- 1Preventive Medicine, University of Southern California, Los Angeles, CA, United States
- 2Department of Kinesiology, University of North Carolina, Greensboro, NC, United States
- 3University of Texas MD Anderson Cancer Center, Houston, TX, United States
Background: Research on the clustering of physical activity, sedentary, and dietary intake behaviors in children has relied on retrospective and parent-report measures, which may obscure true associations. The current study combined objectively-measured moderate-to-vigorous intensity physical activity (MVPA) and sedentary time (ST) data from waist-worn accelerometers, with multiple child-report 24-h dietary recalls to assess specific components of dietary intake (i.e., dietary fat, carbohydrates, protein; glycemic load, fruits and vegetables) in children.
Methods: Participants (n = 136, ages 8–12 years) wore an accelerometer for 7 days. On two of those days, children completed 24-h recall phone interviews to assess dietary intake.
Results: After adjusting for child age, sex, ethnicity, annual household income, and body mass index (BMI) percentile; ST was positively associated with percent dietary fat intake, and negatively associated with percent dietary carbohydrate intake and glycemic load (p's < 0.01). MVPA was positively associated with percent dietary carbohydrate intake and daily glycemic load, and negatively associated with percent dietary fat intake (p's < 0.05).
Conclusion: Despite its direct health benefits, physical activity may be associated with consuming greater proportion of total intake from carbohydrates, especially those with a higher glycemic index. Further research is needed to understand the differential implications of these unique behavioral interrelations for diabetes, cardiovascular, and obesity risk.
Introduction
Low levels of physical activity, high levels of sedentary behavior, and poor dietary intake (e.g., high dietary fat, high glycemic load) in childhood are modifiable health behaviors that have been implicated in elevated lifelong risk of obesity, diabetes, and cardiovascular disease (1–7). Understanding the interrelationships among physical activity, sedentary behavior, and dietary intake in children is relevant to the design and development of health promotion programs. Interventions that are able to successfully bring about change in more than one health behavior (i.e., multiple behavior change) may be more cost-effective than those that only impact single behaviors (8). However, physical activity and sedentary behaviors may be associated with specific aspects of dietary intake in multifaceted ways that are not well understood, particularly in youth (9).
Studies examining the relationships of physical activity and sedentary behavior with diet in children and adolescents suffer from limitations that circumscribe their ability to inform interventions. First, studies on this topic tend to use retrospective and parent-report measures of usual physical activity behavior (9), which are subject to recall and reporting biases, particularly in children (10). A second limitation of these studies is the failure to distinguish between aspects of dietary intake (e.g., fat, carbohydrates, protein, glycemic load, fruits, and vegetables) that may be differentially associated with specific energy expenditure behaviors (9).
The current study aimed to address these limitations by combining objectively-measured moderate-to-vigorous intensity physical activity (MVPA) and sedentary time (ST) using a waist-worn accelerometer with multiple child-report 24-h dietary recalls to assess specific components of dietary intake in children. The primary objective was to determine whether MVPA and ST are differentially related to specific components of dietary intake (i.e., fat, carbohydrates, protein, daily glycemic load, fruits and vegetables) in children.
Materials and Methods
Overview
The current cross-sectional analyses used baseline data from the Mothers' and Their Children's Health (MATCH) study (11), which is a longitudinal investigation of parenting factors and obesity in a sample of mothers and children. This study was approved by the Institutional Review Board at the University of Southern California. Parents provided written consent/permission for children to participate, and children provided written assent.
Participants
Participants included a convenience sample of ethnically-diverse 8–12 year-old children recruited from demographically-representative public elementary schools and after-school programs in the greater Los Angeles metropolitan area. Children were recruited through informational flyers and in-person recruitment events. Inclusion criteria were: (1) child is in the 3rd−6th grade, (2) ≥50% of child's custody resides with the mother, and (3) child is able to read English. Exclusion criteria were: (1) currently taking medications for thyroid function or psychological conditions such as depression, anxiety, mood disorders, and ADHD, (2) health issues that limit physical activity, (3) enrolled in special education programs, (4) currently using oral or inhalant corticosteroids for asthma, (5) mother is pregnant, (6) child classified as underweight by a BMI percentile <5% adjusted for sex and age, and (7) mother works more than two weekday evenings (between the hours of 5–9 p.m.) per week or more than 8 h on any weekend day.
