- 1Department of Geography, University of Zurich, Zurich, Switzerland
- 2Sustainable Agroecosystems Group, Department of Environmental Systems Science, ETH Zürich, Zurich, Switzerland
- 3Swiss Federal Institute for Forest, Snow and Landscape Research (WSL), Birmensdorf, Switzerland
An improved identification of the environmental variables that can be used to predict the content of soil organic carbon (SOC) stored belowground is required to reduce uncertainties in estimating the response of the largest terrestrial carbon reservoir to environmental change. Recent studies indicate that some metal cations can have an active role in the stabilization of SOC, primarily by coordinating the interaction between soil minerals and organic matter through cation bridging and by creating complexes with organic molecules when their hydration shells are displaced. The effective cation exchange capacity (CEC eff.) is a measure that integrates information about available soil surfaces to which metal cations are retained. Therefore, we critically tested the relationship between CEC eff. and SOC content using regression analyses for more than 1000 forest sites across Switzerland, spanning a unique gradient of mean annual precipitation (640–2500 mm), elevation (277–2207 m a.s.l), pH (2.8–8.1) and covering different geologies and vegetation types. Within these sites, SOC content is significantly related to CEC eff., in both topsoils and subsoils. Our results demonstrate that, on a pH-class average, in Swiss forest topsoils (<30 cm depth) there is a strong confounding effect of soil organic matter contributing between 35 and 50% to the total CEC eff. In subsoils, soil organic matter has a negligible contribution to CEC eff., and the variation of CEC eff. is associated to the presence of inorganic surfaces such as clay content as well as iron- and aluminum- oxides and hydroxides. At pH > 5.5, between 59 and 83% of subsoil CEC eff. originates from exchangeable calcium, whereas in acidic soils exchangeable aluminum contributes between 21 and 44% of the CEC eff. Exchangeable iron contributes to less than 1% of the variability in CEC eff. Overall this study indicates that in Swiss forests subsoils, CEC eff. strongly reflects the surface of soil minerals to which SOC can be bound by metal cations. The strength of the relationship between CEC eff. and SOC content depends on the pH of the soil, with the highest amount of variation of SOC content explained by CEC eff. in subsoils with pH > 5.5.
Introduction
Our predictions on the feedback between the terrestrial carbon (C) cycle and climate change are still uncertain, largely due to the difficulty in accurately quantifying the stabilization of soil organic C (SOC) (Jobbágy and Jackson, 2000; Todd-Brown et al., 2013; Friedlingstein et al., 2014; Jackson et al., 2017). Measuring and predicting mechanisms of SOC preservation is particularly challenging due to the long-time periods that are needed to detect changes, as well as the complexity of the soil physicochemical properties which effectively stabilize SOC (van der Voort et al., 2016; Harden et al., 2018). Due to these reasons, offsetting the diversity of mechanisms that drive soil C stabilization and adopting variables which aggregate information about multiple soil characteristics into one, has been proven useful (Bailey et al., 2018).
Climatic variables exert a major control on SOC content and its turnover, likely causing a significant feedback of the terrestrial C cycle to climate change (Chen et al., 2013; Carvalhais et al., 2014). In addition, the biotic activity of plants and microbial communities, as well as soil physicochemical properties, control the fate of SOC dynamics (Torn et al., 1997; Schmidt et al., 2011; Doetterl et al., 2015; Rasmussen et al., 2018). A soil property which is often used to determine variations in SOC stability and to model SOC turnover is the percentage of clay (e.g., Coleman and Jenkinson, 1996; Wieder et al., 2015). In principle, the content of clay controls the stabilization of SOC by promoting the sorption of organic molecules to mineral surfaces <2 μm in size, as well as their occlusion in aggregates (Oades, 1988; Eusterhues et al., 2003; Lützow et al., 2006). Finely textured soils are generally assumed to contain higher amounts of protected SOC as compared to more coarsely textured soils (Rasmussen et al., 2018). Although the use of clay content is widely applicable to large-scale investigations due to its ease of measurement, emerging understanding has indicated that it may oversimplify and not effectively capture SOC preservation mechanisms (Bailey et al., 2018; Rasmussen et al., 2018). Clay merely represents a size class of soil minerals whose surfaces partially interact with SOC (Kaiser and Guggenberger, 2000; Vogel et al., 2014). Moreover, clay content poorly informs about the surface area of soils available for the sorption of organic matter (Farrar and Coleman, 1967). Consequently, other soil properties which represent sorptive processes allowing organic matter to be preserved might represent SOC stabilization better than the amount of clay (Bailey et al., 2018).
An example of an inherent soil property which represents sorptive capacities of soil surfaces is the effective cation exchange capacity (CEC eff.). The CEC eff. represents the total amount of exchangeable cations that a soil can adsorb to its surfaces at the actual pH of the soil. Positively charged ions (cations) such as Na+, K+, Mg2+, Ca2+, Mn2+, Al3, Fe2+, H+ are generally adsorbed on negatively charged soil surfaces. At low pH it is mainly the permanent charges of the 2:1 type clays that adsorb exchangeable cations. With increasing soil pH, the positively charged ions are increasingly adsorbed to surfaces with a variable charge such as 1:1-type clays, allophane, soil organic matter and Fe- and Al- oxides (Weil and Brady, 2016). The pH of the soil specifically controls the amount of negative charges on soil surfaces with a variable charge: either through protonation or deprotonation of H+ from hydroxyl-groups on soil surfaces.
Although soil organic matter surfaces act as loci for cation exchange, and can lead to a correlation between SOC and CEC eff., there are two main reasons why CEC eff. may be causally linked to SOC preservation. First, CEC eff. is strongly associated to the available surface area of the soil (Farrar and Coleman, 1967), and thereby effectively reflects the reactive soil surfaces to which SOC may be adsorbed. With the analytical techniques that are available today, it is not possible to quantify soil surface area for large sample sets. On the other hand, the cation exchange capacity of soils is routinely measured to assess soil fertility for agricultural and forested land and is thus reported in large-scale databases (e.g., the Harmonized World Soil Database). Second, CEC eff. may represent a direct measure of SOC sorption to soil surfaces at the existing pH conditions. Conceptual advances highlighted that polyvalent metal cations such as Al3+ and Ca2+ can play a significant role in the stabilization of SOC by binding organic compounds to mineral surfaces through exchangeable bridges and ionic bonds (Oades, 1988; Rasmussen et al., 2018; Rowley et al., 2018). It has been suggested that the strength of metal cations in binding organic compounds largely depends on the size of the hydration shell and the valence of the cations. For instance, chemical modeling shows that bridges with exchangeable Ca2+ are more stable than bridges with exchangeable monovalent cations because the charge to hydration radius ratio of Ca2+ allows to balance-out the repulsion between negatively charged soil surfaces (Sutton et al., 2005; Iskrenova-Tchoukova et al., 2010). The complexation of organic molecules by metal cations present on mineral surfaces through ionic bonds is mainly related to the displacement of the hydration shell of the cations, the cations ionic potential, as well as types of organic molecules present in the soil solution at the local environmental conditions (Rowley et al., 2018). In acidic soils, Al3+ has been observed to play a relevant role in coordinating the complexation between organic molecules and mineral surfaces. Fe3+ normally forms insoluble precipitates in most environmentally relevant pH conditions and is rarely found as free ion in soils. Ca2+ is weakly polarizable and tends to form ionic bonds with O-containing ligands such as carboxylic acids, and therefore it has the ability to form complexes with organic substrates (Sposito, 2008). The monovalent cation Na+ does not form ionic bonds with organic ligands, and K+ only participates in these complexations in interlayers of certain phyllosilicates (Rowley et al., 2018). Additionally, metal cations can form organo-metal complexes by bridging multiple organic compounds together, which has been observed to cause aggregation and thereby physical protection of soil organic matter (Kunhi Mouvenchery et al., 2012).
Here we used linear regression analyses to assess the relationship between CEC eff. and SOC content for a set of more than 1000 forest soil profiles across Switzerland spanning a strong gradient of climate, elevation, pH, geology and vegetation. We first of all determined the confounding effect of organic surfaces on CEC eff. and detected soils where organic matter does not significantly contribute to CEC eff. We then explored which type of soil surfaces and which cations reflect the variation of CEC eff. and thereby may influence SOC content. In soils where soil organic matter contributes negligibly to CEC eff., we additionally assessed how the variance of SOC content was explained by CEC eff. in comparison to other environmental variables.
Materials and Methods
Study Area and Dataset
The study area covers the complete country of Switzerland (N: 45° 51′ 40.50–47° 42′ 38.07, E: 6° 07′ 31.19–10° 31′ 35.37), situated in the center of Europe. Switzerland offers a wide and intricate range of geology and topography which vary abruptly often within short distances (Walthert et al., 2013; Gosheva et al., 2017). About 30% of the country (∼12.000 km2) is covered by forest, and half of this area is located above 1000 m a.s.l. Forest management is mainly practiced at low elevations, where no large-scale clear-cutting is applied and natural regeneration is often fostered by silvicultural management (Brassel and Brändli, 1999). Forest soil fertilization and liming has always been forbidden in Switzerland.
