- 1Department of Respiratory and Critical Care Medicine, Key Laboratory of Respiratory Disease of Ningbo, First Affiliated Hospital of Ningbo University, Ningbo, China
- 2Department of Hepatology, First Affiliated Hospital of Ningbo University, Ningbo, China
Background: Exposure to brominated flame retardants (BFRs) has been linked to age-related diseases. This study investigates the associations between both individual and combined BFRs exposures and phenotypic age acceleration (PhenoAgeAccel) in U.S. adults.
Methods: Data from 3,908 U.S. adults from NHANES 2005–2010 were analyzed. Generalized linear regression models (GLMs) assessed the associations between individual BFRs and PhenoAgeAccel, while weighted quantile sum (WQS) regression and Bayesian Kernel Machine Regression (BKMR) analyses were used to evaluate the effects of combined BFRs exposures.
Results: GLMs indicated significant positive associations between several BFRs and PhenoAgeAccel, including PBDE28 (β = 0.55, 95% CI: 0.13, 0.96), PBDE85 (β = 0.42, 95% CI: 0.11, 0.74), PBDE47 (β = 0.39, 95% CI: 0.04, 0.75), PBDE99 (β = 0.38, 95% CI: 0.07, 0.68), and PBDE154 (β = 0.37, 95% CI: 0.04, 0.70). RCS analysis confirmed nonlinear dose–response relationships for PBDE47 and PBDE99 (P for nonlinearity = 0.03361 and 0.0233, respectively). Stratified analyses revealed that males were more susceptible to BFRs exposure effects, particularly for PBDE99 (P for interaction = 0.027) and PBDE209 (P for interaction = 0.005). The WQS regression showed a significant association between combined BFRs exposure and increased PhenoAgeAccel (β = 0.504, 95% CI: 0.071, 0.937), with PBB153 and PBDE153 as key contributors. BKMR analysis indicated a trend of increasing PhenoAgeAccel with higher BFR exposure levels, primarily driven by PBDE99.
Conclusion: This study highlights the significant positive associations between individual and combined BFR exposures and PhenoAgeAccel, with males potentially being more vulnerable to these effects.
1 Introduction
Brominated flame retardants (BFRs) are a group of chemicals widely used in plastics, electronics, and furniture to enhance flame resistance. However, due to their persistence in the environment and potential for bioaccumulation, BFRs have emerged as environmental pollutants of global concern. These substances are prevalent in air, soil, water, and biological samples such as human serum and breast milk (1). Despite the phase-out of certain BFRs in many countries (2), they remain in the environment and continue to expose humans through various pathways, including inhalation of dust, dietary intake, and dermal contact (3). Some studies have indicated that BFRs exhibit thyroid toxicity, neurotoxicity, and reproductive and developmental toxicity, posing significant health risks (4–6). Compounds such as polybrominated diphenyl ethers (PBDEs) and polybrominated biphenyls (PBBs), due to their lipophilicity and long half-lives, can accumulate in the human body over time. This means that despite their gradual discontinuation, human exposure to BFRs persists, presenting ongoing risks (7).
Aging is commonly defined as the gradual decline in physiological functions and systemic integrity that occurs over time, leading to increased vulnerability to diseases and an elevated risk of death (8). It involves the progressive accumulation of damage at the cellular, molecular, and tissue levels, resulting in the deterioration of bodily systems and a reduced ability to maintain homeostasis (8). Aging is a multifaceted process influenced by genetic, environmental, and lifestyle factors, manifesting in various biological, functional, and cognitive changes that contribute to the overall aging phenotype. To provide a more precise measure of an individual’s aging state, taking into account their overall health, various biomarkers of aging have been developed (9), such as phenotypic age (10), biological age (11), DNA methylation (12), and HD (13). Among these, phenotypic age—a composite biomarker based on biological markers and mortality risk—has demonstrated high accuracy in predicting disease incidence and mortality (14). While, phenotypic age acceleration (PhenoAgeAccel) is derived from phenotypic age and serves as an indicator of whether an individual’s biological aging process is faster or slower than expected, given their chronological age. A positive PhenoAgeAccel value reflects accelerated aging, meaning the individual’s biological state is older than their chronological age, while a negative value indicates decelerated aging, suggesting a younger biological state. This metric has been linked to increased susceptibility to age-related diseases, functional decline, and mortality. As such, PhenoAgeAccel provides a valuable framework for understanding how external factors, including environmental exposures, may influence the aging process. Given the dual challenges of environmental pollution and population aging, it is critical to investigate how environmental substances affect the aging process. Previous studies have suggested that BFRs exposure are linked to several age-related diseases, including cancer (15), cardiovascular diseases (16), neurodegenerative conditions (17), and metabolic disorders (18). Additionally, in vitro studies have shown that BFRs can induce apoptosis (19), which is one of the 12 hallmarks of biological aging (14).
Therefore, this study aims to explore the potential association between BFRs exposure and phenotypic age acceleration (PhenoAgeAccel) using data from the National Health and Nutrition Examination Survey (NHANES). By examining this relationship, the study seeks to provide new evidence on the role of environmental exposure in the aging process, contributing to the growing body of research on the impact of environmental pollutants on human health.
2 Materials and methods
2.1 Design
This study analyzed data from the 2005–2010 NHANES cycles, spanning three consecutive survey periods. Although BFRs data is available through 2016, a methodology changes in C-reactive protein (CRP) measurement occurred in the 2011–2012 cycle with the introduction of high-sensitivity CRP. Since CRP is a critical marker for calculating phenotypic age, data after 2010 were excluded to ensure methodological consistency.
NHANES, conducted by the CDC, is a nationally representative survey employing a complex, multi-stage sampling design to evaluate the health and nutrition of the U.S. population. Participants undergo interviews, physical exams, and biospecimen collection at mobile examination centers. For this analysis, individuals aged 20 and older were included, providing information on demographics, socio-economic status, dietary intake, chronic diseases, and BFRs exposure from the 2005–2010 cycles. After applying specific inclusion and exclusion criteria, a total of 3,908 participants were selected for the final analysis, as outlined in Figure 1.
