- 1Center for Black Studies Research, University of California, Santa Barbara, Santa Barbara, CA, United States
- 2College of Creative Studies, University of California, Santa Barbara, Santa Barbara, CA, United States
- 3Department of Health Policy and Management, Jiangsu University School of Management, Zhenjiang, Jiangsu, China
- 4Teesside International Business School, Teesside University, Middlesbrough, United Kingdom
Background: Healthcare disparities remain a significant challenge in addressing equitable healthcare access and outcomes for minority populations, including African Americans. Rooted in systemic racism and historical exclusion, these inequities persist as part of broader structural violence. Leveraging health technology holds promise in addressing these disparities by enhancing access to care, improving its quality, and reducing inequities. However, the association between health technology access, use, socioeconomic status (SES), and healthcare disparities among African Americans remains underexplored. This study aims to explore the potential role of technology in mitigating healthcare disparities by investigating the associations between technology access, healthcare technology use, socioeconomic status (SES), and health disparities among African Americans.
Methods: Using data from the Health Information National Trends Survey (HINTS) Wave 6 dataset, a sample of 815 African Americans was analyzed using Partial Least Squares-Structural Equation Modeling (PLS-SEM).
Findings: The results of the study showed that technology access had a significant positive effect on healthcare technology use (β = 0.260, p < 0.000). Technology access (β = −0.086, p = 0.034) and healthcare technology use (β = −0.180, p < 0.001) demonstrated a significant negative effect on healthcare disparity, respectively. Results also revealed SES had a significant positive effect on technology access (β = 0.424, p < 0.001). Additionally, SES was found to significantly moderate the relationship between technology access and healthcare disparities, indicating variability in the impact of technology access based on SES levels among African Americans.
Conclusion: These findings highlight the potential of technology in mitigating healthcare disparities among African Americans. By promoting enhanced health technology access and utilization, particularly in lower SES populations, the healthcare outcomes for vulnerable communities can be significantly improved. Policymakers, healthcare providers, and technology developers are encouraged to collaborate in providing conducive conditions for the adoption and use of technology to advance healthcare equity.
Introduction
In recent years, advancements in technology have transformed various aspects of society, including the healthcare sector. These advancements have revolutionized healthcare delivery, enabling access to improved personalized healthcare, enhanced patient outcomes, and more efficient care systems (1, 2). However, despite these advancements, significant disparities in healthcare outcomes persist among marginalized communities, particularly African Americans (3–7). The Centers for Disease Control and Prevention (CDC) defines health disparities as preventable differences in the burden of disease, injury, violence, or opportunities to achieve optimal health that are experienced by socially disadvantaged populations (8). Technology inequity, characterized by unequal access to and utilization of healthcare technologies, has emerged as a critical factor contributing to these disparities (9).
Healthcare inequities faced by African Americans are not incidental but rooted in systemic racism and historical patterns of exclusion (3, 5, 10). Saidiya Hartman’s ‘Afterlife of Slavery’ highlights how skewed life chances, by extension technological exclusion, and healthcare disparities are enduring legacies of slavery and racial capitalism (11). Similarly, Ruth Wilson Gilmore’s framing of racism as ‘the production of premature death’ (12) underscores how systemic barriers, such as limited access to telehealth, electronic health records, and wearable technologies, disproportionately deny Black populations equitable opportunities for healthcare (13). While efforts have been made to address these disparities, the rapid pace of technological advancements has introduced a new dimension to the problem (14, 15). Technology inequity exacerbates healthcare disparities among African Americans, as they often encounter limited access to essential technologies, such as electronic health records (EHRs), telehealth services, mobile health applications, and wearable devices, and also experience delayed access to needed care, and discrimination (16, 17).
To comprehend the multifaceted impact of technology on healthcare disparities among African Americans, it is crucial to explore the underlying factors that perpetuate this divide. Socioeconomic factors, including income disparities, education, occupation, and lack of health insurance coverage, play a significant role in limiting access to and utilization of healthcare technologies (14). Low socioeconomic status (SES) individuals, including many African Americans, often face barriers to technology access due to limited financial resources, lower digital literacy levels, and inadequate technology infrastructure in their communities. Additionally, continued systemic discrimination (18, 19), delayed access to needed care (20, 21), and health risk factors (18) may contribute to the divide, further impeding African Americans’ ability to leverage technology for improved health outcomes.
Several studies have demonstrated the detrimental effects of technology inequity on healthcare disparities (22) among African Americans (23–26). Research by Mulia et al. indicated that Hispanic/Latinx and African American patients had reduced access to telehealth services compared to their white counterparts, resulting in delayed or inadequate healthcare access (27). Furthermore, Heiney et al. found that African Americans faced barriers to utilizing mobile health applications, limiting their ability to engage in self-management and preventive care practices (28).
This study aims to comprehensively explore the complex relationship between technology inequity and healthcare disparities among African Americans. By analyzing data from the Health Information National Trends Survey (HINTS) Wave 6 dataset, we seek to identify the underlying mechanisms through which technology inequity perpetuates healthcare disparities. Furthermore, we discuss potential strategies and interventions to address these disparities and promote equitable access to healthcare technologies for African Americans.
Methodology
Study population and design
Using data from the National Cancer Institute (NCI)’s Health Information National Trends Survey (HINTS) Wave 6 dataset, this study analyzed responses from 815 African Americans. HINTS 6 is a cross-sectional survey of non-institutionalized civilian adults aged 18+ years in the United States collected from March 7, 2022, to November 8th, 2022 with the goal of investigating the need for, access to, and use of health-related information and health-related behaviors, perceptions and knowledge (29, 30).
HINTS 6 included an embedded methodological experiment comparing two mixed-mode approaches: concurrent and sequential; also referred to as the control and treatment groups, respectively. Households in the control group received a cover letter with the link to the web survey and their unique access code as well as a paper survey with each mailing (including their first mailing). Households in the treatment group received only a cover letter with the link to the web survey and their unique access code with their first mailing, they did not receive a paper survey in their first mailing. In subsequent mailings, these households received the link to the web survey and their unique access code as well as the paper survey. Both conditions used the same sampling frame provided by Marketing Systems Group (MSG) of addresses in the United States. All addresses were grouped into one of four strata; high and low minority, and by rural and urban area using the benchmark for assessing minority populations in census data. This approach enhances the representativeness of minority populations, including African Americans, ensuring sufficient representation of diverse demographic segments and thus facilitating accurate generalization to the African American population in the U.S.
The mailing protocol for HINTS 6 followed a modified Dillman approach (31) with all selected households receiving a total of four mailings: an initial mailing, a reminder postcard, and two follow-up mailings.
