- 1Department of Forest Resource Management, Swedish University of Agricultural Sciences, Umeå, Sweden
- 2Department of Statistics, Umeå School of Business, Economics and Statistics, Umeå University, Umeå, Sweden
Forest information is requested at many levels and for many purposes. Sampling-based national forest inventories (NFIs) can provide reliable estimates on national and regional levels. By combining expensive field plot data with different sources of remotely sensed information, from airplanes and/or satellite platforms, the precision in estimators of forest variables can be improved. This paper focuses on the design-based model-assisted approach to using NFI data together with remotely sensed data to estimate forest variables for small areas, where the variables studied are total growing stock volume, volume of Norway spruce (Picea abies), and volume of broad-leaved trees. Remote sensing variables may be highly correlated with one another and some may have poor predictive ability for target forest variables, and therefore model selection and/or coefficient shrinkage may be appropriate to improve the efficiency of model-assisted estimators of forest variables. For this purpose, one can use modern shrinkage estimators based on lasso, ridge, and elastic net regression methods. In a simulation study using real NFI data, Sentinel 2 remote-sensing data, and a national airborne laser scanning (ALS) campaign, we show that shrinkage estimators offer advantages over the (weighted) ordinary least-squares (OLS) estimator in a model-assisted setting. For example, for a sample size n of about 900 and with 72 auxiliary variables, the RMSE was up to 41% larger when based on OLS. We propose a data-driven method for finding suitable transformations of auxiliary variables, and show that it can improve estimators of forest variables. For example, when estimating volume of Norway spruce, using a smaller expert selection of auxiliary variables, transformations reduced the RMSE by up to 10%. The overall best results in terms of RMSE were obtained using shrinkage estimators and a larger set of 72 auxiliary variables. However, for this larger set of variables, the use of transformations yielded at most small improvements of RMSE, and at worst large increases of RMSE, except in combination with ridge and elastic net regression.
Introduction
Information about forests is needed for many purposes and at various geographical levels. Large area sampling-based national forest inventories (NFIs) provide reliable estimates of mean values or totals on a national and regional level (Tomppo et al., 2011; Fridman et al., 2014). These estimates are used, for example, to form national forest policies, sustainability assessment, and reporting to international conventions. However, terrestrial inventory systems such as NFIs are typically designed to provide reliable estimates on a national and regional scale and may not provide sufficiently precise estimates for small areas without including auxiliary information, for example remote sensing data (McRoberts et al., 2014).
The availability of airborne laser scanning (ALS) data, and spectral data from Sentinel 2 and Landsat 8 satellites that are freely available, offers new possibilities for NFIs to produce more precise statistical estimates than by using field data alone. In order to utilize the full potential of auxiliary remote sensing data for statistical estimates, comprehensive remote sensing data can be combined with sample-based field measurements utilizing sampling theory (Gregoire et al., 2011). An important category of sample-based estimators that can be used for this purpose are known as design-based model-assisted estimators (Särndal et al., 1992). Such estimators use models and auxiliary data to improve the efficiency, while maintaining design-based properties of asymptotic design-unbiasedness and consistency (Breidt and Opsomer, 2016). Thus, model-assisted estimators are asymptotically design-unbiased irrespective of whether the assigned model is correct or not, where design-unbiasedness means that the estimator is unbiased over repeated sampling of field data. In contrast, model-based estimators, which do not utilize the sampling design for the inference, do not share these desirable properties (Kangas et al., 2016; Ståhl et al., 2016). When models are correctly assigned, model-based estimators can be very efficient, but model misspecifications easily result in severely biased estimators (Chambers et al., 2006).
The range of prediction techniques that can be used in a model-assisted estimator has dramatically increased during the last couple of decades. The main reason for this is the rapid development in the field of statistical learning and its very close cousin machine learning (Hastie et al., 2009, 2015; Berk, 2016). Breidt and Opsomer (2016) provide a review of such techniques in a model-assisted context. With a machine learning or statistical learning perspective, model-assisted methods are judged on their ability to produce precise estimates rather than on their ability to build interpretable models (McConville et al., 2020).
The model-assisted framework has gained an increasing popularity in forest inventory, and various prediction techniques have been utilized within this framework. Breidt et al. (2005) considered penalized spline regression together with auxiliary information such as GIS data. Opsomer et al. (2007) applied generalized additive models (GAMs), using three sources of auxiliary data, digital elevation models, Landsat TM imagery, and spatial coordinates. Baffetta et al. (2009, 2010) developed an estimator using k-nearest neighbor regression, and used Landsat 7 ETM+ imagery as auxiliary data. Chirici et al. (2016) compared the performance of k-nearest neighbor regression with linear regression, using auxiliary ALS based metrics. Kangas et al. (2016) considered three different predictions techniques, linear regression (where no transformations were carried out to linearize the relationship), GAM regression, and kernel regression, and used ALS data as auxiliary data. Moser et al. (2017) used non-linear regression and auxiliary ALS data, and explored variable selection techniques based on genetic algorithms and random forests. McConville et al. (2017) considered various lasso regression methods, using auxiliary variables from a national land cover database and Landsat 5 TM imagery, and comparisons were made with other predictions techniques such as linear regression and ridge regression. Further studies on lasso regression and its close cousins ridge regression and elastic net regression were made in McConville et al. (2020), using auxiliary data from Landsat imagery, forest maps, and a digital elevation model, and comparisons were made with standard prediction techniques, including linear regression (for continuous target variables) and logistic regression (for categorical target variables).
Remote sensing data or data that originates from remotely sensed data are used as auxiliary data in many forest inventory applications. This often means that the auxiliary data are known for the entire finite population under consideration, and that the number of potential auxiliary variables is large. As in Moser et al. (2017), methods for variable selection can be used for selecting a “best” set of auxiliary variables. Ridge, lasso, and elastic net regression shrink coefficient estimates toward zero, relative to least-squares estimates in a standard multiple linear regression. In the case of lasso and elastic net, coefficient estimates can be forced to be exactly zero. Consequently, these methods can also perform variable selection.
In this paper, we consider ridge, lasso, and elastic net regression in a model-assisted framework. Since the relationship between the target variable y and an auxiliary variable x can be non-linear, transformations of x may be needed. The key step is the identification of an appropriate transformation. In many applications, the form of transformation is suggested by prior experience. Unfortunately, in many cases, prior knowledge or theory may not suggest a suitable transformation to be used. In such situations, it would be convenient to determine the transformation adaptively, using a data-driven method for selecting appropriate transformations. This is especially useful when the number of auxiliary variables is large. For this reason, we suggest and investigate the performance of a data-driven method for finding suitable transformations in a model-assisted framework, where the method used is based on fractional polynomials (Royston and Altman, 1994).
The objective of this study was to evaluate ridge, lasso, and elastic net regression for prediction of volume per hectare of total growing stock, Norway spruce (Picea abies), and broad-leaved trees in a model-assisted setting, with or without data-driven transformations of auxiliary variables. The evaluation includes comparisons with the most well-known model-assisted estimator, the generalized regression estimator based on a multiple regression model, and is based on Monte Carlo simulations using real data, from the Swedish NFI, Sentinel-2, and a national laser scanning campaign. Also, an expert’s a priori selection of a smaller set of auxiliary variables is compared to using a full set of variables. The influence of outliers is discussed.
Materials and Methods
Data
Test Area
In this study, we used a combination of data from a national ALS campaign, Sentinel 2, and the Swedish NFI to estimate volume per hectare of total growing stock, Norway spruce, and broad-leaved trees. Our test area is in southern Sweden and covers an area of approximately 6.0 million ha for which Sentinel 2 images and Leica ALS data registered during leaf-off conditions were available (Figure 1). The test area was restricted to areas mapped as land in the Swedish National Land Cover Database (NMD; Naturvårdsverket, 2020), except buildings (class 51 in NMD). Coniferous forest dominates the landscape within the test area, and the proportion of tree species are 28, 47, and 25% for Scots pine (Pinus sylvestris), Norway spruce (Picea abies), and broad-leaved trees, respectively, according to the Swedish NFI.
