- 1Department of Obstetrics and Gynecology, School of Medicine, Iranshahr University of Medical Sciences, Iranshahr, Iran
- 2Department of Obstetrics and Gynecology, School of Medicine, Iran University of Medical Sciences, Tehran, Iran
- 3Shahid Akbarabadi Clinical Research Development Unit, Iran University of Medical Sciences, Tehran, Iran
- 4Neonatal Research Center, Shiraz University of Medical Sciences, Shiraz, Iran
- 5Department of Medical Genetics, School of Medicine, Shiraz University of Medical Sciences, Shiraz, Iran
- 6School of Medicine, Tehran University of Medical Sciences, Tehran, Iran
- 7School of Medicine, Shahid Sadoughi University of Medical Sciences, Yazd, Iran
- 8School of Medicine, Shiraz University of Medical Sciences, Shiraz, Iran
- 9Department of Radiology, School of Medicine, Shahid Rahnamoun Hospital, Shahid Sadoughi University of Medical Sciences, Yazd, Iran
- 10Mother and Newborn Health Research Center, Shahid Sadoughi University of Medical Sciences, Yazd, Iran
Artificial Intelligence is revolutionizing prenatal diagnostics by enhancing the accuracy and efficiency of procedures. This review explores AI and machine learning (ML) in the early detection, prediction, and assessment of neural tube defects (NTDs) through prenatal ultrasound imaging. Recent studies highlight the effectiveness of AI techniques, such as convolutional neural networks (CNNs) and support vector machines (SVMs), achieving detection accuracy rates of up to 95% across various datasets, including fetal ultrasound images, genetic data, and maternal health records. SVM models have demonstrated 71.50% accuracy on training datasets and 68.57% on testing datasets for NTD classification, while advanced deep learning (DL) methods report patient-level prediction accuracy of 94.5% and an area under the receiver operating characteristic curve (AUROC) of 99.3%. AI integration with genomic analysis has identified key biomarkers associated with NTDs, such as Growth Associated Protein 43 (GAP43) and Glial Fibrillary Acidic Protein (GFAP), with logistic regression models achieving 86.67% accuracy. Current AI-assisted ultrasound technologies have improved diagnostic accuracy, yielding sensitivity and specificity rates of 88.9% and 98.0%, respectively, compared to traditional methods with 81.5% sensitivity and 92.2% specificity. AI systems have also streamlined workflows, reducing median scan times from 19.7 min to 11.4 min, allowing sonographers to prioritize critical patient care. Advancements in DL algorithms, including Oct-U-Net and PAICS, have achieved recall and precision rates of 0.93 and 0.96, respectively, in identifying fetal abnormalities. Moreover, AI's evolving role in genetic research supports personalized NTD prevention strategies and enhances public awareness through AI-generated health messages. In conclusion, the integration of AI in prenatal diagnostics significantly improves the detection and assessment of NTDs, leading to greater accuracy and efficiency in ultrasound imaging. As AI continues to advance, it has the potential to further enhance personalized healthcare strategies and raise public awareness about NTDs, ultimately contributing to better maternal and fetal outcomes.
Introduction
Artificial intelligence (AI) is transforming various aspects of medicine, including diagnostics, treatment planning, and patient care (1). AI algorithms improve diagnostic accuracy by analyzing extensive clinical data from electronic health records (EHRs) and medical imaging, facilitating the rapid and precise identification of diseases such as coronary artery disease and cancers (2, 3). AI in radiology improves image interpretation, enhances condition detection via computer-assisted diagnosis systems, and advances prenatal ultrasound diagnostics for fetal anomaly identification (4–6). Moreover, machine learning (ML) models are being developed for predictive analytics, enabling healthcare providers to evaluate patient outcomes based on historical data, which is especially beneficial for managing chronic diseases (7). AI's ability to integrate large datasets allows for the creation of personalized treatment plans tailored to individual genetic and clinical profiles, resulting in more effective therapies (8, 9). Additionally, AI supports clinicians with decision support systems that provide real-time recommendations based on current guidelines, which is crucial in urgent care settings for optimizing triage and resource allocation. The automation of routine administrative tasks through AI can mitigate physician burnout, allowing healthcare providers to focus more on patient care and ultimately enhancing job satisfaction. However, the integration of AI in healthcare raises ethical concerns related to data privacy, algorithmic bias, and the implications of machine decision-making (8, 10). This highlights the necessity for diverse training datasets to ensure equitable clinical outcomes. Despite these challenges, the potential of AI and ML to improve healthcare delivery is substantial, empowering healthcare professionals and contributing to a more efficient system (11, 12).
Neural tube defects (NTDs) are major public health issues arising from incomplete closure of the neural tube during early embryonic development (13, 14). These multifactorial conditions, shaped by genetic and environmental factors, include serious birth defects like spina bifida and anencephaly (15, 16). Figure 1 illustrates the different types of NTDs within their developmental contexts. Their prevalence varies significantly by region due to genetic and environmental factors. Globally, the incidence of NTDs is approximately 2.1 per 100,000 (17), with prevalence rates between 0.3 and 199.4 per 10,000 births (18). Sub-Saharan Africa has the highest incidence at about 4.0 per 100,000 (17), while North America has the lowest at around 0.5 (19). Over the past two decades, NTD incidence has generally declined in about 89% of countries, thanks to improved public health initiatives like folic acid fortification. These trends highlight the urgent need for targeted public health strategies to promote folic acid supplementation among women of childbearing age, increasing awareness and access to this crucial preventive measure (17). The evolution of the field has ushered in the integration of AI, presenting a significant opportunity to enhance the detection, management, and comprehension of NTDs, which could transform maternal and child health interventions (20). AI has made notable strides in the early diagnosis of NTDs by employing ML algorithms that analyze complex datasets, including fetal ultrasound images and maternal health records, to uncover subtle patterns that indicate these conditions much earlier than traditional methods would allow (21, 22). ML techniques are being utilized in several vital areas, such as biomarker identification, predictive modeling, and the analysis of diverse datasets. A prominent application of machine learning lies in identifying biomarkers that facilitate early diagnosis, ultimately improving our understanding of the biological mechanisms underlying NTDs and enabling more targeted interventions (23). Furthermore, ML has been utilized to analyze large datasets, such as EHRs, to predict NTD occurrences based on maternal and environmental factors. A systematic review highlighted the potential of deep learning (DL) models to extract meaningful patterns from complex health data, significantly improving predictive accuracy in healthcare, particularly in prenatal care, where timely interventions can reduce risks associated with NTDs (24). Moreover, ML has been integrated into the study of organoids to simulate and analyze the effects of various genetic and environmental factors on neural tube development (25). This integration enhances the analysis of experimental data, leading to more robust conclusions about the causes and potential treatments for NTDs. Furthermore, the combination of ML with advanced imaging technologies has shown promise in enhancing the detection and classification of defects in embryonic development by identifying subtle morphological changes associated with NTDs, aligning with the broader trend of using ML to improve diagnostic accuracy and efficiency in medical imaging (24, 26).