Procedures
During the 7-day data collection period, children wore an accelerometer during waking hours and completed two parent-assisted 24-h dietary recalls by phone with trained interviewers. This study was carried out in accordance with the recommendations of the Human Subjects Institutional Review Board at the University of Southern California. The protocol was approved by the Health Sciences Review Board. All subjects gave written informed consent/assent in accordance with the Declaration of Helsinki. All study protocols, descriptions of analytic methods, materials, and data are available upon request to the authors.
Measures
Accelerometer
The Actigraph, Inc. GT3X model accelerometer was used for measurement of MVPA and ST. The device was worn on the right hip, attached to an adjustable belt, at all times except sleeping, bathing, or swimming. The device was set to collect movement data in activity count units for each 30-s epoch. Meterplus software (Santech, San Diego, CA) was used to identify periods of non-wear (>60 continuous minutes of zero activity counts) and valid days (at least 10 h of wear). Cut-points for time spent in MVPA and ST were consistent with studies of national surveillance data (12, 13) using age-specific thresholds for children generated from the Freedson prediction equation equivalent to 4 METs (13–18). ST was defined as activity < 100 counts per minute (19, 20).
Dietary Assessment
Usual dietary intake was assessed using two 24-h dietary recalls. Each recall was conducted over the phone by trained research staff using a five-pass interview approach (21, 22). The 24-h dietary recalls were collected from children with assistance from mothers. Children were asked to recall what they ate during the course of the prior day (midnight to midnight). It was preferred that the recalls consisted of one weekday and one weekend day. However, if a participant missed one or both scheduled calls, make-up calls were made within the remaining days of the data collection period that may have led to the inclusion of two weekdays or two weekend days in the recall. Dietary intake data were analyzed using Nutrition Data System for Research software versions 2013 and 2015. Usual daily dietary intake was calculated by averaging dietary intake across recall days. Dietary intake amounts for fat, carbohydrate, and protein were reported as a percent of total energy intake (e.g., percent of calories from dietary fat) to account for plausible differences in energy requirements across the study sample (23, 24). Dietary intake for fruit and vegetables was calculated by dividing the reported servings by total kcal of energy consumed. This amount was then multiplied by 1,000 kcals to determine adjusted intake per 1,000 kilocalories consumed. Dietary outcome variables were as follows: percent energy from dietary fats, carbohydrates, and protein; glycemic load; and fruits and vegetables servings per 1,000 kcal. A food's glycemic load is determined by multiplying its glycemic index (i.e., relative ranking of a carbohydrate according to how it quickly it is digested, absorbed in the blood, and metabolized) by the amount of carbohydrate (g) contained in that food and dividing by 100.
Anthropometric Assessments
Height (m) and weight (kg) were measured in duplicate using an electronically calibrated digital scale (Tanita WB-110A) and professional stadiometer (PE-AIM-101). Body mass index (BMI; kg/m2) and CDC age and sex-specific BMI percentiles were determined using EpiInfo 2005, Version 3.2 (CDC, Atlanta, GA).
Demographic Factors
Mothers completed paper questionnaires reporting on their child's ethnicity and annual household income. Children self-reported their age and sex.
Data Analyses
Fruit and vegetable intake was square root transformed to meet normal distribution assumptions in models. Bivariate analyses consisting of Pearson's correlations and t-tests examined the associations of covariates (i.e., child age, sex, ethnicity [Hispanic vs. non-Hispanic], annual household income [divided into quartiles: <$35,000; $35,001–$75,000; $75,001–$105,000; ≥$105,001) with the dietary outcome variables. Multiple linear regression analyses examined the associations of MVPA and ST with dietary outcomes controlling for child age, sex (male vs. female), ethnicity (Hispanic vs. non-Hispanic), annual household income (quartiles), and BMI percentile. Separate models were run to test the associations of MVPA and ST with each dietary outcome.