The dataset used in this study originates from a database of the Swiss Federal Institute for Forest, Snow and Landscape Research (WSL) containing data on 1204 forest sites across Switzerland (for more details see Walthert et al., 2013; and Walthert and Meier, 2017). More than 80% of these sites have been covered for at least 150 years with forests (Gosheva et al., 2017). The parent material of Northern Switzerland is dominated by calcareous bedrocks and sediments. In Southern Switzerland crystalline bedrocks prevail and in the central Alpine regions calcareous and crystalline bedrocks form a complex pattern. Most acidic soils are found in parts of the Swiss plateau and in the central and Southern Alps of Switzerland (Figure 1). The predominant soil types are classified as Cambisols (n = 365), Luvisols (n = 127), and Gleysols (n = 89). The soil variables which we evaluated included SOC content (g kg–1) and other soil physicochemical properties such as pH in CaCl2, CEC eff. (mmolc kg–1), oxalate and dithionite extractable aluminum and iron (mg kg–1), and soil texture (%). In this study we report SOC content rather than SOC stocks. This is because SOC content represents a direct measure of SOC after a single correction for the amount of fine earth in pedogenetic horizons, while SOC stock estimates would require multiple imponderable corrections (Gosheva et al., 2017).
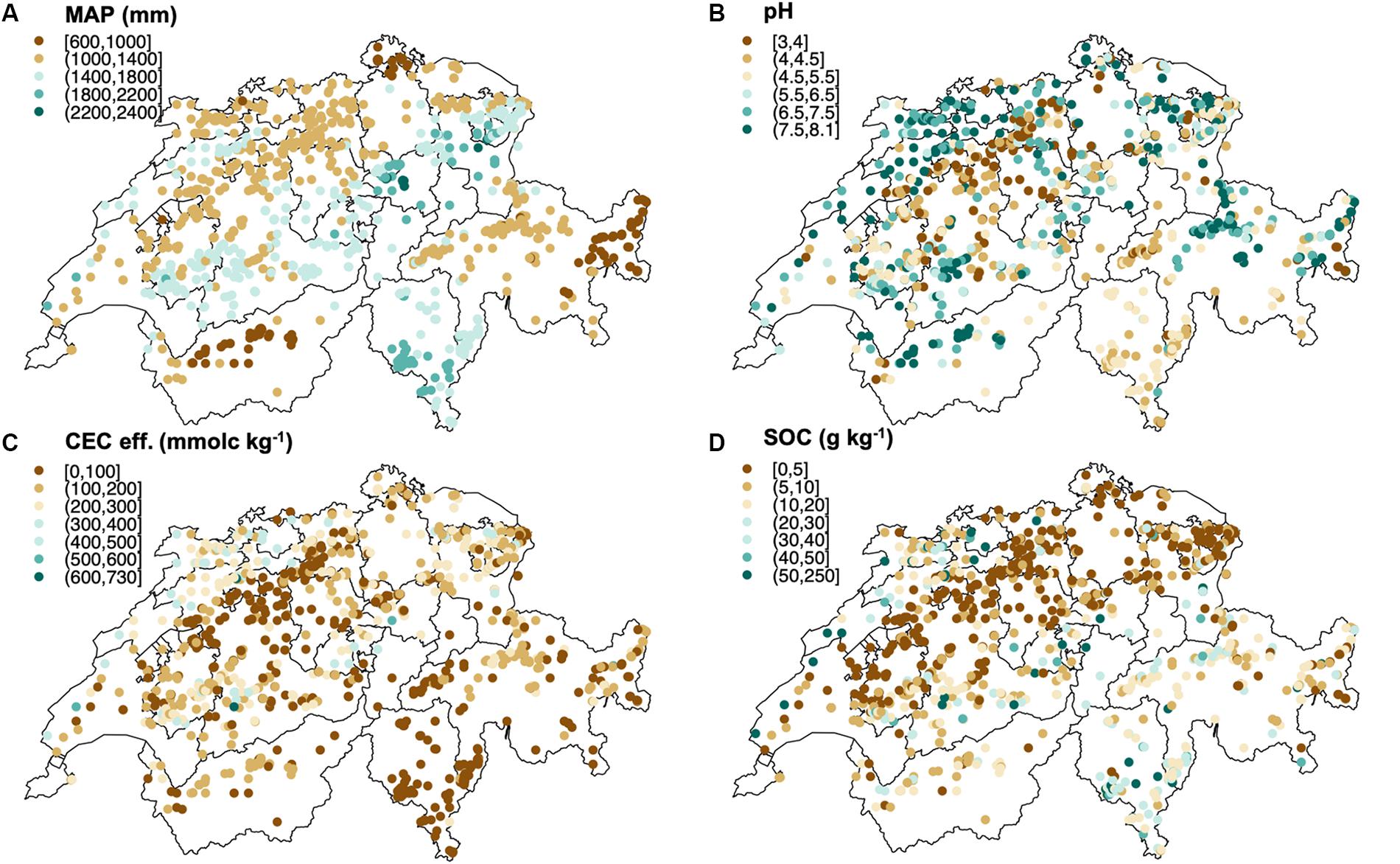
Figure 1. Spatial distribution of (A) mean annual precipitation (MAP), (B) subsoil (30–120 cm depth) pH, (C) subsoil effective cation exchange capacity (CEC eff.), (D) subsoil soil organic carbon (SOC) in the Swiss forest sites analyzed in this study. Maps were built using R packages (“sp,” “lattice,” “RColorBrewer,” “proj4, rworldmap,” “raster,” “rgdal”).
The soil data of each forest site was paired with climatic, vegetation and topographic data. Climatic data was based on the Swiss meteorological network (MeteoSwiss), combined with suitable interpolation algorithms (Walthert et al., 2013). Specifically, mean annual precipitation (MAP) and mean annual temperature (MAT) were provided by Meteotest1 for the period 1981–2010 (for details see Remund et al., 2014). For each forest site, the local Leaf Area Index (LAI) was further estimated according to Schleppi et al. (2011). These estimates were based on data from a vegetation surveys during the vegetation period (May–September) using the Braun-Blanquet cover abundance scale (Braun-Blanquet, 1964; Mueller-Dombois and Ellenberg, 1974). The LAI is a common measure of canopy foliage and indicator of primary production (Asner et al., 2003). No other vegetation variable related to primary production was available. The altitude of the forest sites was extracted from a 25 m digital elevation model (DEM) of the Federal Geo-Information centre swisstopo.
Soil Chemical and Physical Properties
At each forest site a soil profile was sampled by pedogenetic horizons down to a depth of 120 cm if possible, otherwise down to parent rock, with six samples per pit on average. Soil samples were dried at 40–60°C and sieved at 2 mm for chemical analyses. SOC content was measured in milled subsamples by dry combustion using a C/N analyzer (NC 2500, Carlo Erba Instruments, Milan, Italy). Inorganic C was removed in samples with a pH above 6.0 by fumigating with HCl vapor prior to analysis (Walthert et al., 2010). Soil pH was measured potentiometrically in 0.01 M CaCl2 with a soil - extract ratio of 1:2 after 30 min of equilibration. Exchangeable cations were extracted in an unbuffered solution of 1 M NH4Cl for 1 h on an end-over-end shaker using a soil - extract ratio of 1:10. The elemental concentrations were subsequently measured with an ICP-AES (Optima 3000, Perkin–Elmer, Waltham, MA, United States). For soil samples with a pH (CaCl2) < 6.5 concentrations of exchangeable protons were calculated as the difference between the total and the Al-induced exchangeable acidity with the KCl method (Thomas, 1982). For soil samples with a higher pH, concentrations of exchangeable protons were assumed to be negligible. The effective cation exchange capacity (CEC eff.) was finally calculated by summing the charge equivalents of exchangeable Na, K, Mg, Ca, Mn, Al, Fe, and H. Grain size distribution was measured with the sedimentation method according to Gee and Bauder (1986) for 750 soil profiles, to determine the relative amounts of clay, silt and sand. For the remaining soil profiles, we used the field estimates based on ten texture classes from Walthert et al. (2004). Our analysis focused on the following soil-depth intervals: the upper 0–30 cm of the mineral soil (topsoil), the lower 30–120 cm of the mineral soil (subsoil) and the complete 0–120 cm of the mineral soil. For the 0–30, 30–120, and 0–120 soil-depth intervals we calculated the mean content of all the above-mentioned soil properties. This was done by considering the proportional contribution of each of the pedogenetic horizons within those fixed-depth intervals, as described in Walthert et al. (2013) by accounting for the proportional amounts of fine earth <2 mm.
For a subset of soil profiles (n = 238), iron and aluminum oxides and hydroxides were additionally measured by oxalate and dithionite extraction in all of the mineral soil horizons within a soil profile. Oxalate extractable aluminum and iron (Alo and Feo) were obtained by extracting one gram of soil sample with 50 ml of 0.2 M ammonium oxalate and shaking for 4 h in the dark (Leoppert and Inskeep, 1996). Dithionite extractable iron (Fed) was determined according to Holmgren (1967). Two grams of soil were mixed with two grams of Na–dithionite and extracted in a mixture of 10 ml 1 M sodium bicarbonate and 150 ml 0.3 M tri-Na citrate-dihydrate for 24 h on an end over end shaker. The suspensions were filtered using Whatman No. 40 filter paper and the Fe- and Al-concentrations were measured by atomic absorption spectrometry (Philips PU 9200, Eindhoven, Netherlands). The extraction of soils with oxalate dissolves aluminum and iron from more amorphous oxides and hydroxides (e.g., allophane, imogolite, ferrihydrite and organo-metal complexes), while the extraction of soils with dithionite additionally dissolves crystalline iron form (e.g., goethite and hematite) (Parfitt and Childs, 1988; Herold et al., 2014).
Regression Analyses
In this study we used regression analyses to assess the relationship between CEC eff. and SOC content.
Assessment of the Confounding Effect of Organic Surfaces on CEC eff.