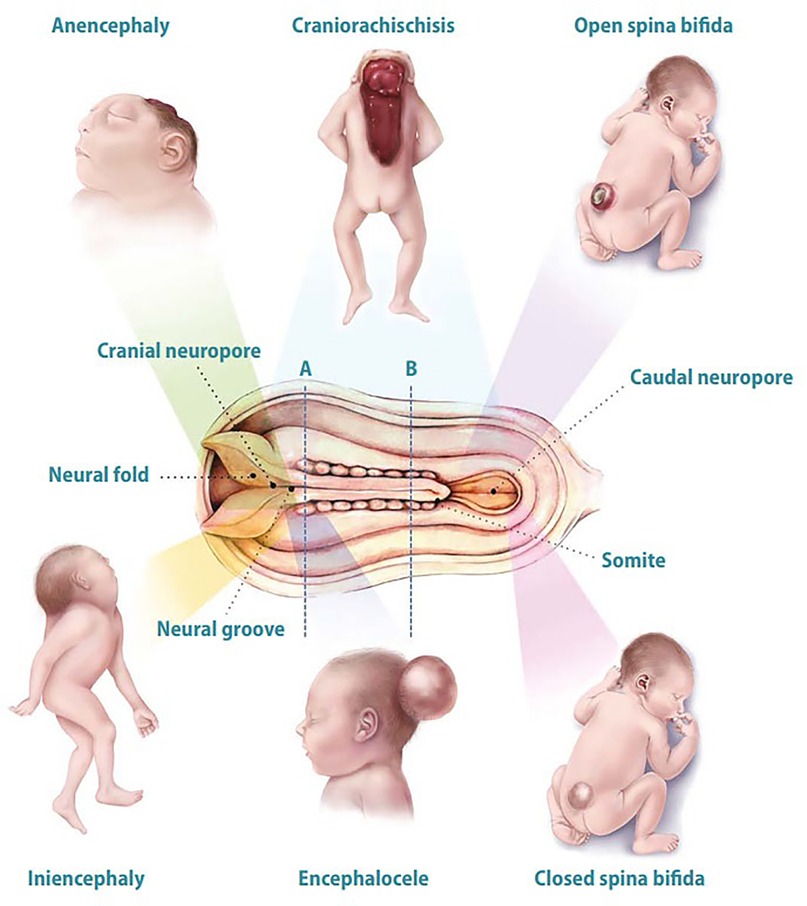
Figure 1. Overview of various NTDs in their developmental contexts. The NTDs depicted include anencephaly (absence of brain and skull), craniorachischisis (complete neural tube failure affecting both skull and spine), open spina bifida (exposed spinal column), closed spina bifida (defect with the spinal cord covered by skin but potential hidden neurological issues), encephalocele (protrusion of brain tissue through an abnormal skull opening), and iniencephaly (malformations of the skull and spine). Key developmental structures—neural groove, neural fold, cranial neuroport, caudal neuroport, and somite—are labeled to contextualize neural tube formation and associated defects. These conditions emerge during the critical phase of neural tube closure, highlighting the importance of proper embryonic development and potential disruptors. In the developing neural tube, area “A” refers to the dorsal aspect involved in patterning, while area “B” represents the ventral aspect where motor neuron progenitors are generated and brain and spinal cord vesicles begin to differentiate. This figure is adapted from Botto et al. (16).
This review examines advancements and future directions in AI applications for diagnosing NTDs. It assesses the effectiveness of various AI techniques for predicting NTDs and classifying related genetic mutations. The review explores methods for biomarker discovery in open NTDs, evaluating their potential to enhance prenatal diagnostics and clinical outcomes. It also highlights the role of AI-assisted ultrasound technology in improving detection accuracy and integrating AI with systems biology to enhance our understanding of NTDs. Innovations in precision medicine, including personalized dietary recommendations for prospective parents and AI-driven health communication strategies for raising awareness about NTD prevention, are emphasized. The review concludes with future research directions, underscoring the need for collaboration between technology developers and clinicians while addressing the ethical implications of AI in this critical area of healthcare.
An overview of machine learning and deep learning
AI, ML, and DL constitute a hierarchical framework of computational intelligence, each with unique methodologies and applications (1). AI is the broad framework for creating intelligent systems that mimic human cognitive functions like problem-solving and learning. ML, a subset of AI, allows systems to learn from data and experiences without explicit programming, using techniques such as supervised, unsupervised, and reinforcement learning (27). DL, a subset of ML, employs multi-layered neural networks to automatically extract features from large datasets. This complexity requires substantial computational power, often provided by Graphics Processing Units (GPUs), which outperform traditional Central Processing Units (CPUs) in parallel processing. While ML is suitable for simpler tasks and smaller datasets, DL excels in complex applications like image and speech recognition due to its advanced architecture. The rise of GPUs and other hardware accelerators has significantly enhanced the speed of training and efficiency of inference in DL (28–30). ML encompasses various algorithms that derive insights and make predictions across fields such as agriculture, healthcare, finance, and e-commerce, with performance heavily reliant on the quality and quantity of training data. In contrast, DL utilizes neural networks, including convolutional neural networks (CNNs) for image processing and recurrent neural networks (RNNs) for sequential data analysis, to handle complex data types (1, 31). Recent advancements in DL, especially in transformers and self-attention mechanisms, have significantly improved capabilities in tasks like language translation, making DL crucial for high-accuracy applications such as autonomous driving and robotics. The integration of ML and DL techniques enhances performance on complex problems, as seen in hybrid models that combine CNNs and long short-term memory networks (LSTMs) for text classification (32, 33). Despite their different methodologies, the interplay between ML and DL creates a robust framework for tackling complex challenges, with ongoing research necessary to address computational demands and model interpretability, optimizing these technologies for broader applications (34). AUROC is a vital metric for evaluating AI algorithms, particularly in binary classification tasks, as it measures a model's ability to distinguish between positive and negative classes across various thresholds, providing insights into sensitivity and specificity. An AUROC of 1 indicates perfect classification, while 0.5 suggests no discriminative ability, akin to random guessing. This metric is especially useful in scenarios with imbalanced class distributions, allowing for a more nuanced comparison of algorithms beyond simple accuracy. Incorporating AUROC into the evaluation process helps researchers and practitioners better understand the trade-offs associated with their AI models, ultimately enhancing decision-making capabilities (35).
Use of AI in prenatal diagnostics
AI, particularly through ML and DL, is revolutionizing prenatal diagnostics by significantly enhancing the accuracy and efficiency of fetal assessments. These technologies are increasingly applied in various facets of prenatal care, such as screening for congenital anomalies, predicting pregnancy complications, and improving ultrasound imaging (21, 36, 37). AI algorithms, especially DL models, excel at analyzing ultrasound images to identify fetal anomalies that may be overlooked by human operators. For example, CNNs have been utilized to standardize fetal anatomy assessments, which minimizes inter-operator variability and boosts diagnostic consistency (38, 39). This advancement allows for more precise evaluations of conditions like congenital heart defects and kidney anomalies. Furthermore, AI has markedly improved non-invasive prenatal testing (NIPT) methods by elevating detection rates of chromosomal abnormalities through the analysis of complex datasets from maternal blood samples, offering safer diagnostic options for expectant mothers (40, 41). Moreover, ML models are employed to develop predictive analytics that can identify pregnancies at risk for complications such as preterm birth and preeclampsia by examining various factors, including maternal history and biophysical parameters, thus enabling personalized care strategies. AI technologies also support continuous monitoring of maternal and fetal health parameters through wearable devices that track vital signs, providing immediate alerts for any deviations and facilitating timely medical interventions (42, 43).
