- 1Faculty of Forestry and Wood Sciences, Czech University of Life Sciences Prague, Prague, Czechia
- 2Forests of Slovak Republic, SOE, Regional Branch of CTL Technologies, Banská Bystrica, Slovakia
Introduction: The performance and consumption of CTL technologies in the forest harvesting process are influenced by a wide range of technological conditions and factors, requiring detailed analysis to increase machine performance and reduce environmental impacts.
Methods: In the presented study, we investigated the efficiency of different CTL (cut-to-length) logging machines in 1,390 forest stands from 2020 to 2022. We focused on analyzing variables that substantially affect machine productivity, fuel consumption, and the resulting CO2 emissions of harvesters and forwarders. Utilizing data from 4,044 work shifts, we determined the effect sizes and order of the variables in terms of their effects on productivity and fuel consumption. We used linear mixed models, one-way ANOVA, and regression and correlation analyses.
Results: Our findings revealed fuel consumption rates ranging from 2.65 to 2.95 liters per cubic meter of timber, directly influencing CO2 emissions, estimated between 7.11 and 7.91 kilograms per cubic meter of timber. Linear Mixed Models showed a significant effect (p<0.05) of the mean volume of the harvested trees (m3), duration of downtime, and other variables on the productivity of the CTL machines. Regression and correlation analyses showed a significant relationship (p<0.05) between variables: fuel consumption (l), mean productivity (m3), number of days worked, and number of down days, which have a substantial effect on CO2 emissions.
Conclusion: This study sheds light on the carbon emissions of modern forestry technologies and underscores the critical importance of optimizing logging operations to reduce them.
1 Introduction
During the last two decades, foresters from industrialized countries witnessed rapid progress in using fully mechanized cut-to-length (CTL) timber harvesting systems (Karjaleinen et al., 2001; Nurminen et al., 2006). Cut-to-length systems are based on the use of machines, such as harvesters and forwarders, that minimize the need for manual labor (Bacescu et al., 2022). For example, in Slovakia during 2021, harvesters logged 116,109 m3 and forwarders (following harvesters or other means of logging) extracted 214,899 m3 of the annual production of 7,687,023 m3 of timber (Ministry of Agriculture and Rural Development of the Slovak Republic, 2022).
Compared to Baltic (Estonia – 80–95%, Latvia – 70%) (Moskalik et al., 2017) or other Central European countries, such as Czechia (44%) (Dvořák et al., 2021) or Poland (20%) (Moskalik et al., 2017), the share of fully mechanized CTL harvesting systems is considerably lower. Several reasons contribute to the low uptake of the highly productive technology in the country. Notably, slope is a limiting factor in forest harvesting, especially when using mechanized harvesting systems (Strandgard et al., 2014). In Slovakia, 39% of forests are situated in terrains over 40% slope (Ministry of Agriculture and Rural Development of the Slovak Republic, 2022). Moreover, the share of hardwoods in the country reaches almost 52% of the tree species mix, which also poses a problem for mechanized harvesting systems due to, among other things, thick and uneven branches forming the crown of the trees (Mederski et al., 2018).
Despite the limitations mentioned above, CTL systems can provide an alternative to conventional harvesting systems (e.g., chainsaw logging combined with choker skidding) for thinning operations in mixed stands and indeed offer several advantages over them, including reduced environmental effects and destruction of advance regeneration and increased fiber recovery (Puttock et al., 2005). Indeed, fully mechanized CTL harvesting reaches high productivity, though at the cost of increased fuel consumption (Prinz et al., 2018), which varies from one system to another (Spinelli et al., 2014), depends on the machinery used, and directly affects the production of greenhouse gasses (GHG).
On the other hand, Lijewski et al. (2013) stated that fully mechanized CTL harvesting is a more ecological solution than using two-stroke engine chain saws when considering the different exhaust emissions. Nevertheless, minimizing fuel consumption in any harvesting system is crucial from an economic and environmental standpoint. Increasing resource efficiency is a critical element of cleaner production, and one measure is to reduce fuel input when producing the same product output (Spinelli et al., 2018). Energy efficiency, the consequent reduction of GHG emissions, and the efficiency of machine usage are some of the most important key performance indicators of forest harvesting operations (Prinz et al., 2018). Furthermore, if productivity increases more than fuel consumption, emissions per unit of output will decrease (Prinz et al., 2018).
While there are multiple publications devoted to fuel consumption, energy efficiency or exhaust emissions (Klvač et al., 2012; Holzleitner et al., 2011; Prinz et al., 2018; Kärhä et al., 2023; Haavikko et al., 2022), they mostly assess these operational parameters in more uniform conditions, such as level (or close to level) terrains, similar soils or favorable tree species composition (prevalence of softwoods). This study evaluates longitudinal operational data series, covering the highly variable technological conditions of Western Carpathians. The main goal of this study was to:
• Analyze the productivity and fuel consumption of various CTL machines in forests of the Carpathians and order the importance of variables that affect them in the forest harvesting process from a long-term perspective.
• Estimate CO2 emissions of CTL machines based on their fuel consumption in forest harvesting.
2 Materials and methods
2.1 Study area
Data were gathered between December 2020 and 2022 at the State Forests of the Slovak Republic, SOE (FSR). The company managed 831,091 ha (41.03%) of forests in Slovakia. In 2022, the company harvested approximately 3.47 million m3 (approximately 56% of hardwoods) of timber (State Forests of the Slovak Republic, 2022). From an organizational point of view, the company consisted of 12 forest management branches and two specialized branches – The Forest machinery branch (FMB) and the Nursery branch. The FMB provided aproximately 5% of all harvesting, mainly cable yarding and CTL harvesting.
2.2 Logging operations and technology
We observed three fully mechanized CTL harvesting systems, which worked in tandem and performed all logging and forwarding operations — John Deere 1270D + 1110D (high performance machine; “high”); 1070D + 810D1 (medium performance machine; “medium”); 770D + 810D2 (low performance machine; “low”) and two backup FW 810D3; 810D4 that forwarded logs and logging residues (810D3) and trucked them between roadside landings (810D4) (Table 1). The machines represented various HR and FW performance classes and were used in various stand conditions (species mix, mean stem volume, terrain variability), typical for forest management in Slovakia and Carpathian Forests. Within the research, HRs were deployed in between 140 and 206 forest stands per machine, whereas FWs were deployed in between 119 and 217 forest stands per machine (Table 2). Mean area of a forest stand at the FSR was approximately 10 ha. The work of CTL machine crews was organized into 12-h shifts and a six-day workweek. Rarely the crews had to work on Sundays, as it was mostly a day of switching between the crews. After each work week, the crews had a six-day rest. The deployed HRs were operated by six (770D) to eight (1270D) operators, and in the case of FW, the number of operators fluctuated between three (810D4) and ten (1110D) on a single machine. The logs produced were between two and six meters long. In thinnings, the machines passed on four-meter-wide trails placed two boom lengths apart. A chainsaw feller aided the machines where natural regeneration was present or when they could not access and their boom reach was insufficient. Supplemental motor-manual felling was carried out for 6,592 m3. During salvage logging, the machine crews proceeded according to local technological conditions at the workplace.