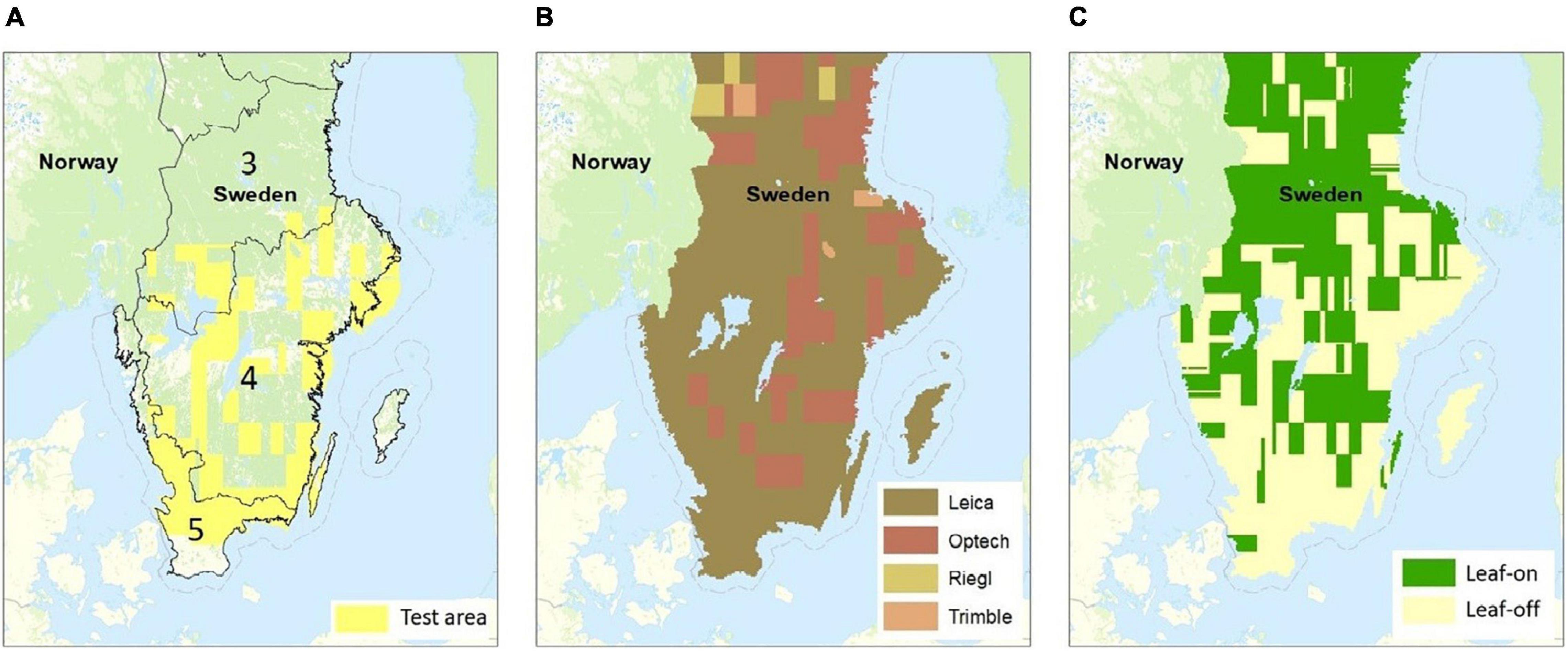
Figure 1. Test area (A), scanner brand (B), and scanning season (C). Three of the strata used in the Swedish NFI are shown in (A) (strata 3, 4, and 5). These strata roughly correspond to the vegetation zones: (3) southern-middle boreal; (4) mainly hemiboreal; and (5) temperate. Copyright Lantmäteriet.
National Forest Inventory Data
The Swedish NFI provides information about forests for regional, national and international policy, planning, and reporting (Fridman et al., 2014). It has been operating since 1923 and at present more than 200 variables are recorded. The NFI covers all forests in Sweden (55–69°N) and the design includes both geographical stratification and clustering of sample plots into square-formed tracts with a side length that varies from 300 to 1,800 m among regions. There are two independent samples, one permanent and one temporary, where trees are measured on concentric sample plots with different radii depending on tree diameter at breast height (Fridman et al., 2014). On both temporary and permanent plots, trees with a diameter less than 4 cm are measured on two 1 m radius plots, and trees with a diameter between 4 and 10 cm are measured on a 3.5 m radius plot (Figure 2). If the diameter is 10 cm or more, the trees are measured on plots with 7 m or 10 m radius for temporary and permanent plots, respectively. Sample plots located on boundaries between forest stands or different land use classes are split and each part is described separately.
The NFI began positioning sample plots using GPS receivers in 1996. As of 2021, Garmin GPSMAP 64 receivers are used for the positioning that give a horizontal positional accuracy of approximately 5–10 m.
In this study, we used NFI data from 2012 to 2016. Split plots were merged and volume per ha of total growing stock, Norway spruce, and broad-leaved trees were calculated for the merged plots (the rest of the growing stock volume was mainly Scots pine). In total, there were 9008 NFI plots within the test area, located in three different geographic strata (Table 1).
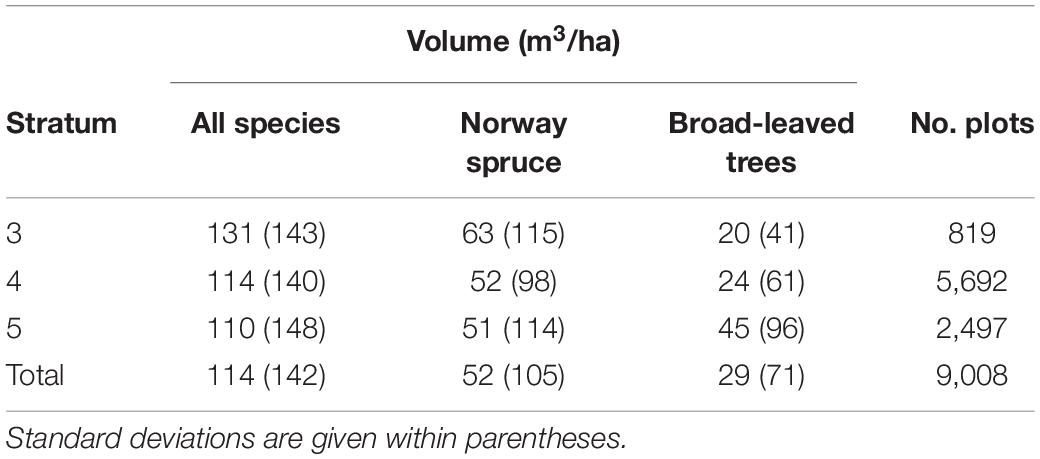
Table 1. Mean volume per hectare of total growing stock, Norway spruce, and broad-leaved trees, and number of plots by stratum.
Airborne Laser Scanning Data
The first national ALS campaign in Sweden started in 2009 and ended in 2019. During the campaign, the National Mapping Agency (Lantmäteriet) collected data from flying heights between 1,700 and 2,300 m and with a point density of 0.5–1.0 pulses/m2. A maximum scanning angle of 20° from nadir with a 20% overlap between adjacent scanning strips was used. For practical reasons, the campaign was divided into 397 blocks with a normal size of 25 km by 50 km. A block was always scanned using one scanner, but the scanner used varied between blocks. In total, 13 different scanners from Leica, Optech, Riegl and Trimble were used. As mentioned above, the study was restricted to areas where ALS data had been acquired with Leica scanners during leaf-off conditions (Figure 1A). All blocks within the test area were laser scanned between 2009 and 2013.
A national DEM (2 m × 2 m grid cell size), derived from the national ALS dataset by the National Mapping Agency, was used to calculate height above ground (normalized height) for all returns. A set of ALS metrics were calculated for each NFI plot using CloudMetrics (McGaughey, 2020) and used together with Sentinel 2 spectral data as auxiliary variables (Table 2).