Thatoi et al. recently conducted a systematic review and meta-analysis on the effectiveness of AI algorithms for detecting congenital fetal abnormalities, particularly in the heart and brain. Analyzing data from 243,456 pregnancies, they found that DL and ML models exhibited high diagnostic performance, with sensitivity between 82% and 99% and specificity from 78% to 99%. Their analysis, utilizing the DerSimonian-Laird technique and Summary Receiver Operating Characteristic (SROC) plots, achieved a significant area under the SROC curve of 0.960, indicating a strong ability of these AI algorithms to distinguish between abnormal and normal cases across the included studies. Individual studies reported AUROC curves ranging from 0.85 to 0.95, demonstrating good to excellent discrimination. These findings highlight the potential of AI algorithms, especially CNNs, to deliver reliable diagnostic predictions, potentially reducing infant mortality and improving treatment outcomes for affected pregnancies (44). Moreover, Spahić et al. (45) advanced the integration of AI in diagnosing fetal neurological impairment disorders with their TRUEAID system. This innovative approach combines the finely tuned Kurjak Antenatal Neurodevelopmental Test (KANET), which utilizes four-dimensional ultrasound technology to assess fetal neurological development during the critical third trimester, with a convolutional neural network to create an AI-powered ultrasound module for automated diagnostic support. The study reports an accuracy of 93.83%, highlighting the system's potential for early detection of conditions like cerebral palsy, epilepsy, and autism spectrum disorder (45). The KANET, developed over the past 15 years, evaluates fetal behavior and movement patterns through a brief 15–20 min ultrasound session, meticulously recording specific criteria such as head, hand, and foot movements, eye movements, facial expressions, and the continuity of complex movements. The research emphasizes the importance of early diagnosis for timely intervention and illustrates the viability of AI technologies in enhancing fetal neurobehavioral assessments. This pilot study lays the groundwork for AI-based diagnostics, with significant implications for improving outcomes for affected children and expanding access to advanced diagnostic capabilities in various healthcare settings (46, 47).
Use of AI for early detection of neural tube defects
AI offers a transformative approach to prenatal diagnostics, but challenges persist, including the requirement for large labeled datasets and the integration of AI tools into clinical practice. Still, AI holds significant potential for enhancing early detection and intervention for NTDs. Table 1 provides an overview of AI applications for the early detection and prediction of NTDs. Mahalle et al. (22) investigated the application of ML techniques, specifically CNNs and gradient boosting machines (GBMs), for the early detection of birth defects. The study emphasized the importance of early identification of these defects to facilitate timely intervention. By utilizing a diverse range of datasets, including fetal ultrasound images, genetic information, maternal health records, and demographic details, the authors trained their ML models on labeled data containing accurate diagnoses of various birth defects. The proposed methodology not only achieved high accuracy in detecting NTDs, fetal heart abnormalities, and chromosomal issues but also aimed to enhance understanding of the biological processes underlying these conditions. The user-friendly interface designed for healthcare professionals further supported its practical implementation, enabling them to provide prompt guidance and assistance to expecting parents (22). Vahedifard et al. (51) conducted a narrative review on the application of AI and ML techniques in fetal brain magnetic resonance imaging (MRI). The study highlights the prevalence of central nervous system abnormalities in fetuses, which occur in approximately 0.1% to 0.2% of live births and 3% to 6% of stillbirths, emphasizing the importance of early detection and categorization of these conditions. The authors discuss various AI models, primarily CNNs and U-Net architectures, that have been employed for automating the processing of anatomical fetal brain MRI. Notably, some models demonstrated accuracy rates exceeding 95%. The review also outlines the capabilities of AI in tasks such as gestational age prediction, fetal brain extraction and segmentation, and placenta detection. Furthermore, the study addresses the use of classification algorithms, including K-nearest neighbor and random forest, for identifying brain pathologies. The authors stress the need for large-scale, labeled datasets to enhance the efficacy of DL methods, as well as the importance of shared fetal brain MRI datasets due to the limited availability of such images. They conclude by highlighting the necessity for healthcare professionals, particularly neuroradiologists, general radiologists, and perinatologists, to understand the role of AI in improving fetal brain MRI diagnostics (51).
Use of AI in prediction and assessment of severity of neural tube defects
Techniques like Support Vector Machines (SVM) and Recursive Feature Elimination (RFE) are effectively employed to analyze genetic and demographic data, enhancing the understanding and prediction of NTDs such as spina bifida (23). SVM classifies NTD occurrences by merging genetic and demographic datasets, clarifying risk factors for these birth defects (49). RFE refines feature selection to identify the most significant genetic markers. This integration improves model accuracy and informs public health initiatives and preventive measures for at-risk populations. The research spans public health, genetics, and maternal-fetal health, highlighting the importance of interdisciplinary approaches to complex health issues (53, 54). Current models show some predictive ability, but further research is necessary to refine methods and incorporate additional risk factors for better prenatal care outcomes. Wang et al. demonstrated that SVM models predicted NTDs with 71.50% accuracy on training datasets and 68.57% on testing datasets, emphasizing the need for robust datasets to enhance predictive performance. While these accuracy rates indicated potential for classifying and forecasting NTD rates at the village level, significant room for improvement remained. Future research should have focused on optimizing model parameters, exploring additional features, and integrating other ML algorithms (48). In contrast, Weaver et al. (20) advanced DL techniques for assessing neurogenic bladder dysfunction in spina bifida patients. Their ensemble model combined volume-pressure recordings—measuring urine volume and bladder pressure—with fluoroscopic imaging data, achieving 70% accuracy (95% CI 66%, 76%). However, a weighted kappa of 0.54 signifies only moderate agreement between the model's predictions and clinical evaluations, indicating substantial improvement is needed. The scale ranges from −1 (complete disagreement) to 1 (perfect agreement). These results underscore the importance of refining and validating the DL model to enhance its clinical applicability and reliability. With the clinical model showing only 61% accuracy and a weighted kappa of 0.37, the findings highlight the advantages of DL over traditional methods for more effectively assessing bladder dysfunction severity in this vulnerable population (20). Moreover, a 2025 study by Qi et al. highlighted DL's immense potential, reporting a patient-level prediction accuracy of 94.5% and an AUROC of 99.3% for various NTDs, with models generating heatmaps to assist clinicians in evaluating anomaly severity (52). Further exploration by Karthik et al. in 2022 employed RFE to identify critical genetic markers linked to open NTDs, emphasizing the multifactorial nature of NTDs and the necessity of integrating genetic insights into predictive models to enhance their accuracy and applicability in clinical settings (23). This holistic approach underscores the importance of multifaceted strategies in advancing the predictive power of models for complex health conditions such as NTDs.
