- 1Department of Cardiology, General Hospital of Ningxia Medical University, Yinchuan, China
- 2The First Clinical College of Ningxia Medical University, Yinchuan, China
- 3Department of Intervention, Tongxin County People’s Hospital, Wuzhong, China
- 4Institute of Basic Medical Sciences, Xi’an Medical University, Xi’an, China
- 5Department of Geriatric, General Hospital of Ningxia Medical University, Yinchuan, China
Background: Abdominal aortic calcification (AAC) is one of the earliest observed forms of atherosclerotic calcification and is crucial for early cardiovascular risk prediction. Frailty, a global clinical and public health challenge, is associated with increased risks of mortality, functional decline, and loss of independence. However, the relationship between the Frailty Index (FI) and AAC among middle-aged and older adults has yet to be explored.
Methods: This study analyzed data from 2013 to 2014 National Health and Nutrition Examination Survey (NHANES) cohort, focusing on individuals aged ≥ 40 years. The FI was calculated using a 49-item model to assess frailty status and participants were stratified into three groups: non-frail (FI ≤ 0.15), pre-frail (0.15 < FI ≤ 0.25), and frail (FI > 0.25). AAC was measured by dual-energy X-ray absorptiometry and quantified by Kauppila scores. Severe AAC was defined as an AAC score > 6. The relationship between FI and AAC was investigated using multivariable logistic regression, sensitivity analyses, and smoothing curve fitting. Subgroup analyses and interaction tests were conducted to assess the stability of this association across different populations.
Results: A total of 2,572 participants were enrolled in this study. Following adjustment for potential confounders, FI exhibited a statistically significant positive association with both AAC score (β = 2.64, 95%CI = 1.20–4.08) and Severe AAC (OR = 6.36, 95%CI = 1.48–27.41). Similar trends (P for trend < 0.05) were observed when FI was analyzed as a categorical variable. Smooth curve fitting and subgroup analysis were used to investigate the relationship between baseline FI Z-score and AAC score and Severe AAC. Interestingly, we found that the FI Z-score was linearly related to the occurrence of severe AAC, while it was nonlinearly related to the AAC score. The FI-Z score was positively associated with the likelihood of AAC score before the breakpoint (K = 0.78), but not significant after the breakpoint. The association between FI-Z score and Severe AAC was stable in the different subgroups (all P for interaction > 0.05).
Conclusion: Our study indicated a stable positive correlation between FI and AAC. FI may serve as a biomarker for early subclinical atherosclerosis detection in middle-aged and older US adults.
1 Introduction
Cardiovascular disease (CVD) is the leading contributor to mortality and morbidity in both the United States (US) and worldwide, leading to a heavy burden on the medical system (1, 2). The prevention of CVD is a matter of concern within the realms of research and health policy (2). Vascular calcification (VC) refers to a prevalent vascular condition characterized by the anomalous accumulation of minerals within the media or lipoproteins within the intima, is a potential risk factor for the progression and rupture of atherosclerotic plaques and has been highly associated with CVD events (3). It is a common condition in individuals with diabetes and chronic kidney disease (CKD), affecting over 70% of patients (4). Even in the young adults with end-stage renal disease (ESRD) who do not exhibit common cardiovascular risk factors like hypertension or dyslipidemia, this phenomenon still occurs (5). To data, the mechanism of VC is complex and not well-defined, possibly linked to uncontrolled mineralization, homeostasis of calcium and phosphorus levels, imbalance between osteochondrogenic signaling, inflammatory responses, endoplasmic reticulum stress, mitochondrial dysfunction, iron homeostasis, cell apoptosis and degradation of substrates (6). Animal studies have demonstrated the potential efficacy of statins and calcium channel blockers (CCBs) in treating VC, however, their effectiveness in human subjects is still a topic of debate (7, 8). While studies in rat models have demonstrated the efficacy of sodium thiosulfate in treating VC, its clinical application in humans requires verification through more extensive randomized controlled trials to ensure both safety and therapeutic effectiveness (9). Given the association of VC with CVDs and mortality, along with the treatment challenges involved, the prevention and improvement method of VC deserve further exploration.
Abdominal aortic calcification (AAC), a prevalent form of VC, develops earlier than coronary artery calcification (CAC) and has demonstrated the ability to forecast subclinical CVDs and subsequent CVD events, irrespective of traditional risk factors. Data from the Framingham Heart Study demonstrate a sex-specific disparity in AAC prevalence, with males under 45 years exhibiting a 22.4% incidence compared to 16.4% in females (10). In a 13-year prospective cohort study of 2,056 community-dwelling U.S. women, the prevalence of AAC increases with age, from 60% in 65–69 years of age to 96% in 85 years and older (11). A long-term prospective follow-up study indicated that AAC not only serves as a marker of subclinical atherosclerotic disease and an independent predictor of cardiovascular morbidity and mortality, but also exhibits a dose–response relationship where the risk of CVD mortality increases proportionally with the severity of AAC scores (12). In order to access AAC, the Kauppila AAC score ranged from 0 to 24, developed by Kauppila and colleagues (13), is a quantifiable methodology utilizing lateral lumbar radiographs to assess abdominal aortic calcification. AAC score > 6 was used as a commonly reported cut-off point for severe AAC has been extensively defined in prior investigations (14, 15) and has been shown to forecast all-cause mortality and CVD mortality independently. In a longitudinal cohort study of 396 Japanese hemodialysis patients spanning from 2005 to 2014, distinct trajectories of abdominal aortic calcification were identified through group-based modeling, demonstrating that rapid nonlinear progression (adjusted HR 1.91, 95%CI 1.02–3.58) and advanced baseline with slow progression (adjusted HR 2.79, 95%CI 1.44–5.11) were independent predictors of heightened all-cause mortality risk when contrasted with stable patterns (16). Furthermore, there was a notable association between AAC progression and the development of CVD and myocardial infarction, with HR of 1.6 and 2.1, respectively (17). Additionally, there is a potential link between AAC and lower bone mineral density, increased fracture risk, and a decline in handgrip strength in the older adult (18, 19). Therefore, prevention and early detection of AAC, to slow its progression, are worth exploring and may be benefit to the patients.
The extension of human lifespan is intricately connected to the concomitant rise in chronic comorbidities and frailty syndromes, significantly impacting healthcare systems (20). Frailty, a geriatric syndrome characterized by diminished physiological capacity and increased vulnerability to stressors, susceptibility to stress, and impaired ability to maintain homeostasis, leading to negative patient outcomes (21). Due to the multiple multisystem dysfunctions associated with frailty, there is currently no single diagnostic tool or biomarker available for identifying and evaluating frailty. At present, the most widely used principal models to operationalize the frailty concept are the frail phenotype (22) (assessed by physical performances) and the frailty index (23) (assessed by multidimensional checklists encompassing biological, psychological, and sociological aspects). Frailty is highly common among the older adult population, substantially increasing the risk of negative health outcomes such as fall episodes, hospitalization, functional impairment, major CVD events and CVD mortality (24, 25). While frailty is also a reversible state, and decreased CVD risks were observed in individuals who reversed their frailty status (26). The multifactorial pathogenesis of frailty emerges through the convergence of age-related biological cascades, principally involving dysregulated neuroplasticity, endocrine axis disruption, persistent pro-inflammatory states, and tissue-specific epigenetic remodeling, which collectively drive systemic vulnerability in older populations (27, 28). Central nervous system dysfunction and musculoskeletal degeneration are considered key pathological events during the course of frailty (27), however, the link between cardiovascular disease (CVD) and frailty has garnered increased attention due to the acknowledgment that the aging process accelerates the onset and progression of age-related illnesses (29). Studies on the association between cardiovascular disease and frailty have mainly focused on endpoint events, with few studies reporting the association between frailty and subclinical atherosclerotic events. Although the FI is associated with various chronic diseases, the relationship between the FI and AAC and its potential clinical value are still unclear.
