- 1School of Public Health, The Second Affiliated Hospital, Zhejiang University School of Medicine, Hangzhou, Zhejiang, China
- 2The Key Laboratory of Intelligent Preventive Medicine of Zhejiang Province, Hangzhou, Zhejiang, China
- 3School of Public Health, Faculty of Medicine, The University of Queensland, Brisbane, QLD, Australia
Background: The role of certain biomarkers in the development of single cardiometabolic disease (CMD) has been intensively investigated. Less is known about the association of biomarkers with multiple CMDs (cardiometabolic multimorbidity, CMM), which is essential for the exploration of molecular targets for the prevention and treatment of CMM. We aimed to systematically synthesize the current evidence on CMM-related biomarkers.
Methods: We searched PubMed, Embase, Web of Science, and Ebsco for relevant studies from inception until August 31st, 2022. Studies reported the association of serum/plasma biomarkers with CMM, and relevant effect sizes were included. The outcomes were five progression patterns of CMM: (1) no CMD to CMM; (2) type 2 diabetes mellitus (T2DM) followed by stroke; (3) T2DM followed by coronary heart disease (CHD); (4) T2DM followed by stroke or CHD; and (5) CHD followed by T2DM. Newcastle-Ottawa Quality Assessment Scale (NOS) was used to assess the quality of the included studies. A meta-analysis was conducted to quantify the association of biomarkers and CMM.
Results: A total of 68 biomarkers were identified from 42 studies, which could be categorized into five groups: lipid metabolism, glycometabolism, liver function, immunity, and others. Lipid metabolism biomarkers were most reported to associate with CMM, including TC, TGs, HDL-C, LDL-C, and Lp(a). Fasting plasma glucose was also reported by several studies, and it was particularly associated with coexisting T2DM with vascular diseases. According to the quantitative meta-analysis, HDL-C was negatively associated with CHD risk among patients with T2DM (pooled OR for per 1 mmol/L increase = 0.79, 95% CI = 0.77–0.82), whereas a higher TGs level (pooled OR for higher than 150 mg/dL = 1.39, 95% CI = 1.10–1.75) was positively associated with CHD risk among female patients with T2DM.
Conclusion: Certain serum/plasma biomarkers were associated with the progression of CMM, in particular for those related to lipid metabolism, but heterogeneity and inconsistent findings still existed among included studies. There is a need for future research to explore more relevant biomarkers associated with the occurrence and progression of CMM, targeted at which is important for the early identification and prevention of CMM.
1 Introduction
With the growth of the aging population, non-communicable diseases (NCDs) have become the major global disease burden and the leading cause of death worldwide (1). Some NCDs may share similar pathogenesis or identical risk factors (2, 3), leading to their simultaneous coexistence in individuals and resulting in multimorbidity (i.e., the coexistence of two or more NCDs) (4). Cardiometabolic multimorbidity (CMM) is one of the most studied patterns of multimorbidity (5), defined as the co-occurrence of two or more cardiometabolic diseases (CMDs), including coronary heart disease (CHD), stroke, and type 2 diabetes mellitus (T2DM) (2, 6, 7). The prevalence of CMM has increased rapidly in the past few decades (8), potentially resulting in worse quality of life, excess morbidity, and mortality (8–11).
Current studies on the risk factors of CMM mainly focused on some macro or external factors, such as lifestyle factors (12), dietary factors (6, 13), and environmental factors (14). Limited studies have explored the role of micro risk factors, such as serum/plasma biomarkers, on CMM. Biomarkers are the most objective and quantifiable medical markers that can be measured and are commonly used as clinical and diagnostic tools (15). Changes in biomarker levels can reflect the interaction effects among genetic factors, lifestyle factors, environmental factors, and health conditions, which can be used to explore new routes of disease occurrence, improve the accuracy of risk prediction, and achieve stratifying prevention and management of these diseases (16, 17). The relationship between specific biomarkers and single CMD was extensively investigated. For example, high levels of triglycerides (TGs), low-density lipoprotein cholesterol (LDL-C) and fasting plasma glucose (FPG), low levels of high-density lipoprotein cholesterol (HDL-C) have been reported to be risk factors of cardiovascular complications in T2DM patients (18–20). A 12-year follow-up longitudinal analysis revealed that high homocysteine (tHcy) and low Methionine (Met) levels were associated with a higher risk of cardiovascular multimorbidity in older age (21). However, there remains a lack of studies investigating the relationship between biomarkers and CMM. Moreover, no systematic review and meta-analysis has yet synthesized existing evidence on the association between biomarkers and the progression of CMM, which is critical for the clinical practice of identifying high-risk populations early via laboratory blood testing. We aimed to synthesize the available scattered evidence on the role of serum/plasma biomarkers in the development and progression of CMM.
2 Methods
2.1 Search strategy
This systematic review was conducted according to the Preferred Reporting Items for Systematic Reviews and Meta-Analyses (PRISMA) guidelines (22). Databases, including PubMed, Embase, Web of Science, and Ebsco, were searched for eligible articles published in English from January 1st, 1900, to August 31st, 2022. Details of search strategies are presented in Supplementary Table S1. Reference lists and Google were also searched to identify any additional or gray literature that met the inclusion criteria.
2.2 Selection criteria
After removing the duplicate records, three authors (JY, XZ, ZY) independently screened a third of the total records for titles and abstracts and evaluated the full text to select eligible articles with exclusion reasons recorded. A total of 5% of the records were randomly selected and cross-checked for verification. Inter-examiner agreements across the three authors were calculated using Cohen κ statistics, with ranges of 0.01–0.20 representing slight agreement, 0.21–0.40 representing fair agreement, 0.41–0.60 representing moderate agreement, 0.61–0.80 representing substantial agreement, and 0.81–0.99 representing almost perfect agreement (23). Discrepancies were discussed with a fourth author (XX) in regular group meetings.
Inclusion criteria were: (1) observational studies such as cohort studies and nested case-control studies; (2) the primary outcome of the study was CMM, which was defined as the co-occurrence of at least two of the following CMDs: CHD, T2DM, and stroke. Myocardial infarction (MI), ischemic heart disease (IHD), angina pectoris, coronary artery bypass grafting, percutaneous transluminal coronary angioplasty, coronary revascularization procedures, and CHD-related death are all examples of CHD occurrences; (3) biomarkers include all detectable and quantifiable biochemical parameters found in plasma or serum, except for gene regulatory molecules such as microRNA. Laboratory examinations were conducted at baseline or before the occurrence of CMM; (4) participants were free of CMM at baseline; (5) effect sizes [e.g., hazard ratio (HR), odds ratio (OR), relative risk (RR), and 95% confidence interval (CI)] of the biomarkers on CMM were reported; and (6) written in English.
2.3 Quality assessment and data extraction
Three authors (JY, XZ, ZY) independently extracted data from all included articles using a pre-designed standardized data extraction form, including title, first author, year of publication, country, sex distribution and age of participants, sample size, follow-up duration, biomarkers, definition of CMM, number (percentage) of participants developing CMM, comparison, adjusted effect sizes of biomarkers on CMM, and covariates. Disagreements on the extraction were discussed with a fourth author (XX).
Three authors independently (JY, XZ, ZY) assessed the methodological quality of each study using Newcastle-Ottawa Quality Assessment Scale (NOS) (24), with discrepancies discussed with a fourth author (XX). Studies scored ≥7 (out of 9) were considered as high quality, and those scored ≤ 3 were of low quality (25).
2.4 Evidence synthesis
A narrative synthesis approach was used to summarize the effect sizes of specific biomarkers on different progression patterns of CMM, which was visualized through a heatmap. However, due to the heterogeneity in the cut-off points or measurement units of biomarkers among included articles, we could not pool the effect sizes of the majority of biomarkers on CMM through meta-analysis. For eligible biomarkers with enough data for meta-analysis, different measurement levels of specific biomarkers were converted into a consistent standard. Categorical variables were converted into binary forms, while effect sizes of continuous variables were transformed into ORs according to the following formulas (26):
The heterogeneity was assessed using Cochran's Q-statistic and I2 statistics, with thresholds of 25%, 50%, and 75% for low, moderate, and high heterogeneity (23). Depending on the degree of heterogeneity, either a fixed effect model or a random effect model was used to estimate the pooled ORs (95% CIs). We weighted studies using the inverse-variance approach. The meta-analysis was conducted using Review Manager (RevMan) Version 5.4. (Copenhagen, Denmark: The Nordic Cochrane Center, The Cochrane Collaboration).