Results
Data Availability
Of the 464 mother-child dyads interested in the study, 132 dyads could not be reached for eligibility screening, 22 dyads declined to be screened, 62 dyads did not meet eligibility criteria, and 46 dyads either did not attend their enrollment session or were no longer interested. A total of 202 children (and their mothers) enrolled in the study. Of this number, 14 children did not have at least two valid days of accelerometer data, an additional 46 children did not have at least 2 days of 24-h diet recall data, and an additional 6 children were missing height and weight data—leaving an analytic sample of 136 children. The analytic and excluded sample did not differ in terms of child age, sex, ethnicity, annual household income, or BMI percentile. Of the analytic sample, 10 children (7.4%) had 24-h dietary recall data for two weekend days, and 23 children (16.9%) had 24-h dietary recall data on two weekdays. Furthermore, 10 children (7.4%) had < 4 valid days (>10 h) of accelerometer data.
Descriptive Statistics and Bivariate Correlations
Table 1 shows demographic characteristics and descriptive statistics for the study variables. MVPA was moderately negatively associated with ST (r = −0.61, p < 0.001). Child BMI percentile was positively associated with percent energy intake from dietary fat (r = 0.22, p = 0.01), and negatively associated with percent energy intake from carbohydrates (r = −0.21, p = 0.02) and daily glycemic load (r = −0.27, p = 0.01). Hispanic children had a greater percent energy intake from protein (M = 16.36, SD = 4.53) than non-Hispanic children (M = 14.16, SD = 3.19) (t = 3.23, p = 0.002). Hispanic children also reported consuming a smaller percent energy intake from carbohydrates (M = 50.83, SD = 8.07) than non-Hispanic children (M = 53.62, SD = 7.24; t = −2.12, p = 0.04). Further, Hispanic children consumed a lower glycemic load (M = 118.67, SD = 42.65) than non-Hispanic children (M = 144.89, SD = 41.20; t = −3.64, p < 0.001). Child age, sex, and annual household income were not related to any of the dietary outcomes.
Covariate-Adjusted Associations of MVPA and ST With Dietary Intake Variables
Results of the multiple linear regression analyses of MVPA and ST predicting dietary intake variables controlling for child age, sex, ethnicity (Hispanic vs. not Hispanic), annual household income (quartiles), and BMI percentile are shown in Tables 2, 3, respectively. MVPA was positively associated with percent energy intake from carbohydrates and glycemic load, and negatively associated with percent energy intake from fat. ST was positively associated with percent energy intake from fat, and negatively associated with percent energy intake from carbohydrates and glycemic load.
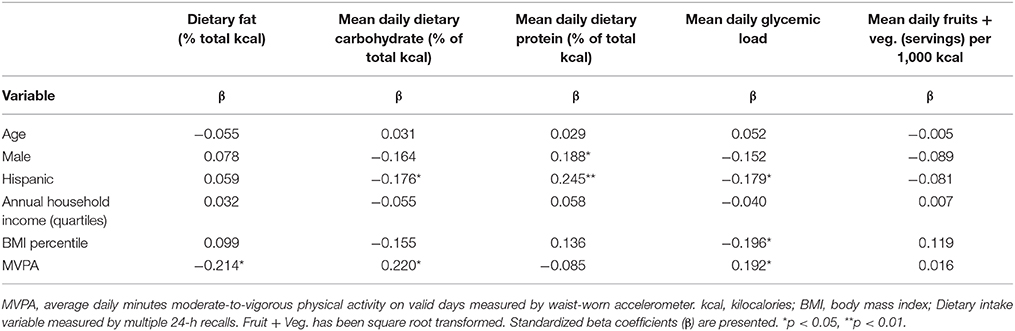
Table 2. Summary of multiple linear regression analysis for moderate-to-vigorous physical activity (MVPA) predicting dietary intake variables in children (8–12 years) (n = 136).
