis (Zhao et al., 2021). The descriptive strength of this relationship is commonly indicated by the q-value, whose calculation is outlined as below:
In the formula, q quantifies the explanatory power of X over Y, on a scale of 0–1, with a higher value indicating a stronger influence; h indexes the specific strata related to Y and X; Nh and N stand for the respective zones of each stratum h and the total area; and
Reflecting on the relevance of environmental factors around the coal gangue heap and the availability of data, six key variables were chosen: the vertical height difference between the sampling point and the coal gangue heap (X1), the slope at the sampling point (X2), the distance from the sampling site to the coal gangue heap (X3), the proximity of the sampling site to the main road (X4), the distance from the sampling site to the nearest residential area (X5), and the Soil pH (X6). Coordinates for X1, X3, X4, and X5 were sourced from 91 Map Assistant 19.4.0, while X2 was derived from ArcGIS 10.2, and X6 was directly measured in this investigation. The natural breakpoint method was applied to segment X1∼X6 into five distinct categories each.
2.3.2 APCS-MLR model
The APCS-MLR model, introduced by Thurston and Spengler (1985), employs APCS from PCA. These scores are combined with MLR to detect potential pollution sources, characterize their nature, and estimate their contributions (Thurston ang Spengler, 1985). The application of this model is prevalent in soil pollution source apportionment studies (Ma J. et al., 2023; Lv, 2019; Gong et al., 2024). The formula for the model is structured as follows:
where
2.3.3 Cd isotope
The isotopic signature of cadmium is determined by measuring the 114Cd/110Cd ratios, presented in the delta (δ) notation. This process standardizes the sample’s isotope ratio to that of the NIST standard (specifically, NIST SRM 3108) (Yao et al., 2024; Imseng et al., 2018), according to the following formula:
2.4 Statistical analysis
Descriptive statistics was conducted with Microsoft Excel 2016, involving calculations of average, peak, and minimal values, along with the coefficient of variation (CV). GeoDetector 2015 software was utilized for GDM analysis. Spatial distribution diagrams were generated using ArcMap 10.2. Statistical analyses, including the APCS-MLR model, correlation tests (Significant p ≤ 0.05, Extremely Significant p ≤ 0.01), principal component analysis (PCA), and independent-samples t-tests (Significant p ≤ 0.05,Extremely Significant p ≤ 0.01), were conducted using SPSS Statistics 24.0.
3 Results and discussions
3.1 Characteristics of HMs contents in SS
Figure 2 and Supplementary Table S1 document the SS metrics. Within the study area, the mean contents of Cd, Hg, As, Pb, Cr, Cu, Ni, and Zn were 1.33, 0.29, 13.8, 32.9, 142, 68.8, 54.6, and 118 mg/kg, respectively. Cd contents surpassed the risk screening values at 16 sites (84.2%), and Cu at 8 sites (42.1%) (MEE and SAMR, 2018). Conversely, the control area recorded average contents of 0.42, 0.17, 10.2, 26.1, 141, 76.1, 58.7, and 125 mg/kg, respectively, with Cd exceeding risk thresholds at 12 sites and Cu at 10 sites (MEE and SAMR, 2018). Thus, the analysis confirmed Cd as the primary pollutant. The contents of Cd, Hg, As, and Pb were significantly elevated in the study area relative to the control, whereas the levels of Cr, Cu, Zn, and Ni displayed no marked differences between the zones, underscoring an intensified pollution level in the study region.
In the study area, the CV values were sorted as follows: Cd (111.4%) > Hg (58.6%) > Cu (38.0%) > Pb (34.5%) > Zn (26.5%) > As (26.2%) > Ni (21.2%) > Cr (9.4%). In contrast, the control area displayed CV values of: Cd (34.4%) > As (29.7%) > Cu (29.2%) > Hg (24.1%) > Ni (22.4%) > Pb (20.5%) > Zn (17.6%) > Cr (16.5%). According to the CV classification proposed by Wilding (1985), Cd, Hg, and Cu in the study area fall into the high variation category (CV > 36%), indicating substantial anthropogenic influence. In contrast, other HMs demonstrated moderate or low variation (CV ≤ 36%). Notably, Cr showed the lowest CV in both zones, suggesting minimal anthropogenic impact (Yang et al., 2021; Delbari et al., 2019).
3.2 Spatial distribution of HMs in SS
Figure 3 illustrates the HM distribution patterns in soil. Sub-Figures 3a–d reveal that Cd, Hg, As, and Pb share similar dispersal trends within the study zone, with contents escalating as proximity to the coal gangue heap decreases. Correlation analysis indicated a strong linkage among these HMs (P ≤ 0.01, r = 0.59–0.77) (Figure 4a), signifying a common pollution source: the prolonged accumulation at the coal gangue heap. Conversely, in the control zone, these elements displayed a more uniform and stable distribution. Prior research has shown that coal gangue heaps are the main culprits when it comes to HM enrichment in nearby SS (Song et al., 2023; Li et al., 2016; Chen et al., 2024; Ge et al., 2016). This is because HMs are transferred from coal gangue heaps to nearby SS by surface runoff, dust, and leachate (Yao et al., 2023; Dong et al., 2024; Ma et al., 2023b). The coal gangue heap’s location in mountainous terrain limits dust diffusion into the atmosphere. Additionally, a mountain barrier that separates the study and control zones effectively protects the soil in the control area from significant impacts. However, due to its higher elevation, the coal gangue heap’s leachate infiltrates the study area’s soil through rainwater runoff. It is thus preliminarily concluded that Cd, Hg, As, and Pb contents near the coal gangue heap in the study area are primarily influenced by surface runoff, while in the control area, these elements are not significantly affected by the coal gangue. The spatial distribution of Cu, Zn, and Ni in the study area was similar (Figures 3f–h); as the proximity to the coal gangue heap decreased, their contents decreased, indicating a lesser influence from surface runoff. Correlation analysis indicated a significant positive correlation among Cu, Zn, and Ni (P ≤ 0.01, r = 0.60–0.73 in the study area; P ≤ 0.01, r = 0.77–0.86 in the control area) (Figures 4a,b), suggesting these metals may share similar sources of pollution, excluding the coal heap. The spatial distribution of Cr was notably dissimilar to other HMs (Figure 3e), leading to the preliminary conclusion that Cr’s pollution sources differ from those of other HMs.