3 Results
3.1 Characteristics of included studies
A total of 79,207 publications were initially identified through a database search. After screening for titles and abstracts, 1,633 were selected for full-text review (inter-reader agreement κ = 0.81/0.85/0.83). A total of 42 studies (7, 27–67) that met the inclusion criteria were finally included in our review after the full-text screen (inter-reader agreement κ=0.86/0.88/0.82). The selection process is shown in Figure 1, and the basic characteristics of included studies are presented in Supplementary Table S2. Among the 42 studies included in our review, we selected five of them for the meta-analysis (40, 43, 48, 49, 62) (Table 1), which were prospective studies focusing on the associations of FPG, LDL-C, HDL-C, and TGs with the progression of CMM among T2DM patients based on populations from Japan, Greece, and Italy. These 42 studies were grouped into five categories by different progression patterns of CMM: (1) participants were free of CMD at baseline and developed CMM during follow-up (n = 3); (2) T2DM followed by stroke (n = 15); (3) T2DM followed by CHD (n = 24); (4) T2DM followed by stroke or CHD (n = 12); and (5) CHD followed by T2DM (n = 3).
A total of 8 studies were from the USA, followed by Japan (n = 7) and the UK (n = 6). The sample size varied considerably among included studies, ranging from 224 to 891,095 participants. The mean age of participants ranged from 46.9 to 72.5 years across included studies. Most studies were of high quality (n = 41), and one study was of moderate quality (Supplementary Figure S1A). The items “Ascertainment of exposure” and “Demonstration that outcome of interest was not present at start of study” were rated as low risk of bias in all included studies, and the item “Adequacy of follow up of cohorts” was rated as high risk in the most of studies (n = 28) (Supplementary Figure S1B).
3.2 Overview of biomarkers
Among the included studies, a total of 68 serum/plasma biomarkers were identified (Figure 2). They are categorized into five groups: lipid metabolism, glycometabolism, liver function, immunity, and others. The most frequently studied biomarkers were HDL-C (n = 19), TGs (n = 18), FPG (n = 15), LDL-C (n = 15), and lipoprotein-A [Lp(a)] (n = 7). Effects of some biomarkers reported in more than two studies were also presented according to races (Supplementary Figure S2). The directions of the association for most biomarkers with CMM were similar among different populations, except for HDL-C, which showed positive association with CMM in Chinese populations and negative association in Japanese. Four biomarkers (i.e., FPG, HDL-C, LDL-C, and TGs) were available for meta-analysis.
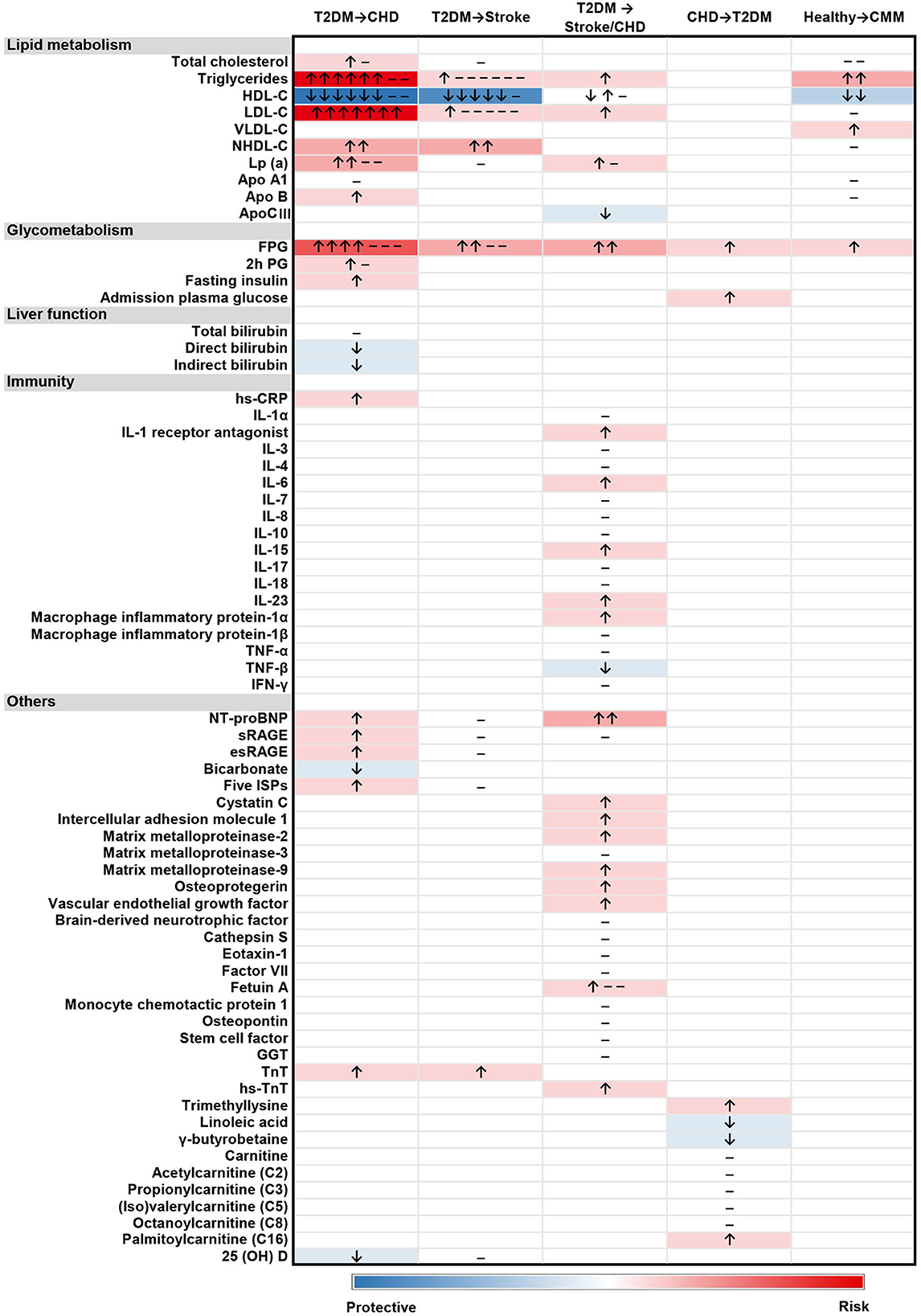
Figure 2. Heatmap of the associations between 68 biomarkers and 5 types of CMM progression. “↑”, positive association; “↓”, negative association; “–”, no significant association. The studies reporting different associations were counted and presented in the heatmap. The intensity of the color depended on the strength of overall association between biomarker level and the outcome.
3.3 The role of biomarkers in different progression patterns of CMM
3.3.1 Healthy participants progressed to CMM
Three studies (7, 66, 67) reported the association of lipid metabolism related biomarkers with the progression of CMM, including total cholesterol (TC), TGs, HDL-C, LDL-C, VLDL-C, NHDL-C, Apo A1, and ApoB. According to these studies, a higher level of TGs or VLDL-C was reported to positively associate with an increased risk of CMM, while HDL-C level was reported to inversely associate with CMM. FPG was the only glycometabolism biomarker associated with CMM, and a higher FPG level was positively associated with an increased risk of CMM.
3.3.2 T2DM followed by CHD
A total of 15 biomarkers were reported to be positively associated with a higher risk of CHD among T2DM patients, including six lipid metabolism biomarkers [i.e., TC, TGs, LDL-C, NHDL-C, Lp(a), and Apo B], three glycometabolism biomarkers (i.e., FPG, 2h PG and fasting insulin), one immunity biomarkers (i.e., hs-CRP), and five other biomarkers [i.e., N-terminal pro-B type natriuretic peptide (NT-proBNP), sRAGE, esRAGE, five inflammation-sensitive plasma proteins (ISPs) and TnT]. Five biomarkers were reported to negatively associate with the risk of CHD following T2DM, including HDL-C, direct bilirubin, indirect bilirubin, bicarbonate, and 25(OH)D. Four articles (40, 43, 48, 62) were available for the meta-analyses, which showed no significant associations of FPG (pooled OR for per 10 mg/dL increase = 1.00, 95% CI = 1.00–1.01) with CHD among T2DM patients. A higher level of TGs was positively associated with CHD risk among female T2DM patients (pooled OR for higher than 150 mg/dL among male T2DM patients = 1.80, 95% CI = 0.76–4.22, pooled OR for higher than 150 mg/dL among female T2DM patients = 1.39, 95%CI: = 1.10–1.75). Besides, an inverse association of HDL-C (pooled OR for per 1 mmol/L increase = 0.79, 95% CI = 0.77–0.82) with CHD risk among T2DM patients was found (Figure 3).
