- 1Institute of Medicine, Chung Shan Medical University, Taichung City, Taiwan
- 2College of Medicine, Chung Shan Medical University, Taichung City, Taiwan
- 3Department of Medical Imaging, Chung Shan Medical University Hospital, Taichung City, Taiwan
- 4Department of Medical Imaging, School of Medicine, Chung Shan Medical University, Taichung City, Taiwan
- 5Department of Medical Imaging and Radiological Sciences, Chung Shan Medical University, Taichung City, Taiwan
- 6Department of Public Health and Institute of Public Health, Chung Shan Medical University, Taichung City, Taiwan
- 7Division of Thoracic Surgery, Department of Surgery, Chung Shan Medical University Hospital, Taichung City, Taiwan
Background: Cigarette smoking and particulate matter (PM) with aerodynamic diameter < 2.5 μm (PM2.5) are major preventable cardiovascular mortality and morbidity promoters. Their joint role in metabolic syndrome (MS) pathogenesis is unknown. We determined the risk of MS based on PM2.5 and cigarette smoking in Taiwanese adults.
Methods: The study included 126,366 Taiwanese between 30 and 70 years old with no personal history of cancer. The Taiwan Biobank (TWB) contained information on MS, cigarette smoking, and covariates, while the Environmental Protection Administration (EPA), Taiwan, contained the PM2.5 information. Individuals were categorized as current, former, and nonsmokers. PM2.5 levels were categorized into quartiles: PM2.5 ≤ Q1, Q1 < PM2.5 ≤ Q2, Q2 < PM2.5 ≤ Q3, and PM2.5 > Q3, corresponding to PM2.5 ≤ 27.137, 27.137 < PM2.5 ≤ 32.589, 32.589 < PM2.5 ≤ 38.205, and PM2.5 > 38.205 μg/m3.
Results: The prevalence of MS was significantly different according to PM2.5 exposure (p-value = 0.0280) and cigarette smoking (p-value < 0.0001). Higher PM2.5 levels were significantly associated with a higher risk of MS: odds ratio (OR); 95% confidence interval (CI) = 1.058; 1.014–1.104, 1.185; 1.134–1.238, and 1.149; 1.101–1.200 for 27.137 < PM2.5 ≤ 32.589, 32.589 < PM2.5 ≤ 38.205, and PM2.5 > 38.205 μg/m3, respectively. The risk of MS was significantly higher among former and current smokers with OR; 95% CI = 1.062; 1.008–1.118 and 1.531; 1.450–1.616, respectively, and a dose-dependent p-value < 0.0001. The interaction between both exposures regarding MS was significant (p-value = 0.0157). Stratification by cigarette smoking revealed a significant risk of MS due to PM2.5 exposure among nonsmokers: OR (95% CI) = 1.074 (1.022–1.128), 1.226 (1.166–1.290), and 1.187 (1.129–1.247) for 27.137 < PM2.5 ≤ 32.589, 32.589 < PM2.5 ≤ 38.205, and PM2.5 > 38.205 μg/m3, respectively. According to PM2.5 quartiles, current smokers had a higher risk of MS, regardless of PM2.5 levels (OR); 95% CI = 1.605; 1.444–1.785, 1.561; 1.409–1.728, 1.359; 1.211–1.524, and 1.585; 1.418–1.772 for PM2.5 ≤ 27.137, 27.137 < PM2.5 ≤ 32.589, 32.589 < PM2.5 ≤ 38.205, and PM2.5 > 38.205 μg/m3, respectively. After combining both exposures, the group, current smokers; PM2.5 > 38.205 μg/m3 had the highest odds (1.801; 95% CI =1.625–1.995).
Conclusion: PM2.5 and cigarette smoking were independently and jointly associated with a higher risk of MS. Stratified analyses revealed that cigarette smoking might have a much higher effect on MS than PM2.5. Nonetheless, exposure to both PM2.5 and cigarette smoking could compound the risk of MS.
Background
Metabolic syndrome (MS) is a condition characterized by the coexistence of at least three metabolic risk markers, including impaired fasting blood glucose (sugar), dyslipidemia (low high-density cholesterol and high triglyceride), abdominal obesity (high waist circumference), and elevated blood pressure (1–4). MS is a public health challenge with a huge global burden: it enhances morbidity and mortality related to chronic diseases such as cancer, stroke, diabetes, asthma, and atherosclerotic and nonatherosclerotic cardiovascular disease (5–7). Metabolic risk factors such as blood pressure, fasting plasma glucose, and high total cholesterol were among the ten largest contributors to global disability-adjusted life years (DALYs) in 2015 (8). MS has multiple promoting factors including, age (9), unhealthy diet (10, 11), obesity (11), alcohol consumption (12, 13), physical inactivity (11, 13), cigarette smoking (13–23), and PM2.5 (24–27).
Cigarette smoking is a major preventable promoter of global cardiovascular mortality and morbidity (16, 28, 29). In 2015, it was among the five top risk factors attributable to global DALYs in 109 countries (8). The influence of cigarette smoking on MS and its components is contentious (14, 30). For instance, cigarette smoking was a significant cause of MS among Chinese (14), Koreans (31–33), and Japanese (18, 23). Nonetheless, it was not significantly associated with MS among Japanese (34) and Chinese (35). Furthermore, heavy cigarette smoking among Turkish women was suggested as being protective against future MS (36).
Air pollution, especially PM2.5 (fine PM) is an urgent global public health concern, with continuously increasing implications (4, 9, 37–46). It significantly enhances neurological and cardiovascular morbidity and mortality (47, 48). Several studies reported contrasting findings regarding the relationship between MS and PM2.5 (14–23, 31–33, 49–51). A recent systematic review and meta-analysis found that PM2.5 could contribute to as much as 12.28% of MS (52). In several original studies, PM2.5 exposure significantly elevated the risk of MS among Chinese (25–27, 53, 54), Saudi (55), and Korean adults (56). On the contrary, PM2.5 did not significantly affect the risk of MS among Germans (57) and Chinese (50).
The positive association between PM2.5 and MS was more prominent in cigarette smokers, alcohol drinkers, and obese people (25, 26, 53). This suggests that smoking and other unhealthy habits could exacerbate the adverse effects of air pollution (25, 26, 53). Smoking could also confound the effect of air pollution on cardiovascular health (58). Hence, pinpointing the combined effect of cigarette smoking and PM2.5 could narrow the data gap for the burden of disease attributable to both exposures (59). Moreover, determining the interaction between PM2.5 and smoking could provide insightful knowledge regarding the susceptibility to PM2.5-related adverse health conditions in smokers and nonsmokers (59). High exposure to PM2.5 among Chinese was recently associated with a higher risk of hypertension caused by smoking (60). However, robust studies have not been conducted to determine the combined effect of PM2.5 and cigarette smoking on MS. In the current study, we determined the independent association of ambient PM2.5 and smoking with MS in Taiwanese adults. Moreover, we determined the interaction between PM2.5 and smoking regarding MS.
