- College of Forestry, Hebei Agricultural University, Baoding, China
Tree productivity is not only determined by stand structure, but also influenced by soil chemical properties, climate and topography. However, the relative importance of each indicator on larch (Larix principis-rupprechtii) productivity were uncertain. In this study, 76 pure larch forest plots were selected based on national forest inventory (NFI) data in Hebei Province, China. Structural equation model (SEM) was used to analyze the direct and indirect effects of stand structure, soil chemical properties, climate and topography on larch productivity, and to quantify the relative importance of each indicator in determining productivity. The results showed that stem volume growth (SVG) of larch was influenced by a combination of stand density, diameter at breast height (DBH), mean winter snow (PAS), annual temperature range (TD), slope, and alkali-hydrolysis nitrogen (AN). SVG tended to increase with decreasing stand density and AN content and increasing DBH. Stand density, DBH and AN were more important than PAS, TD, and slope in explaining SVG variation. The results can provide a scientific basis for adaptive management of larch forests.
1 Introduction
Forests are a major component of terrestrial ecosystems, covering approximately one third of the earth’s land area and sequestering about two thirds of the carbon of terrestrial ecosystems annually (Jin et al., 2022). They play a crucial role in regulating the global carbon balance, reducing greenhouse gas concentrations and mitigating global warming (Li T. et al., 2022). Forest productivity is fundamental to studying nutrient cycling and energy flow in forest ecosystems, reflecting the relationship between forests and the environment, as well as the essential characteristics of community structure (Brown, 2002; Poorter and Sack, 2012), making it a core research area in forest ecosystem studies (Johnsen et al., 2001). Various factors, including stand structure, climate, topography, and soil chemical properties, influence tree growth, adaptability, and ultimately, forest productivity (Johnsen et al., 2001; Knoke et al., 2008; Wang et al., 2013; Zhao et al., 2015). Therefore, clarifying the relative importance of each factor’s impact on productivity is beneficial for guiding adaptive forest management.
Stand structure, particularly stand density, significantly impacts tree growth and productivity (Morin et al., 2011; Forrester et al., 2018; Lu et al., 2021). Different stand densities can lead to changes in community structure and soil environment, ultimately affecting stand productivity levels (Han et al., 2023). Higher stand density often results in intensified tree competition, as limiting space and resources and leading to decreased productivity and increased mortality rates (Kweon and Comeau, 2023). Additionally, tree size is an important factor affecting productivity, with larger trees generally exhibiting higher productivity (Yang et al., 2022; Zhang J. et al., 2022). This is attributed to medium-and large-diameter trees being more adept at occuping environmental resources compared to smaller trees, thereby positively impacting tree growth and resulting in higher productivity (Gersonde et al., 2004; Wright et al., 2014; Augusto et al., 2015). However, the positive relationship between medium-and large-diameter trees and productivity may also be influenced by stand age and density (Canham et al., 2004; Dong et al., 2021).
Forest productivity is influenced not only by stand structure, but also by environmental factors, including soil (Zhou et al., 2018), climate (Li et al., 2019), and topography (Li X. et al., 2022). Nitrogen is an essential element that limits plant growth in forest ecosystems (Li et al., 2012), particularly in boreal forests (Du et al., 2020). Alkali-hydrolysis nitrogen (AN) is an important indicator of soil nitrogen pool dynamics. It directly affects forest productivity (Geng et al., 1999) and is also considered the most effective indicator for evaluating soil fertility (Wang et al., 2018) and stand productivity (Jones et al., 2005). Climate directly affects forest productivity through changes in temperature and precipitation (Lü et al., 2019). The annual temperature range (TD), which measures the range of average daily temperature throughout the year, reflects environmental stability (Lin et al., 2009). TD has a significant impact on productivity (Gao et al., 2019), as a stable living environment can mitigate the adverse impacts of climate extremes on plants (Zhang and Lei, 2010). Moderate climate warming and increased precipitation are beneficial for enhancing the productivity of larch trees in northern China (Zhao et al., 2018; Lü et al., 2019). Topography, in conjunction with climate and soil chemical properties, plays a crucial role in forest productivity. In the context of stable climate change, temperature is identified as the key determinant of tree growth at lower elevations. However, as temperatures rise moderately, precipitation emerges as the dominant influence on tree growth in higher altitude areas (Li X. et al., 2022). Slope is also significantly related to productivity, which tends to initially increase and then decrease as the slope becomes steeper (Zhang Z. H. et al., 2022). This pattern may be attributed to the increased light energy received by stands on steeper slopes, promoting tree growth (Chen and Peng, 1996). Additionally, slope is closely linked to soil physical and chemical properties (Grimm et al., 2008).
Larch (Larix principis-rupprechtii) is a major silvicultural and timber species in northern China, offering various functional benefits (Ye et al., 2018). However, larch forests currently face several issues, including low productivity and ecological efficiency. While existing studies primarily focus on the unilateral effects of stand structure (Xu et al., 2023), climatic factors (Li et al., 2019), and soil physicochemical properties (Jones et al., 2005) on larch productivity, combined effects are less explored. Structural equation model (SEM) is a method for establishing, estimating and testing causal models (Jöreskog, 1978; Bentler, 1980). The model involves both observable explicit variables and potential variables that are not directly observable. To address these gaps, this study utilized national forest inventory (NFI) data and selected 76 pure larch stands in Hebei Province, China. The study aims to explore two key questions: (1) the pathways and magnitude of the effects of various indicator of stand structure on productivity, and (2) the relationship between abiotic factors (climate, topography and soil) and forest productivity. The results of the study can provide a scientific basis for the adaptive management of larch forests in northern China.
2 Study area and methodology
2.1 Study area
Hebei Province is located between 36°05′ N and 42°40′ N latitude, and 113°27′ E and 119°50′ E longitude. It has a terrain that is high in the northwest and low in the southeast. It has a temperate continental monsoon climate, characterized by four distinct seasons. The annual frost-free period ranges from 81 to 204 days and the average annual precipitation amounts to 484.5 mm. The distribution of precipitation follows a pattern of being higher in the southeast and lower in the northwest, with the average annual precipitation ranging from approximately 400 to 800 mm. In January, the average temperature drops below 3°C, while in July, temperatures range from 18°C to 27°C. Notably, the high-altitude areas exhibit average temperatures of −10.3°C in January and 23.4°C in July. In contrast, the low-altitude regions report average temperatures of −4.2°C in January and 27.1°C in July. Hebei Province has a relatively rich tree species diversity. The main tree species include Mongolian oak (Quercus mongolica), birch (Betula platyphylla), larch (Larix principis-rupprechtii) and so on.
2.2 Data collection
The data were obtained from the eighth and ninth NFI data. A systematic sampling method was used in NFI, dividing the province into a 4 km by 4 km grid and setting permanent sampling plots. It was surveyed every 5 years. Plot size was 20 m × 30 m. A total of 99 pure larch forest sample plots were surveyed. The plot data were checked and verified, excluding plots with incomplete data, to obtain valid plot data. Ultimately, a total of 76 plots were selected (Figure 1). Based on data from NFI and Forest Farm archives, the management approaches employed in larch plantations primarily consisted of near-natural management, structural management, and understory thinning. The main management objective was to optimize stand structure. The sampling plot data included tree measurement factors such as DBH, tree height, volume, elevation, stand age and dominant species (Table 1).
2.3 Environmental factors
To obtain temperature and precipitation data for the 76 sampling plots, the high-resolution climate model ClimateAP (Wang et al., 2016) was employed. This model utilized the latitude, longitude, and elevation data to generate the bioclimatic data. Additionally, GIS interpolation analysis method was applied to generate DEM based on vector data of contours and rivers in the study area. Subsequently, slope raster data with a resolution of 30 m × 30 m were extracted from the DEM. The soil data were mainly sourced from the soil feature dataset (Shangguan et al., 2013) with a spatial resolution of 30 arcsec. This dataset contained comprehensive information on commonly used soil physiochemical properties. A raster dataset of 12 soil factors was generated for the study area. In total, 17 environmental factors were initially selected, encompassing two climatic factors, three topographic factors, and 12 soil factors. Considering comprehensively, all environmental factors were resampled at 30 m × 30 m resolution.