Since the variable negative charges on soil organic matter surfaces may contribute significantly to CEC eff., especially alkaline and surface soils, we quantified how much of the CEC eff. is contributed by soil organic matter and by soil minerals in the clay fraction. To separate the contribution of organic matter and inorganic components to CEC eff. of soils, statistical approaches have been previously advantageously adopted over the selective removal of each component by chemical treatment (Wright and Foss, 1972; Klamt and Sombroek, 1986). The reason being that chemical treatment can cause interference on the derived charges due to pH alterations (Salazar et al., 2002). Therefore, we used partial regression coefficient values and the content of soil organic matter, clay and CEC eff. to distinguish how much of the CEC eff. of topsoils and subsoils is driven by soil organic matter and clay minerals. The following equation was adapted from Klamt and Sombroek (1986):
where [X2] is the amount of soil organic matter (where SOC is used as a proxy of soil organic matter), [X1] is the amount of clay in soil. The fitted coefficient a is the CEC eff. of 1 unit of clay in soil, whereas b is the CEC of 1 unit of soil organic matter in soil. The contribution of soil organic matter to soil CEC eff. is then:
and the contribution of clay to the total CEC eff. of soils is then the remaining difference from 100 percent. For this assessment, data was grouped into nine consecutive pH ranges: (pH: <4, 4–4.5, 4.5–5, 5–5.5, 5.5–6, 6–6.5, 6.5–7, 7–7.5, and >7.5).
Variation of CEC eff. in Relation to Soil Inorganic and Organic Surfaces
We used linear mixed effect models to test how the variation of CEC eff. is related to different soil inorganic and organic surfaces, such as clay minerals <2 μm in size (here measured as clay content), pedogenic oxides and hydroxides (here measured as dithionite extractable iron, oxalate extractable aluminum and iron) and soil organic matter (SOC used as a proxy of soil organic matter). This analysis was performed on the subset of 238 soil profiles for which iron and aluminum oxides and hydroxides were measured in each individual soil horizon. To address the non-independent nature of multiple horizons within one soil profile for this subset of data, linear mixed effect models were chosen for this statistical approach as in Rasmussen et al. (2018). We conducted three separate linear models, one considering the entire depth range of the soil profiles, one for topsoils <30 cm and one for subsoils >30 cm depth. Soil profile identity was used as a random effect, allowing both the slope and intercept to vary with soil depth. Thus, we considered that the interactions between CEC eff. and soil inorganic and organic surfaces may vary with soil depth and pH. For the linear model considering the entire depth range of a soil profile, CEC eff. was set as dependent variable and soil depth as well as all the other soil properties as fixed effects. The linear models for topsoils and subsoils were performed with CEC eff. set as dependent variable and pH as well as all the other soil properties as fixed effects.
Assessment of the Relative Contribution of Exchangeable Cations to CEC eff.
Hierarchical variance partitioning based on linear models was used to assess the relative contribution of each exchangeable cation to CEC eff. across pH classes. Specifically, soil-pH data was grouped into nine consecutive pH ranges: (pH: <4, 4–4.5, 4.5–5, 5–5.5, 5.5–6, 6–6.5, 6.5–7, 7–7.5, and >7.5). Exchangeable cations were treated as explanatory variables while CEC eff. was treated as the main response variable. This analysis was only assessed for soils where organic matter contributed negligibly to the overall CEC eff., therefore all topsoils were excluded and all subsoils were included in this assessment.
Contribution of CEC in Explaining the Variance SOC Content
We also adopted hierarchical variance partitioning based on linear models to determine how much of the variance in SOC content is explained by cation exchange capacity, as compared to that of other edaphic, climatic and vegetation variables in soils with pH <5.5 and >5.5. CEC eff., percentage of clay, LAI, MAT, and MAP were treated as explanatory variables while SOC content was treated as main response variable. This analysis was only assessed for soils where organic matter contributed negligibly to the overall CEC eff., excluding all topsoils.
Statistical Analyses and Model Development
To meet the normality assumptions of the applied statistical tests and standardize the variation among variables, we transformed the continuous variables to normal distributions applying log-transformations when required, and subsequently standardized them to a mean of 0 and standard deviation of 1. The linear models were checked for multicollinearity using the variance inflation factor (VIF). The value of VIF > 4 was set as a threshold for evidence of multicollinearity. We checked the model assumptions using the diagnostic plot functions in R (Crawley, 2012), and the normality of the residuals was tested with histograms.
For the hierarchical variance partitioning analyses, we used the“lmg” metric in the R package “relaimpo” (Groemping and Matthias, 2006), which decomposes the R squares of previously built linear models into non-negative contributions that automatically sum to the total R2 of the linear model and takes care of the dependence on orderings by averaging over orderings (Grömping, 2006). The contribution of the explanatory variables was normalized to 100%, i.e., instead of the total R2 (Groemping and Matthias, 2006). All analyses were performed with the R statistical software version 3.5.0 (R Core Team, 2018), and the packages “vegan,” “MASS,” “dplyr,” “plyr,” “car,” “quantPsyc,” “caret,” “relaimpo,” “Psych.”
Results
Environmental Characteristics of the Forest Sites
The Swiss forest sites from which the soils were sampled are distributed between 277 and 2207 m a.s.l. They are characterized by a mean annual precipitation (MAP) ranging between 636 and 2484 mm, and mean annual temperatures (MAT) ranging between 0.1 and 12.0°C. MAT and altitude are strongly negatively correlated (r = −0.97). About half of the sites are covered by broadleaf tree species while the rest are coniferous forests (Gosheva et al., 2017), with LAI values varying between 2 to 7.2. pH values range from 2.8 to 8.1 and the percentage of clay varies between 0.8 and 75.6% (for details about specific ranges in topsoils and in the subsoils see Table 1). The content of SOC varies between 6 and 377 g kg–1 in topsoils (upper 0–30 cm of the mineral soil) and between 0 (below detection limit) and 229 g kg–1 in subsoils (30–120 cm depth). CEC eff. ranges between 20.4 and 1046 mmolc kg–1 in topsoils and between 6.2 and 728 mmolc kg–1 in subsoils. Oxalate extractable aluminum and iron range between 78.9 and 37100 mg kg–1, 63.3 and 42410 mg kg–1 respectively. Dithionite extractable iron varies between 272.4 and 53200 mg kg–1.
Relationship Between CEC eff. and SOC Content
Soil organic carbon content is significantly related to CEC eff. in topsoils and subsoils (p-value < 0.0001), with the strongest positive relationship observed in topsoils with pH > 5.5 (R2 = 0.64) followed by subsoils with pH > 5.5 (R2 = 0.20). In soils with more acidic pH the relationship is weaker (Figure 2).
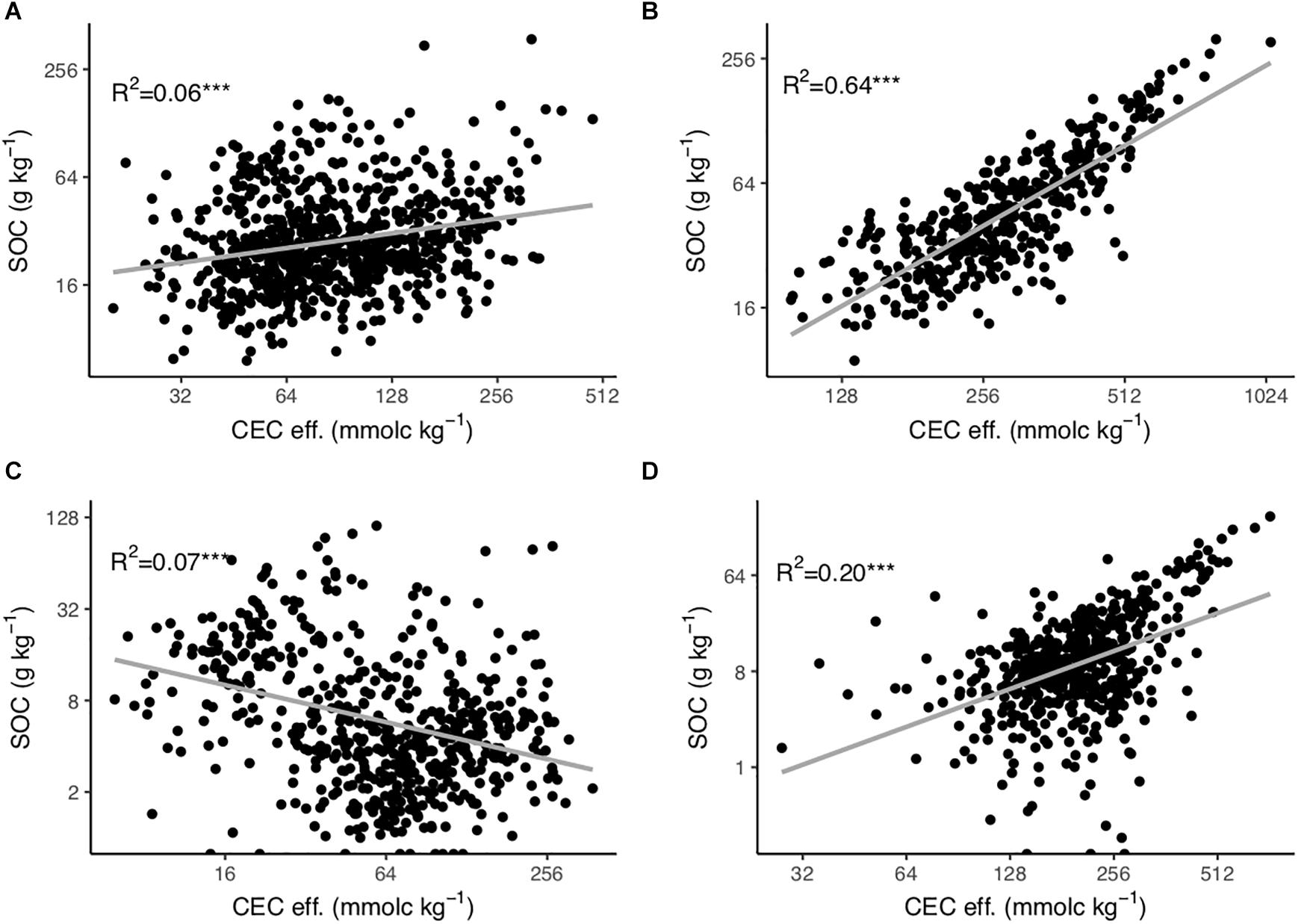
Figure 2. Relationship between effective cation exchange capacity (CEC eff.) and soil organic carbon (SOC) content in (A) topsoils (0–30 cm depth) with soil pH < 5.5, (B) topsoils with soil pH > 5.5, (C) subsoils (30–120 cm depth) with soil pH < 5.5 and (D) subsoils with soil pH > 5.5. The axes are log-log scaled. ***p-value < 0.001.