Methods
Study participants and data acquisition
We acquired information relating to MS, cigarette smoking, sex, age, weight, height, alcohol drinking, exercise, marital status, educational level, secondhand smoke exposure, and duration of residence from the TWB (2008–2020). The TWB database is one of the human biological databases currently supplying data for biomedical research in Taiwan (61). The TWB project is a community-based prospective study whose participants are exclusively Taiwanese adults with no personal history of cancer (62, 63). At the start of the project, only Taiwanese aged 30–70 were eligible for enrolment (63). Currently, individuals between 20 and 70 years old without a diagnosis of cancer can enroll in the project (62). The TWB biobank currently contains over 30 recruitment sites all over Taiwan (62). All volunteers sign informed consent forms before enrolment. At enrolment, each volunteer fills out the TWB questionnaire, undergoes anthropometric examinations, and provides blood/urine samples for biochemical testing. The questionnaire contains information on cigarette smoking, sex, age, alcohol drinking, exercise, etc. The anthropometry examination determines weight, height, waist circumference, and blood pressure. The biochemical tests determine fasting blood glucose (FBG), triglyceride (TG), and high-density lipoprotein cholesterol (HDL-C), among others.
Currently, the TWB database lacks PM2.5 data. Notwithstanding, the Taiwan Environmental Protection Administration (EPA) contains about 71 automated stations that record daily average PM2.5 concentrations. We used the EPA daily average data from 2000 to 2016 and computed the annual average PM2.5 concentrations (μg/m3). The spatial–temporal variability of PM2.5 in 349 areas in Taiwan was assessed using machine learning-coupled land-use regression (LUR) as previously described (64). The PM2.5 data for each area was considered the exposure data for the participants’ current residing there. The initial study sample was 131,498. However, we excluded 5,132 individuals with missing information for at least one variable. The final analysis included 126,366 people with complete data. The Institutional Review Board (IRB) of the Chung Shan Medical University Hospital granted ethical approval for this study (IRB: CS1-20009).
Definition of variables
MS was defined as the presence of at least three of the following metabolic markers: (1) waist circumference ≥ 90 cm in men or ≥ 80 cm in women; (2) systolic blood pressure (SBP) ≥ 130 mmHg or diastolic blood pressure (DBP) ≥ 85 mmHg; (3) FBG ≥ 100 mg/dL; (4) HDL-C < 40 mg/dL for men and < 50 mg/dL for women; (5) triglyceride (TG) ≥ 150 mg/dL. This definition was based on the guidelines of the Health Promotion Administration, Ministry of Health and Welfare, Taiwan. Mean annual PM2.5 concentrations between 2000 and 2016 were grouped into quartiles: PM2.5 ≤ Q1 (PM2.5 ≤ 27.137 μg/m3), Q1 < PM2.5 ≤ Q2 (27.137 < PM2.5 ≤ 32.589 μg/m3), Q2 < PM2.5 ≤ Q3 (32.589 < PM2.5 ≤ 38.205 μg/m3), and PM2.5 > Q3 (PM2.5 > 38.205 μg/m3). Smoking habits were self-reported, and individuals were categorized as current, former, or nonsmokers. Current smokers included those who smoked cigarettes for at least six months and were still smoking during the data collection period. Former smokers were those who smoked cigarettes for at least six months in the past but had quit the habit for over six months. Nonsmokers were those with no personal history of cigarette smoking.
The body mass index (BMI) was computed as weight/height squared (kg/m2). The cutoff values for BMI categories were BMI < 18.5, 18.5 ≤ BMI < 24, 24 ≤ BMI < 27, and BMI ≥ 27 kg/m2, corresponding to normal weight, underweight, overweight, and obesity. Current drinkers were individuals who confirmed having a regular habit of consuming at least 150 mL of alcohol per week continuously for half a year or more. Former drinkers included those who drank 150 mL of alcohol per week continuously for at least half a year in the past but had quit the habit for over six months. Nondrinkers included those who drank <150 mL of alcohol per week. Physically active individuals included those who had a habit of regularly engaging in physical activities (lasting over half an hour) at least three times weekly. Exposure to secondhand smoke referred to habitual exposure to secondhand smoke for 5 min or more in an hour. For marital status, participants were regarded as being married (still married), single, divorced/separated (not yet married/divorced or separated from their spouses), or widowed (lost a partner). Educational level categories included, elementary and below, junior and senior high school, or university and above. The quartiles for the duration of residence were < 7.58, 7.58–17.58, 17.58–29.58, and ≥ 29.58 years.
Statistical analyses
The differences in age (a continuous variable) between participants with and without MS were determined with the Student t-test. The differences in the percentage distribution of categorical variables (e.g., sex, cigarette smoking) between those with and without MS were determined using the Chi-square test. Age was presented in mean ± standard error (SE) while the categorical variables were presented as n (%). The risk of MS based on PM2.5, cigarette smoking, and the interaction between both exposures was determined by multivariate logistic regression. In the logistic regression model assessing the interaction between cigarette smoking and PM2.5 on MS, the p-value was obtained by putting the interaction term (cigarette smoking*PM2.5) as the main exposure (independent variable) and MS as the outcome variable. In all the regression analyses, adjustments were made for sex, age, weight, height, alcohol drinking, exercise, marital status, educational level, secondhand smoke exposure, and duration of residence. A p-value < 0.05 was set as the threshold for statistical significance. Data were managed and analyzed using SAS 9.4 (SAS Institute Inc., Cary, NC).
Results
Table 1 shows the demographic characteristics of the 126,366 study participants comprising 26,767 MS cases and 99,599 individuals without MS. The PM2.5 quartiles were PM2.5 ≤ Q1 (PM2.5 ≤ 27.137), Q1 < PM2.5 ≤ Q2 (27.137 < PM2.5 ≤ 32.589 μg/m3), Q2 < PM2.5 ≤ Q3 (32.589 < PM2.5 ≤ 38.205 μg/m3), and PM2.5 > Q3 (PM2.5 > 38.205 μg/m3). Individuals with and without MS significantly differed in terms of PM2.5 concentration (p-value = 0.0280), cigarette smoking, and other variables, including sex, age, BMI, alcohol intake, marital status, educational level, secondhand smoke exposure, and duration of residence (p-value < 0.0001). Among the 99,599 individuals without MS, 24,378 (24.48%), 27,157 (27.27%), 23,147 (23.24%), 24,917 (25.02%) were within the PM2.5 quartiles, PM2.5 ≤ Q1, Q1 < PM2.5 ≤ Q2, Q2 < PM2.5 ≤ Q3, and PM2.5 > Q3, respectively. Among the 26,767 MS cases, 6,370 (23.80%), 7,250 (27.09%), 6,414 (23.96%), and 6,733 (25.15%) were within the PM2.5 quartiles, PM2.5 ≤ Q1, Q1 < PM2.5 ≤ Q2, Q2 < PM2.5 ≤ Q3, and PM2.5 > Q3, respectively. The group without MS comprised 81,706 (82.03%) nonsmokers, 9,687 (9.73%) former smokers, and 8,206 (8.24%) current smokers. The MS group comprised 19,541 (73.00%) nonsmokers, 3,628 (13.55%) former smokers, and 3,598 (13.44%) nonsmokers.