2.2 BFRs exposure
BFRs were measured in serum using automated liquid–liquid extraction followed by gas chromatography coupled with high-resolution mass spectrometry. In line with previous studies, serum BFR concentrations were used to reflect individual exposure levels. The analysis included polybrominated biphenyl (PBB153) and eight polybrominated diphenyl ethers (PBDEs) with detection rates above 65% (20). The selected PBDEs were: 2,4,4′-Tribromodiphenyl ether (PBDE28), 2,2′,4,4´-Tetrabromodiphenyl ether (PBDE47), 2,2′,3,4,4´-Tetrabromodiphenyl ether (PBDE85), 2,2′,4,4′,5-Pentabromodiphenyl ether (PBDE99), 2,2′,4,4′,6-Pentabromodiphenyl ether (PBDE100), 2,2′,4,4′,5,5´-Hexabromodiphenyl ether (PBDE153), 2,2′,4,4′,5,6´-Hexabromodiphenyl ether (PBDE154), and Decabromodiphenyl ether (PBDE209). In this study, values below the limit of detection (LOD) were imputed using limit divided by the square root of two, in accordance with the NHANES analytical guidelines (21). This approach is widely adopted in environmental health research. Moreover, previous studies have assessed the sensitivity of various imputation methods for handling values below the detection limit, providing support for LOD/√2 as a reasonable and widely accepted estimation method (22).
2.3 Phenotypic age acceleration
Aging acceleration was assessed using phenotypic age, which is calculated based on various biomarkers and a specific algorithm. To control for chronological age, PhenoAgeAccel was determined as the residual from a regression of phenotypic age on chronological age. Participants were categorized into two groups: those with accelerated aging (PhenoAgeAccel ≥ 0) and those with delayed aging (PhenoAgeAccel < 0).
Phenotypic age was calculated using the following formula (14):
Where:
And:
𝑥𝑏 = −19.907–0.0336 × Albumin+0.0095 × Creatinine+0.1953 × Glucose+0.0954 × ln(CRP) − 0.0120 × Lymphocyte Percentage+0.0268 × Mean Cell Volume+0.3306 × Erythrocyte Distribution Width + 0.00188 × Alkaline Phosphatase+0.0554 × Leukocyte Count+0.0804 × Chronological Age. Here, “𝑥𝑏 “represents the linear combination of biomarkers used in the model.
2.4 Covariation
Based on previous literature, covariates that have been proven to be associated with environmental factors and biological aging were included in the analysis. These covariates were: age, sex, race/ethnicity, poverty-to-income ratio (PIR), BMI, marital status, education level, smoking status, alcohol consumption, healthy dietary status (assessed using the Healthy Eating Index-2015 [HEI-2015] (23)), physical activity level, and the presence of chronic diseases, including hypertension, diabetes mellitus (DM), cardiovascular disease (CVD), and cancer, Additionally, the corresponding survey year was included. Hypertension and DM were determined through both self-reports and clinical measurements, while CVD and cancer were self-reported. Estimated glomerular filtration rate (eGFR) was calculated using the Chronic Kidney Disease Epidemiology Collaboration (CKD-EPI) equation. Participants were classified by PIR as low (<1.3), middle (1.3–3.5), and high (>3.5); BMI was categorized based on WHO classifications (normal, overweight, obesity); alcohol consumption was divided into non-drinkers, moderate drinkers (1–3 drinks/day), and heavy drinkers (≥4 drinks/day); and physical activity level was categorized as active, inactive, moderate, or others (24).
2.5 Statistical methods
Although NHANES employs a complex sampling design, weighting was not applied in this study due to the limitations in incorporating weights into mixture analyses. For baseline characteristics, continuous variables were presented as means with standard deviations (SD), while categorical variables were expressed as counts and percentages. Differences in continuous variables between the delayed and accelerated aging groups were assessed using Student’s t-tests, and chi-square tests were employed for categorical variables.
Generalized Linear Models (GLMs) were used to evaluate the associations between BFRs(log-transformed) and PhenoAgeAccel. Three models were applied: the crude model, which was unadjusted; Model 1 adjusted for age, sex, and race/ethnicity; and Model 2 further adjusted for BMI, PIR, marital status, education, physical activity, smoking, alcohol consumption, hypertension, diabetes, cardiovascular disease, cancer, HEI-2015, and survey year. Restricted cubic spline (RCS) analysis was conducted to investigate the dose–response relationship between BFRs exposure and PhenoAgeAccel after adjusting for all confounders. Subgroup and interaction analyses were also performed based on age and sex.
In the second phase, quantile sum (WQS) regression was used to assess the effect of BFRs mixture exposure on PhenoAgeAccel. The bootstrap method (10,000 iterations) was applied to assign weights to each contaminant, identifying the most influential components in the mixture. Contaminant weights ranged from 0 to 1. This method not only captures real-world co-exposures more effectively but also enhances sensitivity in identifying key predictors compared to univariate analysis. The data were randomly split into 40% training and 60% testing samples, with 10,000 bootstrap iterations.
Additionally, Bayesian Kernel Machine Regression (BKMR) was employed to visualize the joint exposure-response relationship between BFRs mixtures and biological aging risk. BKMR’s strength lies in its flexibility to model exposure-response relationships, accounting for potential nonlinear and non-additive effects often seen in environmental epidemiology. The exposure-response functions were modeled using a Gaussian process, and the analysis was run with 10,000 iterations of the Markov Chain Monte Carlo (MCMC) algorithm. Conditional posterior inclusion probabilities (conPIPs) were calculated to identify the key BFRs components most associated with biological aging risk. All statistical analyses were conducted by R version 4.4.12.
3 Results
3.1 Baseline characteristics of study participants
A total of 3,908 U.S. adults were included in the study and categorized into two groups based on PhenoAgeAccel: the delayed aging group (n = 3,004) and the accelerated aging group (n = 904). Participants in the accelerated aging group were significantly older, with a mean age of 54.78 ± 17.46 years, compared to 41.30 ± 17.85 years in the delayed aging group. The accelerated aging group also had a higher proportion of non-Hispanic Black individuals, lower PIR, lower HEI-2015 scores, reduced eGFR, lower education levels, and a lower rate of active physical activity. In contrast, this group exhibited higher BMI, a greater prevalence of widowed/divorced/separated individuals, and an increased incidence of smoking, hypertension, cancer, and diabetes. Furthermore, the accelerated aging group was exposed to higher levels of PBDE28, PBDE47, PBDE85, PBDE99, PBDE100, and PBDE154. Detailed information is provided in Table 1. Besides, the characteristics of study participants by sex in adults are presented in Supplementary Table S1, showing that BFRs exposure levels were significantly higher in males compared to females. Additionally, the characteristics of study participants with accelerated phenotypic age by sex are provided in Supplementary Table S2, where certain BFRs exhibited significantly higher exposure levels in males than in females.