Throughout the observed period, HRs logged 116,146 m3 of timber, of which the majority was softwood timber (81.33%). The hardwood harvesting reached 20,457 m3 and consisted mainly of thinning operations, where the smaller branches and lower grades of produced logs enabled the use of CTL method. Forwarders extracted 170,159 m3 of timber, mostly softwood (91%). The 810D4 was the only machine that extracted mostly hardwood (99%) because it transported logs between roadside landings. Harvester utilization ranged between 53 and 73% and was affected by machine downtime (repairs, maintenance, holidays, lost days) and the frequent changes of operators. In the case of FWs, the utilization ranged between 62% and 77%. Harvesters consumed 150,589 l of diesel during all operations connected to machine operation, i.e., felling, processing, and pre-skidding to trail. The 1270D machine had the highest fuel consumption (57,386 l). FWs consumed 196,283 l of diesel during forwarding of logs and logging residues from the stump or edge of trail to the roadside. The highest consumption recorded for 1110D (51,816 l). The total number of Productive Machine Hours (PMH) over the observed period ranged between 3,722 and 4,822 in the case of HRs, whereas FWs clocked in between 3,552 and 5,183 PMH. Forwarders transported 26,106 loads to roadside landings, of which 19,042 (72.94%) were logs and 7,064 (27.06%) were logging residues (Table 2).
2.3 Data curation
Data were provided as daily and monthly summaries in an Excel spreadsheet by the FMB (Forest machinery branch). Within the daily summary data of HR and FW work, 4,044 work shifts were observed. Regarding the monthly HR and FW data summary, 288 working months were observed. The daily data gathered for each HR, and FW were: actual harvested or forwarded volume (m3) (v1), stand (v2), machine type (v3), operator (v4), species (hardwood or softwood) (v5), downtime (h) (v6), mean stem volume (m3) (v7), forwarding distance (m) (v8), day (v9), month (v10), duration of logging residues extraction (h) (v11), number of timber loads and logging residues forwarded (n) (v12).
Monthly data provided the following variables: fuel consumption (l) (v13), harvesting (forwarding) volume (m3) (v14), percentage share of hardwood (v15), number of days worked (v16), number of off days (v17) and productive machine hours (PMH) (v18).
We used the monthly data to calculate: fuel consumption in l m−3 (v19) (Equation 1); l PMH−1 (v20) (Equation 2); l trip−1 (v21) (Equation 3); m3 PMH−1 (v22) (Equation 4); m3 trip−1 (v23) (Equation 5); GHG emissions of CO2 (Equation 6): kg CO2 m−3 (v24) (Equation 7); kg CO2 PMH−1 (v25) (Equation 8); kg CO2 day−1 (v26) (Equation 9) and kg CO2 trip−1 (v27) (Equation 10):
Emissions of greenhouse gasses were calculated based on fuel consumption, according to the method published by the Environmental Protection Agency (EPA) of the United States of America (EPA.gov, 2023) and according to (Prinz et al., 2018), following (Equation 6):
FC – diesel fuel consumption (l),
CC – carbon content of diesel fuel (0.732 kg/L),
44/12 – ratio of molecular weights of CO2 and carbon.
2.4 Statistical analyses
The data were analyzed using Tibco Statistica 14.0.1 and IBM SPSS statistical software. One–way ANOVA was used to compare the daily productivity (v1) and productivity m3 PMH−1 (v22) between particular HR and FW types (v3) as well as between days of the week (v10); moreover, for comparison also mean stem volume (m3) (v7), fuel consumption (l) (v13), downtime duration (h) (v6). In the case of FWs, the analysis was also used to compare the differences between: forwarding distance (m) (v8), load (m3 trip−1) (v23), log loads, and logging residues loads (n day−1) (v12). The analysis was supplemented by Duncan’s test to identify the differences between groups.
For both HRs and FWs, linear mixed-effects models (LMMs) were used to analyze the relationship between the response variable harvested/forwarded volume (m3) (v1), stand (v2) (random effect) and fixed effects: machine type (v3), operator (v4), mean stem volume (m3) (v7), species (v5), downtime (h) (v6), day (v9), month (v10), forwarding distance (m) (v8), logging residue extraction (h) (v11). To estimate the fixed effect sizes, a separate LMM was created for each fixed effect, in which the response variable was the amount of harvesting or forwarding done (v1) by the HR or FW, and the random effect was the stand variable (v2).
Regression and correlation analysis was used to test the relationships between fuel consumption (v13), harvesting (forwarding) volume (m3) (v14), the share of hardwood in percent (v15), number of days worked (v16), number of off days (v17).
3 Results
3.1 Operational parameters of harvesters
The mean daily productivity of the HR ranged between 62.34 m3 and 112.70 m3 (Table 3). One–way ANOVA and Duncan’s test confirmed that the differences between daily productivities of the HR (∆ = 15.85–44.69%) were significant (F = 131.98; p–0.00) depending on the performance category of the HR (Supplementary Table 1). One–way ANOVA similarly showed significant differences (1270D: F = 8.04, p–0.00; 1070D: F = 10.26, p–0.00; 770D: F = 7.37, p–0.00) in the mean daily productivity based on the days of the week (Figure 1) for all harvesters. Duncan’s test further specified that the differences were mainly between Mondays and Sundays, which differed from other weekdays. The productivity in m3 PMH−1 ranged between 7.20–11.94 m3 PMH−1 for particular HRs, with differences (F = 26.31; p–0.00) being significant (Duncan’s) mainly for 1270D (∆ = 38.19–39.7%).
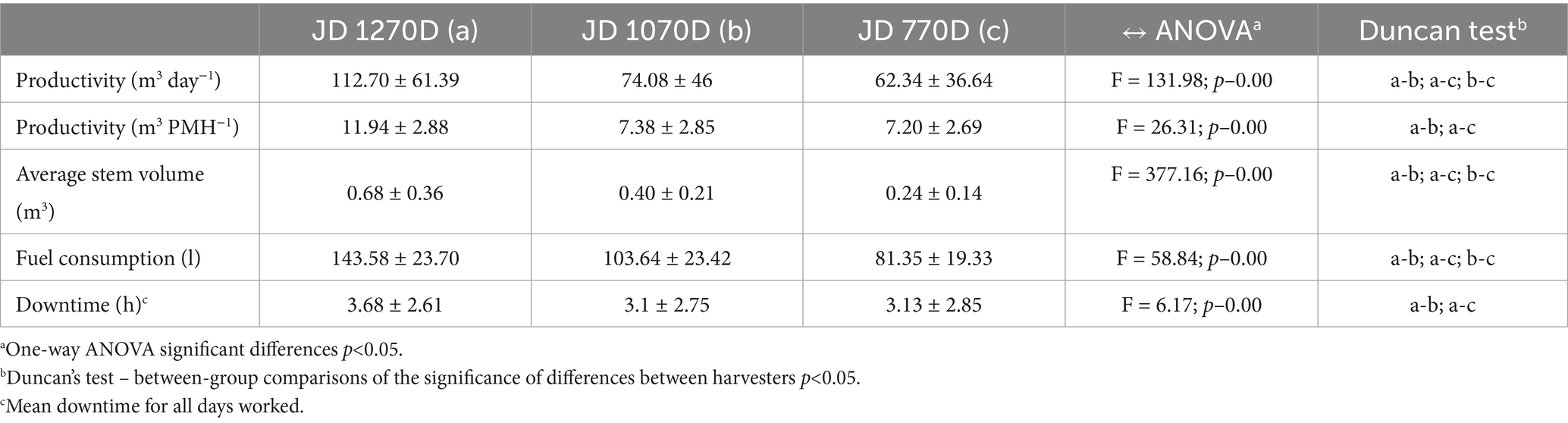
Table 3. Comparison of mean harvester productivity and other operational parameters throughout the work shift (± standard deviation).