Table 2 and Supplementary Figures S1, S2 present the association of MS with PM2.5 and cigarette smoking. Higher compared to lower PM2.5 levels (27.137 < PM2.5 ≤ 32.589, 32.589 < PM2.5 ≤ 38.205, and PM2.5 > 38.205 vs. PM2.5 ≤ 27.137 μg/m3) were significantly associated with a higher risk of MS (OR; 95% CI = 1.058; 1.014–1.104 for 27.137 < PM2.5 ≤ 32.589 μg/m3, 1.185; 1.134–1.238 for 32.589 < PM2.5 ≤ 38.205 μg/m3, and 1.149; 1.101–1.200 for PM2.5 > 38.205 μg/m3). Compared to nonsmokers, former and current smokers had a higher risk of MS (OR = 1.062, 95% CI = 1.008–1.118 for former smokers and 1.531; 1.450–1.616 for current smokers). The dose–response relationship between smoking and MS was significant (p-trend <0.0001). According to the quantity of cigarettes smoked, a weekly consumption of ≥140 cigarettes per week was significantly associated with a higher risk of MS in both former and current smokers (Supplementary Table S1). The interaction between PM2.5 and cigarette smoking was significant: p-value = 0.0157 (Table 2). The risk of MS was also significantly higher among people who were ≥ 50 years (OR = 2.277, 95% CI = 2.190–2.367), overweight (OR; 95% CI = 4.219; 4.056–4.388), obese (OR; 95% CI = 13.232; 12.707–13.778), current alcohol drinkers (OR = 1.162, 95% CI = 1.092–1.236), divorced/separated (OR; 95% CI = 1.097; 1.039–1.159), and widowed (OR; 95% CI = 1.178;1.098–1.264). However, the risk was lower among underweight individuals (OR = 0.084, 95% CI = 0.057–0.124), those who exercised regularly (OR = 0.866, 95% CI = 0.839–0.895), single people (OR; 95% CI = 0.928; 0.882–0.976), those who attained a junior and senior high school level (OR; 95% CI = 0.821; 0.769–0.876), and university education and above (OR = 0.692, 95% CI = 0.648–0.740).
Table 3 shows the association between PM2.5 and MS in current, former, and nonsmokers. PM2.5 was significantly associated with a higher risk of MS among nonsmokers: OR = 1.074, 95% CI = 1.022–1.128, 1.226; 1.166–1.290, and 1.187; 1.129–1.247 for 27.137 < PM2.5 ≤ 32.589, 32.589 < PM2.5 ≤ 38.205, and PM2.5 > 38.205 μg/m3, respectively.
Table 4 illustrates the association between cigarette smoking and MS stratified by PM2.5 quartiles. Compared to nonsmokers, the risk of MS was significantly higher in former smokers when the PM2.5 concentration was ≤32.589 μg/m3: OR (95% CI) = 1.139 (1.027–1.263) for PM2.5 ≤ 27.137 μg/m3 and 1.138 (1.032–1.254) for 27.137 < PM2.5 ≤ 32.589 μg/m3. The risk of MS was significantly higher among current smokers, regardless of the PM2.5 concentration. The ORs; 95% CIs were 1.605; 1.444–1.785, 1.561; 1.409–1.728, 1.359; 1.211–1.524; and 1.585; 1.418–1.772 for PM2.5 ≤ 27.137, 27.137 < PM2.5 ≤ 32.589, 32.589 < PM2.5 ≤ 38.205, and PM2.5 > 38.205 μg/m3, respectively.
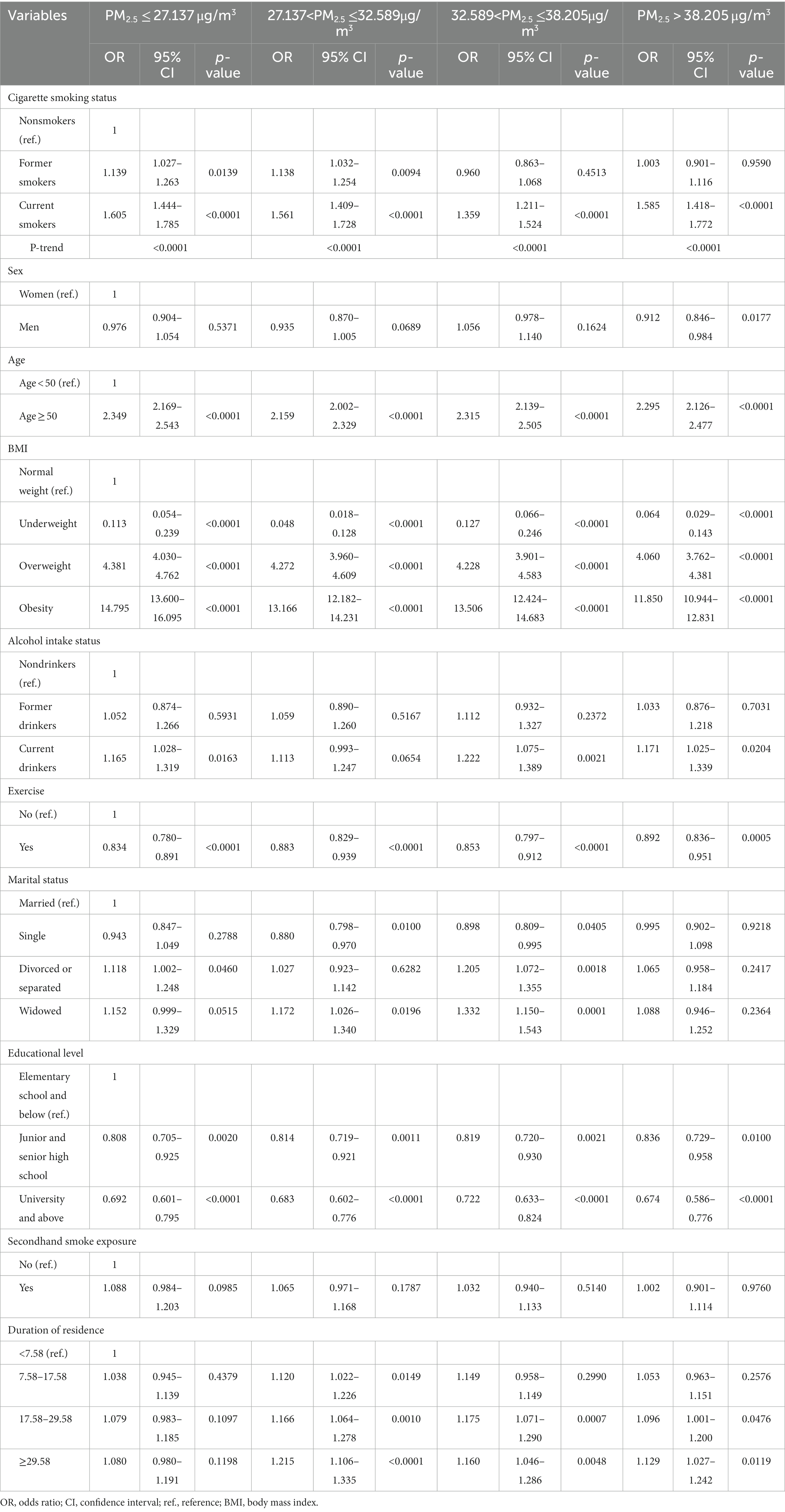
Table 4. Association between cigarette smoking and metabolic syndrome stratified by PM2.5 quartiles.