Integration of AI with biomarkers for detection of neural tube defects
AI models excel at analyzing extensive genomic datasets to identify various genetic variants, such as single-nucleotide variations (SNVs) and copy number variants (CNVs). These models enhance the sensitivity and specificity of variant calling, which is crucial for pinpointing mutations linked to congenital disorders (55). Tools like SpliceAI and MMSplice are often integrated into AI frameworks to prioritize harmful variants that may contribute to NTDs (56). Researchers at Weill Cornell Medicine have applied a ML approach to explore genetic variations associated with spina bifida, a type of NTD. By examining the genomes of 149 individuals with spina bifida and 149 healthy controls, they discovered function-altering variants in genes that differentiate affected individuals from those without the condition. The study highlighted significant pathways related to glucose and lipid metabolism, which are vital for cellular energy and influenced by maternal health factors like diabetes and obesity, known risk factors for NTDs. The findings demonstrate the efficacy of ML algorithms in classifying genetic data, enabling the identification of genes that distinguish spina bifida cases from controls, thus minimizing biases present in traditional studies. These insights could inform targeted preventative strategies, including personalized nutritional advice for couples planning to conceive. Moreover, the researchers aim to establish an international consortium to expand their investigation, enhancing the understanding of the genetic basis of spina bifida across diverse populations and promoting personalized medicine approaches to prevent this serious condition (49). Furthermore, a study by Karthik et al. (23) employed a ML technique, specifically RFE and differential gene expression analysis, to identify key biomarkers related to open NTDs, particularly myelomeningocele, by analyzing amniotic fluid datasets (GSE4182 and GSE101141). The research identified four significant biomarkers: Growth Associated Protein 43 (GAP43), Glial Fibrillary Acidic Protein (GFAP), Repetin (RPTN), and CD44, which are involved in critical processes such as axon growth, astrocyte differentiation, and neuroinflammation. The findings were validated using various binary classifiers, with logistic regression achieving an accuracy of 86.67% and an AUC-ROC of 0.90 in distinguishing affected from healthy samples. GAP43 and GFAP are particularly important for early diagnosis due to their roles in neurodevelopment and response to central nervous system injuries, with elevated levels indicating abnormal neurodevelopment and astroglial activation, respectively. This research highlights the potential of these biomarkers to improve prenatal diagnostic methods for conditions like myelomeningocele, enabling healthcare providers to identify at-risk pregnancies earlier and facilitating timely interventions for better outcomes in newborns (23). In their 2021 study, Mustafa et al. investigated the relationship between gene polymorphism and folic acid interactions in the context of NTDs. The research highlighted the significance of the minimum folate carrier (MFC A80G) gene polymorphism, particularly the homozygous mutant type (GG) genotype, alongside maternal folic acid consumption in reducing the prevalence of NTDs. Utilizing a grey wolf optimizer-assisted deep recurrent neural network, the authors achieved an impressive accuracy rate of 99.5% in predicting the associations between these factors. Their findings indicated a remarkably low error ratio of 0.015%, underscoring the effectiveness of combining genetic information with nutritional factors to mitigate the risk of NTDs in offspring (50). Folic acid plays a crucial role in reducing the risk of NTDs, including spina bifida and anencephaly, during pregnancy. As a vital B vitamin, it is essential for DNA synthesis, repair, and methylation—processes critical for early fetal development. Once converted to tetrahydrofolate (THF) in the body, folic acid supports nucleotide synthesis, aiding in the formation and closure of the neural tube (57). Research indicates that women who consume sufficient folic acid before and during early pregnancy can significantly lower their risk of NTDs (13, 14).
The integration of ML with systems biology is transforming the study of complex genetic conditions like spina bifida by allowing researchers to analyze genetic data in conjunction with environmental factors (49). This multidisciplinary approach enhances the understanding of conditions NTDs, which are influenced by both genetic predispositions and environmental exposures. By utilizing ML algorithms to process large datasets, researchers can effectively identify at-risk populations and develop targeted interventions, overcoming challenges faced by genome-wide association studies (GWAS) in detecting subtle genetic signals associated with rare conditions (58, 59). Ultimately, the goal is to create personalized prevention strategies tailored to individual families, allowing healthcare providers to offer customized advice and interventions that could reduce the risk of spina bifida and other NTDs. As ML continues to evolve, future research will likely focus on sophisticated models that incorporate various datasets, further improving predictive capabilities and deepening our understanding of complex genetic conditions (49). Overall, these studies underscores the transformative role of ML in biomedical research, particularly in identifying critical biomarkers for complex conditions such as open NTDs and advancing personalized maternal-fetal healthcare.
Integration of AI in prenatal ultrasound imaging
Currently, there are relatively few studies utilizing AI to predict NTDs, with most existing models focusing on binary classification (normal vs. abnormal) or identifying general intracranial image patterns (60, 61). However, AI-assisted ultrasound technology is significantly enhancing the detection of NTDs and other fetal anomalies through various mechanisms. One of the most notable advancements is in diagnostic accuracy, where AI algorithms have achieved sensitivity and specificity rates as high as 88.9% and 98.0%, respectively. These rates surpass those of traditional methods, which report lower sensitivity at 81.5% and specificity at 92.2% (24, 26). Moreover, AI systems automate routine tasks, such as capturing standard image planes and measuring biometric parameters, which has reduced median scan times from 19.7 min to 11.4 min, thereby easing the cognitive burden on sonographers and allowing them to concentrate on other critical patient care aspects (26, 62). This streamlining leads to enhanced workflow efficiency, enabling sonographers to conduct smoother examinations with fewer interruptions and fostering better patient interactions. Moreover, AI provides real-time quality assurance during ultrasound examinations, offering immediate feedback to ensure that images meet diagnostic standards and mitigating human judgment errors. It also addresses traditional ultrasound limitations, such as fetal movement and inter-observer variability, by improving measurement consistency and anomaly detection under less-than-ideal conditions. Beyond NTDs, AI applications extend to assessing other fetal conditions, like congenital heart disease and brain abnormalities, with high accuracy in distinguishing normal from abnormal fetal brain structures (39, 63). This integration of AI into ultrasound technology represents a transformative advancement in prenatal care, significantly improving the screening and diagnosis of fetal anomalies and ultimately leading to better outcomes for affected infants and families. As research progresses, further advancements are anticipated, paving the way for fully automated systems and enhanced collaboration between AI developers and medical professionals. Overall, AI is transforming obstetric ultrasound by improving diagnostic capabilities, optimizing workflows, and facilitating timely medical interventions, ultimately enhancing prenatal care and outcomes for affected infants and families (4, 24).