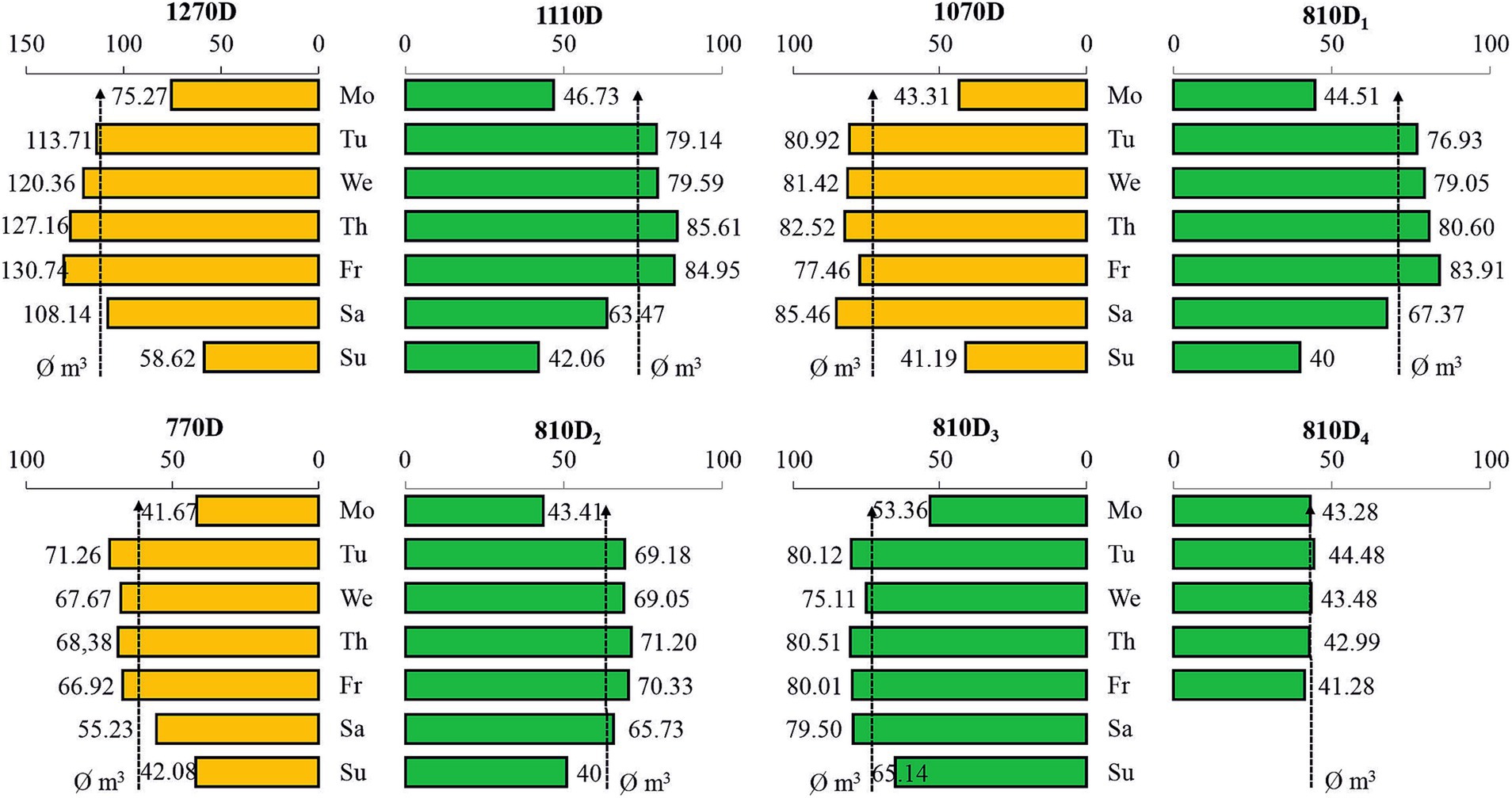
Figure 1. Comparisons of mean daily productivities of harvesters and forwarders on individual days of the week.
Significant differences (F = 377.16; p–0.00) were also found in mean stem volumes (0.24–0.68 m3) of the trees processed by HR (∆ = 40–64.71%). The mean daily fuel consumption ranged between 81.35 l and 143.58 l (F = 58.84; p–0.00) with differences between the particular HR performance categories (∆ = 21.51–43.34%) with the mean downtime duration of 3.10–3.68 h (F = 6.17; p–0.00).
Mean fuel consumption of the HR (l m−3) ranged between 1.30 and 1.78 l m−3 (Figure 2). The highest consumptions were recorded for 770D (1.54 l m−3) and 1070D (1.78 l m−3), which worked in stands with smaller stem volumes (0.26–0.42 m3). Harvester 1070D consumed 36.9% more fuel (l m−3) compared to 1270D and 13.48% more than 770D. Consumption per PMH varied due to operational conditions, with the peak recorded for 1270D (15.22 l PMH-1), while the remaining HR reached smaller values than the high-performance machine (770D: 9.87 l PMH−1; 1070D: 11.55 l PMH−1).
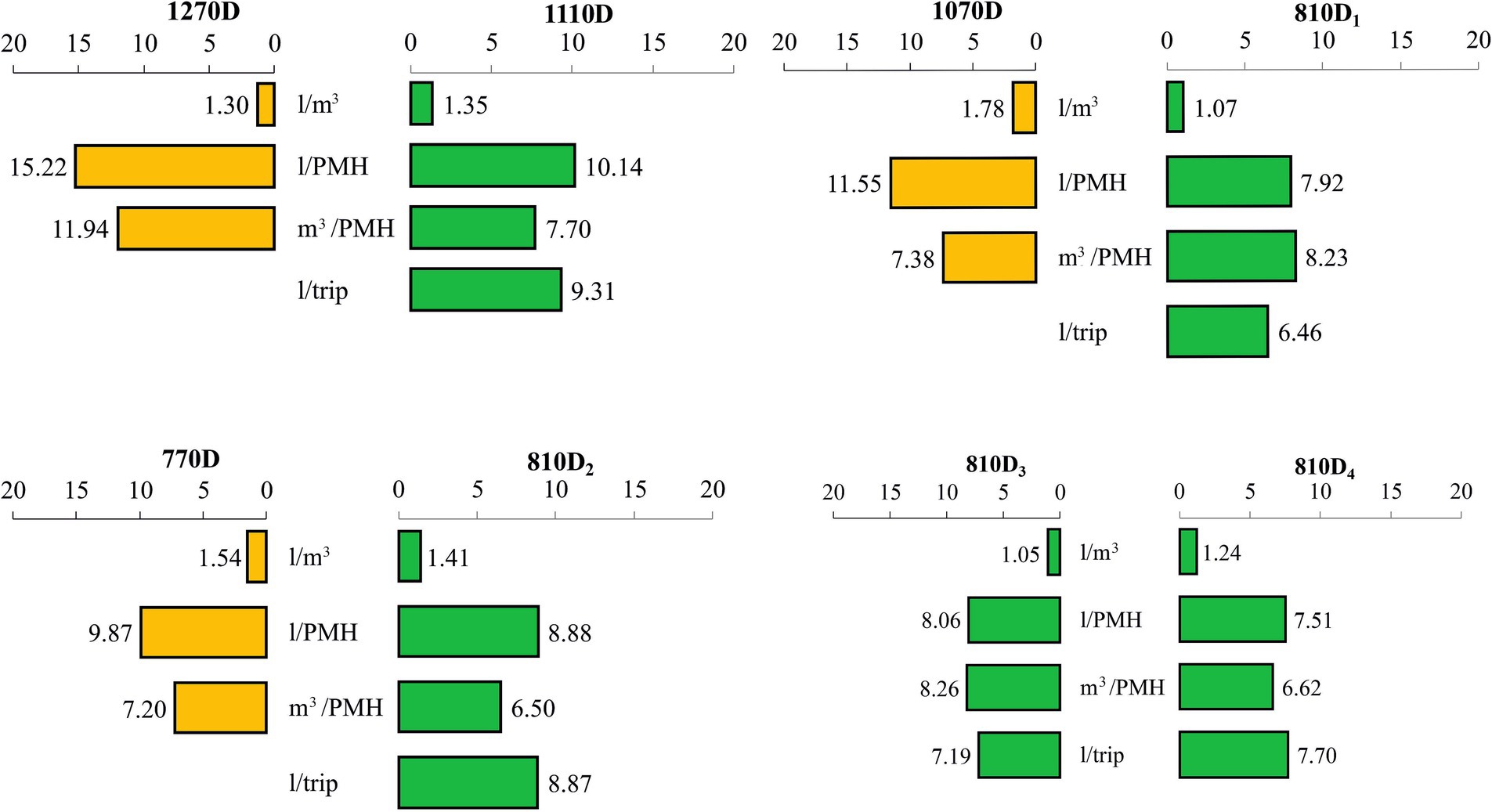
Figure 2. Mean fuel consumptions and productivities of harvesters and forwarders in the observed period of 2020–2022.