Measures
Health disparity (H_DISPARITY)
The outcome variable for this study was health disparity. To assess health disparity, the experiences of discrimination while seeking medical care and delays in accessing necessary care were used. To evaluate delay in accessing needed healthcare, a 3-item scale of “Yes,” “No, I received the medical care I felt I needed” and “I did not need any medical care in the past 12 months,” was used to elicit response to the question “In the past 12 months, did you delay or not get medical care you felt you needed - such as seeing a doctor, a specialist, or other health professional?.” Discrimination was evaluated on a yes/no scale on a question “Have you ever been treated unfairly or been discriminated against when getting medical care because of your race or ethnicity?”
Independent variables
Socioeconomic status (SES)
The relationship between socioeconomic status (SES), technology access, and healthcare disparities is complex and multifaceted. SES, which encompasses factors such as income, education, and occupation, plays a significant role in determining an individual’s access to technology and subsequent healthcare disparities. Many studies have established a strong relationship between socioeconomic factors and how they influence an individual’s access to technology (32, 33). Higher-income individuals are more likely to afford smartphones, computers, and internet access, which are essential for utilizing healthcare technology such as telehealth services or health apps (34). On the other hand, lower-income individuals may face barriers due to the cost of devices, internet access, or limited availability of technology infrastructure in their communities (35). Consequently, disparities in technology access may contribute to disparities in healthcare outcomes. Furthermore, the digital divide, driven by socioeconomic factors, exacerbates healthcare disparities. For instance, lower-income individuals, including African Americans who are more likely to experience economic challenges, may lack the resources or skills to effectively use health technology. This limits their ability to access online health information, engage in telehealth visits, or effectively manage their healthcare. As a result, they may experience delays in accessing care, receive suboptimal healthcare services, or have poorer health outcomes compared to individuals with higher SES (14, 36). SES is closely linked to health literacy, which refers to an individual’s ability to access, understand, and use health information to make informed decisions about their health (37, 38). Individuals with lower SES are more likely to have lower health literacy levels, which can hinder their utilization of healthcare technology. Limited health literacy skills may make it difficult for individuals to navigate complex digital platforms, understand health-related information, or effectively communicate with healthcare providers through technology (37, 38). Another factor that could be influenced by SES is provider-patient communication. Technology-mediated interactions, such as telehealth visits or patient portals, may impact provider-patient communication differently based on SES. Individuals with lower SES may face challenges in effectively communicating their health concerns, understanding medical jargon, or asking necessary questions through these platforms. These communication barriers can impede the delivery of patient-centered care and contribute to disparities in healthcare quality and outcomes (39). Education and technological literacy have the potential to influence the level of SES of an individual (33). Education, another component of SES, influences technological literacy and digital skills. Higher levels of education are associated with better technology proficiency (33, 40, 41), including the ability to navigate digital platforms, access online health resources, and use health technology effectively. Individuals with lower education levels may experience difficulties in adopting and benefiting from healthcare technology, leading to disparities in healthcare access, utilization, and health outcomes (42, 43). Thus, it is imperative to understand how SES plays into technology access and use and how they consequently impact healthcare disparity among African Americans. Respondents’ education level and household income were used to evaluate socioeconomic status. For education, respondents were asked about their highest level of education. The highest level of education was categorized as “Less than high school,” “high school graduate,” “some college,” “bachelor’s degree,” and “post-baccalaureate degree.” Similarly, respondents’ annual household income was assessed with 1 representing “$0 to $9,999”, 2 “$10,000 to $14,999”, 3 “$15,000 to $19,999”, 4 “$20,000 to $34,999”, 5 “$35,000 to $49,999”, 6 “$50,000 to $74,999”, 7 “$75,000 to $99,999”, 8 “$100,000 to $199,999” and 9 as “$200,000 or more”.
Based on the above, we posit the following hypothesis for SES:
H1: SES has a significant negative effect on health disparity; H2: SES has a significant positive effect on technology access, and H6: SES moderates the relationship between technology access and health disparity, such that the higher an individual’s SES level, the lesser their experience of healthcare disparity.
Technology access (TECH_ACC)
Technology and its associated advancements have long been trumpeted to influence the kind of healthcare individuals receive and the quality of such care (44). Nonetheless, through what has been known as the “Digital Divide,” technology might either assist in making things better or perhaps worse. For some populations, having access to digital information might help with self-care and maintaining good health (32). However, the racial and ethnic groups that experience the largest injustices, particularly Blacks and Hispanics, have significantly varying access to digital resources depending on socioeconomic level. The future will depend on improving digital health equality. Broadband access represents one specific major concern. Over 21 million Americans lack broadband access (45). While cities like New York have broadband infrastructure covering 99.9% of the population, 2.2 million adults there do not have a home broadband subscription (46). In more rural areas, such as the mountains of Appalachia in states such as Tennessee, Kentucky, and West Virginia, there are large areas with no or limited broadband access (46). According to the Public Policy Institute of California (PPIC), though broadband has grown slightly from 84% in 2019 to 85% in 2020, racial and ethnic disparities in access persist with 81% of Latino, 83% of Black, 87% of white, and 88% of Asian households report having broadband access at home in 2021 (33). Thus, disparities in broadband infrastructure, driven by uneven geographical deployment, economic affordability issues, and historical digital redlining, constitute critical barriers that disproportionately affect marginalized communities, including African Americans. Aside from broadband access, telehealth access is critically essential in promoting equitable healthcare. The COVID-19 pandemic has facilitated and increased the importance and use of telehealth (46). Because of the risk of person-to-person viral transmission, organizations around the country switched most outpatient care to telehealth essentially overnight (46). Black patients and poorer patients were much more likely to receive telephonic as opposed to video visits (46). The following questions were posed to respondents to measure technology access to access to basic cell phones, smartphones, and access and use of the internet rated on a “Yes”/“No” scale; (i) “Have a basic cell phone?,” (ii) “Have a smartphone?” and (iii) “Do you ever go online to access the Internet or World Wide Web, or to send and receive email?.” Based on the above, this study posits that: H3: Technology access has a significant negative effect on healthcare disparities, H4: Technology access has a significant positive effect on health technology, and H7: There is a significant negative indirect effect of SES on H_DISPARITY through the sequential mediation of TECH_ACC.