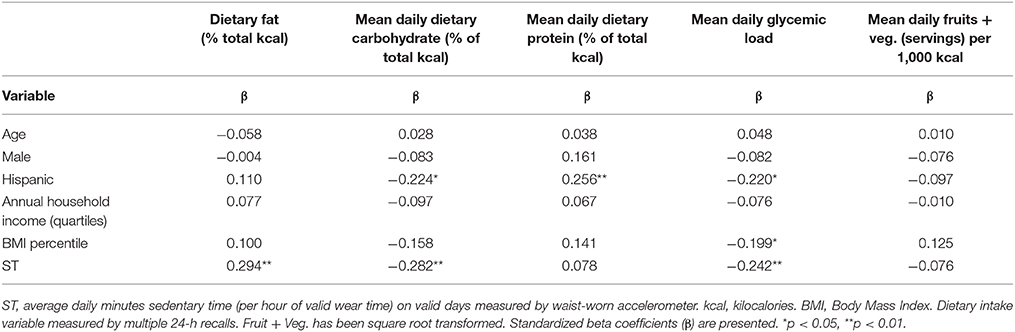
Table 3. Summary of multiple linear regression analysis for sedentary time (ST) predicting dietary intake variables in children (8–12 years) (n = 136).
Discussion
This study makes an important contribution to the literature as one of the first studies to combine an objective monitor-based activity measure with multiple 24-h diet recalls to examine the associations of MVPA and ST with specific components of dietary intake in children. Results indicating that greater ST is associated with a greater proportion of energy intake from dietary fat are consistent with a number of previous studies (25–29). The largest contributors to fat intake in the current study were lunchmeats, chicken nuggets, fast food meals, oils, butter, chips, and ice cream. Children may consume these types of high fat foods and snacks while watching TV or engaging in other screen behaviors (30, 31) as these activities may distract normal satiation cues (32–34) or trigger hunger cues through food advertising (35, 36). The observed association between higher MVPA and a lower proportion of energy intake from dietary fat has appeared less frequently in the literature (37). However, the majority of prior studies in this area use either parent- or self- report measures of children's sedentary or screen time and physical activity, which may be prone to recall or reporting biases that obscure relationships (10). Previous studies also typically measure dietary intake through food frequency measures, which suffer from other methodological weakness such as underestimation and lower reliability compared to the 24-h dietary recall (38).
A novel finding of this study was that greater MVPA and less ST were associated with a greater proportion of total dietary energy intake from carbohydrates particularly foods with higher glycemic load (i.e., simple sugars, white bread, white rice). Children may consume a greater proportion of energy intake from carbohydrates such as granola bars, sweetened cereals, pastry, candy, pretzels, bread, and sports drinks before, during, or after organized sports and at activity-based classes/lessons (e.g., karate, dance). This may be because these foods dominate the concessions sold at these events, are often given out as postgame treats, and serve a substitute for regular meals that are disrupted due to sports games and practices (39–41). In fact, a growing body of evidence suggests that youth sports may expose children to an unhealthy food environment (42, 43). Another potential explanation is that increased physical activity is a behavioral response to carbohydrate intake (44, 45). Consuming foods with a high glycemic index may reduce feelings of fatigue or replenish skeletal muscle energy, which can induce physical activity behaviors (46). Also, the body may increase musculoskeletal activity in a compensatory manner to reduce elevated blood glucose levels in response to high glycemic foods (47–49).
This study had some limitations. Due to the cross-sectional design, inferences about directionality of causal effects cannot be made. Additionally, many of the observed effects sizes are small. Although using 24-h dietary recall methodology in children offers improvements over parent proxy-report and food frequency instruments, there are more objective measures of dietary such as the use of urine metabolites doubly labeled water, and food photography that have improved reliability and validity. Also, approximately a quarter of potential participants did not have complete 24-h dietary recall data, however, these rates of non-response and non-compliance they are comparable to other studies using this methodology in children (50, 51). Furthermore, although the accelerometer provided objective measures of MVPA and ST, amounts may be underestimated due to accelerometer non-wear. For improved accelerometer wear time compliance, a wrist-worn model may be used. Additionally, including children with < 4 valid days of accelerometer wear may reduce the reliability of these data and the ability to detect significant associations. Also, results may not be generalizable to children younger than age 8 or adolescents.