3.2 Associations between individual BFRs exposures and PhenoAgeAccel
Table 2 presents the results of the GLMs, revealing significant positive associations between several BFRs and PhenoAgeAccel. Specifically, PBDE28 (β = 0.55, 95% CI: 0.13, 0.96), PBDE85 (β = 0.42, 95% CI: 0.11, 0.74), PBDE47 (β = 0.39, 95% CI: 0.04, 0.75), PBDE99 (β = 0.38, 95% CI: 0.07, 0.68), and PBDE154 (β = 0.37, 95% CI: 0.04, 0.70) were all significantly associated with PhenoAgeAccel.
RCS analysis confirmed both linear and nonlinear dose–response relationships for several PBDEs (Figure 2). Specifically, PBDE47 (P for overall = 0.0098, P for nonlinearity = 0.0336) and PBDE99 (P for overall = 0.0041, P for nonlinearity = 0.0233) exhibited significant nonlinear associations with PhenoAgeAccel, indicating that increases in exposure were associated with sharper increases in PhenoAgeAccel at higher exposure levels. In contrast, PBDE28 (P for overall = 0.0225, P for nonlinearity = 0.3143), PBDE85 (P for overall = 0.0118, P for nonlinearity = 0.2902), and PBDE154 (P for overall = 0.0432, P for nonlinearity = 0.2062) demonstrated significant overall associations without evidence of nonlinearity, suggesting that their relationships with PhenoAgeAccel were more likely linear.
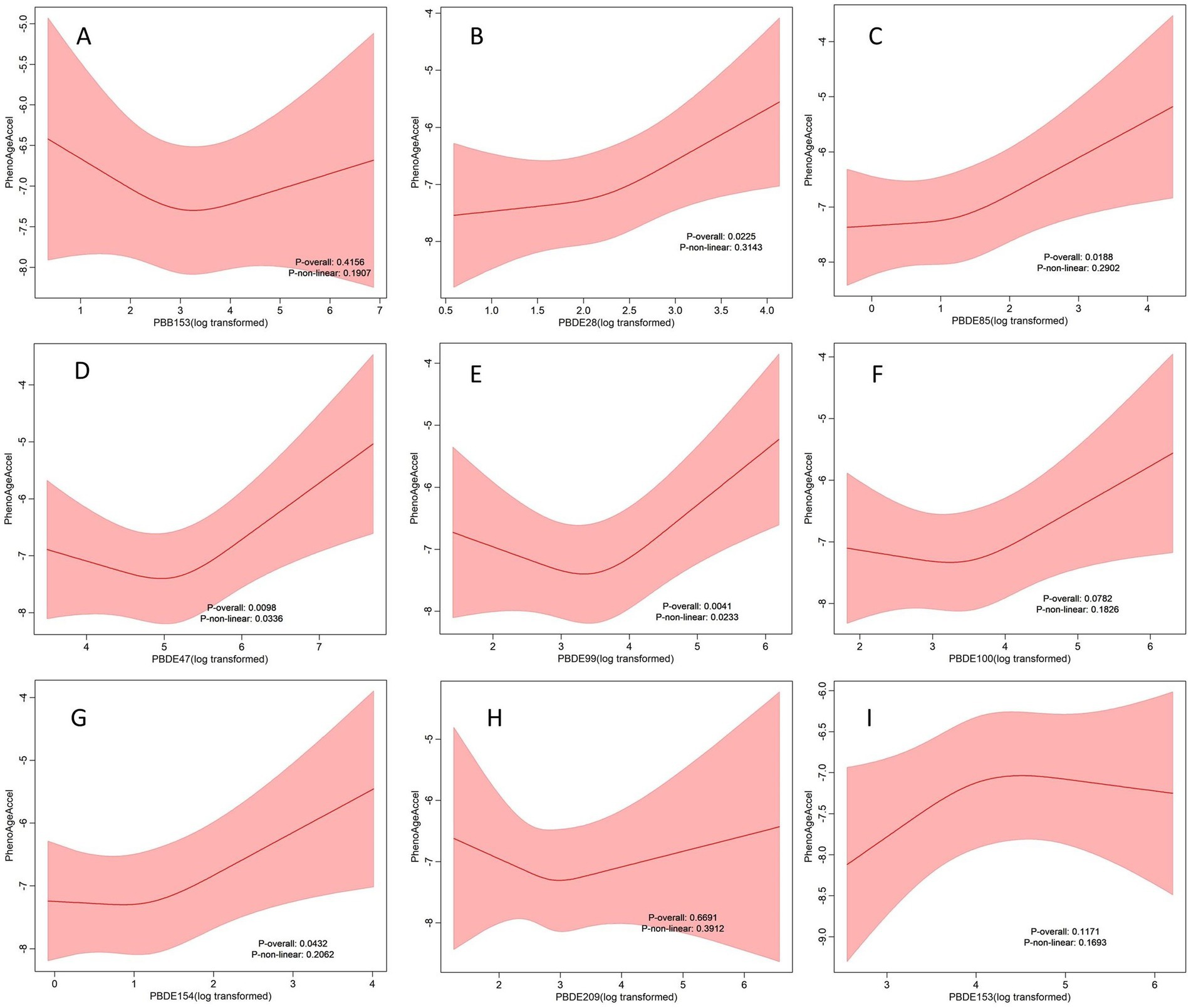
Figure 2. Figures (A–I) depict dose–response relationship between individual BFRs and PhenoAgeAccel in the sample of 3,908 U.S adults from NHANES 2005 to 2010. Red solid lines and Red dotted line represent RCS models and 95%CI, respectively. Multivariable linear regression model is used to estimate the fully adjusted coefficient in PhenoAgeAccel and corresponding 95% CI. Models were adjusted by age, sex, ethnicity, BMI, PIR, marital status, education, physical activity, smoke, drinks, hypertension, DM, CVD, cancer, eGFR, HEI-2015, and year.