Health technology use (hTECH_USE)
Health technology has the potential to significantly impact healthcare outcomes for African Americans, as it may help improve access to care, enhance patient engagement, and promote health equity. For instance, during the COVID-19 pandemic the use of telehealth became more prevalent, helping individuals manage their chronic diseases by enhancing patient-provider communication, promoting medication adherence, and enabling self-monitoring of health conditions (47, 48). However, it is crucial to acknowledge that disparities in health technology use may also contribute to existing healthcare disparities (49), particularly issues of access due to structural and systemic racism. Thus, to measure the health technology use among African Americans, this study evaluated the frequency of watching a health-related video on a social media site like YouTube in the last 12 months. The responses were assessed on a 5-likert scale with 1 as “Almost every day,” 3 as “A few times a month” and 5 as “Never.” Respondents were further asked how often they interacted with people who have similar health or medical issues as them on social media or online forums and how often they shared general health-related information on social media for example, a news article in the last 12 months, respectively. Finally, respondents were asked to indicate whether they had received care from a doctor or health professional using telehealth in the past 12 months using a scale of 1–4, with 1 indicating Yes, by video, 2 as “Yes, by phone call (voice only with no video),” 3 as “Yes, some by video and some by phone call” and 4 as “No telehealth visits in the past 12 months.”
These questions were posed to help understand how health technology use affects healthcare disparities, hence, the study posits that: H5: Health technology use has a significant negative effect on healthcare disparities.
H8: There is a significant negative indirect effect of TECH_ACC on H_DISPARITY through the sequential mediation of hTECH_USE.
Statistical analysis
Due to its robustness of estimations and statistical power (50, 51), the partial least squares (PLS) based on structural equation modeling (SEM) was employed to test and validate the hypothesized model with the aid of SmartPLS 4.0 (52). The model’s validity was determined by analyzing the measurement and structural models.
The measurement model was evaluated using internal consistency reliability, convergent validity, and discriminant validity. This was done using the outer loadings (≥0.70 for reliability), Average of Variance Extracted (AVE; ≥0.50 indicating convergent validity), Composite Reliability (CR; ≥0.70 indicating internal consistency), Fornell and Larcker criterion (square root of AVE exceeding inter-construct correlations indicating discriminant validity), and Heterotrait-Monotrait Ratio of correlation (HTMT; <0.85 demonstrating discriminant validity) (53–55).
The collinearity assessment (VIF) (53, 54), path coefficient (β; showing relationship strength and direction), t-statistics (statistical significance) (53), and the model fit index were used to evaluate the structural model for the main model, mediation, and moderation analysis. The moderating effect was further evaluated using the simple slope analysis. Model fit analysis was performed using the Standardized Root Mean Square Residual (SRMR ≤0.10 acceptable), discrepancy function (d_ULS and d_G), chi-square statistic, and the Normed Fit Index (NFI) (56, 57).
Results
Table 1 shows the summary statistics for the respondents’ demographics. The majority of respondents were females, and the most occurring age was 50–64 years old. Most respondents had some college education, with the majority having only one employment. Lastly, the most observed household income range was between $20,000 and $34,999.
The results for the outer loadings, internal consistency reliability, convergent validity, and discriminant validity are presented in Table 2 above. The outer loadings for all constructs showed values greater than the acceptable threshold of 0.70, except hTU2, which had an outer loading value of 0.694. Also, composite reliability values for all the constructs were greater than 0.70, suggesting strong internal consistency reliability (38). AVE values were all above the recommended level of 0.50 (55), as shown in Table 2. Figure 1 shows the various constructs with their respective loadings. Results of HTMT showed all the constructs had HTMT values less than the threshold of 1. Additionally, the square root of the AVE of all the constructs was greater than their correlation with other constructs, and the diagonal items were larger than the entries in corresponding columns and rows, hence satisfying the Fornell and Larcker criterion (55).
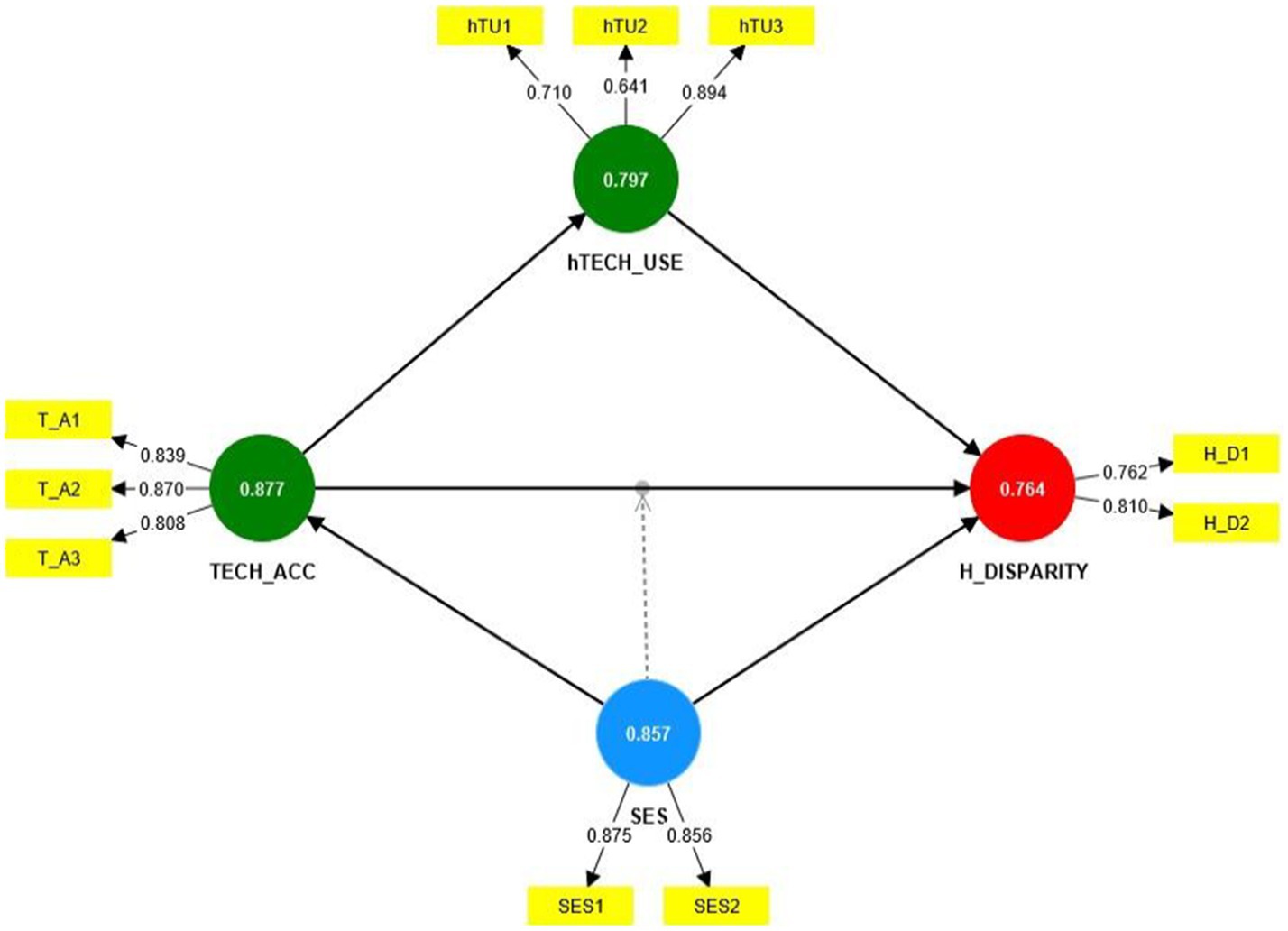
Figure 1. Path diagram showing loadings and composite reliability of the various latent and observed variables.