Satellite Data
A mosaic of Sentinel-2 data from 2015 to 2017 with top-of-the-atmosphere (TOA) reflectance from bands 4, 5, 7, 8, 8a, 11, and 12 were used. About 95% of the test area was covered by images registered on May 27 and July 6, 2017 (Table 3). Additional images from 2015 to 2016 were used to cover the remaining parts of the test area, resulting in an almost cloud free mosaic. All image bands were resampled to 12.5 × 12.5 m pixel size and spectral data from all seven bands were extracted for the NFI plots using nearest neighbor interpolation. Sentinel-2 data were missing for 208 of the 9008 NFI plots due to clouds or cloud shadows. For these plots, spectral values were imputed based on all ALS metrics (Table 2), the sum of all daily mean temperature values exceeding 5° C° (Tsum), altitude, and plot coordinates (x and y) using the knnImputation function (k = 3) in the R package DMwR (Torgo, 2010).
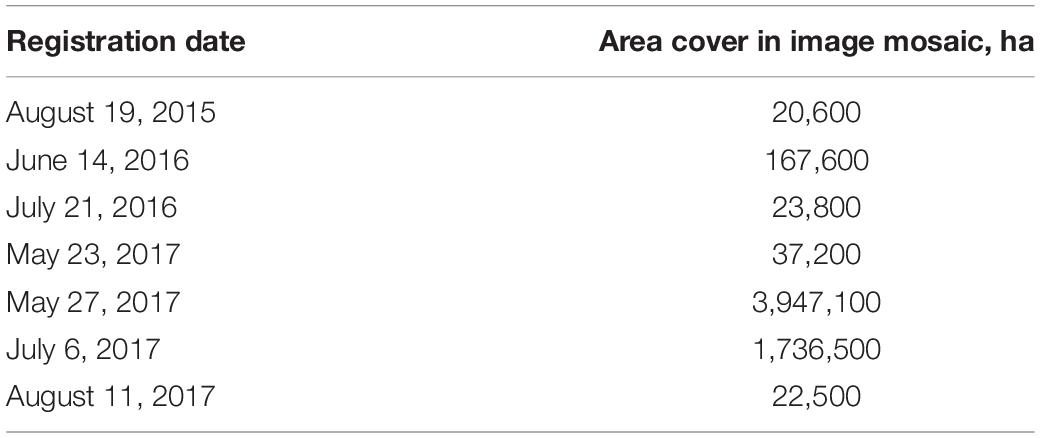
Table 3. Registration dates for Sentinel-2 images used in the study and the area covered at each registration date.
Final Auxiliary Data
Three different datasets were defined from the variables in Table 2. The first dataset consisted of all 72 variables in the table and will be referred to as “all available auxiliary variables.” The two other datasets were subsets of the variables in Table 2, and will be referred to as “expert’s selections of auxiliary variables.” The first subset was used to estimate total growing stock volume and included 90th (P90) ALS height percentile for all laser returns above 1.5 m, proportion of all laser returns above 1.5 m multiplied by P90 (P90 Prop), and standard deviation for all laser returns above 1.5 m (Stddev). These variables were chosen because they previously were used to predict the total growing stock volume in the production of a nationwide raster database of forest variables using data from the first national ALS campaign (Nilsson et al., 2017). The second subset was used to estimate volume for Norway spruce and broad-leaved trees and included 95th height percentile for all laser returns above 1.5 m (P95), the proportion of all laser returns above 1.5 m (Prop), and Sentinel-2 bands 4, 5, 7, 8, 8a, 11, and 12. The metrics were selected based on experiences from an ongoing project with the aim to predict standing volume by tree species from a combination of ALS metrics and Sentinel 2 data.
A correlation matrix was calculated for the 72 auxiliary variables in Table 2, containing 2556 unique correlation coefficients. The absolute values of these were larger than 0.5 in 1209 cases. In 212 cases they were larger than 0.9, and in 39 cases larger than 0.99. The largest absolute correlation coefficient between growing stock volume and an auxiliary variable was 0.75. For volume of Norway spruce and volume of broad-leaved trees, the corresponding values were 0.55 and 0.35, respectively.
Methods
To construct estimators of forest variables, the area of interest was tessellated into a finite number of population units, labeled by {1, 2, …, N}, where the set was denoted by U. In our setting, a square tessellation was used, given by the 12.5 × 12.5 m raster cells in the wall-to-wall auxiliary data. The objective was to estimate the population mean, , where yi denotes value of the target forest variable for the ith unit.
A sample s of units is selected with a view to obtain information about the whole population. In large-area surveys like NFIs and vegetation monitoring programs, samples are usually taken using complex probability sampling designs that include, for example, geographical stratification (Ekström et al., 2018). In these designs, each population unit i typically has a non-zero probability πi of getting included in the sample.
Design-based estimators incorporate sample design characteristics into their formulae, typically to achieve desirable properties such as unbiasedness. The Horvitz and Thompson (1952) estimator (HT) of the population mean, , incorporates design information through inverse-probability weighting,
The HT is a design-unbiased estimator, which means that the mean of the estimator, taken over all possible samples under the sampling design, is equal to . The estimator of the variance of in (1), suggested by Horvitz and Thompson (1952), is
where πij is the probability that both units i and j are included in the sample s, and πii = πi for all i.
Model-Assisted Estimators
One possible approach to improving the efficiency of estimators is to incorporate auxiliary information, and model-assisted estimation is a form of design-based estimation that incorporates both design information (through the inclusion probabilities πi) and auxiliary information (through a model). Many super-population models for this purpose can be written in the form
with random, zero-mean ϵi, and a vector of auxiliary variables for unit i, xi = (1, xi1, …, xip). The predictor function μ(⋅) is typically unknown, but can be estimated using the sample data. Denoting the estimated predictor by , a general class of model-assisted estimators of the population mean, known as generalized regression estimators (GREG), can be defined as
It should be noted that the estimator (4) depends on the sampling design, the form of the model, and the method used for estimating the predictor function μ(⋅). The estimator (4) consists of two parts, the mean of the predicted values over the population and the design bias adjustment consisting of inverse probability-weighted “residuals” . This adjustment term protects against model misspecification, and makes the estimator approximately design-unbiased for many commonly used prediction methods (see, e.g., Breidt and Opsomer (2016) and the references therein).
To estimate the variance for (4) we use a common variance estimator approach based on (2) but replacing the “raw” yi values with the “residuals” (cf. Breidt and Opsomer, 2016). Provided that the residuals have smaller variation than the raw values, we can expect GREG to have a smaller variance than HT.
Under a multiple linear regression model with μ(x) = xTβ, the parameter vector β = (β0, β1, …, βp) can be estimated using weighted least-squares. This approach gives the predictor , where
where arg min means the value of β which minimizes the sum of design-weighted squared residuals. With plugged into (4), we refer to (4) as the regression estimator (REG).
For our analyses, is computed using the glm function in R (R Core Team, 2020). If some auxiliary variables are perfectly or nearly perfectly collinear, the glm function automatically excludes at least one of them and sets the corresponding coefficients to NA (not available). For this reason, we investigate the following two variants for handling this problem:
(i) calculate pairwise correlations among the variables in the sample and, among each pair of variables correlated above a given threshold, exclude the variable least correlated with the target variable;
(ii) if a coefficient is NA, then simply set it to 0.
If, for example, the second variant is used, we refer to (4) as REGii. A benefit of the first variant is that it decreases the danger of multicollinearity, but as argued in Vaughan and Berry (2005), multicollinearity is “not quite as damning” when linear modeling is used for prediction rather than explanation. That is, in case of (severe) multicollinearity, coefficient estimates and their standard errors can become (very) sensitive to small changes in the model, but this usually has little effect on the prediction capability of the model. However, if the fitted model is used to predict values for new data, and the pattern of multicollinearity in the new data differs from that in the data that was fitted, this may introduce large errors in the predictions (Chatterjee et al., 2012).