To reduce the inter-correlation among environmental indicators and identify environmental factors significantly affecting stand volume growth (SVG), Pearson correlation analysis was performed on 17 environmental factors, three forest structure indices, and SVG. Finally, 10 variables with low intercorrelation (|r| < 0.7) and a significant impact on SVG (p < 0.05) were selected to build a linear regression model (Figure 2).
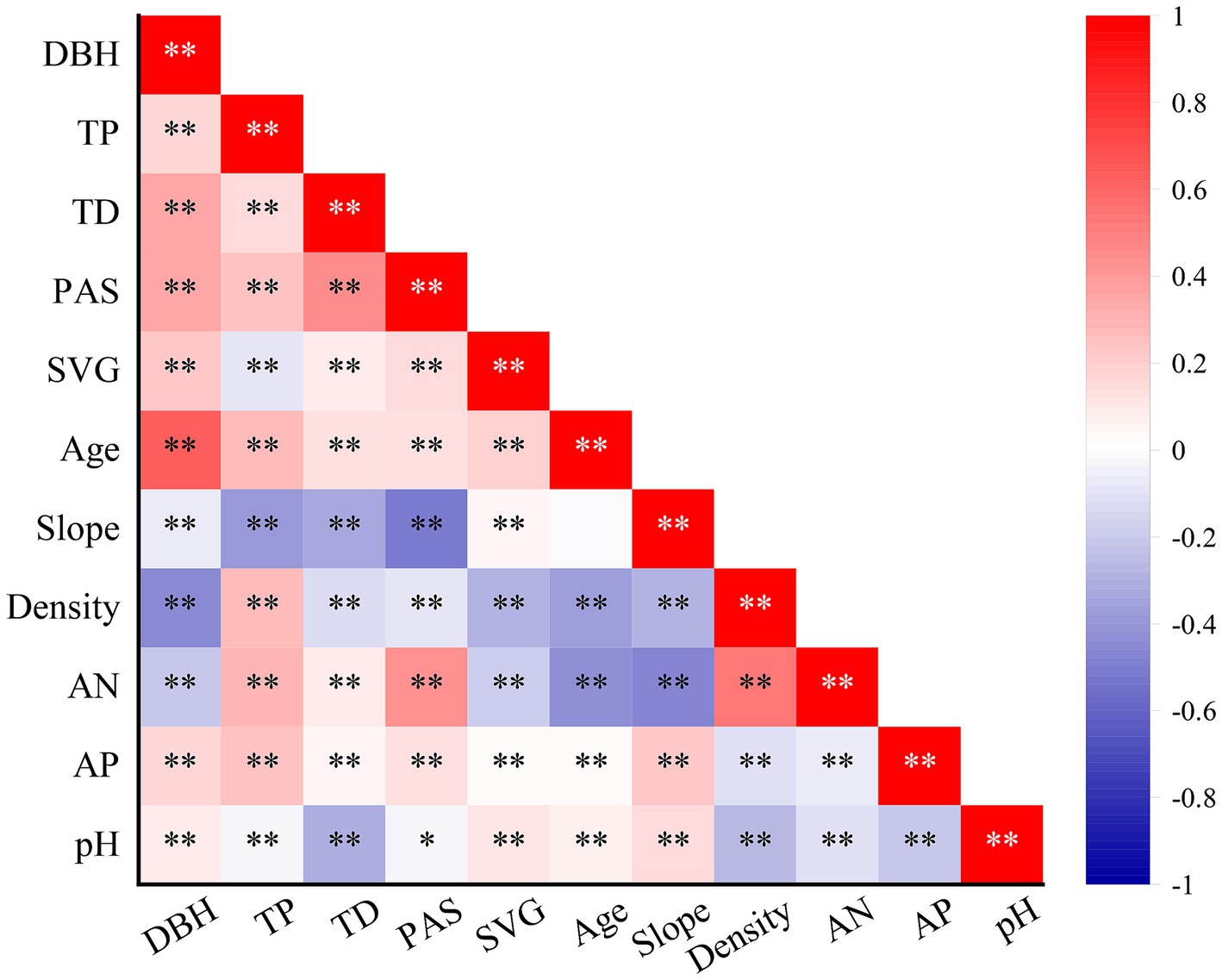
Figure 2. Relationship between ln (SVG) and environmental factors. AN, pH, AK, TP, AP, PAS, and TD denote alkali-hydrolysis nitrogen, potential of hydrogen, available potassium, total phosphorus, available phosphorus, mean winter snow and annual temperature range, respectively. *p < 0.05 and **p < 0.01.
2.4 Calculation of productivity
The volume of each tree was calculated using its DBH and H, while the stand volume was determined by summing the volumes of all trees in each plot. Stand volume growth was then calculated as the difference between two consecutive measurements. Only living trees that were remeasured at least once during both periods were included (Tian et al., 2022); thus, stand volume growth refers to living trees in a specific permanent plot. Therefore, SVG was used to quantify periodic annual increment of living stem volume in each plot and as an indicator to assess productivity, which was calculated based on Eq. 1 and Eq. 2:
where SVGi is the periodic annual increment of living stem volume in the ith sample plot (m3·a−1), is the stem volume of an individual tree (m3); DBH is the diameter at breast height of a single tree (cm); H is the tree height of a single tree (m); and are the living stem volume of an individual tree(j) at plot(i) at time t and t−T years (m3); T is the study period.
2.5 Construction of the linear regression model
Before conducting the regression analysis, the response variables were log-transformed to ensure normal distribution. The explanatory variables were standardized using the Z-score method. Multicollinearity, which can affect the explanatory and predictive power of variables, was assessed using the variance inflation factor (VIF). A VIF value below 10 was considered to ensure that there was no multicollinearity among the variables (Fox and Monette, 1992; Fox, 2008).
The response variable used in this study was SVG. Explanatory variables included factors related to stand structure, topography, soil chemical properties, and climate variables. A linear regression model was constructed using SVG as the response variable, and the importance of each indicator was evaluated using the relative weight indicator (RW). The RW indicates the contribution of each indicator to the model’s R2, considering both its individual contribution and its joint effect with other variables (Johnson, 2004; LeBreton and Tonidandel, 2008). The importance of these predictors in the regression equation was determined through a transformation process that yielded a set of orthogonal variables corresponding to each predictor. The relative weight (1) was calculated according to Eq. 3 (Li et al., 2019). The regression weight vector (β) of the correlated orthogonal variables and the target variable, and the regression coefficient matrix (I) were obtained. By calculating the squares of the elements in β and I (i.e., and ), the relative weight (1) of the first variable could be determined.
2.6 Structural equation model construction
SEM is a statistical technique that assesses direct and indirect effects between variables, tests the statistical significance of the model, and provides an accurate description of the data (Sande et al., 2017). In this study, SEM was constructed to analyze the relationships between stand structure, soil chemical properties, climate, topographic variables, and larch SVG (Figure 3). Based on the linear regression model and RW analysis, variables with higher RW were selected for inclusion in the SEM. The SEM was fitted using the maximum likelihood method. The model’s accuracy was evaluated using the chi-square freedom ratio (χ2/df), comparative fit index (CFI), root mean square error of approximation (RMSEA), and p-value (Howard, 2013). Generally, χ2/df value of 1–3 is considered acceptable, while CFI >0.9, RMSEA <0.08, and p > 0.05. The standardized coefficients of each pathway in the model were utilized to quantify the relative importance of different factors affecting larch SVG. This indicator allowed for the quantification of the direct, indirect and total standardized effects of each variable on the larch SVG (Edwards and Lambert, 2007).
2.7 Statistical analysis
One-way ANOVA was used to compare the differences in SVG among different stand structures and environmental factors. SEM was employed to demonstrate the coupling effects of various indicators on productivity. All the above analyses were performed using R 4.2.2 (R Development Core Team, 2023).
3 Results
3.1 Relative importance of variables
A linear regression model was constructed for each indicator and SVG (Figure 4A). The relative importance of each variable was determined based on the relative weight in the linear regression model (Figure 4B). The following factors with larger RW were selected and included in the SEM: Two stand structures (density, DBH), one soil factor (AN), two climate factors (PAS, TD), and one topographic factor (slope).