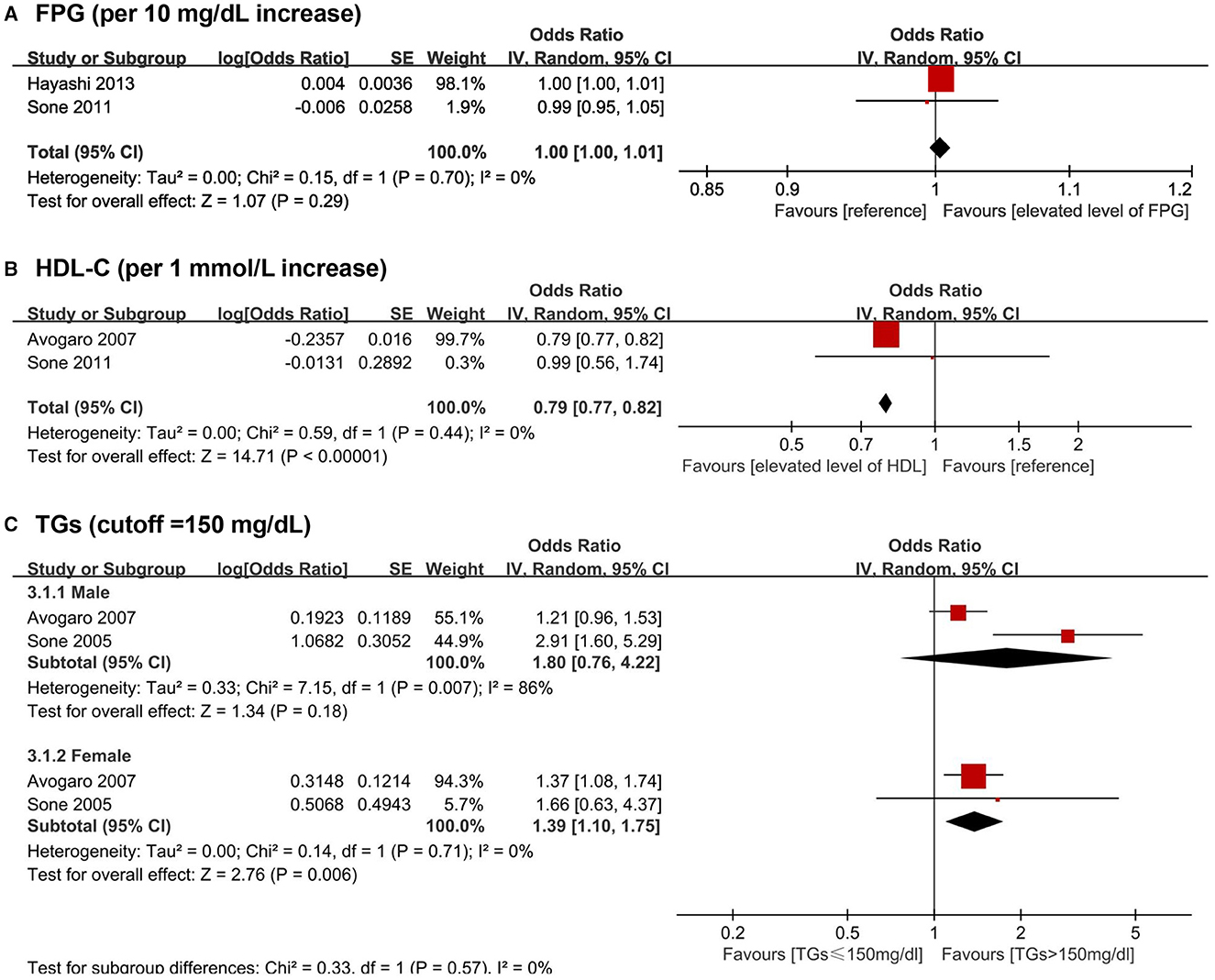
Figure 3. Forest plot of prospective studies examining FPG (A), HDL-C (B), TGs (C) levels and risk of CHD in subjects with T2DM. (A) Pooled analysis of increase risk of CHD in subjects with T2DM for per 10 mg/dL increase of FPG level. (B) Pooled analysis of increase risk of CHD in subjects with T2DM for per 1 mmol/L increase of HDL-C level. (C) 3.1.1 Pooled analysis of increase risk of CHD in male T2DM patients with TGs level higher than 150 mg/dL. (C) 3.1.2 Pooled analysis of increase risk of CHD in female T2DM patients with TGs level higher than 150 mg/dL. CHD, coronary heart disease; CI, confidence interval; FPG, fasting plasma glucose; HDL-C, high-density lipoprotein cholesterol; T2DM, type 2 diabetes mellitus; TGs, triglycerides.
3.3.3 T2DM followed by stroke
A total of five biomarkers were reported to be positively associated with a higher risk of stroke among T2DM patients, including three lipid metabolism biomarkers (i.e., LDL-C, TGs, and NHDL-C), one glycometabolism biomarker (i.e., FPG), and one other biomarker (i.e., TnT). Besides, HDL-C was reported to be inversely associated with the risk of stroke among the population with T2DM. Four articles (43, 48, 49, 62) were available for the meta-analyses: FPG (pooled OR for 10 mg/dL increase = 1.01, 95% CI = 1.00–1.01), LDL-C (pooled OR for 1 mg/dL increase = 1.00, 95% CI = 0.99–1.01), and TGs (pooled OR for higher than 150 mg/dL cutoff = 1.03, 95% CI = 0.92–1.16) showed no significant association with stroke among T2DM patients (Figure 4).
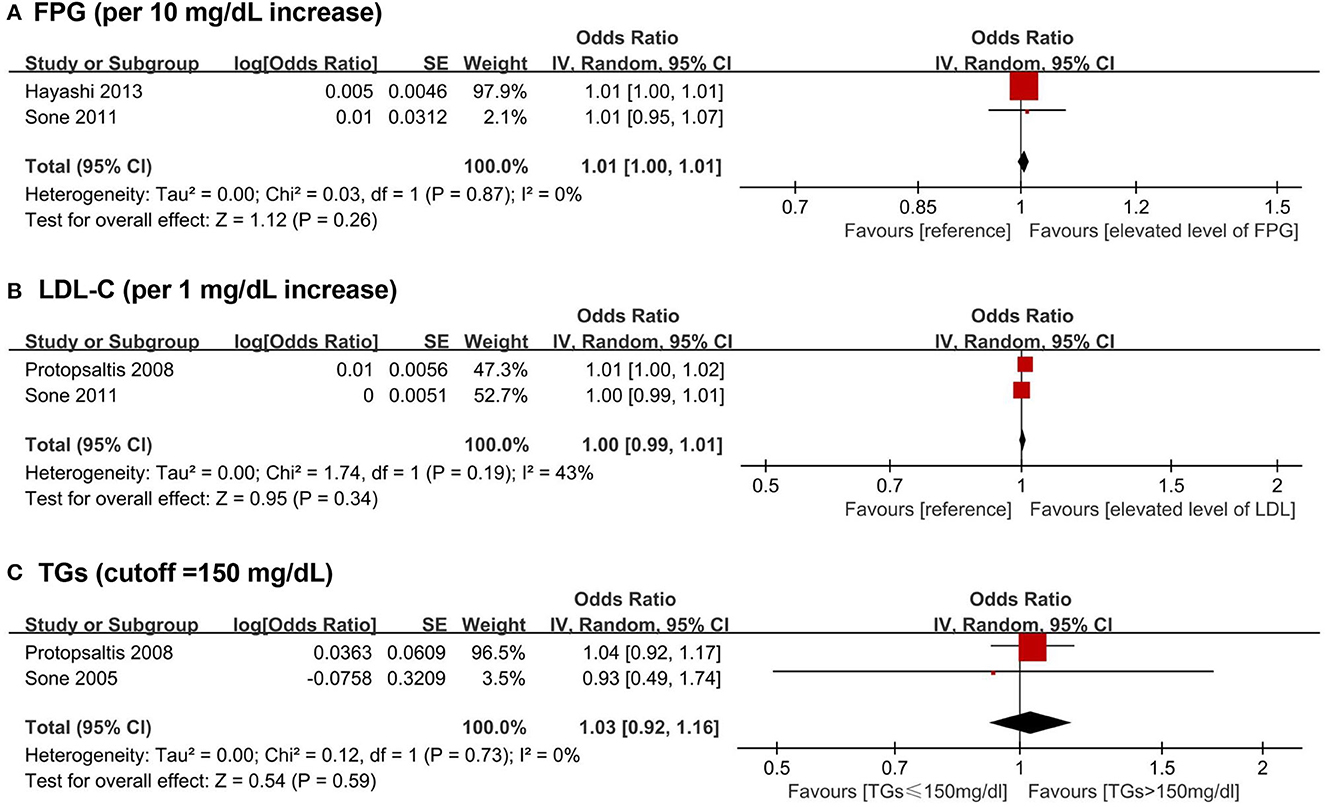
Figure 4. Forest plot of prospective studies examining FPG (A), LDL-C (B), TGs (C) levels and risk of stroke in subjects with T2DM. (A) Pooled analysis of increase risk of stroke in subjects with T2DM for per 10 mg/dL increase of FPG level. (B) Pooled analysis of increase risk of stroke in subjects with T2DM for per 1 mg/dL increase of LDL-C level. (C) Pooled analysis of increase risk of stroke in subjects with T2DM with TGs level higher than 150 mg/dL. CI, confidence interval; FPG, fasting plasma glucose; LDL-C, low-density lipoprotein cholesterol; T2DM, type 2 diabetes mellitus; TGs, triglycerides.