Conclusions
Overall, this project addressed important methodological limitations of prior work in being one of the first studies to use an objective activity monitor combined with multiple child-report 24-h dietary recalls in children. Despite its direct health benefits, physical activity may be associated with consuming a greater proportion of total energy intake from carbohydrates, especially those with a higher glycemic index such as simple sugars, white bread, white rice. These results underscore that diet, physical activity, and sedentary behaviors might not cluster together in a manner that optimizes health outcomes. Further research is needed to understand the differential implications of these unique behavioral interrelations for diabetes, cardiovascular, and obesity risk.
Author Contributions
GD and SS formulated the research questions. GD designed the study. SO processed and cleaned the data. GD analyzed the data and wrote the article. SO, BB, JM, and SS reviewed and edited the article.
Conflict of Interest Statement
GD and JM have received consulting payments from the Dairy Council of California. GD has received travel funding from the National Physical Activity Plan Alliance. GD has received consulting payments from the National Collaborative on Childhood Obesity Research. These organizations had no role in the design, conduct of the study, collection, analysis/interpretation of the data, or preparation, review, approval of the manuscript.
The remaining authors declare that the research was conducted in the absence of any commercial or financial relationships that could be construed as a potential conflict of interest.
Funding
This work was supported by the National Institutes of Health/National Heart Lung and Blood Institute (grant number R01HL119255; grant number F31HL137346); the National Institutes of Health/National Cancer Institute (grant number T32CA009492-31); and the University of Southern California Provost Fellowship. The National Institute of Health and the University of Southern California had no role in the design, analysis or writing of this article.
References
1. Strong WB, Malina RM, Blimkie CJ, Daniels SR, Dishman RK, Gutin B, et al. Evidence based physical activity for school-age youth. J Pediatr. (2005) 146:732–37. doi: 10.1016/j.jpeds.2005.01.055
2. Janssen I, LeBlanc AG. Systematic review of the health benefits of physical activity and fitness in school-aged children and youth. Int J Behav Nutr Phys Act. (2010) 7:40. doi: 10.1186/1479-5868-7-40
3. Tremblay MS, LeBlanc AG, Kho ME, Saunders TJ, Larouche R, Colley RC, et al. Systematic review of sedentary behaviour and health indicators in school-aged children and youth. Int J Behav Nutr Phys Act. (2011) 8:98. doi: 10.1186/1479-5868-8-98
4. Lim SS, Vos T, Flaxman AD, Danaei G, Shibuya K, Adair-Rohani H, et al. A comparative risk assessment of burden of disease and injury attributable to 67 risk factors and risk factor clusters in 21 regions, 1990–2010: a systematic analysis for the Global Burden of Disease Study 2010. Lancet. (2013) 380:2224–60. doi: 10.1016/S0140-6736(12)61766-8
5. Ledoux T, Hingle MD, Baranowski T. Relationship of fruit and vegetable intake with adiposity: a systematic review. Obes Rev. (2011) 12:e143–50. doi: 10.1111/j.1467-789X.2010.00786.x
6. Ailhaud G, Guesnet P. Fatty acid composition of fats is an early determinant of childhood obesity: a short review and an opinion. Obes Rev. (2004) 5:21–6. doi: 10.1111/j.1467-789X.2004.00121.x
7. Greenwood D, Threapleton D, Evans C, Cleghorn CL, Nykjaer C, Woodhead C, et al. Association between sugar-sweetened and artificially sweetened soft drinks and type 2 diabetes: systematic review and dose–response meta-analysis of prospective studies. Br J Nutr. (2014) 112:725–34. doi: 10.1017/S0007114514001329