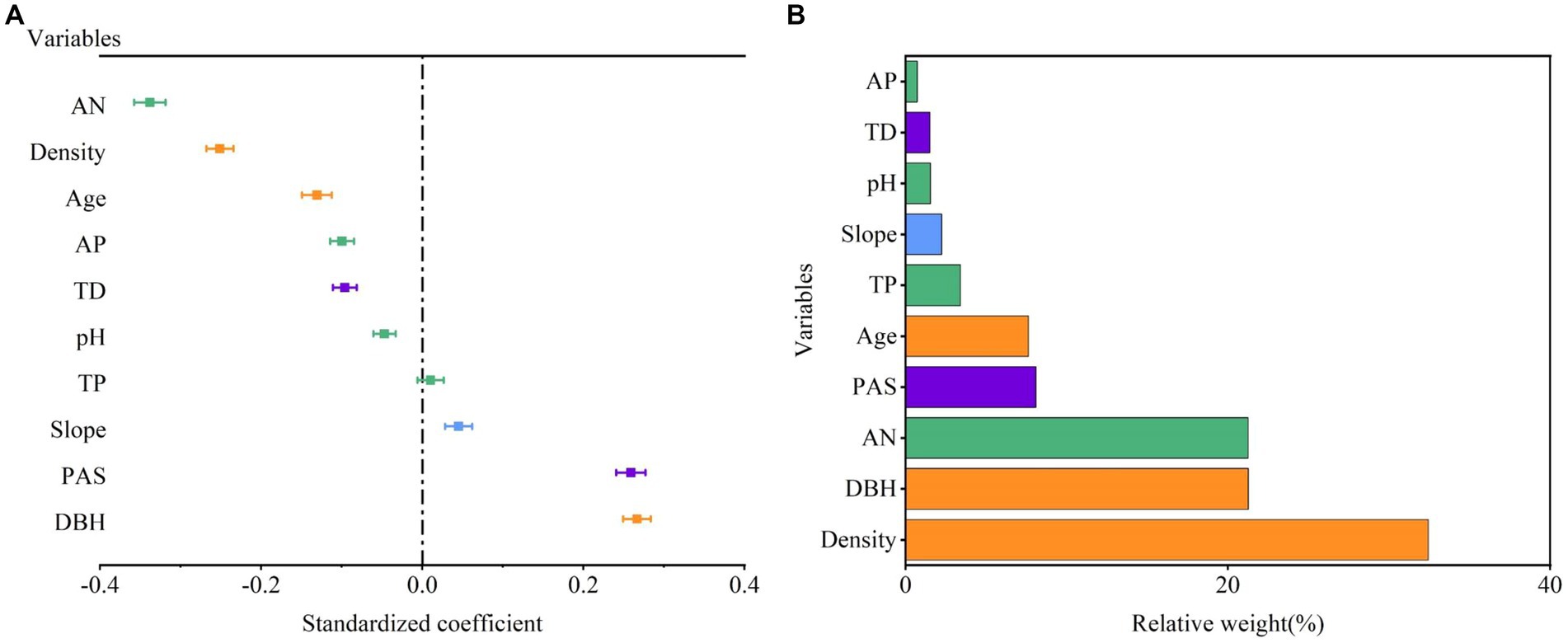
Figure 4. Results of multiple regression model selection. (A) The effects of stand structure, climate, soil and topography indicators on SVG. (B) The relative weights of each indicator.
3.2 Effect of stand structure and environmental factors on SVG
Stand density and DBH significantly influenced SVG (p < 0.05). Specifically, SVG showed a significant decreasing trend with increasing stand density. Conversely, SVG exhibited a significant increasing trend with increasing DBH. SVG increased significantly with increasing slope, reaching its maximum at 21–30° (p < 0.05). SVG decreased significantly with increasing soil AN content (p < 0.05). SVG at precipitation accumulation of 60–89.99 mm was significantly greater than that at <59.99 mm (p < 0.05). Additionally, the SVG in the temperature range of 32–33.99°C was significantly higher than that in the range of 34–35.99°C (p < 0.05) (Figure 5).
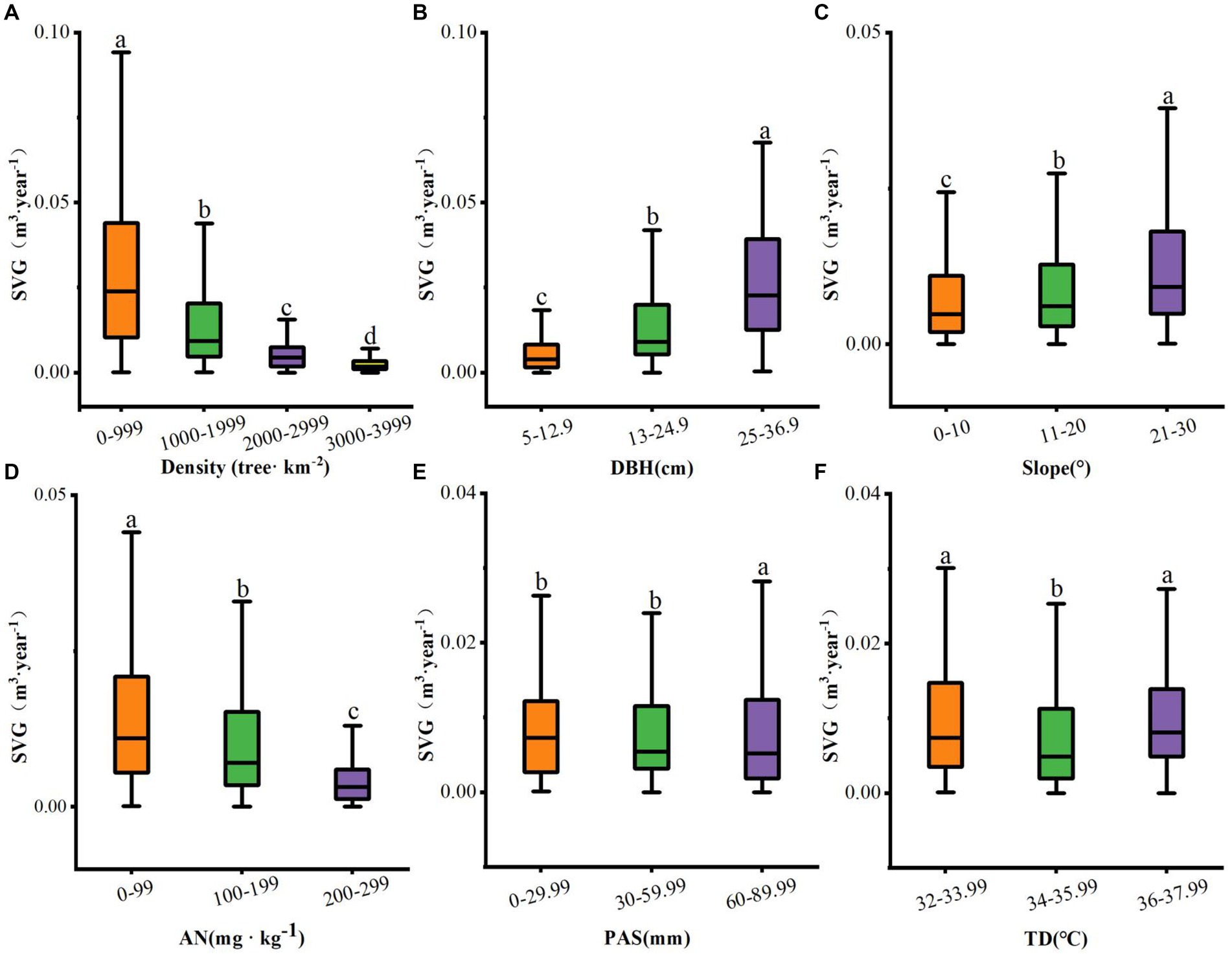
Figure 5. Differences in SVG under different stand densities (A), DBH (B), slope (C), AN (D), PAS (E), and TD (F). Different lowercase letters indicate significant differences among indicators (p < 0.05).