3.3 Vertical distribution of HMs contents in soil profiles
Figure 5 provides data on soil profiles, showing that in the study area, the contents of Cd, Hg, As, Pb, Cu, Zn, and Ni are higher in SS (0–20 cm) than in subsoil (20–60 cm) and substratum (60–100 cm). This pattern indicates that HM pollution in SSs is largely due to anthropogenic sources (Huang et al., 2017; Liang et al., 2021). Notably, in the study area, contents of Cd, Hg, As, and Pb were consistently higher across all soil layers than in the control area. Particularly near coal heaps (T1, T6, T8), elevated levels suggest migration from surface to deeper layers due to coal gangue accumulation, increasing their presence in subsurface soils (Luo et al., 2024). In contrast, the contents of Cu, Zn, and Ni were similar across soil layers in both the study and control zones, indicating that their contamination might be linked to anthropogenic sources other than coal heaps. Additionally, the uniform chemical composition of Cr across various depths suggests it predominantly originates from parent materials (Bini et al., 2011).
3.4 Driving factor analysis based on GDM
Figure 6 illustrates the results of factor test. In the study area, Cd, Hg, As, and Pb demonstrated the strongest explanatory power for variable X3 (distance from the coal gangue heap), with q-values of 0.524, 0.313, 0.712, and 0.442, respectively. This supports the spatial distribution findings, offering quantitative evidence that the coal gangue heap significantly influences these HMs. Conversely, Cr, Cu, Zn, and Ni had the strongest explanatory power for variable X5 (distance from residential zones), with q-values of 0.387, 0.422, 0.514, and 0.338, respectively. In the control area, Cd, As, Pb, Zn, and Ni showed the most significant influence for variable X4 (distance from the main road), with respective q-values of 0.363, 0.391, 0.387, 0.670, and 0.714; Hg, Cr, and Cu were most influenced by variable X5, with q-values of 0.311, 0.392, and 0.456. Prior research has shown that proximity to roads and residential zones often leads to increased human activities, such as road traffic emissions and agricultural practices, which in turn accumulate HMs in soil (Atafar et al., 2010; Cho and Newman, 2005; Zhang et al., 2012; Han et al., 2019). Therefore, it can be inferred that the contents of Cr, Cu, Zn, and Ni in the study area, as well as all HMs in the control area, were influenced by rural residents’ production and lifestyle.
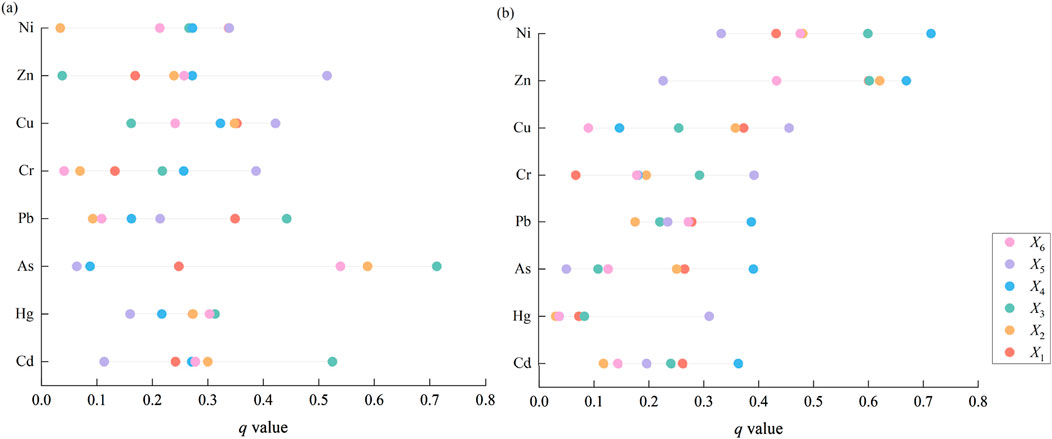
Figure 6. Explanatory power of X1∼X6 on influence of HMs in the study area (a) and control area (b).
3.5 Source apportionment of HMs in soil
The Kaiser-Meyer-Olkin (KMO) values in the study and control zones were 0.568 and 0.677, respectively, both above the threshold of 0.5, validating the appropriateness of conducting principal component analysis (PCA) (Supplementary Table S2) (Wang et al., 2020). The PCA, performed using the APCS-MLR receptor model, indicated strong predictive capabilities for HMs, with R2 values of 0.966, 0.903, 0.669, 0.779, 0.907, 0.830, 0.938, and 0.862 for Cd, Hg, As, Pb, Cr, Cu, Zn, and Ni in the study area; and 0.745, 0.672, 0.744, 0.731, 0.988, 0.623, 0.815, and 0.882 for the same metals in the control area (Duan et al., 2020).
Source apportionment outcomes illustrated in Figure 7 reveal that in the study area (Figures 7a,b), PC1, PC2, and PC3 accounted for 40.1%, 33.7%, and 26.2% of the variance, respectively. Predominantly, Cd, Hg, As, and Pb were influenced by PC1, with contributions of 78.8%, 62.9%, 60.8%, and 60.7%, respectively. The analysis of both surface and profile soil data suggests these elements are majorly affected by anthropogenic factors. Spatial analysis via GIS demonstrated an increase in the contents of these HMs near the coal gangue heap. Moreover, the quantitative analysis employing the GDM revealed that X3 (the distance from the sampling site to the coal gangue heap) provided the most significant explanation for the variation in these HMs. Research indicates that the redox reactions of pyrite in coal gangue lead to the release of HMs as sulfide compounds, which persistently migrate to the adjacent soil, propelled by surface runoff (Sun et al., 2021; Dong et al., 2024; Zhao et al., 2024). Thus, it is posited that PC1 is primarily derived from mining activities, significantly affecting the soil’s content of Cd, Hg, As, and Pb through the accumulation of coal gangue. Conversely, Cu, Zn, and Ni were mostly impacted by PC2, with respective contributions of 79.5%, 78.5%, and 50.6%. GDM analysis showed that X4 (distance to the main road) was most explanatory for these metals. The proximity to residential zones and thoroughfares elevates human activity, enhancing land use and potentially elevating soil contamination levels (Atafar et al., 2010; Cho and Newman, 2005; Zhang et al., 2012; Han et al., 2019). It is hypothesized that PC2 stems predominantly from vehicular emissions and the usage of fertilizers and pesticides. Cr, mainly affected by PC3 with a 69.4% contribution, exhibited the lowest CV in SSs, suggesting uniform levels across different soil depths (Yang et al., 2021; Delbari et al., 2019; Bini et al., 2011). These findings are corroborated by other studies, which attribute natural sources as the principal contributors to the Cr content in Chongqing’s SS (Ma et al., 2024a; Yao et al., 2024; Dong et al., 2018).