To fill the knowledge gap, the purpose of this study is to investigate the association between FI and AAC among middle-aged and older adults in US using the 2013–2014 National Health and Nutrition Examination Survey (NHANES) dataset. Through this study, the FI may become a valuable clinical indicator for early detection and managing subclinical atherosclerosis in clinical practice.
2 Methods
2.1 Participants and study design
This population-based cross-sectional study was conducted using data from the NHANES project, overseen by the National Center for Health Statistics at the Centers for Disease Control and Prevention (CDC) in the United States. The Institutional Review Board of the National Center of Health Statistics approved the initial survey protocol. All participants provided an informed consent form and complied with the Declaration of Helsinki (30). All NHANES data used in this analysis are publicly available at https://www.cdc.gov/nchs/nhanes. Since the dual-energy X-ray absorptiometry (DXA) scan was only performed during 2013–2014 in NHANES, participants from this period were included (31). After excluding participants under 40 years old (n = 6,360), those who were pregnant (n = 3), had invalid scans (n = 190), or were not scanned for other reasons (n = 482), 3,140 participants with complete AAC data were included. Additional exclusion criteria were missing data on covariates: smoking status (n = 2), alcohol consumption (n = 196), poverty status (n = 221), education status (n = 1), marital status (n = 4), stroke (n = 4), chronic kidney disease (CKD) (n = 87), coronary heart disease (CHD) (n = 5), body mass index (BMI) (n = 15), serum calcium (n = 25), and serum uric acid (n = 2). Ultimately, 2,572 adult participants were included in the primary analysis (Figure 1).
2.2 Measurement of frailty index
We assessed frailty using the frailty index (FI) approach proposed by Rockwood et al. The 49-item FI used in previous studies covered different systems, including cognition (Memory lapses and cognitive challenges), dependence (difficulties in performing daily activities), depression (assessed using the PHQ-9), comorbidities (various chronic conditions), hospital utilization and general health, physical performance, body assessment (grip power and BMI), and laboratory data (including blood counts and glucose levels), constructed according to standardized procedures published earlier (23, 32). Scores were aggregated and then normalized by dividing the total by the number of items. A scoring system ranging from 0 to 1 was established to enable the amalgamation of continuous and categorical variables, aligning with the severity of the deficit (Supplementary Table S1). To facilitate analysis, we transformed this continuous score into a categorical variable based on cutoffs established in previous literature (33, 34). We categorized our sample into three groups: non-frail (FI ≤ 0.15), pre-frail (0.15 < FI ≤ 0.25), and frail (FI > 0.25). All items of FI are shown in. A detailed summary of the variables comprising the frailty index along with their respective scores can be found in Supplementary Table S1.
2.3 Abdominal aortic calcification
Each participant’s scan and phantom scan were analyzed by UCSF using standard radiologic techniques and study specific NHANES protocols. The severity of abdominal aorta calcification was quantified using the AAC score. It was calculated based on Kauppila score system (13) by dual-energy X-ray absorptiometry (DXA, Densitometer Discovery A, Hologic, Marlborough, MA, United States) for each participant. The Kauppila scoring method involved dividing the anterior and posterior aortic walls into four segments, corresponding to the areas in front of the lumbar vertebrae L1–L4. Scores were obtained separately for these walls, resulting in a range from 0 to 6 for each vertebral level and 0 to 24 for the total score. Higher AAC scores indicated more severe calcification in the abdominal aorta. An AAC score greater than 6 was used as a commonly reported cut-off point for severe abdominal aortic calcification in previous study (35, 36). Both AAC score and severe AAC were included as outcome variables in our analysis.
2.4 Demographic characteristics and other covariate
In the analysis, we included self-reported data on age, sex, education, race, alcohol consumption, smoking status, and medical history. Race was classified into non-Hispanic Black, non-Hispanic White, Mexican American, and Multiracial/other races. Education level was simplified into three categories: below high school (less than 11th grade), high school graduate or General Educational Development (GED), and some college or higher (Associate’s degree or above). Additionally, marital status was grouped as either married or living with a partner, or as widowed/divorced/separated/never married. The Poverty-Income Ratio (PIR), an index of income relative to federally established poverty thresholds that accounts for economic inflation and family size, was estimated by dividing monthly family income by the poverty level (37). PIR was then classified into three groups: <1.30, 1.31 to 3.50, and >3.50. Nicotine exposure, alcohol use, and histories of diabetes, coronary heart disease (CHD), hypertension, stroke, and chronic kidney disease (CKD) were obtained via self-report questionnaires. Smoking status was assessed and classified as “never smoker” for individuals who smoked less than 100 cigarettes in their lifetime, “former smoker” for those who had smoked more than 100 cigarettes but currently do not smoke, and “current smoker” for individuals who smoked more than 100 cigarettes in their lifetime and smoke some days or every day (38, 39). Alcohol users were categorized into the following groups based on a previous study (40): “non-drinks,” “ex-drinks,” “current-drinks.” Diabetes was defined as meeting one of the following criteria: (1) self-reported diagnosis of diabetes by a doctor, (2) taking hypoglycemic drugs, (3) glycated hemoglobin (HbA1c) ≥ 6.5%, or (4) fasting blood glucose ≥ 7.0 mmol/L. Similarly, hypertension was defined as the self-reported diagnosis of hypertension by a doctor, taking antihypertensive medications, or an average systolic blood pressure ≥ 140 mmHg or average diastolic blood pressure ≥ 90 mmHg. Stroke was diagnosed among those who responded “yes” to the self-reported stroke question (“Has a doctor or other health professional ever told you that you had a stroke?”) (39). CHD was diagnosed among individuals responding “yes” to the self-reported CHD question (“Has a doctor or other health professional ever told you that you had coronary heart disease?”). CKD was diagnosed among individuals responding “yes” to the self-reported CKD question (“Has a doctor or other health professional ever told you had weak/failing kidneys?”). Height and weight were collected at the mobile examination center (MEC), and BMI was calculated using the formula: body weight (kg)/height squared (m2). Clinical indicators such as serum total calcium, serum phosphorus, serum alkaline phosphatase, serum creatinine, serum uric acid, triglycerides (TG), total cholesterol (TC), low-density lipoprotein cholesterol (LDL-C), and high-density lipoprotein cholesterol (HDL-C) were measured in the NHANES laboratory.
2.5 Statistical analysis
For continuous variables that met normal distribution, the mean ± standard deviation (SD) was used for statistical description, and an independent samples t-test was used for inter-group comparison. If the variables did not meet normal distribution, the median (Q1, Q3) was used for description, and the rank sum test was used for inter-group comparison. For categorical data, the number of cases (%) was used for description, and the chi-square test was used for comparison between groups. Fisher’s exact probability was employed when the chi-square test assumptions were not satisfied. Continuous data were compared using the Kruskal-Wallis test or one-way analysis of variance (ANOVA), and categorical data were compared using the chi-squared test. Covariates selected according to previous research (15, 41) and clinical expertise, which could potentially influence the relationship between FI and AAC, were further added to the multivariate Cox model. We used four levels of adjustment. Model 1, no covariates were adjusted. Model 2 was adjusted for age and sex. Model 3 was adjusted for age, sex, race and ethnicity, poverty income ratio (categorical), marital status, and education levels. Model 4 was adjusted for age, sex, race and ethnicity, poverty income ratio (categorical), marital status, education levels, hypertension, body mass index, total cholesterol, serum total calcium, serum phosphorus, serum creatinine, and serum alkaline phosphatase. We further conducted sensitivity analysis after categorizing the FI group to evaluate robustness. To facilitate the interpretation of regression coefficients (42, 43), FI was converted to FI Z-score using Z-score transformations for further analysis. A generalized additive model (GAM) and smooth curve fittings were employed to address nonlinearity between FI Z-score and AAC. When a non-linear correlation was observed, a two-piecewise linear regression model (segmented regression model) was used to fit each interval and calculate the threshold effect. A log-likelihood ratio test comparing the one-line model (non-segmented) to the two-piecewise linear regression model was conducted to determine the existence of a threshold. The breakpoint (K) was further determined by a two-step recursive method (39).