Another possibility is to estimate the parameter vector β using penalized weighted least squares. Elastic net regression (Zou and Hastie, 2005; McConville et al., 2020), introduced as compromise between lasso and ridge regression, is an approach that uses a penalty. Here, the parameter vector is estimated by
where 0 ≤ α ≤ 1. When α = 0, elastic net regression becomes ridge regression, and when α = 1 it becomes lasso regression. Ridge regression tends to give similar coefficient values to highly correlated auxiliary variables, whereas lasso regression tend to give quite different coefficient values to highly correlated variables. Unlike ridge regression, lasso regression performs variable selection by forcing some of the coefficient estimates to be exactly equal to zero (this happens if the “tuning parameter” λ is sufficiently large). Elastic net regression, with α equal to a value between 0 and 1, shrinks together the coefficients of correlated auxiliary variables like ridge, and performs variable selection like the lasso (Zou and Hastie, 2005). Thus, the α value in (5) is the “mixing proportion” that toggles between a pure lasso penalty (when α = 1) and a pure ridge penalty (α = 0). The parameter λ controls the total amount of penalization. Both penalties shrink the coefficient estimates toward zero, relative to the usual (weighted) least-squares estimates, and the more so the larger λ is. As λ increases, the shrinkage of the coefficient estimates reduces the variance of the predictions, at the expense of an increase in bias (James et al., 2021). Selecting a good value for λ is therefore critical for finding a good balance between variance and bias, and cross-validation is commonly used for this purpose.
With the estimator function set to the generalized penalized estimator , we refer to (4) as RIDGE, ELNET, and LASSO, for α = 0, 0.5, and 1, respectively. These three are available through the R package mase (McConville et al., 2018), which uses cross-validation to choose the tuning parameter λ. If there are issues with multicollinearity, McConville et al. (2020) recommend using RIDGE or ELNET rather than REG or LASSO.
In our study and for a given set of auxiliary variables, the parameter vector β is estimated using all data from a sample s. In Supplementary Material, results are presented also for the case where outliers in the sample s are removed before β is estimated. The identified outliers are those where field measured tree height and the 95th height percentile in the ALS data deviate more than 7 m.
Data-Driven Choices of Transformations
A model with assumes a linear relationship between the expected value of the target variable yi in (3) and each auxiliary variable (when the other auxiliary variables are held fixed). If linearity fails to hold, it is sometimes possible to transform the auxiliary variables in the model to improve the linearity. Examples of a non-linear transformation of variable xij are the square root or the reciprocal of xij. Suitable transformations can be found through studies of residual plots, but this is tedious work when the number of variables is large. For this reason, we investigate the performance of a data-driven method for finding suitable transformations. The method is based on fractional polynomials (FPs; Royston and Altman, 1994). FP is an approach that uses a function selection procedure to check whether a non-linear function fits the data significantly better than a linear function. We use the level of significance 5% for the function selection. To reduce the computational burden, the function selection is done for one auxiliary variable at a time.
The class of FP functions is an extension of power transformations of a variable, and in this study the attention is restricted to FPs of the first degree. That is, the powers are selected from the collection {−3,−2,−1,−0.5, 0, 0.5, 1, 2, 3}, where 0 denotes the log transformation, using the sample data and the fp and mfp functions in the R package mfp (Ambler and Banner, 2015). FPs are defined only for positive auxiliary variables, but real data may contain non-positive observations. Therefore, at population level, if non-positive values are encountered (or the range of values of the auxiliary variables is unreasonably large), the auxiliary variables are shifted (and rescaled). The method for doing this is adopted from the mfp algorithm (Sauerbrei et al., 2006; Sabanés Bové and Held, 2011).
In our study, outliers in the sample data are not used in the selection procedure of transformations. Again, the identified outliers are those where field measured tree height and the 95th height percentile in the ALS data deviate more than 7 meters. (In Supplementary Material, results are presented also for the case where transformations are selected based on all sample data).
Evaluation of the Estimators
The performances of estimators were compared using Monte Carlo simulations. The population units were defined by the 9008 pixels that we matched with the corresponding plots given in Table 1. Three strata were defined according to Table 1, and Monte Carlo simulations were implemented with a stratified simple random sampling design. With this design, a simple random sample without replacement is drawn from each strata, the drawings being made independently in different strata. In comparison with the Swedish NFI, the main difference is that we ignored that plots are grouped into tracts. The number of sampled units in each stratum was proportional to the size of the stratum. Two sample sizes were considered in the simulations, n = 901 and n = 2703. In the former case, the sample sizes in the three NFI strata within the study area (Figure 1A) were 82, 569, and 250, and in the latter case, 246, 1708, and 749, respectively. For each forest variable to be estimated and for each estimator considered, we used the same set of samples of size n = 901 or n = 2703. In total, m = 10000 samples of each sample size were drawn.
The estimators of the population mean were evaluated with respect to root mean square error (RMSE), standard deviation (SD; also commonly referred to as the standard error), and bias, obtained with the m = 10000 repeated samples under the aforementioned stratified simple random sampling design. With denoting an estimator of a population mean , and denoting an estimate based on the ith sample, these quantities were computed as
and
For the ease of comparisons across variables, all values of bias, SD, and RMSE are presented as percentages of . That is, as
Likewise, let denote an estimate of the variance of based on the ith sample. For example, in the case of the HT estimator, is computed using formula (2). Then
is the value of an estimated standard deviation, using data from the ith sample, and presented as a percentage of the corresponding population mean. Let
where “ave″ denotes average. If is approximately equal to , then this suggests that the estimator of the standard deviation of is nearly unbiased.
For comparing the RMSE of one estimator (with auxiliary variables in their original scale) to the RMSE of another estimator (with power transformed auxiliary variables), the basic bootstrap confidence interval (e.g., Davison and Hinkley, 1997) for their difference is applied. Let and denote the two estimates based on sample i, where the first is based on auxiliary variables in the original scale while the other uses power transformed auxiliary variables. A bootstrap sample is taken as a random sample with replacement from . Based on the bootstrap sample, bootstrap replicates of the two estimated RMSEs are computed. Based on R = 9999 such bootstrap replicates, a basic bootstrap 95% confidence interval for the difference of the two RMSEs is computed using the boot.ci function in the R package boot (Davison and Hinkley, 1997). In these computations, all RMSEs are expressed as percentages of the corresponding population means. A 95% confidence interval that does not cover zero means that the use of power transformed auxiliary variables significantly changes the efficiency of the estimator at the 5% significance level. If the interval contains only positive values, the conclusion is that the transformations significantly improves the efficiency of the estimator at the 5% level. Thus, as in, for example, Samuels et al. (2012), if we find significant evidence for a change, our conclusion can be directional. Some authors prefer not to draw a directional conclusion in these cases (Samuels et al., 2012).
Results
The results for HT are presented in Table 4, i.e., the results for the case where no auxiliary data were used in the estimation. Since the HT estimator is unbiased, as expected, the values of (estimated) bias in Table 4 were close to zero. In addition, and also as expected, the values of were all close to the corresponding values of , suggesting that the estimator of the standard deviation of [i.e., the square root of the variance estimator (2)] is nearly unbiased.
When comparing the RMSEs in Table 4 with the RMSEs in Table 5 for the various model-assisted estimators based on an expert selection of auxiliary variables, notice that the use of assisting models and auxiliary information improved the efficiency of estimation. For volume of total growing stock, the reduction in RMSE was larger than 40% for each model-assisted estimator used and for both sample sizes considered. Moreover, the confidence intervals in Table 5 show that the use of data-driven choices of transformations of auxiliary variables significantly improved the RMSEs of the estimators. However, the improvements were quite small, except for Norway spruce, with reductions of RMSE by 7.7–10.0%. The performances of REG, LASSO, RIDGE, and ELNET were very similar.