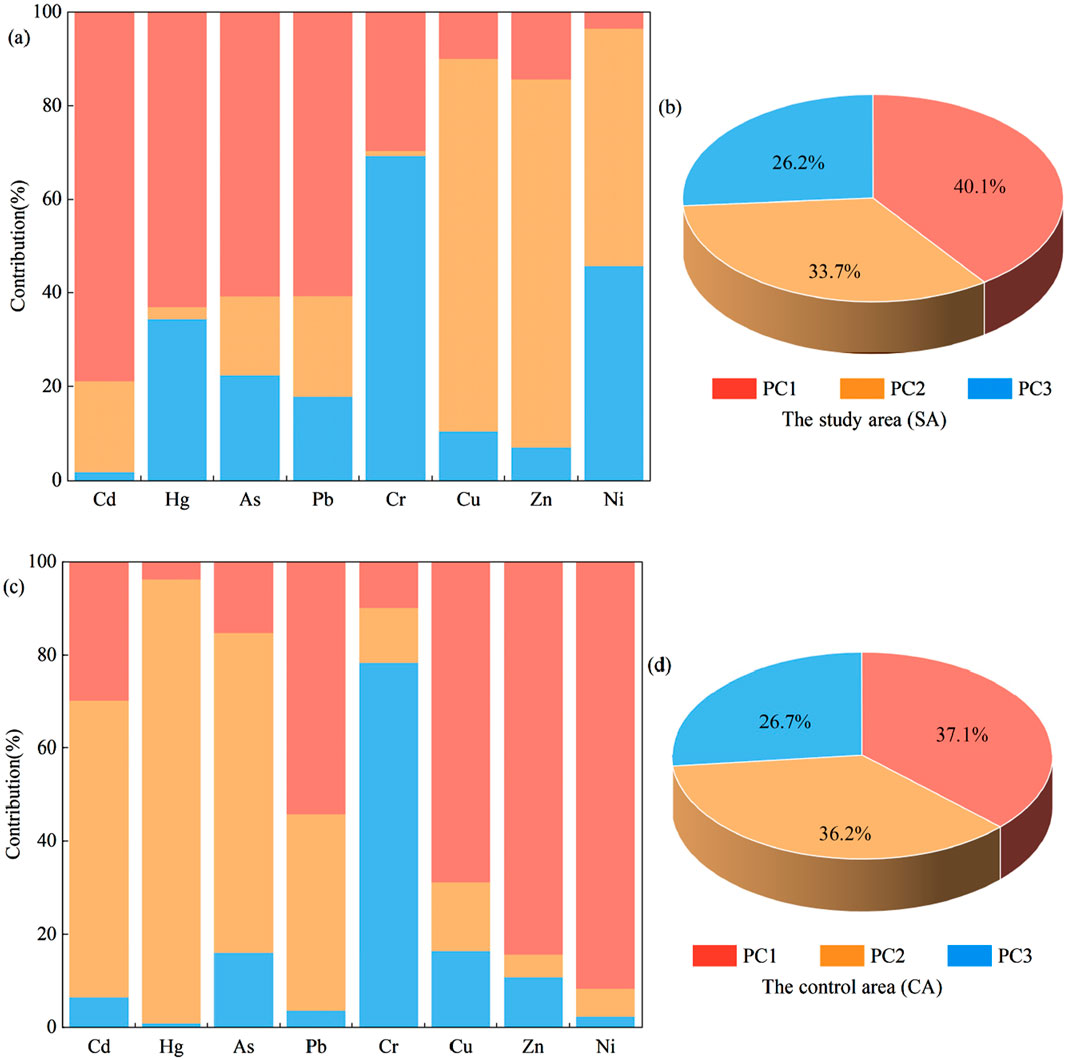
Figure 7. Contributions of sources calculated by APCS-MLR receptor model in the study area (a,b) and control area (c,d).
In the control area (Figures 7c,d), contributions from PC1, PC2, and PC3 were 37.1%, 36.2%, and 26.7%, respectively. Predominantly, Pb, Cu, Ni, and Zn were influenced by PC1, with contribution percentages of 54.1%, 68.7%, 84.3%, and 91.5%, respectively. Numerous studies have shown that elevated levels of these metals primarily arise from vehicular sources. For instance, significant Cu content in brake pads and their subsequent wear contribute to Cu release (Jeong et al., 2022); the inclusion of ZnO and ZnS in tires is a recognized source of Zn from tire wear (Jeong, 2022; Adamiec et al., 2016); historical use of leaded gasoline has notably led to Pb accumulation (Ye et al., 2022; Eichler et al., 2015); Ni, frequently included in tire and brake pad compositions, serves to bolster the resistance of these materials against plastic deformation (Jeong, 2022; Zhang P. et al., 2020). Given the strategic location near roads in the control area, it is theorized that PC1 mainly arises from road traffic emissions. Cd, Hg, and As, influenced by PC2 with contributions of 63.7%, 95.3%, and 68.4%, respectively, alongside Pb, significantly affected at 42.2%, underscore the impact of agricultural practices. Research shows Cd and Pb may precipitate as cadmium and lead phosphates in soils due to their presence in phosphate fertilizers (Jastrzębska et al., 2018; Dharma-wardana, 2018); similarly, phosphorus fertilizers contribute substantially to Hg presence in soils (Tang et al., 2018). Oyedele et al. (2006) noted significant rises in Cd, Pb, and Hg levels following superphosphate fertilizer application. Moreover, arsenic contents in soils are known to increase with the usage of arsenic-containing herbicides and pesticides (Atafar et al., 2010; Defarge et al., 2018). Field studies confirm the control area’s predominant agricultural land use, mainly employing nitrogen and phosphorus fertilizers, leading to the supposition that PC2 originates largely from fertilizer and pesticide application. Finally, Cr, chiefly influenced by PC3 with a 78.5% contribution, showed minimal variability across soil depths, reinforcing the notion that natural sources predominantly dictate Cr distribution, mirroring findings from the study area.
In summary, the soil in the study area was predominantly influenced by the ongoing accumulation of coal gangue (PC1), which accounted for 40.1% of the impact. Additionally, road traffic emissions, together with the use of fertilizers and pesticides (PC2), contributed 33.7%. Natural sources (PC3) also played a role, accounting for 26.2%. Conversely, in the control area, the primary influences were road traffic emissions and the use of fertilizers and pesticides (PC1 and PC2), contributing a combined 73.3%, followed by natural sources (PC3), which accounted for 26.7%.