8. Evers KE, Quintiliani LM. Advances in multiple health behavior change research. Transl Behav Med. (2013) 3:59–61. doi: 10.1007/s13142-013-0198-z
9. Leech RM, McNaughton SA, Timperio A. The clustering of diet, physical activity and sedentary behavior in children and adolescents: a review. Int J Behav Nutr Phys Act. (2014) 11:4. doi: 10.1186/1479-5868-11-4
10. Sallis JF, Saelens BE. Assessment of physical activity by self-report: status, limitations, and future directions. Res Q Exerc Sport. (2000) 71:1–14. doi: 10.1080/02701367.2000.11082780
11. Dunton GF, Liao Y, Dzubur E, Leventhal AM, Huh J, Gruenewald T, et al. Investigating within-day and longitudinal effects of maternal stress on children's physical activity, dietary intake, and body composition: Protocol for the MATCH study. Contemp Clin Trials. (2015) 43:142–54. doi: 10.1016/j.cct.2015.05.007
12. Troiano RP, Berrigan D, Dodd KW, Mâsse LC, Tilert T, McDowell M. Physical activity in the United States measured by accelerometer. Med Sci Sports Exerc. (2008) 40:181–8. doi: 10.1249/mss.0b013e31815a51b3
13. Belcher BR, Berrigan D, Dodd KW, Emken BA, Chou CP, Spruijt-Metz D. Physical activity in US youth: effect of race/ethnicity, age, gender, and weight status. Med Sci Sports Exerc. (2010) 42:2211–21. doi: 10.1249/MSS.0b013e3181e1fba9
14. Laska MN, Murray DM, Lytle LA, Harnack LJ. Longitudinal associations between key dietary behaviors and weight gain over time: transitions through the adolescent years. Obesity (2012) 20:118–25. doi: 10.1038/oby.2011.179
15. Freedson P, Pober D, Janz KF. Calibration of accelerometer output for children. Med Sci Sports Exerc. (2005) 37:S523–30. doi: 10.1249/01.mss.0000185658.28284.ba
16. Harrell JS, McMurray RG, Baggett CD, Pennell ML, Pearce PF, Bangdiwala SI. Energy costs of physical activities in children and adolescents. Med Sci Sports Exerc. (2005) 37:329–36. doi: 10.1249/01.MSS.0000153115.33762.3F
17. Roemmich JN, Clark PA, Walter K, Patrie J, Weltman A, Rogol AD. Pubertal alterations in growth and body composition. V. Energy expenditure, adiposity, and fat distribution. Am J Physiol Endocrinol Metab. (2000) 279:E1426–36. doi: 10.1152/ajpendo.2000.279.6.E1426
18. Freedson PS, Melanson E, Sirard J. Calibration of the Computer Science and Applications, Inc. accelerometer. Med Sci Sports Exerc. (1998) 30:777–81. doi: 10.1097/00005768-199805000-00021
19. Treuth MS, Schmitz K, Catellier DJ, McMurray RG, Murray DM, Almeida MJ, et al. Defining accelerometer thresholds for activity intensities in adolescent girls. Med Sci Sports Exerc. (2004) 36:1259–66.
20. Healy GN, Dunstan DW, Salmon J, Cerin E, Shaw JE, Zimmet PZ, et al. Breaks in sedentary time: beneficial associations with metabolic risk. Diabetes Care. (2008) 31:661–6. doi: 10.2337/dc07-2046
21. McPherson RS, Hoelscher DM, Alexander M, Scanlon KS, Serdula MK. Dietary assessment methods among school-aged children: validity and reliability. Prev Med. (2000) 31:S11–33. doi: 10.1006/pmed.2000.0631
22. Johnson RK, Driscoll P, Goran MI. Comparison of multiple-pass 24-hour recall estimates of energy intake with total energy expenditure determined by the doubly labeled water method in young children. J Am Diet Assoc. (1996) 96:1140–4. doi: 10.1016/S0002-8223(96)00293-3
23. Willett WC, Howe GR, Kushi LH. Adjustment for total energy intake in epidemiologic studies. Am J Clin Nutr. (1997) 65:1220S−8S.
24. National Cancer Institute. Dietary Assessment Primer: Learn More About Energy Adjustment. Available online at: https://dietassessmentprimer.cancer.gov/learn/adjustment.html (Accessed May 7, 2018) (2018).
