- 1Department of Community Health Nursing, School of Nursing, College of Medicine and Health Sciences, University of Gondar, Gondar, Ethiopia
- 2Department of Public Health, College of Medicine and Health Sciences, Samara University, Samara, Ethiopia
- 3Department of Epidemiology and Biostatistics, Institute of Public Health, College of Medicine and Health Sciences, University of Gondar, Gondar, Ethiopia
Background: The postnatal period is a critical period for both mothers and their newborns for their health. Lack of early postnatal care (PNC) services during a 2-day period is a life-threatening situation for both the mother and the babies. However, no data have been examined for PNCs in East Africa. Hence, using the more flexible Bayesian multilevel modeling approach, this study aims to investigate the pooled prevalence and potential factors for PNC utilization among women after delivery in East African countries.
Methods: We retrieved secondary data from the Kids Record (KR) demographic and health surveys (DHS) data from 2015 to 2022 from 10 East African countries. A total of 77,052 weighted women were included in the study. We used R 4.3.2 software for analysis. We fitted Bayesian multilevel logistic regression models. Techniques such as Rhat, effective sample size, density, time series, autocorrelation plots, widely applicable information criterion (WAIC), deviance information criterion (DIC), and Markov Chain Monte-Carlo (MCMC) simulation were used to estimate the model parameters using Hamiltonian Monte-Carlo (HMC) and its extensions, No-U-Turn Sampler (NUTS) techniques. An adjusted odds ratio (AOR) with a 95% credible interval (CrI) in the multivariable model to select variables that have a significant association with PNC was used.
Results: The overall pooled prevalence of PNC within 48 hrs. of delivery was about 52% (95% CrI: 39, 66). A higher rate of PNC usage was observed among women aged 25–34 years (AOR = 1.21; 95% CrI: 1.15, 1.27) and 35–49-years (AOR = 1.61; 95% CrI: 1.5, 1.72) as compared to women aged 15–24 years; similarly, women who had achieved primary education (AOR = 1.96; 95% CrI: 1.88, 2.05) and secondary/higher education (AOR = 3.19; 95% CrI: 3.03, 3.36) as compared to uneducated women; divorced or widowed women (AOR = 0.83; 95% CrI: 0.77, 0.89); women who had currently working status (AOR = 0.9; 95% CrI: 0.87, 0.93); poorer women (AOR = 0.88; 95% CrI: 0.84, 0.92), middle-class women (AOR = 0.83; 95% CrI: 0.79, 0.87), richer women (AOR = 0.77; 95% CrI: 0.73, 0.81), and richest women (AOR = 0.59; 95% CrI: 0.55, 0.63) as compared to the poorest women; women who had media exposure (AOR = 1.32; 95% CrI: 1.27, 1.36), were having 3–5 children (AOR = 0.89; 95% CrI: 0.84, 0.94), had >5 children (AOR = 0.69; 95% CrI: 0.64, 0.75), had first birth at age < 20 years (AOR = 0.82; 95% CrI: 0.79, 0.84), had at least one ANC visit (AOR = 1.93; 95% CrI: 1.8, 2.08), delivered at health facilities (AOR = 2.57; 95% CrI: 2.46, 2.68), had average birth size (AOR = 0.94; 95% CrI: 0.91, 0.98) and small birth size child (AOR = 0.88; 95% CrI: 0.84, 0.92), had twin newborns (AOR = 1.15; 95% CrI: 1.02, 1.3), and fourth and above birth order (AOR = 0.88; 95% CrI: 0.82, 0.95) were individual-driven women who have been independently associated with PNC, respectively. Regarding community-level variables, rural women (AOR = 0.76; 95% CrI: 0.72, 0.79), high media exposure communities (AOR = 1.1; 95% CrI: 1.04, 1.18), communities with high wealth levels (AOR = 0.88 95% CrI: 0.83, 0.94), communities with high antenatal care (ANC) utilization (AOR = 1.13, 95% CrI: 1.07, 1.19), and long distance to health facilities (AOR = 1.5; 95% CrI: 1.38, 1.63) were among the community factors associated with PNC, respectively.
Conclusion: One of the significant public health priorities in East Africa continues to be the underutilization of immediate PNC. The government ought to prioritize improving maternity and child health services, collaborating with interested parties in the area, reducing health disparities, educating mothers about child health, and other connected issues that are very beneficial.
Introduction
According to the various wide range of reports, the damage caused by the world’s health system—especially to the health of mothers and children—continues to be very serious. To achieve Sustainable Development Goal 3 (SDG 3), that is, ensuring healthy lives and promoting wellbeing at all ages, efforts on the mother’s health during pregnancy, childbirth, and the postpartum period are the main agenda (1). The WHO, and United Nations Children’s’ Fund (UNICEF) advise that women who give birth in medical facilities should have their babies and themselves examined for any health-related challenges within 24 h, considering a date to bring them back for additional postnatal care (PNC) even when everything seems to be going well (2, 3). Furthermore, women should be counseled to return back immediately if they notice any warning signs (4). The initial PNC contact should also be held as soon as practicable, ideally within 24 h of the birth, if the delivery occurs at home. Ultimately, it is advocated that all mothers and newborns receive a minimum of three more postnatal examinations: on day 3 (48–72 h), within days 7–14 immediately after delivery, and 6 weeks later (5–7). Globally, although the number of maternal and infant deaths is decreasing, developing countries, especially, sub–Saharan African (SSA) countries, have a significant share in the failure of the sustainable development goal to reduce 70 maternal deaths per 100,000 live births and 12 neonatal deaths per 1,000 live births by 2030 (8, 9). Since the year 2017, pieces of evidence show that globally, 66% of maternal deaths occur in SSA only (10–12), and the issue is more massive and awful. Inaccessibility to healthcare and inadequate use of postnatal care (PNC) contribute to 99% of maternal mortality in low- and middle-income countries (LMICs), including SSA. These issues are particularly concerning because the majority of the deliveries in these regions occur at home, leading to tragic outcomes that affect public health significantly (9, 12, 13). In comparison to the 230 fatalities per 100,000 live births that occurred in LMICs, the maternal mortality ratio similarly remained unacceptably high at 433 (14, 15). All these gaps could be eliminated by integrating maternal child health service care at a high range (16). Therefore, if women can receive the early postnatal care check-up suggested by the WHO, it could significantly reduce the risk of complications and maternal mortality (6, 17). The SSA countries can avoid greater maternal morbidity and related mortality, which could also help the countries achieve SDG 3.
There are significant gaps in understanding early PNC in East Africa. Key issues include outdated knowledge, lack of region-specific studies, insufficient detail on influencing factors, limited use of advanced statistical methods, and large sample sizes. Addressing these gaps with current, localized, and methodologically robust research is crucial for improving PNC outcomes in the region. Although studies were not conducted under large study settings, numerous studies have found links between the continuum of care and factors such as socioeconomic status, family and individual characteristics, community features, characteristics of the newborn, and interactions with the healthcare system (18, 19). For instance, the age of the mother (20, 21), her marital status (22), her education level (20, 21, 23), occupation (23), the order in which her children were born (20), her access to media (18, 21), residence, health insurance, family number, her ability to make decisions, and her wealth index (19, 21, 24) were found to be the determinants of the continuum of maternal and child care.
Therefore, this study aimed to ascertain the number of reproductive-age women who received each of the chosen functions of the PNC during the first 2 days after birth, as well as its predictors of the number of last live births in the 2 years prior to the survey by applying more advanced and flexible modeling approach to the data. As the Bayesian multilevel approach incorporates prior information, allows for more model flexibility, and is a natural approach to expressing uncertainty, this study benefits the East African countries by identifying key factors—both individual and community-level factors—that influence postnatal care use in the region. It is crucial to enhance PNC and lower rates of child morbidity and mortality. Although studies have not been performed to clarify the elements that support or obstruct PNC at the national level, East African countries continue to be one of the leading causes of mother and infant mortality worldwide (25). The results of this study will provide preliminary information for the upcoming researchers in the area, and policymakers and other stakeholders working on issues related to both women’s and children’s health with an up-to-date multicounty result from which they can build, enhance, and implement their plans.
Materials and methods
Study setting and period
For a cross-sectional study design conducted nationwide, data were gathered in East African countries for the period from 2015 to 2022. The study encompassed communities from 10 countries: Burundi, Ethiopia, Kenya, Madagascar, Malawi, Rwanda, Uganda, Tanzania, Zambia, and Zimbabwe. The demographic and health surveys (DHS) Program, funded by the United States Agency for International Development (USAID), provided both financial support and technical assistance for population and health surveys conducted globally. The most recent DHSs dataset encompassing the last 7-year period (2015–2022) in East African nations served as this study’s primary source of information. A standardized dataset was employed (26) to collect a sizable sample size that is representative of the population source and all factors. DHSs gather comparable data on a global scale. The surveys have huge sample sizes, are population-based, and are nationally representative of each nation (26). The 14 nations that make up Eastern Africa are spread throughout the Horn of Africa, the Indian Ocean islands, and the Great Lakes region. These nations struggle with comparable economic, social, and environmental problems and worry that they would not achieve all of the sustainable development objectives (27) East Africa refers to the region of the African continent situated in the Horn and Eastern regions of the Sahara Desert. It is estimated that approximately 486,766,759 individuals inhabit this region, and it spans an area of 6,667,493 km2 (2,574,332 sq. mi), constituting around 6.03% of the global population.