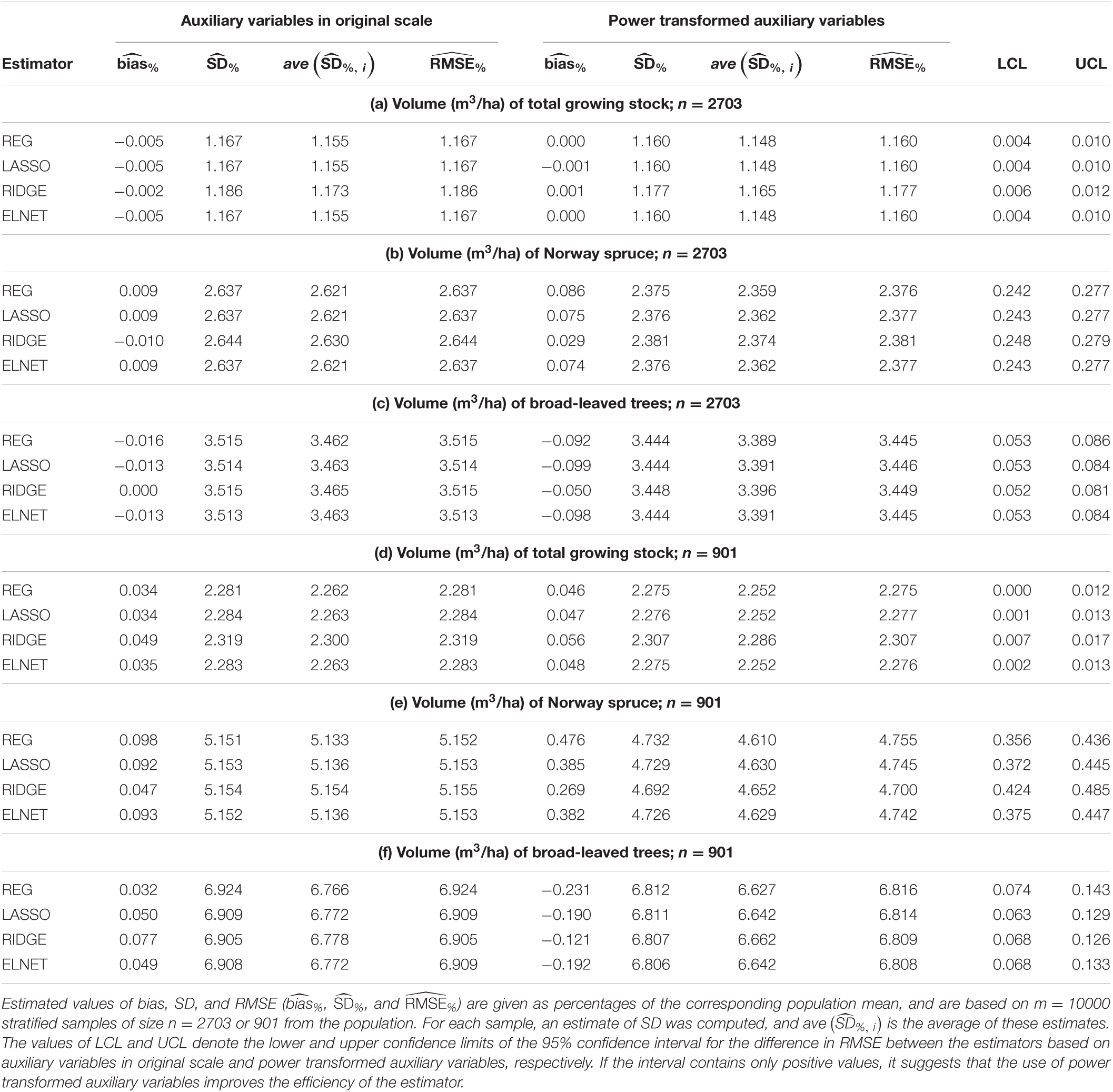
Table 5. Monte Carlo results for REG, LASSO, RIDGE, and ELNET, when based on an expert selection of auxiliary variables.
The results when all 72 available auxiliary variables in Table 2 were used are shown in Table 6. For REGi and the larger sample size, results are presented for the case where we excluded auxiliary variables with correlations above thresholds ± 0.90 and ± 0.95. When we tried ± 0.99 as threshold, then for many of the samples not all model coefficients could be estimated. For many samples of the smaller size (n = 901), this was the case even if the threshold was as low as ± 0.70. Therefore, no results for REGi were presented for the smaller sample size.
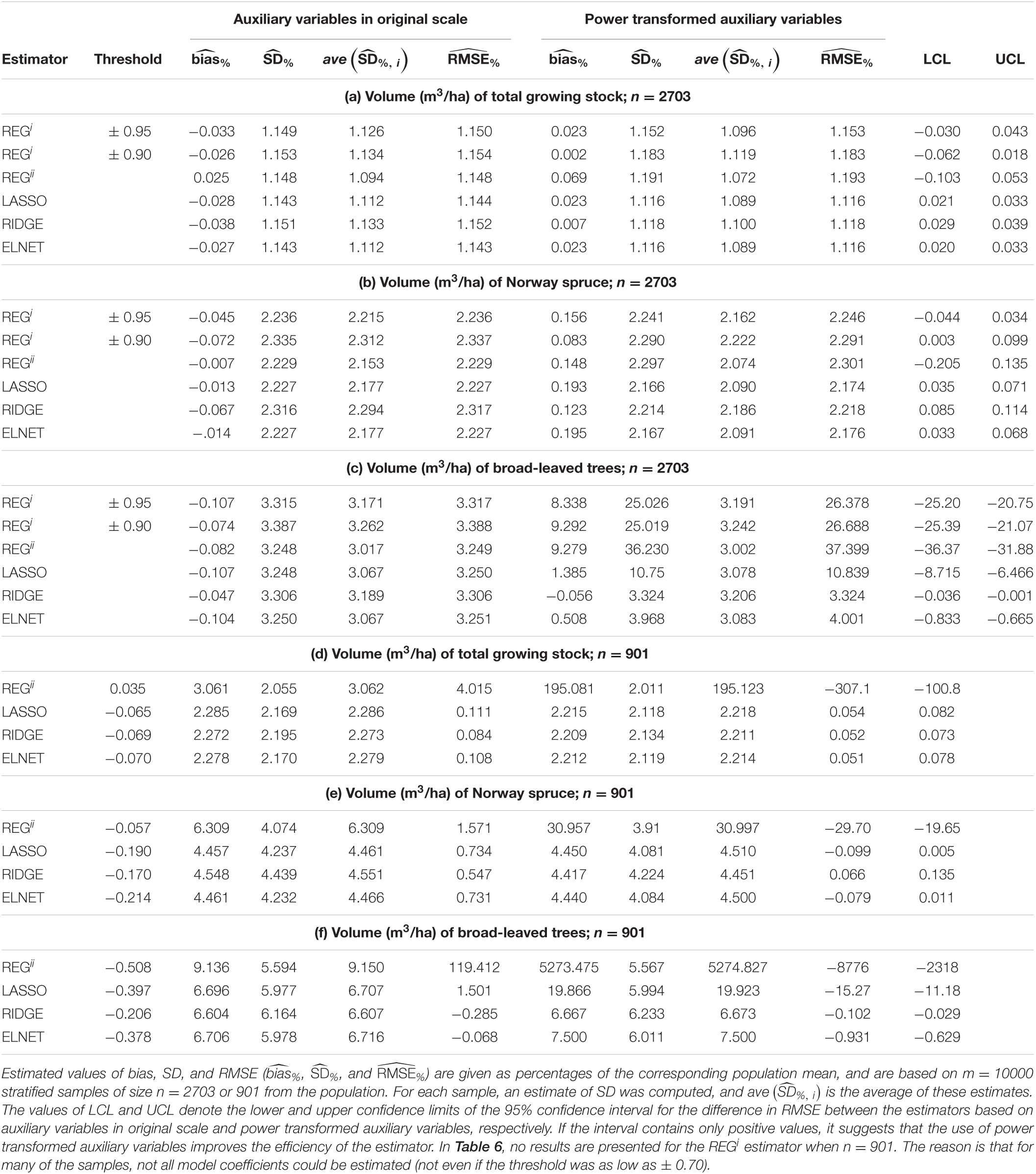
Table 6. Monte Carlo results for REGi, REGii, LASSO, RIDGE, and ELNET, when based on all auxiliary variables.
