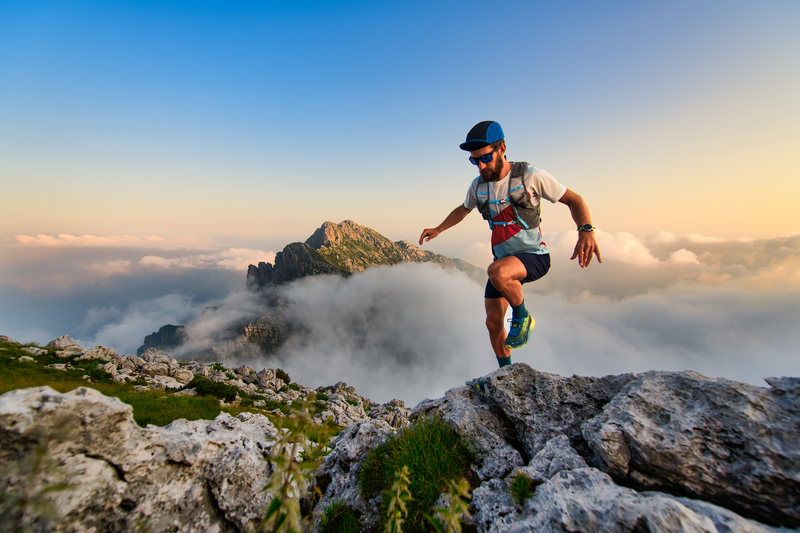
95% of researchers rate our articles as excellent or good
Learn more about the work of our research integrity team to safeguard the quality of each article we publish.
Find out more
ORIGINAL RESEARCH article
Front. Public Health , 02 March 2023
Sec. Environmental Health and Exposome
Volume 11 - 2023 | https://doi.org/10.3389/fpubh.2023.1127566
Background: Chronic Obstructive Pulmonary Disease (COPD) is a common and harmful disease that requires an effective tool to early screen high-risk individuals. Gansu has unique environments and customs, leading to the different prevalence and etiology of COPD from other regions. The association between altitude and COPD once attracted epidemiologists' attention. However, the prevalence in Gansu and the role of altitude are still unclarified.
Methods: In Gansu, a multistage stratified cluster sampling procedure was utilized to select a representative sample aged 40 years or older. The questionnaire and spirometry examination were implemented to collect participants' information. The diagnosis and assessment of COPD were identified by the Global Initiative for Chronic Obstructive Lung Disease (GOLD) criterion, while post-bronchodilator FEV1/FVC < LLN was for sensitivity analysis. Furthermore, the effect of high altitude on COPD was evaluated by the logistic regression model after propensity score matching (PSM). Finally, the participants were randomly divided into training and validation sets. The training set was used to screen the relative factors and construct a nomogram which was further assessed by the receiver operating characteristic (ROC) curve, calibration curve, and decision curve analysis (DCA) in the two sets.
Results: There were 2,486 eligible participants in the final analysis, of which 1,584 lived in low altitudes and 902 lived in high altitudes. Based on the GOLD criterion, the crude and standardized prevalences in Gansu were 20.4% (18.7–22.0) and 19.7% (17.9–21.6). After PSM, the logistic regression model indicated that high altitude increased COPD risk [PSM OR: 1.516 (1.162–1.978)]. Altitude, age, sex, history of tuberculosis, coal as fuel, and smoking status were reserved for developing a nomogram that demonstrated excellent discrimination, calibration, and clinical benefit in the two sets.
Conclusions: COPD has become a serious public health problem in Gansu. High altitude is a risk factor for COPD. The nomogram has satisfactory efficiency in screening high-risk individuals.
Chronic Obstructive Pulmonary Disease (COPD) is the most common chronic respiratory disease and is the third cause of mortality leading to 3.2 million deaths worldwide (1, 2). A population-based survey conducted from 2002 to 2004 indicated that the prevalence of Chinese older than 40 was about 8.2% (3). The rate rose to 13.6% just a decade later, which was higher in men and the southwest region than in women and other regions (4). Airway limitation and respiratory symptoms would seriously disturb the patient's life and work due to the irreversible and progressive lung lesions (5). Besides, data demonstrated that the direct medical cost of COPD accounted for 33.33%−118.09% of the average annual income in China (6). COPD has increasingly become a serious public health problem in China, leading to tremendous economic, social, and healthcare burdens.