Results of the structural model as shown in Table 3 revealed a statistically significant relationship between SES → TECH_ACC (β = 0.424, t-statistics = 16.444, p < 0.001), TECH_ACC → H_DISPARITY (β = −0.086, t-statistics = 1.828, p-value = 0.034), TECH_ACC → hTECH_USE (β = 0.260, t-statistics = 11.363, p < 0.001), and hTECH_USE → H_DISPARITY (β = −0.180, t-statistics = 4.458, p < 0.001), thus, supporting hypothesis H2, H3, H4, and H5, respectively. However, SES → H_DISPARITY (β = −0.021, t-statistics = 0.503, p-value = 0.307) was not significant, therefore not supporting H1. Mediation results shows a partial mediation SES → TECH_ACC → H_DISPARITY (β = −0.036, t-statistics = 1.794, p-value = 0.036) and TECH_ACC → hTECH_USE → H_DISPARITY (β = −0.047, t-statistics = 4.332, p < 0.001), hence satisfying H7 and H8, respectively. The path diagram for the bootstrapped results is shown in Figure 2 below.
The moderating effect of SES x TECH_ACC → H_DISPARITY (β = 0.097, t-statistics = 2.674, p-value = 0.004) produced a statistically significant result, thus, satisfying H6. The simple slope analysis of the moderating effect is shown in Figure 3.
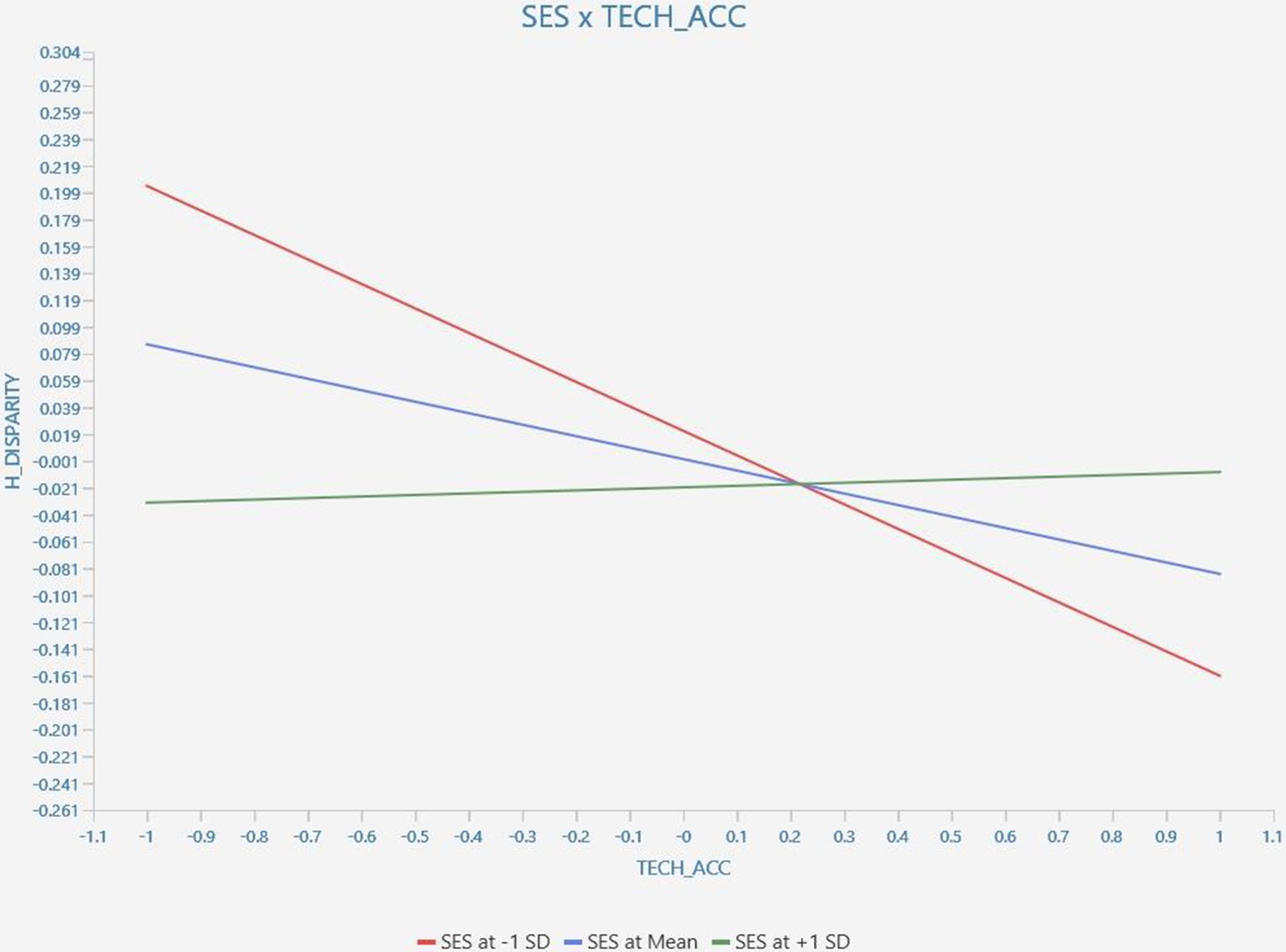
Figure 3. Result of the simple slope analysis of the moderating effect of SES on TECH_ACC → H_DISPARITY.
Finally, the summary of the fit indexes showed SRMR of 0.096, Unweighted Least Squares discrepancy (d_ULS) and Bentler’s Comparative Fit Index (d_G) values of 0.504 and 0.201, respectively, and NFI of 0.424, suggesting model fit.