The stratified analysis by age and sex revealed differential associations between BFRs exposure and PhenoAgeAccel (Supplementary Table S3). Males were more susceptible to the effects of PBB153, PBDE28, PBDE85, PBDE47, PBDE99, and PBDE154 compared to females, with significant sex interactions (P for interaction <0.0001, 0.002, 0.003, 0.017, 0.003, and 0.025, respectively). Additionally, PBDE99 showed a stronger effect in participants aged 60 and older (P for interaction = 0.027), while PBDE209 had a more pronounced effect in the 40–59 age group (P for interaction = 0.005).
3.3 Associations between BFRs mixture and PhenoAgeAccel
When constraining the analysis in the positive direction, the WQS regression analysis (Figure 3) revealed a significant association between combined exposure to BFRs and an increase in PhenoAgeAccel (β = 0.504, 95% CI: 0.071, 0.937), with this effect being particularly pronounced among males. The chemicals that contributed most to this increase in PhenoAgeAccel were PBB153, PBDE153, PBDE28, and PBDE85. Interaction analyses indicated that males were more strongly affected by this exposure. Conversely, in the negative direction, no significant association was found (β = 0.424, 95% CI: −0.084, 0.932).
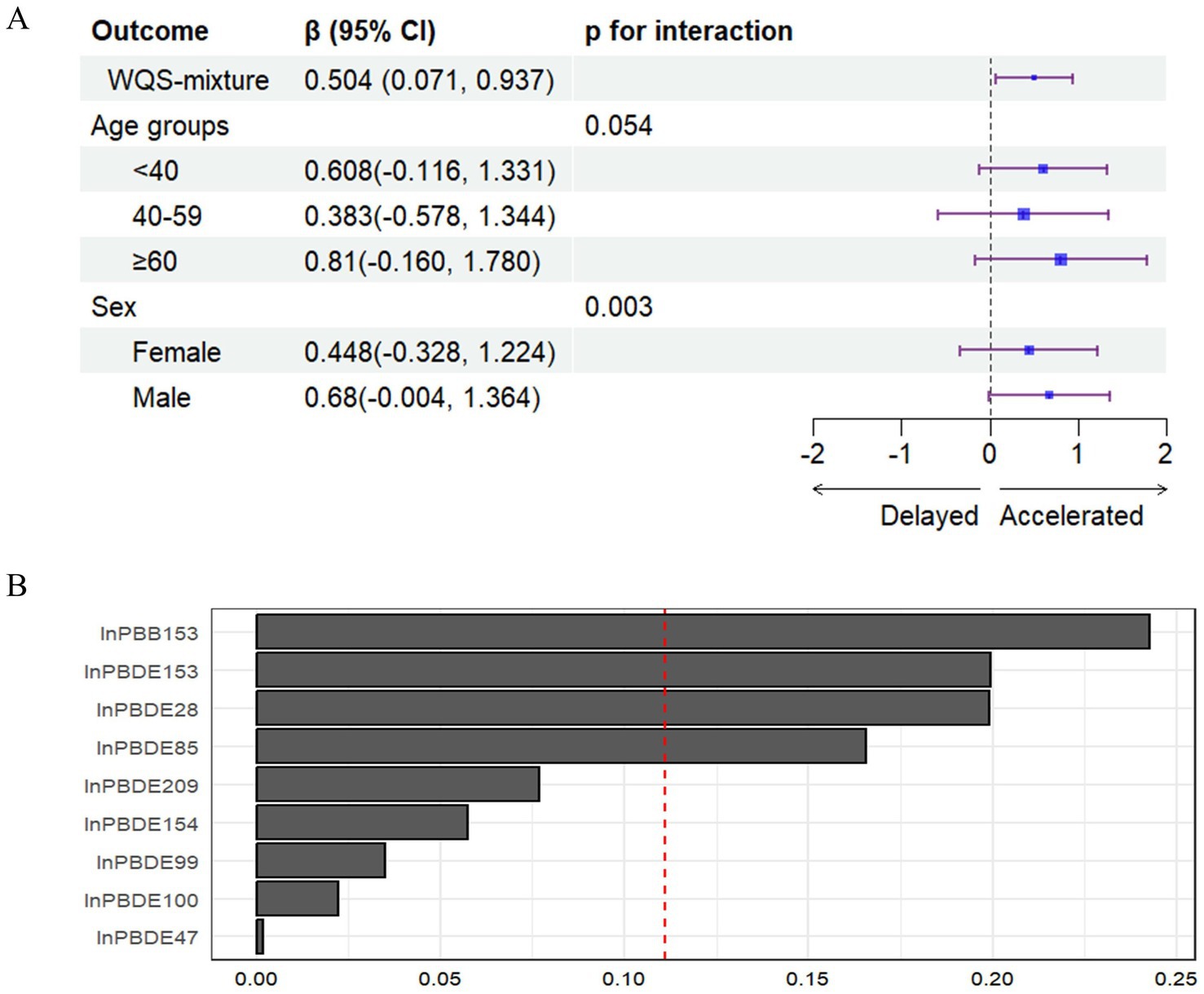
Figure 3. (A) The association between WQS mixture of BFRs and PhenoAgeAccel and stratified results by age group and sex. (B) Weights from weighted quantile sum regression for the mixture and PhenoAgeAccel.
The BKMR analysis (Figure 4A) indicated as exposure to BFRs increases from lower to higher quantiles, there is a noticeable trend in the estimated effects, suggesting that higher mixed BFRs exposure may be associated with increased PhenoAgeAccel, particularly in males (red circles). The posterior inclusion probabilities (PIPs) identified PBDE99 as the primary drivers of this effect, Other BFRs, such as PBDE28 and PBDE85, also show significant probabilities, but to a lesser extent than lnPBDE99 (Figure 4B). Males exhibited a stronger response to the BFRs mixture compared to females. Supplementary Figure S2A shows the univariate exposure-response analysis for each BFR, indicating a positive monotonic relationship between PBDE100, PBDE85, and PBDE99 with PhenoAgeAccel. In contrast, PBDE28, PBDE47, and PBDE154 exhibited a negative monotonic trend. As shown in Supplementary Figure S2B, when other chemicals were held constant at the 25th, 50th, and 75th percentiles, a positive association between PBDE100, PBDE85, and PBDE99 and PhenoAgeAccel was observed. Additionally, no statistically significant interactions between BFR components were detected (Supplementary Figure S2C).
