- 1Department of Pharmacy Practice, Nims Institute of Pharmacy, Nims University Rajasthan, Jaipur, India
- 2School of Health Sciences, Faculty of Biology, Medicine and Health, Manchester Academic Health Science Centre, University of Manchester, Manchester, United Kingdom
- 3Public Health and Preventive Medicine, University of Alberta, Edmonton, AB, Canada
- 4Department of Biochemistry, National Institute of Medical Sciences and Research, Nims University Rajasthan, Jaipur, India
- 5Department of Endocrinology, National Institute of Medical Sciences and Research Nims University Rajasthan, Jaipur, India
- 6Department of Clinical Studies, Fourth Hospital of Yulin (Xingyuan), Yulin, Shaanxi, China
- 7Department of Clinical Sciences, Shenmu Hospital, Shenmu, Shaanxi, China
- 8Institute of Pediatric Gastroenterology and Hepatology, National Institute of Medical Sciences and Research, Nims University Rajasthan, Jaipur, India
Background: The emergence of the COVID-19 pandemic created an unprecedented global health crisis, resulting in major disruptions to healthcare systems worldwide. The pandemic has also significantly reshaped healthcare utilization patterns. This study aimed to assess healthcare utilization during the three waves of COVID-19.
Methods: We conducted a retrospective study involving 1,308 patients admitted to the COVID-19 care facility at the National Institute of Medical Sciences and Research in Jaipur, Rajasthan, India. The study analyzed healthcare utilization patterns during the first, second, and third waves of COVID-19, focusing on patient hospitalization duration across the three waves.
Results: The hospitalization rate increased during the second wave compared to the first wave and subsequently declined in the third wave. Hospitalization durations varied significantly across the waves. In all three waves, 30% of the population was hospitalized for 0–5 days, 25.9% for 9–13 days, 24.08% for 6–8 days, and 19.5% of patients were hospitalized for more than 14 days. A p-value of 0.032 indicated a statistically significant difference in length of hospital stay (LHS) across the three waves of COVID-19. A threshold p-value of 0.05 was used to assess healthcare utilization and to estimate future healthcare requirements for similar pandemic scenarios.
Conclusion: Our findings highlight the dynamic nature of healthcare demands during pandemic waves and underscore the need for flexible healthcare systems capable of adapting to fluctuating patient loads. Proactive planning and resource allocation are crucial to managing future pandemics effectively.
Introduction
The COVID-19 pandemic has had a profound impact on healthcare systems globally. The initial outbreak was first detected in Wuhan, China. As the virus’s behavior in China was studied, other countries began preparing for the impending waves of the pandemic. However, many nations, including India, lacked sufficient time to prepare adequately. In response to the pandemic’s waves, India implemented various healthcare and treatment strategies (1–3).
During the first wave, the rapid spread of the virus, coupled with uncertainty about treatment and outcomes, put immense pressure on India’s healthcare system (4). Hospitals prioritized COVID-19 care to manage the surge in patients, while non-essential medical procedures were postponed or avoided by patients (5).
The second wave brought additional challenges, severely disrupting healthcare services across the country. Shortages of medical supplies, an inadequate number of healthcare personnel, and overwhelmed hospitals led to increased mortality and morbidity rates from COVID-19 (6). In response, hospitals began streamlining resources and implementing standard operating procedures. This allowed the country to address healthcare challenges more effectively by expanding local facilities, advancing telemedicine, and integrating e-health services (7, 8).
With the onset of the third wave, the emergence of new SARS-CoV-2 variants introduced further complexities in healthcare management. The availability and distribution of vaccines, alongside vaccine hesitancy, significantly influenced healthcare decisions. The evolution of additional variants highlighted the importance of timely and appropriate care-seeking behaviors (9, 10).
To date, no comprehensive study has compared healthcare utilization across the three waves of COVID-19 in terms of hospital stay durations, admission units, and medication regimens. Such research is crucial to understanding the full burden of the pandemic and providing insights that can improve healthcare preparedness for future crises.
This study aims to analyze the factors influencing healthcare utilization patterns during the three waves of COVID-19 in India, with a focus on hospital admissions, and the determinants of Health Care Utilization (HCU). With this, a clearer understanding of the pandemic’s impact on hospital admissions and guide improvements in future healthcare responses.
Methodology
Study design
The acute COVID care unit of National Institute of Medical Sciences and Research, Nims Hospital Rajasthan, Jaipur, India admitted 1,620 COVID-19 patients over the three waves of pandemic. We included data of the patients admitted during the first, second, and third waves.
Data collection
A retrospective analysis was performed to investigate the healthcare utilization of hospitalized COVID-19 patients during the first wave (March–November 2020), the second wave (March–May 2021), and the third wave (January–February 2022) of the pandemic (11). This assessment was carried out utilizing records from the Medical Record Department (MRD) of the Nims Hospital, Rajasthan, Jaipur, India. The Nims hospital admitted 1,000 RT-PCR-positive patients in the first wave, 597 in the second wave, and only 23 in the third wave. We extracted medical information from 1,620 patients and assessed them for research inclusion and exclusion criteria. Of them, we found 1,308 to be eligible for the study. A total of 42 (3.21%) admitted patients had missing dataset, participants with missing values were excluded entirely from the analysis.