Sample size determination and sampling methods
In every country, the DHSs followed the stratified multistage sampling method, which involves stratification, clustering, and sample selection over two stages. Urban and rural areas were grouped into separate clusters to form the basis for stratification. The administered questionnaire was responded to by women who consented and agreed to participate in the study. Enumeration areas (EAs) inside each sampling stratum were initially chosen with a probability proportional to their size. The households were carefully sampled in the second step. Each of the chosen clusters underwent a household listing operation (28). Demographic and health survey reports were accessible for approximately 10 out of the 13 East African nations. Periodically, every 5 years, a systematic gathering of DHSs surveys is carried out in low- and middle-income countries, utilizing pretested, validated, and structured questionnaires. Using a standardized approach for sampling, questionnaires, data collection, and coding across DHSs surveys enables the possibility of conducting multicountry analyses. In each of the surveys conducted in the specified nations, the most recent conventional census frame was utilized.
The DHSs’ samples are often divided into urban and rural areas within each administrative geographic region. The DHSs employ a stratified two-stage cluster sampling technique. In the first stage, clusters and enumeration areas (EAs) are randomly selected from the sample frame, typically derived from the most recent national census available. Subsequently, systematic sampling is utilized in the second stage to select households within each cluster or EA. During the initial sampling round, EAs are chosen with a probability proportional to the size of each stratum. A predetermined number of households is systematically selected within designated EAs in the second stage. Following the listing of households, equal probability systematic sampling is employed to select a specific number of households within the defined cluster (26).
Data source and study population
For this study, we utilized DHSs that were conducted over the span of the last 7 years, from 2015 to 2022. Among the approximately 14 countries in East Africa that carried out DHSs during this period, only approximately 10 countries’ surveys contained the necessary information on the outcome variable. Thus, they were included in our analysis. After appending the data from each country, the final analysis included a total weighted sample of 78,213 women and a total unweighted sample of 78,536 women (refer to Table 1). The source population consisted of mothers who had given birth 2 years prior to the survey. Therefore, only mothers who met this criterion were included in the analysis. To account for any unequal sample distributions during the data collection process, weightings were applied to the children’s samples during the estimation process. This adjustment ensured that the results were representative and accurately reflected the studied population.
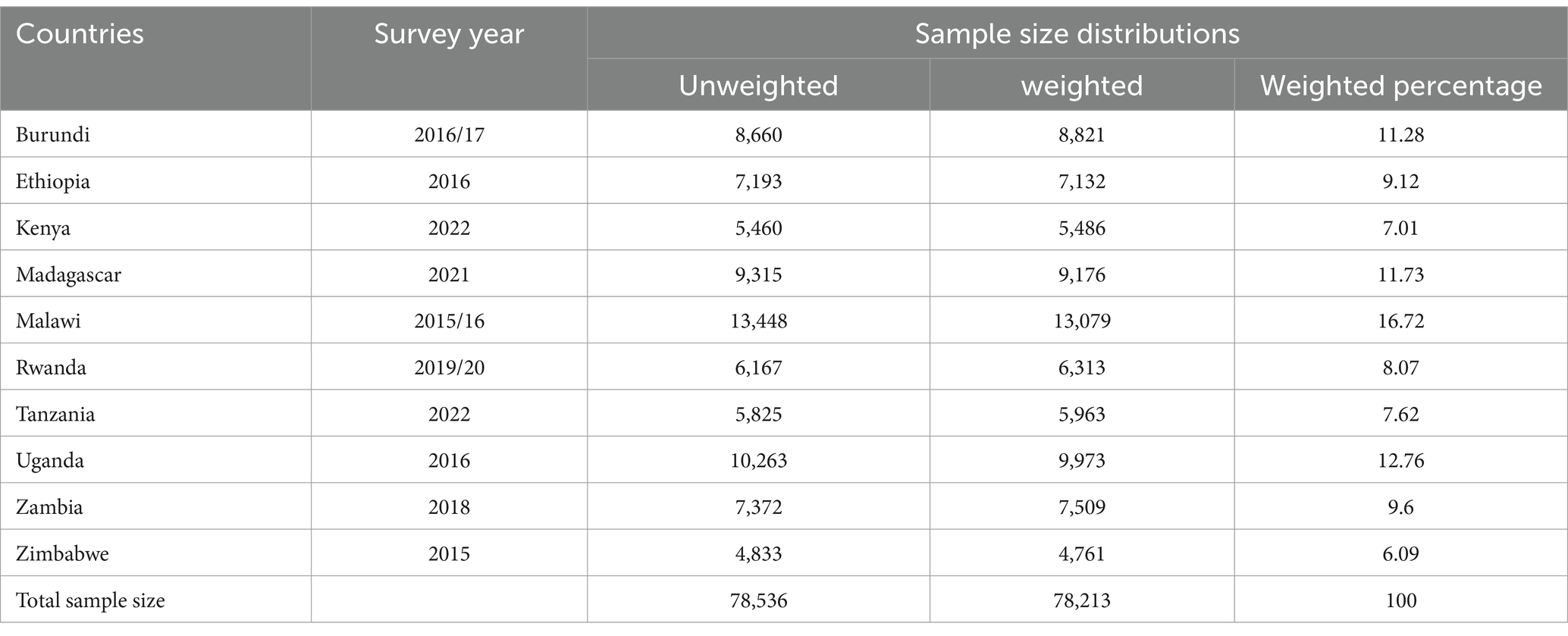
Table 1. List of countries, survey years, and sample size distribution for PNC uptake in East African countries from 2015–2022.
Data quality control
To ensure data quality in each country, the DHSs implemented several measures. These included providing comprehensive training to data collectors, supervisors, and field editors, as well as conducting ongoing supervision to maintain high standards. Standardized questionnaires were used, which were translated into national and local languages to accommodate participants’ preferred languages. Data processing specialists were involved in the data entry and management phase, ensuring accurate and efficient handling of the collected data. Systematic biases were carefully addressed during this phase to minimize their impact on the results. Once the data were obtained from the DHSs, proper data management procedures were followed. This involved appending women’s and men’s data, addressing missing observations through appropriate techniques such as missing completely at random, and conducting necessary recoding and variable recategorization. These rigorous data management practices were consistently implemented across all countries with similar working systems, ensuring a standardized and reliable approach to data handling and analysis. The DHSs guidance provides additional details regarding the data-collection process. Details can be accessed from the Guide to DHSs statistics (26).
Variables of the study
Dependent variable
The study’s outcome variable is early postnatal care (PNC) within the first 2 days of delivery. To calculate the desired measure of PNC for newborns within the first 2 days after birth, along with the associated variables, the under-five datasets (KR files) from DHSs data of 10 East African countries were utilized as the data source for this analysis. The factors considered for computation included cord examination (m78a), temperature measurement (m78b), counseling on danger symptoms (m78c), breastfeeding counseling (m78d), and nursing observation (m78e) (29). All of these were summed up, and if a newborn did not receive a postnatal check within 2 days of birth, it was recorded as “No (0).” Conversely, if a newborn did receive a postnatal check within 2 days, it was recorded as “Yes (1)“.
Independent variables
Both individual and community-level factors that have been identified by earlier researchers and scientific backgrounds as potential factors of PNC checkups were included in this study (Table 2).
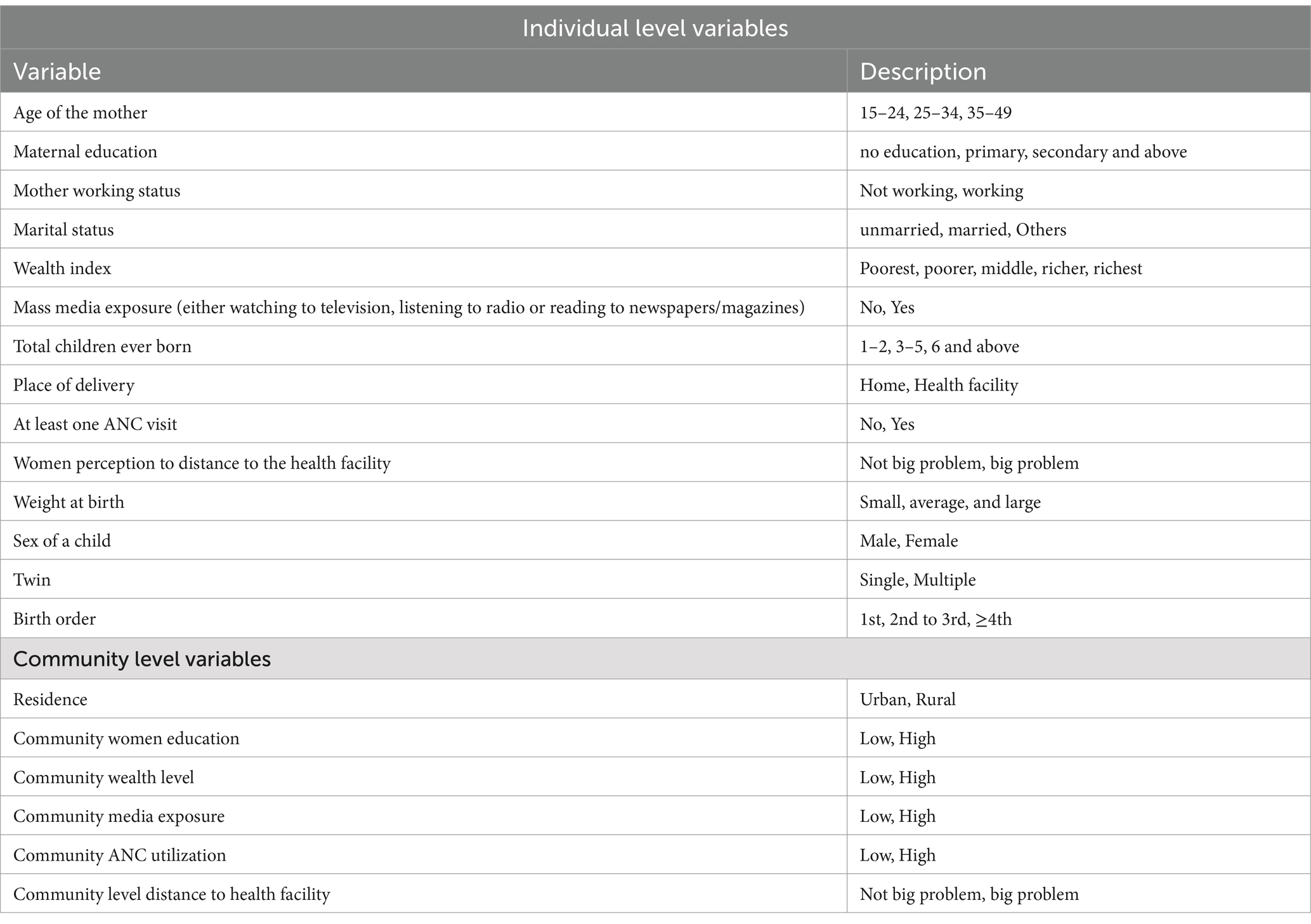
Table 2. Shows independent variable categorizations among women in East Africa countries, 2015–2022.