3.3 Structural equation model
The SEM was constructed using selected parameters (Figure 6). The χ2/df, CFI, RMSEA, and p-values of the SEM were 1.716, 1, 0.012, and 0.190, respectively, indicating the predictive results was acceptable. The model showed that all the variables together accounted for 32.6% of the variation in larch SVG. Stand density had a negative influence on SVG, indicated by a path coefficient of −0.260, while DBH exhibited a positive correlation with SVG, with a path coefficient of 0.180. Stand density had a more significant direct and indirect effect on SVG than DBH (Figures 6, 7A). Among the climate factors, TD was negatively correlated with SVG, represented by a path coefficient of −0.065. PAS had a positive effect on SVG, with a path coefficient of 0.200. Furthermore, PAS had a higher positive effect on SVG compared to TD (Figures 6, 7A). While slope did not have a direct effect on SVG, it exerted an indirect influence by affecting soil AN (Figures 6, 7A). AN had a negative relationship, with a path coefficient of −0.279 (Figures 6, 7A). Stand density and AN had the largest contribution to SVG, followed by DBH, PAS, slope, and TD in pure larch stands (Figure 7B).
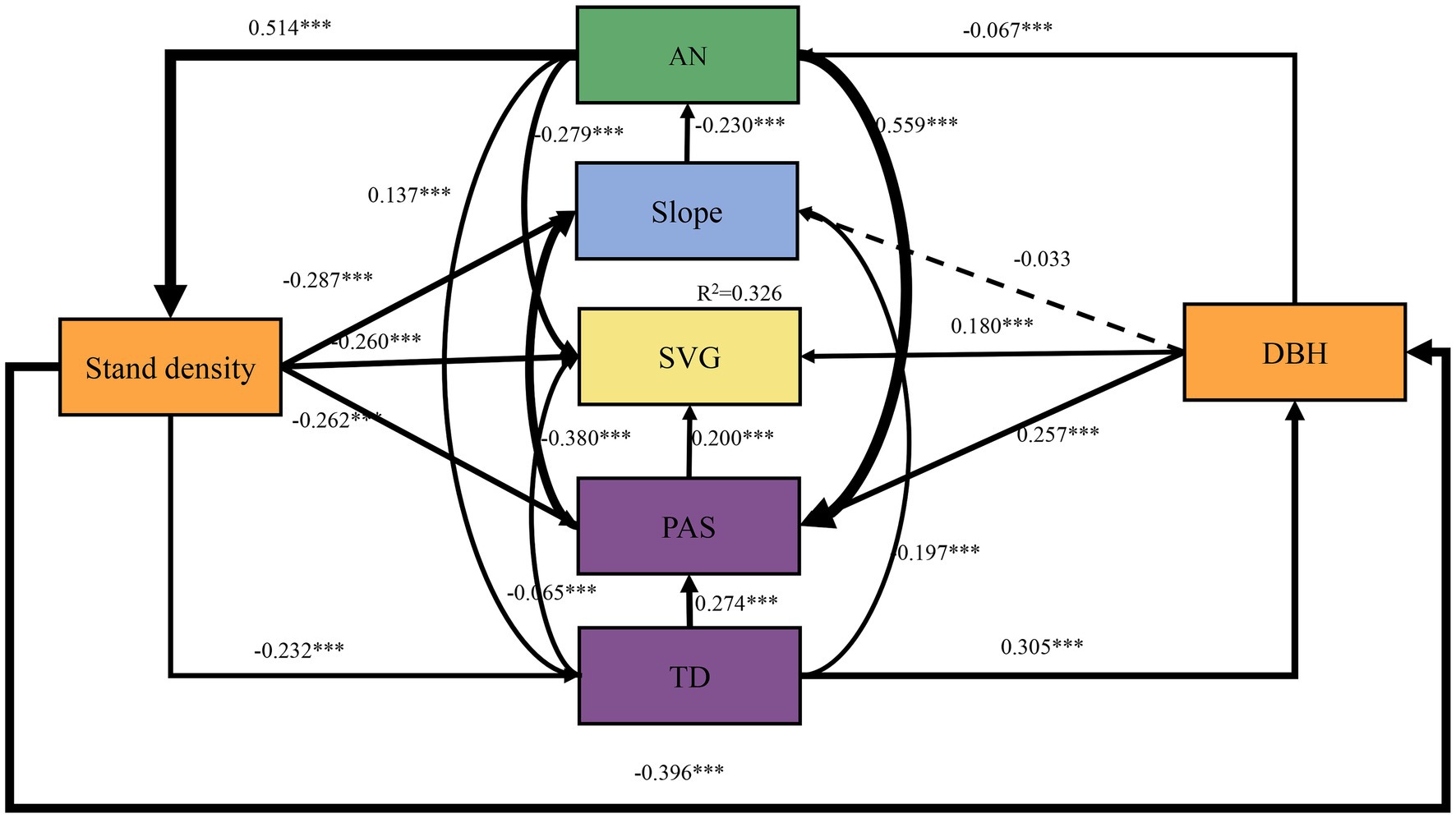
Figure 6. Results of SEM analysis of the effects of stand structure (stand density and DBH), topography (slope), climate (PAS and TD), and soil chemical properties (AN) on SVG in larch forests. The values next to the arrows are standardized path coefficients with corresponding statistical significance. R2 indicates the total variation in the dependent variable explained by the combined independent variables. ***p < 0.001. In the figure, the solid line indicates a significant effect, the dashed line indicates a non-significant effect, and the thickness of the line indicates the size of the standardized coefficient.
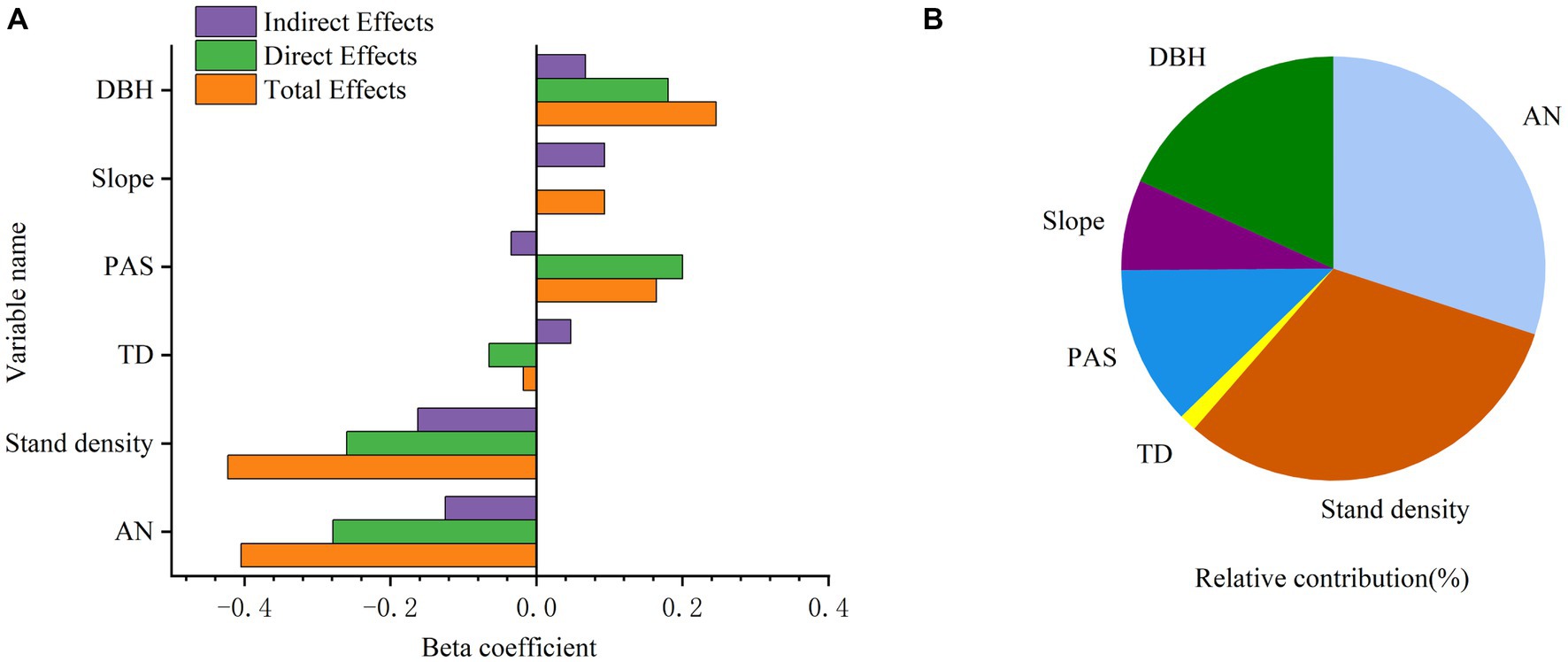
Figure 7. Direct, indirect and total effects of stand structure (stand density and DBH), topography (slope), climate (PAS and TD), and soil chemical properties (AN) on SVG of larch (A) and the total relative contribution of each factor to SVG (B).