All symptomatic and asymptomatic patients with a positive RT-PCR report were included in the study, while patients with incomplete data and those who died during their hospital stay were excluded.
The retrospective data for these patients were acquired using a data collecting form and then entered into a Microsoft Excel spreadsheet. The data included demographic information such as age, gender, and address type (urban versus rural), as well as clinical information such as duration of hospital stay (LHS), High-resolution computed tomography (HRCT) score categorized into mild (<8), moderate (9–15), and severe levels (16–25) (12), Admission unit including ICU, CCU, General ward, Deluxe and super deluxe and Isolation ward, Comorbidities such as diabetes, hypertension, dyslipidemia, respiratory disease were documented based on the patient history, treatments including Corticosteroids, Hydroxychloroquine, Monoclonal antibodies, and Antiviral drugs such as Remdesivir, Favipiravir, Molnupiravir, Daclizumab, Bevacizumab. The age of patient population were grouped into 5 and length of hospital (LHS) stay into 4 categories (refer Tables 1, 2).
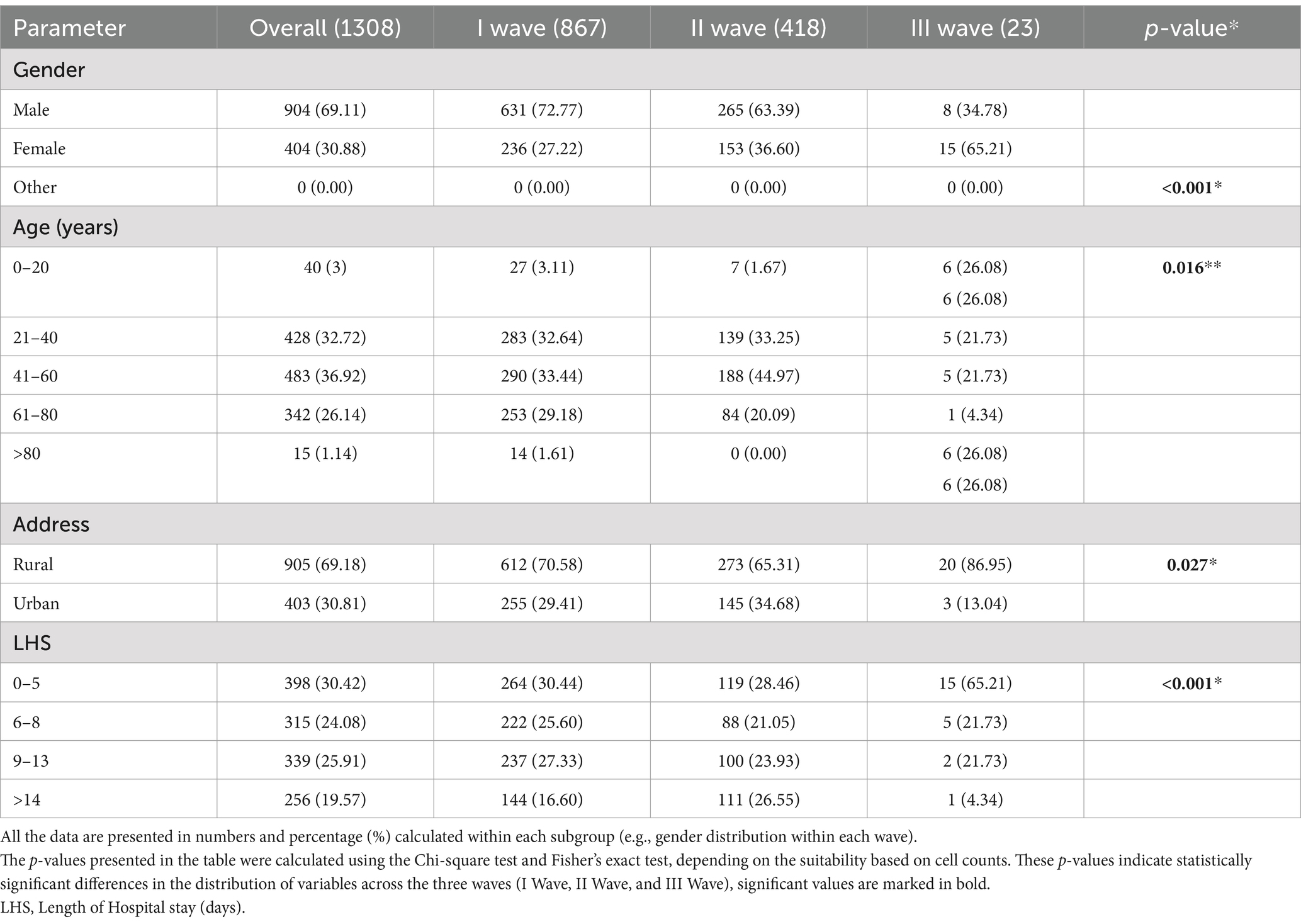
Table 1. Demographic characteristics of 1,308 patients diagnosed with COVID-19 across all the three waves.