3.3.4 T2DM followed by stroke or CHD
A total of 18 biomarkers were identified to be positively associated with a higher risk of stroke or CHD among T2DM patients, including three lipid metabolism biomarkers (i.e., Lp(a), LDL-C, and TGs), one glycometabolism biomarker (i.e., FPG), five immunity biomarkers (i.e., IL-1 receptor antagonist, IL-6, IL-15, IL-23, and macrophage inflammatory protein-1α) and nine other biomarkers [i.e., NT-proBNP, cystatin C, intercellular adhesion molecule 1 (ICAM-1), matrix metalloproteinase-2 (MMP-2), matrix metalloproteinase-9 (MMP-9), osteoprotegerin (OPG), vascular endothelial growth factor (VEGF), fetuin-A, and hs-TnT]. Besides, two biomarkers were inversely associated with the risk of stroke or CHD in T2DM patients, including apolipoprotein C III (Apo CIII) and tumor necrosis factor-β (TNF-β).
3.3.5 CHD followed by T2DM
Two glycometabolism biomarkers (i.e., FPG and admission plasma glucose) and two additional biomarkers [trimethyllysine and palmitoylcarnitine (C16)] were reported to positively associated with a greater risk of T2DM in CHD patients. In addition, linoleic acid and γ-butyrobetaine were revealed to be inversely related to a greater risk of T2DM among CHD patients.
4 Discussion
4.1 Principle findings
We obtained 68 types of serum/plasma biomarkers from 42 included studies and summarized the evidence of associations between these biomarkers and five progression patterns of CMM. Lipid metabolism biomarkers were most reported to associate with the risk of CMM, including TC, TGs, HDL-C, LDL-C, and Lp(a). Only four biomarkers (FPG, HDL-C, LDL-C, TGs) were available for meta-analysis due to methodological heterogeneity among studies. A higher level of HDL-C was shown to significantly associate with lower risks of CHD among T2DM patients; on the other hand, a higher level of TGs was positively associated with CHD risk among female T2DM patients.
4.2 Comparison with previous studies
Lipid metabolism biomarkers were the most studied type of biomarkers. The meta-analysis showed that HDL-C was negative associated with CHD risk among patients with T2DM, and a higher TGs level was positively associated with CHD risk among female patients with T2DM.
Among all included studies, our heatmap suggested that elevated levels of TGs, LDL-C, NHDL-C, and Lp(a) were associated with a higher risk of CMM, while HDL-C presented a significant inverse association with the progression of CMM. In line with our findings, strong associations between lipid metabolism biomarkers (e.g., elevated levels of LDL-C, TGs and Lp(a), decreased level of HDL-C) and CMM have been found, especially in patients with T2DM (18, 19, 68–70). Also, it is worth noting that T2DM patients with high HDL-cholesterol levels had paradoxically higher risk of composite CVD outcomes in an included study (33). Previous studies (71, 72) also suggested that the association between HDL-C concentrations and CVD events might be a U-shaped curve, indicating that abnormally low or high HDL-C levels were both inversely associated with health status. However, due to the limited number and heterogeneity of included studies, we could not identify such an association in our analysis. There also existed sex differences in the effect of lipid metabolism biomarkers on the risk of coronary heart disease in patients with T2DM. One included study (40) reported the risk effect of TGs, and the protective effect of HDL-C on CHD only existed in female participants. Another study (43) showed a significant association between high levels of LDL-C and CHD outcome only in males. It can be explained that women with T2DM were more susceptible to the atherogenic effects of non-LDL-C factors (i.e., high level of TGs and low level of HDL-C) (73, 74). Future studies are warranted to validate such associations.
Several glycometabolism biomarkers were also reported to associate with CMM, especially for FPG. Hyperglycemia and uncontrolled glycemia have been extensively discussed as risk factors for the development of stroke and CHD in previous studies (20, 75, 76) in both the general population and T2DM patients. Hyperglycemia can detrimentally affect normal endothelial function, contributing to plaque formation and rupture, and finally, thrombosis (76, 77), thus correlating with a higher risk of atherosclerotic cardiovascular diseases (ASCVD). In addition, a J-shaped relationship between FPG and adverse cardiovascular events has been reported in people with T2DM (78, 79), especially in older patients with high comorbidity load, consistent with our results (54, 63). As a traditional diagnostic biomarker for T2DM, the level of FPG may provide more information for health status that could also serve as an indicator of the deterioration of T2DM and a potential biomarker for CMM.
Several inflammatory factors were also identified to associate with CMM in our study. In addition to what we found, many previous studies reported that an elevated concentration of inflammatory factors (e.g., IL-6, hs-CRP, TNF-α) were positively correlated with ASCVD outcomes (80–82) due to their effects on plaque development and rupture, endothelial dysfunction, and coronary thrombosis (82). However, the role of some other immunity factors in the progression of CVD is controversial, and there remains a lack of evidence to assess the association between immunity biomarkers and the development of CMM.
The majority of the immunity biomarkers in our research were collected from one study (57), which intended to choose predictive biomarkers for CVD in T2DM patients. More research is needed to determine the relevance of immunity biomarkers as potential indications of CMM progression.
Liver function related biomarkers were also crucial in the progression of CMM, but current findings of their impacts on CMM were scarce and inconsistent. For example, bilirubin, an essential marker of liver function, has been indicated as an antioxidant with anti-inflammatory and antiapoptotic effects (83–85). One included study (60) revealed that higher levels of serum direct and indirect bilirubin were related to decreased CHD risk in T2DM patients. But the evidence from prospective studies was limited. Another study (86) suggested that serum bilirubin could add predictive value to future cardiovascular deaths in patients with T2DM. Besides, NT-proBNP was widely used in detecting heart failure and was also a prognostic marker in patients with acute decompensated heart failure (87, 88). Another review (89) regarded NT-proBNP as a predictor of CVD events in T2DM patients, which was consistent with our findings.
Many included studies recruited patients with one initial CMD at baseline and most had T2DM. It has been proved that hypertension and dyslipidemia were quite common in patients with T2DM, even in prediabetic individuals (90–92). Many studies reported using current diabetes treatments, antihypertensive therapy, and lipid-lowering treatments at baseline, which could interfere with the biomarker measurements and the association between biomarkers and CMD (93, 94). Several studies addressed the influence of medication and incorporated it into the multivariate model. Some studies compared the risk effect before and after further adjustment for treatment and observed similar results. However, the impact of treatment and drugs was not accounted for in some studies due to the complexity of potential interactions. The role of medication in the association between biomarkers and CMM requires further investigation to prevent the development of CMM in people who already have one basis disease.