Table 5 and Supplementary Figure S3 show the risk of MS according to cigarette smoking and PM2.5 exposure. Compared to nonsmokers with low PM2.5 exposure (PM2.5 ≤ 27.137 μg/m3), the risk of MS was significantly higher in all the categories. Of note, the category comprising current smokers and PM2.5 > 38.205 μg/m3 had the highest risk of MS (OR = 1.801, 95% CI = 1.625–1.995).
Discussion
Cigarette smoking and PM2.5 have significant adverse effects on individual and public health. A systematic analysis of the global burden of disease ranked PM2.5 and cigarette smoking among the ten leading causes of death and disability in 2015 (8). We evaluated the independent and joint association of both factors with MS in Taiwan Biobank volunteers. Smoking and PM2.5 were independently associated with higher odds of MS. Moreover, both exposures were interactively associated with MS in a significant manner.
Cigarette smoking has been associated with CVD risk factors such as elevated heart rate, dyslipidemia, hyperinsulinemia, and glucose intolerance (15–17). In line with our study, several original studies and meta-analyses reported cigarette smoking as a metabolic syndrome-promoting factor (14–23, 31–33, 51). For instance, in a meta-analysis including 13 prospective studies, active smoking was positively associated with MS (51). In an original study, life-course cigarette smoking was associated with a higher risk of MS among Chinese, particularly those under 70 years (14). Moreover, a cross-sectional study among Koreans below 40 years found a higher likelihood of MS in smokers than nonsmokers (33). Furthermore, a community-based study involving Taiwanese aged 40 years and above revealed a dose-dependent positive relationship of current smoking with MS and some of its components, including high TG and low HDL (22). In addition, a study among Japanese aged 35–65 also showed a higher incidence of MS among both current and former smokers (23). Another study among Japanese between 20 and 93 years found that the risk of MS in individuals who smoked over 40 cigarettes per day persisted even after 20 years of quitting (18). A cross-sectional study among male Korean former smokers aged at least 19 years showed a higher risk of MS, hypertriglyceridemia, and hyperglycemia among those who had smoked for over 20 years (32). Another cross-sectional among male Koreans aged over 20 years also showed a higher risk of MS among former and current smokers who smoked more than ten packs of cigarettes annually (31). In a cross-sectional study involving individuals of Western European ancestry, cigarette smoking was significantly linked to a higher prevalence of MS, regardless of BMI and sex (65). In the DESIR (Données Epidémiologiques sur le Syndrome d’Insulino-Résistance) study (a longitudinal study involving French), male smokers had a significantly higher risk of MS (66). In another longitudinal study in Norway, heavy smoking increased the incidence of MS in both men and women (13). Using the Third National Health and Nutrition Examination Survey (NHANES) data, a study in the US found a lower risk of MS among normal weight and overweight men and women with no history of smoking (67).
The positive association of PM2.5 and MS in the current study is comparable to findings from previous studies (14–23, 31–33, 51). For example, exposure to PM2.5 exacerbated the risk of MS among Saudi adults (55) and Korean adults without CVDs (56). Moreover, several original studies found a positive relationship between long-term exposure to PM2.5 and MS in adult Chinese (25–27, 53, 54). A meta-analysis of observational studies revealed a borderline positive association between PM2.5 and MS (49). Exposure to PM2.5 has also been associated with an elevated risk of MS components, including high abdominal obesity (56), FBG (54–56, 68–70), high BP (55, 56, 71), and dyslipidemia (54, 56, 70). Analyses of data from the Heinz Nixdorf Recall (HNR) cohort study in Germany revealed a borderline positive association between PM2.5 and MS (57). A study in the US using data from the Normative Aging study found a significantly increased risk of MS due to increasing PM2.5 concentrations (70). Nonetheless, data from the Adolescent to Adult Health (Add Health) study (a longitudinal study in the US) showed no significant association between long-term PM2.5 exposure and MS (72).
In our study, the interaction of PM2.5 and cigarette smoking on MS was significant. It is worth noting that the joint role of both exposures in MS pathogenesis has not received considerable attention. However, some studies investigated the joint role of PM and cigarette smoking on cardiovascular and pulmonary morbidity and mortality (59, 73–75). For instance, Turner and colleagues (59) reported an increased risk of cardiovascular mortality (i.e., about 32 extra deaths per 100,000 person-years) due to smoking-PM2.5 interaction. Even though a study on cardiovascular mortality found no interaction between PM2.5 and smoking, current smokers with higher exposure to PM2.5 had a high relative risk for mortality (76). Exposure to both smoking and PM2.5 was associated with a relative excess risk of lung cancer mortality (74). Exposure to particulate matter, especially PM2.5, was also significantly associated with a higher risk of cardio-cerebrovascular disease among nonsmokers (73).
The potential mechanisms underpinning the role of smoking and PM2.5 on MS are unclear. Nonetheless, the available evidence points toward insulin resistance, induced oxidative stress, inflammation, and endothelial dysfunction. That is, smoking is believed to promote MS by inducing insulin resistance, reducing insulin sensitivity, and causing hyperglycemia, high blood pressure, hyperinsulinemia, oxidative stress, endothelial dysfunction, and systemic inflammation (15, 16, 77, 78). Air pollution, especially PM2.5, enhances MS susceptibility by disrupting insulin signaling, inducing inflammation and oxidative stress (73, 79–82). Sung Kyun Park and colleagues (83) found that in MS patients, PM could particularly affect CVDs by causing cardiac autonomic dysfunction.
The current study has some limitations. First, we included only Taiwanese adults aged 30 and 70 who were enrolled in the TWB project. The restriction of enrolment to only Taiwanese within a specific age cohort is a possible source of selection bias. As such, our conclusions may not be generalizable to non-Taiwanese and Taiwanese outside the 30–70 age group. Second, we could not ascertain PM2.5 exposure at individual levels since data were obtained from fixed monitoring stations. The non-definitive ascertainment of smoking and PM2.5 exposures could have resulted in measurement error or information bias and consequently, wrong classification. Nonetheless, we believe that the misclassification could be nondifferential as it involved both cases and controls from a community-based cohort. The nondifferential misclassification could have resulted in the underestimation of MS risk. We recommend that the findings from this study should be replicated in other populations. Moreover, studies in Taiwan should consider including adults outside the 30–70 years age group. Furthermore, to get the actual effect of cigarette smoking on MS, future studies should consider the number of cigarettes smoked and determine the levels of cotinine (a biomarker of tobacco consumption).