25. Barr-Anderson DJ, Larson NI, Nelson MC, Neumark-Sztainer D, Story M. Does television viewing predict dietary intake five years later in high school students and young adults? Int J Behav Nutr Phys Act. (2009) 6:7. doi: 10.1186/1479-5868-6-7
26. Utter J, Neumark-Sztainer D, Jeffery R, Story M. Couch potatoes or French fries: are sedentary behaviors associated with body mass index, physical activity, and dietary behaviors among adolescents? J Am Diet Assoc. (2003) 103:1298–305. doi: 10.1016/S0002-8223(03)01079-4
27. Lake A, Townshend T, Alvanides S, Stamp E, Adamson A. Diet, physical activity, sedentary behaviour and perceptions of the environment in young adults. J Hum Nutr Diet. (2009) 22:444–54. doi: 10.1111/j.1365-277X.2009.00982.x
28. Zabinski MF, Norman GJ, Sallis JF, Calfas KJ, Patrick K. Patterns of sedentary behavior among adolescents. Health Psychol. (2007) 26:113. doi: 10.1037/0278-6133.26.1.113
29. Haerens L, Craeynest M, Deforche B, Maes L, Cardon G, De Bourdeaudhuij I. The contribution of psychosocial and home environmental factors in explaining eating behaviours in adolescents. Eur J Clin Nutr. (2008) 62:51–9. doi: 10.1038/sj.ejcn.1602681
30. Matheson DM, Killen JD, Wang Y, Varady A, Robinson TN. Children's food consumption during television viewing. Am J Clin Nutr. (2004) 79:1088–94. doi: 10.1093/ajcn/79.6.1088
31. Feldman S, Eisenberg ME, Neumark-Sztainer D, Story M. Associations between watching TV during family meals and dietary intake among adolescents. J Nutr Educ Behav. (2007) 39:257–63. doi: 10.1016/j.jneb.2007.04.181
32. Mittal D, Stevenson RJ, Oaten MJ, Miller LA. Snacking while watching TV impairs food recall and promotes food intake on a later TV free test meal. Appl Cogn Psychol. (2011) 25:871–7. doi: 10.1002/acp.1760
33. Blass EM, Anderson DR, Kirkorian HL, Pempek TA, Price I, Koleini MF. On the road to obesity: television viewing increases intake of high-density foods. Physiol Behav. (2006) 88:597–604. doi: 10.1016/j.physbeh.2006.05.035
34. Bellissimo N, Pencharz PB, Thomas SG, Anderson GH. Effect of television viewing at mealtime on food intake after a glucose preload in boys. Pediatr Res. (2007) 61:745–9. doi: 10.1203/pdr.0b013e3180536591
35. Halford JC, Gillespie J, Brown V, Pontin EE, Dovey TM. Effect of television advertisements for foods on food consumption in children. Appetite (2004) 42:221–5. doi: 10.1016/j.appet.2003.11.006
36. Chamberlain LJ, Wang Y, Robinson TN. Does children's screen time predict requests for advertised products? Cross-sectional and prospective analyses. Arch Pediatr Adolesc Med. (2006) 160:363–8. doi: 10.1001/archpedi.160.4.363
37. Sabbe D, De Bourdeaudhuij I, Legiest E, Maes L. A cluster-analytical approach towards physical activity and eating habits among 10-year-old children. Health Educ Res. (2007) 23:753–62. doi: 10.1093/her/cyl135
38. Schaefer EJ, Augustin JL, Schaefer MM, Rasmussen H, Ordovas JM, Dallal GE, et al. Lack of efficacy of a food-frequency questionnaire in assessing dietary macronutrient intakes in subjects consuming diets of known composition. Am J Clin Nutr. (2000) 71:746–51. doi: 10.1093/ajcn/71.3.746
39. Irby MB, Drury-Brown M, Skelton JA. The food environment of youth baseball. Child Obes. (2014) 10:260–5. doi: 10.1089/chi.2013.0161
40. Thomas M, Nelson TF, Harwood E, Neumark-Sztainer D. Exploring parent perceptions of the food environment in youth sport. J Nutr Educ Behav. (2012) 44:365–71. doi: 10.1016/j.jneb.2011.11.005