Additionally, subgroup analysis of the associations between FI Z-score and AAC (AAC score and Severe AAC) was conducted using stratified multivariable logistic regression models. Stratified factors included sex, age (40–65, and ≥65 years), DM, CHD, CKD, PIR (<1.30, 1.31–3.50, >3.50), Marital Status (status 1: married or living with a partner, status 2:widowed/divorced/separated/never married) which were also treated as potential effect modifiers. An interaction term was added to evaluate heterogeneity using the likelihood ratio test. All analyses were performed using R version 4.1.3 (http://www.R-project.org, The R Foundation) and Empower software (www.empowerstats.com; X&Y Solutions, Inc., Boston, MA). Statistical significance was considered at a two-sided p < 0.05.
3 Results
3.1 Baseline characteristics of the study population
Table 1 shows that a total of 2,572 participants were enrolled in this study, with an average age of 58.95 ± 11.95 years, including 1,244 males (48.37%). The prevalence of the non-frail state (FI ≤ 0.15), pre-frail state (0.15 < FI ≤ 0.25), and frail state (FI > 0.25) was 53.97, 29.24, and 17.61%, respectively. The median AAC score was 0 (Q1, Q3: 0.00–2.00) in the overall cohort, showing progressive increases across FI categories [non-frail: 0 (Q1, Q3: 0.00–1.00); pre-frail:0.00 (Q1, Q3: 0.00–2.00); frail: 0 (Q1, Q3: 0.00–3.00), p < 0.001]. The overall prevalence of severe AAC was 11.12%, and it also increased with the FI index (non-frail: 7.13%; pre-frail: 14.34%; frail: 18.20%; p < 0.001). Those with higher FI were notably older, more likely to be female, non-Hispanic white, below high school education, with a poverty ratio < 1.3, and never married, widowed, divorced, or separated (all p values < 0.05). Furthermore, individuals with a history of hypertension, diabetes, stroke, heart disease, or CKD had higher FI (all p values < 0.05). Participants with higher FI displayed higher BMI, serum phosphorus, serum uric acid, serum alkaline phosphatase, serum creatinine, and triglycerides, but lower total cholesterol, HDL-C, and LDL-C (all p values < 0.05). However, there was no significant difference in serum total calcium (p > 0.05) (Table 1).
3.2 Relationship between FI and severe ACC
Table 2 shows the association between FI and severe AAC. We found that higher FI Z-score and FI were correlated with severe AAC in both unadjusted and adjusted models. After full adjustment (adjusted for age, sex, race and ethnicity, poverty income ratio, marital status, education levels, hypertension, body mass index, total cholesterol, serum total calcium, serum phosphorus, serum creatinine, and serum alkaline phosphatase), participants with a unit higher FI Z-score had a 20% increased risk of severe AAC (OR = 1.20, 95% CI 1.04–1.39). Participants with a unit higher FI had a 5.36-fold increased risk of severe AAC (OR = 6.36, 95% CI 1.48–27.41). When FI was categorized as non-frail, pre-frail, and frail, participants in the pre-frail status exhibited a significantly 0.41-fold increased likelihood compared to the non-frail status (OR = 1.41, 95% CI 1.02–1.97; P for trend = 0.038).
In addition, a linear relationship was detected according to the results of smooth curve fitting between FI and severe AAC after full adjustment (p value for LRT test = 0.1) [adjusted for age, sex, race and ethnicity, poverty income ratio (categorical), marital status, education levels, hypertension, body mass index, total cholesterol, serum total calcium, serum phosphorus, serum creatinine, and serum alkaline phosphatase] (Figure 2B; Table 3).
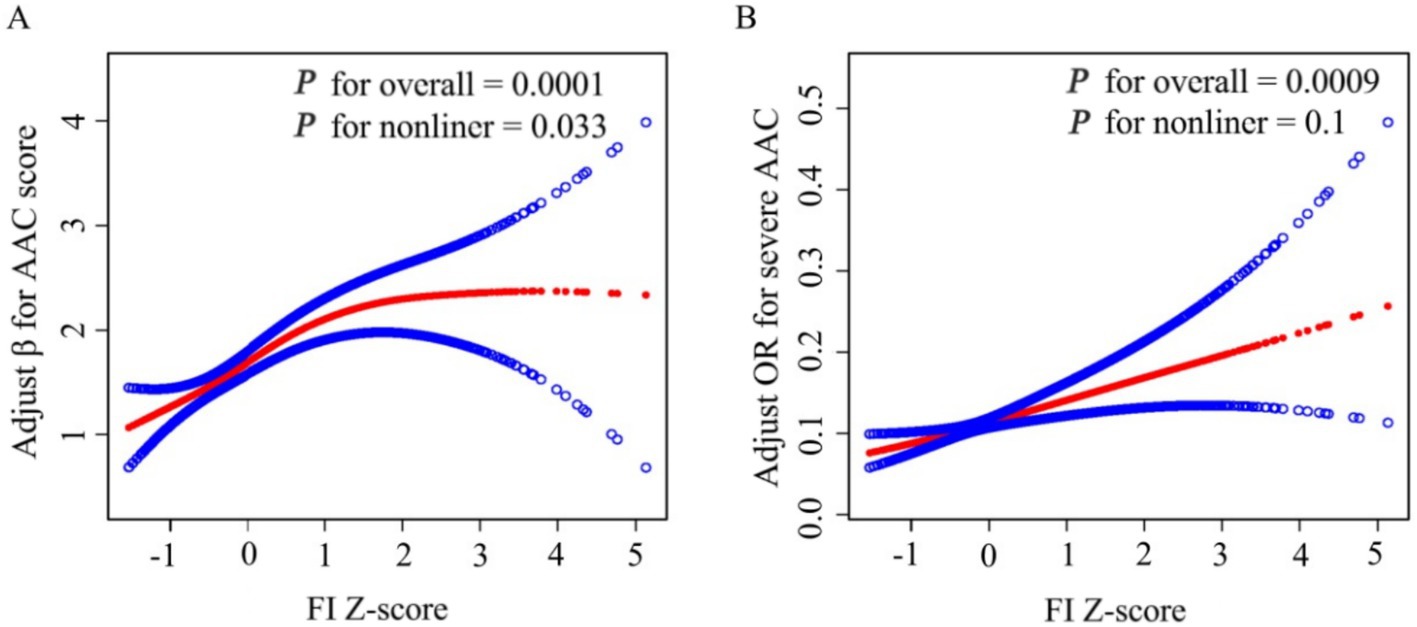
Figure 2. Smooth Curve Fitting Detected A nonlinear positive relationship between FI-Z score and AAC score (A) and a Liner relationship between FI Z-score and Sever AAC (B) by the generalized additive model. β/OR (Red lines) and 95% CI (under the blue curve areas) were adjusted for age, sex, race and ethnicity, poverty income ratio (category), marital status, education levels, hypertension, body mass index, total cholesterol, serum total calcium, serum phosphorus, serum creatinine, serum alkaline phosphatase.