Confounding Effect of Soil Organic Matter on CEC eff. in Topsoils but Not in Subsoils
The partial regression analysis following the approach by Klamt and Sombroek (1986) indicated that soil organic matter contributes, on average for the pH classes assessed in this study, between 35 and 50% to the total CEC eff. in topsoils (0–30 cm depth) (Figure 3). In subsoils (30–120 cm depth), soil organic matter contributes to CEC eff. to a much lower extent: between 0 and 11% in soils with pH below 5.5 and between 2 and 34% for soils with higher pH (Figure 3).
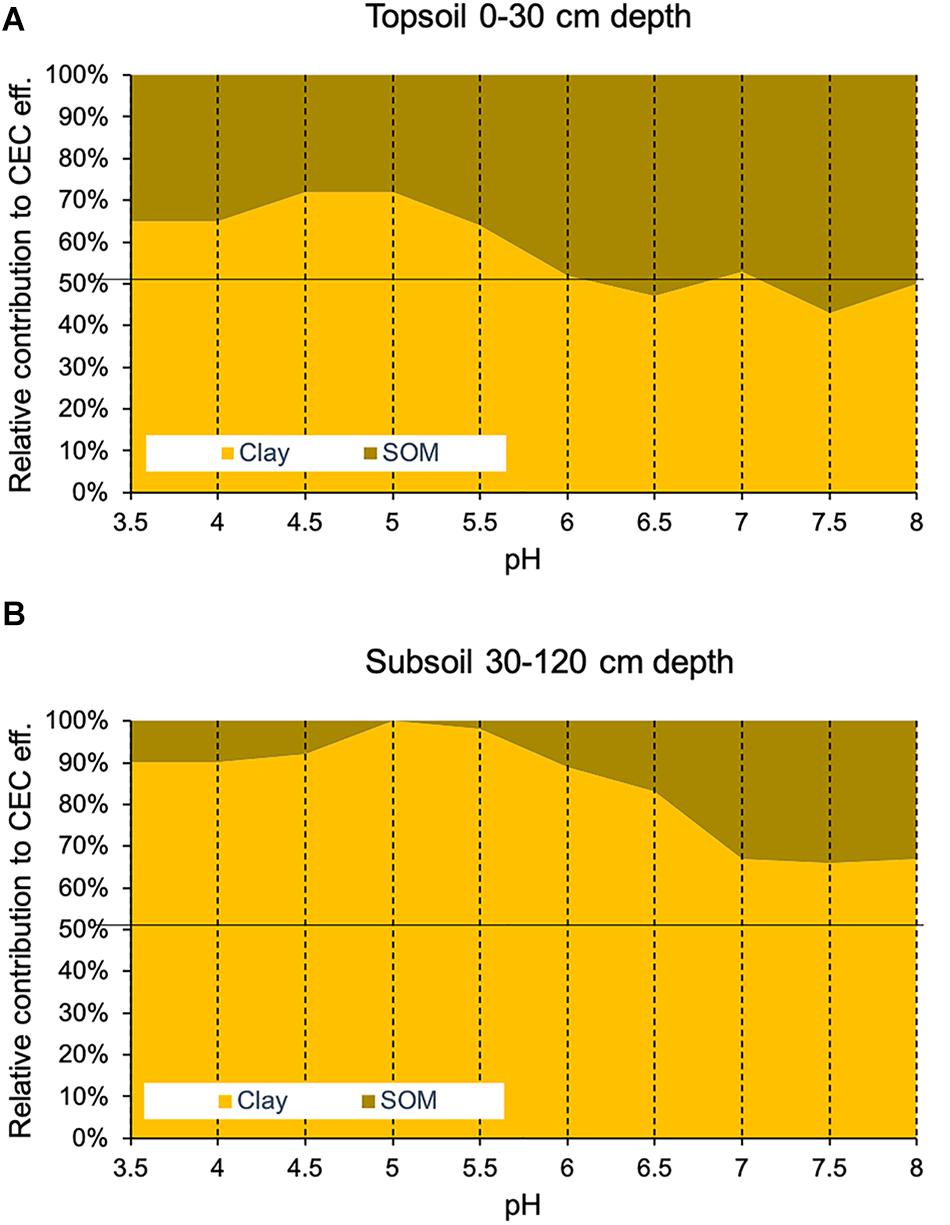
Figure 3. Relative contribution of soil organic matter (SOM) and the percentage of clay to the effective cation exchange capacity across pH classes of (A) Swiss forest topsoils (0–30 cm depth) and (B) subsoils (30–120 cm depth). The contribution of SOM and clay was estimated using partial regression coefficient values as well as the content of SOM, clay and CEC eff.
Relationship Between the Variance of CEC eff. and Soil Properties
Corroborating the outcome of our partial regression analysis, the results of the linear mixed effect model run to test the interactions between CEC eff. and soil surfaces across complete vertical soil profiles, showed a significant influence of the interaction between soil organic matter and soil depth on CEC eff. (p-value < 0.01, Table 2A). The separation between topsoils (<30 cm depth) and subsoils further indicated that the variance of CEC eff. is significantly related to alterations in soil organic matter in topsoils (p-value < 0.0001, Table 2B) but not in subsoils (p-value n.s., Table 2C). Due to the major confounding effect and significant influence of soil organic matter on CEC eff. in topsoils (<30 cm depth), this paper predominantly focuses on presenting and discussing the relationship between CEC eff. and SOC content in subsoils.
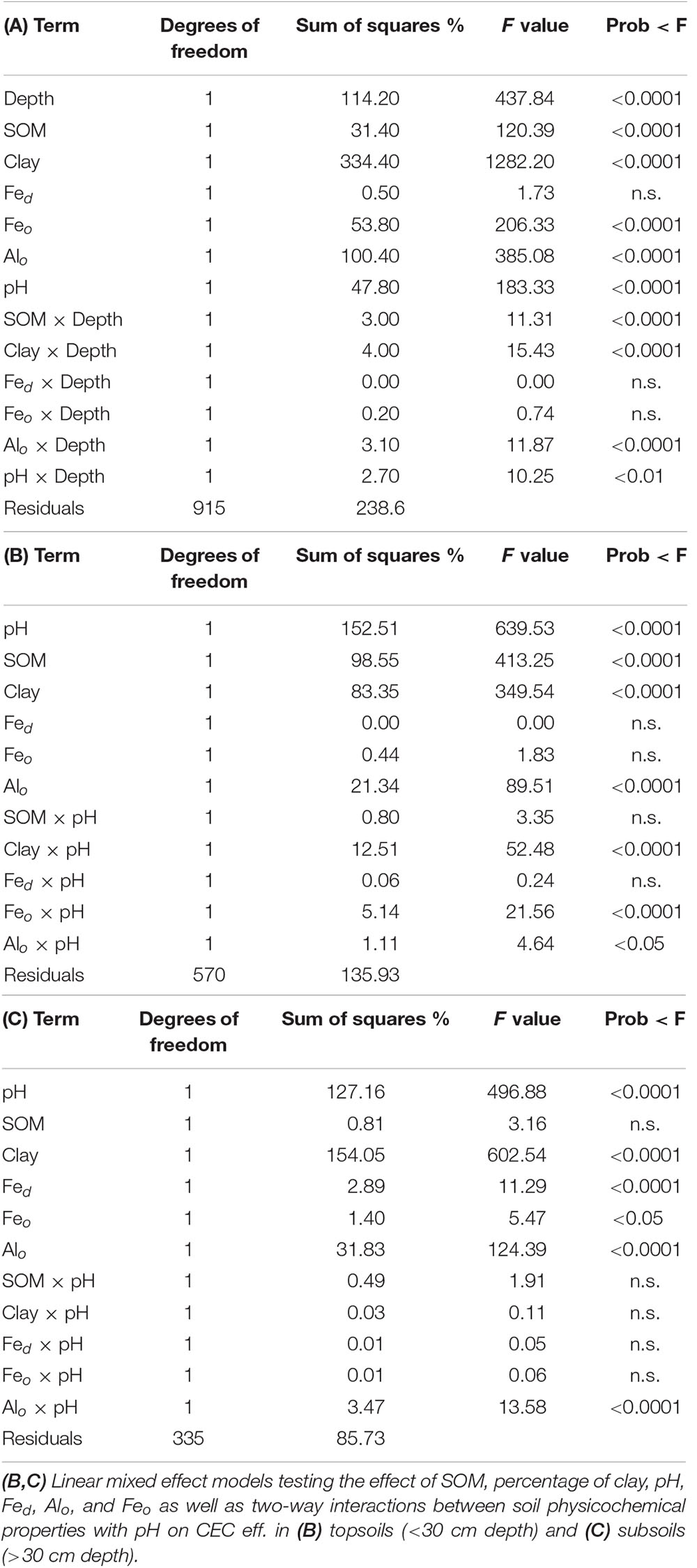
Table 2. (A) Linear mixed effect model testing the effect of soil depth, soil organic matter (SOM, as a proxy of SOC), percentage of clay, pH, dithionite extractable iron (Fed), oxalate extractable aluminum and iron (Alo and Feo), as well as two-way interactions between soil physicochemical properties with depth on the effective cation exchange capacity (CEC eff.) across complete soil profiles.