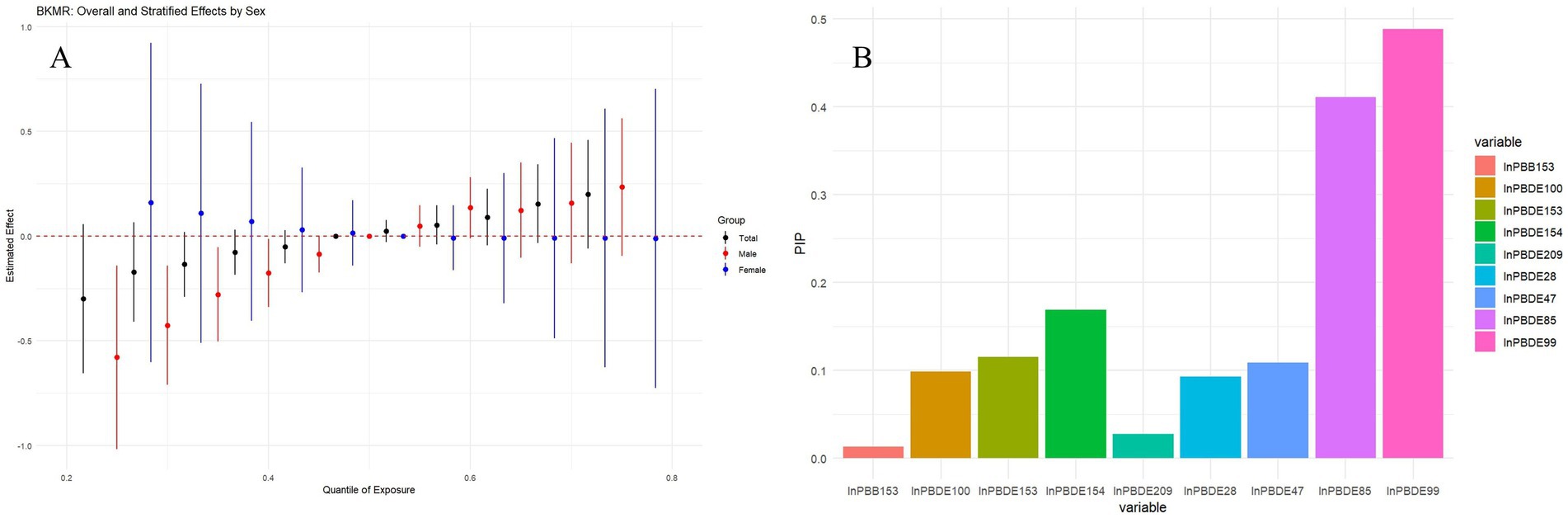
Figure 4. Association between combined BFRs exposure and PhenoAgeAccel analyzed by BKMR model. (A) Overall effects of BFRs mixture on PhenoAgeAccel and stratified results by sex at all concentrations ranged from the first quantile (10%) to the third quantile (90%) relative to the median (50%) level. (B) Posterior inclusion probabilities (PIPs) of each BFRs for PhenoAgeAccel. Models adjusted for age, sex, ethnicity, BMI, PIR, marital status, education, physical activity, smoke, drinks, hypertension, DM, CVD, cancer, eGFR, HEI-2015, and year.
4 Discussion
This study is the first to systematically examine the relationship between brominated flame retardants (BFRs) exposure and PhenoAgeAccel using U.S. NHANES data. Our findings reveal significant positive associations between several BFRs including PBDE28, PBDE85, PBDE47, PBDE99, and PBDE154 and PhenoAgeAccel. Notably, PBDE47 and PBDE99 demonstrated non-linear dose–response relationships. Both WQS mixture analysis and BKMR confirmed a positive link between BFR mixtures and PhenoAgeAccel. WQS regression identified PBB153, PBDE153, PBDE28, and PBDE85 as the primary contributors to this association, while BKMR analysis highlighted PBDE99, PBDE85, and PBDE154 as key drivers of PhenoAgeAccel. Additionally, stratified analyses revealed sex-specific differences, with males showing greater susceptibility to the adverse effects of BFR exposure.
Epidemiological studies exploring the relationship between BFRs exposure and aging remain limited. However, previous research has identified associations between BFRs and several age-related diseases. For instance, PBDE100 and PBDE153 have been independently linked to hypertension prevalence, while BFR mixtures have been associated with an increased risk of hypertension (25). Che et al. found a relationship between BFR exposure and metabolic syndrome, identifying PBB153, PBDE209, and PBDE28 as significant contributors (18). Furthermore, BFRs exposure has been positively correlated with CVD risk, with PBB153 identified as a key factor, particularly in relation to congestive heart failure and coronary heart disease (16). These findings are consistent with our study. Additionally, our study also revealed that sex modulates the relationship between BFRs exposure and PhenoAgeAccel, with males being more sensitive to PBB153, PBDE28, PBDE85, PBDE47, PBDE99, and PBDE154. One potential explanation for this sex difference lies in the influence of sex hormones, particularly testosterone and estrogen, which play critical roles in regulating metabolic and physiological processes (26). As endocrine disruptors, BFRs may interfere with these hormonal pathways, potentially leading to more pronounced metabolic dysregulation in males (27). Moreover, the higher proportion of lean body mass in males compared to females could alter the distribution, storage, and metabolism of lipophilic compounds like BFRs, further contributing to these differences (28, 29). Another plausible factor is differential exposure pathways. For example, occupational or lifestyle variations may result in higher BFRs exposure in males (30), as suggested by the data presented in Supplementary Tables S1, S2, although this warrants further investigation. Additionally, sex-based metabolic differences, such as variations in liver enzyme activity or fat metabolism, may influence the biotransformation and clearance of BFRs, exacerbating the observed disparities in sensitivity (26, 31). While these findings underscore important sex-specific variations, further studies are needed to elucidate the underlying mechanisms and to explore additional factors that may contribute to the differential susceptibility observed in males.