The correlation matrix (Supplementary Table 5) of fuel consumption, harvesting volume, number of days worked, and number of downtime days showed a relatively strong and significant relationship (p–0.00), likely strongly affected by the state of the HRs (machine breakdowns, downtimes) and work organization. The share of deciduous trees harvested showed a significant relationship with fuel consumption in the case of the observed machines.
The LMM constructed for daily productivity of HR (response variable), stand, in which the machine worked (random effect), and fixed effects (type of HR: F = 3.69, p = 0.03; operator: F = 10.87, p–0.00; mean stem volume (m3): F = 5.80, p–0.00; species: F = 20.37, p–0.00; downtime (h): F = 29.66, p–0.00; harvesting month: F = 2.77, p–0.00; day: F = 12.59, p–0.00) showed that the fixed effects significantly affected (p–0.00, R2m = 0.73, and R2c = 0.78) the response variable (Supplementary Table 2). The analysis showed that the fixed effects caused 73% of the variability of daily HR productivity, and the combined fixed and random effects caused 78%, i.e., the stand conditions only contributed 5% explanatory power of the variability of daily HR productivity.
Considering the fixed effects (Table 4), the mean stem size (m3): R2m = 0.421 (42.1%) had the most prominent effect on daily HR productivity, followed by downtime duration (h): R2m = 0.271 (27.1%), operator: R2m = 0.177 (17.7%), species: R2m = 0.134 (13.4%), day: R2m = 0.125 (12.5%), type of HR: R2m = 0.115 (11.5%), and the month in which the harvesting took place: R2m = 0.023 (2.3%) had the smallest effect.
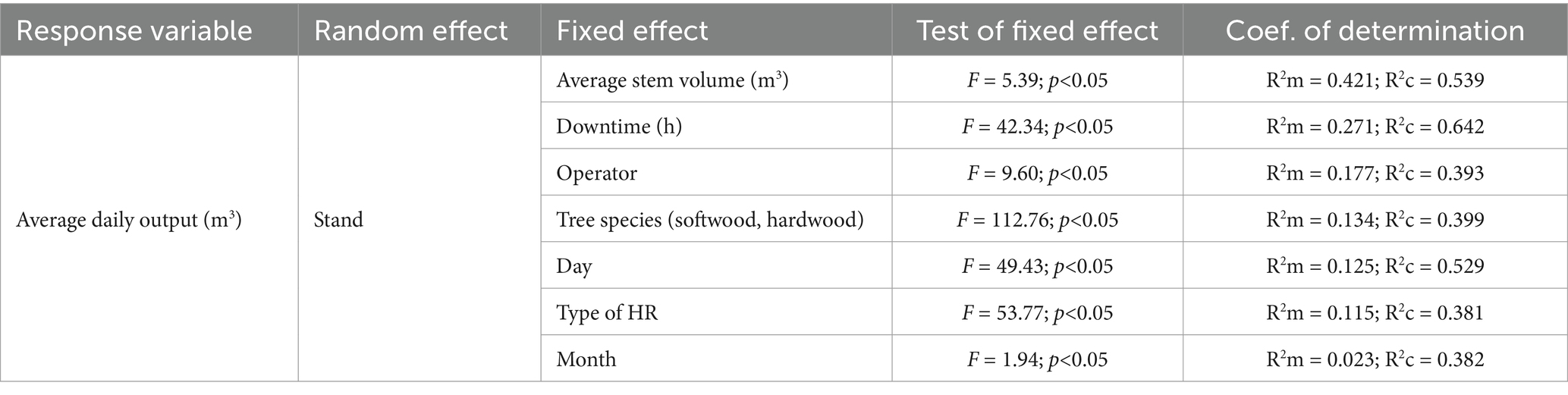
Table 4. Order of sizes of fixed effects for the mean daily (m3) output of the harvester (HR) response variable.
3.2 Operational parameters of forwarders
Analysis of variance showed that the differences between daily productivities of FW were significant (F = 80.27, p–0.00) (Table 5), ranging between 64.66–74.24 m3 (∆ = 0.35–15.22%) (Supplementary Table 3) and 43.16 m3 in the case of 810D4. One–way ANOVA showed significant differences in the mean daily productivity of FW between the days of the week (1110D: =19.43, p–0.00; 810D1: F = 13.89, p–0.00; 810D2: F = 9.53, p–0.00; 810D3: F = 4.22, p–0.00) except 810D4 (F = 0.97, p–0.42), where the differences were not significant since it forwarded timber between roadside landings and as a result of the five-day workweek (Figure 1). Duncan’s test confirmed that the forwarders achieved different productivity on Mondays and Sundays, with the exception of 810D3, where the difference only showed on Mondays. The productivity in m3 PMH−1 ranged between 6.50–8.26 m3 PMH−1 (∆ = 0.36–27.08%) for particular FWs, with the differences between machines being significant (F = 5.17; p–0.00).
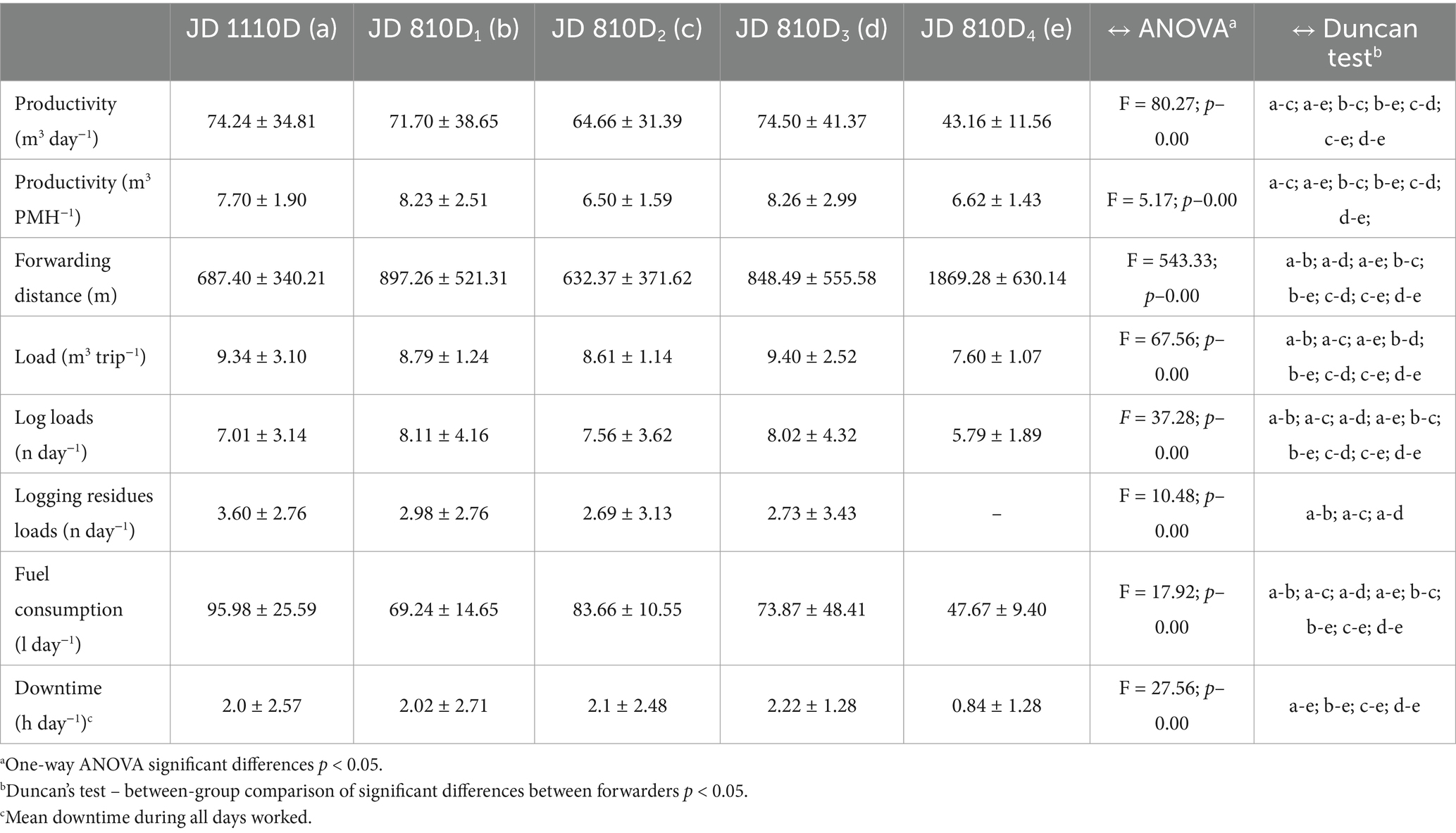
Table 5. Comparison of mean forwarder productivity and other operational parameters throughout the work shift (± standard deviation).