Gansu province, located in northwestern China, is characterized by various altitudes (900–3,800 m) and multi-ethnic habitation (7, 8). Compared to the flat region, the high-altitude region has heterogeneity in the prevalence and etiology of many diseases due to particular environments (cold temperatures, low humidity, hypobaric, hypoxic, etc.) and lifestyles (coal or biomass as fuel, etc.) (9–11). But, the prevalence of COPD in Gansu is still unclarified. In addition, COPD is mediated by environmental and genetic factors, in which smoking is the most recognized risk factor (2). However, quite a few non-smokers developing COPD indicate its etiological complexity (12). Although some researchers advocated that altitude was associated with COPD, it has yet to reach a consensus (13–15).
In this study, we conducted a cross-sectional study to explore the prevalence of COPD in Gansu and clarify the association between altitude and COPD. Also, a nomogram enrolling candidate risk factors was constructed to screen the high-risk population.
Based on Equations 1, 2, we took deff = 5, α = 95%, p = 10.2%, r = 20%, and response rate = 90% to calculate the sample size. The referenced prevalence was derived from previous research (4).
During 2018–2019, a multistage stratified cluster sampling procedure was utilized to collect a representative sample from Gansu (Figure 1). Specifically, four monitoring sites in Gansu were selected by a probability proportional to size method (Longnan City, Jiuquan City, Qingyang City, and Gannan City). Using the same method, three towns were selected from each city, and more than two villages were further selected from each town. Subsequently, we selected a village with more than 150 households by the cluster random sampling method and randomly selected 100 families with members older than 40. Finally, the KISH table was used to select an eligible member from each family for investigation.
All participants were Chinese residents older than 40. Those physically unable to complete the spirometry examination were excluded (active tuberculosis, pregnancy, cardiovascular and cerebrovascular accidents in the past month, heart rate >120 beats/min or blood pressure >180/120 mmHg, related surgeries in the past 3 months, etc.) (16). The flowchart of screening participants was exhibited in Figure 2. Our study was approved by the ethics review committee of Guangzhou Medical University and Xi'an Jiaotong University Health Science Center.
A professional questionnaire was implemented to collect information about demographic characteristics, respiratory symptoms, and exposure to COPD-related risk factors (17). Specifically, the smokers were defined as those who had continually smoked for more than 6 months. The former smokers were defined as those who had quit smoking for more than 1 month when they were interviewed. Biomass was defined as wood, grass, animal dung, and crop waste. Coal was defined as coal and lignite. Occupational exposure was defined as exposure to dust or chemicals in the workplace for more than 1 year (4, 17). According to some references, the physiological alteration of the respiratory and circulatory systems began at altitudes >1,500 m (18–21). Thus, we chose 1,500 m to divide low and high altitudes.
Lung function was examined by EasyOne Spirometer (NDD Medizintechnik AG, Switzerland) following the operation of the American Thoracic Society and the European Respiratory Society (16). In brief, eligible participants were asked to estimate basic lung function and rechecked 15 min after inhalation of 400 μg salbutamol. Each test was acceptable only when the quality was rated A, B, or C. The overall proportion of A should be more than 70%.
The questionnaire and the spirometry examination were performed by trained staff from local medical systems.
According to the Global Initiative for Chronic Obstructive Lung Disease (GOLD) 2019, participants were diagnosed with COPD if forced expiratory volume in one second (FEV1): forced vital capacity (FVC) was <70% after inhaling bronchodilators. The patients were categorized as the GOLD stage I (mild group: FEV1 ≥80% predicted), GOLD stage II (moderate group: FEV1 ≥50 to <80% predicted), GOLD stage III (severe group: FEV1 ≥30 to <50% predicted), and GOLD stage IV (very severe group: FEV1 <30% predicted). The modified Medical Research Council (mMRC) dyspnea scale was used to estimate the dyspnea of COPD patients (5).
In this study, another diagnostic criterion was available for sensitivity analysis: post-bronchodilator FEV1/FVC < the lower normal limit (LLN). The LLN, predicted FEV1 and FVC were calculated by specialized formulas that considered the characteristics of the Chinese population (22).
Continuous variables were described by mean [standard deviation (SD)] or median (lower quartile, upper quartile) and were compared by T-test or Mann–Whitney test. Categorical variables were described by rate [95% confidence interval (95% CI)] and were compared by Chi-square test or Fisher's exact test. The Cochran-Armitage test was used to compare the difference between two groups of ordinal data. The overall standardized prevalence of COPD was calculated based on the sample structure of the 2010 census of the Chinese population (23).