For the larger sample size (n = 2703), the estimators based on auxiliary data in their original scale in Table 6 had lower RMSEs than the corresponding estimators based on the smaller selection of auxiliary variables in Table 5. For example, for Norway spruce the RMSEs were about 15% lower and for broad-leaved trees about 7% lower, except for RIDGE where the gain was somewhat smaller. For the smaller sample size (n = 901) and LASSO, RIDGE, and ELNET, the corresponding reductions of RMSEs were 11% or larger for Norway spruce. For total growing stock and broad-leaved trees, the reduction was only 2 and 4%, respectively, for RIDGE, and even smaller than that for LASSO and ELNET. For the smaller sample size, REGii based on all the 72 auxiliary variables had RMSEs 22–34% larger than when using REG and a small expert selection of variables. For volume of broad-leaved trees, its performance was worse than the Horvitz-Thompson estimator.
The results for the larger sample size in Table 6 show that the estimators based on all available auxiliary variables in their original scale had about the same performance in terms of RMSE. The corresponding results for the smaller sample size show that LASSO, RIDGE, and ELNET were very close in terms of RMSE, and that they performed much better than REGii. More precisely, the latter estimator had RMSEs 34–41% larger than those for LASSO, RIDGE, and ELNET.
When for example estimating total growing stock volume (both sample sizes) or volume of Norway spruce (the larger sample size), the confidence intervals in Table 6 show that the use of data-driven choices of transformations of auxiliary variables significantly improved the RMSEs of LASSO, RIDGE, and ELNET. Although there were significant improvements when using transformations, the improvements in Table 6 were never larger than 5%. When estimating volume of broad-leaved trees using a large number of auxiliary variables, the data-driven method for selecting transformations did not perform well. For REGii and LASSO, the use of transformations sometimes resulted in extreme and unreasonable estimates of volume of broad-leaved trees, which in turn resulted in very large values of RMSE. This was also the case for the REGii estimator of total growing stock and volume of Norway spruce when using the smaller sample size. In comparison, RIDGE was quite robust against poor choices of transformations, and to a lesser degree, ELNET.
In Tables 5, 6, each value of is smaller than the corresponding value of . This implies that the estimated standard deviations, , i = 1, …, n, were somewhat too small, on average, which is quite typical in model-assisted estimation (cf. Kangas et al., 2016). As suggested by simulation results in McConville et al. (2020), it is better to estimate standard deviations (or variances) of model-assisted estimators by using a bootstrap method, especially as the number of explanatory variables grows. However, because of the additional computational burden generated by bootstrapping, we did not use this estimator in our study.
In summary for the larger sample size, when estimating total growing stock volume or volume of Norway spruce, the best results in terms of RMSE were obtained when using all available auxiliary variables. Here, for LASSO, RIDGE, and ELNET, the use of data-driven choices of transformations significantly improved the RMSEs, but the improvements were small. For volume of broad-leaved trees, LASSO, ELNET, and REGii based on all available auxiliary variables in their original scale produced the best results, and were slightly better than the corresponding REGi (with threshold ± 0.95) and RIDGE estimators. Finally, the use of data-driven choices of transformations was most successful when estimating volume of Norway spruce, using an expert selection of auxiliary variables. Here, the transformations reduced the RMSEs by up to 10%.
In summary for the smaller sample size, when estimating total growing stock volume or volume of Norway spruce, LASSO, RIDGE, and ELNET, with or without the use of data-driven choices of transformations, performed the best and were close in terms of RMSE. For volume of broad-leaved trees, LASSO, RIDGE, and ELNET with auxiliary variables in their original scale showed the best results. For all target variables, REGii based on all available auxiliary variables in their original scale had 34–41% higher RMSEs than the corresponding LASSO, RIDGE, and ELNET estimators, and performed worse in terms of RMSE than using REG and an expert selection of variables. For REGi it was often not possible to estimate the model coefficients. Data-driven choices of transformations reduced the RMSEs by about 8% for Norway spruce when using an expert selection of auxiliary variables. For all other cases, the transformations resulted in at best minor improvements of RMSE, and at worst very large increases of RMSE. Of the estimators considered, RIDGE, and to a lesser extent, ELNET, were found robust against poor choices of transformations.
Remark: In our population, 18% of the units (raster cells) had a height difference larger than 7 m between the field measured tree height and the 95th height percentile in the ALS data. We may consider these units as outliers, and we may ask ourselves: (i) Is it better to perform data-driven choices of transformations of auxiliary variables with these outliers present in the sample? (ii) Is it better to estimate the parameter vector β (after possible transformations of auxiliary variables) with these outliers present in the sample? In order to find out, we performed Monte Carlo simulations for each of the four possible combinations of answers to questions i and ii (No-No, Yes-No, Yes-Yes, or No-Yes), and for both the sample sizes, n = 2703 and n = 901. The case No-Yes is presented in Tables 5, 6. Results for all other possible cases are given in Supplementary Material. For each sample size and in terms of RMSE, it turned out that it was generally better to remove the outliers in the sample prior to performing data-driven choices of transformations, but to estimate the parameter vector β without removing the outliers in the sample of auxiliary variable data values (where variables may have been transformed before the estimation is performed). For auxiliary variables in their original scale, the following increases of RMSEs were obtained if outliers were removed before the parameter vector β was estimated: (a) 0.5–1.7% when the models were based on an expert selection of auxiliary variables; (b) 0.9–6.0% when using all available auxiliary variables and n = 2703; and (c) 1.4–68% when using all available auxiliary variables and n = 901. In (c), the increases of RMSEs were in the range 35–68% for REGii, but less than 9% for LASSO, RIDGE, and ELNET.
Discussion
In this paper, we have compared the performances of the Horvitz-Thompson estimator and several model-assisted estimators, using Monte Carlo simulations and real data, from the Swedish NFI, Sentinel-2, and a national laser scanning campaign. The model-assisted estimators were based either on modern prediction techniques (lasso, ridge, and elastic net regression), or on a traditional working model of multiple regression.
When based on an expert selection of a rather small set of auxiliary variables, the performances of the model-assisted estimators were quite similar in terms of RMSE. Our proposed data-driven method for finding suitable transformations of auxiliary variables was shown to improve the efficiency of these estimators. For Norway spruce, improvements by up to 10% were obtained. Rather than using an expert selection of a smaller set of auxiliary variables, it can be tempting to use auxiliary information contained in a larger set of variables. In such cases, a standard use of REG often fails due to (near) collinearity, and some auxiliary variables may need to be excluded before the estimate can be computed. We considered two different approaches of excluding “problematic” auxiliary variables, and the variant of the REG estimator that excluded as few variables as possible (the REGii estimator) provided the best results (with a few exceptions). The simulations showed that the efficiency in terms of RMSE improved when using the large set of auxiliary variables for LASSO, RIDGE, and ELNET, but that this was not necessarily the case for REG estimators. When estimating, for example, total growing stock volume (for both sample sizes considered) or volume of Norway spruce (for the larger sample size), the data-driven method for selecting transformations of auxiliary variables further improved the efficiency of LASSO, RIDGE, and ELNET. Although these improvements were statistically significant at the 5% level, they were all small.
When estimating total growing stock volume or volume of Norway spruce, LASSO, RIDGE, and ELNET based on the large set of auxiliary variables were the best in terms of RMSE. For the smaller sample size, they performed much better than the corresponding REGii estimator. For volume of broad-leaved trees, LASSO, RIDGE, and ELNET based on the large set of auxiliary variables in their original scale showed the best performance. Here, for the smaller sample size, they performed much better than REGii, which in this case had an RMSE even larger than the Horvitz-Thompson estimator.