The ANOVA confirmed significant differences between forwarding distances (F = 543.33, p–0.00). The mean forwarding distance ranged between 632.37–897.26 m (∆ = 5.44–34.18%), and the longest was 1,869.28 m (810D4) when forwarding between two roadside landings. The mean load of the forwarders ranged between 7.60 and 9.40 m3 (∆ = 0.64–9.18%) (F = 67.56, p–0.00) and was connected to the performance of the HR. The mean number of loads during the work shifts ranged between 5.79 and 8.11 (F = 37.25, p–0.00) (∆ = 1.11–15.69%) and in the case of logging residue forwarding (F = 10.48, p–0.00), it was 2.69–3.60 (∆ = 1.49–25.28%). The highest mean daily fuel consumption (F = 17.92, p–0.00) was recorded for 1110D (95.98 L), conversely and the lowest for 810D1 (69.24 L) (∆ = 6.69–27.86%), 810D4 consumed the least fuel per day (47.67 L). The mean downtime of the FW ranged between 0.84 and 2.22 h (F = 27.56, p–0.00). The short downtimes of JD 810D4 (0.84 h) were likely due to its forwarding between roadside landings, in less demanding conditions and over forest roads, and the fact that the smallest number of operators rotated on the machine.
The LMM (Supplementary Table 4) constructed for the mean daily productivity as a response variable, the stand, where the machines were deployed (random effect), and fixed effects (machine type: F = 17.91, p–0.00; operator: F = 18.80, p–0.00; species (softwood or hardwood): F = 25.24, p–0.00; downtime: F = 979.85, p–0.00; forwarding distance: F = 60.53, p–0.00; month: F = 5.98, p–0.00; day: F = 19.78, p–0.00; logging residue forwarding duration (h): F = 125.0, p–0.00) showed that all fixed effects had a significant effect on the response variable (p–0.00). The R2m = 0.497 and R2c = 0.646 showed that 49.7% of the variability of the response variable was due to the fixed effects and 64.6% due to both fixed and random effects, i.e., the stand conditions affected the FW productivity by nearly 15%.
Of the fixed effects, machine downtime had the most considerable effect (Table 6) on the response variable: R2m = 0.245 (24.5%), followed by species: R2m = 0.168 (16.8%), operator: R2m = 0.150 (15%), and FW type: R2m = 0.120 (12%). The forwarding distance had a relatively small effect with R2m = 0.09 (9%), similar to the day of the week, the month in the year, and the duration of logging residues extraction (h) throughout the work shifts.
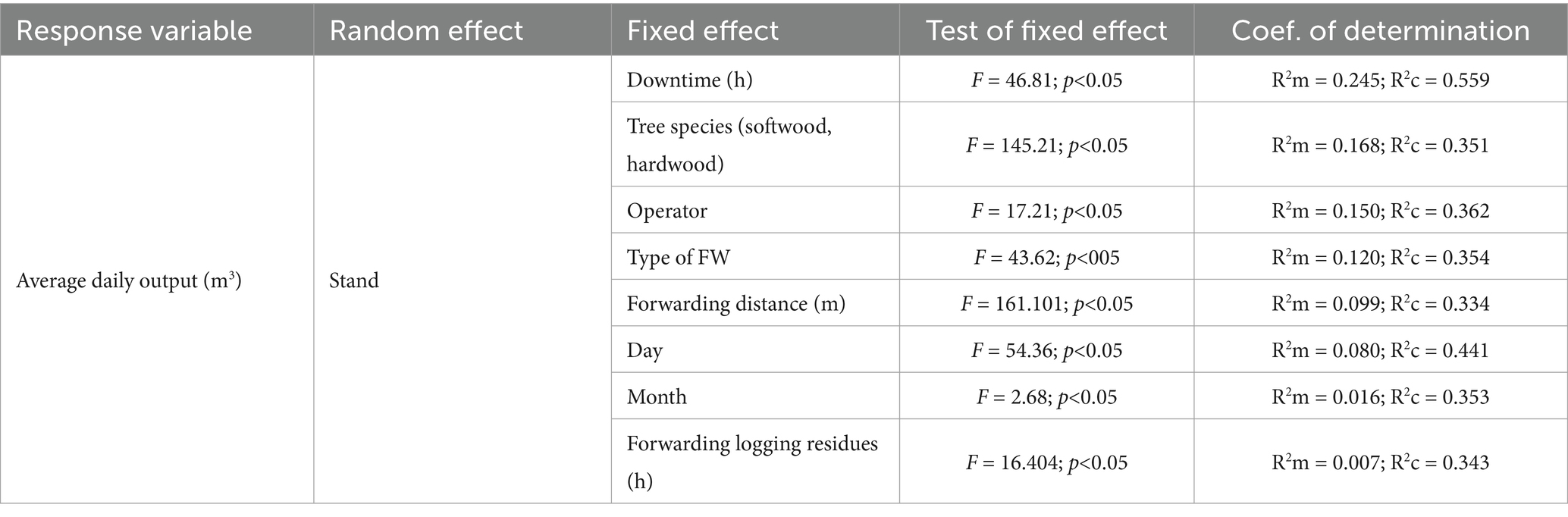
Table 6. Order of sizes of fixed effects for the mean daily output (m3) of the forwarder (FW) response variable.
In the case of FWs that worked in tandem with HRs, the mean consumption ranged between 1.07 (810D1) and 1.41 (810D2) l m-3, while the consumption per PMH was in the interval of 7.92 (JD 810D1) to 10.14 (1110D) l PMH−1. We must state that the consumption per m3 included the consumption for logging residue forwarding because that was carried out simultaneously with forwarding logs and was not explicitly recorded. Consumption per trip (l trip−1) ranged between 6.46 (810D1) and 9.31 (1110D) l trip−1. Forwarder 810D3 reached values similar to those of the FWs mentioned before, as it supported them in similar technological conditions, while 810D4 had higher fuel consumption per m3 and trip due to forwarding heavier hardwood loads over longer distances. Regression and correlation analysis showed a significant relationship (p–0.00) between FW fuel consumption, the volume of extracted timber, the number of days worked, and the number of off days (Supplementary Table 6). The share of hardwood trees had a significant relationship (p–0.00) only in the case of 810D1.