Table 2 showcases advancements in DL algorithms for diagnosing fetal abnormalities, such as NTDs, using ultrasound and MRI, highlighting their potential to enhance diagnostic accuracy and efficiency in clinical settings. Recent studies emphasize notable progress in DL techniques for classifying and detecting fetal brain abnormalities through these imaging modalities. Chen et al. (62) developed an enhanced convolutional neural network algorithm called Oct-U-Net, aimed at improving the automatic recognition and diagnosis of fetal spina bifida in three-dimensional (3D) ultrasound images. This research involved 3,300 pregnant women who underwent 3D ultrasound examinations, with Oct-U-Net evaluated using various metrics such as recall rate, precision rate, pixel accuracy (PA), mean intersection over union (MIoU), and mean standard error. The findings revealed that Oct-U-Net outperformed both the fully convolutional network (FCN) and the original U-Net algorithm, achieving recall and precision rates of 0.93 and 0.96, respectively, alongside a PA of 0.949 and an MIoU of 0.917. Moreover, Oct-U-Net demonstrated a notably lower mean standard error of 4.1243 and a reduced average running time of 12.15 s compared to other algorithms, suggesting its superior diagnostic capabilities for fetal spina bifida in 3D ultrasound imaging (67). Xie et al. (64) examined the feasibility of DL algorithms on a large dataset from a hospital database, which included 10,251 normal and 2,529 abnormal pregnancies, ultimately analyzing 15,372 normal and 14,047 abnormal ultrasound images. Their algorithms achieved impressive segmentation precision, recall, and Dice's coefficient of 97.9%, 90.9%, and 94.1%, respectively, with an overall classification accuracy of 96.3%, sensitivity of 96.9%, and specificity of 95.9% (64). Lin et al. (65) introduced the Prenatal ultrasound diagnosis Artificial Intelligence Conduct System (PAICS), utilizing a dataset of 43,890 images and 169 videos from 16,297 pregnancies. PAICS demonstrated robust diagnostic performance in identifying ten types of intracranial abnormalities, achieving macro- and microaverage areas under the receiver-operating-characteristics curve (AUC) of 0.933 and 0.977 for internal validation, respectively (65). Chowdhury et al. (66) developed StackFBAs, a novel DL framework for detecting fetal brain abnormalities from MRI images, achieving an overall accuracy of 80% and incorporating federated learning techniques (66). Qi et al. (52) advanced the field further with a model focused on detecting central nervous system anomalies, achieving a patient-level prediction rate of 94.5% and an AUROC of 99.3% (52). These studies collectively illustrate the transformative potential of DL in prenatal diagnostics, significantly enhancing healthcare delivery and patient outcomes.
Future directions
The integration of AI into genetic research is transforming NTD prevention by enabling personalized strategies for prospective parents. AI allows for the thorough analysis of genetic data to identify mutations and variations that increase the risk of NTDs, while also factoring in environmental influences. This comprehensive approach enables healthcare providers to offer tailored recommendations, such as personalized nutritional advice on folic acid, which effectively reduces NTD risks. The goal is to empower families by considering their unique genetic backgrounds, lifestyle choices, and dietary needs. Moreover, AI enhances traditional GWAS to overcome challenges associated with the rarity of NTDs, leading to more robust findings (68–70). As AI technology advances, its role in genetic research is expected to expand, promoting interdisciplinary collaboration that translates findings into clinical practice and paving the way for personalized NTD prevention strategies.
AI-generated health messages are proving effective in raising public awareness about NTD prevention, particularly regarding the importance of folic acid. Research shows that these messages often match or exceed the clarity and effectiveness of human-created content, as seen in studies like the Folic Acid Message Engine (71, 72). This technology produces tailored messages that effectively engage specific demographics, ensuring broader reach on social media. Moreover, AI can generate a consistent flow of fresh content, addressing challenges in maintaining long-term health communication campaigns. By providing timely reminders and educational materials for prospective parents, AI helps close significant knowledge gaps about folic acid's role in reducing NTD risks. As AI technology evolves, its application in health communication may grow, potentially leading to more sophisticated algorithms that analyze audience responses in real-time, while also emphasizing the importance of ethical considerations. Overall, AI-generated health messages offer a valuable opportunity to enhance public understanding of NTD prevention and improve health outcomes for at-risk communities.
Conclusions
The integration of AI into the study and prevention of NTDs represents a significant advancement in research and clinical practice. Studies showcase various applications of these technologies, such as predictive modeling, genetic mutation identification, biomarker discovery, and AI-assisted imaging, which enhance diagnostic accuracy, patient care, and personalized prevention strategies based on individual genetic and environmental factors. As research evolves, the potential for precision medicine grows, allowing healthcare providers to offer targeted recommendations based on genetic predispositions and lifestyle choices. Furthermore, AI-driven health awareness campaigns can improve public understanding of NTD prevention, guiding informed decisions by prospective parents. Moving forward, combining ML techniques with traditional medical practices will enhance our understanding of complex conditions like spina bifida and promote innovative solutions. Collaborative efforts across disciplines will be essential for translating these advancements into practical benefits for at-risk patients. This ongoing exploration underscores AI's crucial role in the future of maternal-fetal medicine and public health initiatives aimed at reducing NTD incidence.
Author contributions
MY: Conceptualization, Writing – original draft, Writing – review & editing. MD: Conceptualization, Writing – original draft, Writing – review & editing. SA: Investigation, Writing – original draft, Writing – review & editing. FJ: Investigation, Writing – original draft, Writing – review & editing. RB: Methodology, Writing – original draft, Writing – review & editing. SD: Conceptualization, Methodology, Writing – original draft, Writing – review & editing. HR: Investigation, Writing – original draft, Writing – review & editing. AM: Investigation, Writing – original draft, Writing – review & editing. AS: Methodology, Writing – original draft, Writing – review & editing. KA: Methodology, Writing – original draft, Writing – review & editing. MN: Conceptualization, Validation, Writing – review & editing. HN: Conceptualization, Investigation, Methodology, Writing – original draft, Writing – review & editing.
Funding
The author(s) declare that no financial support was received for the research and/or publication of this article.
Conflict of interest
The authors declare that the research was conducted in the absence of any commercial or financial relationships that could be construed as a potential conflict of interest.
Generative AI statement
The authors declare that no Gen AI was used in the creation of this manuscript.
Publisher's note
All claims expressed in this article are solely those of the authors and do not necessarily represent those of their affiliated organizations, or those of the publisher, the editors and the reviewers. Any product that may be evaluated in this article, or claim that may be made by its manufacturer, is not guaranteed or endorsed by the publisher.
Abbreviations
AI, artificial intelligence; NTDs, neural tube defects; EHRs, electronic health records; NIPT, non-invasive prenatal testing; DL, deep learning; ML, machine learning; CNNs, convolutional neural networks; RNNs, recurrent neural networks; AUROC, area under the receiver operating characteristic curve; AUC, area under the curve; SROC, summary receiver operating characteristic; GBMs, gradient boosting machines; SVM, support vector machine; RFE, recursive feature elimination; SNVs, single-nucleotide variants; CNVs, copy number variants; GWAS, genome-wide association studies; MFC, minimum folate carrier; MFC A80G, minimum folate carrier A80G; THF, tetrahydrofolate; GAP43, growth associated protein 43; GFAP, glial fibrillary acidic protein; RPTN, repetin; PA, pixel accuracy; MIoU, mean intersection over union; PAICS, prenatal ultrasound diagnosis artificial intelligence conduct system; GPUs, graphics processing units; CPUs, central processing units; KANET, kurjak antenatal neurodevelopmental test; MRI, magnetic resonance imaging.