Lifestyle and diet habits are also important factors associated with certain biomarkers levels and CMM progression according to previous studies (6, 12, 95–97). Many included studies adjusted alcohol intake and physical activity as covariates in the multivariate analysis. However, few of them estimated the effect sizes of these factors with biomarkers or further discuss other lifestyle or diet factors. The interaction between lifestyle, diet habits, biomarkers, and CMM awaits more investigations and explorations in the future.
4.3 Implications
For future research, there remains a lack of observational studies, especially longitudinal cohort studies, to provide more evidence on the associations between biomarkers and the progression of CMM. Evidence on individuals from an apparently healthy state to CMM and various CMM patterns is needed since the majority of included studies in our review focused on populations with T2DM at baseline. Also, the role and effect of medications, treatment, lifestyle, and diet factors in the association between biomarkers and cardiometabolic diseases await more evidence and further exploration. Furthermore, it is also worth exploring the variation of biomarkers over time in the progression of CMM. Finally, a few included studies reported the joint assessments of multiple biomarkers, but the prediction performance of multiple biomarkers in comparison with a single biomarker still needs more evidence.
Our results highlight the value of serum/plasma biomarkers in the primary prevention of CMM among healthy people and secondary prevention in people who already have CMD. Lowering levels of risk biomarkers could be considered potential preventive targets of lifestyle and therapeutic interventions. Our finding may also provide important clinical implications for the early screening and prediction of CMM through targeted biomarkers. Measurements of serum biomarkers in the general population may help to identify individuals at high risk and maximize healthcare resources.
4.4 Limitations
Some limitations of our current review should be addressed. First, because of the limited number of available studies, several biomarkers were only reported once or twice, suggesting that there may be publication bias. Additionally, most included studies were conducted in specific regions or countries, recruiting participants of specific race. Previous studies have shown the various clinical impacts of some metabolic characteristics [e.g., metabolic syndrome (43), serum triglyceride levels (62)] on diabetes across different races. The results could not be fully generalized to the overall population. Third, although many studies included potential confounders in the multivariate models, the adjustments differed in the originally included studies, and some studies' confounders were unavailable. Finally, as mentioned above, integrative analysis of some biomarkers failed due to methodological heterogeneity across studies, which may lead to unconvincing results of the meta-analysis.
5 Conclusion
Our systematic review and meta-analysis summarized the evidence on the role of a broad number of biomarkers in the development and progression of CMM. However, studies focusing on the association of biomarkers and CMM were scarce, requesting more evidence on this topic to provide implications for early prevention, detection, and intervention of CMM.
Data availability statement
The original contributions presented in the study are included in the article/Supplementary material, further inquiries can be directed to the corresponding author.
Author contributions
XX: Conceptualization, Methodology, Project administration, Supervision, Validation, Writing—review & editing. YJ: Data curation, Formal analysis, Investigation, Methodology, Validation, Visualization, Writing—original draft. ZX: Data curation, Formal analysis, Investigation, Methodology, Validation, Writing—original draft. YutZ: Data curation, Investigation, Methodology, Validation, Writing—original draft. YueZ: Writing—review & editing. DW: Methodology, Visualization, Writing—review & editing. YC: Writing—review & editing. YZho: Writing—review & editing. MF: Writing—review & editing.
Funding
The author(s) declare that no financial support was received for the research, authorship, and/or publication of this article.
Acknowledgments
We would like to thank the study authors who provided information about their studies, enabling the synthesis of evidence.
Conflict of interest
The authors declare that the research was conducted in the absence of any commercial or financial relationships that could be construed as a potential conflict of interest.
Publisher's note
All claims expressed in this article are solely those of the authors and do not necessarily represent those of their affiliated organizations, or those of the publisher, the editors and the reviewers. Any product that may be evaluated in this article, or claim that may be made by its manufacturer, is not guaranteed or endorsed by the publisher.
Supplementary material
The Supplementary Material for this article can be found online at: https://www.frontiersin.org/articles/10.3389/fpubh.2023.1280185/full#supplementary-material
References
1. GBD 2016 Causes of Death Collaborators. Global, regional, and national age-sex specific mortality for 264 causes of death, 1980-2016: a systematic analysis for the global burden of disease study 2016. Lancet. (2017) 390:1151–210. doi: 10.1016/S0140-6736(17)32152-9
2. Xu X, Mishra GD, Dobson AJ, Jones M. Progression of diabetes, heart disease, and stroke multimorbidity in middle-aged women: a 20-year cohort study. PLoS Med. (2018) 15:e1002516. doi: 10.1371/journal.pmed.1002516
3. Martín-Timón I, Sevillano-Collantes C, Segura-Galindo A, Del Cañizo-Gómez FJ. Type 2 diabetes and cardiovascular disease: have all risk factors the same strength? World J Diabetes. (2014) 5:444–70. doi: 10.4239/wjd.v5.i4.444
4. Xu X, Mishra GD, Jones M. Evidence on multimorbidity from definition to intervention: an overview of systematic reviews. Ageing Res Rev. (2017) 37:53–68. doi: 10.1016/j.arr.2017.05.003
5. Busija L, Lim K, Szoeke C, Sanders KM, McCabe MP. Do replicable profiles of multimorbidity exist? Systematic review and synthesis. Eur J Epidemiol. (2019) 34:1025–53. doi: 10.1007/s10654-019-00568-5
6. Jeong D, Kim J, Lee H, Kim DY, Lim H. Association of cardiometabolic multimorbidity pattern with dietary factors among adults in South Korea. Nutrients. (2020) 12:2730. doi: 10.3390/nu12092730
7. Singh-Manoux A, Fayosse A, Sabia S, Tabak A, Shipley M, Dugravot A, et al. Clinical, socioeconomic, and behavioural factors at age 50 years and risk of cardiometabolic multimorbidity and mortality: a cohort study. PLoS Med. (2018) 15:e1002571. doi: 10.1371/journal.pmed.1002571
8. Di Angelantonio E, Kaptoge S, Wormser D, Willeit P, Butterworth AS, Bansal N, et al. Association of cardiometabolic multimorbidity with mortality. JAMA. (2015) 314:52–60. doi: 10.1001/jama.2015.7008
9. Fishbook BN, Brinton CD, Siever J, Klassen TD, Sakakibara BM. Cardiometabolic multimorbidity and activity limitation: a cross-sectional study of adults using the canadian longitudinal study on aging data. Fam Pract. (2022) 39:455–63. doi: 10.1093/fampra/cmab129
10. Zhang D, Tang X, Shen P, Si Y, Liu X, Xu Z, et al. Multimorbidity of cardiometabolic diseases: prevalence and risk for mortality from one million chinese adults in a longitudinal cohort study. BMJ Open. (2019) 9:e024476. doi: 10.1136/bmjopen-2018-024476