The 1:1 propensity score matching (PSM), with the caliper of 0.02 and in the nearest method, was implemented to balance the populations' characteristics between low and high altitudes. Subsequently, logistic regression models estimated odd ratios (Ors) and 95% CI to evaluate the association between high altitude and COPD risk (24).
Furthermore, the participants were randomly divided into the training and validation sets at the ratio of 6:4. In the training set, the univariable and stepwise logistic regression models were performed to screen the related factors to construct a nomogram for COPD (GOLD criterion). The nomogram was assessed by the receiver operating characteristic (ROC) curve, calibration curve, and decision curve analysis (DCA), which was reassessed in the validation set for internal validation.
Statistical analysis was performed by SPSS 26.0 and R 4.13. The result was considered statistically significant only when the two-sided P was < 0.05.
We interviewed 2,925 residents. Finally, 2,486 eligible participants were included in the analysis, of which 1,584 lived in low altitudes (mean: 1,190.56 m, range: 996–1,442 m) and 902 lived in high altitudes (mean: 2,684.92 m, range: 2,416–2,896 m). Compared to the low-altitude population, the high-altitude population showed significant differences in demographic characteristics except for body mass index (BMI), history of tuberculosis (TB), and coal as fuel (P < 0.05; Table 1).
There were 508 individuals diagnosed with COPD by post-bronchodilator FEV1/FVC < 70%. The overall and overall standardized prevalences were 20.4% (18.7–22.0) and 19.7% (17.9–21.6). Taking 1,500 m as the boundary to divide low and high altitudes, we found that the prevalence in high altitudes was significantly higher than in low altitudes [high altitudes vs. low altitudes: 23.4% (20.7–26.4) vs. 18.8% (16.9–20.5), P = 0.006]. This tendency was also statistically significant in some subgroups (P < 0.05). The details were summarized in Table 2.
Table 2. Prevalence of COPD in Gansu participants aged 40 years and older by different characteristics (GOLD criterion).
There were 538 individuals diagnosed with COPD by post-bronchodilator FEV1/FVC < LLN. The overall and overall standardized prevalences were 21.7% (20.2–23.3) and 21.3% (19.5–23.3). The prevalence in high altitudes was significantly higher than in low altitudes [high altitudes vs. low altitudes: 24.7% (22.0–27.8) vs. 20.0% (18.0–22.1), P = 0.006]. This tendency was also statistically significant in some subgroups (P < 0.05). The details were summarized in Supplementary Table S1.
The formula calculating the predicted FEV1 was suitable for the participants aged 40–81 years, so five patients older than 81 were excluded. Finally, 505 patients were included in the severity assessment. Among the eligible patients, the proportion of GOLD stage I, GOLD stage II, and GOLD stage III or IV was 67.1% (63.0–71.5), 28.7% (24.8–33.1), and 4.2% (2.6–5.9), respectively. The result of the mMRC dyspnea scale showed that the proportion of Grade 0, Grade 1, Grade 2, Grade 3, and Grade 4 was 55.1% (51.0–59.3), 17.3% (14.0–20.7), 16.7% (13.8–20.3), 5.3% (3.31–7.3), and 5.3% (3.31–7.3), respectively. Furthermore, compared to low-altitude patients, high-altitude patients had worse lung function and more serious dyspnea (both P < 0.05; Table 3, Supplementary Table S2).
Biomass as fuel and ethnic minorities almost occurred at high altitudes (Table 1). Taking them into PSM would enormously decrease the sample size. In addition, the distributions of ethnicity and biomass as fuel between cases and controls were similar to the altitude, which might lead to multicollinearity and conceal the actual association between altitude and COPD if we take them into the PSM-processed and multivariate logistic regression models. Thus, the two variables were not considered in calculating propensity score (PS) and the multivariate logistic regression model.
After matching, 699 participants were retained in each group; the two populations have similar distributions of PS and comparable characteristics (SMD < 0.2, P < 0.05; Figure 3, Supplementary Table S3). Further, logistic regression models indicated that high altitude increased COPD risk in both the criteria of FEV1/FVC < 0.7 [crude OR: 1.323 (1.084–1.615); Adjusted OR: 1.408 (1.107–1.79); PSM OR: 1.516 (1.162–1.978)] and FEV1/FVC < LLN [crude OR: 1.318 (1.084–1.602); adjusted OR: 1.544 (1.227–1.944); PSM OR: 1.599 (1.232–2.076); Table 4].