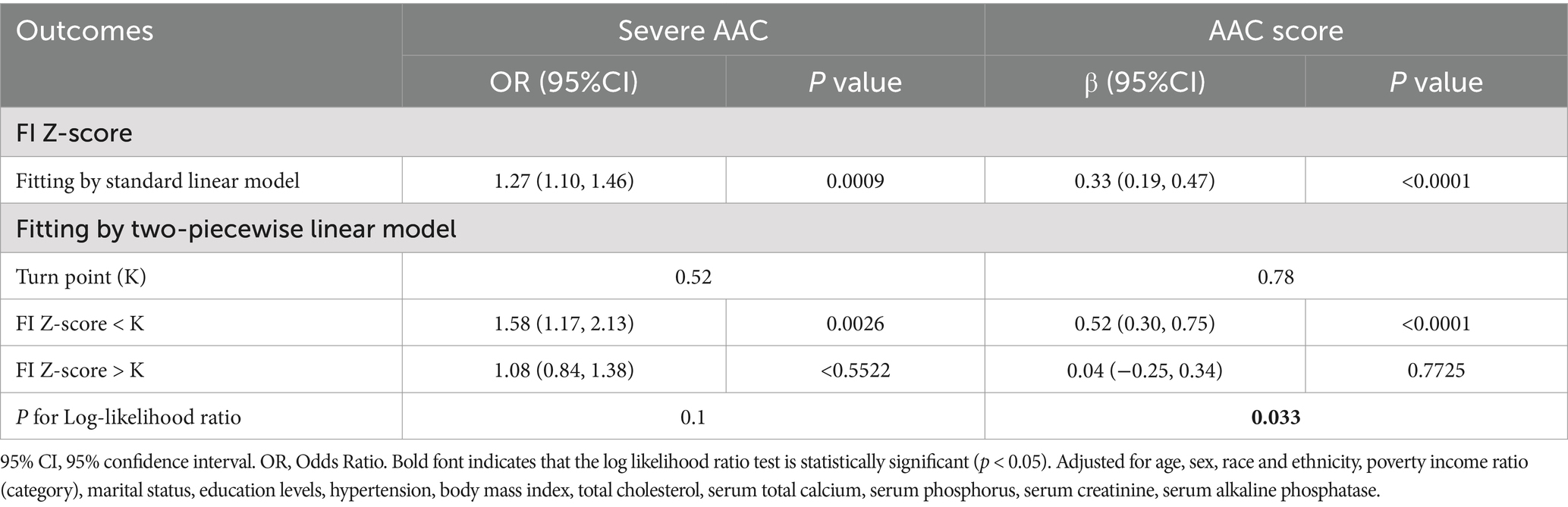
Table 3. Threshold effect analysis of FI Z-Score on AAC using a two-piecewise linear regression model.
3.3 A nonlinear relationship between FI and AAC score
FI was positively associated with the likelihood of a higher AAC score with statistical significance, and this association remained stable across our four models. After full adjustment, each unit increase in FI Z-score was associated with a 0.26 unit increase in AAC score (β = 0.26, 95% CI: 0.12–0.41). Each unit increase in FI was associated with a 1.64-fold increase in AAC score (β = 2.64, 95% CI: 1.20–4.08). Sensitivity analysis was conducted with frailty status as a categorical variable (non-frail, pre-frail, frail). In the fully adjusted model, compared with the non-frail status, the adjusted β for participants in pre-frail status and frail status were 0.51 and 0.65, respectively (Pre-frail: β = 0.51, 95% CI: 0.21–0.81; Frail: β = 0.65, 95% CI: 0.27–1.03; P for trend = 0.0001) (Table 2).
However, smooth curve fitting exhibited a non-linear relationship between FI and AAC score after full adjustment (p value for LRT test < 0.05) [adjusted for age, sex, race and ethnicity, poverty income ratio (categorical), marital status, education levels, hypertension, body mass index, total cholesterol, serum total calcium, serum phosphorus, serum creatinine, and serum alkaline phosphatase] (Figure 2A). Then, FI was converted to FI Z-score to analysis the dose-relationship. A generalized additive model (GAM) and smooth curve fittings were employed to address nonlinearity between FI Z-score and AAC. We further calculated the turning point (K) to be 0.78. On the left of the turning point, a positive relationship between FI Z-score and AAC score (β = 0.52, 95% CI: 0.30–0.75; p < 0.0001) was detected. However, no statistically significant relationship between FI Z-score and AAC score was found on the right of the turning point (β = 0.04, 95% CI: −0.25–0.34; p = 0.7725) (Table 3).
3.4 Subgroup analysis and interaction test
To evaluate whether the association between FI Z-score and AAC was consistent in the overall population and to identify potential differences among subgroups, we conducted subgroup analysis and interaction tests stratified by sex, age, DM, CHD, CKD, PIR, and marital status. In the subgroup analysis, the association between FI Z-score and AAC score was not consistently significant across certain groups (Figure 3A). Interaction testing indicated that gender, DM, CHD, CKD, PIR, and marital status did not significantly impact the association between FI and AAC score (all P for interaction > 0.05). However, the age subgroup significantly influenced this association (P-interaction < 0.05). The positive association between FI and AAC score appeared stronger in populations older than 65 years (β per 1 SD increase, 0.68; 95% CI: 0.46–0.90). We also found that FI Z-score was positively associated with severe AAC in subgroups stratified by gender (male or female), history of DM and CHD, CKD (yes or no), PIR > 1.3, and marital status. For severe AAC, we detected no statistical significance for gender, age, DM, CHD, CKD, PIR, and marital status (P-interaction > 0.05) (Figure 3B).