Data analysis
We analyzed the data with IBM SPSS (version 26; IBM, Armonk, NY, USA). We expressed all categorical variables in percentages and analyzed them using the chi-square test or Fisher exact test to identify significant differences between the first, second, and third waves calculated within each subgroup (e.g., gender distribution within each wave). A p-value of <0.05 was considered statistically significant. We used Poisson analysis to investigate the relationships between demographic characteristics (age, gender, comorbidities) and the occurrence of COVID-19 over three waves as a dependent variable. Multivariable Poisson regression is a type of generalized linear model (GLM), this model assumes that the dependent variable (COVID-19 occurrence) follows a poisson distribution. Since the study spans three COVID-19 waves, poisson regression is useful in assessing whether the incidence of cases changed significantly over time while adjusting for demographic variables. All graphical representations were made using Microsoft Excel version 2019.
Results
A total of 1,620 cases of COVID 19 were admitted to acute COVID care facility of the National Institute of Medical Sciences and Research, Nims Hospital Rajasthan, Jaipur, India, were categorized based on their occurrence during the first, second, and third waves. Out of 1,620 cases, 1,308 patients were found eligible for the inclusion in this study. Of these 1,308 patients, 867 patients were admitted in the first wave, 418 in the second wave, and 23 cases in the third wave. In this retrospective observational study, we found that the total number of patients admitted subsequently decreased from I wave to III wave. Males to female ratio was 2.23 (p-value <0.001) indicating males were more affected than females in all the three waves. The age group most impacted by COVID-19 among the patients were 41–60 year range, which comprises 36.9% of the total. In comparison, 26.14% of the patients were in the 61–80 year range, and only 1.14% of the patients were older than 80 years. This suggests that middle-aged individuals (41–60 years) are more affected by the virus than those who are over 80 years. Patients were divided into four groups as per the length of hospitalization. In these 30% of the patients were hospitalized for 0–5 days, 25.9% for 9–13 days, 24.08% for 6–8 days, and only 19.5% of patients hospitalized for >14 days (refer Table 1). The healthcare utilization data during the COVID-19 pandemic, categorized by area of living, gender, and age groups is shown in Figure 1. Rural residents show higher utilization across all age groups compared to urban counterparts, potentially due to limited access to healthcare in rural areas. Similarly, males and middle age group (41–60 years) utilizes more