Although the precise mechanisms through which BFRs influence aging remain unclear, several hypotheses, supported by various studies, provide potential explanations. One mechanism involves the endocrine-disrupting properties of BFRs, particularly PBDEs. PBDEs are recognized as endocrine disruptors that can potentially interfere with estrogen receptor and thyroid hormone signaling, both of which are involved in regulating adipose tissue metabolism and maintaining metabolic homeostasis (32, 33). These disruptions are hypothesized to contribute to metabolic dysregulation, which has been associated with markers of accelerated aging in prior studies. Another mechanism that may influence aging is oxidative stress and inflammation (34). In vitro studies have demonstrated that exposure to PBDE-47, PBDE-209, and PBDE-99 induces oxidative stress and inflammatory responses in bronchial epithelial cells, which are linked to DNA damage and apoptosis. These processes are fundamental to cellular aging (34). Experiments involving HepG2 cells, wild-type N2 worms, and adipocytes have shown that PBDE-47 exposure may contribute to a dose-dependent increase in oxidative stress (35–37). Reactive oxygen species, a hallmark of oxidative stress, are recognized as one of the 12 hallmarks of aging. In human umbilical vein endothelial cells, PBDE-209 has been shown to induce ROS production, further linking BFR exposure to cellular senescence (38). Furthermore, epidemiological evidence suggests an association between BFR exposure and oxidative stress biomarkers, which may provide insight into potential pathways linking BFRs to biological aging. A study analyzing NHANES data from 2007 to 2016 revealed a positive association between BFR levels and oxidative stress biomarkers in U.S. adults (39). Aging presents the many characteristics including high bioavailability of oxidative stress and inflammation (40). Overall, BFRs may influence biological processes potentially relevant to aging, including endocrine disruption, oxidative stress, inflammation, and organ-specific toxicity. While these mechanisms are plausible, further research is needed to clarify their roles and interactions in relation to biological aging.
Although our study provides the first preliminary evidence linking BFRs exposure to PhenoAgeAccel, further research is necessary to validate these findings due to several limitations. First, this study’s cross-sectional design precludes establishing causal relationships between BFRs exposure and PhenoAgeAccel. The mechanisms discussed are based on associations observed in this study and prior experimental findings, and should be interpreted as hypotheses requiring further validation through longitudinal and experimental research. Second, there is currently no consensus on the optimal methods for measuring lipophilic chemicals in serum. The use of serum BFRs concentrations as markers of exposure in this study is appropriate given their widespread application in environmental health research. However, serum measurements have limitations in capturing long-term or cumulative exposure, particularly for lipophilic and bioaccumulative compounds like BFRs. While serum levels reflect recent or circulating exposure, they may not fully account for the total body burden of BFRs, which can be stored in adipose tissue and other compartments over time. Alternative approaches, such as measuring BFRs concentrations in fat biopsies, breast milk, or hair, could provide more comprehensive assessments of cumulative exposure but were not feasible within the constraints of this study. Future research should consider integrating these biomarkers to better capture the full scope of BFRs exposure. Third, the pathogenic characteristics and mechanisms of BFRs in both animals and humans remain unclear. Additionally, the long-term accumulation effects of BFRs in the body may require extended follow-up periods for a comprehensive assessment.
Overall, our study demonstrates a significant association between BFRs exposure and PhenoAgeAccel, revealing gender differences in exposure effects. This finding offers new insights into how environmental pollutants can influence biological aging. These results underscore the importance of public health interventions aimed at reducing BFRs exposure. Moreover, given the greater susceptibility observed in males, targeted exposure reduction strategies—such as regulatory restrictions, workplace safety measures, and public awareness—are essential to mitigate potential health risks. Future research should include more longitudinal studies to determine the causal relationship between BFRs exposure and aging and validate these findings in broader populations, while also exploring the underlying mechanisms involved.
Data availability statement
Publicly available datasets were analyzed in this study. This data can be found at: nhanes: https://wwwn.cdc.gov/nchs/nhanes/Default.aspx.
Ethics statement
The studies involving humans were approved by National Center for Health Statistics (NCHS), the Centers for Disease Control and Prevention (CDC). The studies were conducted in accordance with the local legislation and institutional requirements. The participants provided their written informed consent to participate in this study.
Author contributions
WK: Conceptualization, Investigation, Software, Validation, Visualization, Writing – original draft, Writing – review & editing. YX: Conceptualization, Data curation, Formal analysis, Methodology, Writing – original draft. YJ: Methodology, Project administration, Resources, Writing – original draft.
Funding
The author(s) declare that financial support was received for the research and/or publication of this article. This work was supported by Zhejiang Traditional Chinese Medicine Administration (2024ZF027), Medical Science and Technology Project of Zhejiang Province (2024KY320), and Ningbo Municipal Bureau of Science and Technology (2023Y94).
Acknowledgments
The authors thank National Health and Nutrition Examination Survey for publicly available data.
Conflict of interest
The authors declare that the research was conducted in the absence of any commercial or financial relationships that could be construed as a potential conflict of interest.
Generative AI statement
The authors declare that no Gen AI was used in the creation of this manuscript.
Publisher’s note
All claims expressed in this article are solely those of the authors and do not necessarily represent those of their affiliated organizations, or those of the publisher, the editors and the reviewers. Any product that may be evaluated in this article, or claim that may be made by its manufacturer, is not guaranteed or endorsed by the publisher.
Supplementary material
The Supplementary material for this article can be found online at: https://www.frontiersin.org/articles/10.3389/fpubh.2025.1513757/full#supplementary-material
Abbreviations
BFRs, Brominated flame retardants; NHANES, National Health and Nutrition Examination Survey; BMI, Body mass index (weight [kg]/height[m]2); CI, Confidence intervals; eGFR, Estimations of glomerular filtration rate; DM, Diabetes mellitus; CDC, Centers for disease control and prevention; MEC, Mobile examination center; LOD, Limit of detection; PIR, Poverty-to-income ratio; PhenoAgeAccel, Phenotypic age acceleration; CVD, Cardiovascular disease; SD, Standard deviations; RCS, Restricted cubic spline; WQS, Weighted quantile sum; BKMR, Bayesian Kernel Machine Regression.