References
1. Ali M, Dewan A, Sahu AK, Taye MM. Understanding of machine learning with deep learning: architectures, workflow, applications and future directions. Computers. (2023) 12:91. doi: 10.3390/COMPUTERS12050091
2. Krishnan G, Singh S, Pathania M, Gosavi S, Abhishek S, Parchani A, et al. Artificial intelligence in clinical medicine: catalyzing a sustainable global healthcare paradigm. Front Artif Intell. (2023) 6:1227091. doi: 10.3389/FRAI.2023.1227091
3. Najjar R. Redefining radiology: a review of artificial intelligence integration in medical imaging. Diagnostics. (2023) 13:2760. doi: 10.3390/DIAGNOSTICS13172760
4. He F, Wang Y, Xiu Y, Zhang Y, Chen L. Artificial intelligence in prenatal ultrasound diagnosis. Front Med. (2021) 8:729978. doi: 10.3389/FMED.2021.729978
5. Nafissi N, Heiranizadeh N, Shirinzadeh-Dastgiri A, Vakili-Ojarood M, Naseri A, Danaei M, et al. The application of artificial intelligence in breast cancer. Eurasian J Med Oncol. (2024) 8:235–44. doi: 10.14744/EJMO.2024.45903
6. HaghighiKian SM, Shirinzadeh-Dastgiri A, Vakili-Ojarood M, Naseri A, Barahman M, Saberi A, et al. A holistic approach to implementing artificial intelligence in lung cancer. Indian J Surg Oncol. (2024) 2024:1–22. doi: 10.1007/S13193-024-02079-6
7. Maleki Varnosfaderani S, Forouzanfar M. The role of AI in hospitals and clinics: transforming healthcare in the 21st century. Bioengineering. (2024) 11:337. doi: 10.3390/BIOENGINEERING11040337
8. Alowais SA, Alghamdi SS, Alsuhebany N, Alqahtani T, Alshaya AI, Almohareb SN, et al. Revolutionizing healthcare: the role of artificial intelligence in clinical practice. BMC Med Educ. (2023) 23:689. doi: 10.1186/s12909-023-04698-z
9. Dixon D, Sattar H, Moros N, Kesireddy SR, Ahsan H, Lakkimsetti M, et al. Unveiling the influence of AI predictive analytics on patient outcomes: a comprehensive narrative review. Cureus. (2024) 16:e59954. doi: 10.7759/CUREUS.59954
10. Rony MKK, Parvin MR, Ferdousi S. Advancing nursing practice with artificial intelligence: enhancing preparedness for the future. Nurs Open. (2023) 11:10.1002/nop2.2070. doi: 10.1002/NOP2.2070
11. Vakili-Ojarood M, Naseri A, Shirinzadeh-Dastgiri A, Saberi A, HaghighiKian SM, Rahmani A, et al. Ethical considerations and equipoise in cancer surgery. Indian J Surg Oncol. (2024) 15:363–73. doi: 10.1007/S13193-024-02023-8
12. Danaei M, Yeganegi M, Azizi S, Jayervand F, Shams SE, Sharifi MH, et al. Machine learning applications in placenta accreta spectrum disorders. Eur J Obstet Gynecol Reprod Biol X. (2025) 25:100362. doi: 10.1016/J.EUROX.2024.100362
13. Soleimani-Jadidi S, Meibodi B, Javaheri A, Tabatabaei RS, Hadadan A, Zanbagh L, et al. Association between fetal MTHFR A1298C (rs1801131) polymorphism and neural tube defects risk: a systematic review and meta-analysis. Fetal Pediatr Pathol. (2022) 41:116–33. doi: 10.1080/15513815.2020.1764682
14. Tabatabaei RS, Fatahi-Meibodi N, Meibodi B, Javaheri A, Abbasi H, Hadadan A, et al. Association of fetal MTHFR C677T polymorphism with susceptibility to neural tube defects: a systematic review and update meta-analysis. Fetal Pediatr Pathol. (2022) 41:225–41. doi: 10.1080/15513815.2020.1775734
15. Greene NDE, Copp AJ. Neural tube defects NTDs: neural tube defects. Annu Rev Neurosci. (2014) 37:221–42. doi: 10.1146/annurev-neuro-062012-170354
16. Botto LD, Moore CA, Khoury MJ, Erickson JD. Neural-tube defects. N Engl J Med. (1999) 341:1509–19. doi: 10.1056/NEJM199911113412006
17. Lu VM. Global, regional, and national epidemiological trends in neural tube defects between 1990 and 2019: a summary. Childs Nerv Syst. (2023) 39:3103–9. doi: 10.1007/S00381-023-05985-2
18. Zaganjor I, Sekkarie A, Tsang BL, Williams J, Razzaghi H, Mulinare J, et al. Describing the prevalence of neural tube defects worldwide: a systematic literature review. PLoS One. (2016) 11:e0151586. doi: 10.1371/journal.pone.0151586
19. Brinkhof M, Meier S, Chamberlain J, Mader L. The impact of folic acid food fortification on global incidence of neural tube defects: systematic review, meta-analysis and estimate of preventable cases. Int J Epidemiol. (2015) 44:i143–i143. doi: 10.1093/IJE/DYV096.160
20. Weaver JK, Martin-Olenski M, Logan J, Broms R, Antony M, Van Batavia J, et al. Deep learning of videourodynamics to classify bladder dysfunction severity in patients with spina Bifida. J Urol. (2023) 209:994–1003. doi: 10.1097/JU.0000000000003267
21. Miskeen E, Alfaifi J, Alhuian DM, Alghamdi M, Alharthi MH, Alshahrani NA, et al. Prospective applications of artificial intelligence in fetal medicine: a scoping review of recent updates. Int J Gen Med. (2025) 18:237. doi: 10.2147/IJGM.S490261
22. Mahalle PN, Mahajan RA, Takale DG. Early Detection of Birth Defects Using Machine Learning. Hershey, PA: IGI Global (2024). p. 1–30. doi: 10.4018/979-8-3693-3711-0.CH001
23. Karthik KV, Rajalingam A, Shivashankar M, Ganjiwale A. Recursive feature elimination-based biomarker identification for open neural tube defects. Curr Genomics. (2022) 23:195–206. doi: 10.2174/1389202923666220511162038
24. Xiao S, Zhang J, Zhu Y, Zhang Z, Cao H, Xie M, et al. Application and progress of artificial intelligence in fetal ultrasound. J Clin Med. (2023) 12:3298. doi: 10.3390/JCM12093298
25. Bai L, Wu Y, Li G, Zhang W, Zhang H, Su J. AI-enabled organoids: construction, analysis, and application. Bioact Mater. (2024) 31:525–48. doi: 10.1016/J.BIOACTMAT.2023.09.005
26. Day TG, Matthew J, Budd SF, Farruggia A, Venturini L, Wright R, et al. Artificial intelligence to assist in the screening fetal anomaly ultrasound scan (PROMETHEUS): a randomised controlled trial. medRxiv. (2024) 2024:05.23.24307329. doi: 10.1101/2024.05.23.24307329
27. Jin L, Zhao Q, Fu S, Cao F, Hou B, Ma J. Development and validation of machine learning models to predict survival of patients with resected stage-III NSCLC. Front Oncol. (2023) 13:1092478. doi: 10.3389/FONC.2023.1092478