Data management and analysis process
We conducted a secondary study using the Kids Records (KR) dataset, which involved analyzing the data from the DHSs. To carry out the analysis, we utilized R 4.3.2 software (68, 69) and Microsoft Excel version 19 for tasks such as data downloading, appending, cleaning, recoding, and handling missing values. In the DHSs’ data, infants were nested within clusters, and neonates within the same cluster showed more remarkable similarity than those in different clusters. This violated the assumptions of independence of observations and equal variance across clusters in the classical logistic regression model. Therefore, a more sophisticated modeling approach was required to account for between-cluster factors. We constructed a Bayesian multilevel random logistic regression model to investigate the relationship between individual- and community-level variables and the risk of not receiving immediate PNC within 2 days of birth. In total, four models were developed. The first model, known as the empty or null model, was fitted without any explanatory variables. This model helped reduce community differences and served as a baseline for understanding community variances. It also assisted in determining whether a multilevel statistical framework should be used instead of a standard logistic regression model. The null model was evaluated using various measures, including the log–likelihood ratio (LLR), median odds ratio (MOR), intraclass correlation coefficient (ICC), proportional change of variance (PCV), and the widely applicable information criterion (WAIC). The second model included only individual or household-level characteristics, while the third included only community-level factors without individual-level factors. Finally, the fourth and final model included components from both the individual and community levels. These models were constructed to assess the impact of individual and community factors on mothers’ behavior regarding immediate PNC. Then, we conducted a Bayesian meta-analysis to compute the pooled prevalence and construct the forest plot (30). Subsequently, a two-level Bayesian logistic regression was employed to identify the determinants of PNC.
Bayesian mixed model and convergence of algorithm
To identify the factors influencing early PNC uptake, we utilized multilevel mixed-effects logistic regression analysis employing the Bayesian approach. The Bayesian approach to credible intervals serves a similar purpose to classical confidence intervals (CIs); however, their composition and interpretation philosophies differ significantly (31). The Bayesian statistical approach offers the ability to incorporate additional prior information external to the data through prior distributions. By leveraging this additional prior information, the accuracy and credibility of effect size estimations can be improved. Consequently, applying the classical confidence interval interpretation is inappropriate, as the Bayesian statistical approach provides a more reasonable alternative. The Bayesian credible interval interpretation is more intuitive in this context, while frequentist confidence intervals are often misinterpreted as Bayesian credible intervals.
We used the random variable EAs to account for the variation of early postnatal care (PNC) utilization across different EAs within the country. An ICC value >5% was used as a threshold to consider the variation across EAs. Since the outcome variable was dichotomized (no/yes), children within households were treated as level-1 unit, while EAs were considered as level-2 units. This hierarchical structure resulted in children being nested within EAs. We employed a Bayesian multilevel logistic regression model to address the hierarchical nature of the data and account for the dependency of observations within the same cluster. This approach allowed us to obtain accurate and credible estimates of effect sizes, considering the clustering of children within EAs and the potential impact of the EA on the outcome variable.
Hence, the dependent variable was represented by:
The likelihood function
The key ingredients to a Bayesian analysis are the likelihood function, which reflects information about the parameters contained in the data, and the prior distribution, which quantifies what is known about the parameters before observing data. The prior distribution and likelihood can be easily combined to form the posterior distribution, which represents total knowledge about the parameters after the data have been observed. Bayesian multilevel logistic analysis specifies a dichotomous dependent variable as a function of a set of explanatory variables (32, 33).
Likelihood contribution from the subject in the group is Bernoulli:
where represents the probability of the event for subject i in group j that has a covariate vector and indicates the presence ( ) or absence ( ) of the event for that subject. In multilevel logistic regression, we know that
where is a fixed part of the model and is a random part of the model and . is the probability of the child in the the group having PNC uptake so that the likelihood contribution to the subject in the group is
Since individual subjects in the group are assumed to be independent of each other, the likelihood function over a dataset of subjects in the group is then
Prior distribution
For a flat normal prior, we will assume a normal distribution for the coefficients and with mean and variance .
Let us denote the prior distribution for the parameters as follows:
Posterior distribution
The full conditional distribution for parameter is given by (34):
For parameter , the full conditional distribution is (34):
where is the total number of observations.
To estimate the parameters of the variable and the extent of random variations between clusters, we used the Brms-R package (70–72). It uses Hamiltonian Monte-Carlo (HMC) and its extensions No-U-Turn Sampler (NUTS) that uses a recursive algorithm to build a set of likely candidate points that spans a wide swath of the target distribution, stopping automatically when it starts to double back and retrace its steps. These features allow it to converge to high-dimensional target distributions much more quickly than simpler methods, such as the random walk Metropolis or Gibbs sampler (35). Currently, in a multilevel framework, Brms provides an intuitive, powerful, and flexible formula syntax that extends the well-known formula syntax of lme4, especially when modeling categorical and ordinal data (36). Hence, we employed this package to fit multilevel logistic regression models based on the Bayesian approach.
The regression coefficients for each parameter and variance were estimated using a flat prior with a normal distribution (0, 1,000) and a half-Cauchy distribution (0, 25), respectively. In addition, we employed iteration = 4,000, warm-up (number of discarded iterations) = 2,000, cores = 2 (specifying the number of cores used for the algorithm), chains = 2, adapt delta (controlling divergent transitions) = 0.95, and initials (starting values for the iterations) = 0 to estimate the posterior distribution.
For the multilevel binary logistic regression analysis, four models were developed. These models included an empty model, which had no independent variables and served to assess the extent of cluster variation in early PNC utilization. Additionally, there were models with individual-level variables, community-level variables, and a full model incorporating both types of variables. The widely applicable information criterion (WAIC) was utilized to identify the best-fitting model,. This criterion is preferred over the commonly used deviance information criterion (DIC) for model selection (37, 38). A model with the lowest WAIC was the best fitted model. The results obtained from a given HMC analysis are not believed to be reliable until the chain has reached its stationary distribution and converged much more quickly (39, 40). Hence, to assess the convergence diagnostics criteria, we employed the following indicators: (37, 73, 74) Rhat = 1, effective sample sizes (both Bulk_ESS and Tail_ESS) exceeding 1,000. Additionally, we examined the time series plots to ensure well-mixed chains, observed smooth density plots, and verified that the autocorrelation plots showed minimal correlation with zero for the majority of parameters (Supplementary Figures S1–S3).
Measure of variations between levels
To estimate the EA effects on early PNC uptake outcome and to quantify the variation in the early PNC uptake outcome between EAs (i.e., clusters), we applied the variance partition coefficient (VPC) and the median odds ratio (MOR), respectively.
Intra-class correlation (ICC): The VPC measures the proportion of the total observed individual variation in the outcome that is explained by the between cluster variation. The VPC requires an estimate of the variance at the individual level (level 1), and for the standard logistic distribution, this variance is equal to (41). Therefore, in the multilevel logistic regression models M1 and M2 with a logit link, the PCV or ICC is approximated by
where σ2μ is the variance of the random parameter at the cluster level representing the amount of unobserved heterogeneity between clusters; and is a parameter representing the amount of unobserved heterogeneity between women (individual-level variance).
Median Odds Ratio (MRR): The MOR measures how much variability in the early PNC uptake exists between EAs (i.e., clusters) by comparing two persons from two randomly chosen, different EAs (42). Consider two persons chosen randomly from two different EAs but with the same values of covariates in the model. The MOR is the median odds ratio between the person at EA with a higher probability of receiving early PNC services and the person at Survey EA with a lower probability of receiving early PNC services. It is a function of the estimated EAs, that is, cluster, variance and is given by
where σ2 is the variance of each model, and ƒ−1 is the inverse of the standard normal cumulative distribution function is the 75th percentile, and is the exponential function (42, 43).
Proportional change in variance (PCV) was calculated as , where V0 is the variance of the null model, and Vx is the variance of each model at each level with variables (41).
Operational definitions for community-level factors
Community women education
This metric reflects the collective value of women’s educational attainment, which is determined based on the median distribution of educational levels within the neighborhood. If the proportion of women in the community with at least a secondary education fell below the median, it was considered low, while if it exceeded the median, it was considered high. On average, the median proportion was 0.50.
Community media exposure
This variable was created based on individual responses regarding exposure to radio or television media. If the proportion of women in the community who reported media exposure was between 0 and 48%, it was classified as low. Conversely, if the proportion ranged from 49 to 100%, it was considered high.
Community ANC utilization rate
This variable is derived from individual data on antenatal care (ANC) utilization. If the proportion of women in the community who attended at least one ANC visit fell between 0 and 50%, it was classified as low. The median value for this proportion was 0.51.