According to our analysis in subsoils, the variance of CEC eff. is in addition to soil pH predominantly related to changes in clay content, oxalate extractable aluminum, dithionite extractable iron and to a smaller extent to oxalate extractable iron (Table 2C). The interactions of pH with clay content, iron oxides and hydroxides and soil organic matter are not statistically significant (p-value n.s., Table 2C). Instead, the interaction between oxalate extractable aluminum and pH meaningfully contributes to the variance of CEC eff. (p-value < 0.0001, Table 2C). The distributions of clay content, oxalate extractable aluminum, SOC, and CEC eff. in subsoils (>30 cm depth) are presented in Figure 4, where it is possible to observe that the lowest values of CEC eff. are present in acidic soils.
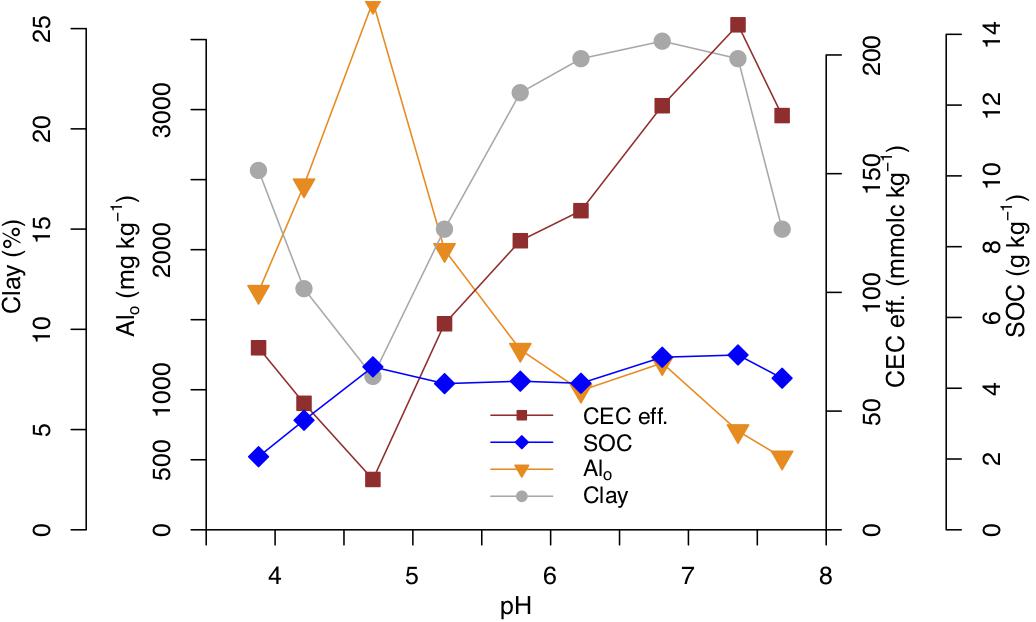
Figure 4. Median values of effective cation exchange capacity (CEC eff., brown squares), soil organic carbon (SOC, blue diamonds), oxalate extractable Al (Alo, orange triangles) and clay content (gray circles), across pH classes in subsoils (>30 cm depth) of Swiss forests.
Contribution of Different Cations to CEC eff. in Subsoils
In subsoils, 59 to 83% of the CEC eff. originates, on average for the assessed pH classes, from exchangeable Ca at pH levels higher or equal to 5.5 (Figure 5). Instead, in acidic soils with pH < 5 exchangeable Al contributes between 21 and 44% of the CEC eff. Exchangeable Fe contributes to less than 1% of the variability in CEC eff.
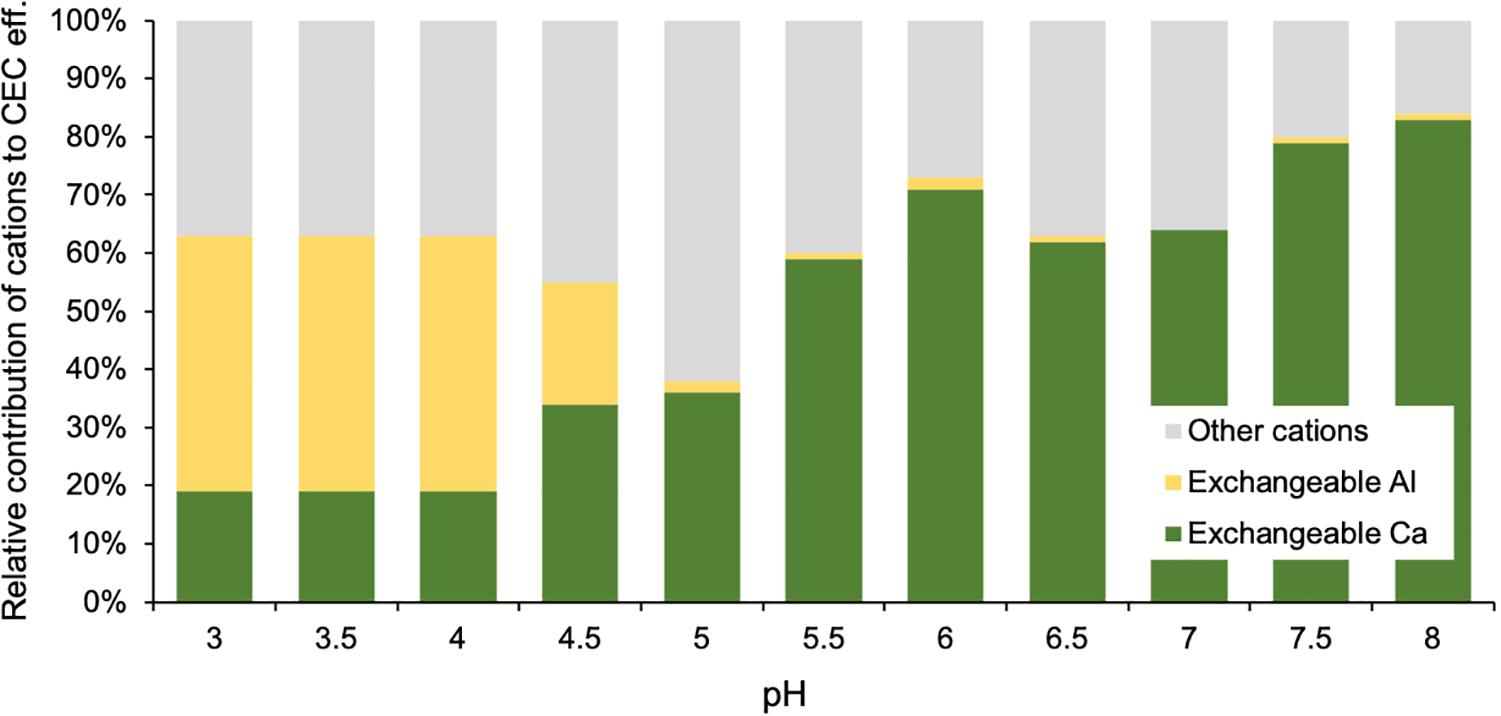
Figure 5. Relative contribution of exchangeable Ca, Al and other cations (Na+, K+, Mg2+, Mn2+, Fe2+, H+) to the effective cation exchange capacity (CEC eff.) across different pH classes in subsoils (30–120 cm depth) of Swiss forests.
Variance of Subsoil SOC Content Explained by CEC eff. in Comparison to Other Environmental Variables
The results of the linear model analysis aimed at analyzing how the variance of subsoil SOC (30–120 cm depth) is influenced by different environmental variables showed that CEC eff. explains the greatest amount of variance of SOC content in soils with a pH > 5.5 (63% of total R2), followed by LAI (13% of total R2) and MAT (12% of total R2) (Table 3). In more acidic soils, MAP explains the highest amount of variation of SOC content (50% of total R2), followed by MAT (25% of total R2) and CEC (13% of total R2) (Table 3). In comparison to CEC eff., clay content explains a smaller amount of variation in SOC content (7% of total R2 at pH ≤ 5.5, and 10% of total R2 at pH > 5.5).