healthcare resources. Understanding these patterns can inform targeted interventions to address disparities and optimize healthcare delivery during pandemics. The detail description of patients with area of living, age and LHS across all the three waves on the basis of gender has been demonstrated in Figure 2 with the respective p values. Table 2 shows hospital burden across pandemic outbreak, the HRCT score indicates that the progression in the number of severe cases from the first to the third wave with more severe cases (61.72%) in second wave, requiring more intensive medical attention and resources. The significant p-value (<0.001) suggests a substantial increase in the strain on hospital resources, especially during the second wave, which necessitated more intensive management of severe cases. Admissions to various hospital units such as ICU, CCU, general wards, and isolation wards. 39.39% of the patients were admitted in deluxe and super deluxe ward and 33.40% in critical care unit (CCU) utilizing expensive facilities and treatments, reflecting a greater strain on these critical areas of the hospital. The prevalence of comorbidities among COVID-19 patients, including diabetes 390 (29.82%), hypertension 356 (27.22%), dyslipidemia 245 (18.73%), and respiratory disease 148 (11.31%). Based on the prevalence data provided for comorbidities among COVID-19 patients we can infer that diabetes and hypertension are the most prevalent comorbidities among COVID-19 patients.
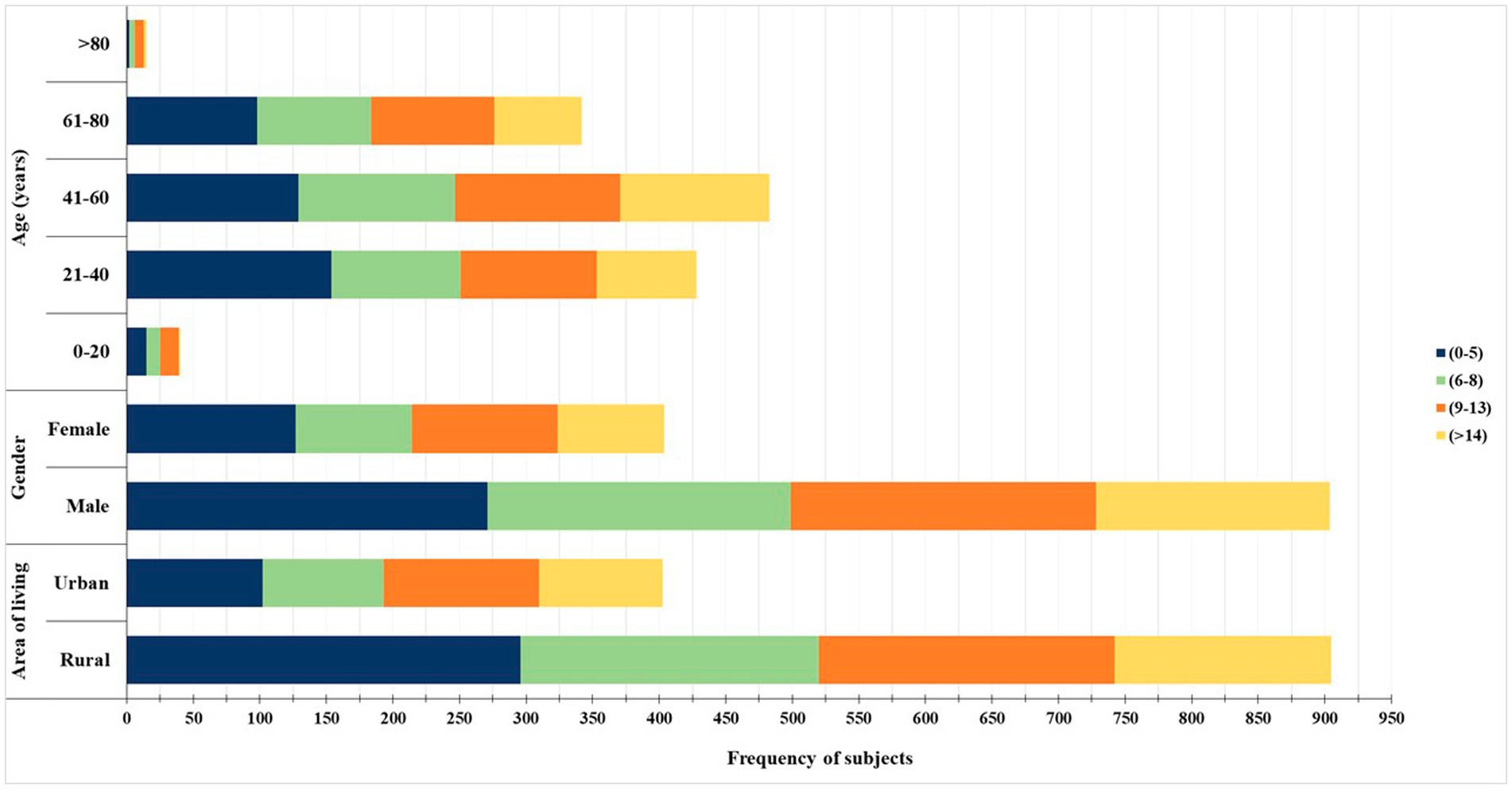
Figure 1. Distribution of healthcare utilization based on age group, gender, and area of residence. The bar chart illustrates the frequency of healthcare utilization among study participants stratified by age (in years), gender (male and female), and area of residence (urban and rural). The duration of healthcare utilization is categorized into four groups: 0–5 days (dark blue), 6–8 days (light green), 9–13 days (orange), and more than 14 days (yellow). Middle-aged individuals (21–60 years), males, and those from rural areas demonstrated longer healthcare usage durations.
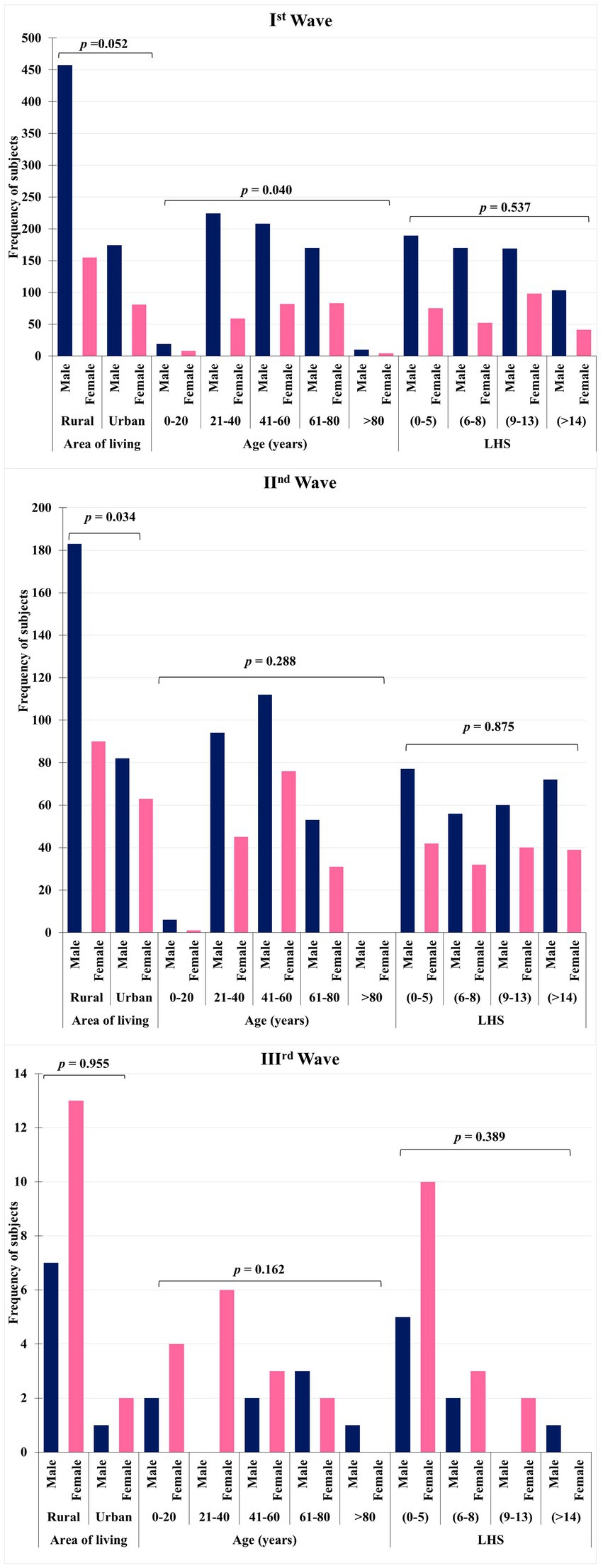
Figure 2. Comparison of healthcare utilization across the three COVID-19 waves based on gender, age, and area of residence. The grouped bar charts display the frequency of healthcare utilization among COVID-19 patients during the first, second, and third waves, categorized by gender, age group, area of residence, and duration of utilization (LHS: length of healthcare stay). Each chart presents statistical comparisons (p-values) for gender-based, age-based, and residence-based differences in healthcare usage. The data highlight gender and rural–urban disparities and trends in age-specific healthcare engagement across different waves.