4 Discussion
4.1 Relationship between stand structure and productivity
This study found a significant negative correlation between productivity in larch forests and stand density within a given range, irrespective of abiotic factors. These findings are consistent with previous studies (Magruder et al., 2013; Tun et al., 2018). Lower stand density reduces competition among individual trees in the stand (Dong et al., 2021) and allows for increased resources allocation to individual tree, thereby enhancing stand productivity. However, excessively low stand density can lead to stand structure damage, soil erosion, and detrimental effects on tree growth (Powers et al., 2010). In addition, the impact of stand density on productivity was also affected by the soil physicochemical properties and tree size. Excessively high or low stand densities can cause soil degradation and acidification, adversely affecting the normal growth of trees (Zhao et al., 2019). Different stand densities can lead to distinct patterns of tree DBH distribution (Forrester, 2019), which can have either positive (Dănescu et al., 2016) or negative (Bourdier et al., 2016) effects on productivity. Similar results were obtained in this study, that is, stand density exhibited a significant negative correlation with pH and DBH (Figures 2, 6).
Tree size, generally represented by DBH, served as an important indicator of productivity. The results of this study demonstrated a significant positive correlation between DBH and larch productivity. These results align with the findings of Bordin et al. (2021), indicating a positive correlation between DBH and productivity. This relationship can be attributed to the vertical and horizontal stratification structure within the canopy layer, which allows larger trees to occupy dominant positions and gain more access to light, water and nutrients. Consequently, larger trees exhibit enhanced competitive abilities, leading to increased stand productivity (Bordin et al., 2021). However, it has also been found that larch productivity shows a trend of increasing and then decreasing with increasing DBH (Hui et al., 2012). This phenomenon may be attributed to previous studies considering the effect of stand age on productivity, as the positive relationship between tree size and productivity diminishes with increasing stand age (Canham et al., 2004; Zhao et al., 2015). In this study, stand age was not included in the SEM as its contribution to productivity was significantly lower than that of stand density and DBH. Moreover, large trees can positively influence carbon sequestration through the regulation of above-and below-ground resource allocation, facilitated by abundant roots and branches (Luyssaert et al., 2008; Lutz et al., 2012). In northern China, larch forests serve as major potential carbon sinks and play an important role in timber production. Therefore, studying these important stand structures can provide valuable insights for forestry production and sustainable forest management.
4.2 Relationship between abiotic factors and productivity
Temperature and precipitation are crucial climatic indicators that significantly influence productivity (Ratcliffe et al., 2016; Schaphoff et al., 2016). In this study, two climate factors, PAS and TD, were selected and analyzed through correlation analysis and SEM to explore their relationship with productivity and the underlying mechanisms. The results showed that PAS had a positive impact on productivity, consistent with previous studies (Cui and Xiang, 2017). This positive relationship can be attributed to the contribution of sufficient snowfall, which provides abundant water supply to plants during the warming period when snow melts, thereby promoting an increase in productivity (Kholdaenko et al., 2023). This interpretation is indirectly supported by the finding that isolated precipitation treatments lead to a significant reduction in productivity (Zhong et al., 2016). On the other hand, TD was significantly negatively correlated with productivity, which is consistent with earlier findings (Zhang et al., 2018). This negative relationship can be attributed to the more pronounced seasonal temperature fluctuations associated with higher TD values (Zhang and Lei, 2010). Higher temperature differences in a given year can lead to extreme weather events that are unfavorable for tree growth. Our study found that the cumulative temperature above 5°C was higher in the plots with TD of 34–35.99°C compared to those with TD of 32–33.99°C and 36–37.99°C. This observation may explain the higher productivity in the former one, as cumulative temperature is an important factor influencing plant growth and development (Zheng et al., 2021). Additionally, the study found that the effect of PAS on productivity was significantly higher than that of TD. This difference may be attributed to the study area’s high-altitude nature, where precipitation plays a dominant role in affecting tree growth (Li X. et al., 2022).
Soil chemical properties are essential indicators to characterize soil fertility and have a direct impact on tree growth and forest productivity (Zhang et al., 2019). Nitrogen content in the soil is a critical measures of soil fertility, and the selection of key soil indicators varies based on research objectives. Previous studies have suggested that temperate forests are primarily limited by nitrogen supply (Fyllas et al., 2009; LeBauer and Treseder, 2008). In this study, after a comparative analysis of variables, AN was chosen as an indicator to assess its impact on productivity. The results showed a significant and negative relationship between productivity and AN. This negative correlation could be attributed to the adverse spillover effects of high AN content on other soil factors. For instance, an excessive amount of AN may lead to soil acidification and the dilution and loss of other trace elements, adversely affecting tree growth and ultimately decrease productivity (Fan et al., 2007). Previous nitrogen deposition experiments indirectly support this conclusion, suggesting that an overabundance of nitrogen in the soil could reduce AP and hinder the mineralization of soil organic matter (Cai et al., 2016), thereby impacting tree growth. However, it is worth noting that research by Zhou et al. (2005) showed a positive correlation between soil AN content and productivity, while studies by Gower and Son (1992) and Gower et al. (1993) found no correlation between productivity and AN. These findings diverge from the results of the current study, possibly due to differences in forest types, soil types, and geographical gradients across various studies. Additionally, the widespread high AN content in the study area may exert negative impacts on the soil, inhibiting tree growth and leading to a negative correlation between AN and productivity.
The effect of slope on productivity was investigated in this study, revealing no significant direct relationship between slope and larch productivity in Hebei Province. Instead, slope primarily influenced productivity indirectly by affecting AN (based on direct effects). Nonetheless, a positive correlation (based on total effects) between slope and productivity was observed, as shown in the linear regression model and correlation analyses presented earlier. The study found that the highest productivity occurred on slopes between 20° and 30°. This finding is consistent with previous findings. For instance, Zhang Z. H. et al. (2022) and Tian (2018) reported optimal slope ranges for larch productivity between 20° to 27° and 21° to 30°, respectively. Both studies showed that tree growth is markedly inhibited on slopes exceeding 30°. The suppression of productivity on steeper slopes may stem from several factors. Up to a slope of 30°, increased slope enhances sunlight exposure in the canopy, thereby augmenting the trees’ capacity to absorb and fix CO2 (Chen and Peng, 1996), which in turn boosts tree productivity. Conversely, flat terrains lead to shading and waterlogging issues, while steep slopes cause soil erosion and reduced soil fertility, both detrimental to tree growth. Additionally, while some studies (e.g., Li et al., 2016) have reported larch productivity decreasing with increasing slope, our findings differ, possibly due to lower altitude and higher temperatures at previous study sites, mitigating thermal variations’ impact on tree growth. However, the growth-limiting effects of thinning soil layers and soil erosion due to increased slope are still significant and evident.
5 Conclusion
In this study, the relationship between stand structure, soil chemical properties, climate, topography, and larch productivity was explored using a structural equation model based on NFI data in Hebei Province, China. The SEM model indicates that in pure larch forests, AN has the highest impact on productivity, followed by stand density, PAS, DBH, TD, and Slope (based on direct effects). However, when considering the interactions among these factors, the results differ, with stand density having the highest contribution, followed by AN, DBH, PAS, Slope, and TD (based on total effects). This suggests that the effects of various factors on productivity in larch forest ecosystems are not simply positively or negatively correlated, but rather involve a combination of multiple factors with direct or indirect relationships, as well as facilitative or inhibitory effects. Therefore, the application of SEM provides a deeper understanding of the relationships between these factors, offering a theoretical foundation for enhancing forest productivity and formulating management strategies. However, the study has certain limitations due to the selection of representative factors from stand structure, soil properties, and climatic factors, potentially overlooking the roles of other factors. Consequently, further analysis of the interactions among the remaining indicators and their interplay with productivity is highlighted as a key area for future research. In practical forestry production, it is essential to consider the interplay between stand structure, climate, soil chemical properties, topography, and stand growth. Implementing appropriate management approaches, such as controlling stand density conducive to tree growth and applying appropriate fertilization, can significantly enhance the productivity of larch in Hebei Province.