Conclusion
Summarily, PM2.5 and smoking were independently and interactively associated with a higher risk of MS. Stratified analyses revealed that cigarette smoking might have a much higher effect on MS than PM2.5. After integrating smoking and PM2.5 exposure in the same model, the risk of MS was highest among current cigarette smokers exposed to the highest level of PM2.5. Quitting smoking could reduce the incidence of MS in individuals exposed to PM2.5. As PM2.5 could affect nonsmokers, targeting it could also be very beneficial in reducing the risk of MS in these individuals. To curb smoking, PM2.5, and their adverse effects, the government could enforce stronger and more sustainable policies such as funding mass media campaigns on the dangers of environmental factors. The government could also provide incentives for smoking cessation treatments.
Data availability statement
The data analyzed in this study is subject to the following licenses/restrictions: the data that support the findings of this study are available from Taiwan Biobank but restrictions apply to the availability of these data, which were used under license for the current study, and so are not publicly available. Data are, however available from the corresponding author, Y-PL upon reasonable request and with permission of Taiwan Biobank. Requests to access these datasets should be directed to Y-PL, TGlhd3lwQGNzbXUuZWR1LnR3.
Ethics statement
The studies involving humans were approved by the Institutional Review Board (IRB) of the Chung Shan Medical University Hospital granted ethical approval for this study (IRB: CS1-20009). The studies were conducted in accordance with the local legislation and institutional requirements. The participants provided their written informed consent to participate in this study.
Author contributions
H-HT, DT, WL, C-YC, and Y-PL did the literature search, conceived, and designed the study. WL and Y-PL analyzed the data. H-HT, DT, and C-YC drafted the manuscript. DT edited the paper. All authors contributed to the article and approved the submitted version.
Funding
The Ministry of Science and Technology (MOST), Taiwan, funded this study (MOST 111-2121-M-040-002).
Acknowledgments
We gratefully acknowledge Chih-Da Wu for generously providing air pollution data, which significantly contributed to the research and findings presented in this paper. This collaboration was invaluable to the successful completion of our study. We are grateful to the professionals and students who provided enormous support in the recruitment and data collection of this study, and the participants who took the time to cooperate with the survey.
Conflict of interest
The authors declare that the research was conducted in the absence of any commercial or financial relationships that could be construed as a potential conflict of interest.
Publisher’s note
All claims expressed in this article are solely those of the authors and do not necessarily represent those of their affiliated organizations, or those of the publisher, the editors and the reviewers. Any product that may be evaluated in this article, or claim that may be made by its manufacturer, is not guaranteed or endorsed by the publisher.
Supplementary material
The Supplementary material for this article can be found online at: https://www.frontiersin.org/articles/10.3389/fpubh.2023.1234799/full#supplementary-material
References
1. Rochlani, Y , Pothineni, NV , Kovelamudi, S , and Mehta, JL . Metabolic syndrome: pathophysiology, management, and modulation by natural compounds. Ther Adv Cardiovasc Dis. (2017) 11:215–25. doi: 10.1177/1753944717711379
2. Huang, PL . A comprehensive definition for metabolic syndrome. Dis Model Mech. (2009) 2:231–7. doi: 10.1242/dmm.001180
3. Alberti, KG , Eckel, RH , Grundy, SM , Zimmet, PZ , Cleeman, JI , Donato, KA, et al. Harmonizing the metabolic syndrome: a joint interim statement of the international diabetes federation task force on epidemiology and prevention; national heart, lung, and blood institute; American heart association; world heart federation; international atherosclerosis society; and international association for the study of obesity. Circulation. (2009) 120:1640–5. doi: 10.1161/CIRCULATIONAHA.109.192644
4. Eckel, RH , Grundy, SM , and Zimmet, PZ . The metabolic syndrome. Lancet. (2005) 365:1415–28. doi: 10.1016/S0140-6736(05)66378-7
5. Lee, JA , Yoo, JE , and Park, HS . Metabolic syndrome and incidence of breast cancer in middle-aged Korean women: a nationwide cohort study. Breast Cancer Res Treat. (2017) 162:389–93. doi: 10.1007/s10549-017-4131-x
6. Battelli, MG , Bortolotti, M , Polito, L , and Bolognesi, A . Metabolic syndrome and cancer risk: the role of xanthine oxidoreductase. Redox Biol. (2019) 21:101070. doi: 10.1016/j.redox.2018.101070
7. O'Neill, S , and O'Driscoll, L . Metabolic syndrome: a closer look at the growing epidemic and its associated pathologies. Obes Rev. (2015) 16:1–12. doi: 10.1111/obr.12229
8. Forouzanfar, MH , Afshin, A , Alexander, LT , Anderson, HR , Bhutta, ZA , Biryukov, S, et al. Global, regional, and national comparative risk assessment of 79 behavioural, environmental and occupational, and metabolic risks or clusters of risks, 1990–2015: a systematic analysis for the global burden of disease study 2015. Lancet. (2016) 388:1659–724. doi: 10.1016/S0140-6736(16)31679-8
9. Aguilar, M , Bhuket, T , Torres, S , Liu, B , and Wong, RJ . Prevalence of the metabolic syndrome in the United States, 2003-2012. JAMA. (2015) 313:1973–4. doi: 10.1001/jama.2015.4260
10. Guo, H , Gao, X , Ma, R , Liu, J , Ding, Y , Zhang, M, et al. Prevalence of metabolic syndrome and its associated factors among multi-ethnic adults in rural areas in Xinjiang, China. Sci Rep. (2017) 7:1–9. doi: 10.1038/s41598-017-17870-5
11. Kaur, J . Assessment and screening of the risk factors in metabolic syndrome. Med Sci. (2014) 2:140–52. doi: 10.3390/medsci2030140
12. Baik, I , and Shin, C . Prospective study of alcohol consumption and metabolic syndrome. Am J Clin Nutr. (2008) 87:1455–63. doi: 10.1093/ajcn/87.5.1455