Participants were randomly divided into two sets. The training set had 1,491 individuals, while the validation set had 995 individuals. The difference in the characteristics between the two populations was not statistically significant (P > 0.05; Supplementary Table S4). The univariable logistic regression model first eliminated the factors that did not statistically associate with COPD. Then, the stepwise logistic regression model reserved altitude, age, sex, TB, coal as fuel, and smoking status for developing a nomogram (Table 5, Figure 4).
Figure 4. Nomogram for COPD reserving altitude, age, sex, history of tuberculosis, coal as fuel, and smoking status as predictors.
The ROC curve, calibration curve, and DCA were used to assess the efficiency of the nomogram. In the training set, the AUC was 0.722 (0.690–0.754); the predicted probabilities were almost identical to the actual probabilities; when the threshold probability ranged from 0.08 to 0.45, the clinical benefit was positive (Figures 5A–C). In the validation set, the AUC was 0.678 (0.634–0.722); the predicted probabilities also fluctuated around the actual probabilities; when the threshold probability ranged from 0.09 to 0.36, the clinical benefit was positive (Figures 5D–F). If removed altitude from the nomogram, the discrimination, calibration, and clinical benefit declined to some degree (Figures 5G–I).
Figure 5. The assessment of the nomogram. (A) AUC of the ROC curve in training set was 0.722 (0.690–0.754). (B) Calibration curve in training set demonstrated that the predicted probabilities were almost identical to the actual probabilities. (C) Decision curve analysis in training set suggested that the clinical benefit was positive when the threshold probability ranged from 0.08 to 0.45. (D) AUC of the ROC curve in validation set was 0.678 (0.634–0.722). (E) Calibration curve in validation set demonstrated that the predicted probabilities fluctuated around the actual probabilities. (F) Decision curve analysis in validation set suggested that the clinical benefit was positive when the threshold probability ranged from 0.09 to 0.36. (G) Eliminating altitude, AUC of the ROC curve in training set was 0.719 (0.688–0.751). (H) Eliminating altitude, calibration curve in training set demonstrated that the predicted probabilities were more deviated from the actual probabilities. (I) Eliminating altitude, Decision curve analysis in training set suggested that the clinical benefit was positive when the threshold probability ranged from 0.07 to 0.37.
In this cross-section study, we investigated a represented sample with 2,486 individuals aged 40 years or above in Gansu by the multistage stratified cluster sampling procedure. Using both the criteria of post-bronchodilator FEV1/FVC < 0.7 or LLN, we found that the prevalence and severity of COPD in high altitudes were higher than in low altitudes. Furthermore, the results of univariable, multivariable, and PSM-processed logistic regression models showed that high altitude was a risk factor for COPD. In addition, we identified altitude, age, sex, TB, coal as fuel, and smoking status as the risk factors and developed a nomogram for screening the high-risk population. The nomogram showed excellent discrimination, calibration, and clinical benefit in the internal validation.
The prevalence of COPD in China has risen rapidly in recent years. Among residents older than 40, the overall prevalence was about 8.2% (7.9–8.6) in 2002–2004. However, that increased to 13.6% (12.0–15.2) just a decade later (3, 4). Fang et al. (4) invoked data from the surveillance points in northwest China, only two of which were located in Gansu, and concluded that the standardized prevalence in northwest China was 13.6% (9.5–17.6). However, after investigating four representative regions in Gansu, we found that the standardized prevalence in Gansu was 19.7% (17.9–21.6) diagnosed by the GOLD criterion, while that was 21.3% (19.5–23.3) diagnosed by post-bronchodilator FEV1/FVC < LLN. Previous studies found that unclean fuels were frequently used in northwest China (coal as fule: 49.4%; biomass as fule: 48.1%), but our study found a higher frequency in Gansu (coal as fule: 53.7%; biomass as fule: 83.9%), which might lead to the much higher prevalence in Gansu than in northwest China (4). Notedly, in the total, low-altitude and high-altitude populations, the prevalences diagnosed by post-bronchodilator FEV1/FVC < LLN were higher in the middle-aged and lower in the elderly when compared to the prevalences diagnosed by the GOLD criterion (Figures 6A–C). LLN as the diagnostic criterion might have an advantage in improving diagnostic sensitivity in the middle-aged and avoiding overdiagnosis in the elderly (22).