41. Fulkerson JA, Story M, Neumark-Sztainer D, Rydell S. Family meals: perceptions of benefits and challenges among parents of 8-to 10-year-old children. J Am Diet Assoc. (2008) 108:706–9. doi: 10.1016/j.jada.2008.01.005
42. Nelson TF, Stovitz SD, Thomas M, LaVoi NM, Bauer KW, Neumark-Sztainer D. Do youth sports prevent pediatric obesity? A systematic review and commentary. Curr Sports Med Rep. (2011) 10:360. doi: 10.1249/JSR.0b013e318237bf74
43. Dortch KS, Gay J, Springer A, Kohl HW III, Sharma S, Saxton D, et al. The association between sport participation and dietary behaviors among fourth graders in the school physical activity and nutrition survey, 2009–2010. Am J Health Promot. (2014) 29:99–106. doi: 10.4278/ajhp.130125-QUAN-47
44. Spruijt-Metz D, Belcher B, Anderson D, Lane CJ, Chou CP, Salter-Venzon D, et al. A high-sugar/low-fiber meal compared with a low-sugar/high-fiber meal leads to higher leptin and physical activity levels in overweight Latina females. J Am Diet Assoc. (2009) 109:1058–63. doi: 10.1016/j.jada.2009.03.013
45. O'Reilly GA, Belcher BR, Davis JN, Martinez LT, Huh J, Antunez-Castillo L, et al. Effects of high-sugar and high-fiber meals on physical activity behaviors in Latino and African American adolescents. Obesity (Silver Spring) (2015) 23:1886–94. doi: 10.1002/oby.21169
46. Gibson EL. Carbohydrates and mental function: feeding or impeding the brain? Nutr Bull. (2007) 32:71–83. doi: 10.1111/j.1467-3010.2007.00606.x
47. McLaughlin T, Allison G, Abbasi F, Lamendola C, Reaven G. Prevalence of insulin resistance and associated cardiovascular disease risk factors among normal weight, overweight, and obese individuals. Metabolism (2004) 53:495–9. doi: 10.1016/j.metabol.2003.10.032
48. Baron AD, Steinberg H, Brechtel G, Johnson A. Skeletal muscle blood flow independently modulates insulin-mediated glucose uptake. Am J Physiol Endocrinol Metab. (1994) 266:E248–53. doi: 10.1152/ajpendo.1994.266.2.E248
49. Vincent MA, Clerk LH, Lindner JR, Klibanov AL, Clark MG, Rattigan S, et al. Microvascular recruitment is an early insulin effect that regulates skeletal muscle glucose uptake in vivo. Diabetes (2004) 53:1418–23. doi: 10.2337/diabetes.53.6.1418
50. Lipsky LM, Nansel TR, Haynie DL, Liu D, Li K, Pratt CA, et al. Diet quality of US adolescents during the transition to adulthood: changes and predictors, 2. Am J Clin Nutr. (2017) 105:1424–32. doi: 10.3945/ajcn.116.150029
Keywords: physical activity, sedentary behavior, dietary intake, accelerometer, 24 h dietary recall, children
Citation: Dunton GF, O'Connor SG, Belcher BR, Maher JP and Schembre SM (2018) Objectively-Measured Physical Activity and Sedentary Time are Differentially Related to Dietary Fat and Carbohydrate Intake in Children. Front. Public Health 6:198. doi: 10.3389/fpubh.2018.00198
Received: 31 March 2018; Accepted: 27 June 2018;
Published: 20 July 2018.
Edited by:
Yoram Chaiter, Israel Defense Forces Medical Corps, IsraelReviewed by:
Wasantha Jayawardene, Indiana University Bloomington, United StatesSusan Elizabeth Esposito, Life University, United States
Copyright © 2018 Dunton, O'Connor, Belcher, Maher and Schembre. This is an open-access article distributed under the terms of the Creative Commons Attribution License (CC BY). The use, distribution or reproduction in other forums is permitted, provided the original author(s) and the copyright owner(s) are credited and that the original publication in this journal is cited, in accordance with accepted academic practice. No use, distribution or reproduction is permitted which does not comply with these terms.
*Correspondence: Genevieve F. Dunton, ZHVudG9uQHVzYy5lZHU=