3.6 Cd isotope
Figure 8a depicts the Cd isotopic composition relative to the inverse Cd content in soils and coal gangues. Previous research has demonstrated that weathering and surface runoff enhance the release of HMs from coal gangue, particularly those that are surface-weathered compared to unweathered samples (Guo et al., 2024; Li et al., 2021). Leaching from mine tailings into creeks causes heavier Cd isotopes to be preferentially transported into aqueous solutions, while lighter isotopes tend to remain in the solid phase (Imseng et al., 2018; Wang et al., 2023; Zhang et al., 2016). This study observed a similar trend in coal gangue samples, where δ114/110Cd values ranged from −0.208‰ to 0.024‰, with samples CG1 and CG2 (0–50 cm in depth) exhibiting higher values than CG3, CG4, and CG5. Furthermore, Cd isotopic ratios in soil samples from the study area varied from −0.038‰–0.316‰, indicating higher ratios than those in the control area. Notably, the content of heavy Cd isotopes in the SS decreases as the distance from the coal heap increases, as shown by the sequence: T1 (0.316‰) > T6 (0.117‰) > T8 (0.092‰) > T10 (-0.038‰). This suggests that heavy Cd isotopes from coal gangue migrate to the surrounding soil via surface runoff, enriching nearby soil with these isotopes. This aligns with findings by Yao et al. (2023). In contrast, Cd isotopic ratios in the control area’s soil samples were consistently lower, spanning −0.441‰ to −0.187‰. Research by Yan et al. (2021) has shown that both compound and phosphate fertilizers, as well as road traffic emissions, contain light Cd isotopes, with values of −0.43 ± 0.03‰, −0.15 ± 0.04‰, and −0.21 ± 0.03‰, respectively. Zhang X. W. et al. (2020) also indicated similar isotopic values in fertilizers and emissions, with −0.51 ± 0.06‰ and −0.40 ± 0.01‰, respectively, closely matching the range found in the control area soils. By integrating results from the APSC-MLR model and GDM, it is speculated that soil Cd in the control area is primarily affected by road traffic emissions and the application of fertilizers and pesticides.
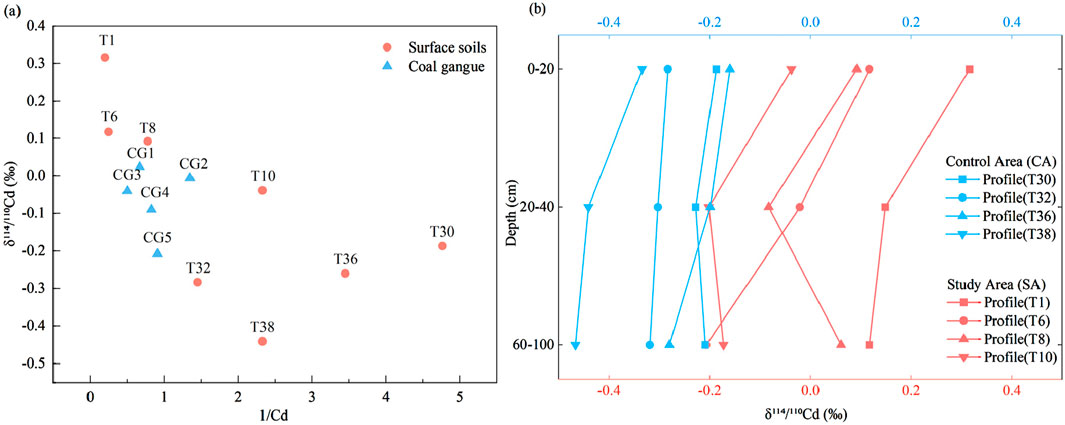
Figure 8. Cd isotopic composition versus the inverse Cd content (mg/kg) of soils (a) and coal gangues, Cd isotopic composition versus the depth of soils (b).
Figure 8b illustrates the isotopic composition of cadmium in relation to soil depth. In the research site, the mean δ114Cd/110Cd ratios for the surface, subsurface, and bottom soils were recorded at 0.122‰, −0.039‰, and −0.051‰, respectively. In the control area, the observed values were −0.241‰, −0.293‰, and −0.319‰, respectively, suggesting a decline in the δ114Cd/110Cd ratio as soil depth increased. This indicates that topsoil is more profoundly affected by human activities (Yao et al., 2023). The elevated ratios of Cd isotopes observed in each soil layer of the study area, in contrast to the control area, indicate that heavy Cd isotopes are likely migrating downward from the surface, possibly facilitated by rainwater infiltration (Gao et al., 2021). Consequently, the longstanding accumulation of coal gangue has significantly impacted the deep soil surrounding it.
4 Conclusion
In this study, we designated specific study and control zones, utilizing the APCS-MLR model, GDM, and Cd isotope fingerprints to ascertain the origins of HMs in the soil surrounding coal gangue heaps. The findings revealed that pollution levels were elevated in the study area compared to the control area, with Cd emerging as the primary contaminant. The integration of the GDM, APCS-MLR model, and Cd isotope fingerprints significantly improved the precision and dependability of our source apportionment efforts. In the study area, soil contamination was predominantly due to the persistent accumulation of coal gangue (PC1: Cd, Hg, As, and Pb), emissions from road traffic, and the usage of fertilizers and pesticides (PC2: Cu, Zn, and Ni), along with natural sources (PC3: Cr), contributing 40.1%, 33.7%, and 26.2% respectively. In contrast, the control area’s soil was mainly influenced by road traffic emissions and the application of fertilizers and pesticides (PC1 and PC2: Cd, Hg, As, Pb, Cu, Zn, and Ni), and by natural sources (PC3: Cr), with respective contribution rates of 73.3% and 26.7%. Surface runoff from coal gangue accumulation emerged as the dominant pathway for soil contamination in the study area. Thus, forthcoming research should prioritize effective coal gangue management strategies and address the ecological threats posed by elevated Cd levels in the study area.
Data availability statement
The raw data supporting the conclusions of this article will be made available by the authors, without undue reservation.
Author contributions
JM: Conceptualization, Formal Analysis, Investigation, Visualization, Writing – original draft, Writing – review and editing. YJ: Data curation, Investigation, Visualization, Writing – original draft. ZS: Data curation, Investigation, Visualization, Writing – original draft. BZ: Data curation, Investigation, Visualization, Writing – original draft. HZ: Conceptualization, Formal Analysis, Investigation, Writing – review and editing. XY: Conceptualization, Formal Analysis, Investigation, Writing – review and editing. XF: Investigation, Writing – original draft. DS: Investigation, Writing – original draft.
Funding
The author(s) declare that financial support was received for the research and/or publication of this article. This study was supported by Chongqing Science and Technology Bureau Project (CSTB2024JXJL-YFX0061) (CSTB2024JXJL-YFX0017), and National Natural Science Foundation of China Project (42077001).