The role of altitude on COPD is still unclear (13). Some epidemiological studies indicated that high altitude was a protective factor for COPD (25–29). Recently, an epidemiological study combining several databases even suggested that high altitude (>1,500 m) did not associate with COPD (15). However, the participants of those studies were westerners whose genetic background might differ from Chinese (15, 25–29). In some research, the exposure frequencies of some known risk factors were lower at high altitudes, which contracted to our country (15, 25, 29). In addition, some studies either did not perform multivariate analysis or missed crucial risk factors in the model for adjusting confoundings (coal as fuel, etc.) (15, 26, 27, 29). We advocated that high altitude increased COPD risk and severity, which was also one of the mainstream views (14, 29–33). There were two reasonable explanations. On the one hand, high-altitude residents tended to use unclean energy for cooking and warming. Long-term exposure to indoor air pollutants might promote inflammation and oxidative stress in the lung (34). On the other hand, a climate like hypobaric and hypoxic would stimulate hypoxia-related genes, which might play a harmful role in a series of physiological and biochemical processes such as inflammation, lung development, pulmonary hypertension or remodeling, and vascular permeability (35, 36).
Our result only regarded age, sex, TB, coal as fuel, and smoking as the COPD-relative predictors. In the univariable analysis, childhood hospital admission for severe respiratory disease, educational level, biomass as fuel, occupational exposure, and BMI were not significantly associated with COPD. One of the reasons might be the crude evaluation of the exposures like biomass as fuel and occupational exposure. Besides, similar exposure frequencies of the above factors in cases and controls required a larger sample to identify the association between the risk factors and COPD (37). Noteworthily, ethnicity was significantly associated with COPD in the univariable analysis. But the stepwise logistic regression model eliminated ethnicity, which might cause by its strong association with altitude (38).
Based on the multivariable regression model, the nomogram provides a quick, visual, and accurate tool to predict the probability of clinical outcomes and is popular among medical workers (39). As we know, this tool is rarely utilized for screening the COPD high-risk population (40, 41). In this study, we creatively developed a nomogram for COPD screening, which was evaluated by the ROC curve, calibration curve, and DCA. In the training and validation sets, the nomogram demonstrated excellent calibration and clinical benefit. However, the AUC in the training set was 0.722 (0.690–0.754), while that in the validation set was 0.678 (0.634–0.722), merely indicating acceptable discrimination of the nomogram. More candidate predictors should be enrolled to improve the efficiency of the nomogram by expanding the sample size in future work. We also found that the discrimination, calibration, and clinical benefit declined after removing altitude from the nomogram, reiterating that altitude is an important risk factor for COPD.
This study has some limitations: (1) As a retrospective and cross-section study, the recall bias and defect in causal inference are inevitable. (2) The evaluation of some risk factors is crude, which may lead to false negative results. (3) The sample in high altitudes is relatively small, requiring a larger sample to detect the candidate risk factors with a similar distribution in cases and controls (37). (4) Our nomogram needs further external validation.
COPD has become a severe public health problem in Gansu. High altitude is a critical risk factor besides aging, male, TB, coal as fuel, and smoking. The nomogram with the above risk factors has satisfactory efficiency in screening high-risk individuals.
The raw data supporting the conclusions of this article will be made available by the authors, without undue reservation.
The studies involving human participants were reviewed and approved by the Institutional Review Board of Guangzhou Medical University and Xi'an Jiaotong University Health Science Center—approval: GZMC2007-07-0676 and XJTU2016-411. The patients/participants provided their written informed consent to participate in this study.
AL analyzed and interpreted the data and completed the writing. CM, BR, YW, GY, and HL took part in investigation. JL, XW, HZ, and XZ made contributions to conceptualization, participants recruitment, and project management. JL, YD, CX, and DH guided the methodology and revised the manuscript. All authors have read and approved the final manuscript.
This study was supported by the following grants: National Natural Science Foundation of China 82173609, 81872694, and 81673267 (JL), Local Innovative and Research Teams Project of Guangdong Pearl River Talents Program 2017BT01S155 (JL), the Science and Technology Project of Guangzhou 202103000073 (JL), National Key Research and Development Project of China 2017YFC0907202 (XW), National Natural Science Foundation of China 81460123 (YD), Guangxi Natural Foundation 2018GXNSFAA281187 (YD), and National Natural Science Foundation of China 82260889 (XZ).