11. Lyall DM, Celis-Morales CA, Anderson J, Gill JM, Mackay DF, McIntosh AM, et al. Associations between single and multiple cardiometabolic diseases and cognitive abilities in 474 129 UK biobank participants. Eur Heart J. (2017) 38:577–83. doi: 10.1093/eurheartj/ehw528
12. Han Y, Hu Y, Yu C, Guo Y, Pei P, Yang L, et al. Lifestyle, cardiometabolic disease, and multimorbidity in a prospective chinese study. Eur Heart J. (2021) 42:3374–84. doi: 10.1093/eurheartj/ehab413
13. Luo Y, He L, Ma T, Li J, Bai Y, Cheng X, et al. Associations between consumption of three types of beverages and risk of cardiometabolic multimorbidity in uk biobank participants: a prospective cohort study. BMC Med. (2022) 20:273. doi: 10.1186/s12916-022-02456-4
14. Luo H, Zhang Q, Yu K, Meng X, Kan H, Chen R. Long-term exposure to ambient air pollution is a risk factor for trajectory of cardiometabolic multimorbidity: a prospective study in the UK biobank. EBioMedicine. (2022) 84:104282. doi: 10.1016/j.ebiom.2022.104282
15. Strimbu K, Tavel JA. What are biomarkers? Curr Opin HIV AIDS. (2010) 5:463–6. doi: 10.1097/COH.0b013e32833ed177
16. Aronson JK, Ferner RE. Biomarkers—A general review. Curr Protoc Pharmacol. (2017) 76:9.23.1-9.23.17. doi: 10.1002/cpph.19
17. Dhingra R, Vasan RS. Biomarkers in cardiovascular disease: statistical assessment and section on key novel heart failure biomarkers. Trends Cardiovasc Med. (2017) 27:123–33. doi: 10.1016/j.tcm.2016.07.005
18. Cullen P, von Eckardstein A, Souris S, Schulte H, Assmann G. Dyslipidaemia and cardiovascular risk in diabetes. Diabetes Obes Metab. (1999) 1:189–98. doi: 10.1046/j.1463-1326.1999.00030.x
20. Sarwar N, Gao P, Seshasai SR, Gobin R, Kaptoge S, Di Angelantonio E, et al. Diabetes mellitus, fasting blood glucose concentration, and risk of vascular disease: a collaborative meta-analysis of 102 prospective studies. Lancet. (2010) 375:2215–22. doi: 10.1016/S0140-6736(10)60484-9
21. Calderón-Larrañaga A, Saadeh M, Hooshmand B, Refsum H, Smith AD, Marengoni A, et al. Association of homocysteine, methionine, and Mthfr 677c>T polymorphism with rate of cardiovascular multimorbidity development in older adults in Sweden. JAMA Netw Open. (2020) 3:e205316. doi: 10.1001/jamanetworkopen.2020.5316
22. Moher D, Liberati A, Tetzlaff J, Altman DG. Preferred reporting items for systematic reviews and meta-analyses: the prisma statement. BMJ. (2009) 339:b2535. doi: 10.1136/bmj.b2535
23. Higgins JP, Thompson SG, Deeks JJ, Altman DG. Measuring inconsistency in meta-analyses. BMJ. (2003) 327:557–60. doi: 10.1136/bmj.327.7414.557
24. Stang A. Critical evaluation of the newcastle-ottawa scale for the assessment of the quality of nonrandomized studies in meta-analyses. Eur J Epidemiol. (2010) 25:603–5. doi: 10.1007/s10654-010-9491-z
25. Trujillo J, Vieira MC, Lepsch J, Rebelo F, Poston L, Pasupathy D, et al. A systematic review of the associations between maternal nutritional biomarkers and depression and/or anxiety during pregnancy and postpartum. J Affect Disord. (2018) 232:185–203. doi: 10.1016/j.jad.2018.02.004
26. Huang S, Zhang X, Huang J, Lu X, Liu F, Gu D. Ambient air pollution and body weight status in adults: a systematic review and meta-analysis. Environ Pollut. (2020) 265:114999. doi: 10.1016/j.envpol.2020.114999
27. Stacey RB, Zgibor J, Leaverton PE, Schocken DD, Peregoy JA, Lyles MF, et al. Abnormal fasting glucose increases risk of unrecognized myocardial infarctions in an elderly cohort. J Am Geriatr Soc. (2019) 67:43–9. doi: 10.1111/jgs.15604
28. Knudsen EC, Seljeflot I, Abdelnoor M, Eritsland J, Mangschau A, Arnesen H, et al. Abnormal glucose regulation in patients with acute St- elevation myocardial infarction-a cohort study on 224 patients. Cardiovasc Diabetol. (2009) 8:6. doi: 10.1186/1475-2840-8-6
29. Hayashi T, Kawashima S, Nomura H, Itoh H, Watanabe H, Ohrui T, et al. Age, gender, insulin and blood glucose control status alter the risk of ischemic heart disease and stroke among elderly diabetic patients. Cardiovasc Diabetol. (2011) 10:86. doi: 10.1186/1475-2840-10-86
30. Li L, Ambegaonkar BM, Reckless JP, Jick S. Association of a reduction in low-density lipoprotein cholesterol with incident cardiovascular and cerebrovascular events among people with type 2 diabetes mellitus. Eur J Prev Cardiol. (2014) 21:855–65. doi: 10.1177/2047487313481752
31. Liu F, Yang X, Li J, Cao J, Chen J, Li Y, et al. Association of fasting glucose levels with incident atherosclerotic cardiovascular disease: an 8-year follow-up study in a Chinese population. J Diabetes. (2017) 9:14–23. doi: 10.1111/1753-0407.12380
32. Aryan Z, Ghajar A, Faghihi-Kashani S, Afarideh M, Nakhjavani M, Esteghamati A. Baseline high-sensitivity C-reactive protein predicts macrovascular and microvascular complications of type 2 diabetes: a population-based study. Ann Nutr Metab. (2018) 72:287–95. doi: 10.1159/000488537
33. Wu Z, Huang Z, Lichtenstein AH, Jin C, Chen S, Wu S, et al. Different associations between Hdl cholesterol and cardiovascular diseases in people with diabetes mellitus and people without diabetes mellitus: a prospective community-based study. Am J Clin Nutr. (2021) 114:907–13. doi: 10.1093/ajcn/nqab163
34. Faghihi-Kashani S, Bonnet F, Hafezi-Nejad N, Heidari B, Aghajani Nargesi A, Sheikhbahaei S, et al. Fasting hyperinsulinaemia and 2-H glycaemia predict coronary heart disease in patients with type 2 diabetes. Diabetes Metab. (2016) 42:55–61. doi: 10.1016/j.diabet.2015.10.001
35. Birukov A, Polemiti E, Jäger S, Stefan N, Schulze MB. Fetuin-a and risk of diabetes-related vascular complications: a prospective study. Cardiovasc Diabetol. (2022) 21:6. doi: 10.1186/s12933-021-01439-8
36. Aroner SA, St-Jules DE, Mukamal KJ, Katz R, Shlipak MG, Criqui MH, et al. Fetuin-a, glycemic status, and risk of cardiovascular disease: the multi-ethnic study of atherosclerosis. Atherosclerosis. (2016) 248:224–9. doi: 10.1016/j.atherosclerosis.2016.03.029
37. Sluik D, Beulens JW, Weikert C, van Dieren S, Spijkerman AM, van der AD, et al. Gamma-glutamyltransferase, cardiovascular disease and mortality in individuals with diabetes mellitus diabetes. Metab Res Rev. (2012) 28:284–8. doi: 10.1002/dmrr.2261
38. Qi Q, Workalemahu T, Zhang C, Hu FB Qi L. Genetic variants, plasma lipoprotein(a) levels, and risk of cardiovascular morbidity and mortality among two prospective cohorts of type 2 diabetes. Eur Heart J. (2012) 33:325–34. doi: 10.1093/eurheartj/ehr350
39. Hayashi T, Kawashima S, Itoh H, Yamada N, Sone H, Watanabe H, et al. Importance of lipid levels in elderly diabetic individuals - baseline characteristics and 1-year - survey of cardiovascular events. Circ J. (2008) 72:218–25. doi: 10.1253/circj.72.218
40. Avogaro A, Giorda C, Maggini M, Mannucci E, Raschetti R, Lombardo F, et al. Incidence of coronary heart disease in type 2 diabetic men and women - impact of microvascular complications, treatment, and geographic location. Diabetes Care. (2007) 30:1241–7. doi: 10.2337/dc06-2558
41. Engström G, Stavenow L, Hedblad B, Lind P, Eriksson KF, Janzon L, et al. Inflammation-sensitive plasma proteins, diabetes, and mortality and incidence of myocardial infarction and stroke: a population-based study. Diabetes. (2003) 52:442–7. doi: 10.2337/diabetes.52.2.442