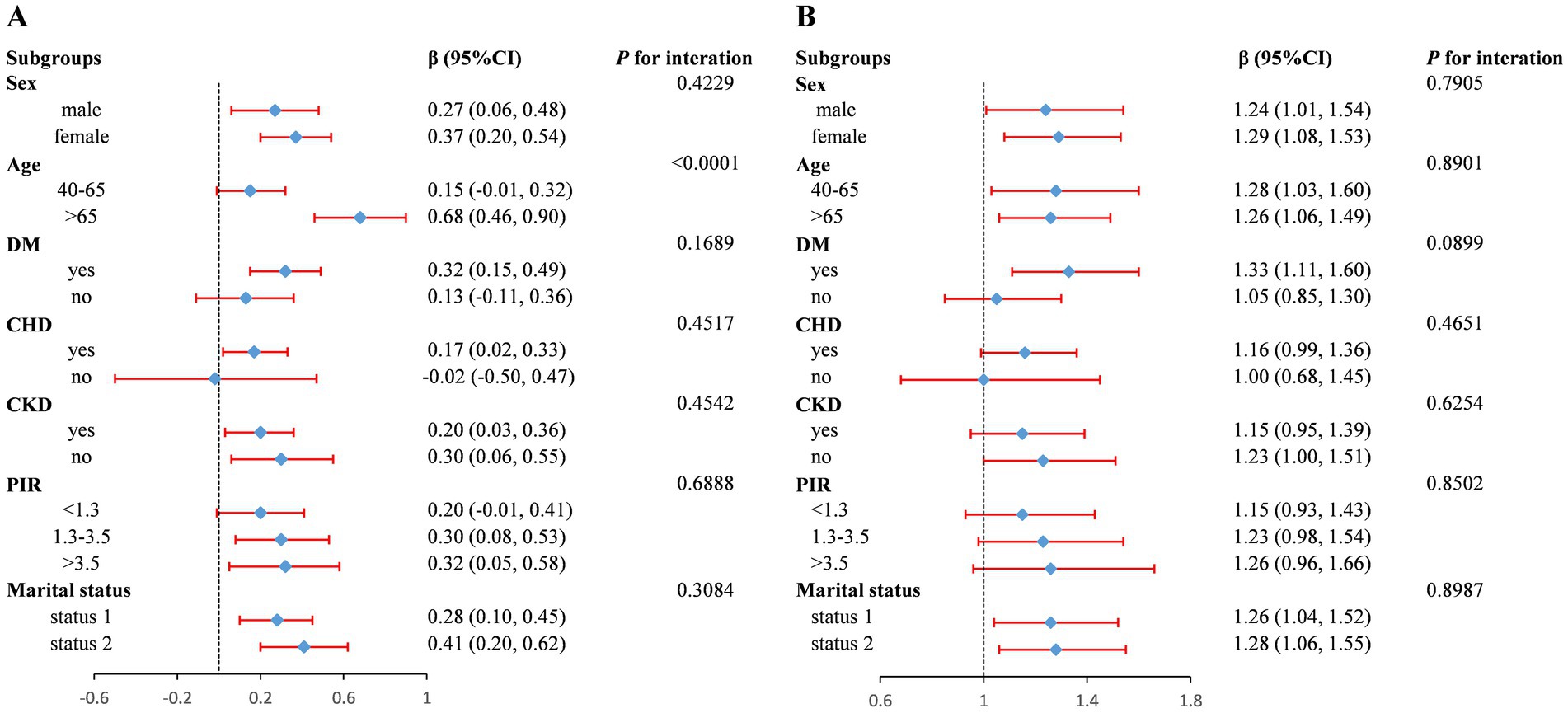
Figure 3. Forest plot of subgroup analysis for the association between FI Z-score and AAC score (A) and Severe AAC (B). Adjusted for age, sex, race and ethnicity, poverty income ratio (category), marital status, education levels, hypertension, body mass index, total cholesterol, serum total calcium, serum phosphorus, serum creatinine, serum alkaline phosphatase, except for the subgroup variable. OR, odds ratio; 95% CI, 95% confidence interval. Status 1: married or living with a partner; Status 2: widowed/divorced/separated/never married.
4 Discussion
In this cross-sectional study with a total of 2,572 subjects, we observed a positive correlation between the FI and AAC. Whether AAC was considered a continuous or categorical variable, the FI was found to be an independent risk factor for AAC. Interestingly, we found that the FI Z-score was linearly related to the occurrence of severe AAC through smoothing curves, while it was nonlinearly related to the AAC score. Different relationships of FI Z-score on AAC score were detected on the left and right sides of the breakpoint. FI Z-score was positively associated with the likelihood of AAC score on the left side of breakpoint (K = 0.78), while the association on the right of breakpoint was of no statistical significance. Our findings demonstrate a significant association between FI and AAC, suggesting FI may serve as a correlational biomarker requiring further validation of its temporal relationship with AAC pathogenesis.
To our knowledge, this is the first study to examine the relationship between FI and AAC, highlighting a direct link between FI severity and increased subclinical cardiovascular risk. The Frailty has been consistently associated with higher risks of multiple adverse health outcomes, including loss of ability to carry out daily activities (ADL), falls and fractures, disability, multiple chronic diseases, hospitalization, and even death. Additionally, a prospective cohort study of the Chinese population showed that the FI was associated with all-cause and cause-specific mortality, independent of the actual age of both young and older adult individuals (44). Among 2,894 adults diagnosed with type 2 diabetes mellitus (T2DM) in the NHANES study, a positive linear relationship was observed between higher FI and an increased risk of congestive heart failure (OR = 3.60, 95CI%: 2.34–5.54). Furthermore, individuals with higher FI exhibited an 86% higher risk of all-cause mortality (HR = 1.86, 95%CI:1.55–2.24) and a 66% elevated risk of cardiovascular mortality (HR = 1.66, 95%CI:1.18–2.33) in comparison to non-frail patients. A meta-analysis has shown that a genetically determined higher FI is associated with an odds ratio of 1.46 for coronary artery disease, 1.62 for myocardial infarction, and 1.46 for heart failure (45). Emerging evidence demonstrates that reversing frailty status significantly reduces the risk of cardiovascular events, underscoring the critical need to integrate frailty assessment into preclinical risk stratification (26). The Multi-Ethnic Study of Atherosclerosis (MESA) revealed that AAC exhibited a more robust association with cardiovascular mortality and all-cause mortality than CAC, indicating its earlier appearance in the progression of atherosclerosis. Thus, AAC emerges as a more clinically relevant and sensitive early biomarker of systemic atherosclerosis (46). Existing studies have mainly examined the link between frailty and clinical outcomes. However, the connection between the frailty index (FI) and subclinical cardiovascular conditions, specifically AAC as an early indicator of atherosclerosis, lacks thorough investigation. Moreover, Lin CH’ s research report that a significant association between frailty and subclinical peripheral arterial disease (measured by the Ankle-Brachial Index), and this relationship remains significant even when considering age, blood pressure, and blood lipid levels. Subsequently, The Multicenter AIDS Cohort Study (MACS) reveals that frailty is independently associated with subclinical coronary atherosclerosis in HIV-uninfected men, but not in HIV-infected men. In our study we also found that the FI was positively correlated with AAC and we observe a linear positive correlation between the FI and severe AAC in both unadjusted and adjusted models. Our study broadens existing knowledge by extending the findings to middle-aged cohorts and using a nationally representative sample from the United States. Additionally, we applied a more accurate and commonly used approach to define frailty, specifically the frailty index, which includes up to 49 items for assessment. Collectively, these findings indicate that the FI could function as a valuable biomarker for early identification of subclinical atherosclerosis and prediction cardiovascular events, as demonstrated in our research. Incorporating FI into routine screenings for symptom-free adults could improve preventive healthcare strategies.
The potential mechanisms of this positive association between FI and AAC has not been fully understood. Frailty is associated with diminished antioxidant defense and elevated oxidative stress markers, hastening the progression from prefrailty to frailty (47).Elevated inflammatory markers (such as TNF-α, IL-1β, IL-1, IL-6, and IL-18) and pro-inflammatory cytokines contribute to frailty by promoting protein catabolism (48, 49). The release of inflammatory mediators leads to endothelial dysfunction and vascular wall degradation, which increases the likelihood of AAC (50). Previous studies have also indicated that inflammatory mediators can activate vascular smooth muscle cell calcification through various pathways, leading to the occurrence and development of AAC (51, 52). Additionally, some studies have shown that the systemic immune-inflammatory index (SII), a new inflammation assessment indicator, is positively correlated with AAC. This association is more significant in the older adult and has potential value in identifying the severity of AAC (53). In addition to elevated levels of inflammation, frail older adult individuals often experience disturbances in calcium/phosphate metabolism and decreased bone mineral density, which are important causes of VC (54). Epidemiological studies have also reported an association between AAC and osteoporosis, accelerated bone loss, and vertebral and hip fractures (55). Notably, increased oxidative stress and oxidative stress-induced signaling are common features in frail populations (56), studies have shown that elevated intracellular oxidative stress, through oxidized lipids and H2O2, induces VSMC osteogenic differentiation and calcification (57). In chronic kidney disease patients, increased systemic oxidative stress is a key feature of the uremic state (58), which is highly correlated with increased VC (59). In summary, inflammatory response, metabolic imbalance, oxidative stress, and other factors are common pathophysiological changes in individuals with a high FI, and these changes trigger the occurrence of VC, including AAC, ultimately increasing the incidence and mortality of cardiovascular disease. Therefore, these studies explain the association between FI and AAC at the molecular mechanism level.