Conflict of interest
Author ZS was employed by China Merchants Ecological Environmental Protection Technology Co., Ltd.
The remaining authors declare that the research was conducted in the absence of any commercial or financial relationships that could be construed as a potential conflict of interest.
Generative AI statement
The authors declare that no Generative AI was used in the creation of this manuscript.
Publisher’s note
All claims expressed in this article are solely those of the authors and do not necessarily represent those of their affiliated organizations, or those of the publisher, the editors and the reviewers. Any product that may be evaluated in this article, or claim that may be made by its manufacturer, is not guaranteed or endorsed by the publisher.
Supplementary material
The Supplementary Material for this article can be found online at: https://www.frontiersin.org/articles/10.3389/fenvs.2025.1582799/full#supplementary-material
References
Adamiec, E., Jarosz-Krzemińska, E., and Wieszała, R. (2016). Heavy metals from non-exhaust vehicle emissions in urban and motorway road dusts. Environ. Monit. Assess. 188, 369. doi:10.1007/s10661-016-5377-1
Ali, W., and Muhammad, S. (2023). Compositional data analysis of heavy metal contamination and eco-environmental risks in Himalayan agricultural soils, northern Pakistan. J. Geochem. Explor. 255, 107323. doi:10.1016/j.gexplo.2023.107323
Arora, N. K., and Chauhan, R. (2021). Heavy metal toxicity and sustainable interventions for their decontamination. Environ. Sustain 4, 1–3. doi:10.1007/s42398-021-00164-y
Atafar, Z., Mesdaghinia, A., Nouri, J., Homaee, M., Yunesian, M., Ahmadimoghaddam, M., et al. (2010). Effect of fertilizer application on soil heavy metal concentration. Environ. Monit. Assess. 160, 83–89. doi:10.1007/s10661-008-0659-x
Bini, C., Sartori, G., Wahsha, M., and Fontana, S. (2011). Background levels of trace elements and soil geochemistry at regional level in NE Italy. J. Geochem. Explor. 109 (1), 125–133. doi:10.1016/j.gexplo.2010.07.008
Chen, Y. Y., Zhao, J. Y., Chen, X., and Zheng, L. G. (2024). Analysis of the source apportionment and pathways of heavy metals in soil in a coal mining area based on machine learning and an APCS-MLR model. Minerals 14 (1), 54. doi:10.3390/min14010054
Cho, S. H., and Newman, D. H. (2005). Spatial analysis of rural land development. For. Policy. Econ. 7 (5), 732–744. doi:10.1016/j.forpol.2005.03.008
Cloquet, C., Carignan, J., Libourel, G., Sterckeman, T., and Perdrix, E. (2006). Tracing source pollution in soils using cadmium and lead isotopes. Environ. Sci. Technol. 40 (8), 2525–2530. doi:10.1021/es052232+
Defarge, N., Spiroux de Vendômois, J., and Séralini, G. E. (2018). Toxicity of formulants and heavy metals in glyphosate-based herbicides and other pesticides. Toxicol. Rep. 5, 156–163. doi:10.1016/j.toxrep.2017.12.025
Delbari, M., Afrasiab, P., Gharabaghi, B., Amiri, M., and Salehian, A. (2019). Spatial variability analysis and mapping of soil physical and chemical attributes in a salt-affected soil. Arab. J. Geosci. 12 (3), 68. doi:10.1007/s12517-018-4207-x
Dharma-wardana, M. W. C. (2018). Fertilizer usage and cadmium in soils, crops and food. Environ. Geochem Hlth 40, 2739–2759. doi:10.1007/s10653-018-0140-x
Din, I. U., Muhammad, S., Faisal, S., Rehman, I. U., and Ali, W. (2024). Heavy metal(loid)s contamination and ecotoxicological hazards in coal, dust, and soil adjacent to coal mining operations, Northwest Pakistan. J. Geochem. Explor. 256, 107332. doi:10.1016/j.gexplo.2023.107332
Din, I. U., Muhammad, S., and Rehman, I. U. (2023). Heavy metal(loid)s contaminations in soils of Pakistan: a review for the evaluation of human and ecological risks assessment and spatial distribution. Environ. Geochem. Health. 45, 1991–2012. doi:10.1007/s10653-022-01312-x
Dong, J. H., Li, J. B., Huang, Y., Zhong, J. Y., Dun, K., Wu, M., et al. (2024). Understanding the release, migration, and risk of heavy metals in coal gangue: an approach by combining experimental and computational investigations. J. Hazard Mater 461, 132707. doi:10.1016/j.jhazmat.2023.132707
Dong, R. Z., Jia, Z. M., and Li, S. Y. (2018). Risk assessment and sources identification of soil heavy metals in a typical county of Chongqing Municipality, Southwest China. Process Saf. Environ. 113, 275–281. doi:10.1016/j.psep.2017.10.021
Dong, S. W., Pan, Y. C., Guo, H., Gao, B. B., and Li, M. M. (2021). Identifying influencing factors of agricultural soil heavy metals using a geographical detector: a case study in Shunyi district, China. Land 10 (10), 1010. doi:10.3390/land10101010
Duan, Y. X., Zhang, Y. M., Li, S., Fang, Q. L., Miao, F. F., and Lin, Q. G. (2020). An integrated method of health risk assessment based on spatial interpolation and source apportionment. J. Clean. Prod. 276, 123218. doi:10.1016/j.jclepro.2020.123218
Eichler, A., Gramlich, G., Kellerhals, T., Tobler, L., and Schwikowski, M. (2015). Pb pollution from leaded gasoline in South America in the context of a 2000-year metallurgical history. Sci. Adv. 1, e1400196. doi:10.1126/sciadv.1400196
Gao, S., Zhao, G. H., Guo, L. H., Zhou, L. Q., and Yuan, K. K. (2021). Utilization of coal gangue as coarse aggregates in structural concrete. Constr. Build. Mater 268, 121212. doi:10.1016/j.conbuildmat.2020.121212
Ge, H., Feng, Y., Li, Y., Yang, W. L., and Gong, N. (2016). Heavy metal pollution diagnosis and ecological risk assessment of the surrounding soils of coal waste pile at Naluo coal mine, Liupanshui, Guizhou. Int. J. Min. Reclam. Env. 30 (4), 312–318. doi:10.1080/17480930.2015.1050840
Gong, C., Xia, X., Lan, M., Shi, Y. C., Lu, H. C., Wang, S. X., et al. (2024). Source identification and driving factor apportionment for soil potentially toxic elements via combining APCS-MLR, UNMIX, PMF and GDM. Sci. Rep. 14 (1), 10918. doi:10.1038/s41598-024-58673-9