The suggested data-driven choices of transformations performed the best when estimating volume of Norway spruce, using an expert selection of auxiliary variables, where they reduced the RMSEs by 7–10%. Although the transformations resulted in statistically significant reductions of RMSE in many other cases, too, these improvements cannot be regarded as practically significant. In addition, for the smaller sample size, the data-driven choices of transformations sometimes resulted in huge increases of RMSE, in particular when combined with REGii, and to a lesser degree with LASSO. In comparison, RIDGE (and to some extent also ELNET) was found to be quite robust against poor choices of transformations. Thus, the data-driven method for selecting transformations has not been proven promising enough to be recommended for the type of applications considered in this paper, except possibly in combination with RIDGE and ELNET.
Cook’s distance is a commonly used metric to indicate the influence of a data point when performing a multiple regression analysis. In an attempt to make the data-driven method more robust and in an additional simulation study not presented here, we disallowed transformations that caused an excessive increase in Cook’s distance. This improved the performance of the estimators of volume of broad-leaved trees, but it was still found that for broad-leaved trees it is better to use auxiliary variables in their original scale.
In our proposed data-driven method for finding suitable transformations, the transformation selection was done for one auxiliary variable at a time. To improve the method, and the efficiency of the resulting model-assisted estimators, one can use multivariable fractional polynomials, which simultaneously determine a functional form for continuous auxiliary variables and delete uninfluential auxiliary variables (Sauerbrei et al., 2006; Sauerbrei and Royston, 2017). For our simulation study, however, the additional computational burden of using multivariable fractional polynomials was considered too high. Another topic for further studies is the inclusion of interaction terms in the models. Except for one interaction term in the model for total growing stock volume based on an expert selection of auxiliary variables, only main effects were included in our models. Potentially, many interactions can be used. To avoid overfitting, and not only for models with interactions, a possibility is to use an information criterion, such as the Akaike information criterion (Akaike, 1974).
Although the methods might be further improved, our results indicate that model-assisted methods like LASSO, RIDGE, and ELNET could be used by the Swedish NFI to provide reliable estimates for smaller areas than possible using field data alone. Today, counties are the smallest unit for which the NFI present reliable estimates. The smallest area for which reliable results can be presented depends in large part on how the model-assisted estimators perform when using smaller sample sizes than the ones used in this study (n < 901). Thus, it remains to be investigated how small areas can be to produce reliable estimates of different forest variables with a sufficiently low RMSE.
A relatively large proportion of the units (raster cells) in our population (18%) had a difference between P95 and field measured tree height that was greater than 7 m. These units were considered as outliers. Many of them were units that were clear felled after the field survey, but before the laser scanning took place. The large proportion of outliers could also be a consequence of using merged split-plots for which the linkage with laser data is more sensitive to plot location errors compared to un-split plots. In the Monte Carlo study, it was found better to perform the data-driven choices of transformations without using these outliers in a sample, but to estimate model parameters with the outliers in a sample of auxiliary variable data values (where variables may have been transformed before the estimation is done). In addition to these outliers, there were additional units in the population with an unusual relationship between field data and laser metrics. This could be, for example, due to thinning cuttings, wind-thrown trees, and other changes. It was noticed that the proportion of such units was higher for plots with a high proportion of broad-leaved trees. To some extent, this can be an effect of using laser data acquired during leaf-off conditions, which gives lower laser density metrics for broad-leaved forests than using data acquired during leaf-on conditions (White et al., 2013). Although the number of such units was relatively low, they might have a large influence on the selection of transformations, and may explain why the use of data-driven choices of transformations was not successful when estimating volume of broad-leaved trees.
Data Availability Statement
The raw data supporting the conclusions of this article are available from the Dryad Digital Repository: doi: 10.5061/dryad.s4mw6m97k.
Author Contributions
ME conceived the study, was in charge of overall direction and planning, and carried out the Monte Carlo simulations. MN retrieved all data and contributed to the analysis with expertise in remote sensing. ME wrote the first draft of the manuscript, except for section “Materials and Methods,” written by MN. Both authors contributed to manuscript revision, read, and approved the submitted version. Both authors involved the participatory research process.
Funding
This research was financially supported by a research grant from the Swedish National Space Board.
Conflict of Interest
The authors declare that the research was conducted in the absence of any commercial or financial relationships that could be construed as a potential conflict of interest.
Publisher’s Note
All claims expressed in this article are solely those of the authors and do not necessarily represent those of their affiliated organizations, or those of the publisher, the editors and the reviewers. Any product that may be evaluated in this article, or claim that may be made by its manufacturer, is not guaranteed or endorsed by the publisher.
Acknowledgments
We acknowledge the Swedish National Forest Inventory for providing field data. We thank Håkan Olsson, Anton Grafström, guest associate editor BW, and two referees for their comments on the manuscript.
Supplementary Material
The Supplementary Material for this article can be found online at: https://www.frontiersin.org/articles/10.3389/ffgc.2021.764495/full#supplementary-material
References
Akaike, H. (1974). A new look at the statistical model identification. IEEE Trans. Automat. Control 19, 716–723. doi: 10.1109/TAC.1974.1100705
Ambler, G., and Banner, A. (2015). MFP: Multivariable Fractional Polynomials. R package version 1.5.2.
Baffetta, F., Corona, P., and Fattorini, L. (2010). Design-based diagnostics for k-nn estimators of forest resources. Can. J. For. Res. 41, 59–72. doi: 10.1139/X10-157
Baffetta, F., Fattorini, L., Franceschi, S., and Corona, P. (2009). Design-based approach to k-nearest neighbours technique for coupling field and remotely sensed data in forest surveys. Remote Sens. Environ. 113, 463–475. doi: 10.1016/j.rse.2008.06.014
Berk, R. A. (2016). Statistical Learning from a Regression Perspective, 2nd Edn. Cham: Springer International Publishing. doi: 10.1007/978-3-319-44048-4
Breidt, F. J., and Opsomer, J. D. (2016). Model-assisted survey estimation with modern prediction techniques. Stat. Sci. 32, 190–205. doi: 10.1214/16-STS589
Breidt, F. J., Claeskens, G., and Opsomer, J. D. (2005). Model-assisted estimation for complex surveys using penalised splines. Biometrika 92, 831–846. doi: 10.1093/biomet/92.4.831
Chambers, R., van den Brakel, J., Hedlin, D., Lehtonen, R., and Zhang, L.-C. (2006). Future challenges of small area estimation. Stat. Transit. 7, 759–769.
Chatterjee, S., Hadi, A. S., and Price, B. (2012). Regression Analysis by Example, 5th Edn. Hoboken, NJ: Wiley.