Although our study utilized a complex multi-stage probability sampling design, which enhanced the reliability and representativeness of our findings, we ultimately identified a significant positive association between FI and AAC. The findings of this study could provide a valuable insight for the management and prevention of subclinical atherosclerotic events. However, our study also possesses several potential limitations. Firstly, the cross-sectional design of this study prevented the establishment of causal relationships between FI and AAC. Future longitudinal, experimental studies or Mendelian randomization are crucial for elucidating these causal relationships, investigating whether the FI raises the risk of developing AAC or if the presence of AAC contributes to the deterioration of FI. Secondly, this study utilized self-reported retrospective data resulting in unavoidable recall bias and social desirability bias. Owing to the absence of data on these variables in NHANES, which are not included in the original dataset, thus constraining our inclusion of these covariates in the analysis. Thirdly, our study, like other observational studies, could not eliminate residual or unknown confounding factors or accidental confounding effects resulting from measurement errors and unmeasured variables such as psychosocial stress or genetic susceptibility. Future research is essential to explore the relationship and biological mechanisms between FI and AAC by incorporating advanced technologies such as machine learning and multi-omics approaches. Fourthly, NHANES was a study conducted in US, thus we could only evaluate the association between FI and AAC in US adults, the sample’s geographical and demographic specificity may restrict generalizability to other populations.
Our study has important clinical and public health implications. The findings of this study emphasize the notable link between FI and AAC, emphasizing the need for targeted clinical strategies to assess and manage frailty in older adults. By integrating FI assessments into routine clinical evaluations, healthcare providers can enhance early identification of individuals at elevated risk for subclinical atherosclerosis, ultimately promoting better cardiovascular health outcomes in aging populations. These investigations may ultimately contribute to the development of effective prevention and intervention aimed at mitigating the impact of subclinical atherosclerosis in vulnerable populations.
5 Conclusion
This cross-sectional study demonstrated that elevated FI levels were associated with higher severe AAC and a nonlinear positive relationship with AAC score, indicating that FI may serve as a biomarker for early subclinical atherosclerosis detection in US adults aged ≥ 40 years. Additionally, high-quality studies are necessary to validate our findings and establish a causal link between the two variables.
Data availability statement
All NHANES data used in this analysis are publicly available at https://www.cdc.gov/nchs/nhanes.
Ethics statement
This study was based on public datasets (NHANES). Ethical review and approval was not required for the study on human participants in accordance with the local legislation and institutional requirements. Written informed consent from the patients/participants or patients’/participants’ legal guardian/next of kin was not required to participate in this study in accordance with the national legislation and the institutional requirements.
Author contributions
ZZ: Data curation, Formal analysis, Writing – original draft. PW: Data curation, Formal analysis, Writing – original draft. SY: Data curation, Writing – original draft. BZ: Data curation, Writing – original draft. DC: Supervision, Writing – original draft. XL: Supervision, Writing – original draft. YW: Conceptualization, Methodology, Writing – review & editing, Supervision. NY: Methodology, Supervision, Writing – review & editing, Project administration, Conceptualization.
Funding
The author(s) declare that financial support was received for the research and/or publication of this article. This work was supported by the Natural Science Foundation of Ningxia, China (Grant numbers 2022AAC05058 and 2023AAC03620).
Acknowledgments
We acknowledged the contributions of NHANES staff for creating and updating the NHANES database.
Conflict of interest
The authors declare that the research was conducted in the absence of any commercial or financial relationships that could be construed as a potential conflict of interest.
Generative AI statement
The author(s) declare that no Gen AI was used in the creation of this manuscript.
Publisher’s note
All claims expressed in this article are solely those of the authors and do not necessarily represent those of their affiliated organizations, or those of the publisher, the editors and the reviewers. Any product that may be evaluated in this article, or claim that may be made by its manufacturer, is not guaranteed or endorsed by the publisher.
Supplementary material
The Supplementary material for this article can be found online at: https://www.frontiersin.org/articles/10.3389/fpubh.2025.1546647/full#supplementary-material
References
1. McClellan, M, Brown, N, Califf, RM, and Warner, JJ. Call to action: urgent challenges in cardiovascular disease: a presidential advisory from the American Heart Association. Circulation. (2019) 139:e44–54. doi: 10.1161/cir.0000000000000652
2. Khan, SS, and Greenland, P. Comprehensive cardiovascular health promotion for successful prevention of cardiovascular disease. JAMA. (2020) 324:2036–7. doi: 10.1001/jama.2020.18731
3. Nguyen, HL, Liu, J, Del Castillo, M, and Shah, T. Role of coronary calcium score to identify candidates for ASCVD prevention. Curr Atheroscler Rep. (2019) 21:53. doi: 10.1007/s11883-019-0812-8
4. Yahagi, K, Kolodgie, FD, Lutter, C, Mori, H, Romero, ME, Finn, AV, et al. Pathology of human coronary and carotid artery atherosclerosis and vascular calcification in diabetes mellitus. Arterioscler Thromb Vasc Biol. (2017) 37:191–204. doi: 10.1161/atvbaha.116.306256
5. Goodman, WG, Goldin, J, Kuizon, BD, Yoon, C, Gales, B, Sider, D, et al. Coronary-artery calcification in young adults with end-stage renal disease who are undergoing dialysis. N Engl J Med. (2000) 342:1478–83. doi: 10.1056/NEJM200005183422003
6. Pan, W, Jie, W, and Huang, H. Vascular calcification: molecular mechanisms and therapeutic interventions. MedComm. (2020) 4:e200. doi: 10.1002/mco2.200
7. Shimizu, T, Tanaka, T, Iso, T, Kawai-Kowase, K, and Kurabayashi, M. Azelnidipine inhibits Msx2-dependent osteogenic differentiation and matrix mineralization of vascular smooth muscle cells. Int Heart J. (2012) 53:331–5. doi: 10.1536/ihj.53.331