Discussion
This study aimed to investigate the relationships between socioeconomic status (SES), technology access, health technology use, and healthcare disparities. The findings revealed a positive association between SES and technology access, suggesting that individuals with higher socioeconomic status are more likely to have more access to technology. This aligns with previous research indicating that socioeconomic factors play a crucial role in determining technology access (32, 33, 58). The statistically significant relationship between SES and technology access highlights the role of structural racism in shaping economic opportunities and digital inclusion for African Americans. These disparities are not simply socioeconomic but are rooted in what Ruth Wilson Gilmore terms ‘group-differentiated vulnerability to premature death.’ (12) Communities with limited access to health technology face compounded disadvantages, reflecting patterns of exclusion embedded in racialized spatial dynamics.
Consistent with previous studies (14, 59, 60), this study found a negative association between technology access and healthcare disparities. Individuals with better technology access were found to experience reduced healthcare disparities. This finding underscores the potential of technology in bridging healthcare gaps and improving access to care, especially for underserved populations, if, and only if, systemic barriers to access and utilization are dismantled. Telehealth specifically plays a crucial role in addressing healthcare disparities by providing access to medical care for individuals in underserved communities. It enables remote consultations, reduces wait times, and facilitates continuity of care, particularly for managing chronic diseases. Mobile health applications and electronic health records further support patient engagement by providing real-time health monitoring and improved access to medical information (47, 61). Thus, by providing remote access to healthcare services, technology can overcome geographical barriers and ensure timely delivery of care to individuals who may face challenges in accessing traditional healthcare facilities.
The study also found a positive association between technology access and health technology use, which is consistent with previous research (44, 62–64), suggesting that individuals with better technology access are more likely to engage with health technology tools. This finding emphasizes the importance of ensuring equitable technology access to facilitate health technology adoption and engagement among diverse populations. By leveraging health technology, individuals can actively manage their health, access educational resources, and engage in shared decision-making with healthcare providers, potentially leading to improved health outcomes (65).
Furthermore, the negative association between health technology use and healthcare disparities supports the notion that health technology can help reduce disparities in healthcare outcomes. Individuals who actively utilize health technology may benefit from improved health management, increased access to information, and better communication with healthcare providers (66, 67). These factors contribute to more equitable healthcare experiences and outcomes.
The interaction effect between SES and technology access on healthcare disparities highlights the importance of considering the interplay between socioeconomic factors and technology access. This finding suggests that addressing both SES disparities and technology access is crucial in reducing healthcare disparities. Government initiatives such as telehealth subsidies, broadband expansion programs, and Medicaid coverage for virtual healthcare services have the potential to improve access to telehealth for low-income populations (68). These policies have the potential to reduce financial and technological barriers that limit healthcare access for marginalized groups (69). Thus, strategies should focus not only on enhancing technology access but also on addressing underlying socioeconomic inequalities to achieve equitable healthcare outcomes for all individuals.
Regarding the mediation analysis, this study found that the joint mediation of technology access and health technology use in the association between SES and healthcare disparities was statistically significant, suggesting that health technology use plays a crucial role in reducing healthcare disparities among individuals with different socioeconomic backgrounds (70, 71).
As better technology access facilitates greater engagement with health technology, it is imperative that digital literacy programs are introduced to help individuals navigate telehealth platforms, use mobile health applications, use social media to seek accurate health information and access electronic health records. Community-based initiatives and partnerships between healthcare providers and technology organizations should be developed to enhance digital skills, particularly among older adults and low-income populations, ensuring equitable utilization of health technology, and consequently leading to improved healthcare outcomes.
Furthermore, our results suggest that health technology use partially mediates the relationship between technology access and healthcare disparities. Health technology use, such as health apps or remote monitoring devices, helps bridge gaps in healthcare access and improve patient engagement. By promoting health technology use, healthcare providers can enhance patient-provider communication, empower patients to take an active role in their care, and ultimately reduce disparities in healthcare outcomes.
Interestingly, while we observed a significant indirect effect of SES on health technology use through technology access, the mediation of health technology use in the relationship between SES and healthcare disparities was not statistically significant. This suggests that the effect of SES on healthcare disparities is not solely mediated by health technology use in the studied population. Other factors, such as access to healthcare facilities, provider-patient communication, and cultural competence in healthcare, may also contribute to healthcare disparities among individuals with varying SES levels.
Conclusion
The results of this study suggest that socioeconomic status (SES), technology access, and healthcare technology use are all important factors that can influence healthcare disparity. Additionally, the results suggest that the effect of technology access on healthcare disparity is different for people with different SES levels. These findings have important implications for the design of policies and programs aimed at reducing healthcare disparities. Policies aimed at reducing healthcare disparities must prioritize racial justice, addressing digital redlining, technological exclusion, and the economic marginalization of Black communities. By leveraging health technology effectively, healthcare systems can work towards reducing disparities, enhancing patient engagement, and improving health outcomes for all populations. Future research and policy efforts should continue to explore innovative ways to maximize the potential of technology in promoting health equity and reducing healthcare disparities.
Limitations
Despite the findings discussed above, there are a number of limitations to this study that should be considered in interpreting the results. This current study was conducted in the United States of America, and the results may not be generalizable to other countries. Additionally, the study relied on self-reported data, which may introduce response biases and rely on participants’ subjective perceptions. Future studies could employ objective measures and longitudinal designs to strengthen the validity of the findings. Furthermore, the study was cross-sectional, hence, the effect of these constructs on healthcare disparity in the long term is contestable. It is imperative to understand the effect of these constructs in the long term over time through a longitudinal study. Lastly, the technology access measures from HINTS Wave 6 captured only basic device ownership and general internet use, without details on internet quality or broadband access. Future research should consider more detailed measures of internet infrastructure.
Data availability statement
Publicly available datasets were analyzed in this study. This data can be found at: https://hints.cancer.gov/data/download-data.aspx#H6.
Ethics statement
Ethical approval was not required for the study involving humans in accordance with the local legislation and institutional requirements. Written informed consent to participate in this study was not required from the participants or the participants’ legal guardians/next of kin in accordance with the national legislation and the institutional requirements.
Author contributions
EL: Conceptualization, Formal analysis, Methodology, Validation, Writing – original draft, Writing – review & editing. ST: Writing – original draft, Writing – review & editing. JN: Data curation, Formal analysis, Writing – original draft, Writing – review & editing. SA-D: Writing – original draft, Writing – review & editing. FA: Writing – original draft, Writing – review & editing.