13. Wilsgaard, T , and Jacobsen, BK . Lifestyle factors and incident metabolic syndrome: the Tromsø Study 1979–2001. Diabetes Res Clin Pract. (2007) 78:217–24. doi: 10.1016/j.diabres.2007.03.006
14. Wang, J , Bai, Y , Zeng, Z , Wang, J , Wang, P , Zhao, Y, et al. Association between life-course cigarette smoking and metabolic syndrome: a discovery-replication strategy. Diabetol Metab Syndr. (2022) 14:1–11. doi: 10.1186/s13098-022-00784-2
15. Reaven, G , and Tsao, PS . Insulin resistance and compensatory hyperinsulinemia: the key player between cigarette smoking and cardiovascular disease? J Am Coll Cardiol. (2003) 41:1044–7. doi: 10.1016/S0735-1097(02)02982-0
16. Frati, AC , Iniestra, F , and Ariza, CR . Acute effect of cigarette smoking on glucose tolerance and other cardiovascular risk factors. Diabetes Care. (1996) 19:112–8. doi: 10.2337/diacare.19.2.112
17. Razay, G , and Heaton, K . Smoking habits and lipoproteins in British women. QJM. (1995) 88:503–8. doi: 10.1093/oxfordjournals.qjmed.a069093
18. Wada, T , Urashima, M , and Fukumoto, T . Risk of metabolic syndrome persists twenty years after the cessation of smoking. Intern Med. (2007) 46:1079–82. doi: 10.2169/internalmedicine.46.0026
19. Kawada, T , Otsuka, T , Inagaki, H , Wakayama, Y , Li, Q , Li, YJ, et al. Association of smoking status, insulin resistance, body mass index, and metabolic syndrome in workers: a 1-year follow-up study. Obes Res Clin Pract. (2010) 4:e163–9. doi: 10.1016/j.orcp.2009.12.004
20. Nakanishi, N , Takatorige, T , and Suzuki, K . Cigarette smoking and the risk of the metabolic syndrome in middle-aged Japanese male office workers. Ind Health. (2005) 43:295–301. doi: 10.2486/indhealth.43.295
21. Miyatake, N , Wada, J , Kawasaki, Y , Nishii, K , Makino, H , and Numata, T . Relationship between metabolic syndrome and cigarette smoking in the Japanese population. Intern Med. (2006) 45:1039–43. doi: 10.2169/internalmedicine.45.1850
22. Chen, C-C , Li, T-C , Chang, P-C , Liu, CS , Lin, WY , Wu, MT, et al. Association among cigarette smoking, metabolic syndrome, and its individual components: the metabolic syndrome study in Taiwan. Metabolism. (2008) 57:544–8. doi: 10.1016/j.metabol.2007.11.018
23. Ishizaka, N , Ishizaka, Y , Toda, E-I , Hashimoto, H , Nagai, R , and Yamakado, M . Association between cigarette smoking, metabolic syndrome, and carotid arteriosclerosis in Japanese individuals. Atherosclerosis. (2005) 181:381–8. doi: 10.1016/j.atherosclerosis.2005.01.026
24. Zhang, J-S , Gui, Z-H , Zou, Z-Y , Yang, BY , Ma, J , Jing, J, et al. Long-term exposure to ambient air pollution and metabolic syndrome in children and adolescents: a national cross-sectional study in China. Environ Int. (2021) 148:106383. doi: 10.1016/j.envint.2021.106383
25. Yang, B-Y , Qian, ZM , Li, S , Fan, S , Chen, G , Syberg, KM, et al. Long-term exposure to ambient air pollution (including PM1) and metabolic syndrome: the 33 Communities Chinese Health Study (33CCHS). Environ Res. (2018) 164:204–11. doi: 10.1016/j.envres.2018.02.029
26. Wang, Y , Liu, F , Yao, Y , Chen, M , Wu, C , Yan, Y, et al. Associations of long-term exposure to ambient air pollutants with metabolic syndrome: the Wuhan Chronic Disease Cohort Study (WCDCS). Environ Res. (2022) 206:112549. doi: 10.1016/j.envres.2021.112549
27. Hou, J , Liu, X , Tu, R , Dong, X , Zhai, Z , Mao, Z, et al. Long-term exposure to ambient air pollution attenuated the association of physical activity with metabolic syndrome in rural Chinese adults: a cross-sectional study. Environ Int. (2020) 136:105459. doi: 10.1016/j.envint.2020.105459
28. Ezzati, M , and Lopez, AD . Regional, disease specific patterns of smoking-attributable mortality in 2000. Tob Control. (2004) 13:388–95. doi: 10.1136/tc.2003.005215
29. Pan, A , Wang, Y , Talaei, M , and Hu, FB . Relation of smoking with total mortality and cardiovascular events among patients with diabetes mellitus: a meta-analysis and systematic review. Circulation. (2015) 132:1795–804. doi: 10.1161/CIRCULATIONAHA.115.017926
30. Wang, S , Chen, J , Wang, Y , Yang, Y , Zhang, D , Liu, C, et al. Cigarette smoking is negatively associated with the prevalence of type 2 diabetes in middle-aged men with normal weight but positively associated with stroke in men. J Diabetes Res. (2019) 2019:1–8. doi: 10.1155/2019/1853018
31. Oh, JE . Association between smoking status and metabolic syndrome in men. Korean J Obes. (2014) 23:99–105. doi: 10.7570/kjo.2014.23.2.99
32. Youn, JA , Lee, YH , and Noh, MS . Relationship between smoking duration and metabolic syndrome in Korean former smokers. J Korean Soc Res Nicotine Tob. (2018) 9:18–25. doi: 10.25055/JKSRNT.2018.9.1.18
33. Kim, SW , Kim, HJ , Min, K , Lee, H , Lee, SH , Kim, S, et al. The relationship between smoking cigarettes and metabolic syndrome: a cross-sectional study with non-single residents of Seoul under 40 years old. PLoS One. (2021) 16:e0256257. doi: 10.1371/journal.pone.0256257
34. Katano, S , Nakamura, Y , Nakamura, A , Murakami, Y , Tanaka, T , Nakagawa, H, et al. Relationship among physical activity, smoking, drinking and clustering of the metabolic syndrome diagnostic components. J Atheroscler Thromb. (2010) 17:644–50. doi: 10.5551/jat.3699
35. Yu, S , Guo, X , Yang, H , Zheng, L , and Sun, Y . An update on the prevalence of metabolic syndrome and its associated factors in rural Northeast China. BMC Public Health. (2014) 14:1–9. doi: 10.1186/1471-2458-14-877
36. Onat, A , Özhan, H , Esen, AM , Albayrak, S , Karabulut, A , Can, G, et al. Prospective epidemiologic evidence of a “protective” effect of smoking on metabolic syndrome and diabetes among Turkish women—without associated overall health benefit. Atherosclerosis. (2007) 193:380–8. doi: 10.1016/j.atherosclerosis.2006.07.002
37. Borsi, SH , Goudarzi, G , Sarizadeh, G , Dastoorpoor, M , Geravandi, S , Shahriyari, HA, et al. Health endpoint of exposure to criteria air pollutants in ambient air of on a populated in Ahvaz City, Iran. Front Public Health. (2022) 10:869656. doi: 10.3389/fpubh.2022.869656