8. Saremi, A, Bahn, G, and Reaven, PD. Progression of vascular calcification is increased with statin use in the veterans affairs diabetes trial (VADT). Diabetes Care. (2012) 35:2390–2. doi: 10.2337/dc12-0464
9. Pasch, A, Schaffner, T, Huynh-Do, U, Frey, BM, Frey, FJ, and Farese, S. Sodium thiosulfate prevents vascular calcifications in uremic rats. Kidney Int. (2008) 74:1444–53. doi: 10.1038/ki.2008.455
10. Chuang, ML, Massaro, JM, Levitzky, YS, Fox, CS, Manders, ES, Hoffmann, U, et al. Prevalence and distribution of abdominal aortic calcium by gender and age group in a community-based cohort (from the Framingham heart study). Am J Cardiol. (2012) 110:891–6. doi: 10.1016/j.amjcard.2012.05.020
11. Rodondi, N, Taylor, BC, Bauer, DC, Lui, LY, Vogt, MT, Fink, HA, et al. Association between aortic calcification and total and cardiovascular mortality in older women. J Intern Med. (2007) 261:238–44. doi: 10.1111/j.1365-2796.2007.01769.x
12. Wilson, PW, Kauppila, LI, O’Donnell, CJ, Kiel, DP, Hannan, M, Polak, JM, et al. Abdominal aortic calcific deposits are an important predictor of vascular morbidity and mortality. Circulation. (2001) 103:1529–34. doi: 10.1161/01.CIR.103.11.1529
13. Kauppila, LI, Polak, JF, Cupples, LA, Hannan, MT, Kiel, DP, and Wilson, PW. New indices to classify location, severity and progression of calcific lesions in the abdominal aorta: a 25-year follow-up study. Atherosclerosis. (1997) 132:245–50. doi: 10.1016/s0021-9150(97)00106-8
14. Lewis, JR, Schousboe, JT, Lim, WH, Wong, G, Wilson, KE, Zhu, K, et al. Long-term atherosclerotic vascular disease risk and prognosis in elderly women with abdominal aortic calcification on lateral spine images captured during bone density testing: a prospective study. J Bone Miner Res. (2018) 33:1001–10. doi: 10.1002/jbmr.3405
15. Cai, Z, Liu, Z, Zhang, Y, Ma, H, Li, R, Guo, S, et al. Associations between Life's essential 8 and abdominal aortic calcification among middle-aged and elderly populations. J Am Heart Assoc. (2023) 12:e031146. doi: 10.1161/jaha.123.031146
16. Inoue, H, Shimizu, S, Watanabe, K, Kamiyama, Y, Shima, H, Nakase, A, et al. Impact of trajectories of abdominal aortic calcification over 2 years on subsequent mortality: a 10-year longitudinal study. Nephrol Dial Transplant. (2018) 33:676–83. doi: 10.1093/ndt/gfx253
17. Bolland, MJ, Wang, TK, van Pelt, NC, Horne, AM, Mason, BH, Ames, RW, et al. Abdominal aortic calcification on vertebral morphometry images predicts incident myocardial infarction. J Bone Miner Res. (2010) 25:505–12. doi: 10.1359/jbmr.091005
18. Lewis, JR, Eggermont, CJ, Schousboe, JT, Lim, WH, Wong, G, Khoo, B, et al. Association between abdominal aortic calcification, bone mineral density, and fracture in older women. J Bone Miner Res. (2019) 34:2052–60. doi: 10.1002/jbmr.3830
19. Ramírez-Vélez, R, García-Hermoso, A, Correa-Rodríguez, M, Lobelo, F, González-Ruiz, K, and Izquierdo, M. Abdominal aortic calcification is associated with decline in handgrip strength in the U.S. adult population ≥40 years of age. Nutr Metab Cardiovasc Dis. (2021) 31:1035–43. doi: 10.1016/j.numecd.2020.11.003
20. Dent, E, Martin, FC, Bergman, H, Woo, J, Romero-Ortuno, R, and Walston, JD. Management of frailty: opportunities, challenges, and future directions. Lancet (London, England). (2019) 394:1376–86. doi: 10.1016/s0140-6736(19)31785-4
21. Collard, RM, Boter, H, Schoevers, RA, and Oude Voshaar, RC. Prevalence of frailty in community-dwelling older persons: a systematic review. J Am Geriatr Soc. (2012) 60:1487–92. doi: 10.1111/j.1532-5415.2012.04054.x
22. Fried, LP, Tangen, CM, Walston, J, Newman, AB, Hirsch, C, Gottdiener, J, et al. Frailty in older adults: evidence for a phenotype. J Gerontol A Biol Sci Med Sci. (2001) 56:M146–57. doi: 10.1093/gerona/56.3.m146
23. Searle, SD, Mitnitski, A, Gahbauer, EA, Gill, TM, and Rockwood, K. A standard procedure for creating a frailty index. BMC Geriatr. (2008) 8:24. doi: 10.1186/1471-2318-8-24
24. Taylor, JA, Greenhaff, PL, Bartlett, DB, Jackson, TA, Duggal, NA, and Lord, JM. Multisystem physiological perspective of human frailty and its modulation by physical activity. Physiol Rev. (2023) 103:1137–91. doi: 10.1152/physrev.00037.2021
25. Shrauner, W, Lord, EM, Nguyen, XT, Song, RJ, Galloway, A, Gagnon, DR, et al. Frailty and cardiovascular mortality in more than 3 million US veterans. Eur Heart J. (2022) 43:818–26. doi: 10.1093/eurheartj/ehab850
26. He, D, Wang, Z, Li, J, Yu, K, He, Y, He, X, et al. Changes in frailty and incident cardiovascular disease in three prospective cohorts. Eur Heart J. (2024) 45:1058–68. doi: 10.1093/eurheartj/ehad885
27. Brivio, P, Paladini, MS, Racagni, G, Riva, MA, Calabrese, F, and Molteni, R. From healthy aging to frailty: in search of the underlying mechanisms. Curr Med Chem. (2019) 26:3685–701. doi: 10.2174/0929867326666190717152739
28. Clegg, A, Young, J, Iliffe, S, Rikkert, MO, and Rockwood, K. Frailty in elderly people. Lancet (London, England). (2013) 381:752–62. doi: 10.1016/s0140-6736(12)62167-9
29. Nadruz, W Jr, Kitzman, D, Windham, BG, Kucharska-Newton, A, Butler, K, Palta, P, et al. Cardiovascular dysfunction and frailty among older adults in the community: the ARIC study. J Gerontol Ser A Biomed Sci Med Sci. (2017) 72:958–64. doi: 10.1093/gerona/glw199
30. Bundy, JD, Mills, KT, He, H, LaVeist, TA, Ferdinand, KC, Chen, J, et al. Social determinants of health and premature death among adults in the USA from 1999 to 2018: a national cohort study. Lancet Public Health. (2023) 8:e422–31. doi: 10.1016/s2468-2667(23)00081-6
31. Schousboe, JT, Lewis, JR, and Kiel, DP. Abdominal aortic calcification on dual-energy X-ray absorptiometry: methods of assessment and clinical significance. Bone. (2017) 104:91–100. doi: 10.1016/j.bone.2017.01.025
32. Hakeem, FF, Bernabé, E, and Sabbah, W. Association between Oral health and frailty among American older adults. J Am Med Dir Assoc. (2021) 22:559–563.e2. doi: 10.1016/j.jamda.2020.07.023
33. Hubbard, RE, Goodwin, VA, Llewellyn, DJ, Warmoth, K, and Lang, IA. Frailty, financial resources and subjective well-being in later life. Arch Gerontol Geriatr. (2014) 58:364–9. doi: 10.1016/j.archger.2013.12.008
34. Kang, MG, Kim, OS, Hoogendijk, EO, and Jung, HW. Trends in frailty prevalence among older adults in Korea: a Nationwide study from 2008 to 2020. J Korean Med Sci. (2023) 38:e157. doi: 10.3346/jkms.2023.38.e157
35. Wang, F, and Zheng, J. Association between serum alpha-klotho and severe abdominal aortic calcification among civilians in the United States. Nutr Metab Cardiovasc Dis. (2022) 32:1485–92. doi: 10.1016/j.numecd.2022.02.017