The total fuel consumption per m3 reached 2.65 l m−3 in the case of the high-performance machine combination (1270D + 1110D). In the case of the mid-performance machine combination, it was 2.85 l m−3 (1070D + 810D1), and the low-performance machine combination (770D + 810D2) reached the highest fuel consumption of 2.96 l m−3, likely because it forwarded timber in forest stands with the lowest mean stem volume.
3.3 CO2 emissions of the machines
Carbon dioxide emissions primarily relate to fuel consumption. HR produced between 3.49 (1270D) and 4.78 kg m−3 (1070D) of CO2. Emissions per PMH ranged between 26.49 (770D) and 40.85 kg CO2 PMH−1 (1270D), and the daily production ranged between 218.34 (770D) and 385.37 kg (1270D) CO2 day−1. FW produced between 2.82 (810D3) and 3.78 (810D2) kg CO2 m−3 and 20.16 (810D4) to 27.22 (1110D) kg CO2 PMH−1. Daily emissions of FW ranged between 127.95 (810D4) and 256.54 (1110D) kg CO2 day−1 (Figure 3).
The high-performance HR/FW combination (node) (1270D + 1110D) produced 7.11 kg CO2 m−3 of timber, while the mid-performance machines (1070D + 810D2) 7.65 kg CO2 m−3, and the low-performance machines (770D + 810D2) produced 7.91 kg CO2 m−3. The low-performance CTL machine node (770D + 810D1) emitted 11.25% more CO2 m−3 than the high-performance machine combination (1270D + 1110D) and 3.4% more than the mid-performance machine combination (1070D + 810D1).
4 Discussion
4.1 Harvester and forwarder productivity
The productivity of HR and FW is affected by several variables. Through LMMs, we could explain more than three-quarters of the variability of HR productivity and almost two-thirds of the variability of productivity in the case of the FWs. The effects of forest stands (random effect) where the machines worked were minor for HRs and slightly more substantial for FWs, which showed the higher sensitivity of the FW to the stand characteristics, such as slope, tree species, soil type and others. The mean stem volume proved to be the decisive variable in the case of HR productivity. Several studies (Eriksson and Lindroos, 2014; Polowy and Molińska-Glura, 2023; Ackerman et al., 2024; Liski et al., 2020; Louis et al., 2022) stated that the mean stem size was the most influential variable for harvesting productivity. Moreover, according to She et al. (2018), harvesting productivity is influenced by machine utilization and is inversely related to downtime, i.e., a higher amount of downtime lowers utilization. In our case, the utilization of HRs ranged between 53 and 73%, and for FWs, it ranged between 62 and 77%. These rates were similar to those reported by Holzleitner et al. (2011), who observed utilization of 62% (HRs) to 63% (FWs) using the same mode of operation. Spinelli et al. (2011) report higher utilization between 70% (HRs) and 78% (FWs). In this study, the age of the machines and their related higher rate of failure likely substantially contributed to the amount of downtimes. Liski et al. (2020) reported that the operator alone explained ca. 30–60% of the productivity variation of CTL harvesting. Purfürst and Erler (2011) and Kärhä et al. (2004) reported that the operator causes 37.3% or 40% of HR productivity variability, respectively. In our case, the size of the effect of the operator was substantially smaller, between 15% (FWs) and 17% (HRs).
According to Labelle et al. (2016), the frequent forks in the top part of a tree and thick branches in the case of harvesting hardwood trees can reduce the harvester efficiency by 15 to 20%, similar to our findings. George et al. (2022) too attributed longer processing times to hardwoods. The under-representation of hardwood forests in the sample, compared to their overall representation in Slovakia, likely caused the small effect of species composition on machine productivity.
Only a handful of studies observe the development of machine productivity based on the day of the week or the month. Most studies that deal with these variables consider them from the point of view of the duration of the work shifts (Passicot and Murphy, 2013) or by comparing the effects of day and night shifts (Nicholls et al., 2004). In our case, the effect of the day of the week only showed in the case of HRs (12.5%), when the change of the squads likely caused the significant differences observed between Monday and Sunday. Considering the seasonal effects, Kymäläinen et al. (2023) reported significant differences in productivity between the summer and winter seasons, while in our case, the month’s effect was negligible for HRs and FWs. Haavikko et al. (2022) report that in forwarding, travel distance affects productivity and fuel consumption the most, which was not the case in our study, as we identified downtime, tree species, operator, and forwarder type as more influential variables.
4.2 Fuel consumption and CO2 emissions
Kärhä et al. (2023) reported that the mean fuel consumption of CTL machines is 1.36 l m−3 in final fellings (cutting 0.77 l m−3; forwarding 0.59 l m−3) and 3.06 (cutting 2.12 l m−3; forwarding 0.94 l m−3) and 2.23 l m−3 (cutting 1.39 l m−3; forwarding 0.84 l m−3) in first and later thinnings, respectively. Haavikko et al. (2022) reported that the mean calculated fuel consumption of timber harvesting was 1.59 l m−3 (cutting 0.91 l m−3; forwarding 0.68 l m−3). Similarly, Klvac et al. (2003) reported fuel consumption in the range between 1.28 and 1.73 l m−3 for various machine performance classes in Ireland, and the results are in line with our findings for both HRs and FWs. The increased fuel consumption of FWs was caused by including the forwarding of logging residues for chipping into the overall fuel consumption, which we could not separate from other machine operations in the data. Holzleitner et al. (2011) state that the mean hourly fuel consumption is 15.6 lPMH−1 for HRs, and 11.1 l PMH−1 for FWs. Magagnotti et al. (2021) reported that fuel consumption for three HRs with engine performance of 165 to 205 kW ranged between 8.4 and 5.3 l PMH−1. We reached similar results, with HR fuel consumption between 9.87 and 15.22 l PMH−1.
Mean productivity per PMH was affected mainly by the conditions in which the machines operated. Gerasimov et al. (2012), in their longitudinal study of HR productivity in Russia, revealed that HR productivity ranges between 4.3 and 14.9 m3 PMH−1, with a mean of 10.7 m3 PMH−1, while in our study, it ranged between 7.20 and 11.94 m3 PMH−1. Similarly, Dvořák et al. (2019) report an 8.3–9.9 m3 PMH−1 interval for similar machine classes.
Greenhouse gas emissions also depend on numerous factors, but mainly on the fuel consumption within the forest harvesting process. Kärhä et al. (2023), in their study conducted in Finland, reported a 6.6 kg CO2 m−3 in thinnings and 3.6 kg CO2 m−3 in final fellings for JD 1110D HR and JD 1110E FW. Similarly, Haavikko et al. (2022) found that the average GHG emissions of the CTL machines from first and later thinnings were 7.3 and 5.3 kg CO2 m−3, respectively, and 3.1 kg CO2 m−3 from final fellings. These values correspond with our results, which ranged between 7.11 and 7.91 kg CO2 m−3. On the other hand, Bacescu et al. (2022) found that the mean GHG emissions of HR (JD 1270G) and FW (1510G) were 2.1 and 2.56 kg CO2 m−3 respectively, while Kärhä et al. (2024) state that the CO2 emissions produced during fully mechanized felling-processing averaged 3.62 kg m−3 (ranging from 1.25 to 5.67 kg m−3) in thinnings and 2.56 kg m−3 (ranging from 1.71 to 3.33 kg m−3) in final fellings. Cosola et al. (2016) realized that the CO2 emissions incurred by fully mechanized CTL harvesting are lower in plantation forests (average 4.23 kg m−3) than in close-to-nature forests (average 6.64 kg m−3), which points towards a strong effect of felling type and management system on the machine fuel consumption, also observed by Alex et al. (2024). Furthermore, Kärhä et al. (2024) reported that CO2 emissions decreased with increasing machine size, due to the higher productivity of the larger machines, which corresponds to our results, as we observed the highest fuel consumption for the small CTL machine node. However, this was likely caused by the use of appropriate machine size for the operations being conducted, rather than a general statement of better efficiency of large (or higher engine performance) machines. Indeed, using inappropriate machine sizes can lead to excessive residual stand damage and decreased fuel efficiency because the large machine necessitates more space and wider trails, and its engine performance exceeds the power needs of most thinning operations.