38. Abbasi-Kangevari, M , Malekpour, M-R , Masinaei, M , Moghaddam, SS , Ghamari, SH , Abbasi-Kangevari, Z, et al. Effect of air pollution on disease burden, mortality, and life expectancy in North Africa and the Middle East: a systematic analysis for the global burden of disease study 2019. Lancet Planet Health. (2023) 7:e358–69. doi: 10.1016/S2542-5196(23)00053-0
39. Faraji Ghasemi, F , Dobaradaran, S , Saeedi, R , Nabipour, I , Nazmara, S , Ranjbar Vakil Abadi, D, et al. Levels and ecological and health risk assessment of PM 2.5-bound heavy metals in the northern part of the Persian Gulf. Environ Sci Pollut Res. (2020) 27:5305–13. doi: 10.1007/s11356-019-07272-7
40. Moradi, M , Mokhtari, A , Mohammadi, MJ , Hadei, M , and Vosoughi, M . Estimation of long-term and short-term health effects attributed to PM 2.5 standard pollutants in the air of Ardabil (using air Q+ model). Environ Sci Pollut Res. (2022) 29:21508–16. doi: 10.1007/s11356-021-17303-x
41. Momtazan, M , Geravandi, S , Rastegarimehr, B , Valipour, A , Ranjbarzadeh, A , Yari, AR, et al. An investigation of particulate matter and relevant cardiovascular risks in Abadan and Khorramshahr in 2014–2016. Toxin Rev. (2018) 38:290–7. doi: 10.1080/15569543.2018.1463266
42. Khaefi, M , Geravandi, S , Hassani, G , Yari, AR , Soltani, F , Dobaradaran, S, et al. Association of particulate matter impact on prevalence of chronic obstructive pulmonary disease in Ahvaz, Southwest Iran during 2009-2013. Aerosol Air Qual Res. (2017) 17:230–7. doi: 10.4209/aaqr.2015.11.0628
43. Tahery, N , Zarea, K , Cheraghi, M , Hatamzadeh, N , Farhadi, M , Dobaradarn, S, et al. Chronic obstructive pulmonary disease (COPD) and air pollution: a review. Jundishapur J Chronic Dis Care. (2021) 10:10. doi: 10.5812/jjcdc.110273
44. Effatpanah, M , Effatpanah, H , Jalali, S , Parseh, I , Goudarzi, G , Barzegar, G, et al. Hospital admission of exposure to air pollution in Ahvaz megacity during 2010–2013. Clin Epidemiol Global Health. (2020) 8:550–6. doi: 10.1016/j.cegh.2019.12.001
45. Dastoorpoor, M , Sekhavatpour, Z , Masoumi, K , Mohammadi, MJ , Aghababaeian, H , Khanjani, N, et al. Air pollution and hospital admissions for cardiovascular diseases in Ahvaz, Iran. Sci Total Environ. (2019) 652:1318–30. doi: 10.1016/j.scitotenv.2018.10.285
46. Idani, E , Geravandi, S , Akhzari, M , Goudarzi, G , Alavi, N , Yari, AR, et al. Characteristics, sources, and health risks of atmospheric PM10-bound heavy metals in a populated middle eastern city. Toxin Rev. (2020) 39:266–74. doi: 10.1080/15569543.2018.1513034
47. Kioumourtzoglou, M-A , Schwartz, JD , Weisskopf, MG, et al. Long-term PM2.5 exposure and neurological hospital admissions in the northeastern United States. Environ Health Perspect. (2016) 124:23–9. doi: 10.1289/ehp.1408973
48. Brook, RD , Rajagopalan, S , Pope, CA III, Brook, JR , Bhatnagar, A , Diez-Roux, AV, et al. Particulate matter air pollution and cardiovascular disease: an update to the scientific statement from the American Heart Association. Circulation. (2010) 121:2331–78. doi: 10.1161/CIR.0b013e3181dbece1
49. Zang, S-T , Luan, J , Li, L , Wu, QJ , Chang, Q , Dai, HX, et al. Air pollution and metabolic syndrome risk: evidence from nine observational studies. Environ Res. (2021) 202:111546. doi: 10.1016/j.envres.2021.111546
50. Woo, KS , Kwok, TCY , Chook, P , Hu, YJ , Yin, YH , Lin, C, et al. Independent effects of metabolic syndrome and air pollution (PM2. 5) on atherosclerosis in modernizing China. Austin J Public Health Epidemiol. (2021) doi: 10.26420/austinjpublichealthepidemiol.2021.1097
51. Sun, K , Liu, J , and Ning, G . Active smoking and risk of metabolic syndrome: a meta-analysis of prospective studies. PLoS One. (2012) 7:e47791. doi: 10.1371/journal.pone.0047791
52. Ning, J , Zhang, Y , Hu, H , Hu, W , Li, L , Pang, Y, et al. Association between ambient particulate matter exposure and metabolic syndrome risk: a systematic review and meta-analysis. Sci Total Environ. (2021) 782:146855. doi: 10.1016/j.scitotenv.2021.146855
53. Guo, Q , Zhao, Y , Xue, T , Zhang, J , and Duan, X . Association of PM2.5 and its chemical compositions with metabolic syndrome: a nationwide study in middle-aged and older Chinese adults. Int J Environ Res Public Health. (2022) 19:14671. doi: 10.3390/ijerph192214671
54. Zheng, X-y , Tang, S-l , Liu, T , Wang, Y , and Xu, XJ . Effects of long-term PM2.5 exposure on metabolic syndrome among adults and elderly in Guangdong, China. Environ Health. (2022) 21:1–11. doi: 10.1186/s12940-022-00888-2
55. Shamy, M , Alghamdi, M , Khoder, MI , Mohorjy, A , Alkhatim, A , Alkhalaf, A, et al. Association between exposure to ambient air particulates and metabolic syndrome components in a Saudi Arabian population. Int J Environ Res Public Health. (2018) 15:27. doi: 10.3390/ijerph15010027
56. Lee, S , Park, H , Kim, S , Lee, EK , Lee, J , Hong, YS, et al. Fine particulate matter and incidence of metabolic syndrome in non-CVD patients: a nationwide population-based cohort study. Int J Hyg Environ Health. (2019) 222:533–40. doi: 10.1016/j.ijheh.2019.01.010
57. Matthiessen, C , Lucht, S , Hennig, F , Ohlwein, S , Jakobs, H , Jöckel, KH, et al. Long-term exposure to airborne particulate matter and NO2 and prevalent and incident metabolic syndrome–results from the Heinz Nixdorf recall study. Environ Int. (2018) 116:74–82. doi: 10.1016/j.envint.2018.02.035
58. Sheppard, L , Burnett, RT , Szpiro, AA , Kim, SY , Jerrett, M , Pope, CA III, et al. Confounding and exposure measurement error in air pollution epidemiology. Air Qual Atmos Health. (2012) 5:203–16. doi: 10.1007/s11869-011-0140-9
59. Turner, MC , Cohen, A , Burnett, RT , Jerrett, M , Diver, WR , Gapstur, SM, et al. Interactions between cigarette smoking and ambient PM2. 5 for cardiovascular mortality. Environ Res. (2017) 154:304–10. doi: 10.1016/j.envres.2017.01.024
60. Chen, Q , Ma, X , Geng, Y , Liao, J , and Ma, L . Association between smoking and hypertension under different PM2.5 and green space exposure: a nationwide cross-sectional study. Front Public Health. (2022) 10:1026648. doi: 10.3389/fpubh.2022.1026648