The authors declare that the research was conducted in the absence of any commercial or financial relationships that could be construed as a potential conflict of interest.
All claims expressed in this article are solely those of the authors and do not necessarily represent those of their affiliated organizations, or those of the publisher, the editors and the reviewers. Any product that may be evaluated in this article, or claim that may be made by its manufacturer, is not guaranteed or endorsed by the publisher.
The Supplementary Material for this article can be found online at: https://www.frontiersin.org/articles/10.3389/fpubh.2023.1127566/full#supplementary-material
1. Soriano JB, Kendrick PJ, Paulson KR, Gupta V, Abrams EM, Adedoyin RA, et al. Prevalence and attributable health burden of chronic respiratory diseases, 1990-2017: a systematic analysis for the Global Burden of Disease Study 2017. Lancet Respir Med. (2020) 8:585–96. doi: 10.1016/S2213-2600(20)30105-3
2. Christenson SA, Smith BM, Bafadhel M, Putcha N. Chronic obstructive pulmonary disease. Lancet. (2022) 399:2227–42. doi: 10.1016/S0140-6736(22)00470-6
3. Zhong N, Wang C, Yao W, Chen P, Kang J, Huang S, et al. Prevalence of chronic obstructive pulmonary disease in China: a large, population-based survey. Am J Respir Crit Care Med. (2007) 176:753–60. doi: 10.1164/rccm.200612-1749OC
4. Fang L, Gao P, Bao H, Tang X, Wang B, Feng Y, et al. Chronic obstructive pulmonary disease in China: a nationwide prevalence study. Lancet Respir Med. (2018) 6:421–30. doi: 10.1016/S2213-2600(18)30103-6
5. Singh D, Agusti A, Anzueto A, Barnes PJ, Bourbeau J, Celli BR, et al. Global strategy for the diagnosis, management, and prevention of chronic obstructive lung disease: the GOLD science committee report 2019. Eur Respir J. (2019) 53:1900164. doi: 10.1183/13993003.00164-2019
6. Zhu B, Wang Y, Ming J, Chen W, Zhang L. Disease burden of COPD in China: a systematic review. Int J Chron Obstruct Pulmon Dis. (2018) 13:1353–64. doi: 10.2147/COPD.S161555
7. Fan J, Liu X, Pan W, Douglas MW, Bao S. Epidemiology of coronavirus disease in Gansu Province, China, 2020. Emerg Infect Dis. (2020) 26:1257–65. doi: 10.3201/eid2606.200251
8. Bai J, Li L, Li Y, Zhang L. Genetic and immune changes in Tibetan high-altitude populations contribute to biological adaptation to hypoxia. Environ Health Prev Med. (2022) 27:39. doi: 10.1265/ehpm.22-00040
9. West JB. High-altitude medicine. Am J Respir Crit Care Med. (2012) 186:1229–37. doi: 10.1164/rccm.201207-1323CI
10. Sharma V, Varshney R, Sethy NK. Human adaptation to high altitude: a review of convergence between genomic and proteomic signatures. Hum Genomics. (2022) 16:21. doi: 10.1186/s40246-022-00395-y
11. Mallet RT, Burtscher J, Richalet JP, Millet GP, Burtscher M. Impact of high altitude on cardiovascular health: current perspectives. Vasc Health Risk Manag. (2021) 17:317–35. doi: 10.2147/VHRM.S294121
12. Salvi SS, Barnes PJ. Chronic obstructive pulmonary disease in non-smokers. Lancet. (2009) 374:733–43. doi: 10.1016/S0140-6736(09)61303-9
13. Xiong H, Huang Q, He C, Shuai T, Yan P, Zhu L, et al. Prevalence of chronic obstructive pulmonary disease at high altitude: a systematic review and meta-analysis. PeerJ. (2020) 8:e8586. doi: 10.7717/peerj.8586
14. Guo Y, Xing Z, Shan G, Janssens JP, Sun T, Chai D, et al. Prevalence and risk factors for COPD at high altitude: a large cross-sectional survey of subjects living between 2,100-4,700 m above sea level. Front Med. (2020) 7:581763. doi: 10.3389/fmed.2020.581763
15. Horner A, Soriano JB, Puhan MA, Studnicka M, Kaiser B, Vanfleteren L, et al. Altitude and COPD prevalence: analysis of the PREPOCOL-PLATINO-BOLD-EPI-SCAN study. Respir Res. (2017) 18:162. doi: 10.1186/s12931-017-0643-5