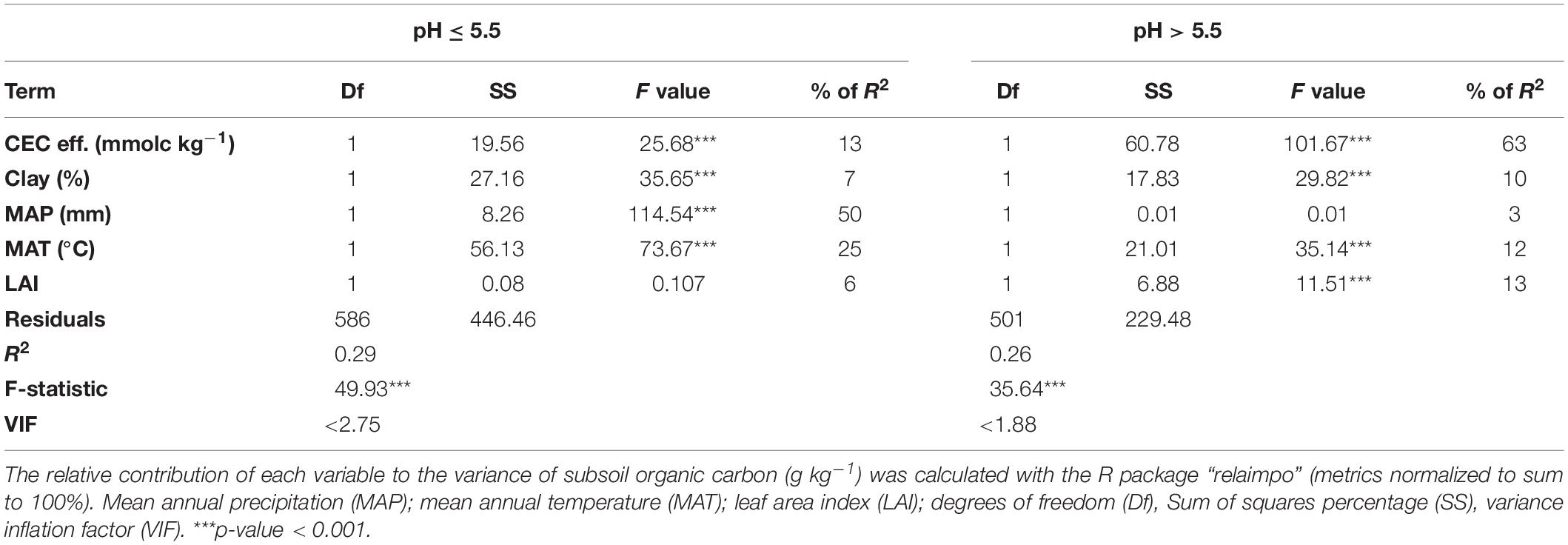
Table 3. Results of the linear model used to analyze how the variance of subsoil organic carbon (30–120 cm depth) is influenced by the effective cation exchange capacity (CEC eff.) and other environmental variables.
Discussion
Our analysis indicated that throughout 1204 forest sites in Switzerland, the negative charges of soil organic matter contribute substantially (35 to 50%, on average for the pH classes assessed in this study) to CEC eff. in topsoils (<30 cm depth), but negligibly in subsoils (Figure 3). In the deeper soil (>30 cm depth) the variance of CEC eff. was rather strongly associated with clay content, dithionite extractable iron, oxalate extractable aluminum, and to a smaller extent oxalate extractable iron (Table 2C). The negative charges on clay minerals and pedogenic oxides and hydroxides adsorb oppositely-charged metal cations (Weil and Brady, 2016), which may thereby bind SOC through exchangeable bridges and ionic bonds (Oades, 1988; Rasmussen et al., 2018; Rowley et al., 2018).
We observed that the contribution of different exchangeable cations to subsoil CEC eff. is strongly influenced by the pH of the soil. In acidic soils exchangeable Al contributes to 21 to 44% of the CEC eff. (on pH-class average), while at pH levels higher than 5.5, between 59 and 83% of the CEC eff. originates from exchangeable Ca (Figure 5). Although many geologic substrates in Switzerland contain carbonates and several soils are genetically young so that carbonates are still present in many soil horizons, this pattern could be partially linked to the precipitation of specific soil minerals. For instance, the precipitation of gibbsite [Al(OH)3] and calcite (CaCO3) was previously found to be reflected by soil pH at the global scale (Slessarev et al., 2016). Slessarev et al. (2016) showed that at the global scale, soils containing exchangeable Al have a pH averaging around 5, while CaCO3 is in equilibrium with atmospheric CO2 at much higher pH ranges (averaging at 8). Aluminum oxides and calcite usually precipitate from Al3+ and Ca2+ ions, respectively, releasing protons and thereby buffering pH during their precipitation processes. We found that in Swiss forest subsoils exchangeable Fe contributes little to CEC eff. (less than 1%). Fe3+ is seldom observed in large amounts as a free ion in soils (Rowley et al., 2018). Nevertheless, Fe-oxides are assumed to contribute substantially to SOM stabilization by the clay fraction (Inagaki et al., 2020).
Our assessment showed that the relationship between CEC eff. and subsoil organic C content evolves as a function of soil pH. CEC eff. explains a greater amount of variation of SOC content in subsoils with a pH > 5.5 (Table 3). Although this pattern could be partially explained by the increase in cation exchange sites provided by soil organic matter (Yuan et al., 1967), some studies have evidenced that some metal cations such as Ca2+ can influence SOC dynamics (Whittinghill and Hobbie, 2012; Minick et al., 2017; Martí-Roura et al., 2019; Rowley et al., 2020). Our observation that subsoil CEC eff. mainly originates from exchangeable Ca at pH levels higher than 5.5 (Figure 5) could indeed suggest that Ca2+ could play a role in stabilizing organic compounds in Swiss alkaline subsoils. In Switzerland, the regions with less precipitation largely maintain circum-neutral to alkaline pH values (Figure 1). It has previously been observed that regions with a drier climate favor the development of permanent charge clay minerals with a high abundance of negatively charged sites in alkaline soils (Douglas, 1989). This is in line with the increase in clay content that we observed at pH values > 5.5 (Figure 4). In Switzerland, however, geology may contribute to the observed linkage between precipitation and alkaline soils as drier regions are primarily located on calcareous bedrock. When polyvalent cations, such as Ca2+ occupy negatively charged exchange sites on permanent charge clay minerals, they can effectively bridge negatively charged organic compounds such as carboxylic acids which are commonly present in soils (Lützow et al., 2006; Mikutta et al., 2007). Moreover, Ca2+ can bridge negatively charged organic compounds together, forming organo-metal structures which protect soil organic compounds (Kunhi Mouvenchery et al., 2012). Overall, our finding of a complex influence of soil pH in shaping the relationship between CEC eff. and subsoil organic C strongly corroborates previous experimental and conceptual studies suggesting that soil pH can act as an essential indicator of the mechanisms controlling SOC stabilization (Newcomb et al., 2017; Rasmussen et al., 2018; Rowley et al., 2018).
In subsoils with pH values lower than 5.5, we observed that climate explains a higher amount of variance in subsoil organic C content as compared to CEC eff. (Table 3). The strong influence of MAP on organic C content in acidic subsoils is likely primarily related to the reaction of organic ligands with aluminum cations (Al3+) and aluminum oxides that occurs in many soils of regions with a high MAP and thus high water availabilities (Blaser and Sposito, 1987; Blaser et al., 1997; Torn et al., 1997; Chadwick and Chorover, 2001; Eckmeier et al., 2010; Inagaki et al., 2020). In acidic soils the presence of Al3+ cations is commonly associated with the production of organo-metal complexes during weathering. A strong chelation of organic ligands with Al3+ and the association of SOC to aluminum oxides in Swiss forest soils characterized by a high MAP and acid soils is pointed out by the high levels of exchangeable aluminum as well as oxalate extractable aluminum found at low pH classes (Figures 4, 5), and by the fact that the acidic soils occur primarily in regions with the highest MAP regime (Figure 1). Moreover, previous results have shown that the relatively humid climate of the Southern Alps promotes both the formation of pedogenic oxides and the leaching of dissolved organic C from the organic layer to the mineral soil, where organic matter i.e., tannins and other polyphenols, form organo-metal complexes (Eckmeier et al., 2010).
The finding that clay content explains a smaller amount of variation in SOC content as compared to CEC eff. strongly supports the emerging conceptual understanding that other edaphic properties, such as soil mineralogy and metal cations interacting with soil organic matter, could predict the potential persistence of SOC better than the amount of clay (e.g., Rasmussen et al., 2018; Rowley et al., 2018).
In conclusion, our study in Swiss forest soils indicates that soil organic matter contributes negligibly to the overall CEC eff. in Swiss forest subsoils (>30 cm). The relationship between CEC eff. and subsoil SOC is to a large extent mediated by the pH of soils and the local climate which largely shape soil physicochemical conditions. These conditions in turn reflect the respective effects of Ca2+ and Al3+ in protecting organic C, mainly by cation bridging or through the formation of organo-metal complexes. Due to the relatively narrow ranges of MAT and MAP in Switzerland, further studies are encouraged to examine the relationship between CEC eff. and SOC content also in other geographical locations and ecosystem types, especially in regions characterized by different climatic, biotic and geologic settings (such as tropical forests or arid lands). This would provide higher certainty in the mechanisms behind the functional relationship between CEC eff. and SOC, which might be used in larger-scale modeling applications. This information could be compiled starting from available soil profile samples, soil surveys and monitoring programs at the country, continental or climatic zone level2,3. Some global gridded data about cation exchange capacity has also already been derived by the international soil reference and information center ISRIC (Hengl et al., 2017). In our study, CEC eff. and SOC content are more strongly related at pH > 5.5. Since 73% of the global-land surface is covered by soils with pH > 5.5 [area calculated according to the global pH dataset IGBP-DIS (1998)], the consideration of CEC eff. as a variable reflecting the surface of soil minerals to which subsoil organic C can be stabilized by metal cations could strengthen our ability to predict future changes in the belowground C reservoir.
Data Availability Statement
All datasets presented in this study are included in the article/Supplementary Material.
Author Contributions
ES, MS, SZ, and FH conceived the idea of this analysis. SZ and LW provided and quality checked the data. ES developed the ideas for this manuscript and did the statistical analyses considering the preliminary analyses by VW. ES prepared and wrote the manuscript with contributions from all co-authors. All authors contributed to the article and approved the submitted version.