Community perception distance to health facility
This community-level variable was constructed based on individual responses regarding the major challenge in accessing health facilities, specifically related to the distance to the nearest facility. If the proportion of women in the community who perceived distance as a significant problem to visit health facilities ranged from 0 to 14%, it was categorized as a low problem. The median value for this proportion was 0.15.
Community wealth
The procedure employed to obtain this variable was similar to that used for the household’s wealth index. If the proportion of women in a community belonging to the two lowest wealth quintiles ranged from 50 to 100%, it was classified as high. Conversely, if the proportion fell between 0 and 49%, it was considered low.
Results
Sociodemographic characteristics of the study participants
In this analysis, a total of 78,213 weighted numbers of mothers were included. Out of the total mothers interviewed 44.7% of them were between 25 and 34 years of age. Close to 49% of the women had primary education, while 29% had secondary and above education. The majority (64%) of mothers were employed, and 67% were married. In addition, 45.67% of the participants were poor, and about 57% had media exposure. Nearly 93% of the women had ANC visits during their pregnancy time. Moreover, 77% of them gave birth at a health facility. About 58% of mothers give birth for the first time at the age of 19 years and below. Only 20% of the households had a number of under-5-year-old children above the age of 5 years. Close to half (51%) of the children were males, and only 1.7% were twins. Approximately 53.47% of the children had average size, and 41% of the children were the fourth or above child in the family. Regarding community-level variables, nearly 53% of the participants were from four countries (namely, Burundi, Madagascar, Malawi, and Uganda), and 77% were rural residents. Approximately 53% of the population had low exposure to mass media. Nearly 51% of women in the community had low educational levels. Regarding the community utilization of health facility delivery was low at 52%. Approximately 52% of the community had low ANC utilization and poverty level, respectively (Table 3).
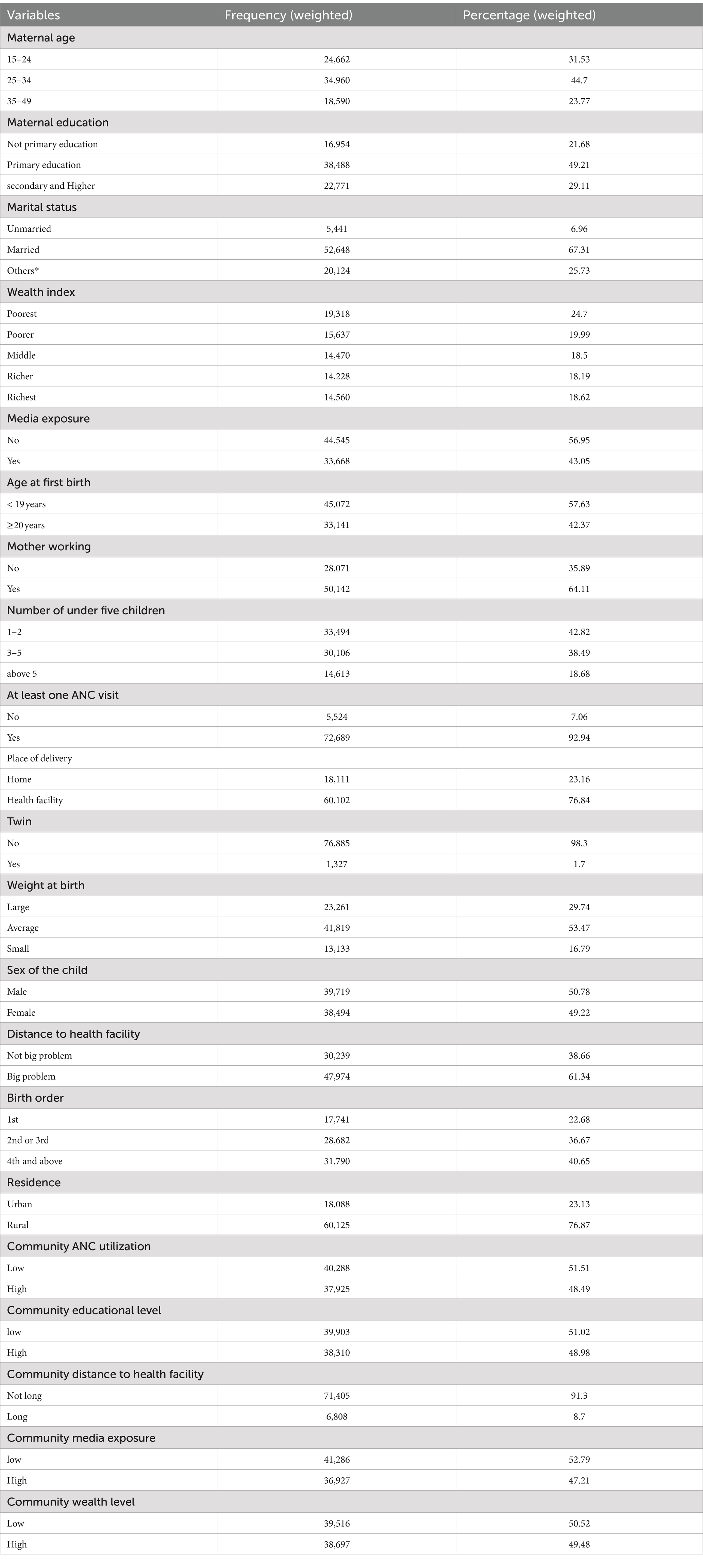
Table 3. Participant characteristics early PNC uptake and its associated factors following child birth in East Africa: using recent DHS data from 2015 to 2022.
Pooled magnitude of early PNC uptake in East Africa
The pooled magnitude of early PNC uptake in the East African countries was 52% (95% CI: 39, 66). The highest magnitude of early PNC uptake was found in Zimbabwe (71%), followed by Kenya (69%) and Rwanda (63%). The lowest magnitude of early PNC utilization was reported in Uganda (40%). The between-country difference in magnitude of early PNC uptake (τ) was 22% (95% CI: 11, 44) (Figure 1).
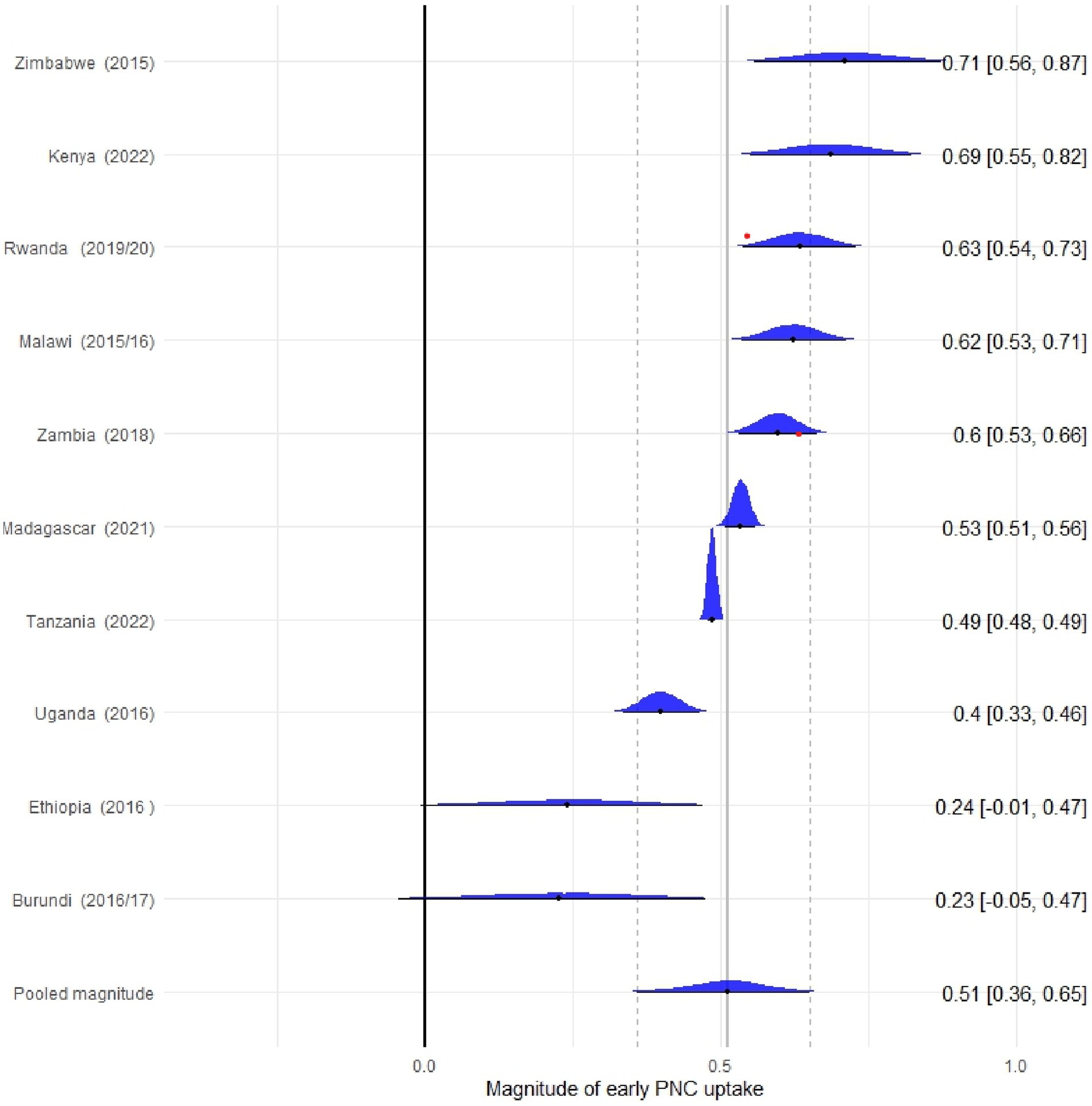
Figure 1. Forest plot of pooled prevalence of early PNC uptake in East Africa across 10 countries from 2015 to 2022.