Data availability statement
The raw data supporting the conclusions of this article will be made available by the authors, without undue reservation.
Author contributions
ZZ, YW, and NF contributed to the study design and performed the formal analysis. YW performed the software analysis and wrote the first draft of the manuscript. JQ, JZ, ZG, and CL contributed data curation. ZZ contributed to the writing, review and editing. All authors contributed to the article and approved the submitted version.
Funding
The author(s) declare that financial support was received for the research, authorship, and/or publication of this article. This work was funded by the State Key Research and Development Program, Grant No. 2023YFD2200803, the National Natural Science Foundation of China, Grant No. 32071759; the Natural Science Foundation of Hebei Province, China, Grant No. C2020204026, the Hebei Province Forest and Grass Science and Technology Demonstration Project, Grant No. TG [2022]018 and the Hebei Province Key R & D Program of China, Grant No. 22326803D.
Acknowledgments
The authors greatly appreciate everyone who helped with the field survey and the reviewers for their valuable comments.
Conflict of interest
The authors declare that the research was conducted in the absence of any commercial or financial relationships that could be construed as a potential conflict of interest.
Publisher’s note
All claims expressed in this article are solely those of the authors and do not necessarily represent those of their affiliated organizations, or those of the publisher, the editors and the reviewers. Any product that may be evaluated in this article, or claim that may be made by its manufacturer, is not guaranteed or endorsed by the publisher.
References
Augusto, L., De Schrijver, A., Vesterdal, L., Smolander, A., Prescott, C., and Ranger, J. (2015). Influences of evergreen gymnosperm and deciduous angiosperm tree species on the functioning of temperate and boreal forests. Biol. Rev. 90, 444–466. doi: 10.1111/brv.12119
Bentler, P. M. (1980). Multivariate analysis with latent variables: causal modeling. Annu. Rev. Psychol. 31, 419–456. doi: 10.1146/annurev.ps.31.020180.002223
Bordin, K. M., Esquivel-Muelbert, A., Bergamin, R. S., Klipel, J., Picolotto, R. C., Frangipani, M. A., et al. (2021). Climate and large-sized trees, but not diversity, drive above-ground biomass in subtropical forests. Forest. Ecol. Manag. 490:119126. doi: 10.1016/j.foreco.2021.119126
Bourdier, T., Cordonnier, T., Kunstler, G., Piedallu, C., Lagarrigues, G., and Courbaud, B. (2016). Tree size inequality reduces forest productivity: an analysis combining inventory data for ten European species and a light competition model. PLoS One 11:e0151852. doi: 10.1371/journal.pone.0151852
Brown, S. (2002). Measuring carbon in forests: current status and future challenges. Environ. Pollut. 116, 363–372. doi: 10.1016/S0269-7491(01)00212-3
Cai, Q. K., Duan, H. L., Liu, W. F., Wu, J. P., Yuan, Y. H., and Fan, H. B. (2016). Impacts of nitrogen deposition on soil available N and P contents in the Chinese fir plantation. J. For. Environ. 36, 342–348. doi: 10.13324/j.cnki.jfcf.2016.03.015
Canham, C. D., LePage, P. T., and Coates, K. D. (2004). A neighborhood analysis of canopy tree competition: effects of shading versus crowding. Can. J. For. Res. 34, 778–787. doi: 10.1139/x03-232
Chen, C. G., and Peng, H. (1996). Standing crops and productivity of the major forest-types at the Huoditang forest region of the Qinling Mountains. J. Northwest For. Coll. 11, 92–102.
Cui, S. M., and Xiang, W. (2017). Effects of thinning and climate factors on Larix olgensis tree-ring width. Sci. Silv. Sin. 53, 1–11. doi: 10.11707/j.1001-7488.20171201
Dănescu, A., Albrecht, A. T., and Bauhus, J. (2016). Structural diversity promotes productivity of mixed, uneven-aged forests in southwestern Germany. Oecologia 182, 319–333. doi: 10.1007/s00442-016-3623-4
Dong, X. T., Zhang, J., Zhang, Z. D., and Huang, X. R. (2021). Effects of tree species interaction, stand density, and tree size on the productivity of Larix principis-rupprechtii. Chin. J. Appl. Ecol. 32, 2722–2728. doi: 10.13287/j.1001-9332.202108.006
Du, E., Terrer, C., Pellegrini, A. F. A., Ahlström, A., van Lissa, C. J., Zhao, X., et al. (2020). Global patterns of terrestrial nitrogen and phosphorus limitation. Nat. Geosci. 13, 221–226. doi: 10.1038/s41561-019-0530-4
Edwards, J. R., and Lambert, L. S. (2007). Methods for integrating moderation and mediation: a general analytical framework using moderated path analysis. Psychol. Methods 12, 1–22. doi: 10.1037/1082-989X.12.1.1
Fan, H. B., Liu, W. F., Li, Y. Y., Liao, Y. C., Yuan, Y. H., and Xu, L. (2007). Tree growth and soil nutrients in response to nitrogen deposition in a subtropical Chinese fir plantation. Acta Ecol. Sin. 27, 4630–4642. doi: 10.3321/j.issn:1000-0933.2007.11.031
Forrester, D. I. (2019). Linking forest growth with stand structure: tree size inequality, tree growth or resource partitioning and the asymmetry of competition. For. Ecol. Manag. 447, 139–157. doi: 10.1016/j.foreco.2019.05.053
Forrester, D. I., Ammer, C., Annighöfer, P. J., Barbeito, I., Bielak, K., Bravo-Oviedo, A., et al. (2018). Effects of crown architecture and stand structure on light absorption in mixed and monospecific Fagus sylvatica and Pinus sylvestris forests along a productivity and climate gradient through Europe. J. Ecol. 106, 746–760. doi: 10.1111/1365-2745.12803
Fox, J. (2008). Applied regression analysis and generalized linear models. London: SAGE Publications, Inc.