16. Graham BL, Steenbruggen I, Miller MR, Barjaktarevic IZ, Cooper BG, Hall GL, et al. Standardization of spirometry 2019 update. An official american thoracic society and european respiratory society technical statement. Am J Respir Crit Care Med. (2019) 200:e70–88. doi: 10.1164/rccm.201908-1590ST
17. Yang L, Lu X, Deng J, Zhou Y, Huang D, Qiu F, et al. Risk factors shared by COPD and lung cancer and mediation effect of COPD: two center case-control studies. Cancer Causes Control. (2015) 26:11–24. doi: 10.1007/s10552-014-0475-2
18. Muza SR, Beidleman BA, Fulco CS. Altitude preexposure recommendations for inducing acclimatization. High Alt Med Biol. (2010) 11:87–92. doi: 10.1089/ham.2010.1006
19. Zheng C, Wang X, Tang H, Chen Z, Zhang L, Wang S, et al. Habitation altitude and left ventricular diastolic function: a population-based study. J Am Heart Assoc. (2021) 10:e018079. doi: 10.1161/JAHA.120.018079
20. Grissom CK, Jones BE. Respiratory health benefits and risks of living at moderate altitude. High Alt Med Biol. (2018) 19:109–15. doi: 10.1089/ham.2016.0142
21. Talaminos-Barroso A, Roa-Romero LM, Ortega-Ruiz F, Cejudo-Ramos P, Marquez-Martin E, Reina-Tosina J. Effects of genetics and altitude on lung function. Clin Respir J. (2021) 15:247–56. doi: 10.1111/crj.13300
22. Jian W, Gao Y, Hao C, Wang N, Ai T, Liu C, et al. Reference values for spirometry in Chinese aged 4-80 years. J Thorac Dis. (2017) 9:4538–49. doi: 10.21037/jtd.2017.10.110
23. National Bureau of Statistics. (2013). 2010 Population Census. Available online at: http://www.stats.gov.cn/english/Statisticaldata/CensusData/ (accessed November 18, 2022).
24. Zhao QY, Luo JC, Su Y, Zhang YJ, Tu GW, Luo Z. Propensity score matching with R: conventional methods and new features. Ann Transl Med. (2021) 9:812. doi: 10.21037/atm-20-3998
25. Menezes AM, Perez-Padilla R, Jardim JR, Muino A, Lopez MV, Valdivia G, et al. Chronic obstructive pulmonary disease in five Latin American cities (the PLATINO study): a prevalence study. Lancet. (2005) 366:1875–81. doi: 10.1016/S0140-6736(05)67632-5
26. Havryk AP, Gilbert M, Burgess KR. Spirometry values in Himalayan high altitude residents (Sherpas). Respir Physiol Neurobiol. (2002) 132:223–32. doi: 10.1016/S1569-9048(02)00072-1
27. Wood S, Norboo T, Lilly M, Yoneda K, Eldridge M. Cardiopulmonary function in high altitude residents of Ladakh. High Alt Med Biol. (2003) 4:445–54. doi: 10.1089/152702903322616191
28. Jaganath D, Miranda JJ, Gilman RH, Wise RA, Diette GB, Miele CH, et al. Prevalence of chronic obstructive pulmonary disease and variation in risk factors across four geographically diverse resource-limited settings in Peru. Respir Res. (2015) 16:40. doi: 10.1186/s12931-015-0198-2
29. Laniado-Laborin R, Rendon A, Batiz F, Alcantar-Schramm JM, Bauerle O. High altitude and chronic obstructive pulmonary disease prevalence: a casual or causal correlation? Arch Bronconeumol. (2012) 48:156–60. doi: 10.1016/j.arbr.2011.12.004
30. Hwang J, Jang M, Kim N, Choi S, Oh YM, Seo JB. Positive association between moderate altitude and chronic lower respiratory disease mortality in United States counties. PLoS ONE. (2018) 13:e0200557. doi: 10.1371/journal.pone.0200557
31. Ezzati M, Horwitz ME, Thomas DS, Friedman AB, Roach R, Clark T, et al. Altitude, life expectancy and mortality from ischaemic heart disease, stroke, COPD and cancers: national population-based analysis of US counties. J Epidemiol Community Health. (2012) 66:e17. doi: 10.1136/jech.2010.112938