Measures of variation (random effect estimates) analysis
The model with both individual and community-level factors has the smallest Widely Applicable Information Criteria (WAIC = 98,050.2) compared to the random intercept-only model (WAIC 107,081.7), the model with only individual-level factors (WAIC = 98,361.9), and the model with only community-level factors (WAIC = 105,612). Therefore, this model is the best fitted model for the data because it has the smallest WAIC compared to the other models. Interpretations and reports were made based on this model. The random effect estimates have been determined by fitting four models (Model I (null model), Model II, Model III, and Model IV). The null model showed significant variability in the likelihood of early PNC uptake among East African countries (σ2 = 0.202). The ICC of 0.06 in the null model implied that the difference between clusters contributed 6% of the total variation in the uptake of early PNC services. The MOR of 1.54 in the null model implied that if children were picked randomly from two different clusters (EAs), children within a cluster had a higher probability of receiving early PNC, with a 1.54 times higher chance of receiving early PNC services compared to children within a cluster with a lower chance of uptake of early PNC services. The proportional change in variance (PCV) in the full model was 55.7%, showing that both individual- and community-level factors explained 55.7% of the observed variation in uptake of early PNC (see Table 4).
Factor affecting early PNC uptake
In a multilevel multivariable Bayesian logistic regression analysis, variables, namely, maternal age, maternal education, marital status, mother’s working status, wealth index, media exposure, age at first birth, parity, place of delivery, at least one ANC, birth size, birth order number, while residence, community media exposure, community wealth level, community ANC utilization and community distance to health facility were identified as significant individual and community-level factors of receiving early PNC service (Table 5).
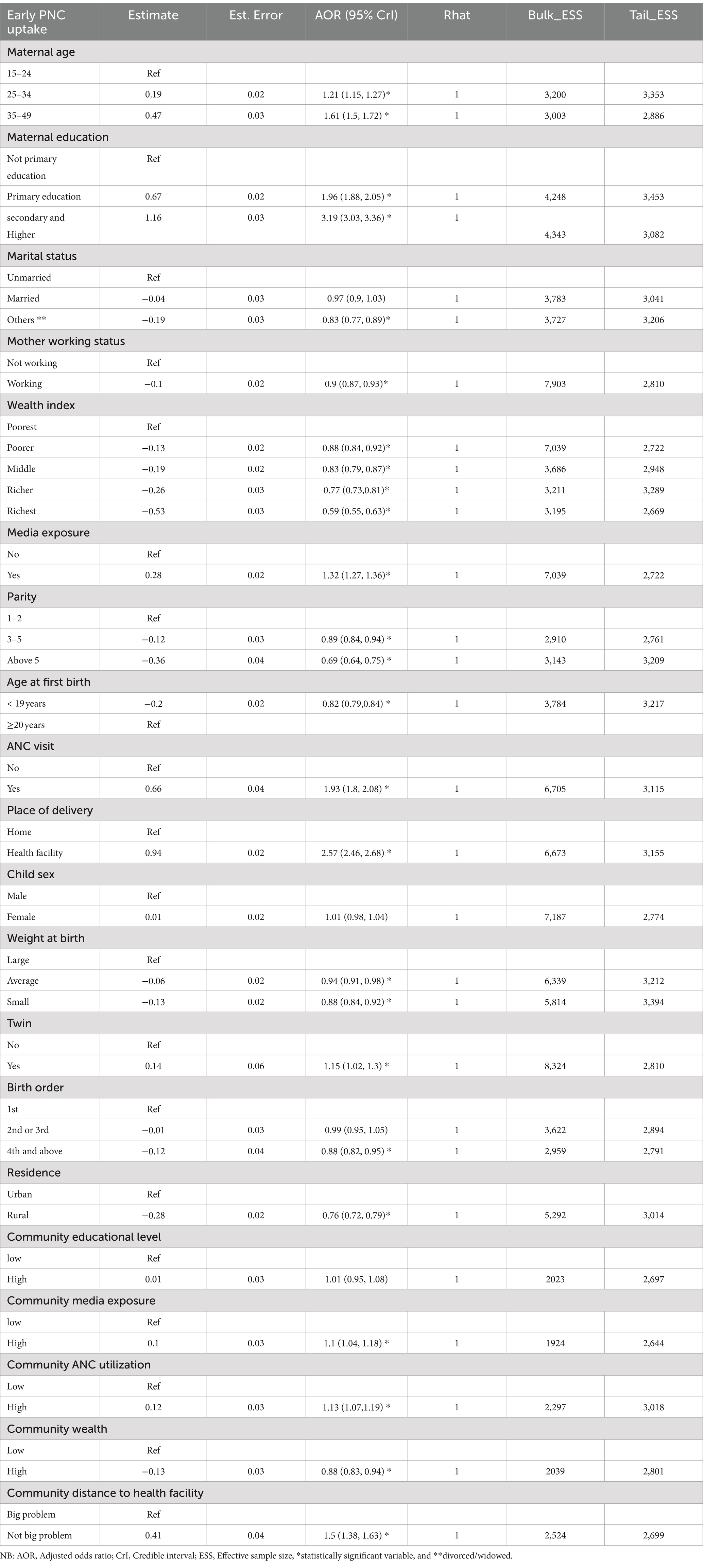
Table 5. Individual and community level factors associated with early PNC uptake among women in East African countries using the recent DHS datasets, 2015–2022.
The odds of early PNC service uptake among women in the age group of 25–34 years (AOR = 1.21; 95% CrI: 1.15, 1.27) and 35–49 years (AOR = 1.61; 95% CrI: 1.5, 1.72) were higher by 21 and 61%, respectively, compared to women aged 15–24 years old. Similarly, women who had achieved primary education (AOR = 1.96; 95% CrI: 1.88, 2.05) and secondary/higher education (AOR = 3.19; 95% CrI: 3.03, 3.36) were 1.96 and 3.19 times more likely to receive early PNC services than formally uneducated women, respectively. Divorced or widowed women (AOR = 0.83; 95% CrI: 0.77, 0.89) had 17% lower odds of early PNC service uptake than unmarried women. Currently working women (AOR = 0.9; 95% CrI: 0.87, 0.93) had 10% lower odds of early PNC service uptake than those not working. Regarding household wealth indexes, taking the poorest households as a reference, poorer women (AOR = 0.88; 95% CrI: 0.84, 0.92), middle-class women (AOR = 0.83; 95% CrI: 0.79, 0.87), richer (AOR = 0.77; 95% CrI: 0.73, 0.81), and richest women (AOR = 0.59; 95% CrI: 0.55, 0.63) all had decreased odds of early PNC service uptake as wealth increased. Women with media exposure (AOR = 1.32; 95% CrI: 1.27, 1.36) had 32% higher odds of early PNC service uptake than those without media exposure. Women with 3–5 children (AOR = 0.89; 95% CrI: 0.84, 0.94) and those with more than 5 children (AOR = 0.69; 95% CrI: 0.64, 0.75) had lower odds of early PNC service uptake compared to those with one to two children. Women with an age at first birth <20 years (AOR = 0.82; 95% CrI: 0.79, 0.84) had slightly lower odds of early PNC service uptake compared to those with an age at first birth ≥20 years. Women with at least one ANC visit (AOR = 1.93, 95% CrI: 1.8, 2.08) had 93% higher odds of early PNC service uptake than those without an ANC visit. Women delivering in health facilities (AOR = 2.57; 95% CrI: 2.46, 2.68) were 2.57 times more likely to receive early PNC service uptake than those at home. Women with a child who had an average birth size (AOR = 0.94; 95% CrI: 0.91, 0.98) and small birth size (AOR = 0.88; 95% CrI: 0.84, 0.92) had slightly lower odds of early PNC service uptake compared to those with large birth size. Women with twins (AOR = 1.15; 95% CrI: 1.02, 1.3) had 15% higher odds of early PNC service uptake than those without twins. Fourth- or above-born children (AOR = 0.88; 95% CrI: 0.82, 0.95) had slightly lower odds of early PNC service uptake compared to first-born children. Regarding community-level variables, rural women (AOR = 0.76; 95% CrI: 0.72, 0.79) had lower odds of early PNC service uptake by 24% compared to urban women. Communities with high media exposure (AOR = 1.1; 95% CrI: 1.04, 1.18) had 10% higher odds of early PNC service uptake than their counterparts. Communities with high wealth levels (AOR = 0.88; 95% CrI: 0.83, 0.94) had 12% lower odds of early PNC service uptake. Communities with high ANC utilization (AOR = 1.13; 95% CrI: 1.07, 1.19) had 13% higher odds of early PNC service uptake than those with low ANC utilization. Communities in which the distance to a health facility is not a big problem (AOR = 1.5; 95% CrI: 1.38, 1.63) had 50% higher odds of early PNC service uptake than communities where it is a big problem (Table 5).
Discussion
Using the Bayesian multilevel modeling approach, the goal of this study was to pinpoint the underlying individual and community-level factors that contributed to the PNC uptake among mothers immediately following birth within 24–48 h. Based on our final model analysis, we found that the pooled prevalence of PNC following birth within 2 days of the delivery was about 52% (95% CrI: 39, 66). Although this pooled prevalence was still low, however, it has been shown to have approximately 20% increase as compared to the previous research subgroup analysis findings in SSA (44). In comparison to the prevalence recorded in Ethiopia (15.71%) (45), and Gambia (46), this prevalence was greater. Although the prevalence of PNC has increased over time in East Africa, this number is not very spectacular when compared to other countries such as Bangladesh (63%) (47), Benin (68%) (48), and Zambia (63%) (49). These differences may reflect the variety of country-specific factors, analysis model type, sampling, and other methodological changes, as well as the effectiveness of the health care system delivering mother and child care.