Chirici, G., McRoberts, R. E., Fattorini, L., Mura, M., and Marchetti, M. (2016). Comparing echo-based and canopy height model-based metrics for enhancing estimation of forest aboveground biomass in a model-assisted framework. Remote Sens. Environ. 174, 1–9. doi: 10.1016/j.rse.2015.11.010
Davison, A. C., and Hinkley, D. V. (1997). Bootstrap Methods and Their Application. Cambridge: University Press. doi: 10.1017/CBO9780511802843
Ekström, M., Esseen, P.-A., Westerlund, B., Grafström, A., Jonsson, B. G., and Ståhl, G. (2018). Logistic regression for clustered data from environmental monitoring programs. Ecol. Informatics 43, 165–173. doi: 10.1016/j.ecoinf.2017.10.006
Fridman, J., Holm, S., Nilsson, M., Nilsson, P., Ringvall, A. H., and Ståhl, G. (2014). Adapting national forest inventories to changing requirements – the case of the Swedish National Forest Inventory at the turn of the 20th century. Silva Fenn. 48:1095. doi: 10.14214/sf.1095
Gregoire, T., Ståhl, G., Næsset, E., Gobakken, T., Nelson, R., and Holm, S. (2011). Model-assisted estimation of biomass in a LiDAR sample survey in Hedmark county, Norway. Can. J. For. Res. 41, 83–95. doi: 10.1139/X10-195
Hastie, T., Tibshirani, R., and Friedman, J. (2009). The Elements of Statistical Learning: Data Mining, Inference and Prediction, 2nd Edn. New York, NY: Springer. doi: 10.1007/978-0-387-84858-7
Hastie, T., Tibshirani, R., and Wainwright, M. (2015). Statistical Learning with Sparsity: The Lasso and Generalizations. Boca Raton, FL: CRC Press. doi: 10.1201/b18401
Horvitz, D. G., and Thompson, D. J. (1952). A generalization of sampling without replacement from a finite universe. J. Am. Stat. Assoc. 47, 663–685. doi: 10.1080/01621459.1952.10483446
Hosking, J. R. M. (1990). L-moments: analysis and estimation of distributions using linear combinations of order statistics. J. R. Stat. Soc. Ser. B 52, 105–124. doi: 10.1111/j.2517-6161.1990.tb01775.x
James, G., Witten, D., Hastie, T., and Tibshirani, R. (2021). An Introduction to Statistical Learning, 2nd Edn. New York, NY: Springer. doi: 10.1007/978-1-0716-1418-1
Kangas, A., Myllymäki, M., Gobakken, T., and Næsset, E. (2016). Model-assisted forest inventory with parametric, semiparametric, and nonparametric models. Can. J. For. Res. 46, 855–868. doi: 10.1139/cjfr-2015-0504
McConville, K. S., Breidt, F. J., Lee, T. C. M., and Moisen, G. G. (2017). Model-assisted survey regression estimation with the lasso. J. Surv. Stat. Methodol. 5, 131–158. doi: 10.1093/jssam/smw041
McConville, K. S., Moisen, G. G., and Frescino, T. S. (2020). A tutorial on model-assisted estimation with application to forest inventory. Forests 11:244. doi: 10.3390/f11020244
McConville, K., Tang, B., Zhu, G., Cheung, S., and Li, S. (2018). Mase: Model-Assisted Survey Estimation. R package version 0.1.2.
McGaughey, R. J. (2020). FUSION/LDV: Software For LIDAR Data Analysis and Visualization. Available online at: http://forsys.cfr.washington.edu/fusion/ (accessed January 18, 2021).
McRoberts, R. E., Liknes, G., and Domke, G. M. (2014). Using a remote sensing-based, percent tree cover map to enhance forest inventory estimation. For. Ecol. Manag. 331, 12–18. doi: 10.1016/j.foreco.2014.07.025
Moser, P., Vibrans, A. C., McRoberts, R. E., Næsset, E., Gobakken, T., Chirici, G., et al. (2017). Methods for variable selection in LiDAR-assisted forest inventories. Forestry 90, 112–124. doi: 10.1093/forestry/cpw041
Naturvårdsverket (2020). Nationella Marktäckedata 2018, Basskikt – Produktbeskrivning. Utgåva 2.2, Naturvårdsverket. Available online at: http://gpt.vic-metria.nu/data/land/NMD/NMD_Produktbeskrivning_NMD2018Basskikt_v2_2.pdf (accessed November 26, 2021).
Nilsson, M., Nordkvist, K., Jonzén, J., Lindgren, N., Axensten, P., Wallerman, J., et al. (2017). A nationwide forest attribute map of Sweden predicted using airborne laser scanning data and field data from the National Forest Inventory. Remote Sens. Environ. 194, 447–454. doi: 10.1016/j.rse.2016.10.022
Opsomer, J. D., Breidt, F. J., Moisen, G. G., and Kauermann, G. (2007). Model-assisted estimation of forest resources with generalized additive models (with discussion). J. Am. Stat. Assoc. 102, 400–416. doi: 10.1198/016214506000001491
R Core Team (2020). R: A Language and Environment for Statistical Computing. Vienna: R Foundation for Statistical Computing.
Royston, P., and Altman, D. G. (1994). Regression using fractional polynomials of continuous covariates: Parsimonious parametric modelling. J. R. Stat. Soc. Ser. C 43, 429–467. doi: 10.2307/2986270
Sabanés Bové, D., and Held, L. (2011). Bayesian fractional polynomials. Stat. Comput. 21, 309–324. doi: 10.1007/s11222-010-9170-7
Samuels, M. L., Witmer, J. A., and Schaffner, A. A. (2012). Statistics for the Life Sciences, 4th Edn. Boston, FL: Prentice Hall.
Särndal, C.-E., Swensson, B., and Wretman, J. (1992). Model Assisted Survey Sampling. New York, NY: Springer. doi: 10.1007/978-1-4612-4378-6
Sauerbrei, W., and Royston, P. (2017). “The multivariable fractional polynomial approach, with thoughts about opportunities and challenges in big data,” in Big Data Clustering: Data Preprocessing, Variable Selection, And Dimension Reduction. WIAS Report 29, ed. H.-J. Mucha (Berlin: Weierstraß-Institut für Angewandte Analysis und Stochastik), 36–54.
Sauerbrei, W., Meier-Hirmer, C., Benner, A., and Royston, P. (2006). Multivariable regression model building by using fractional polynomials: description of SAS, STATA and R programs. Comput. Stat. Data Anal. 50, 3464–3485. doi: 10.1016/j.csda.2005.07.015
Ståhl, G., Saarela, S., Schnell, S., Holm, S., Breidenbach, J., Healey, S. P., et al. (2016). Use of models in large-area forest surveys: comparing model-assisted, model-based and hybrid estimation. For. Ecosyst. 3:5.
Tomppo, E., Heikkinen, J., Henttonen, H. M., Ihalainen, A., Katila, M., Mäkelä, H., et al. (2011). “Designing and conducting a forest inventory – case: 9th national forest inventory of finland,” in Managing Forest Ecosystems, Vol. 22, ed. K. von Gadow (Dordrecht: Springer). doi: 10.1007/978-94-007-1652-0
Torgo, L. (2010). Data Mining With R: Learning With Case Studies. Boca Raton, FL: Chapman and Hall/CRC. doi: 10.1201/b10328
Vaughan, T. S., and Berry, K. E. (2005). Using Monte Carlo techniques to demonstrate the meaning and implications of multicollinearity. J. Stat. Educ. 13, 1–9. doi: 10.1080/10691898.2005.11910640
White, J. C., Wulder, M. A., Vastaranta, M., Coops, N. C., Pitt, D., and Woods, M. (2013). The utility of image-based point clouds for forest inventory: a comparison with airborne laser scanning. Forests 4, 518–536.
Keywords: model-assisted estimation, generalized regression estimators, data-driven transformations, lasso, ridge, elastic net, forest inventory, remote sensing
Citation: Ekström M and Nilsson M (2021) A Comparison of Model-Assisted Estimators, With and Without Data-Driven Transformations of Auxiliary Variables, With Application to Forest Inventory. Front. For. Glob. Change 4:764495. doi: 10.3389/ffgc.2021.764495
Received: 25 August 2021; Accepted: 19 November 2021;
Published: 15 December 2021.
Edited by:
Barry Wilson, Northern Research Station, United States Forest Service, United States Department of Agriculture (USDA), United StatesReviewed by:
Michael Goerndt, Missouri State University, United StatesJacob Strunk, Pacific Northwest Research Station, United States Forest Service, United States Department of Agriculture (USDA), United States
Copyright © 2021 Ekström and Nilsson. This is an open-access article distributed under the terms of the Creative Commons Attribution License (CC BY). The use, distribution or reproduction in other forums is permitted, provided the original author(s) and the copyright owner(s) are credited and that the original publication in this journal is cited, in accordance with accepted academic practice. No use, distribution or reproduction is permitted which does not comply with these terms.
*Correspondence: Magnus Ekström, TWFnbnVzLkVrc3Ryb21Ac2x1LnNl