32. Cote TR, Stroup DF, Dwyer DM, Horan JM, Peterson DE. Chronic obstructive pulmonary disease mortality. A role for altitude. Chest. (1993) 103:1194–7. doi: 10.1378/chest.103.4.1194
33. Brakema EA, Tabyshova A, Kasteleyn MJ, Molendijk E, van der Kleij R, van Boven JFM, et al. High COPD prevalence at high altitude: does household air pollution play a role? Eur Respir J. (2019) 53:1801193. doi: 10.1183/13993003.01193-2018
34. Olloquequi J, Silva OR. Biomass smoke as a risk factor for chronic obstructive pulmonary disease: effects on innate immunity. Innate Immun. (2016) 22:373–81. doi: 10.1177/1753425916650272
35. Miele CH, Grigsby MR, Siddharthan T, Gilman RH, Miranda JJ, Bernabe-Ortiz A, et al. Environmental exposures and systemic hypertension are risk factors for decline in lung function. Thorax. (2018) 73:1120–7. doi: 10.1136/thoraxjnl-2017-210477
36. Prabhakar NR, Semenza GL. Adaptive and maladaptive cardiorespiratory responses to continuous and intermittent hypoxia mediated by hypoxia-inducible factors 1 and 2. Physiol Rev. (2012) 92:967–1003. doi: 10.1152/physrev.00030.2011
37. Schmidt SAJ, Lo S, Hollestein LM. Research techniques made simple: sample size estimation and power calculation. J Invest Dermatol. (2018) 138:1678–82. doi: 10.1016/j.jid.2018.06.165
38. Vatcheva KP, Lee M, McCormick JB, Rahbar MH. Multicollinearity in regression analyses conducted in epidemiologic studies. Epidemiology. (2016) 6:227 doi: 10.4172/2161-1165.1000227
39. Wang X, Lu J, Song Z, Zhou Y, Liu T, Zhang D. From past to future: bibliometric analysis of global research productivity on nomogram (2000-2021). Front Public Health. (2022) 10:997713. doi: 10.3389/fpubh.2022.997713
40. Lee SC, An C, Yoo J, Park S, Shin D, Han CH. Development and validation of a nomogram to predict pulmonary function and the presence of chronic obstructive pulmonary disease in a Korean population. BMC Pulm Med. (2021) 21:32. doi: 10.1186/s12890-021-01391-z
Keywords: COPD, altitude, prevalence, risk factor, nomogram
Citation: Lin A, Mao C, Rao B, Zhao H, Wang Y, Yang G, Lei H, Xie C, Huang D, Deng Y, Zhang X, Wang X and Lu J (2023) Development and validation of nomogram including high altitude as a risk factor for COPD: A cross-sectional study based on Gansu population. Front. Public Health 11:1127566. doi: 10.3389/fpubh.2023.1127566
Received: 19 December 2022; Accepted: 13 February 2023;
Published: 02 March 2023.
Edited by:
Weihong Chen, Huazhong University of Science and Technology, ChinaReviewed by:
Carlos A. Torres-Duque, Fundación Neumológica Colombiana, ColombiaCopyright © 2023 Lin, Mao, Rao, Zhao, Wang, Yang, Lei, Xie, Huang, Deng, Zhang, Wang and Lu. This is an open-access article distributed under the terms of the Creative Commons Attribution License (CC BY). The use, distribution or reproduction in other forums is permitted, provided the original author(s) and the copyright owner(s) are credited and that the original publication in this journal is cited, in accordance with accepted academic practice. No use, distribution or reproduction is permitted which does not comply with these terms.
*Correspondence: Jiachun Lu, amNMdUBnemhtdS5lZHUuY24=; Xinhua Wang, d3hoQGdzenkuZWR1LmNu
†These authors have contributed equally to this work
Disclaimer: All claims expressed in this article are solely those of the authors and do not necessarily represent those of their affiliated organizations, or those of the publisher, the editors and the reviewers. Any product that may be evaluated in this article or claim that may be made by its manufacturer is not guaranteed or endorsed by the publisher.
Research integrity at Frontiers
Learn more about the work of our research integrity team to safeguard the quality of each article we publish.