Fox, J., and Monette, G. (1992). Generalized collinearity diagnostics. J. Am. Stat. Assoc. 87, 178–183. doi: 10.1080/01621459.1992.10475190
Fyllas, N. M., Patiño, S., Baker, T. R., Bielefeld Nardoto, G., Martinelli, L. A., Quesada, C. A., et al. (2009). Basin-wide variations in foliar properties of Amazonian forest: phylogeny, soils and climate. Biogeosciences 6, 2677–2708. doi: 10.5194/BG-6-2677-2009
Gao, R. N., Xie, Y. S., Lei, X. D., Lu, Y. C., and Sui, X. Y. (2019). Study on prediction of natural forest productivity based on random forest model. J. Cent. South Univ. For. Technol. 39, 39–46. doi: 10.14067/j.cnki.1673-923x.2019.04.008
Geng, Y. Q., Sun, X. Y., Kang, X. G., Tan, X., Zhao, Y. Q., Li, D. L., et al. (1999). Soil fertility of different Forest types in the Changbai Mountains. J. Beijing For. Univ. 21, 101–105. doi: 10.13332/j.1000
Gersonde, R., Battles, J. J., and O’Hara, K. L. (2004). Characterizing the light environment in Sierra Nevada mixed-conifer forests using a spatially explicit light model. Can. J. For. Res. 34, 1332–1342. doi: 10.1139/x04-013
Gower, S. T., Reich, P. B., and Son, Y. (1993). Canopy dynamics and above ground production of five tree species with different leaf longevities. Tree Physiol. 12, 327–345. doi: 10.1093/treephys/12.4.327
Gower, S. T., and Son, Y. (1992). Differences in soil and leaf litter-fall nitro gen dynamics for five forest plantations. Soil Sci. Soc. Am. J. 56, 1959–1966. doi: 10.2136/SSSAJ1992.03615995005600060051X
Grimm, R., Behrens, T., Märker, M., and Elsenbeer, H. (2008). Soil organic carbon concentrations and stocks on Barro Colorado Island—digital soil mapping using random forests analysis. Geoderma 146, 102–113. doi: 10.1016/j.geoderma.2008.05.008
Han, X. S., Xu, H., Guo, Y. Z., Zhang, Y. R., Mei, S. G., Wang, S. G., et al. (2023). Influence of stand density on growth and stand volume of Larix principis-rupprechtii plantations on the west side of Liupan Mountain. J. Gansu Agric.Univ. 58, 169–179. doi: 10.13432/j.cnki.jgsau.2023.02.021
Howard, A. L. (2013). Handbook of structural equation modeling. Struct. Equ. Modeling 20, 354–360. doi: 10.1080/10705511.2013.769397
Hui, D., Wang, J., Le, X., Shen, W. J., and Ren, H. (2012). Influences of biotic and abiotic factors on the relationship between tree productivity and biomass in China. For. Ecol. Manag. 264, 72–80. doi: 10.1016/j.foreco.2011.10.012
Jin, J., Xiang, W. H., Zeng, Y. L., Ouyang, S., Zhou, X. L., Hu, Y. T., et al. (2022). Stand carbon storage and net primary production in China’s subtropical secondary forests are predicted to increase by 2060. Carbon Balance Manag. 17:6. doi: 10.1186/s13021-022-00204-y
Johnsen, K., Samuelson, L., Teskey, R., McNulty, S., and Fox, T. (2001). Process models as tools in forestry research and management. For. Sci. 47, 2–8. doi: 10.1093/FORESTSCIENCE/47.1.2
Johnson, J. W. (2004). Factors affecting relative weights: the influence of sampling and measurement error. Organ. Res. Methods 7, 283–299. doi: 10.1177/1094428104266018
Jones, D. L., Healey, J. R., Willett, V. B., Farrar, J. F., and Hodge, A. (2005). Dissolved organic nitrogen uptake by plants—an important N uptake pathway? Soil Biol. Biochem. 37, 413–423. doi: 10.1016/j.soilbio.2004.08.008
Jöreskog, K. (1978). Structural analysis of covariance and correlation matrices. Psychometrika 43, 443–477. doi: 10.1007/BF02293808
Kholdaenko, Y. A., Babushkina, E. A., Belokopytova, L. V., Zhirnova, D. F., Koshurnikova, N. N., Yang, B., et al. (2023). The more the merrier or the fewer the better fare? Effects of stand density on tree growth and climatic response in a scots pine plantation. Forests 14:915. doi: 10.3390/f14050915
Knoke, T., Ammer, C., Stimm, B., and Mosandl, R. (2008). Admixing broadleaved to coniferous tree species: a review on yield, ecological stability and economics. Eur. J. For. Res. 127, 89–101. doi: 10.1007/s10342-007-0186-2
Kweon, D., and Comeau, P. G. (2023). Factors influencing productivity of pine-dominated stands in South Korea. J. Environ. Manag. 330:117250. doi: 10.1016/j.jenvman.2023.117250
LeBauer, D. S., and Treseder, K. K. (2008). Nitrogen limitation of net primary productivity in terrestrial ecosystems is globally distributed. Ecology 89, 371–379. doi: 10.1890/06-2057.1
LeBreton, J. M., and Tonidandel, S. (2008). Multivariate relative importance: extending relative weight analysis to multivariate criterion spaces. J. Appl. Psychol. 93, 329–345. doi: 10.1037/0021-9010.93.2.329
Li, J., Li, Y. F., Li, G. S., Mu, L., Meng, F. J., and Zhang, J. L. (2016). Effect of site factors on the growth status of Larix principis-rupprechtii in the northern mountain region of Hebei Province. Hebei. J. For. Res. 31, 122–127. doi: 10.13320/j.cnki.hjfor.2016.0024
Li, T., Li, M. Y., Ren, F., and Tian, L. (2022). Estimation and spatio-temporal change analysis of NPP in subtropical forests: a case study of Shaoguan, Guangdong, China. Remote Sens. 14:2541. doi: 10.3390/rs14112541
Li, W. B., Lü, Z. G., Huang, X. R., and Zhang, Z. D. (2019). Predicting productivity and spatial distribution of Larix principis-rupprechtii plantation. J. Nat. Resour. 34, 1365–1375. doi: 10.31497/zrzyxb.20190702
Li, X., Plaluang, B., Kang, W. H., Ji, X.-D., Zhang, H.-J., Xue, Z.-G., et al. (2022). Responses of radial growth to climate change over the past decades in secondary Betula platyphylla forests in the mountains of Northwest Hebei, China. Chin. J. Plant Ecol. 46, 919–931. doi: 10.17521/cjpe.2021.0253
Li, M. J., Yan, W. D., Li, S. Z., Zhao, D. Y., and Duo, Y. F. (2012). Effects of controlling carbon input on nitrogen mineralization in soils of broadleaved-needle mixed forest plantation. J. Cent. South Univ. For. Technol. 32, 108–112. doi: 10.14067/j.cnki.1673-923x.2012.05.010
Lin, X., Wang, Z., Tang, Z., Zhao, S., and Fang, J. (2009). Geographic patterns and environmental correlates of terrestrial mammal species richness in China. Biodivers. Sci. 17, 652–663. doi: 10.3724/SP.J.1003.2009.09065
Lü, Z. G., Li, W. B., Huang, X. R., and Zhang, Z. D. (2019). Larix principis-rupprechtii growth suitability based on potential NPP under climate change scenarios in Hebei Province. Sci. Silvae Sin. 55, 37–44. doi: 10.11707/j1001-7488.20191105
Lu, J., Wu, Z., Zhang, C., and Zhao, X. (2021). Influence of forest strate structure on productivity of coniferous and broad-leaved mixed forest in Jiaohe, Jilin. Acta Ecol. Sin. 41, 2024–2032. doi: 10.5846/stxb202005131220
Lutz, J. A., Larson, A. J., Swanson, M. E., and Freund, J. A. (2012). Ecological importance of large-diameter trees in a temperate mixed-conifer forest. PLoS One 7:e36131. doi: 10.1371/journal.pone.0036131
Luyssaert, S., Schulze, E. D., Börner, A., Knohl, A., Hessenmöller, D., Law, B. E., et al. (2008). Old-growth forests as global carbon sinks. Nature 455, 213–215. doi: 10.1038/nature07276
Magruder, M., Chhin, S., Palik, B., and Bradford, J. B. (2013). Thinning increases climatic resilience of red pine. Can. J. For. Res. 43, 878–889. doi: 10.1139/cjfr-2013-0088
Morin, X., Fahse, L., Scherer-Lorenzen, M., and Bugmann, H. (2011). Tree species richness promotes productivity in temperate forests through strong complementarity between species. Ecol. Lett. 14, 1211–1219. doi: 10.1111/j.1461-0248.2011.01691.x
Poorter, H., and Sack, L. (2012). Pitfalls and possibilities in the analysis of biomass allocation patterns in plants. Front. Plant Sci. 3:259. doi: 10.3389/fpls.2012.00259
Powers, M. D., Palik, B. J., Bradford, J. B., Fraver, S., and Webster, C. R. (2010). Thinning method and intensity influence long-term mortality trends in a red pine forest. For. Ecol. Manag. 260, 1138–1148. doi: 10.1016/j.foreco.2010.07.002