28. Hsu JC, Nguyen PA, Phuc PT, Lo TC, Hsu MH, Hsieh MS, et al. Development and validation of novel deep-learning models using multiple data types for lung cancer survival. Cancers. (2022) 14:5562. doi: 10.3390/CANCERS14225562
29. Jeon W, Ko G, Lee J, Lee H, Ha D, Ro WW. Deep learning with GPUs. Adv Compu. (2021) 122:167–215. doi: 10.1016/BS.ADCOM.2020.11.003
30. Al Maruf M, Azim A, Auluck N, Sahi M. Optimizing DNN training with pipeline model parallelism for enhanced performance in embedded systems. J Parallel Distrib Comput. (2024) 190:104890. doi: 10.1016/J.JPDC.2024.104890
31. Sarker IH. Machine learning: algorithms, real-world applications and research directions. SN Comput Sci. (2021) 2:1–21. doi: 10.1007/S42979-021-00592-X/FIGURES/11
32. Pandey M, Fernandez M, Gentile F, Isayev O, Tropsha A, Stern AC, et al. The transformational role of GPU computing and deep learning in drug discovery. Nat Mach Intell. (2022) 43:211–21. doi: 10.1038/s42256-022-00463-x
33. Ahmed N, Saha AK, Al Noman MA, Jim JR, Mridha MF, Kabir MM. Deep learning-based natural language processing in human–agent interaction: applications, advancements and challenges. Nat Lang Process J. (2024) 9:100112. doi: 10.1016/J.NLP.2024.100112
34. Chakraborty C, Bhattacharya M, Pal S, Lee SS. From machine learning to deep learning: advances of the recent data-driven paradigm shift in medicine and healthcare. Curr Res Biotechnol. (2024) 7:100164. doi: 10.1016/J.CRBIOT.2023.100164
35. Marzban C. The ROC curve and the area under it as performance measures. Weather Forecast. (2004) 19:1106–14. doi: 10.1175/825.1
36. Masoudi A, Omidi A, Shams SE, Yeganegi M, Danaei M, Shiri A, et al. Applications of artificial intelligence chatbots in congenital diplopodia: a real-world perspective on a rare case of duplicated lower limb. World J Peri Neonatol. (2024) 7:7–15. doi: 10.18502/WJPN.V7I1.17323
37. Naseri A, Antikchi MH, Barahman M, Shirinzadeh-Dastgiri A, HaghighiKian SM, Vakili-Ojarood M, et al. AI Chatbots in oncology: a comparative study of sider fusion AI and perplexity AI for gastric cancer patients. Indian J Surg Oncol. (2024) 15:1–10. doi: 10.1007/S13193-024-02145-Z/METRICS
38. Ramirez Zegarra R, Ghi T. Use of artificial intelligence and deep learning in fetal ultrasound imaging. Ultrasound Obstet Gynecol. (2023) 62:185–94. doi: 10.1002/UOG.26130
39. Yousefpour Shahrivar R, Karami F, Karami E. Enhancing fetal anomaly detection in ultrasonography images: a review of machine learning-based approaches. Biomimetics. (2023) 8:519. doi: 10.3390/BIOMIMETICS8070519
40. Ejaz H, Thyyib T, Ibrahim A, Nishat A, Malay J. Role of artificial intelligence in early detection of congenital heart diseases in neonates. Front Digit Heal. (2024) 5:1345814. doi: 10.3389/FDGTH.2023.1345814
41. Lee J, Lee SM, Ahn JM, Lee TR, Kim W, Cho EH, et al. Development and performance evaluation of an artificial intelligence algorithm using cell-free DNA fragment distance for non-invasive prenatal testing (aiD-NIPT). Front Genet. (2022) 13:999587. doi: 10.3389/FGENE.2022.999587/BIBTEX
42. Mennickent D, Rodríguez A, Opazo MC, Riedel CA, Castro E, Eriz-Salinas A, et al. Machine learning applied in maternal and fetal health: a narrative review focused on pregnancy diseases and complications. Front Endocrinol. (2023) 14:1130139. doi: 10.3389/FENDO.2023.1130139/BIBTEX
43. Kaya Y, Bütün Z, Çelik Ö, Salik EA, Tahta T. Risk assessment for preeclampsia in the preconception period based on maternal clinical history via machine learning methods. J Clin Med. (2024) 14:155. doi: 10.3390/JCM14010155
44. Thatoi P, Choudhary R, Khairari A, Rout SS, Selvarathinam AL. Bridging prenatal diagnostics and AI: a systematic review and meta-analysis of the efficacy of advanced algorithms in identifying congenital fetal abnormalities. Asian J Biol. (2024) 20:10–21. doi: 10.9734/AJOB/2024/V20I10439
45. Spahić L, Badnjević A, Kurjak A, Gurbeta Pokvić L. Integration of artificial intelligence-based systems in diagnostic pathways: tRUEAID case study. Artif Intell Ind 40 Futur That Comes True. (2024):270–9. doi: 10.5644/PI2024.215.15
46. Honemeyer U, Talic A, Therwat A, Paulose L, Patidar R. The clinical value of KANET in studying fetal neurobehavior in normal and at-risk pregnancies. J Perinat Med. (2013) 41:187–97. doi: 10.1515/JPM-2011-0251/MACHINEREADABLECITATION/RIS
47. Hata T, Kanenishi K, Mori N, AboEllail MAM, Hanaoka U, Koyano K, et al. Mini KANET: simple fetal antenatal neurodevelopmental test. Donald Sch J Ultrasound Obstet Gynecol. (2019) 13:59–63. doi: 10.5005/JP-JOURNALS-10009-1586
48. Wang JF, Liu X, Liao YL, Chen HY, Li WX, Zheng XY. Prediction of neural tube defect using support vector machine. Biomed Environ Sci. (2010) 23:167–72. doi: 10.1016/S0895-3988(10)60048-7
49. Aguiar-Pulido V, Wolujewicz P, Martinez-Fundichely A, Elhaik E, Thareja G, Aleem AA, et al. Systems biology analysis of human genomes points to key pathways conferring spina bifida risk. Proc Natl Acad Sci U. (2021) 118:e2106844118. doi: 10.1073/PNAS.2106844118/-/DCSUPPLEMENTAL
50. Mustafa I, Saad A, Mahmoud MH, Alamery S, Mahmoud NM. Analyzing gene polymorphism and metal folic acid interactions in neural tube defects using optimized deep recurrent neural networks. Pers Ubiquitous Comput. (2023) 27:861–73. doi: 10.1007/S00779-021-01538-Z
51. Vahedifard F, Adepoju JO, Supanich M, Ai HA, Liu X, Kocak M, et al. Review of deep learning and artificial intelligence models in fetal brain magnetic resonance imaging. World J Clin Cases. (2023) 11:3725. doi: 10.12998/WJCC.V11.I16.3725
52. Qi Y, Cai J, Lu J, Xiong R, Chen R, Zheng L, et al. Multi-Center Study on Deep Learning-Assisted Detection and Classification of Fetal Central Nervous System Anomalies Using Ultrasound Imaging. (2025) http://arxiv.org/abs/2501.02000 (accessed January 25, 2025).