In this study, educated and older mothers have more PNC than their counterparts. According to a study conducted in SSA, Vietnam, and China, educated and older mothers have more PNC than their counterparts (44, 50, 51). Several previous studies have shown that a mother’s education and age have a significant impact on a child’s health (52). It is closely linked to her knowledge of health, which influences her use of maternal and child health services. Women with higher levels of education and age tend to have better knowledge about maternal health, enabling them to make informed decisions and seek appropriate medical care, including maternal health services. Higher education and being aged are associated with improved health-seeking behaviors, being more susceptible to maternal- and pregnancy-related complications, and are seen as a crucial way to empower women to access maternal healthcare services (53, 54). Therefore, the evidence suggests that educated and older women are more likely to have better knowledge and utilize maternal health services because they understand the importance of these services and can make informed choices.
When enabling factors are taken into account, mothers who had at least one ANC visit and gave birth in health facilities had a higher chance of getting a PNC checkup than their counterparts, which is supported by earlier research from India (55), Pakistan (56), Ethiopia (45, 57), Bangladesh (47) as well as Nigeria (58), and Uganda (59). This finding could be explained by the fact that pregnant women who receive counseling and information about the health risks of the postpartum period during ANC visits are more likely to use adequate PNC services, which maintains their advantageous position regardless of their socioeconomic status. This finding might be explained by the fact that pregnant women who are exposed to counseling and information about the health risks of the postpartum period during ANC visits and during their delivery at medical facilities are more likely to use adequate PNC services, which maintain their advantageous position regardless of their socioeconomic status. Additionally, these women who gave birth in a hospital might be allowed to stay for an additional 2 days or advised to have a follow-up appointment 2 days after giving birth so that they can receive the proper medical attention for both themselves and their newborns. Mothers who give birth at home typically face cultural restrictions that prevent them from leaving their homes for a certain amount of time, exhibit negative attitudes, and use fewer postpartum services.
Although previous research did not provide specific explanations for why widowed mothers show less health-seeking behavior for themselves and their children, it can be inferred from the broader literature that the loss of a spouse has a significant impact on a woman’s motivation and ability to seek healthcare. This impact includes increased levels of depression, anxiety, and psychological distress, which can affect a woman’s willingness to access healthcare for herself and her children (60). Additionally, widowed mothers often lack social support, making it more difficult for them to seek healthcare (60). Moreover, the death of a spouse can lead to economic hardships, which further hinder a woman’s ability to access healthcare for herself and her children. Furthermore, in some communities, widows may face stigmatization or discrimination, which can negatively influence their healthcare-seeking behavior (61). While the specific reasons for the lower health-seeking behavior among widowed mothers may vary depending on individual circumstances and cultural contexts, it is crucial to offer targeted support and interventions to address the unique challenges faced by this population.
The majority of the studies have investigated those urban women who had more PNC than rural women due to the unequaled distribution of sources and various factors of women. Several factors could explain this difference. One reason is that rural areas often have limited transportation options and poor road infrastructure (62). This makes it difficult for women to travel to healthcare facilities for PNC visits. Additionally, rural women may have less information about PNC services and their importance, which can lead to lower utilization rates (62). Rural women may also face more socioeconomic challenges, such as poverty and a lack of resources, which can prevent them from seeking PNC services. Furthermore, rural areas typically have fewer healthcare facilities and resources, resulting in longer travel and wait times for PNC services, and women may face challenges in attending PNC visits due to the distance of the nearest health facility from their homes (63). Finally, in some rural communities, there may be cultural or social norms that discourage women from seeking PNC services (64). For example, there might be a preference for traditional birth attendants or a belief that PNC visits are unnecessary. All these could be caused by differences in the accessibility and availability of healthcare facilities, as well as the caliber of healthcare provided in the various regions.
This study showed that mothers who come from areas with high levels of media exposure and who frequently consume any form of media, including radio and television, are more likely to use PNC from any provider, regardless of qualification, than their counterparts who are not exposed to any media. This outcome is consistent with the research performed in Bangladesh (47), Uganda (59), Ethiopia (65), and Nigeria (66), where only qualified providers were considered. The fact that highly educated women who are already aware of the seeking behavior are more likely to be exposed to media can be used to explain this better occurrence. This demonstrates how the country can improve communication hurdles, PNC use, and other health services by leveraging the power of media.
Compared to those at parity 1, women at parity 4 and more had reduced probabilities of using PNC for their offspring. The most likely explanation is that women with higher parities may rely on the knowledge they gained from prior PNC visits. Studies from Uganda and Bangladesh have shown their agreement with this figure (47, 59). On the contrary, it is believed that a variety of obligations, such as raising younger and older children, frequently prevent women from seeking PNC, particularly in situations where access to a health facility is regarded to be difficult, mainly if they have more children and have not used contraception.
Compared to women who had single infants, twin mothers were more likely to seek PNC. Utilizing PNC services is crucial since they provide health professionals with a platform to identify and treat problems affecting expectant mothers and newborns. Women must use these services as a result, regardless of the manner of birth. Perhaps the stigma associated with twin pregnancies pushes women to seek PNC; however, more research on twin pregnancies and PNC uptake is required to fully understand the phenomena. Currently, studies from Bangladesh and Papua New Guinea have discovered a comparable number (47, 67). In the same way, if their babies are overweight or have obesity-like tendencies that are different from the babies they knew before and around them, they do not consider it as a good opportunity, so they are more likely to go to the health facility for a checkup.
This study found that wealthier and employed mother have a less likelihood chance of having PNC within 2 days following birth compared to their counterparts. According to a study conducted in Vietnam and SSA, women in wealthier households were significantly associated with postnatal health checks for their newborns. The proportion of postnatal health checks increased among households of near-poor, middle, rich, and richest as compared with the poorest households (44, 50). Although the author did not find earlier evidence, several factors could explain why wealthier and employed mothers may not return to health facilities within 2 days after giving birth, compared to poor and unemployed women. These factors include access to underreported private healthcare, self or relative postpartum support, dissatisfaction, and cultural or personal preferences. Wealthier women may have other options for postpartum care, such as home visits from healthcare providers. Cultural or personal preferences may also play a role in when women choose to visit health facilities after giving birth. Additionally, employed mothers may face challenges in taking time off work for these visits. These factors contribute to the disparities in postpartum health facility visits among different socioeconomic groups. Further research and data analysis on this topic would provide a more comprehensive understanding of the underlying reasons.
Strengths and limitations of the study
Regarding the study’s advantages, East African countries’ nationally representative data, which is indicative of the entire nation, is used. To offer a more believable conclusion that considers the hierarchical nature of the survey data by incorporating prior information, this study also used a Bayesian multilevel modeling technique and sizable samples. This will improve the estimation of the results toward the reality of the country’s current status. The study does have some flaws, though. Due to the self-reported nature of the interview, the survey is subject to social desirability, and the cross-sectional form of the study may not be able to account for the temporal connection of the independent and outcome variables. Furthermore, although there was a relatively short time variation among the countries in the dataset, time was not considered an independent factor in the regression. Therefore, readers should keep this in mind when interpreting and using the findings of this study.
Conclusion, recommendations, and implications of the study
Low utilization of immediate PNC uptake remains among the top public health importance agendas in East Africa. In the final model, the study at hand revealed several crucial individual and community-level factors, including older maternal age, higher education levels, being divorced/widowed women, better household wealth index, maternal working status, having mass media exposure, higher parity, having ANC follow up, institutional delivery, age at first birth, twin status, child weight at birth, and birth order were individual-level factors that have been independently associated with early PNC uptake. Similarly, regarding community-level factors, variables such as high community mass media exposure, being from a rural area, being from high ANC follow-up coverage communities, being from high-wealth communities and having a long distance to the health facilities were associated with the outcome variable, respectively, among women in East Africa.
Hence, targeted education and awareness campaigns are crucial. Given the positive correlation between higher education levels and early PNC uptake, it is important to implement campaigns that specifically target women with lower levels of education. These campaigns should emphasize the benefits of PNC and highlight the importance of seeking PNC services early in the postnatal period. Additionally, they should address any misconceptions or barriers that may exist. Similarly, it is important to strengthen ANC and institutional delivery services. The study has identified ANC follow-up and institutional delivery as factors that are positively associated with early PNC uptake. By improving access to quality ANC services, ensuring the presence of skilled birth attendants, and establishing adequate referral systems, the likelihood of women receiving timely PNC can be increased. Furthermore, community-based interventions are also crucial. The study has found that community-level factors, such as mass media exposure and ANC follow-up coverage, can influence early PNC uptake. Therefore, implementing community health education programs and utilizing mass media platforms to disseminate PNC-related information can help improve awareness and promote early PNC-seeking behaviors. The future research should explore how the identified factors influence early PNC uptake and evaluate the effectiveness of interventions targeting these factors. Longitudinal studies could provide insights into the long-term impact of early PNC uptake on maternal and child health outcomes. Overall, the study underscores the importance of addressing both individual and community factors to enhance early PNC uptake in East Africa. By implementing targeted interventions and policies, healthcare systems can ensure that all women have equal access to timely and high-quality PNC services, improving maternal and child health outcomes.
Data availability statement
The raw data supporting the conclusions of this article will be made available by the authors, without undue reservation.
Ethics statement
Ethical approval and a letter of permission were requested online at www.dhsprogram.com, and the DHS program was given permission through email to access the data for this study. This study made use of freely accessible data that was completely devoid of any personal information. The East African countries DHS provided the secondary data used in the study. Concerns about informed consent, confidentiality, anonymity, and privacy of the study sample were ethically addressed by the DHS authorities, and we did not alter or utilize the data in any other way. The study’s data set was publicly accessible and devoid of any private information. Both patients and the general public were not involved in this investigation. The studies were conducted in accordance with the local legislation and institutional requirements.