Funding
The author(s) declare that no financial support was received for the research and/or publication of this article.
Acknowledgments
Thanks to Lyla Washaha for her administrative roles performed in getting this manuscript ready for publication.
Conflict of interest
The authors declare that the research was conducted in the absence of any commercial or financial relationships that could be construed as a potential conflict of interest.
Generative AI statement
The authors declare that no Gen AI was used in the creation of this manuscript.
Publisher’s note
All claims expressed in this article are solely those of the authors and do not necessarily represent those of their affiliated organizations, or those of the publisher, the editors and the reviewers. Any product that may be evaluated in this article, or claim that may be made by its manufacturer, is not guaranteed or endorsed by the publisher.
References
1. Brewer, LC, Fortuna, KL, Jones, C, Walker, R, Hayes, SN, Patten, CA, et al. Back to the future: achieving health equity through health informatics and digital health. JMIR Mhealth Uhealth. (2020) 8:e14512. doi: 10.2196/14512
2. Koonin, LM, Hoots, B, Tsang, CA, Leroy, Z, Farris, K, Jolly, B, et al. Trends in the use of telehealth during the emergence of the COVID-19 pandemic - United States, January-March 2020. MMWR Morb Mortal Wkly Rep. (2020) 69:1595–9. doi: 10.15585/mmwr.mm6943a3
3. Massey, DS, and Denton, NA. American apartheid: segregation and the making of the underclass In: Social stratification, class, race, and gender in sociological perspective. 2nd ed. Milton Park, Abingdon, Oxfordshire, United Kingdom: Routledge (2019). 660–70.
4. Bonilla-Silva, E. Rethinking racism: toward a structural interpretation. Am Sociol Rev. (1997) 62:465–80. doi: 10.2307/2657316
5. Braveman, PA, Arkin, E, Proctor, D, Kauh, T, and Holm, N. Systemic and structural racism: definitions, examples, health damages, and approaches to dismantling. Health Aff. (2022) 41:171–8. doi: 10.1377/hlthaff.2021.01394
6. Williams, DR, and Sternthal, M. Understanding racial-ethnic disparities in health: sociological contributions. J Health Soc Behav. (2010) 51:S15–27. doi: 10.1177/0022146510383838
7. Pollack, CE, Cubbin, C, Sania, A, Hayward, M, Vallone, D, Flaherty, B, et al. Do wealth disparities contribute to health disparities within racial/ethnic groups? J Epidemiol Community Health. (2013) 67:439–45. doi: 10.1136/jech-2012-200999
8. Centers for Disease Control and Prevention. Community health and program services (CHAPS): health disparities among racial/ethnic populations. Clifton Road, Atlanta, GA, USA: US Department of Health and Human Services (2008).
9. Huh, J, Koola, J, Contreras, A, Castillo, A, Ruiz, M, Tedone, K, et al. Consumer health informatics adoption among underserved populations: thinking beyond the digital divide. Yearb Med Inform. (2018) 27:146–55. doi: 10.1055/s-0038-1641217
10. Jones, CP. Levels of racism: a theoretic framework and a gardener's tale. Am J Public Health. (2000) 90:1212–5. doi: 10.2105/ajph.90.8.1212
11. Hartman, S. Lose your mother: a journey along the Atlantic slave route. New York, NY, USA: Macmillan (2008).
13. Connell, CL, Wang, SC, Crook, LS, and Yadrick, K. Barriers to healthcare seeking and provision among African American adults in the rural Mississippi Delta region: community and provider perspectives. J Community Health. (2019) 44:636–45. doi: 10.1007/s10900-019-00620-1
14. Saeed, SA, and Masters, RM. Disparities in health care and the digital divide. Curr Psychiatry Rep. (2021) 23:61. doi: 10.1007/s11920-021-01274-4
15. Badr, J, Motulsky, A, and Denis, J-L. Digital health technologies and inequalities: a scoping review of potential impacts and policy recommendations. Health Policy. (2024) 146:105122. doi: 10.1016/j.healthpol.2024.105122
16. Ferryman, K. Framing inequity in health technology: the digital divide, data bias, and racialization. Brooklyn, NY, USA: Just Tech Social Science Research Council (2022).
17. Lorence, DP, Park, H, and Fox, S. Racial disparities in health information access: resilience of the digital divide. J Med Syst. (2006) 30:241–9. doi: 10.1007/s10916-005-9003-y
18. Simons, RL, Lei, MK, Klopack, E, Beach, SRH, Gibbons, FX, and Philibert, RA. The effects of social adversity, discrimination, and health risk behaviors on the accelerated aging of African Americans: further support for the weathering hypothesis. Soc Sci Med. (2021) 282:113169. doi: 10.1016/j.socscimed.2020.113169
19. National Academies of Sciences, E. and Medicine. Communities in action: pathways to health equity. Washington (DC): National Academies Press (US) (2017).
20. Adinkrah, EK, Cobb, S, and Bazargan, M. Delayed medical Care of Underserved Middle-Aged and Older African Americans with chronic disease during COVID-19 pandemic. Healthcare. (2023) 11:595. doi: 10.3390/healthcare11040595
21. Krawczyk, CS, Funkhouser, E, Kilby, JM, and Vermund, SH. Delayed access to HIV diagnosis and care: special concerns for the southern United States. AIDS Care. (2006) 18:35–44. doi: 10.1080/09540120600839280
22. Lee, J. The impact of health information technology on disparity of process of care. Int J Equity Health. (2015) 14:34. doi: 10.1186/s12939-015-0161-3
23. Ward-Sutton, C, Williams, NF, Moore, CL, and Manyibe, EO. Assistive technology access and usage barriers among African Americans with disabilities: a review of the literature and policy. J Appl Rehabil Couns. (2020) 51:115–33. doi: 10.1891/JARC-D-19-00011
24. Mavrou, K, Meletiou-Mavrotheris, M, Kärki, A, Sallinen, M, and Hoogerwerf, EJ. Opportunities and challenges related to ICT and ICT-AT use by people with disabilities: an explorative study into factors that impact on the digital divide. Technol Disabil. (2017) 29:63–75. doi: 10.3233/TAD-170174
25. Subramony, D. Reframing the digital divide within a ‘flat world’context: the McJulien lecture. Jacksonville, FL: Association for Educational Communications and Technology (AECT) International Convention (2014).
26. Subramony, DP. Understanding the complex dimensions of the digital divide: lessons learned in the Alaskan Arctic. J Negro Educ. (2007) 76:57–67.