Funding
This study was financed by the Swiss National Science Foundation project “Deep C” (number 172744), the Swiss National Foundation Ambizione Project “PZ00P2_180030” and the University Research Priority Program for Global Change and Biodiversity of the University of Zurich.
Conflict of Interest
The authors declare that the research was conducted in the absence of any commercial or financial relationships that could be construed as a potential conflict of interest.
Acknowledgments
Evaluations were based on data from the Swiss Long-term Forest Ecosystem Research programme LWF (www.lwf.ch), which is part of the UNECE Co-operative Programme on Assessment and Monitoring of Air Pollution Effects on Forests ICP Forests (www.icp-forests.net). We are in particular grateful to Peter Jakob for his contribution to the soil database, and the technical staff of the WSL research unit Forest Soils and Biogeochemistry for collecting and analyzing the soil samples. We would like to acknowledged the fruitful discussions with Rose Abramoff (Lawrence Berkeley Laboratory) on early versions of the data analyses.
Supplementary Material
The Supplementary Material for this article can be found online at: https://www.frontiersin.org/articles/10.3389/ffgc.2020.00098/full#supplementary-material
DATA S1 | Data file originating from a database of the Swiss Federal Institute for Forest, Snow and Landscape Research WSL (Switzerland), containing soil data on 1204 forest sites across the country of Switzerland.
DATA S2 | Data file originating from a database of the Swiss Federal Institute for Forest, Snow and Landscape Research WSL (Switzerland), containing a subset of measurements of oxalate and dithionite extractable aluminum and iron.
Footnotes
References
Asner, G. P., Scurlock, J. M., and Hicke, A. J. (2003). Global synthesis of leaf area index observations: implications for ecological and remote sensing studies. Glob. Ecol. Biogeogr. 12, 191–205. doi: 10.1046/j.1466-822x.2003.00026.x
Bailey, V. L., Bond-Lamberty, B., DeAngelis, K., Grandy, A. S., Hawkes, C. V., Heckman, K., et al. (2018). Soil carbon cycling proxies: understanding their critical role in predicting climate change feedbacks. Glob. Change Biol. 24, 895–905. doi: 10.1111/gcb.13926
Blaser, P., Kernebeek, P., Tebbens, L., Van Breemen, N., and Luster, J. (1997). Cryptopodzolic soils in Switzerland. Eur. J. Soil Sci. 48, 411–423. doi: 10.1111/j.1365-2389.1997.tb00207.x
Blaser, P., and Sposito, G. (1987). Spectrofluorometric investigation of trace metal complexation by an aqueous chestnut leaf litter extract1. Soil Sci. Soc. Am. J. 51, 612–619. doi: 10.2136/sssaj1987.03615995005100030011x
Brassel, P., and Brändli, U. (1999). Schweizerisches Landesforstinventar. Ergebnisse der Zweitaufnahme 1993-1995, Eidgenössische Forschungsanstalt für Wald und Schnee und Landschaft, Bundesamt für Umwelt und Wald und Landschaft. Bern: Haupt Verlag.
Carvalhais, N., Forkel, M., Khomik, M., Bellarby, J., Jung, M., Migliavacca, M., et al. (2014). Global covariation of carbon turnover times with climate in terrestrial ecosystems. Nature 514, 213–217. doi: 10.1038/nature13731
Chadwick, O. A., and Chorover, J. (2001). The chemistry of pedogenic thresholds. Geoderma 100, 321–353. doi: 10.1016/S0016-7061(01)00027-1
Chen, S., Huang, Y., Zou, J., and Shi, Y. (2013). Mean residence time of global topsoil organic carbon depends on temperature, precipitation and soil nitrogen. Global and Planetary Change 100, 99–108. doi: 10.1016/j.gloplacha.2012.10.006
Coleman, K., and Jenkinson, D. (1996). “RothC-26.3-A Model for the turnover of carbon in soil,” in Evaluation of Soil Organic Matter Models, eds J. U. Smith, P. Smith, and D. S. Powlson (Cham: Springer), 237–246. doi: 10.1007/978-3-642-61094-3_17
Doetterl, S., Stevens, A., Six, J., Merckx, R., Van Oost, K., Pinto, M. C., et al. (2015). Soil carbon storage controlled by interactions between geochemistry and climate. Nat. Geosci. 8, 780–783. doi: 10.1038/ngeo2516
Douglas, L. A. (1989). Vermiculites. Miner. Soil Environ. 1, 635–674. doi: 10.2136/sssabookser1.2ed.c13
Eckmeier, E., Egli, M., Schmidt, M. W. I., Schlumpf, N., Nötzli, M., Minikus-Stary, N., et al. (2010). Preservation of fire-derived carbon compounds and sorptive stabilisation promote the accumulation of organic matter in black soils of the Southern Alps. Geoderma 159, 147–155. doi: 10.1016/j.geoderma.2010.07.006
Eusterhues, K., Rumpel, C., Kleber, M., and Kögel-Knabner, I. (2003). Stabilisation of soil organic matter by interactions with minerals as revealed by mineral dissolution and oxidative degradation. Organ. Geochem. 34, 1591–1600. doi: 10.1016/j.orggeochem.2003.08.007
Farrar, D. M., and Coleman, J. D. (1967). The correlation of surface area with other properties of nineteen british clay soils. J. Soil Sci. 18, 118–124. doi: 10.1111/j.1365-2389.1967.tb01493.x
Friedlingstein, P., Meinshausen, M., Arora, V. K., Jones, C. D., Anav, A., Liddicoat, S. K., et al. (2014). Uncertainties in CMIP5 climate projections due to carbon cycle feedbacks. J. Clim. 27, 511–526. doi: 10.1175/jcli-d-12-00579.1
Gee, G. W., and Bauder, J. W. (1986). Particle-Size Analysis 1. Madison: Soil Science Society of America.
Gosheva, S., Walthert, L., Niklaus, P. A., Zimmermann, S., Gimmi, U., and Hagedorn, F. (2017). Reconstruction of historic forest cover changes indicates minor effects on carbon stocks in swiss forest soils. Ecosystems 20, 1512–1528. doi: 10.1007/s10021-017-0129-9
Groemping, U., and Matthias, L. (2006). Relaimpo: Relative Importance of Regressors in Linear Models. R Package Version 1.1–1.
Grömping, U. (2006). Relative importance for linear regression in R: the package relaimpo. J. Stat. Softw. 17, 1–27.
Harden, J. W., Hugelius, G., Ahlström, A., Blankinship, J. C., Bond-Lamberty, B., Lawrence, C. R., et al. (2018). Networking our science to characterize the state, vulnerabilities, and management opportunities of soil organic matter. Glob. Change Biol. 24, e705–e718. doi: 10.1111/gcb.13896
Hengl, T., de Jesus, J. M., Heuvelink, G. B., Gonzalez, M. R., Kilibarda, M., Blagotiæ, A., et al. (2017). SoilGrids250m: global gridded soil information based on machine learning. PLoS One 12:e0169748. doi: 10.1371/journal.pone.0169748
Herold, N., Schöning, I., Michalzik, B., Trumbore, S., and Schrumpf, M. (2014). Controls on soil carbon storage and turnover in German landscapes. Biogeochemistry 119, 435–451. doi: 10.1007/s10533-014-9978-x
Holmgren, G. G. S. (1967). A rapid citrate-dithionite extractable iron procedure. Soil Sci. Soc. Am. J. 31, 210–211. doi: 10.2136/sssaj1967.03615995003100020020x
IGBP-DIS (1998). SoilData(V.0) A Program for Creating Global Soil-Property Databases. France: IGBP Global Soils Data Task.
Inagaki, T. M., Possinger, A. R., Grant, K. E., Schweizer, S. A., Mueller, C. W., Derry, L. A., et al. (2020). Subsoil organo-mineral associations under contrasting climate conditions. Geochim. Cosmochim. Acta 270, 244–263. doi: 10.1016/j.gca.2019.11.030
Iskrenova-Tchoukova, E., Kalinichev, A. G., and Kirkpatrick, R. J. (2010). Metal cation complexation with natural organic matter in aqueous solutions: molecular dynamics simulations and potentials of mean force. Langmuir 26, 15909–15919. doi: 10.1021/la102535n
Jackson, R. B., Lajtha, K., Crow, S. E., Hugelius, G., Kramer, M. G., and Piñeiro, G. (2017). The ecology of soil carbon: pools, vulnerabilities, and biotic and abiotic controls. Annu. Rev. Ecol. Evol. Syst. 48, 419–445. doi: 10.1146/annurev-ecolsys-112414-054234
Jobbágy, E. G., and Jackson, R. B. (2000). The vertical distribution of soil organic carbon and its relation to climate and vegetation. Ecol. Applic. 10, 423–436. doi: 10.1890/1051-0761(2000)010[0423:tvdoso]2.0.co;2
Kaiser, K., and Guggenberger, G. (2000). The role of DOM sorption to mineral surfaces in the preservation of organic matter in soils. Organ. Geochem. 31, 711–725. doi: 10.1016/S0146-6380(00)00046-2
Klamt, E., and Sombroek, W. (1986). “Contribution of organic matter to exchange properties of Oxisols. Contribution of organic matter to exchange properties of Oxisols,” in Proceedings of the 8th Internation soil classification workshop on Classification ISRIC Report 1986/05. ISRIC Report 1986/05: Contribution of Organic Matter to Exchange Properties of Oxisols, (Wageningen: ISRIC).