Guo, G. H., Li, K., Zhang, D. G., and Lei, M. (2022). Quantitative source apportionment and associated driving factor identification for soil potential toxicity elements via combining receptor models, SOM, and geo-detector method. Sci. Total Environ. 830, 154721. doi:10.1016/j.scitotenv.2022.154721
Guo, Y. W., Li, X. D., Li, Q. Z., and Hu, Z. Q. (2024). Environmental impact assessment of acidic coal gangue leaching solution on groundwater: a coal gangue pile in Shanxi, China. Environ. Geochem. Hlth 46, 120. doi:10.1007/s10653-024-01861-3
Han, Z. Y., Ye, C. W., Zhang, Y., Dan, Z., Zou, Z. Y., Liu, D., et al. (2019). Characteristics and management modes of domestic waste in rural areas of developing countries: a case study of China. Environ. Sci. Pollut. Res. 26, 8485–8501. doi:10.1007/s11356-019-04289-w
Huang, B., Li, Z. W., Li, D. Q., Yuan, Z. J., Chen, Z. L., and Huang, J. Q. (2017). Distribution characteristics of heavy metal(loid)s in aggregates of different size fractions along contaminated paddy soil profile. Environ. Sci. Pollut. R. 24 (30), 23939–23952. doi:10.1007/s11356-017-0012-4
Huang, Y., Deng, M. H., Wu, S. F., Japenga, J., Li, T. Q., Yang, X., et al. (2018). A modified receptor model for source apportionment of heavy metal pollution in soil. J. Hazard Mater 354, 161–169. doi:10.1016/j.jhazmat.2018.05.006
Imseng, M., Wiggenhauser, M., Keller, A., Müller, M., Rehkämper, M., Murphy, K., et al. (2018). Fate of cd in agricultural soils: a stable isotope approach to anthropogenic impact, soil formation, and soil-plant cycling. Environ. Sci. Technol. 52 (4), 1919–1928. doi:10.1021/acs.est.7b05439
Jastrzębska, M., Saeid, A., Kostrzewska, M., and Baśladyńska, S. (2018). New phosphorus biofertilizers from renewable raw materials in the aspect of cadmium and lead contents in soil and plants: P-biofertilizers from waste and Cd and Pb in soil and plant. Open Chem. 16, 35–49. doi:10.1515/chem-2018-0004
Jeong, H. (2022). Toxic metal concentrations and Cu–Zn–Pb isotopic compositions in tires. J. Anal. Sci. Technol. 13, 2. doi:10.1186/s40543-021-00312-3
Jeong, H., Ryu, J. S., and Ra, K. (2022). Characteristics of potentially toxic elements and multi-isotope signatures (Cu, Zn, Pb) in non-exhaust traffic emission sources. Environ. Pollut. 292, 118339. doi:10.1016/j.envpol.2021.118339
Li, C., Zheng, L. G., Jiang, C. I., Chen, X., and Ding, S. S. (2021). Characteristics of leaching of heavy metals from low-sulfur coal gangue under different conditions. Int. J. Coal Sci. Techn. 8, 780–789. doi:10.1007/s40789-021-00416-6
Li, K. J., Gu, Y. S., Li, M. Z., Zhao, L., Ding, J. J., Lun, Z. J., et al. (2016). Spatial analysis, source identification and risk assessment of heavy metals in a coal mining area in Henan, Central China. Int. Biodeter. Biodegr. 128, 148–154. doi:10.1016/j.ibiod.2017.03.026
Liang, B., Ye, Q. T., and Shi, Z. Q. (2024). Stable isotopic signature of cadmium in tracing the source, fate, and translocation of cadmium in soil: a review. J. Hazard Mater 472, 134531. doi:10.1016/j.jhazmat.2024.134531
Liang, X. Y., Wang, C., Song, Z. C., Yang, S. C., Bi, X. Y., Li, Z. G., et al. (2021). Soil metal(loid)s pollution around a lead/zinc smelter and source apportionment using isotope fingerprints and receptor models. Appl. Geochem. 135, 105118. doi:10.1016/j.apgeochem.2021.105118
Liu, X., and Song, G. (2024). Development status of coal industries in the worlds major coal-producing countries. J. S. Afr. Inst. Min. Metall. 124, 383–386. doi:10.17159/2411-9717/1504/2024
Luo, Y., Wang, Z., Zhang, Y. D., Zhang, J. Q., Zeng, Q. P., Zhang, Z. L., et al. (2024). Vertical migration behavior simulation and prediction of Pb and Cd in co-contaminated soil around Pb-Zn smelting slag site. J. Hazard Mater 469, 133990. doi:10.1016/j.jhazmat.2024.133990
Lv, J. S. (2019). Multivariate receptor models and robust geostatistics to estimate source apportionment of heavy metals in soils. Environ. Pollut. 244, 72–83. doi:10.1016/j.envpol.2018.09.147
Ma, J., Chu, L. J., Sun, J., Wang, S. L., Ge, M., and Deng, L. (2024a). Health risk assessment of trace metal(loid)s in agricultural soils based on Monte Carlo simulation coupled with positive matrix factorization model in Chongqing, southwest China. J. Mt. Sci. 21 (1), 100–112. doi:10.1007/s11629-023-8304-2
Ma, J., Shen, Z. J., Jiang, Y., Liu, P., Sun, J., Li, M. S., et al. (2024b). Potential ecological risk assessment for trace metal(loid)s in soil surrounding coal gangue heaps based on source-oriented. Sci. Total Environ. 954, 176465. doi:10.1016/j.scitotenv.2024.176465
Ma, J., Shen, Z. J., Wang, S. L., Deng, L., Sun, J., Liu, P., et al. (2023). Source apportionment of heavy metals in soils around a coal gangue heap with the APCS-MLR and PMF receptor models in Chongqing, southwest China. J. Mt. Sci. 20 (04), 1061–1073. doi:10.1007/s11629-022-7819-2
Ma, J. W., Lanwang, K. N., Liao, S. Y., Zhong, B., Chen, Z. H., Ye, Z. Q., et al. (2023). Source apportionment and model applicability of heavy metal pollution in farmland soil based on three receptor models. Toxics 11 (3), 265. doi:10.3390/toxics11030265
MEE, (1997). Soil quality-determination of lead, cadmium-graphite furnace atomic absorption spectrophotometry. (In Chinese)
MEE, (2015). Soil and sediment-determination of inorganic element-wavelength dispersive X-ray fluorescence spectrometry. (In Chinese)
MEE and SAMR, (2018). Soil environmental quality - risk control standard for soil contamination of agricultural land. (In Chinese)
Muhammad, S., Zeb, A., Shaik, M. R., and Assal, M. E. (2024). Spatial distribution of potentially toxic elements pollution and ecotoxicological risk of sediments in the high-altitude lakes ecosystem. Phys. Chem. Earth. 135, 103655. doi:10.1016/j.pce.2024.103655
Oyedele, D. J., Asonugho, C., and Awotoye, O. O. (2006). Heavy metals in soil and accumulation by edible vegetables after phosphate fertilizer application. Electron. J. Environ. Agric. Food Chem. 5 (4), 1446–1453.