R Development Core Team (2023). R: the R project for statistical computing. Available at: https://www.r-project.org/. (Accessed June 26, 2023)
Ratcliffe, S., Liebergesell, M., Ruiz-Benito, P., Madrigal González, J., Muñoz Castañeda, J. M., Kändler, G., et al. (2016). Modes of functional biodiversity control on tree productivity across the European continent: functional biodiversity control on tree growth. Glob. Ecol. Biogeogr. 25, 251–262. doi: 10.1111/geb.12406
Sande, M. T., Peña-Claros, M., Ascarrunz, N., Arets, E. J. M. M., Licona, J. C., Toledo, M., et al. (2017). Abiotic and biotic drivers of biomass change in a Neotropical forest. J. Ecol. 105, 1223–1234. doi: 10.1111/1365-2745.12756
Schaphoff, S., Reyer, C. P. O., Schepaschenko, D., Gerten, D., and Shvidenko, A. (2016). Tamm review: observed and projected climate change impacts on Russia’s forests and its carbon balance. For. Ecol. Manag. 361, 432–444. doi: 10.1016/j.foreco.2015.11.043
Shangguan, W., Dai, Y. J., Liu, B. Y., Zhu, A. X., Duan, Q. Y., Wu, L. Z., et al. (2013). A China data set of soil properties for land surface modeling. J. Adv. Model. Earth Syst. 5, 212–224. doi: 10.1002/jame.20026
Tian, R. (2018). Influence of site conditions on the growth of Larix principis-rupprechtii. Xiandai. Hortic. 19, 76–77. doi: 10.14051/j.cnki.xdyy.2018.19.043
Tian, H., Zhu, J., He, X., Chen, X., Jian, Z., Li, C., et al. (2022). Using machine learning algorithms to estimate stand volume growth of Larix and Quercus forests based on national-scale forest inventory data in China. For. Ecosyst. 9:100037. doi: 10.1016/j.fecs.2022.100037
Tun, T. N., Guo, J., Fang, S., and Tian, Y. (2018). Planting spacing affects canopy structure, biomass production and stem roundness in poplar plantations. Scand. J. For. Res. 33, 464–474. doi: 10.1080/02827581.2018.1457711
Wang, X. X., Dong, S. K., Gao, Q. Z., Zhang, Y., Hu, G. Z., and Luo, W. T. (2018). The rate of soil nitrogen transformation decreased by the degradation of alpine grasslands in the Qinghai Tibet plateau. Acta Agrestia Sin. 27, 1–9. doi: 10.11686/cyxb2017291
Wang, T. L., Wang, G. Y., Innes, J., Nitschke, C., and Kang, H. J. (2016). Climatic niche models and their consensus projections for future climates for four major forest tree species in the Asia–Pacific region. For. Ecol. Manag. 360, 357–366. doi: 10.1016/j.foreco.2015.08.004
Wang, Y. N., Xiong, W., Wang, Y. H., Yu, P. T., Cao, G. X., Guo, H., et al. (2013). The interannual variation of net primary productivity of three coniferous forests in Liupan Mountains of Ningxia and its responses to climatic factors. Acta Ecol. Sin. 33, 4002–4010. doi: 10.5846/stxb201211041533
Wright, A., Schnitzer, S. A., and Reich, P. B. (2014). Living close to your neighbors: the importance of both competition and facilitation in plant communities. Ecology 95, 2213–2223. doi: 10.1890/13-1855.1
Xu, X. Q., Yu, P. T., Wang, Y. H., Xu, L. H., Yu, Y. P., and Liu, Z. B. (2023). The variation of stand structure with age and its hydrological effects of larch plantation in Liupan Mountains. For. Res. 36, 109–116. doi: 10.12403/j.1001-1498.20220218
Yang, B. Y., Ali, A., Xu, M. S., Guan, M. S., Li, Y., Zhang, X. N., et al. (2022). Large plants enhance aboveground biomass in arid natural forest and plantation along differential abiotic and biotic conditions. Front. Plant Sci. 13:999793. doi: 10.3389/fpls.2022.999793
Ye, S. Y., Dong, G., Shao, C. L., and Liu, Y. (2018). Selection of tree species in accurate quality improvement of Larix principis-rupprechtii plantation. Bull. Soil Water Conserv. 38, 162–168. doi: 10.13961/j.cnki.stbctb.2018.06.025
Zhang, J., Fu, H. Y., and Qin, G. W. (2018). Spatio-temporal changes of farmland productivity in Hanjiang River basin. J. Northwest Norm. Univ. 54, 128–134. doi: 10.16783/j.cnki.nwnuz.2018.05.022
Zhang, W. W., Han, H. R., Cheng, X. Q., Shang, T. X., and Wu, R. (2019). Effects of thinning on soil active organic carbon contents and enzyme activities in Larix principis-rupprechtii plantation. Chin. J. Appl. Ecol. 30, 3347–3355. doi: 10.13287/j.1001-9332.201910.007
Zhang, X., and Lei, Y. (2010). A linkage among whole-stand model, individual-tree model and diameter-distribution model. J. For. Sci. 56, 600–608. doi: 10.17221/102/2009-JFS
Zhang, Z. H., Wang, Y. H., Guo, J. B., and Wang, X. (2022). Response of individual height of Larix principis-rupprechtii plantation to site factors and stand characteristics in Liupan Mountain area. For. Res. 35, 1–9. doi: 10.13275/j.cnki.lykxyj.2022.01.001
Zhang, J., Zhao, J. M., Cheng, R. M., Ge, Z. X., and Zhang, Z. D. (2022). Effects of neighborhood competition and stand structure on the productivity of pure and mixed Larix principis-rupprechtii forests. Forests 13:1318. doi: 10.3390/f13081318
Zhao, W. W., Liang, W. J., and Wei, X. (2019). Soil nutrient characteristics of Larix principis-rupprechtii plantations with different stand densities. J. Southwest China Norm. Univ. Nat. Sci. Ed. 44, 84–92. doi: 10.13718/j.cnki.xsxb.2019.04.015
Zhao, Y. H., Lü, H. C., Zhen, Z., Li, F. R., and Wei, Q. B. (2018). Interpolation optimization of meteorological factors and its correlation analysis with the larch NPP in Heilongjiang Province, China. J. Nanjing For. Univ. 42, 1–9. doi: 10.3969/j.issn.1000-2006.201709002
Zhao, K. J., Wang, L. D., Wang, L. J., Jia, Z. K., and Ma, L. Y. (2015). Stock volume and productivity of Larix principis-rupprechtii in northern and northwestern China. J. Beijing For. Univ. 37, 24–31. doi: 10.13332/j.cnki.jbfu.2015.02.011
Zheng, Q. Y., Zhang, G. S., Zhao, B. Q., and Wang, X. C. (2021). Xylem anatomical characteristics of Fraxinus mandshurica and relationship with climate in different slope positions. Chin. J. Appl. Ecol. 32, 3428–3436. doi: 10.13287/j.1001-9332.202110.012
Zhong, B. Y., Xiong, D. C., Shi, S. Z., Feng, J. X., Xu, C. S., Deng, F., et al. (2016). Effects of precipitation exclusion on fine-root biomass and functional traits of Cunninghamia lanceolata seedlings. Chin. J. Appl. Ecol. 27, 2807–2814. doi: 10.13287/j.1001-9332.201609.023
Zhou, Y. Q., Kang, W. X., Chen, R., Tian, D. L., and Xiang, W. H. (2018). Nutrient uptake, accumulation, and utilization efficiency comparisons in plantations containing different generations of Chinese fir. Acta Ecol. Sin. 38, 3868–3878. doi: 10.5846/stxb201705230956
Keywords: larch, tree growth, stand structure, soil nutrient, climatic factor
Citation: Wang Y, Fan N, Qian J, Zhang J, Ge Z, Liu C and Zhang Z (2024) Assessing Larix principis-rupprechtii productivity and its determinants based on national forest inventory data in Hebei Province, China. Front. For. Glob. Change. 7:1249298. doi: 10.3389/ffgc.2024.1249298
Edited by:
Manfred J. Lexer, University of Natural Resources and Life Sciences Vienna, AustriaReviewed by:
Maciej Pach, University of Agriculture in Krakow, PolandD. R. Bhardwaj, Dr. Yashwant Singh Parmar University of Horticulture and Forestry, India
Copyright © 2024 Wang, Fan, Qian, Zhang, Ge, Liu and Zhang. This is an open-access article distributed under the terms of the Creative Commons Attribution License (CC BY). The use, distribution or reproduction in other forums is permitted, provided the original author(s) and the copyright owner(s) are credited and that the original publication in this journal is cited, in accordance with accepted academic practice. No use, distribution or reproduction is permitted which does not comply with these terms.
*Correspondence: Zhidong Zhang, emhhbmd6ZEBoZWJhdS5lZHUuY24=
†These authors have contributed equally to this work