36. Qin, Z, Chang, K, Liao, R, Jiang, L, Yang, Q, and Su, B. Greater dietary inflammatory potential is associated with higher likelihood of abdominal aortic calcification. Front Cardiovasc Med. (2021) 8:720834. doi: 10.3389/fcvm.2021.720834
37. Liu, F, Liu, F, and Wang, H. Association between Life's essential 8 and rheumatoid arthritis. Clin Rheumatol. (2024) 43:2467–77. doi: 10.1007/s10067-024-07036-w
38. Zhang, J, Chen, Y, Zou, L, and Gong, R. Prognostic nutritional index as a risk factor for diabetic kidney disease and mortality in patients with type 2 diabetes mellitus. Acta Diabetol. (2023) 60:235–45. doi: 10.1007/s00592-022-01985-x
39. Li, Y, Liu, X, Lv, W, Wang, X, Du, Z, Liu, X, et al. Metformin use correlated with lower risk of cardiometabolic diseases and related mortality among US cancer survivors: evidence from a nationally representative cohort study. BMC Med. (2024) 22:269. doi: 10.1186/s12916-024-03484-y
40. Xing, W, Gao, W, Zhao, Z, Xu, X, Bu, H, Su, H, et al. Dietary flavonoids intake contributes to delay biological aging process: analysis from NHANES dataset. J Transl Med. (2023) 21:492. doi: 10.1186/s12967-023-04321-1
41. Liu, Q, Xiang, H, Chen, S, Ouyang, J, Liu, H, Zhang, J, et al. Associations between Life's essential 8 and abdominal aortic calcification among US adults: a cross-sectional study. BMC Public Health. (2024) 24:1090. doi: 10.1186/s12889-024-18622-7
42. Gu, X, Luo, S, Sun, J, Jin, F, Chen, Z, and Song, J. Association between "a body shape index" (ABSI) with periodontitis in a hypertension population from the NHANES 2009-2014. Sci Rep. (2024) 14:23378. doi: 10.1038/s41598-024-73998-1
43. Li, J, Wu, Z, Xin, S, Xu, Y, Wang, F, Liu, Y, et al. Body mass index mediates the association between four dietary indices and phenotypic age acceleration in adults: a cross-sectional study. Food Funct. (2024) 15:7828–36. doi: 10.1039/d4fo01088d
44. Fan, J, Yu, C, Guo, Y, Bian, Z, Sun, Z, Yang, L, et al. Frailty index and all-cause and cause-specific mortality in Chinese adults: a prospective cohort study. Lancet Public Health. (2020) 5:e650–60. doi: 10.1016/S2468-2667(20)30113-4
45. Li, J, Chen, H, He, W, Luo, L, and Guo, X. Frailty index and risk of cardiovascular diseases: a mendelian randomization study. Ann Transl Med. (2022) 10:1007. doi: 10.21037/atm-22-4239
46. Criqui, MH, Denenberg, JO, McClelland, RL, Allison, MA, Ix, JH, Guerci, A, et al. Abdominal aortic calcium, coronary artery calcium, and cardiovascular morbidity and mortality in the multi-ethnic study of atherosclerosis. Arterioscler Thromb Vasc Biol. (2014) 34:1574–9. doi: 10.1161/atvbaha.114.303268
47. El Assar, M, Rodríguez-Sánchez, I, Álvarez-Bustos, A, and Rodríguez-Mañas, L. Biomarkers of frailty. Mol Asp Med. (2024) 97:101271. doi: 10.1016/j.mam.2024.101271
48. Ferrucci, L, and Fabbri, E. Inflammageing: chronic inflammation in ageing, cardiovascular disease, and frailty. Nat Rev Cardiol. (2018) 15:505–22. doi: 10.1038/s41569-018-0064-2
49. Soysal, P, Arik, F, Smith, L, Jackson, SE, and Isik, AT. Inflammation, frailty and cardiovascular disease. Adv Exp Med Biol. (2020) 1216:55–64. doi: 10.1007/978-3-030-33330-0_7
50. Mulholland, M, Jakobsson, G, Lei, Y, Sundius, L, Ljungcrantz, I, Rattik, S, et al. IL-2Rβγ signalling in lymphocytes promotes systemic inflammation and reduces plasma cholesterol in atherosclerotic mice. Atherosclerosis. (2021) 326:1–10. doi: 10.1016/j.atherosclerosis.2021.04.010
51. Kurozumi, A, Nakano, K, Yamagata, K, Okada, Y, Nakayamada, S, and Tanaka, Y. IL-6 and sIL-6R induces STAT3-dependent differentiation of human VSMCs into osteoblast-like cells through JMJD2B-mediated histone demethylation of RUNX2. Bone. (2019) 124:53–61. doi: 10.1016/j.bone.2019.04.006
52. Sánchez-Duffhues, G, García de Vinuesa, A, van de Pol, V, Geerts, ME, de Vries, MR, Janson, SG, et al. Inflammation induces endothelial-to-mesenchymal transition and promotes vascular calcification through downregulation of BMPR2. J Pathol. (2019) 247:333–46. doi: 10.1002/path.5193
53. Xie, R, Liu, X, Wu, H, Liu, M, and Zhang, Y. Associations between systemic immune-inflammation index and abdominal aortic calcification: results of a nationwide survey. Nutr Metab Cardiovasc Dis. (2023) 33:1437–43. doi: 10.1016/j.numecd.2023.04.015
54. Figueiredo, CP, Rajamannan, NM, Lopes, JB, Caparbo, VF, Takayama, L, Kuroishi, ME, et al. Serum phosphate and hip bone mineral density as additional factors for high vascular calcification scores in a community-dwelling: the Sao Paulo Ageing & Health Study (SPAH). Bone. (2013) 52:354–9. doi: 10.1016/j.bone.2012.10.019
55. Thompson, B, and Towler, DA. Arterial calcification and bone physiology: role of the bone–vascular axis. Nat Rev Endocrinol. (2012) 8:529–43. doi: 10.1038/nrendo.2012.36
56. Kmiołek, T, Filipowicz, G, Bogucka, D, Wajda, A, Ejma-Multański, A, Stypińska, B, et al. Aging and the impact of global DNA methylation, telomere shortening, and total oxidative status on sarcopenia and frailty syndrome. Immun Ageing. (2023) 20:61. doi: 10.1186/s12979-023-00384-2
57. Byon, CH, Heath, JM, and Chen, Y. Redox signaling in cardiovascular pathophysiology: a focus on hydrogen peroxide and vascular smooth muscle cells. Redox Biol. (2016) 9:244–53. doi: 10.1016/j.redox.2016.08.015
58. Yamada, S, and Taniguchi, M. Kidney and bone update: the 5-year history and future of CKD-MBD. Disorders of musculoskeletal system in CKD; bone fracture and periarticular calcification. Clin Calcium. (2012) 22:1000–7.
Keywords: subclinical atherosclerosis, vascular calcification, abdominal aortic calcification, frailty index, NHANES
Citation: Zhang Z, Wu P, Yang S, Zhu B, Chen D, Li X, Wang Y and Yan N (2025) The association between frailty index and abdominal aortic calcification in the middle-aged and older US adults: NHANES 2013–2014. Front. Public Health. 13:1546647. doi: 10.3389/fpubh.2025.1546647
Edited by:
Yuquan Chen, Monash University, AustraliaReviewed by:
Xiaofei Hu, Army Medical University, ChinaMohammad Islam, Southern Connecticut State University, United States
Grzegorz K. Jakubiak, Medical University of Silesia, Poland
Copyright © 2025 Zhang, Wu, Yang, Zhu, Chen, Li, Wang and Yan. This is an open-access article distributed under the terms of the Creative Commons Attribution License (CC BY). The use, distribution or reproduction in other forums is permitted, provided the original author(s) and the copyright owner(s) are credited and that the original publication in this journal is cited, in accordance with accepted academic practice. No use, distribution or reproduction is permitted which does not comply with these terms.
*Correspondence: Yarong Wang, bnh5a2RsdXhAMTYzLmNvbQ==; Ning Yan, eWFubmluZzE2OUB5YWhvby5jb20=
†These authors have contributed equally to this work