27. Mulia, N, Ye, Y, Greenfield, TK, Martinez, P, Patterson, D, Kerr, WC, et al. Inequitable access to general and behavioral healthcare in the US during the COVID-19 pandemic: a role for telehealth? Prev Med. (2023) 169:107426. doi: 10.1016/j.ypmed.2023.107426
28. Heiney, SP, Donevant, SB, Arp Adams, S, Parker, PD, Chen, H, and Levkoff, S. A smartphone app for self-management of heart failure in older African Americans: feasibility and usability study. JMIR Aging. (2020) 3:e17142. doi: 10.2196/17142
29. Hesse, BW, Moser, RP, Rutten, LJF, and Kreps, GL. The health information national trends survey: research from the baseline. J Health Commun. (2006) 11:vii–xvi. doi: 10.1080/10810730600692553
30. Nelson, D, Kreps, G, Hesse, B, Croyle, R, Willis, G, Arora, N, et al. The health information national trends survey (HINTS): development, design, and dissemination. J Health Commun. (2004) 9:443–60. doi: 10.1080/10810730490504233
31. Dillman, DA, Smyth, JD, and Christian, LM. Internet, phone, mail, and mixed-mode surveys: the tailored design method. New York: John Wiley & Sons (2014).
32. Paccoud, I, Baumann, M, le Bihan, E, Pétré, B, Breinbauer, M, Böhme, P, et al. Socioeconomic and behavioural factors associated with access to and use of personal health records. BMC Med Inform Decis Mak. (2021) 21:18. doi: 10.1186/s12911-020-01383-9
33. Scherer, R, and Siddiq, F. The relation between students’ socioeconomic status and ICT literacy: findings from a meta-analysis. Comput Educ. (2019) 138:13–32. doi: 10.1016/j.compedu.2019.04.011
34. Dixit, RR. Factors influencing Healthtech literacy: an empirical analysis of socioeconomic, demographic, technological, and health-related variables. Appl Res Artif Intell Cloud Comput. (2018) 1:23–37.
35. Reddick, CG, Enriquez, R, Harris, RJ, and Sharma, B. Determinants of broadband access and affordability: an analysis of a community survey on the digital divide. Cities. (2020) 106:102904. doi: 10.1016/j.cities.2020.102904
36. Gergen Barnett, K, Mishuris, RG, Williams, CT, Bragg, A, Semenya, AM, Baldwin, M, et al. Telehealth's double-edged sword: bridging or perpetuating health inequities? J Gen Intern Med. (2022) 37:2845–8. doi: 10.1007/s11606-022-07481-w
37. de Buhr, E, and Tannen, A. Parental health literacy and health knowledge, behaviours and outcomes in children: a cross-sectional survey. BMC Public Health. (2020) 20:1096. doi: 10.1186/s12889-020-08881-5
38. Jansen, T, Rademakers, J, Waverijn, G, Verheij, R, Osborne, R, and Heijmans, M. The role of health literacy in explaining the association between educational attainment and the use of out-of-hours primary care services in chronically ill people: a survey study. BMC Health Serv Res. (2018) 18:394. doi: 10.1186/s12913-018-3197-4
39. Seljelid, B, Varsi, C, Solberg Nes, L, Øystese, KA, and Børøsund, E. Feasibility of a digital patient-provider communication intervention to support shared decision-making in chronic health care, InvolveMe: pilot study. JMIR Form Res. (2022) 6:e34738. doi: 10.2196/34738
40. Spooner, KK, Salemi, JL, Salihu, HM, and Zoorob, RJ. eHealth patient-provider communication in the United States: interest, inequalities, and predictors. J Am Med Inform Assoc. (2016) 24:e18–27. doi: 10.1093/jamia/ocw087
41. Lee, HY, Jin, SW, Henning-Smith, C, Lee, J, and Lee, J. Role of health literacy in health-related information-seeking behavior online: cross-sectional study. J Med Internet Res. (2021) 23:e14088. doi: 10.2196/14088
42. Busse, TS, Nitsche, J, Kernebeck, S, Jux, C, Weitz, J, Ehlers, JP, et al. Approaches to improvement of digital health literacy (eHL) in the context of person-centered care. Int J Environ Res Public Health. (2022) 19:8309. doi: 10.3390/ijerph19148309
43. Lee, J, and Tak, SH. Factors associated with eHealth literacy focusing on digital literacy components: a cross-sectional study of middle-aged adults in South Korea. Digit Health. (2022) 8:20552076221102765. doi: 10.1177/20552076221102765
44. Borges do Nascimento, IJ, Abdulazeem, HM, Vasanthan, LT, Martinez, EZ, Zucoloto, ML, Østengaard, L, et al. The global effect of digital health technologies on health workers’ competencies and health workplace: an umbrella review of systematic reviews and lexical-based and sentence-based meta-analysis. Lancet Digit Health. (2023) 5:e534–44. doi: 10.1016/S2589-7500(23)00092-4
45. Eruchalu, CN, Pichardo, MS, Bharadwaj, M, Rodriguez, CB, Rodriguez, JA, Bergmark, RW, et al. The expanding digital divide: digital health access inequities during the COVID-19 pandemic in New York City. J Urban Health. (2021) 98:183–6. doi: 10.1007/s11524-020-00508-9
46. Bates, D. Health inequities and technology. J Health Care Poor Underserved. (2021) 32:viii–xii. doi: 10.1353/hpu.2021.0044
47. Lee, M, and Nam, S. Telehealth utilization among patients with chronic disease: insights from the 2022 health information national trends survey. J Telemed Telecare. (2024) 1:1357633X241289158. doi: 10.1177/1357633x241289158
48. Oluyede, L, Cochran, AL, Wolfe, M, Prunkl, L, and McDonald, N. Addressing transportation barriers to health care during the COVID-19 pandemic: perspectives of care coordinators. Transp Res A Policy Pract. (2022) 159:157–68. doi: 10.1016/j.tra.2022.03.010
49. Park, J-H, Lee, MJ, Tsai, MH, Shih, HJ, and Chang, J. Rural, regional, racial disparities in telemedicine use during the COVID-19 pandemic among US adults: 2021 national health interview survey (NHIS). Patient Prefer Adherence. (2023) 17:3477–87. doi: 10.2147/PPA.S439437
50. Larnyo, E, Dai, B, Larnyo, A, Nutakor, JA, Ampon-Wireko, S, Nkrumah, ENK, et al. Impact of actual use behavior of healthcare wearable devices on quality of life: a cross-sectional survey of people with dementia and their caregivers in Ghana. Healthcare. (2022) 10:275. doi: 10.3390/healthcare10020275
51. Reinartz, W, Haenlein, M, and Henseler, J. An empirical comparison of the efficacy of covariance-based and variance-based SEM. Int J Res Mark. (2009) 26:332–44. doi: 10.1016/j.ijresmar.2009.08.001
52. Ringle, CM, Wende, S, and Becker, J-M. SmartPLS 4. Oststeinbek: SmartPLS (2022). Available at: https://www.smartpls.com/
53. Hair, JF, Hult, GTM, Ringle, CM, Sarstedt, M, Danks, NP, Ray, S, et al. Evaluation of formative measurement models In: JF Hair Jr, GTM Hult, CM Ringle, M Sarstedt, NP Danks, and S Ray, editors. Partial least squares structural equation modeling (PLS-SEM) using R: A workbook. Cham: Springer International Publishing (2021). 91–113.