References
1. Jiang, L, Yang, J, Yang, H, Kong, L, Ma, H, Zhu, Y, et al. Advanced understanding of the polybrominated diphenyl ethers (PBDEs): insights from total environment to intoxication. Toxicology. (2024) 509:153959. doi: 10.1016/j.tox.2024.153959
2. Sharkey, M, Harrad, S, Abou-Elwafa Abdallah, M, Drage, DS, and Berresheim, H. Phasing-out of legacy brominated flame retardants: the UNEP Stockholm convention and other legislative action worldwide. Environ Int. (2020) 144:106041. doi: 10.1016/j.envint.2020.106041
3. Zhu, ZC, Chen, SJ, Zheng, J, Tian, M, Feng, AH, Luo, XJ, et al. Occurrence of brominated flame retardants (BFRs), organochlorine pesticides (OCPs), and polychlorinated biphenyls (PCBs) in agricultural soils in a BFR-manufacturing region of North China. Sci Total Environ. (2014) 481:47–54. doi: 10.1016/j.scitotenv.2014.02.023
4. Chen, L, Yu, K, Huang, C, Yu, L, Zhu, B, Lam, PKS, et al. Prenatal transfer of polybrominated diphenyl ethers (PBDEs) results in developmental neurotoxicity in zebrafish larvae. Environ Sci Technol. (2012) 46:9727–34. doi: 10.1021/es302119g
5. Kuriyama, SN, Wanner, A, Fidalgo-Neto, AA, Talsness, CE, Koerner, W, and Chahoud, I. Developmental exposure to low-dose PBDE-99: tissue distribution and thyroid hormone levels. Toxicology. (2007) 242:80–90. doi: 10.1016/j.tox.2007.09.011
6. Emond, C, Raymer, JH, Studabaker, WB, Garner, CE, and Birnbaum, LS. A physiologically based pharmacokinetic model for developmental exposure to BDE-47 in rats. Toxicol Appl Pharmacol. (2010) 242:290–8. doi: 10.1016/j.taap.2009.10.019
7. Feiteiro, J, Mariana, M, and Cairrão, E. Health toxicity effects of brominated flame retardants: from environmental to human exposure. Environ Pollut. (2021) 285:117475. doi: 10.1016/j.envpol.2021.117475
8. López-Otín, C, Blasco, MA, Partridge, L, Serrano, M, and Kroemer, G. Hallmarks of aging: an expanding universe. Cell. (2023) 186:243–78. doi: 10.1016/j.cell.2022.11.001
9. Xia, X, Wang, Y, Yu, Z, Chen, J, and Han, JJ. Assessing the rate of aging to monitor aging itself. Ageing Res Rev. (2021) 69:101350. doi: 10.1016/j.arr.2021.101350
10. Kuo, CL, Pilling, LC, Liu, Z, Atkins, JL, and Levine, ME. Genetic associations for two biological age measures point to distinct aging phenotypes. Aging Cell. (2021) 20:e13376. doi: 10.1111/acel.13376
11. Earls, JC, Rappaport, N, Heath, L, Wilmanski, T, Magis, AT, Schork, NJ, et al. Multi-Omic biological age estimation and its correlation with wellness and disease phenotypes: a longitudinal study of 3,558 individuals. J Gerontol A Biol Sci Med Sci. (2019) 74:S52–s60. doi: 10.1093/gerona/glz220
12. Hannum, G, Guinney, J, Zhao, L, Zhang, L, Hughes, G, Sadda, SV, et al. Genome-wide methylation profiles reveal quantitative views of human aging rates. Mol Cell. (2013) 49:359–67. doi: 10.1016/j.molcel.2012.10.016
13. Cohen, AA, Milot, E, Yong, J, Seplaki, CL, Fülöp, T, Bandeen-Roche, K, et al. A novel statistical approach shows evidence for multi-system physiological dysregulation during aging. Mech Ageing Dev. (2013) 134:110–7. doi: 10.1016/j.mad.2013.01.004
14. Levine, ME, Lu, AT, Quach, A, Chen, BH, Assimes, TL, Bandinelli, S, et al. An epigenetic biomarker of aging for lifespan and healthspan. Aging (Albany NY). (2018) 10:573–91. doi: 10.18632/aging.101414
15. Frenoy, P, Perduca, V, Cano-Sancho, G, Antignac, JP, Severi, G, and Mancini, FR. Application of two statistical approaches (Bayesian kernel machine regression and principal component regression) to assess breast cancer risk in association to exposure to mixtures of brominated flame retardants and per- and polyfluorinated alkylated substances in the E3N cohort. Environ Health. (2022) 21:27. doi: 10.1186/s12940-022-00840-4
16. Yin, W, Xu, R, Zou, J, Wang, Y, and Zhang, Y. Single and combined association between brominated flame retardants and cardiovascular disease: a large-scale cross-sectional study. Front Public Health. (2024) 12:1357052. doi: 10.3389/fpubh.2024.1357052
17. Pham-Lake, C, Aronoff, EB, Camp, CR, Vester, A, Peters, SJ, and Caudle, WM. Impairment in the mesohippocampal dopamine circuit following exposure to the brominated flame retardant, HBCDD. Environ Toxicol Pharmacol. (2017) 50:167–74. doi: 10.1016/j.etap.2017.02.003
18. Che, Z, Jia, H, Chen, R, Pan, K, Fan, Z, Su, C, et al. Associations between exposure to brominated flame retardants and metabolic syndrome and its components in U.S. adults. Sci Total Environ. (2023) 858:159935. doi: 10.1016/j.scitotenv.2022.159935
19. Abe, N, Sasaki, M, and Nakajima, A. Tetrabromobisphenol a and hexabromocyclododecane, brominated flame retardants, trigger endoplasmic reticulum stress and activate necroptosis signaling in PC12 cells. Environ Toxicol Pharmacol. (2023) 98:104056. doi: 10.1016/j.etap.2022.104056
20. Pan, Y, Chen, Q, Yu, Y, Yang, H, Liu, Z, Xie, B, et al. Association between brominated flame retardants (BFRs) and periodontitis: results from a large population-based study. BMC Oral Health. (2024) 24:1025. doi: 10.1186/s12903-024-04796-4
21. Prevention. CfDCa. National Health and nutrition examination survey. Available online at: https://wwwncdcgov/nchs/nhanes/Defaultaspx.