61. Purpose of Taiwan BioBank 2014 (2023). Available at: https://taiwanview.twbiobank.org.tw/index.)
62. Feng, Y-CA , Chen, C-Y , Chen, T-T , Kuo, PH , Hsu, YH , Yang, HI, et al. Taiwan biobank: a rich biomedical research database of the Taiwanese population. Cell. Genomics. (2022) 2:100197. doi: 10.1016/j.xgen.2022.100197
63. Fan, C-T , Lin, J-C , and Lee, C-H . Taiwan biobank: a project aiming to aid Taiwan’s transition into a biomedical island. Pharmacogenomics. (2008) 9:235–46. doi: 10.2217/14622416.9.2.235
64. Wong, P-Y , Su, H-J , Lee, H-Y , Chen, YC , Hsiao, YP , Huang, JW, et al. Using land-use machine learning models to estimate daily NO2 concentration variations in Taiwan. J Clean Prod. (2021) 317:128411. doi: 10.1016/j.jclepro.2021.128411
65. Slagter, SN , van Vliet-Ostaptchouk, JV , Vonk, JM , Boezen, HM , Dullaart, RPF , Kobold, ACM, et al. Associations between smoking, components of metabolic syndrome and lipoprotein particle size. BMC Med. (2013) 11:1–15. doi: 10.1186/1741-7015-11-195
66. Geslain-Biquez, C , Vol, S , Tichet, J , Caradec, A , D'Hour, A , and Balkau, B . The metabolic syndrome in smokers. The D.E.S.I.R. Study. Diabetes Metab. (2003) 29:226–34. doi: 10.1016/S1262-3636(07)70031-9
67. Zhu, S , St-Onge, M-P , Heshka, S , and Heymsfield, SB . Lifestyle behaviors associated with lower risk of having the metabolic syndrome. Metabolism. (2004) 53:1503–11. doi: 10.1016/j.metabol.2004.04.017
68. Mazidi, M , and Speakman, JR . Ambient particulate air pollution (PM2.5) is associated with the ratio of type 2 diabetes to obesity. Sci Rep. (2017) 7:9144. doi: 10.1038/s41598-017-08287-1
69. Ma, R , Zhang, Y , Sun, Z , Xu, D , and Li, T . Effects of ambient particulate matter on fasting blood glucose: a systematic review and meta-analysis. Environ Pollut. (2020) 258:113589. doi: 10.1016/j.envpol.2019.113589
70. Wallwork, RS , Colicino, E , Zhong, J , Kloog, I , Coull, BA , Vokonas, P, et al. Ambient fine particulate matter, outdoor temperature, and risk of metabolic syndrome. Am J Epidemiol. (2017) 185:30–9. doi: 10.1093/aje/kww157
71. Yang, B-Y , Qian, Z , Howard, SW , Vaughn, MG , Fan, SJ , Liu, KK, et al. Global association between ambient air pollution and blood pressure: a systematic review and meta-analysis. Environ Pollut. (2018) 235:576–88. doi: 10.1016/j.envpol.2018.01.001
72. Bravo, MA , Fang, F , Hancock, DB , Johnson, EO , and Harris, KM . Long-term air pollution exposure and markers of cardiometabolic health in the National Longitudinal Study of adolescent to adult health (add health). Environ Int. (2023) 177:107987. doi: 10.1016/j.envint.2023.107987
73. Fiorito, G , Vlaanderen, J , Polidoro, S , Gulliver, J , Galassi, C , Ranzi, A, et al. Oxidative stress and inflammation mediate the effect of air pollution on cardio-and cerebrovascular disease: a prospective study in nonsmokers. Environ Mol Mutagen. (2018) 59:234–46. doi: 10.1002/em.22153
74. Turner, MC , Cohen, A , Jerrett, M , Gapstur, SM , Diver, WR , Pope, CA III, et al. Interactions between cigarette smoking and fine particulate matter in the risk of lung cancer mortality in Cancer prevention study II. Am J Epidemiol. (2014) 180:1145–9. doi: 10.1093/aje/kwu275
75. Puett, RC , Schwartz, J , Hart, JE , Yanosky, JD , Speizer, FE , Suh, H, et al. Chronic particulate exposure, mortality, and coronary heart disease in the nurses’ health study. Am J Epidemiol. (2008) 168:1161–8. doi: 10.1093/aje/kwn232
76. Lepeule, J , Laden, F , Dockery, D , and Schwartz, J . Chronic exposure to fine particles and mortality: an extended follow-up of the Harvard six cities study from 1974 to 2009. Environ Health Perspect. (2012) 120:965–70. doi: 10.1289/ehp.1104660
77. Rönnemaa, T , Rönnemaa, EM , Puukka, P , Pyörälä, K , and Laakso, M . Smoking is independently associated with high plasma insulin levels in nondiabetic men. Diabetes Care. (1996) 19:1229–32. doi: 10.2337/diacare.19.11.1229
78. Akter, S , Goto, A , and Mizoue, T . Smoking and the risk of type 2 diabetes in Japan: a systematic review and meta-analysis. J Epidemiol. (2017) 27:553–61. doi: 10.1016/j.je.2016.12.017
79. Haberzettl, P , O’Toole, TE , Bhatnagar, A , and Conklin, DJ . Exposure to fine particulate air pollution causes vascular insulin resistance by inducing pulmonary oxidative stress. Environ Health Perspect. (2016) 124:1830–9. doi: 10.1289/EHP212
80. Miller, MR . The role of oxidative stress in the cardiovascular actions of particulate air pollution. Biochem Soc Trans. (2014) 42:1006–11. doi: 10.1042/BST20140090
81. Wolf, K , Popp, A , Schneider, A , Breitner, S , Hampel, R , Rathmann, W, et al. Association between long-term exposure to air pollution and biomarkers related to insulin resistance, subclinical inflammation, and adipokines. Diabetes. (2016) 65:3314–26. doi: 10.2337/db15-1567
82. Xu, M-X , Ge, C-X , Qin, Y-T, et al. 5 exposure elevates risk of oxidative stress-driven nonalcoholic fatty liver disease by triggering increase of dyslipidemia. Free Radic Biol Med. (2019) 130:542–56. doi: 10.1016/j.freeradbiomed.2018.11.016
Keywords: cigarette smoking, PM2.5, interaction, adults, Taiwan
Citation: Tsai H-H, Tantoh DM, Lu WY, Chen C-Y and Liaw Y-P (2024) Cigarette smoking and PM2.5 might jointly exacerbate the risk of metabolic syndrome. Front. Public Health. 11:1234799. doi: 10.3389/fpubh.2023.1234799
Edited by:
Ghulam Mujtaba Kayani, Hubei University of Economics, ChinaReviewed by:
Yang Wang, Yunnan Normal University, ChinaMohammad Javad Mohammadi, Ahvaz Jundishapur University of Medical Sciences, Iran
Feng Hong, Guizhou Medical University, China
Copyright © 2024 Tsai, Tantoh, Lu, Chen and Liaw. This is an open-access article distributed under the terms of the Creative Commons Attribution License (CC BY). The use, distribution or reproduction in other forums is permitted, provided the original author(s) and the copyright owner(s) are credited and that the original publication in this journal is cited, in accordance with accepted academic practice. No use, distribution or reproduction is permitted which does not comply with these terms.
*Correspondence: Yung-Po Liaw, TGlhd3lwQGNzbXUuZWR1LnR3; Chih-Yi Chen, Q3NoeTE1NjZAY3NoLm9yZy50dw==