SAMR and NSA, (2008). Soil quality-analysis of total mercury, arsenic and lead contents in soils-Atomic fluorescence pectrometryPart 1: analysis of total mercury contents in soils. (In Chinese)
Shahbaz, M., Farhani, S., and Ozturk, I. (2015). Do coal consumption and industrial development increase environmental degradation in China and India? Environ. Sci. Pollut. R. 22, 3895–3907. doi:10.1007/s11356-014-3613-1
Shi, B., Meng, J., Wang, T. Y., Li, Q. Q., Zhang, Q. F., and Su, G. J. (2024). The main strategies for soil pollution apportionment: a review of the numerical methods. J. Environ. Sci. 136, 95–109. doi:10.1016/j.jes.2022.09.027
Shi, H. Y., Wang, P., Zheng, J. T., Deng, Y. R., Zhuang, C. W., Huang, F., et al. (2023). A comprehensive framework for identifying contributing factors of soil trace metal pollution using Geodetector and spatial bivariate analysis. Sci. Total Environ. 857, 159636. doi:10.1016/j.scitotenv.2022.159636
Shi, T. Z., Hu, Z. W., Shi, Z., Guo, L., Chen, Y. Y., Li, Q. Q., et al. (2018). Geo-detection of factors controlling spatial patterns of heavy metals in urban topsoil using multisource data. Sci. Total Environ. 643, 451–459. doi:10.1016/j.scitotenv.2018.06.224
Song, S. J., Peng, R. S., Wang, Y., Cheng, X., Niu, R. L., and Ruan, H. (2023). Spatial distribution characteristics and risk assessment of soil heavy metal pollution around typical coal gangue hill located in Fengfeng Mining area. Environ. Geochem. Hlth 45 (10), 7215–7236. doi:10.1007/s10653-023-01530-x
Sun, Y. Q., Xiao, K., Wang, X. D., Lv, Z. H., and Mao, M. (2021). Evaluating the distribution and potential ecological risks of heavy metal in coal gangue. Environ. Sci. Pollut. R. 28 (15), 18604–18615. doi:10.1007/s11356-020-11055-w
Tang, Z. Y., Fan, F. L., Wang, X. Y., Shi, X. J., Deng, S. P., and Wang, D. Y. (2018). Mercury in rice (Oryza sativa L.) and rice-paddy soils under long-term fertilizer and organic amendment. Ecotox. Environ. Safe 150, 116–122. doi:10.1016/j.ecoenv.2017.12.021
Thurston, G. D., and Spengler, J. D. (1985). A quantitative assessment of source contributions to inhalable particulate matter pollution in metropolitan Boston. Atmos. Environ. 19 (1), 9–25. doi:10.1016/0004-6981(85)90132-5
Tiwari, V., Garg, A., Kapshe, M., Deshpande, A., and Vishwanathan, S. (2023). Assessing possibilities for coal continuance in India under climate constraints. Int. J. Greenh. Gas. Con. 122, 103811. doi:10.1016/j.ijggc.2022.103811
Tokatlı, C., Ustaoğlu, F., Muhammad, S., Yüksel, B., Gülbaşı, A., Özmen, İ., et al. (2025). Spatial-temporal variations of inorganic contaminants and associated risks for sediment of Felent stream basin flowing along with silver mines in the midwestern Türkiye. Soil Sediment. Contam., 1–18. doi:10.1080/15320383.2025.2464153
Wang, C., Xu, G., Sun, C. L., Xu, J., Xu, K., Jiang, L., et al. (2024). Modeling and forecasting of coal price based on influencing factors and time series. J. Clean. Prod. 467, 143030. doi:10.1016/j.jclepro.2024.143030
Wang, J. F., and Hu, Y. (2012). Environmental health risk detection with GeogDetector. Environ. Modell. Softw. 33, 114–115. doi:10.1016/j.envsoft.2012.01.015
Wang, L. W., Guo, J. M., Tsang, D. C. W., and Hou, D. Y. (2023). Cadmium isotope fractionation during sorption to soil minerals: lab evidence and field implication. Chem. Geol. 634, 121607. doi:10.1016/j.chemgeo.2023.121607
Wang, L. W., Jin, Y. L., Weiss, D. J., Schleicher, N. J., Wilcke, W., Wu, L. H., et al. (2021). Possible application of stable isotope compositions for the identification of metal sources in soil. J. Hazard Mater 407, 124812. doi:10.1016/j.jhazmat.2020.124812
Wang, S., Cai, L. M., Wen, H. H., Luo, J., Wang, Q. S., and Liu, X. (2019). Spatial distribution and source apportionment of heavy metals in soil from a typical county-level city of Guangdong Province, China. Sci. Total Environ. 655, 92–101. doi:10.1016/j.scitotenv.2018.11.244
Wang, S. B., and Wang, X. (2018). Potentially useful elements (Al, Fe, Ga, Ge, U) in coal gangue: a case study in Weibei coal mining area, Shaanxi Province, northwestern China. Environ. Sci. Pollut. R. 25 (12), 11893–11904. doi:10.1007/s11356-018-1476-6
Wang, Y. Z., Duan, X. J., and Wang, L. (2020). Spatial distribution and source analysis of heavy metals in soils influenced by industrial enterprise distribution: case study in Jiangsu Province. Sci. Total Environ. 710, 134953. doi:10.1016/j.scitotenv.2019.134953
Wilding, L. P. (1985). “Spatial variability: its documentation, accommodation and implication to soil survey,” in Soil spatial variability, 166–194.