4.3 Study limitations
Analyzing the operational parameters of CTL machinery in various conditions is important as a decision support for increasing machine productivity, decreasing fuel consumption, and reducing GHG emissions. The study takes advantage of a relatively large data set of HRs and FWs observed during their work under a wide array of technological conditions. In the future, the research could be expanded by including different machine makes and brands to increase the generalizability of the results. Another avenue would be to include the inputs directly from the forest machine systems of the observed HRs and FWs, which would enable us to analyze the effects of additional variables and improve our ability to analyze the harvesting site’s role. This study was unique in its focus on CTL machines in the specific and varied technological conditions of Western Carpathians.
5 Conclusion
Based on our findings, we reached the following conclusions:
i. LMMs confirmed that the most important variables regarding HR productivity were mean stem volume (m3), downtime duration, operator, and tree species. In the case of FW, the most influential variables were downtime (h), tree species, operator, and FW type.
ii. Regression and correlation analysis showed a significant relationship between fuel consumption, harvesting volume, extracted timber volume, number of days worked, and the number of down days for HR and FW.
iii. With decreasing mean stem volume and size of the machines, fuel consumption per cubic meter of timber and CO2 emissions per cubic meter of timber increase.
Data availability statement
The data that support the findings of this study are available from the corresponding author upon reasonable request.
Author contributions
MA: Conceptualization, Data curation, Formal analysis, Methodology, Resources, Supervision, Visualization, Writing – original draft, Writing – review & editing. MJ: Conceptualization, Formal analysis, Writing – original draft. VB: Data curation, Methodology, Validation, Writing – review & editing. ZD: Conceptualization, Data curation, Formal analysis, Methodology, Writing – review & editing.
Funding
The author(s) declare that financial support was received for the research and/or publication of this article. Grant No. SML-00007-2022-99-47. Analysis of electronic timber scaling on the roadside landing and the selection of optimal technologies for the needs of Forests of the Czech Republic, GOE supported this work.
Conflict of interest
The authors declare that the research was conducted in the absence of any commercial or financial relationships that could be construed as a potential conflict of interest.
Generative AI statement
The author(s) declare that no Gen AI was used in the creation of this manuscript.
Publisher’s note
All claims expressed in this article are solely those of the authors and do not necessarily represent those of their affiliated organizations, or those of the publisher, the editors and the reviewers. Any product that may be evaluated in this article, or claim that may be made by its manufacturer, is not guaranteed or endorsed by the publisher.
Supplementary material
The Supplementary material for this article can be found online at: https://www.frontiersin.org/articles/10.3389/ffgc.2025.1567136/full#supplementary-material
References
Ackerman, S., Bekker, J., Astrup, R., and Talbot, B. (2024). Understanding the influence of tree size distribution on the CTL harvesting productivity of two different size harvesting machines. Eur. J. For. Res. 143, 1199–1211. doi: 10.1007/s10342-024-01680-2
Alex, A., Kizha, A. R., George, A. K., Bick, S., Wang, J., Klein, S., et al. (2024). Carbon footprint of the predominant mechanized timber harvesting methods in the northeastern US. Clean. Circ. Bioecon. 9:100097. doi: 10.1016/j.clcb.2024.100097
Bacescu, N. M., Cadei, A., Moskalik, T., Wiśniewski, M., Talbot, B., and Grigolato, S. (2022). Efficiency assessment of fully mechanized harvesting system through the use of Fleet management system. Sustain. For. 14:16751. doi: 10.3390/su142416751
Cosola, G., Grigolato, S., Ackerman, P., Monterotti, S., and Cavalli, R. (2016). Carbon footprint of Forest operations under different management regimes. Croat. J. For. Eng. J. Theory Appl. For. Eng. 37, 201–217. Available at: https://hrcak.srce.hr/file/22612
Dvořák, J., Chytrỳ, M., Natov, P., Jankovskỳ, M., and Beljan, K. (2019). Long-term cost analysis of mid-performance harvesters in Czech conditions. Austrian J. For. Sci. 136. Available online at: https://search.ebscohost.com/login.aspx?direct=true&profile=ehost&scope=site&authtype=crawler&jrnl=03795292&AN=142541735&h=S%2FVS8HWxIk%2Bh3%2FqHQWluf17Q6LK71ATpFVnGON08pLa82ZJ6Gv88l9uK0EeF1%2BuxAaczeL9KMHcMHfIJtHhftw%3D%3D&crl=c
Dvořák, J., Jankovskỳ, M., Chytrỳ, M., Nuhlíček, O., Natov, P., Kormanek, M., et al. (2021). Operational costs of mid-performance forwarders in Czech Forest bioeconomy. Forests 12:435. doi: 10.3390/f12040435
EPA.gov (2023). Greenhouse gas inventory guidance: direct emissions from Mobile combustion source. Available at: https://www.epa.gov/sites/default/files/2020-12/documents/mobileemissions.pdf (Accessed December 15, 2023).
Eriksson, M., and Lindroos, O. (2014). Productivity of harvesters and forwarders in CTL operations in northern Sweden based on large follow-up datasets. Int. J. For. Eng. 25, 179–200. doi: 10.1080/14942119.2014.974309
George, A. K., Kizha, A. R., and Kenefic, L. (2022). Timber harvesting on fragile ground and impacts of uncertainties in the operational costs. Int. J. For. Eng. 33, 12–21. Available at: https://www.tandfonline.com/doi/abs/10.1080/14942119.2022.1988432 (Accessed March 22, 2025).
Gerasimov, Y., Senkin, V., and Väätäinen, K. (2012). Productivity of single-grip harvesters in clear-cutting operations in the northern European part of Russia. Eur. J. For. Res. 131, 647–654. doi: 10.1007/s10342-011-0538-9
Haavikko, H., Kärhä, K., Poikela, A., Korvenranta, M., and Palander, T. (2022). Fuel consumption, greenhouse gas emissions, and energy efficiency of wood-harvesting operations: a case study of Stora Enso in Finland. Croat. J. For. Eng. J. Theory Appl. For. Eng 43, 79–97. doi: 10.5552/crojfe.2022.1101
Holzleitner, F., Stampfer, K., and Visser, R. (2011). Utilization rates and cost factors in timber harvesting based on long-term machine data. Croat. J. For. Eng. J. Theory Appl. For. Eng 32, 501–508. Available at: https://hrcak.srce.hr/file/108152
Kärhä, K., Eliasson, L., Kühmaier, M., and Spinelli, R. (2024). Fuel consumption and CO2 emissions in fully mechanized cut-to-length (CTL) harvesting operations of industrial Roundwood: a review. Curr. For. Rep. 10, 255–272. doi: 10.1007/s40725-024-00219-3
Kärhä, K., Haavikko, H., Kääriäinen, H., Palander, T., Eliasson, L., and Roininen, K. (2023). Fossil-fuel consumption and CO2eq emissions of cut-to-length industrial roundwood logging operations in Finland. Eur. J. For. Res. 142, 547–563. doi: 10.1007/s10342-023-01541-4
Kärhä, K., Rönkkö, E., and Gumse, S.-I. (2004). Productivity and cutting costs of thinning harvesters. Int. J. For. Eng. 15, 43–56. doi: 10.1080/14942119.2004.10702496
Karjaleinen, T., Zimmer, B., Berg, S., Welling, J., Schwaiger, H., Finér, L., et al. (2001). Energy, carbon and other material flows in the life cycle assessment of forestry and forest products: Achievements of the working group 1 of the COST action E. Joensuu: European Forest Institute.