42. Shen Y, Shi L, Nauman E, Katzmarzyk PT, Price-Haywood EG, Bazzano AN, et al. Inverse association between Hdl (high-density lipoprotein) cholesterol and stroke risk among patients with type 2 diabetes mellitus. Stroke. (2019) 50:291–7. doi: 10.1161/strokeaha.118.023682
43. Sone H, Mizuno S, Fujii H, Yoshimura Y, Yamasaki Y, Ishibashi S, et al. Is the Diagnosis of metabolic syndrome useful for predicting cardiovascular disease in Asian diabetic patients? Analysis from the Japan diabetes complications study. Diabetes Care. (2005) 28:1463–71. doi: 10.2337/diacare.28.6.1463
44. Howard BV, Robbins DC, Sievers ML, Lee ET, Rhoades D, Devereux RB, et al. Ldl cholesterol as a strong predictor of coronary heart disease in diabetic individuals with insulin resistance and low Ldl: the strong heart study. Arterioscler Thromb Vasc Biol. (2000) 20:830–5. doi: 10.1161/01.ATV.20.3.830
45. Saeed A, Sun W, Agarwala A, Virani SS, Nambi V, Coresh J, et al. Lipoprotein(a) levels and risk of cardiovascular disease events in individuals with diabetes mellitus or prediabetes: the atherosclerosis risk in communities study. Atherosclerosis. (2019) 282:52–6. doi: 10.1016/j.atherosclerosis.2018.12.022
46. Hayashi T, Kawashima S, Itoh H, Yamada N, Sone H, Watanabe H, et al. Low Hdl cholesterol is associated with the risk of stroke in elderly diabetic individuals: changes in the risk for atherosclerotic diseases at various ages. Diabetes Care. (2009) 32:1221–3. doi: 10.2337/dc08-1677
47. Moosaie F, Firouzabadi FD, Abouhamzeh K, Esteghamati S, Meysamie A, Rabizadeh S, et al. Lp(a) and apo-lipoproteins as predictors for micro- and macrovascular complications of diabetes: a case-cohort study. Nutr Metab Cardiovasc Dis. (2020) 30:1723–31. doi: 10.1016/j.numecd.2020.05.011
48. Hayashi T, Araki A, Kawashima S, Sone H, Watanabe H, Ohrui T, et al. Metabolic predictors of ischemic heart disease and cerebrovascular attack in elderly diabetic individuals: difference in risk by age. Cardiovasc Diabetol. (2013) 12:10. doi: 10.1186/1475-2840-12-10
49. Protopsaltis I, Korantzopoulos P, Milionis HJ, Koutsovasilis A, Nikolopoulos GK, Dimou E, et al. Metabolic syndrome and its components as predictors of ischemic stroke in type 2 diabetic patients. Stroke. (2008) 39:1036–8. doi: 10.1161/STROKEAHA.107.498311
50. Gori M, Gupta DK, Claggett B, Selvin E, Folsom AR, Matsushita K, et al. Natriuretic peptide and high-sensitivity troponin for cardiovascular risk prediction in diabetes: the atherosclerosis risk in communities (Aric) study. Diabetes Care. (2016) 39:677–85. doi: 10.2337/dc15-1760
51. Birukov A, Eichelmann F, Kuxhaus O, Polemiti E, Fritsche A, Wirth J, et al. Opposing associations of Nt-Probnp with risks of diabetes and diabetes-related complications. Diabetes Care. (2020) 43:2930–7. doi: 10.2337/dc20-0553
52. Pertiwi K, Wanders AJ, Harbers MC, Küpers LK, Soedamah-Muthu SS, de Goede J, et al. Plasma and dietary linoleic acid and 3-year risk of type 2 diabetes after myocardial infarction: a prospective analysis in the alpha omega cohort. Diabetes Care. (2020) 43:358–65. doi: 10.2337/dc19-1483
53. Tanaka S, Tanaka S, Iimuro S, Yamashita H, Katayama S, Akanuma Y, et al. Predicting macro- and microvascular complications in type 2 diabetes. Diabetes Care. (2013) 36:1193–9. doi: 10.2337/dc12-0958
54. Lee SH, Han K, Kim HS, Cho JH, Yoon KH, Kim MK. Predicting the development of myocardial infarction in middle-aged adults with type 2 diabetes: a risk model generated from a nationwide population-based cohort study in Korea. Endocrinol Metab. (2020) 35:636–46. doi: 10.3803/EnM.2020.704
55. Abu-Lebdeh HS, Hodge DO, Nguyen TT. Predictors of macrovascular disease in patients with type 2 diabetes mellitus. Mayo Clin Proc. (2001) 76:707–12. doi: 10.4065/76.7.707
56. Shai I, Schulze MB, Manson JE, Stampfer MJ, Rifai N, Hu FB, et al. Prospective study of lipoprotein(a) and risk of coronary heart disease among women with type 2 diabetes. Diabetologia. (2005) 48:1469–76. doi: 10.1007/s00125-005-1814-3
57. Looker HC, Colombo M, Agakov F, Zeller T, Groop L, Thorand B, et al. Protein biomarkers for the prediction of cardiovascular disease in type 2 diabetes. Diabetologia. (2015) 58:1363–71. doi: 10.1007/s00125-015-3535-6
58. Turner RC, Millns H, Neil HA, Stratton IM, Manley SE, Matthews DR, et al. Risk factors for coronary artery disease in non-insulin dependent diabetes mellitus: United Kingdom prospective diabetes study (Ukpds: 23). BMJ. (1998) 316:823–8. doi: 10.1136/bmj.316.7134.823
59. Paul Chubb SA, Davis WA, Peters KE, Davis TM. Serum bicarbonate concentration and the risk of cardiovascular disease and death in type 2 diabetes: the fremantle diabetes study. Cardiovasc Diabetol. (2016) 15:143. doi: 10.1186/s12933-016-0462-x
60. Wang J, Wu X, Li Y, Han X, Hu H, Wang F, et al. Serum bilirubin concentrations and incident coronary heart disease risk among patients with type 2 diabetes: the Dongfeng-Tongji cohort. Acta Diabetol. (2017) 54:257–64. doi: 10.1007/s00592-016-0946-x
61. Strand E, Rebnord EW, Flygel MR, Lysne V, Svingen GFT, Tell GS, et al. Serum carnitine metabolites and incident type 2 diabetes mellitus in patients with suspected stable angina pectoris. J Clin Endocrinol Metab. (2018) 103:1033–41. doi: 10.1210/jc.2017-02139
62. Sone H, Tanaka S, Tanaka S, Iimuro S, Oida K, Yamasaki Y, et al. Serum level of triglycerides is a potent risk factor comparable to Ldl cholesterol for coronary heart disease in japanese patients with type 2 diabetes: subanalysis of the Japan diabetes complications study (Jdcs). J Clin Endocrinol Metab. (2011) 96:3448–56. doi: 10.1210/jc.2011-0622
63. Lee JH, Han K, Huh JH. The sweet spot: fasting glucose, cardiovascular disease, and mortality in older adults with diabetes: a nationwide population-based study. Cardiovasc Diabetol. (2020) 19:44. doi: 10.1186/s12933-020-01021-8
64. Colhoun HM, Betteridge DJ, Durrington P, Hitman G, Neil A, Livingstone S, et al. Total soluble and endogenous secretory receptor for advanced glycation end products as predictive biomarkers of coronary heart disease risk in patients with type 2 diabetes: an analysis from the cards trial. Diabetes. (2011) 60:2379–85. doi: 10.2337/db11-0291
65. Wan Z, Geng T, Li R, Chen X, Lu Q, Lin X, et al. Vitamin D status, genetic factors, and risks of cardiovascular disease among individuals with type 2 diabetes: a prospective study. Am J Clin Nutr. (2022) 116:1389—99. doi: 10.1093/ajcn/nqac183
66. Qiao Y, Liu S, Li G, Lu Y, Wu Y, Ding Y, et al. Metabolic syndrome, its components and risk of cardiometabolic multimorbidity: findings from the Uk biobank cohort. (2021). doi: 10.21203/rs.3.rs-189744/v1
67. Tajik B, Voutilainen A, Kauhanen J, Mazidi M, Lip GYH, Tuomainen TP, et al. Lipid Profile, lipid ratios, apolipoproteins, and risk of cardiometabolic multimorbidity in men: the Kuopio ischaemic heart disease risk factor study. Lipids. (2022) 57:141–9. doi: 10.1002/lipd.12337
68. LeRoith D. Hyperglycemia, hypertension, and dyslipidemia in type 2 diabetes mellitus: goals for diabetes management. Clin Cornerstone. (2008) 9:S8–S16. doi: 10.1016/S1098-3597(09)60021-1
69. Mooradian AD. Cardiovascular disease in type 2 diabetes mellitus: current management guidelines. Arch Intern Med. (2003) 163:33–40. doi: 10.1001/archinte.163.1.33
70. Chan GC, Ghazali O, Khoo EM. Management of type 2 diabetes mellitus: is it in accordance with the guidelines? Med J Malaysia. (2005) 60:578–84.