The types of treatments administered, ranging from non-invasive methods and high-flow oxygen to specific regimen like corticosteroids, hydroxychloroquine, monoclonal antibodies, and antiviral drugs including Ramdesivir, Favipiravir, Molnupiravir, Daclizumab, Bevacizumab, all these expensive treatment regimens directly influenced the healthcare utilization. The significant values across different categories highlight a clear trend toward increased hospital burden, particularly marked by an increase in severe cases, higher admissions to resource-intensive units, and shifts in treatment strategies. The future trajectory of a pandemic similar to COVID-19 was forecasted by conducting a multivariable poisson regression analysis. The analysis used age, gender, residence (region of living), HRCT, and treatment as independent factors. The results showed a statistically significant relationship male gender (p = 0.037; OR 0.90; 95% confidence interval [CI] 0.818 to 0.998), middle age and below (41–60: OR 1.26; 95% CI 0.78–2.06; 0–20: OR; 95% CI 0.78–2.33), rural population (OR 1.01; 95% CI 0.91–1.12), Non-invasive mechanical ventilation + Hydroxychloroquine + Antiviral therapy + Monoclonal antibodies (OR 1.15; 95% CI 0.93–1.43) were associated with higher utilizations of resources. In contrast less hospital stay 9–13 days (OR 0.91; 95% CI 0.79–1.05), HRCT score (mild: OR 0.96; 95% CI 0.84–1.09; moderate: OR 0.93; 95% CI 0.83–1.04) are associated with lower utilization of overall resources.
Other variables, such as age group, vaccination status, comorbidities, and HRCT severity score, did not show statistically significant associations in the multivariate model (p > 0.05). However, some variables demonstrated trends that may have clinical relevance. For instance, participants with moderate to severe HRCT scores showed a slightly increased incidence rate ratio, although not reaching statistical significance (adjusted IRR = 1.188; 95% CI: 0.913–1.546; p = 0.199) (refer Table 3).
Discussion
In this study, we examined healthcare utilization patterns among COVID-19 patients admitted to the acute COVID care center at NIMS Hospital, Jaipur, Rajasthan, India. A total of 1,308 individuals were included, and their utilization patterns were analyzed based on length of hospital stay, treatment protocols, and the hospital units where they were admitted. During the second wave, a higher number of patients required admission to the ICU and CCU compared to isolation and general wards, indicating greater severity and mortality during this wave. This surge in severity led to a shortage of essential medical supplies, including medications, oxygen, hospital beds, and healthcare personnel. Similar studies have shown that the second wave was more widespread and severe, whereas the first wave was marked by stringent social distancing measures, national lockdowns, and travel restrictions (13–15). Consequently, the strain on healthcare resources during the COVID-19 pandemic exceeded that experienced during recent pandemics involving influenza and other coronaviruses (16).
In the second wave, 26.55% of patients were hospitalized for more than 14 days, compared to 16.60% in the first wave and 4.34% in the third wave. The significant difference in hospital stay duration (p < 0.001) highlights the increased severity of illness and higher resource utilization during the second wave. A study by Tendulkar P et al. and Singh S et al. similarly reported that the average hospital stay was longer during the second wave compared to the first (17, 18). Study also demonstrated a marked decline in the number of infected patients during the third wave when compared to the first and second waves. This trend suggests a potential shift in transmission dynamics or the impact of increased immunity and public health interventions over time.
During the second wave, treatments such as Hydroxychloroquine, Monoclonal antibodies, Antiviral therapy, and high-flow oxygen therapy were used more frequently than in the first and third waves. The use of drugs like Remdesivir and Bevacizumab was found to be more effective in preventing disease progression and reducing hospital stay durations (19–21).
In both the first and second waves, the majority of COVID-19 patients were from rural areas, with a p-value of 0.028, corroborating findings from Cuadros DF et al. Rural populations often face challenges such as limited access to healthcare resources, leading to delayed diagnoses and treatment, which in turn results in more severe cases and longer hospital stays. Factors such as lack of knowledge about social distancing, limited availability or use of face masks, and delayed vaccination uptake may have contributed to this trend (8, 22). In contrast, a study by Bhocal U. et al. claimed that rural populations had fewer infections and shorter hospital stays due to a stronger immune response, presenting contrary results (23). This study’s significant strengths include its large and diverse patient cohort, along with its comprehensive evaluation of healthcare utilization following a COVID-19 diagnosis.