Klvač, R., Fischer, R., and Skoupý, A. (2012). Energy use of and emissions from the operation phase of a medium distance cableway system. Croat. J. For. Eng. J. Theory Appl. For. Eng. 33, 79–88. Available at: https://hrcak.srce.hr/file/128029
Klvac, R., Ward, S., Owende, P. M. O., and Lyons, J. (2003). Energy audit of wood harvesting systems. Scand. J. For. Res. 18, 176–183. doi: 10.1080/02827580310003759
Kymäläinen, H., Hujala, T., Häggström, C., and Malinen, J. (2023). Workability and productivity among CTL machine operators – associations with sleep, fitness, and shift work. Int. J. For. Eng. 34, 426–438. doi: 10.1080/14942119.2023.2216113
Labelle, E. R., Soucy, M., Cyr, A., and Pelletier, G. (2016). Effect of tree form on the productivity of a cut-to-length harvester in a hardwood dominated stand. Croat. J. For. Eng. J. Theory Appl. For. Eng 37, 175–183. Available at: https://hrcak.srce.hr/file/226119
Lijewski, P., Merkisz, J., and Fuć, P. (2013). Research of exhaust emissions from a harvester diesel engine with the use of portable emission measurement system. Croat. J. For. Eng. J. Theory Appl. For. Eng 34, 113–122. Available at: https://hrcak.srce.hr/file/172583
Liski, E., Jounela, P., Korpunen, H., Sosa, A., Lindroos, O., and Jylhä, P. (2020). Modeling the productivity of mechanized CTL harvesting with statistical machine learning methods. Int. J. For. Eng. 31, 253–262. doi: 10.1080/14942119.2020.1820750
Louis, L. T., Kizha, A. R., Daigneault, A., Han, H.-S., and Weiskittel, A. (2022). Factors affecting operational cost and productivity of ground-based timber harvesting machines: a Meta-analysis. Curr. For. Rep. 8, 38–54. doi: 10.1007/s40725-021-00156-5
Magagnotti, N., Spinelli, R., Kärhä, K., and Mederski, P. S. (2021). Multi-tree cut-to-length harvesting of short-rotation poplar plantations. Eur. J. For. Res. 140, 345–354. doi: 10.1007/s10342-020-01335-y
Mederski, P. S., Bembenek, M., Karaszewski, Z., Pilarek, Z., and Łacka, A. (2018). Investigation of log length accuracy and harvester efficiency in processing of oak trees. Croat. J. For. Eng. J. Theory Appl. For. Eng. 39, 173–181. Available at: https://hrcak.srce.hr/file/300545
Ministry of Agriculture and Rural Development of the Slovak Republic (2022). Green report, Available online at: https://www.mpsr.sk/lesne-hospodarstvo/123 (Accessed December 14, 2023).
Moskalik, T., Borz, S. A., Dvořák, J., Ferencik, M., Glushkov, S., Muiste, P., et al. (2017). Timber harvesting methods in eastern European countries: a review. Croat. J. For. Eng. J. Theory Appl. For. Eng 38, 231–241. Available at: https://hrcak.srce.hr/file/281488
Nicholls, A., Bren, L., and Humphreys, N. (2004). Harvester productivity and operator fatigue: working extended hours. Int. J. For. Eng. 15, 57–65. doi: 10.1080/14942119.2004.10702497
Nurminen, T., Korpunen, H., and Uusitalo, J. (2006). Time consumption analysis of the mechanized cut-to-length harvesting system. Silva Fenn. 40. doi: 10.14214/sf.346
Passicot, P., and Murphy, G. E. (2013). Effect of work schedule design on productivity of mechanised harvesting operations in Chile. N. Z. J. For. Sci. 43:2. doi: 10.1186/1179-5395-43-2
Polowy, K., and Molińska-Glura, M. (2023). Data Mining in the Analysis of tree harvester performance based on automatically collected data. Forests 14:165. doi: 10.3390/f14010165
Prinz, R., Spinelli, R., Magagnotti, N., Routa, J., and Asikainen, A. (2018). Modifying the settings of CTL timber harvesting machines to reduce fuel consumption and CO2 emissions. J. Clean. Prod. 197, 208–217. doi: 10.1016/j.jclepro.2018.06.210
Purfürst, F. T., and Erler, J. (2011). The human influence on productivity in harvester operations. Int. J. For. Eng. 22, 15–22. doi: 10.1080/14942119.2011.10702606
Puttock, D., Spinelli, R., and Hartsough, B. R. (2005). Operational trials of cut-to-length harvesting of poplar in a mixed wood stand. Int. J. For. Eng. 16, 39–49. doi: 10.1080/14942119.2005.10702506
She, J., Chung, W., and Kim, D. (2018). Discrete-event simulation of ground-based timber harvesting operations. Forests. 9:683. doi: 10.3390/f9110683
Spinelli, R., de Arruda, C., Moura, A., and Manoel da Silva, P. (2018). Decreasing the diesel fuel consumption and CO2 emissions of industrial in-field chipping operations. J. Clean. Prod. 172, 2174–2181. doi: 10.1016/j.jclepro.2017.11.196
Spinelli, R., Lombardini, C., and Magagnotti, N. (2014). The effect of mechanization level and harvesting system on the thinning cost of Mediterranean softwood plantations. Silva Fenn. 48, 1–15. Available at: https://www.silvafennica.fi/article/1003/keyword/biomass (Accessed January 25, 2024).
Spinelli, R., Magagnotti, N., and Picchi, G. (2011). Annual use, economic life and residual value of cut-to-length harvesting machines. J. For. Econ. 17, 378–387. doi: 10.1016/j.jfe.2011.03.003
State Forests of the Slovak Republic (2022). Annual Report, Available at: https://www.lesy.sk/files/lesy/o-nas/vyrocne-spravy/zoznam/2022/vyrocna-sprava-za-rok-2022-lesy-sr-final-web.pdf (Accessed January 10, 2024).
Strandgard, M., Alam, M., and Mitchell, R. (2014). Impact of slope on productivity of a self-levelling processor. Croat. J. For. Eng. J. Theory Appl. For. Eng. 35, 193–200. Available at: https://hrcak.srce.hr/file/187578
Keywords: CO2 emissions, forwarder, fuel consumption, harvester, machine productivity, sustainable forestry
Citation: Allman M, Jankovský M, Bobrík V and Dudáková Z (2025) Operational efficiency and environmental impacts: a study on cut-to-length logging in the Carpathian forests. Front. For. Glob. Change. 8:1567136. doi: 10.3389/ffgc.2025.1567136
Edited by:
Kevin Boston, University of Arkansas at Monticello, United StatesReviewed by:
Tolga Ozturk, Istanbul University-Cerrahpasa, TürkiyeAshish Alex, University of Maine, United States
Mariana Carmelia Dragomir Balanica, Dunarea de Jos University, Romania
Alex Kunnathu George, Paul Smith’s College, United States
Copyright © 2025 Allman, Jankovský, Bobrík and Dudáková. This is an open-access article distributed under the terms of the Creative Commons Attribution License (CC BY). The use, distribution or reproduction in other forums is permitted, provided the original author(s) and the copyright owner(s) are credited and that the original publication in this journal is cited, in accordance with accepted academic practice. No use, distribution or reproduction is permitted which does not comply with these terms.
*Correspondence: Michal Allman, YWxsbWFuQGZsZC5jenUuY3o=
†ORCID: Michal Allman, http://orcid.org/0009-0009-1071-5214
Martin Jankovský, http://orcid.org/0000-0001-6597-3469
Zuzana Dudáková, http://orcid.org/0000-0003-4776-6903