71. Ko DT, Alter DA, Guo H, Koh M, Lau G, Austin PC, et al. High-density lipoprotein cholesterol and cause-specific mortality in individuals without previous cardiovascular conditions: the canheart study. J Am Coll Cardiol. (2016) 68:2073–83. doi: 10.1016/j.jacc.2016.08.038
72. Madsen CM, Varbo A, Nordestgaard BG. Extreme high high-density lipoprotein cholesterol is paradoxically associated with high mortality in men and women: two prospective cohort studies. Eur Heart J. (2017) 38:2478–86. doi: 10.1093/eurheartj/ehx163
73. Russo G, Pintaudi B, Giorda C, Lucisano G, Nicolucci A, Cristofaro MR, et al. Age- and gender-related differences in ldl-cholesterol management in outpatients with type 2 diabetes mellitus. Int J Endocrinol. (2015) 2015:957105. doi: 10.1155/2015/957105
74. Russo GT, Giandalia A, Romeo EL, Marotta M, Alibrandi A, De Francesco C, et al. Lipid and non-lipid cardiovascular risk factors in postmenopausal type 2 diabetic women with and without coronary heart disease. J Endocrinol Invest. (2014) 37:261–8. doi: 10.1007/s40618-013-0023-z
75. Yosefy C. Hyperglycaemia and its relation to cardiovascular morbidity and mortality: has it been resolved? Acta Diabetol. (2003) (40 Suppl)2:S380–8. doi: 10.1007/s00592-003-0124-9
76. Reusch JE, Wang CC. Cardiovascular disease in diabetes: where does glucose fit in? J Clin Endocrinol Metab. (2011) 96:2367–76. doi: 10.1210/jc.2010-3011
77. Park C, Guallar E, Linton JA, Lee DC, Jang Y, Son DK, et al. Fasting glucose level and the risk of incident atherosclerotic cardiovascular diseases. Diabetes Care. (2013) 36:1988–93. doi: 10.2337/dc12-1577
78. Group IHS. Hypoglycaemia, cardiovascular disease, and mortality in diabetes: epidemiology, pathogenesis, and management. Lancet Diabetes Endocrinol. (2019) 7:385–96. doi: 10.1016/S2213-8587(18)30315-2
79. DECODE Study Group EDEG. Is the current definition for diabetes relevant to mortality risk from all causes and cardiovascular and noncardiovascular diseases? Diabetes Care. (2003) 26:688–96. doi: 10.2337/diacare.26.3.688
80. Folsom AR, Aleksic N, Catellier D, Juneja HS, Wu KK. C-reactive protein and incident coronary heart disease in the atherosclerosis risk in communities (Aric) study. Am Heart J. (2002) 144:233–8. doi: 10.1067/mhj.2002.124054
81. Kaptoge S, Di Angelantonio E, Lowe G, Pepys MB, Thompson SG, Collins R, et al. C-reactive protein concentration and risk of coronary heart disease, stroke, and mortality: an individual participant meta-analysis. Lancet. (2010) 375:132–40. doi: 10.1016/S0140-6736(09)61717-7
82. Guo L, Liu MF, Huang JN Li JM, Jiang J, Wang JA. Role of interleukin-15 in cardiovascular diseases. J Cell Mol Med. (2020) 24:7094–101. doi: 10.1111/jcmm.15296
83. Wu TW, Fung KP, Wu J, Yang CC, Weisel RD. Antioxidation of human low density lipoprotein by unconjugated and conjugated bilirubins. Biochem Pharmacol. (1996) 51:859–62. doi: 10.1016/0006-2952(95)02395-X
84. Dong H, Huang H, Yun X, Kim DS, Yue Y, Wu H, et al. Bilirubin increases insulin sensitivity in leptin-receptor deficient and diet-induced obese mice through suppression of Er stress and chronic inflammation. Endocrinology. (2014) 155:818–28. doi: 10.1210/en.2013-1667
85. Kawamura K, Ishikawa K, Wada Y, Kimura S, Matsumoto H, Kohro T, et al. Bilirubin from heme oxygenase-1 attenuates vascular endothelial activation and dysfunction. Arterioscler Thromb Vasc Biol. (2005) 25:155–60. doi: 10.1161/01.ATV.0000148405.18071.6a
86. Chen SC, Lin CP, Hsu HC, Shu JH, Liang Y, Hsu PF, et al. Serum bilirubin improves the risk predictions of cardiovascular and total death in diabetic patients. Clin Chim Acta. (2019) 488:1–6. doi: 10.1016/j.cca.2018.10.028
87. Januzzi JL, van Kimmenade R, Lainchbury J, Bayes-Genis A, Ordonez-Llanos J, Santalo-Bel M, et al. Nt-Probnp testing for diagnosis and short-term prognosis in acute destabilized heart failure: an international pooled analysis of 1256 patients: the international collaborative of Nt-Probnp study. Eur Heart J. (2006) 27:330–7. doi: 10.1093/eurheartj/ehi631
88. Santaguida PL, Don-Wauchope AC, Oremus M, McKelvie R, Ali U, Hill SA, et al. Bnp and Nt-Probnp as prognostic markers in persons with acute decompensated heart failure: a systematic review. Heart Fail Rev. (2014) 19:453–70. doi: 10.1007/s10741-014-9442-y
89. Malachias MVB, Wijkman MO, Bertoluci MC. Nt-Probnp as a predictor of death and cardiovascular events in patients with type 2 diabetes. Diabetol Metab Syndr. (2022) 14:64. doi: 10.1186/s13098-022-00837-6
90. Haffner SM, Stern MP, Hazuda HP, Mitchell BD, Patterson JK. Cardiovascular risk factors in confirmed prediabetic individuals. Does the clock for coronary heart disease start ticking before the onset of clinical diabetes? JAMA. (1990) 263:2893–8. doi: 10.1001/jama.263.21.2893
91. de Boer IH, Bangalore S, Benetos A, Davis AM, Michos ED, Muntner P, et al. Diabetes and hypertension: a position statement by the American diabetes association. Diabetes Care. (2017) 40:1273–84. doi: 10.2337/dci17-0026
92. Uk Prospective Diabetes Study. Xi: biochemical risk factors in type 2 diabetic patients at diagnosis compared with age-matched normal subjects. Diabet Med. (1994) 11:534–44. doi: 10.1111/j.1464-5491.1994.tb02032.x
93. Ridker PM, Cushman M, Stampfer MJ, Tracy RP, Hennekens CH. Inflammation, aspirin, and the risk of cardiovascular disease in apparently healthy men. N Engl J Med. (1997) 336:973–9. doi: 10.1056/NEJM199704033361401
94. Thompson PD, Panza G, Zaleski A, Taylor B. Statin-associated side effects. J Am Coll Cardiol. (2016) 67:2395–410. doi: 10.1016/j.jacc.2016.02.071
95. Ballesteros MN, Cabrera RM, Saucedo MS, Yepiz-Plascencia GM, Ortega MI, Valencia ME. Dietary fiber and lifestyle influence serum lipids in free living adult men. J Am Coll Nutr. (2001) 20:649–55. doi: 10.1080/07315724.2001.10719163
96. Zhao Y, Liu X, Mao Z, Hou J, Huo W, Wang C, et al. Relationship between multiple healthy lifestyles and serum lipids among adults in rural China: a population-based cross-sectional study. Prev Med. (2020) 138:106158. doi: 10.1016/j.ypmed.2020.106158
Keywords: biomarker, cardiometabolic multimorbidity, type 2 diabetes mellitus, coronary heart disease, stroke, systematic review
Citation: Jin Y, Xu Z, Zhang Y, Zhang Y, Wang D, Cheng Y, Zhou Y, Fawad M and Xu X (2023) Serum/plasma biomarkers and the progression of cardiometabolic multimorbidity: a systematic review and meta-analysis. Front. Public Health 11:1280185. doi: 10.3389/fpubh.2023.1280185
Received: 19 August 2023; Accepted: 27 October 2023;
Published: 23 November 2023.
Edited by:
Sen Li, Beijing University of Chinese Medicine, ChinaReviewed by:
Benli Su, Second Hospital of Dalian Medical University, ChinaDongshan Zhu, Shandong University, China
Copyright © 2023 Jin, Xu, Zhang, Zhang, Wang, Cheng, Zhou, Fawad and Xu. This is an open-access article distributed under the terms of the Creative Commons Attribution License (CC BY). The use, distribution or reproduction in other forums is permitted, provided the original author(s) and the copyright owner(s) are credited and that the original publication in this journal is cited, in accordance with accepted academic practice. No use, distribution or reproduction is permitted which does not comply with these terms.
*Correspondence: Xiaolin Xu, eGlhb2xpbi54dUB6anUuZWR1LmNu
†These authors have contributed equally to this work