Several studies have shown that males were more susceptible to COVID-19 compared to females, resulting in higher hospitalization and healthcare resource utilization among men. In our study, 904 males (69.11% of the total) were infected, possibly due to biological differences in immune responses and pre-existing health conditions (24–27), both of which may contribute to higher rates of hospitalization (28). Additionally, the middle-aged population was more affected by COVID-19 than pediatric and geriatric populations in this study (27, 29, 30). In comparison with the findings of the study by Gunjan Kumar et al., which analyzed data from 31 hospitals across India, our study similarly observes a higher proportion of middle-aged individuals affected by post-COVID sequelae. The broader dataset reported by Kumar et al. reinforces our findings, indicating that the middle-aged population represents a significant demographic among COVID-19 survivors experiencing long-term health impacts (30).
This could be attributed to the fact that vulnerable groups, such as children and the older adult, were often kept under strict preventive measures, despite being more immunosensitive (25). Underlying health conditions, such as obesity, diabetes, hypertension, and respiratory diseases, which were prevalent in our study, can exacerbate the severity of COVID-19. This underscores the importance of managing comorbidities in reducing the impact of the virus (31, 32). Although the older adult may have a higher risk of mortality from COVID-19, preventive measures like shielding and vaccination campaigns may have reduced their infection rates compared to other age groups (33, 34).
To the best of our knowledge, this is the first study to forecast future pandemic trends similar to COVID-19 while comparing healthcare utilization patterns across the three waves of the COVID-19 outbreak, based on hospital stay length, admission unit, and treatment regimen in a tertiary care hospital in Jaipur, Rajasthan, India.
Limitations
Further study of healthcare utilization in multicenter involving bed charge, food, lack of transportation, shortage of manpower, poor cooperation from beneficiaries, details about the health care card are required to find further trend of utilization pattern.
Conclusion
The healthcare utilization pattern observed during three waves reveals notable shifts. The initial wave witnessed heightened healthcare utilization, primarily driven by increased hospitalizations. The second wave displayed a further surge, indicating evolving patient needs. However, the third wave demonstrated a decline, possibly reflecting enhanced preventive measures. These trends emphasize the importance of adaptable healthcare systems to accommodate varying demands while maintaining a proactive approach to healthcare management. Therefore, any future similar pandemic situation will be more dangerous for females, middle aged population, individuals residing in rural population and will stay for a long period of time in the hospital.
Data availability statement
The original contributions presented in the study are included in the article/supplementary material, further inquiries can be directed to the corresponding author.
Ethics statement
The studies involving humans were approved by the Institutional Ethics Committee of National Institute of Medical Sciences and Research. The studies were conducted in accordance with the local legislation and institutional requirements. Written informed consent for participation was not required from the participants or the participants’ legal guardians/next of kin in accordance with the national legislation and institutional requirements.
Author contributions
BC: Data curation, Formal analysis, Methodology, Software, Writing – original draft, Writing – review & editing. PR: Formal analysis, Visualization, Writing – original draft. PS: Writing – review & editing. HB: Conceptualization, Methodology, Validation, Writing – original draft. AS: Data curation, Software, Validation, Writing – original draft. MS: Conceptualization, Investigation, Methodology, Resources, Writing – original draft, Writing – review & editing. DN: Funding acquisition, Resources, Supervision, Validation, Writing – review & editing. BT: Funding acquisition, Project administration, Supervision, Writing – review & editing.
Funding
The author(s) declare that no financial support was received for the research and/or publication of this article.
Acknowledgments
We are writing to express my sincere gratitude to swift administration support of Nims University. We also thank to Mr. Raju Ji for allowing data collection from MRD, we also appreciate the professionalism and efficiency with which the entire process was handled, from the initial request for access to the MRD to the final approval and data collection.
Conflict of interest
The authors declare that the research was conducted in the absence of any commercial or financial relationships that could be construed as a potential conflict of interest.
Generative AI statement
The authors declare that no Gen AI was used in the creation of this manuscript.
Publisher’s note
All claims expressed in this article are solely those of the authors and do not necessarily represent those of their affiliated organizations, or those of the publisher, the editors and the reviewers. Any product that may be evaluated in this article, or claim that may be made by its manufacturer, is not guaranteed or endorsed by the publisher.