Kunhi Mouvenchery, Y., Kučerík, J., Diehl, D., and Schaumann, G. E. (2012). Cation-mediated cross-linking in natural organic matter: a review. Rev. Environ. Sci. Bio Technol. 11, 41–54. doi: 10.1007/s11157-011-9258-3
Leoppert, R., and Inskeep, W. (1996). Colorimetric determination of ferrous iron and ferric iron by the 1, 10-phenanthroline method. Methods Soil Anal. Part 3, 659–661.
Lützow, M. V., Kögel-Knabner, I., Ekschmitt, K., Matzner, E., Guggenberger, G., Marschner, B., et al. (2006). Stabilization of organic matter in temperate soils: mechanisms and their relevance under different soil conditions – a review. Eur. J. Soil Sci. 57, 426–445. doi: 10.1111/j.1365-2389.2006.00809.x
Martí-Roura, M., Hagedorn, F., Rovira, P., and Romanyà, J. (2019). Effect of land use and carbonates on organic matter stabilization and microbial communities in Mediterranean soils. Geoderma 351, 103–115. doi: 10.1016/j.geoderma.2019.05.021
Mikutta, R., Mikutta, C., Kalbitz, K., Scheel, T., Kaiser, K., and Jahn, R. (2007). Biodegradation of forest floor organic matter bound to minerals via different binding mechanisms. Geochim. Cosmochim. Acta 71, 2569–2590. doi: 10.1016/j.gca.2007.03.002
Minick, K. J., Fisk, M. C., and Groffman, P. M. (2017). Soil Ca alters processes contributing to C and N retention in the Oa/A horizon of a northern hardwood forest. Biogeochemistry 132, 343–357. doi: 10.1007/s10533-017-0307-z
Mueller-Dombois, D., and Ellenberg, H. (1974). Aims and Methods of Vegetation Ecology. New York, NY: Blackburn Press.
Newcomb, C. J., Qafoku, N. P., Grate, J. W., Bailey, V. L., and De Yoreo, J. J. (2017). Developing a molecular picture of soil organic matter–mineral interactions by quantifying organo–mineral binding. Nat. Commun. 8:396. doi: 10.1038/s41467-017-00407-9
Oades, J. M. (1988). The retention of organic matter in soils. Biogeochemistry 5, 35–70. doi: 10.1007/bf02180317
Parfitt, R., and Childs, C. (1988). Estimation of forms of Fe and Al-a review, and analysis of contrasting soils by dissolution and Mossbauer methods. Soil Res. 26, 121–144.
R Core Team (2018). R: A Language and Environment for Statistical Computing. Vienna: R Foundation for Statistical Computing.
Rasmussen, C., Heckman, K., Wieder, W. R., Keiluweit, M., Lawrence, C. R., Berhe, A. A., et al. (2018). Beyond clay: towards an improved set of variables for predicting soil organic matter content. Biogeochemistry 137, 297–306. doi: 10.1007/s10533-018-0424-3
Remund, J., Rihm, B., and Huguenin-Landl, B. (2014). Klimadaten für die Waldmodellierung für das 20. und 21. Jahrhundert Schlussbericht. Forschungsprogramm, Wald und Klimawandel“ des Bundesamtes für Umwelt BAFU, Bern und der Eidg. Forschungsanstalt WSL, Birmensdorf. Bern: Meteotest.
Rowley, M. C., Grand, S., Adatte, T., and Verrecchia, E. P. (2020). A cascading influence of calcium carbonate on the biogeochemistry and pedogenic trajectories of subalpine soils, Switzerland. Geoderma 361:114065. doi: 10.1016/j.geoderma.2019.114065
Rowley, M. C., Grand, S., and Verrecchia, ÉP. (2018). Calcium-mediated stabilisation of soil organic carbon. Biogeochemistry 137, 27–49. doi: 10.1007/s10533-017-0410-1
Salazar, I., Escudey, M., Guajardo, J., and Goulding, K. W. (2002). Effects of organic matter and iron oxides on cation exchange equilibria and potassium selectivity in a volcanic ash soil of Chile. Commun. Soil Sci. Plant Anal. 33, 3663–3677. doi: 10.1081/css-120015914
Schleppi, P., Thimonier, A., and Walthert, L. (2011). Estimating leaf area index of mature temperate forests using regressions on site and vegetation data. For. Ecol. Manag. 261, 601–610. doi: 10.1016/j.foreco.2010.11.013
Schmidt, M. W., Torn, M. S., Abiven, S., Dittmar, T., Guggenberger, G., Janssens, I. A., et al. (2011). Persistence of soil organic matter as an ecosystem property. Nature 478, 49–56.
Slessarev, E. W., Lin, Y., Bingham, N. L., Johnson, J. E., Dai, Y., Schimel, J. P., et al. (2016). Water balance creates a threshold in soil pH at the global scale. Nature 540, 567–569. doi: 10.1038/nature20139
Sutton, R., Sposito, G., Diallo, M. S., and Schulten, H.-R. (2005). Molecular simulation of a model of dissolved organic matter. Environ. Toxicol. Chem. 24, 1902–1911. doi: 10.1897/04-567r.1
Thomas, G. W. (1982). “Exchangeable cations. Methods of soil analysis. Part 2. Chemical and microbiological properties (methodsofsoilan2),” in Agronomy, No. 9, Part 2, American Society of Agronomy, ed. A.L. Page (Madison, WL: Soil Science Society of America), 159–165. doi: 10.2134/agronmonogr9.2.2ed.c9
Todd-Brown, K. E. O., Randerson, J. T., Post, W. M., Hoffman, F. M., Tarnocai, C., Schuur, E. A. G., et al. (2013). Causes of variation in soil carbon simulations from CMIP5 Earth system models and comparison with observations. Biogeosciences 10, 1717–1736. doi: 10.5194/bg-10-1717-2013
Torn, M. S., Trumbore, S. E., Chadwick, O. A., Vitousek, P. M., and Hendricks, D. M. (1997). Mineral control of soil organic carbon storage and turnover. Nature 389:170. doi: 10.1038/38260
van der Voort, T. S., Hagedorn, F., McIntyre, C., Zell, C., Walthert, L., Schleppi, P., et al. (2016). Variability in 14C contents of soil organic matter at the plot and regional scale across climatic and geologic gradients. Biogeosci. Discuss 2016, 1–28. doi: 10.5194/bg-2015-649
Vogel, C., Mueller, C. W., Höschen, C., Buegger, F., Heister, K., Schulz, S., et al. (2014). Submicron structures provide preferential spots for carbon and nitrogen sequestration in soils. Nat. Commun. 5, 1–7.
Walthert, L., Graf, U., Kammer, A., Luster, J., Pezzotta, D., Zimmermann, S., et al. (2010). Determination of organic and inorganic carbon, δ13C, and nitrogen in soils containing carbonates after acid fumigation with HCl. J. Plant Nutr. Soil Sci. 173, 207–216. doi: 10.1002/jpln.200900158
Walthert, L., Graf Pannatier, E., and Meier, E. S. (2013). Shortage of nutrients and excess of toxic elements in soils limit the distribution of soil-sensitive tree species in temperate forests. For. Ecol. Manag. 297, 94–107. doi: 10.1016/j.foreco.2013.02.008
Walthert, L., and Meier, E. S. (2017). Tree species distribution in temperate forests is more influenced by soil than by climate. Ecol. Evol. 7, 9473–9484. doi: 10.1002/ece3.3436
Walthert, L., Zimmermann, S., Blaser, P., Luster, J., and Lüscher, P. (2004). Waldböden der Schweiz. Switzerland: Hep Verlag Bern.
Weil, R., and Brady, N. C. (2016). Nature and Properties of Soils, the: Pearson New International Edition. London: Pearson Higher Ed.
Whittinghill, K. A., and Hobbie, S. E. (2012). Effects of pH and calcium on soil organic matter dynamics in Alaskan tundra. Biogeochemistry 111, 569–581. doi: 10.1007/s10533-011-9688-6
Wieder, W. R., Grandy, A. S., Kallenbach, C. M., Taylor, P. G., and Bonan, G. B. (2015). Representing life in the Earth system with soil microbial functional traits in the MIMICS model. Geosci. Model Dev. 8, 1789–1808. doi: 10.5194/gmd-8-1789-2015
Wright, W. R., and Foss, J. E. (1972). Contributions of clay and organic matter to the cation exchange capacity of maryland soils1. Soil Sci. Soc. Am. J. 36, 115–118. doi: 10.2136/sssaj1972.03615995003600010027x
Keywords: effective cation exchange capacity, soil organic carbon content, forests, subsoil, carbon stabilization
Citation: Solly EF, Weber V, Zimmermann S, Walthert L, Hagedorn F and Schmidt MWI (2020) A Critical Evaluation of the Relationship Between the Effective Cation Exchange Capacity and Soil Organic Carbon Content in Swiss Forest Soils. Front. For. Glob. Change 3:98. doi: 10.3389/ffgc.2020.00098
Received: 28 May 2020; Accepted: 20 July 2020;
Published: 04 September 2020.
Edited by:
Yunting Fang, Institute of Applied Ecology (CAS), ChinaReviewed by:
Xiankai Lu, South China Botanical Garden, Chinese Academy of Sciences, ChinaLei Duan, Tsinghua University, China
Copyright © 2020 Solly, Weber, Zimmermann, Walthert, Hagedorn and Schmidt. This is an open-access article distributed under the terms of the Creative Commons Attribution License (CC BY). The use, distribution or reproduction in other forums is permitted, provided the original author(s) and the copyright owner(s) are credited and that the original publication in this journal is cited, in accordance with accepted academic practice. No use, distribution or reproduction is permitted which does not comply with these terms.
*Correspondence: Emily F. Solly, ZW1pbHkuc29sbHlAdXN5cy5ldGh6LmNo