Author contributions
BT: Conceptualization, Data curation, Formal analysis, Investigation, Methodology, Project administration, Resources, Software, Validation, Visualization, Writing – original draft. DA: Investigation, Methodology, Resources, Software, Supervision, Validation, Writing – review & editing. FA: Data curation, Formal analysis, Funding acquisition, Investigation, Writing – review & editing. SA: Data curation, Formal analysis, Funding acquisition, Investigation, Supervision, Validation, Visualization, Writing – review & editing. TA: Funding acquisition, Investigation, Methodology, Project administration, Resources, Supervision, Validation, Visualization, Writing – review & editing.
Funding
The author(s) declare that no financial support was received for the research, authorship, and/or publication of this article.
Acknowledgments
The study’s ethical consideration was acknowledged by the authors as being handled by the DHSs Program office.
Conflict of interest
The authors declare that the research was conducted in the absence of any commercial or financial relationships that could be construed as a potential conflict of interest.
Publisher’s note
All claims expressed in this article are solely those of the authors and do not necessarily represent those of their affiliated organizations, or those of the publisher, the editors and the reviewers. Any product that may be evaluated in this article, or claim that may be made by its manufacturer, is not guaranteed or endorsed by the publisher.
Supplementary material
The Supplementary material for this article can be found online at: https://www.frontiersin.org/articles/10.3389/fpubh.2024.1439280/full#supplementary-material
References
1. Howden-Chapman, P, Siri, J, Chisholm, E, Chapman, R, and Doll, CN. Capon AJAgtSifstiP, France: ensure healthy lives and promote wellbeing for all at all ages. Int Council Sci. (2017):81–126.
2. WHO . Standards for improving quality of maternal and newborn care in health facilities. Geneva: WHO (2016).
3. UNICEF . The state of the world's children 2009: Maternal and newborn health. New York, NY: UNICEF (2008).
4. WHO . WHO recommendations on maternal and newborn care for a positive postnatal experience. Geneva: WHO (2022).
5. WHO . Pocket book of hospital care for children: Guidelines for the management of common childhood illnesses. Geneva: WHO (2013).
6. WHO Guidelines Approved by the Guidelines Review Committee . WHO recommendations on postnatal Care of the Mother and Newborn. Geneva: World Health Organization (2013).
7. WHO Guidelines Approved by the Guidelines Review Committee . Home visits for the newborn child: A strategy to improve survival. Geneva: World Health Organization (2009).
8. Nations U . (2020). The sustainable development goals report 2020. Available at: https://unstats.un.org/sdgs/report/2020/The-Sustainable-Development-Goals-Report-2020.pdf
9. Nations U . Progress towards the sustainable development goals report of the secretary- general 2019. Geneva: United Nations (2019).
10. Langlois, EV, Miszkurka, M, Ziegler, D, Karp, I, and Zunzunegui, MV. Protocol for a systematic review on inequalities in postnatal care services utilization in low- and middle-income countries. Syst Rev. (2013) 2:55. doi: 10.1186/2046-4053-2-55
11. Madaj, B, Smith, H, Mathai, M, Roos, N, and van den Broek, N. Developing global indicators for quality of maternal and newborn care: a feasibility assessment. Bull World Health Organ. (2017) 95:445–452I. doi: 10.2471/BLT.16.179531
12. Organization WH . Trends in maternal mortality 2000 to 2017: estimates by WHO, UNICEF, UNFPA, World Bank Group and the United Nations population division: executive summary. Geneva: World Health Organization (2019).
13. McMahon, SA, George, AS, Chebet, JJ, Mosha, IH, Mpembeni, RN, and Winch, PJ. Experiences of and responses to disrespectful maternity care and abuse during childbirth; a qualitative study with women and men in Morogoro region. Tanzania BMC Preg Childbirth. (2014) 14:268. doi: 10.1186/1471-2393-14-268
14. World Bank . World Bank data. Mortality rate, under-5 (per 1,000 live births). Washington, DC: World Bank (2019).
15. WHO . UNFPA, the World Bank and the United Nations population division. Trends in maternal mortality: 1990 to 2013 estimates by WHO, UNICEF, UNFPA, the World Bank and the United Nations population division. Geneva: World Health Organization (2014).
16. Bhutta, ZA, Das, JK, Bahl, R, Lawn, JE, Salam, RA, Paul, VK, et al. Can available interventions end preventable deaths in mothers, newborn babies, and stillbirths, and at what cost? Lancet. (2014) 384:347–70. doi: 10.1016/S0140-6736(14)60792-3
17. Okawa, S, Ansah, EK, Nanishi, K, Enuameh, Y, Shibanuma, A, Kikuchi, K, et al. High incidence of neonatal danger signs and its implications for postnatal Care in Ghana: a cross-sectional study. PLoS One. (2015) 10:e0130712. doi: 10.1371/journal.pone.0130712
18. Chalise, B, Chalise, M, Bista, B, Pandey, AR, and Thapa, S. Correlates of continuum of maternal health services among Nepalese women: evidence from Nepal multiple Indicator cluster survey. PLoS One. (2019) 14:e0215613. doi: 10.1371/journal.pone.0215613
19. Shibanuma, A, Yeji, F, Okawa, S, Mahama, E, Kikuchi, K, Narh, C, et al. The coverage of continuum of care in maternal, newborn and child health: a cross-sectional study of woman-child pairs in Ghana. BMJ Glob Health. (2018) 3:e000786. doi: 10.1136/bmjgh-2018-000786
20. Wang, W, and Hong, R. Levels and determinants of continuum of care for maternal and newborn health in Cambodia-evidence from a population-based survey. BMC Pregnancy Childbirth. (2015) 15:62. doi: 10.1186/s12884-015-0497-0
21. Iqbal, S, Maqsood, S, Zakar, R, Zakar, MZ, and Fischer, F. Continuum of care in maternal, newborn and child health in Pakistan: analysis of trends and determinants from 2006 to 2012. BMC Health Serv Res. (2017) 17:189. doi: 10.1186/s12913-017-2111-9
22. Yeji, F, Shibanuma, A, Oduro, A, Debpuur, C, Kikuchi, K, Owusu-Agei, S, et al. Continuum of Care in a Maternal, newborn and child health program in Ghana: low completion rate and multiple obstacle factors. PLoS One. (2015) 10:e0142849. doi: 10.1371/journal.pone.0142849
23. Sakuma, S, Yasuoka, J, Phongluxa, K, and Jimba, M. Determinants of continuum of care for maternal, newborn, and child health services in rural Khammouane, Lao PDR. PLoS One. (2019) 14:e0215635. doi: 10.1371/journal.pone.0215635
24. Singh, K, Story, WT, and Moran, AC. Assessing the continuum of care pathway for maternal health in South Asia and sub-Saharan Africa. Matern Child Health J. (2016) 20:281–9. doi: 10.1007/s10995-015-1827-6
26. Croft, TN, Marshall, AM, Allen, CK, Arnold, F, Assaf, S, and Balian, S. Guide to DHS statistics. Rockville, MD: ICF (2018).
27. Union, A. Report on sustainable development goals for the eastern Africa subregion. (2015) Addis Ababa, Ethiopia: United Nations Economic Commission for Africa.
28. Gambia Bureau of Statistics – GBoS, ICF . The Gambia demographic and health survey 2019–20. Banjul: GBoS/ICF (2021).
29. WHO . WHO recommendations on postnatal care of the mother and newborn. Geneva: World Health Organization (2014).
30. Williams, DR, Rast, P, and Bürkner, P-C. Bayesian meta-analysis with weakly informative prior distributions. (2018).
31. Lesaffre, E, and Lawson, A. Statistics in practice: Bayesian biostatistics. Somerset, NJ: Wiley (2012).
32. Correa-Álvarez, CD, Salazar-Uribe, JC, and Pericchi-Guerra, LR. Bayesian multilevel logistic regression models: a case study applied to the results of two questionnaires administered to university students. Comput Stat. (2023) 38:1791–810. doi: 10.1007/s00180-022-01287-4
33. Draper, D. Bayesian multilevel analysis and MCMC. Handbook of multilevel analysis. Cham: Springer (2008), p. 77–139.
34. Gelman, A, Carlin, JB, Stern, HS, and Rubin, DB. Bayesian data analysis. London: Chapman and Hall/CRC (1995).
35. Hoffman, MD, and Gelman, A. The no-U-turn sampler: adaptively setting path lengths in Hamiltonian Monte Carlo. J Mach Learn Res. (2014) 15:1593–623.
36. Bürkner, P-C . Advanced Bayesian multilevel modeling with the R package BRMS. (2018) New Zealand: University of Auckland.
37. Vehtari, A, Gelman, A, and Gabry, J.. Practical Bayesian model evaluation using leave one-out cross-validation and WAIC. Stat Comput. (2017) 27:1413–32. doi: 10.1007/s11222-016-9696-4
38. Gelman, A, Hwang, J, and Vehtari, A. Understanding predictive information criteria for Bayesian models. Springer Nature (2014), 24.
39. Kruschke, J . Doing Bayesian data analysis: a tutorial with R, JAGS, and Stan. Academic Press (2014).
40. Vehtari, A, Gelman, A, Simpson, D, Carpenter, B, and Bürkner, PC. Rank-normalization, folding, and localization: an improved Rhat for assessing convergence of MCMC. International Society for Bayesian Analysis (ISBA). Bayesian Analysis (2019) arXiv.