References
1. Cheng, ZJ, Zhan, Z, Xue, M, Zheng, P, Lyu, J, Ma, J, et al. Public health measures and the control of COVID-19 in China. Clin Rev Allergy Immunol. (2021) 64:1–6. doi: 10.1007/s12016-021-08900-2
2. Faruqui, N, Raman, VR, Shiv, J, Chaturvedi, S, Muzumdar, M, and Prasad, V. Informal collectives and access to healthcare during India’s COVID-19 second wave crisis. BMJ Glob Health. (2021) 6:e006731. doi: 10.1136/bmjgh-2021-006731
3. Zhu, H, Wei, L, and Niu, P. The novel coronavirus outbreak in Wuhan, China. Global Health Res Policy. (2020) 5:1–3. doi: 10.1186/s41256-020-00135-6
4. Guan, WJ, Ni, ZY, Hu, Y, Liang, WH, Ou, CQ, He, JX, et al. Clinical characteristics of coronavirus disease 2019 in China. N Engl J Med. (2020) 382:1708–20. doi: 10.1056/NEJMoa2002032
5. Hollander, JE, and Carr, BG. Virtually perfect? Telemedicine for COVID-19. N Engl J Med. (2020) 382:1679–81. doi: 10.1056/NEJMp2003539
6. Wosik, J, Fudim, M, Cameron, B, Gellad, ZF, Cho, A, Phinney, D, et al. Telehealth transformation: COVID-19 and the rise of virtual care. J Am Med Inform Assoc. (2020) 27:957–62. doi: 10.1093/jamia/ocaa067
7. Bouabida, K, Lebouché, B, and Pomey, MP. Telehealth and COVID-19 pandemic: an overview of the telehealth use, advantages, challenges, and opportunities during COVID-19 pandemic. Healthcare. (2022) 10:2293. doi: 10.3390/healthcare10112293
8. Oduro, MS, Peprah, P, Morgan, AK, and Agyemang-Duah, W. Staying in or out? COVID-19-induced healthcare utilization avoidance and associated socio-demographic factors in rural India. BMC Public Health. (2023) 23:1439. doi: 10.1186/s12889-023-16282-7
9. Adams, JG, and Walls, RM. Supporting the health care workforce during the COVID-19 global epidemic. JAMA. (2020) 323:1439–40. doi: 10.1001/jama.2020.3972
10. Gómez, CE, Perdiguero, B, and Esteban, M. Emerging SARS-CoV-2 variants and impact in global vaccination programs against SARS-CoV-2/COVID-19. Vaccine. (2021) 9:243. doi: 10.3390/vaccines9030243
11. Zirpe, KG, Dixit, S, Kulkarni, AP, Pandit, RA, Ranganathan, P, Prasad, S, et al. The second-vs first-wave COVID-19: more of the same or a lot worse? A comparison of mortality between the two waves in patients admitted to intensive care units in nine hospitals in Western Maharashtra. Indian J Crit Care Med. (2021) 25:1343–8. doi: 10.5005/jp-journals-10071-24042
12. Sharma, S, Aggarwal, A, Sharma, RK, Patras, E, and Singhal, A. Correlation of chest CT severity score with clinical parameters in COVID-19 pulmonary disease in a tertiary care hospital in Delhi during the pandemic period. Egypt J Radiol Nucl Med. (2022) 53:166. doi: 10.1186/s43055-022-00832-x
13. Sharma, S, Singh, L, Yadav, J, Gupta, U, Singh, KJ, and Rao, MV. Impact of COVID-19 on utilization of maternal and child health services in India: health management information system data analysis. Clin Epidemiol Global Health. (2023) 21:101285. doi: 10.1016/j.cegh.2023.101285
14. Bager, P, Wohlfahrt, J, Rasmussen, M, Albertsen, M, and Krause, TG. Hospitalisation associated with SARS-CoV-2 delta variant in Denmark. Lancet Infect Dis. (2021) 21:1351. doi: 10.1016/S1473-3099(21)00580-6
15. Twohig, KA, Nyberg, T, Zaidi, A, Thelwall, S, Sinnathamby, MA, Aliabadi, S, et al. Hospital admission and emergency care attendance risk for SARS-CoV-2 delta (B. 1.617. 2) compared with alpha (B. 1.1. 7) variants of concern: a cohort study. Lancet Infect Dis. (2022) 22:35–42. doi: 10.1016/S1473-3099(21)00475-8
16. Huang, BZ, Creekmur, B, Yoo, MS, Broder, B, and Sharp, AL. Healthcare utilization among patients diagnosed with COVID-19 in a large integrated health system. J Gen Intern Med. (2022) 37:830–7. doi: 10.1007/s11606-021-07139-z
17. Tendulkar, P, Pandey, P, Panda, PK, Bhadoria, AS, Kulshreshtha, P, Mishra, M, et al. Comparative study between the first and second wave of COVID-19 deaths in India: a single center study. Cureus. (2023) 15:e0248029. doi: 10.7759/cureus.37472
18. Singh, S, Sharma, A, Gupta, A, Joshi, M, Aggarwal, A, Soni, N, et al. Demographic comparison of the first, second and third waves of COVID-19 in a tertiary care hospital at Jaipur, India. Lung India. (2022) 39:525–31. doi: 10.4103/lungindia.lungindia_265_22
19. Costanzo, M, De Giglio, MA, and Roviello, GN. SARS-CoV-2: recent reports on antiviral therapies based on lopinavir/ritonavir, darunavir/umifenovir, hydroxychloroquine, remdesivir, favipiravir and other drugs for the treatment of the new coronavirus. Curr Med Chem. (2020) 27:4536–41. doi: 10.2174/0929867327666200416131117