Xia, Y. F., Gao, T., Liu, Y. H., Qi, M., Zhu, J. M., Tong, H., et al. (2025). Cd/Pb behavior during combustion in a coal-fired power plant and their spatiotemporal impacts on soils: new insights from Cd/Pb isotopes. J. Environ. Sci. 150, 582–593. doi:10.1016/j.jes.2024.03.002
Xiao, L., Guan, D. S., Chen, Y. J., Dai, J., Ding, W. H., Peart, M. R., et al. (2019). Distribution and availability of heavy metals in soils near electroplating factories. Environ. Sci. Pollut. R. 26 (22), 22596–22610. doi:10.1007/s11356-019-04706-0
Yan, Y., Sun, Q. Q., Yang, J. J., Zhang, X. W., and Guo, B. L. (2021). Source attributions of Cadmium contamination in rice grains by Cadmium isotope composition analysis: a field study. Ecotox Environ. Safe 210, 111865. doi:10.1016/j.ecoenv.2020.111865
Yang, S. Y., David, T., Yang, D., He, M. J., Liu, X. M., and Xu, J. M. (2021). A synthesis framework using machine learning and spatial bivariate analysis to identify drivers and hotspots of heavy metal pollution of agricultural soils. Environ. Pollut. 287, 117611. doi:10.1016/j.envpol.2021.117611
Yao, C., Shen, Z. J., Wang, Y. M., Mei, N., Li, C. X., Liu, Y. J., et al. (2023). Tracing and quantifying the source of heavy metals in agricultural soils in a coal gangue stacking area: insights from isotope fingerprints and receptor models. Sci. Total Environ. 863, 160882. doi:10.1016/j.scitotenv.2022.160882
Yao, C., Yang, Y. D., Li, C. X., Shen, Z. J., Li, J. Q., Mei, N., et al. (2024). Heavy metal pollution in agricultural soils from surrounding industries with low emissions: assessing contamination levels and sources. Sci. Total Environ. 917, 170610. doi:10.1016/j.scitotenv.2024.170610
Ye, J. X., Li, J. J., Wang, P. C., Ning, Y. Q., Liu, J. L., Yu, Q. Q., et al. (2022). Inputs and sources of Pb and other metals in urban area in the post leaded gasoline era. Environ. Pollut. 306, 119389. doi:10.1016/j.envpol.2022.119389
Yüksel, B., and Ustaoğlu, F. (2025). Pollution analysis of metals in the sediments of lagoon lakes in Türkiye: toxicological risk assessment and source insights. Process. Saf. Environ. 193, 665–682. doi:10.1016/j.psep.2024.11.085
Zeng, J. Q., Ke, W. S., Deng, M., Tan, J. Q., Li, C. X., Cheng, Y. Z., et al. (2023). A practical method for identifying key factors in the distribution and formation of heavy metal pollution at a smelting site. J. Environ. Sci. 127, 552–563. doi:10.1016/j.jes.2022.06.026
Zhang, F., Yan, X. D., Zeng, C., Zhang, M., Shrestha, S., Devkota, L. P., et al. (2012). Influence of traffic activity on heavy metal concentrations of roadside farmland soil in mountainous areas. Int. J. Environ. Res. Public Health 9 (5), 1715–1731. doi:10.3390/ijerph9051715
Zhang, M. C., Cheng, L. S., Yue, Z. H., Peng, L. H., and Xiao, L. (2024). Assessment of heavy metal(oid) pollution and related health risks in agricultural soils surrounding a coal gangue dump from an abandoned coal mine in Chongqing, Southwest China. Sci. Rep. 14 (1), 18667. doi:10.1038/s41598-024-69072-5
Zhang, P., Zhang, L., Wei, D. B., and Qu, X. H. (2020). Effect of matrix alloying on braking performance of copper-based brake pad under continuous emergency braking. J. Tribol-T Asme 142 (8), 081703. doi:10.1115/1.4046624
Zhang, X. W., Yan, Y., Wadood, S. A., Sun, Q. Q., and Guo, B. L. (2020). Source apportionment of cadmium pollution in agricultural soil based on cadmium isotope ratio analysis. Appl. Geochem 123, 104776. doi:10.1016/j.apgeochem.2020.104776
Zhang, Y. X., Wen, H. J., Zhu, C. W., Fan, H. F., Luo, C. G., Liu, J., et al. (2016). Cd isotope fractionation during simulated and natural weathering. Environ. Pollut. 216, 9–17. doi:10.1016/j.envpol.2016.04.060
Zhang, Y. Y., Xu, L., Sridhar, S., Liu, L. L., Wang, X. D., and Zhang, Z. T. (2015). Effects of chemistry and mineral on structural evolution and chemical reactivity of coal gangue during calcination: towards efficient utilization. Mater Struct. 48 (9), 2779–2793. doi:10.1617/s11527-014-0353-0
Zhao, R., Wu, X., Zhu, G., Zhang, X., Liu, F., and Mu, W. P. (2024). Revealing the release and migration mechanism of heavy metals in typical carbonate tailings, East China. J. Hazard Mater 464, 132978. doi:10.1016/j.jhazmat.2023.132978
Zhao, Y. H., Liu, L., Kang, S. Z., Ao, Y., Han, L., and Ma, C. Q. (2021). Quantitative analysis of factors influencing spatial distribution of soil erosion based on Geo-Detector model under diverse geomorphological types. Land 10 (6), 604. doi:10.3390/land10060604
Zhong, Q. H., Qi, J. Y., Liu, J., Wang, J., Lin, K., Ouyang, Q., et al. (2022). Thallium isotopic compositions as tracers in environmental studies: a review. Environ. Int. 162, 107148. doi:10.1016/j.envint.2022.107148
Keywords: APCS-MLR, coal gangue, geo-detector, heavy metal(loid)s, isotope, soil, source
Citation: Ma J, Jiang Y, Shen Z, Zhang B, Zhao H, Yang X, Feng X and Sun D (2025) A perspective on heavy metal(loid)s traceability in soil surrounding coal gangue areas: combined application of multiple source analysis methods. Front. Environ. Sci. 13:1582799. doi: 10.3389/fenvs.2025.1582799
Received: 25 February 2025; Accepted: 15 April 2025;
Published: 30 April 2025.
Edited by:
Pavol Midula, Czech University of Life Sciences Prague, CzechiaReviewed by:
Said Muhammad, University of Peshawar, PakistanBayram Yuksel, Giresun University, Türkiye
Copyright © 2025 Ma, Jiang, Shen, Zhang, Zhao, Yang, Feng and Sun. This is an open-access article distributed under the terms of the Creative Commons Attribution License (CC BY). The use, distribution or reproduction in other forums is permitted, provided the original author(s) and the copyright owner(s) are credited and that the original publication in this journal is cited, in accordance with accepted academic practice. No use, distribution or reproduction is permitted which does not comply with these terms.
*Correspondence: Hongjing Zhao, emp6aGpAMTYzLmNvbQ==; Xiaoxia Yang, eXh4aHdqQGxpdmUuY24=