54. Hair, JF, Sarstedt, M, and Ringle, CM. Rethinking some of the rethinking of partial least squares. Eur J Mark. (2019) 53:566–84. doi: 10.1108/EJM-10-2018-0665
55. Hair, JF, Risher, JJ, Sarstedt, M, and Ringle, CM. When to use and how to report the results of PLS-SEM. Eur Bus Rev. (2019) 31:2–24. doi: 10.1108/EBR-11-2018-0203
56. Kline, R.B., Principles and practice of structural equation modeling (3rd ed). New York, NY: Guilford, (2011). 14: p. 1497–1513.
57. Hu, LT, and Bentler, PM. Cutoff criteria for fit indexes in covariance structure analysis: conventional criteria versus new alternatives. Struct Equ Model Multidiscip J. (1999) 6:1–55. doi: 10.1080/10705519909540118
58. Pick, JB, and Azari, R. Global digital divide: influence of socioeconomic, governmental, and accessibility factors on information technology. Inf Technol Dev. (2008) 14:91–115. doi: 10.1002/itdj.20095
59. Barnett, ML, Ray, KN, Souza, J, and Mehrotra, A. Trends in telemedicine use in a large commercially insured population, 2005-2017. JAMA. (2018) 320:2147–9. doi: 10.1001/jama.2018.12354
60. Haimi, M. The tragic paradoxical effect of telemedicine on healthcare disparities- a time for redemption: a narrative review. BMC Med Inform Decis Mak. (2023) 23:95. doi: 10.1186/s12911-023-02194-4
61. Bouabida, K, Lebouché, B, and Pomey, M-P. Telehealth and COVID-19 pandemic: an overview of the telehealth use, advantages, challenges, and opportunities during COVID-19 pandemic. Healthcare. (2022) 10:2293. doi: 10.3390/healthcare10112293
62. Sawesi, S, Rashrash, M, Phalakornkule, K, Carpenter, JS, and Jones, JF. The impact of information technology on patient engagement and health behavior change: a systematic review of the literature. JMIR Med Inform. (2016) 4:e1. doi: 10.2196/medinform.4514
63. Kruse, CS, and Beane, A. Health information technology continues to show positive effect on medical outcomes: systematic review. J Med Internet Res. (2018) 20:e41. doi: 10.2196/jmir.8793
64. Nielsen, P, and Sahay, S. A critical review of the role of technology and context in digital health research. Digit Health. (2022) 8:20552076221109554. doi: 10.1177/20552076221109554
65. Chatterjee, A, Shahaab, A, Gerdes, MW, Martinez, S, and Khatiwada, P. Chapter 22 - leveraging technology for healthcare and retaining access to personal health data to enhance personal health and well-being In: S Bhattacharyya, P Dutta, D Samanta, A Mukherjee, and I Pan, editors. Recent trends in computational intelligence enabled research : Academic Press (2021). 367–76.
66. van Veen, T, Binz, S, Muminovic, M, Chaudhry, K, Rose, K, Calo, S, et al. Potential of mobile health technology to reduce health disparities in underserved communities. West J Emerg Med. (2019) 20:799–802. doi: 10.5811/westjem.2019.6.41911
67. ElKefi, S, and Asan, O. How technology impacts communication between cancer patients and their health care providers: a systematic literature review. Int J Med Inform. (2021) 149:104430. doi: 10.1016/j.ijmedinf.2021.104430
68. Horrigan, JB, Whitacre, BE, and Galperin, H. Understanding uptake in demand-side broadband subsidy programs: the affordable connectivity program case. Telecommun Policy. (2024) 48:102812. doi: 10.1016/j.telpol.2024.102812
69. Dullet, NW, Geraghty, EM, Kaufman, T, Kissee, JL, King, J, Dharmar, M, et al. Impact of a university-based outpatient telemedicine program on time savings, travel costs, and environmental pollutants. Value Health. (2017) 20:542–6. doi: 10.1016/j.jval.2017.01.014
70. Zhang, X, Hailu, B, Tabor, DC, Gold, R, Sayre, MH, Sim, I, et al. Role of health information technology in addressing health disparities: patient, clinician, and system perspectives. Med Care. (2019) 57:S115–20. doi: 10.1097/mlr.0000000000001092
Keywords: technology access, healthcare technology use, technology inequity, socioeconomic status, healthcare disparity/health disparities, African American
Citation: Larnyo E, Tettegah S, Nutakor JA, Addai-Dansoh S and Arboh F (2025) Technology access, use, socioeconomic status, and healthcare disparities among African Americans in the US. Front. Public Health. 13:1547189. doi: 10.3389/fpubh.2025.1547189
Edited by:
Rajiv Janardhanan, SRM Institute of Science and Technology, IndiaReviewed by:
Victoria Ramos Gonzalez, Carlos III Health Institute (ISCIII), SpainDhruva Nandi, SRM University, India
A.H. Sruthi Anil Kumar, SRM Medical College Hospital and Research Centre, India
Copyright © 2025 Larnyo, Tettegah, Nutakor, Addai-Dansoh and Arboh. This is an open-access article distributed under the terms of the Creative Commons Attribution License (CC BY). The use, distribution or reproduction in other forums is permitted, provided the original author(s) and the copyright owner(s) are credited and that the original publication in this journal is cited, in accordance with accepted academic practice. No use, distribution or reproduction is permitted which does not comply with these terms.
*Correspondence: Ebenezer Larnyo, ZWxhcm55b0B1Y3NiLmVkdQ==
†ORCID: Ebenezer Larnyo, orcid.org/0000-0001-5837-0707
Jonathan Aseye Nutakor, orcid.org/0000-0002-6294-2701
Stephen Addai-Dansoh, orcid.org/0000-0002-4070-6273