41. Hesketh, SR, and Skrondal, A. Multilevel and longitudinal modeling using. Stata: STATA press (2021).
42. Larsen, K, and Merlo, J. Appropriate assessment of neighborhood effects on individual health: integrating random and fixed effects in multilevel logistic regression. Am J Epidemiol. (2005) 161:81–8. doi: 10.1093/aje/kwi017
44. Benova, L, Owolabi, O, Radovich, E, Wong, KL, Macleod, D, Langlois, EV, et al. Provision of postpartum care to women giving birth in health facilities in sub-Saharan Africa: a cross-sectional study using demographic and health survey data from 33 countries. PLoS Med. (2019) 16:e1002943. doi: 10.1371/journal.pmed.1002943
45. Kassie, DG, Assimamaw, NT, Tamir, TT, Alemu, TG, Techane, MA, Wubneh, CA, et al. Spatial distribution and determinants of new borns not receiving postnatal check-up within two days after birth in Ethiopia: a spatial and multilevel analysis of EDHS 2016. BMC Pediatr. (2022) 22:495. doi: 10.1186/s12887-022-03506-9
46. Kinteh, B, Barrow, A, Nget, M, Touray, E, Touray, J, Kinteh, SL, et al. Maternal and child health Services in Rural Settings of the Gambia: contextual determinants of postnatal care from mothers’ perspectives—a community-based analytical cross-sectional study. Adv. Pub. Health. (2022) 2022:1–7. doi: 10.1155/2022/3558676
47. Sheba, IT, Sarker, AR, and Tasnim, A. Factors associated with post-Natal Care utilization among the women of reproductive age group: evidence from Bangladesh demographic and health survey 2017–18. Health Services Res. Manage. Epidemiol. (2022) 9:23333928221136393. doi: 10.1177/23333928221136393
48. Dansou, J, Adekunle, AO, and Arowojolu, AO. Factors associated with antenatal care services utilisation patterns amongst reproductive age women in Benin Republic: an analysis of 2011/2012 Benin Republic's demographic and health survey data. Nigerian Postgraduate Med. J. (2017) 24:67–74. doi: 10.4103/npmj.npmj_16_17
49. Chungu, C, Makasa, M, Chola, M, and Jacobs, CN. Place of delivery associated with postnatal care utilization among childbearing women in Zambia. Front Public Health. (2018) 6:94. doi: 10.3389/fpubh.2018.00094
50. Lam, ND, Anh, ND, Ha, NTT, Vinh, TQ, Anh, VTM, and Kien, VD. Socioeconomic inequalities in post-natal health checks for the newborn in Vietnam. Int J Equity Health. (2019) 18:1–7. doi: 10.1186/s12939-019-1029-8
51. Yang, Y, and Yu, M. Disparities and determinants of maternal health services utilization among women in poverty-stricken rural areas of China: a cross-sectional study. BMC Pregnancy Childbirth. (2023) 23:1–14. doi: 10.1186/s12884-023-05434-7
52. Paul, S, Paul, S, Gupta, AK, and James, K. Maternal education, health care system and child health: evidence from India. Soc Sci Med. (2022) 296:114740. doi: 10.1016/j.socscimed.2022.114740
53. Wang, H, Frasco, E, Takesue, R, and Tang, K. Maternal education level and maternal healthcare utilization in the Democratic Republic of the Congo: an analysis of the multiple indicator cluster survey 2017/18. BMC Health Serv Res. (2021) 21:1–13. doi: 10.1186/s12913-021-06854-x
54. Taye, BT, Kebede, AA, and Wondie, KY. Intention to use maternal health services and associated factors among women who gave birth at home in rural Sehala Seyemit district: a community-based cross-sectional study. BMC Pregnancy Childbirth. (2022) 22:213. doi: 10.1186/s12884-022-04447-y
55. Krishnamoorthy, Y, and Majella, MG. Determinants of postnatal care coverage among mothers and new-borns in India: evidence from a nationally representative survey. Int J Health Plann Manag. (2021) 36:1276–86. doi: 10.1002/hpm.3179
56. Yunus, A, Iqbal, S, Munawar, R, Zakar, R, Mushtaq, SK, Sadaf, F, et al. Determinants of postnatal care services utilization in Pakistan-insights from Pakistan demographic and health survey (PDHS) 2006-07. Middle-East J Sci Res. (2013) 18:1440–7. doi: 10.5829/idosi.mejsr.2013.18.10.12418
57. Teshale, AB, Tesema, GA, Yeshaw, Y, Tesema, AK, Alem, AZ, and Liyew, AM. Individual and community level factors associated with delayed first postnatal care attendance among reproductive age group women in Ethiopia. BMC Pregn. Childbirth. (2021) 21:1–8. doi: 10.1186/s12884-020-03523-5
58. Oluwaseyi, SD . Determinants of postnatal care non-utilization among women in Nigeria. Johannesburg: University of the Witwatersrand, Faculty of Humanities (2014).
59. Ndugga, P, Namiyonga, NK, and Sebuwufu, D. Determinants of early postnatal care attendance: analysis of the 2016 Uganda demographic and health survey. BMC Pregnancy Childbirth. (2020) 20:163. doi: 10.1186/s12884-020-02866-3
60. Holm, AL, Berland, AK, and Severinsson, E. Factors that influence the health of older widows and widowers—a systematic review of quantitative research. Nurs Open. (2019) 6:591–611. doi: 10.1002/nop2.243
61. Akter, S, Azad, T, Rahman, MH, and Raihan, MF. Morbidity patterns and determinants of healthcare-seeking behavior among older women in selected rural areas of Bangladesh. Dr Sulaiman Al Habib Medical Journal. (2023) 5:70–9. doi: 10.1007/s44229-023-00033-2
62. Amsalu, G, Talie, A, Gezimu, W, and Duguma, A. Non-utilization of postnatal care and its associated factors among women who gave birth in rural districts of northern Ethiopia: a community-based mixed-method study. Womens Health. (2022) 18:17455057221125091. doi: 10.1177/17455057221125091
63. Kanté, AM, Chung, CE, Larsen, AM, Exavery, A, Tani, K, and Phillips, JF. Factors associated with compliance with the recommended frequency of postnatal care services in three rural districts of Tanzania. BMC Pregnancy Childbirth. (2015) 15:1–10. doi: 10.1186/s12884-015-0769-8
64. Akhter, S, Dasvarma, GL, and Saikia, U. Reluctance of women of lower socio-economic status to use maternal healthcare services–does only cost matter? PLoS One. (2020) 15:e0239597. doi: 10.1371/journal.pone.0239597
65. Ayele, BG, Woldu, MA, Gebrehiwot, HW, Gebre-Egziabher, EG, Gebretnsae, H, Hadgu, T, et al. Magnitude and determinants for place of postnatal care utilization among mothers who delivered at home in Ethiopia: a multinomial analysis from the 2016 Ethiopian demographic health survey. Reprod Health. (2019) 16:1–10. doi: 10.1186/s12978-019-0818-2
66. Agho, KE, Ezeh, OK, Issaka, AI, Enoma, AI, Baines, S, and Renzaho, AM. Population attributable risk estimates for factors associated with non-use of postnatal care services among women in Nigeria. BMJ Open. (2016) 6:e010493. doi: 10.1136/bmjopen-2015-010493
67. Appiah, F, Fenteng, JOD, Dare, F, Salihu, T, Darteh, AO, Takyi, M, et al. Understanding the determinants of postnatal care uptake for babies: a mixed effects multilevel modelling of 2016–18 Papua New Guinea demographic and health survey. BMC Pregnancy Childbirth. (2021) 21:1–13. doi: 10.1186/s12884-021-04318-y
68. R Core Team . (2023a). R: A Language and Environment for Statistical Computing. R Foundation for Statistical Computing. Available at: https://www.r-project.org
69. R Core Team . (2023b). R 4.3.2 Release Notes. Available at: https://cran.r-project.org/doc/manuals/r-release/NEWS.html#R-4-3-2
70. Bürkner, PC. brms: An R Package for Bayesian Multilevel Models using Stan. J Stat Softw. (2017) 80:1–28. doi: 10.18637/jss.v080.i01
71. Bürkner, PC. brms: An R Package for Bayesian Multilevel Models using Stan. GitHub repository. (2023b). Available at: https://github.com/paul-buerkner/brms
72. Bürkner, PC. brms: An R Package for Bayesian Multilevel Models using Stan. CRAN Repository. (2023a). Available at: https://cran.r-project.org/web/packages/brms/index.html
73. Gelman, A., and Rubin, DB. Inference from Iterative Simulation Using Multiple Sequences. Stat. Sci. (1992) 7:457–472. doi: 10.1214/ss/1177011136
Keywords: Bayesian hierarchical model, East Africa, factors, newborns, postnatal care, women
Citation: Terefe B, Asgedom DK, Arage FG, Aychiluhm SB and Ayele TA (2024) Early postnatal care uptake and its associated factors following childbirth in East Africa—a Bayesian hierarchical modeling approach. Front. Public Health. 12:1439280. doi: 10.3389/fpubh.2024.1439280
Edited by:
Lihua Ren, Peking University, ChinaReviewed by:
Gemeda Wakgari Kitil, Mattu University, EthiopiaFlorence Mbuthia, Dedan Kimathi University of Technology, Kenya
Copyright © 2024 Terefe, Asgedom, Arage, Aychiluhm and Ayele. This is an open-access article distributed under the terms of the Creative Commons Attribution License (CC BY). The use, distribution or reproduction in other forums is permitted, provided the original author(s) and the copyright owner(s) are credited and that the original publication in this journal is cited, in accordance with accepted academic practice. No use, distribution or reproduction is permitted which does not comply with these terms.
*Correspondence: Bewuketu Terefe, d29sZGVhYndvbWFyaWFtQGdtYWlsLmNvbQ==