22. Ronald, CA. Evaluation of statistical treatments of left-censored environmental data using coincident uncensored data sets. II. Group comparisons. Environ Sci Technol. (2015) 49:13439–46. doi: 10.1021/acs.est.5b02385
23. Krebs-Smith, SM, Pannucci, TE, Subar, AF, Kirkpatrick, SI, Lerman, JL, Tooze, JA, et al. Update of the healthy eating index: HEI-2015. J Acad Nutr Diet. (2018) 118:1591–602. doi: 10.1016/j.jand.2018.05.021
24. Kim, D, Vazquez-Montesino, LM, Li, AA, Cholankeril, G, and Ahmed, A. Inadequate physical activity and sedentary behavior are independent predictors of nonalcoholic fatty liver disease. Hepatology. (2020) 72:1556–68. doi: 10.1002/hep.31158
25. Cheng, D, Chen, Z, Zhou, J, Cao, Y, Xie, X, Wu, Y, et al. Association between brominated flame retardants (PBDEs and PBB153) exposure and hypertension in U.S. adults: results from NHANES 2005-2016. Environ Health. (2024) 23:64. doi: 10.1186/s12940-024-01103-0
26. Varlamov, O, Bethea, CL, and Roberts, CT Jr. Sex-specific differences in lipid and glucose metabolism. Front Endocrinol (Lausanne). (2015) 5:241. doi: 10.3389/fendo.2014.00241
27. Meerts, IA, Letcher, RJ, Hoving, S, Marsh, G, Bergman, A, Lemmen, JG, et al. In vitro estrogenicity of polybrominated diphenyl ethers, hydroxylated PDBEs, and polybrominated bisphenol a compounds. Environ Health Perspect. (2001) 109:399. doi: 10.2307/3454900
28. Plunk, EC, and Richards, SM. Endocrine-disrupting air pollutants and their effects on the hypothalamus-pituitary-gonadal axis. Int J Mol Sci. (2020) 21:9191. doi: 10.3390/ijms21239191
29. Allen, JG, Gale, S, Zoeller, RT, Spengler, JD, Birnbaum, L, and McNeely, E. PBDE flame retardants, thyroid disease, and menopausal status in U.S. women. Environ Health. (2016) 15:60. doi: 10.1186/s12940-016-0141-0
30. Andreas, S, Lee-Yang, W, Richard, SJ, Park, A, Zhang, Y, Hodge, C, et al. Serum concentrations of polybrominated diphenyl ethers (PBDEs) and polybrominated biphenyl (PBB) in the United States population: 2003-2004. Environ Sci Technol. (2008) 42:1377–84. doi: 10.1021/es702451p
31. Zucker, I, and Prendergast, BJ. Sex differences in pharmacokinetics predict adverse drug reactions in women. Biol Sex Differ. (2020) 11:32. doi: 10.1186/s13293-020-00308-5
32. Welshons, WV, Thayer, KA, Judy, BM, Taylor, JA, Curran, EM, and vom Saal, FS. Large effects from small exposures. I. Mechanisms for endocrine-disrupting chemicals with estrogenic activity. Environ Health Perspect. (2003) 111:994–1006. doi: 10.1289/ehp.5494
33. Qin, WP, Li, CH, Guo, LH, Ren, XM, and Zhang, JQ. Binding and activity of polybrominated diphenyl ether sulfates to thyroid hormone transport proteins and nuclear receptors. Environ Sci Process Impacts. (2019) 21:950–6. doi: 10.1039/C9EM00095J
34. Montalbano, AM, Albano, GD, Anzalone, G, Moscato, M, Gagliardo, R, di Sano, C, et al. Cytotoxic and genotoxic effects of the flame retardants (PBDE-47, PBDE-99 and PBDE-209) in human bronchial epithelial cells. Chemosphere. (2020) 245:125600. doi: 10.1016/j.chemosphere.2019.125600
35. Wang, Y, Li, X, Qu, T, Huang, F, Xu, J, Gao, H, et al. Defective ferritinophagy and imbalanced iron metabolism in PBDE-47-triggered neuronal ferroptosis and salvage by Canolol. Sci Total Environ. (2024) 934:173118. doi: 10.1016/j.scitotenv.2024.173118
36. Messina, CM, Espinosa Ruiz, C, Regoli, F, Manuguerra, S, D'Agostino, F, Avellone, G, et al. BDE-47 exposure modulates cellular responses, oxidative stress and biotransformation related-genes in Mytilus galloprovincialis. Fish Shellfish Immunol. (2020) 107:537–46. doi: 10.1016/j.fsi.2020.11.015
37. Zhang, C, Li, P, Zhang, S, Lei, R, Li, B, Wu, X, et al. Oxidative stress-elicited autophagosome accumulation contributes to human neuroblastoma SH-SY5Y cell death induced by PBDE-47. Environ Toxicol Pharmacol. (2017) 56:322–8. doi: 10.1016/j.etap.2017.10.007
38. Hou, Y, Fu, J, Sun, S, Jin, Y, Wang, X, and Zhang, L. BDE-209 induces autophagy and apoptosis via IRE1α/Akt/mTOR signaling pathway in human umbilical vein endothelial cells. Environ Pollut. (2019) 253:429–38. doi: 10.1016/j.envpol.2019.07.030
39. Han, L, and Wang, Q. Association between brominated flame retardants exposure and markers of oxidative stress in US adults: an analysis based on the National Health and nutrition examination survey 2007-2016. Ecotoxicol Environ Saf. (2023) 263:115253. doi: 10.1016/j.ecoenv.2023.115253
Keywords: phenotypic age, aging, brominated flame retardants, NHANES, sex-specific
Citation: Kong W, Xie Y and Jin Y (2025) Sex-specific associations between brominated flame retardants exposure and phenotypic age acceleration in NHANES 2005–2010. Front. Public Health. 13:1513757. doi: 10.3389/fpubh.2025.1513757
Edited by:
Yuquan Chen, Monash University, AustraliaReviewed by:
Christopher Kirk Thompson, Virginia Tech, United StatesAudrei Pavanello, University Center of Maringá (UniCesumar), Brazil
Copyright © 2025 Kong, Xie and Jin. This is an open-access article distributed under the terms of the Creative Commons Attribution License (CC BY). The use, distribution or reproduction in other forums is permitted, provided the original author(s) and the copyright owner(s) are credited and that the original publication in this journal is cited, in accordance with accepted academic practice. No use, distribution or reproduction is permitted which does not comply with these terms.
*Correspondence: Weiliang Kong, TGl2ZWtvbmdAaG90bWFpbC5jb20=
†These authors have contributed equally to this work