20. Horby, PW, Mafham, M, Bell, JL, Linsell, L, Staplin, N, Emberson, J, et al. Lopinavir–ritonavir in patients admitted to hospital with COVID-19 (RECOVERY): a randomised, controlled, open-label, platform trial. Lancet. (2020) 396:1345–52. doi: 10.1016/S0140-6736(20)32013-4
21. Lam, S, Lombardi, A, and Ouanounou, A. COVID-19: a review of the proposed pharmacological treatments. Eur J Pharmacol. (2020) 886:173451. doi: 10.1016/j.ejphar.2020.173451
22. Cuadros, DF, Branscum, AJ, Mukandavire, Z, Miller, FD, and MacKinnon, N. Dynamics of the COVID-19 epidemic in urban and rural areas in the United States. Ann Epidemiol. (2021) 59:16–20. doi: 10.1016/j.annepidem.2021.04.007
23. Bhocal, U, Katyal, A, Dhull, D, Raghuraman, K, Nandal, N, and Gill, PS. Assessment of clinical and virological outcomes of rural and urban populations: COVID-19. J Family Med Prim Care. (2022) 11:6074–80. doi: 10.4103/jfmpc.jfmpc_151_22
24. Kushwaha, S, Khanna, P, Rajagopal, V, and Kiran, T. Biological attributes of age and gender variations in Indian COVID-19 cases: a retrospective data analysis. Clin Epidemiol Global Health. (2021) 11:100788. doi: 10.1016/j.cegh.2021.100788
25. Samanta, T, Gopalan, K, and Devi, T. Blocked by gender: disparities in COVID19 infection detection in Tamil Nadu, India. Front Public Health. (2022) 10:966490. doi: 10.3389/fpubh.2022.966490
26. Salerno, S, Sun, Y, Morris, EL, He, X, Li, Y, Pan, Z, et al. Comprehensive evaluation of COVID-19 patient short- and long-term outcomes: disparities in healthcare utilization and post-hospitalization outcomes. PLoS One. (2021) 16:e0258278. doi: 10.1371/journal.pone.0258278
27. Matsunaga, N, Hayakawa, K, Asai, Y, Tsuzuki, S, Terada, M, Suzuki, S, et al. Clinical characteristics of the first three waves of hospitalised patients with COVID-19 in Japan prior to the widespread use of vaccination: a nationwide observational study. Lancet Reg Health Western Pac. (2022) 22:100421. doi: 10.1016/j.lanwpc.2022.100421
28. Bwire, GM. Coronavirus: why men are more vulnerable to COVID-19 than women? SN Comprehen Clin Med. (2020) 2:874–6. doi: 10.1007/s42399-020-00341-w
29. Kapoor, M, Nidhi Kaur, K, Saeed, S, Shannawaz, M, and Chandra, A. Impact of COVID-19 on healthcare system in India: a systematic review. J Public Health Res. (2023) 12:22799036231186349. doi: 10.1177/22799036231186349
30. Kumar, G, Bhalla, A, Mukherjee, A, Turuk, A, Talukdar, A, Mukherjee, S, et al. Post COVID sequelae among COVID-19 survivors: insights from the Indian National Clinical Registry for COVID-19. BMJ Glob Health. (2023) 8:e012245. doi: 10.1136/bmjgh-2023-012245
31. Hamza, A, Shah, NN, Azad, AM, Ghanshyam, OS, and Khan, Z. Impact of age, gender and comorbidities affecting the severity of COVID-19 infection in Kashmir. J Family Med Prim Care. (2022) 11:1519–24. doi: 10.4103/jfmpc.jfmpc_278_21
32. Iftimie, S, López-Azcona, AF, Vallverdú, I, Hernández-Flix, S, de Febrer, G, Parra, S, et al. First and second waves of coronavirus disease-19: a comparative study in hospitalized patients in Reus, Spain. PLoS One. (2021) 16:e0248029. doi: 10.1371/journal.pone.0248029
33. Liu, JQ, Xu, JW, Sun, CY, Wang, JN, Wang, XT, Chen, X, et al. Age-stratified analysis of SARS-CoV-2 infection and case fatality rate in China, Italy, and South Korea. Eur Rev Med Pharmacol Sci. (2020) 24:12575–8. doi: 10.26355/eurrev_202012_24054
Keywords: COVID-19, healthcare utilization, length of hospital stay, pandemic, SARS-CoV-2
Citation: Chaturvedi B, Raj P, Singh P, Bareth H, Sharma A, Singh M, Nathiya D and Tomar BS (2025) Exploring the diverse factors influencing healthcare utilization during the COVID-19 crisis. Front. Public Health. 13:1512735. doi: 10.3389/fpubh.2025.1512735
Edited by:
Nelly Lagos San Martín, University of the Bío Bío, ChileReviewed by:
Indika Varuna Mallawaarachchi, University of Virginia, United StatesAmit Tak, RVRS Medical College, India
Anthony Kwame Morgan, Hong Kong Polytechnic University, Hong Kong SAR, China
Copyright © 2025 Chaturvedi, Raj, Singh, Bareth, Sharma, Singh, Nathiya and Tomar. This is an open-access article distributed under the terms of the Creative Commons Attribution License (CC BY). The use, distribution or reproduction in other forums is permitted, provided the original author(s) and the copyright owner(s) are credited and that the original publication in this journal is cited, in accordance with accepted academic practice. No use, distribution or reproduction is permitted which does not comply with these terms.
*Correspondence: Mahaveer Singh, ZHJtcy5tYW1jbWVkQGdtYWlsLmNvbQ==
†ORCID: Deepak Nathiya, orcid.org/0000-0001-7231-0620
Balvir Singh Tomar, https://orcid.org/0000-0001-8788-5152