53. Lin X, Yang F, Zhou L, Yin P, Kong H, Xing W, et al. A support vector machine-recursive feature elimination feature selection method based on artificial contrast variables and mutual information. J Chromatogr B Anal Technol Biomed Life Sci. (2012) 910:149–55. doi: 10.1016/J.JCHROMB.2012.05.020
54. Han Y, Huang L, Zhou F. A dynamic recursive feature elimination framework (dRFE) to further refine a set of OMIC biomarkers. Bioinformatics. (2021) 37:2183–9. doi: 10.1093/BIOINFORMATICS/BTAB055
55. Lin Q, Tam PKH, Tang CSM. Artificial intelligence-based approaches for the detection and prioritization of genomic mutations in congenital surgical diseases. Front Pediatr. (2023) 11:1203289. doi: 10.3389/FPED.2023.1203289/BIBTEX
56. Strauch Y, Lord J, Niranjan M, Baralle D. CI-SpliceAI—improving machine learning predictions of disease causing splicing variants using curated alternative splice sites. PLoS One. (2022) 17:e0269159. doi: 10.1371/JOURNAL.PONE.0269159
57. Greenberg JA, Bell SJ, Guan Y, Yu Y. Folic acid supplementation and pregnancy: more than just neural tube defect prevention. Rev Obstet Gynecol. (2011) 4:52. doi: 10.18370/2309-4117.2017.34.57-63
58. Sigala RE, Lagou V, Shmeliov A, Atito S, Kouchaki S, Awais M, et al. Machine learning to advance human genome-wide association studies. Genes. (2023) 15:34. doi: 10.3390/GENES15010034
59. Cano-Gamez E, Trynka G. From GWAS to function: using functional genomics to identify the mechanisms underlying complex diseases. Front Genet. (2020) 11:505357. doi: 10.3389/FGENE.2020.00424/BIBTEX
60. Yotsu RR, Ding Z, Hamm J, Blanton RE. Deep learning for AI-based diagnosis of skin-related neglected tropical diseases: a pilot study. PLoS Negl Trop Dis. (2023) 17:e0011230. doi: 10.1371/JOURNAL.PNTD.0011230
61. Avagliano L, Massa V, George TM, Qureshy S, Pietro BG, Finnell RH. Overview on neural tube defects: from development to physical characteristics. Birth Defects Res. (2018) 111:1455. doi: 10.1002/BDR2.1380
62. Chen Z, Liu Z, Du M, Wang Z. Artificial intelligence in obstetric ultrasound: an update and future applications. Front Med. (2021) 8:733468. doi: 10.3389/FMED.2021.733468/BIBTEX
63. Enache IA, Iovoaica-Rămescu C, Ciobanu Ștefan G, Berbecaru EIA, Vochin A, Băluță ID, et al. Artificial intelligence in obstetric anomaly scan: heart and brain. Life. (2024) 14:166. doi: 10.3390/LIFE14020166
64. Xie HN, Wang N, He M, Zhang LH, Cai HM, Xian JB, et al. Using deep-learning algorithms to classify fetal brain ultrasound images as normal or abnormal. Ultrasound Obstet Gynecol. (2020) 56:579–87. doi: 10.1002/UOG.21967
65. Lin M, He X, Guo H, He M, Zhang L, Xian J, et al. Use of real-time artificial intelligence in detection of abnormal image patterns in standard sonographic reference planes in screening for fetal intracranial malformations. Ultrasound Obstet Gynecol. (2022) 59:304–16. doi: 10.1002/UOG.24843
66. Chowdhury AA, Hasan Mahmud SM, Shahjalal Hoque KK, Ahmed K, Bui FM, Lio P, et al. StackFBAs: detection of fetal brain abnormalities using CNN with stacking strategy from MRI images. J King Saud Univ Comput Inf Sci. (2023) 35:101647. doi: 10.1016/J.JKSUCI.2023.101647
67. Chen L, Tian Y, Deng Y. Neural network algorithm-based three-dimensional ultrasound evaluation in the diagnosis of fetal spina Bifida. Sci Program. (2021) 4:1–9. doi: 10.1155/2021/3605739
68. Shetty PH, Patil MS, Yadav S, Reddy IV. The role of AI and machine learning in revolutionizing prenatal screening and genetic analysis. Int J Innov Sci Res Technol. (2024) 9:2065–7. doi: 10.38124/IJISRT/IJISRT24OCT1344
69. Sharma A, Kala S, Kumar A, Sharma S, Gupta G, Jaiswal V. Deep learning in genomics, personalized medicine, and neurodevelopmental disorders. Intell Data Anal Bioinforma Biomed Syst. (2024):235–64. doi: 10.1002/9781394270910.CH10
70. Mieth B, Rozier A, Rodriguez JA, Höhne MMC, Görnitz N, Müller KR. DeepCOMBI: explainable artificial intelligence for the analysis and discovery in genome-wide association studies. NAR Genomics Bioinforma. (2021) 3:lqab065. doi: 10.1093/NARGAB/LQAB065
71. Lim S, Schmälzle R. Artificial intelligence for health message generation: theory, method, and an empirical study using prompt engineering. Front Commun. (2022) 8:1129082. doi: 10.3389/fcomm.2023.1129082
Keywords: artificial intelligence, prenatal diagnostics, machine learning, neural tube defects, ultrasound imaging
Citation: Yeganegi M, Danaei M, Azizi S, Jayervand F, Bahrami R, Dastgheib SA, Rashnavadi H, Masoudi A, Shiri A, Aghili K, Noorishadkam M and Neamatzadeh H (2025) Research advancements in the Use of artificial intelligence for prenatal diagnosis of neural tube defects. Front. Pediatr. 13:1514447. doi: 10.3389/fped.2025.1514447
Received: 5 November 2024; Accepted: 3 February 2025;
Published: 17 April 2025.
Edited by:
Sandeep Kumar Singh, Banaras Hindu University, IndiaReviewed by:
Sunil Kumar Rai, University of Medicine and Health Sciences, Saint Kitts and NevisSonali Vishal, Yale University, United States
Copyright: © 2025 Yeganegi, Danaei, Azizi, Jayervand, Bahrami, Dastgheib, Rashnavadi, Masoudi, Shiri, Aghili, Noorishadkam and Neamatzadeh. This is an open-access article distributed under the terms of the Creative Commons Attribution License (CC BY). The use, distribution or reproduction in other forums is permitted, provided the original author(s) and the copyright owner(s) are credited and that the original publication in this journal is cited, in accordance with accepted academic practice. No use, distribution or reproduction is permitted which does not comply with these terms.
*Correspondence: Mahsa Danaei, ZHIubWFoc2FkYW5haWVAZ21haWwuY29t