- 1Department of Workplace Health Promotion and Prevention, University of the Bundeswehr Munich, Neubiberg, Germany
- 2Agito Gesundheit GmbH, Cologne, Germany
Background: Occupational workload can contribute to significant health problems such as chronic stress, fatigue and burnout. To investigate the underlying mechanisms, it is necessary to monitor brain activity in real work environments. Functional near-infrared spectroscopy (fNIRS) is a portable, non-invasive neuroimaging method that captures neural correlates of occupational workload under natural conditions. However, despite its increasing application, a comprehensive overview of fNIRS-based research in this field is lacking. Therefore, this systematic review examines how fNIRS can be utilized to investigate occupational workload.
Methods: Following PRISMA 2020 guidelines, we conducted our systematic review by searching Web of Science, PubMed, and Scopus between November 15, 2023 and March 20, 2025. We included all studies published in English or German at any date, as long as they examined healthy adult professionals performing occupational tasks with functional near-infrared spectroscopy (fNIRS). Extracted data included study characteristics, workload details, signal processing methods, main fNIRS findings, and study quality, assessed using the JBI Critical Appraisal Tool.
Results: We included 41 studies. Of these, 23 reported a significant increase in oxygenated hemoglobin (HbO) concentration and functional connectivity in the prefrontal cortex (PFC) under higher occupational workload conditions. Only five studies examined typical office tasks. Nine studies analyzed differences in cortical activation between experts and novices, with experts showing increased HbO concentration in the PFC than novices. Regarding methodology, 26 studies used standardized optode placements, while only 17 applied systemic and extracerebral artifact correction. Small sample sizes and the absence of randomized controlled trials limited the reliability and reproducibility of the findings.
Conclusion: Functional near-infrared spectroscopy effectively detects neural correlates of occupational workload and provides objective insights into cognitive demands in real-world work settings. Standardizing optode placement, harmonizing signal-processing methods, and increasing sample sizes would enhance the validity and comparability of future research. Expanding investigations to typical office environments is also crucial for understanding daily workload and for developing interventions that promote employee well-being and productivity. Overall, fNIRS represents a promising tool for establishing evidence-based workplace health promotion strategies across diverse occupational settings.
1 Introduction
Occupational workload significantly impacts the well-being and performance of workers in industrialized nations (1). To effectively address this challenge, it is crucial to understand and accurately measure occupational workload. Several key theoretical frameworks have been developed to facilitate this understanding, including Cognitive Load Theory (2, 3), the concept of mental workload (4–6), the Job Demand-Control model (7), and the Effort-Reward Imbalance model (8). These theories conceptualize occupational workload as a multidimensional construct that encompasses cognitive, physical, and psychosocial demands, which in turn shape the available cognitive resources an individual can allocate to meet task demands (9). Whilst excessive workload decreases human performance, an overly low workload potentially reduces motivation and interest (10). Thus, the level of attentional resources required to meet both objective and subjective performance criteria can be influenced by task demands, external support, and previous experiences (8). Increasing human-computer interaction, globalization, and demographic changes in industrialized nations are widely recognized as factors that heighten pressure on employees (11–13). These challenges are intensified by constant availability, faster workflows, greater complexity in modern work environments, and often insecure job conditions, all of which increase pressure on employees (14). When occupational demands chronically exceed an individual’s capacity to cope, this sustained imbalance can lead to serious health issues such as exhaustion and burnout (15). These health issues have become major socio-economic challenges for companies (16), and impose a substantial burden on health care systems (17–19).
Traditionally, occupational workload has been assessed using standardized questionnaires like the NASA Task Load Index (20). Since physiological measurements allow for unobtrusive data collection without interfering with primary tasks, interest in these objective methods has been increasing (21). Techniques like electrocardiography (ECG), eye tracking, respiration, and electromyography (EMG) provide quantifiable insights but do not directly capture the underlying neural mechanisms of occupational workload (22, 23). To address this limitation, traditional neuroimaging methods such as electroencephalography (EEG) and functional magnetic resonance imaging (fMRI) have emerged as critical tools for assessing the neural correlates of occupational workload (9, 23–25). These methods offer objective insights that surpass traditional physiological measures, allowing for a more precise characterization of neuronal activity (26). Specifically, fNIRS facilitates the detection of neural overloads within executive function networks, which are critical for processes such as decision-making and attentional control (27, 28). Neural overload occurs when cognitive demands exceed the brain’s processing capacity, particularly in executive functions (29, 30). This overload leads to heightened activity in regions like the dorsolateral prefrontal cortex (DLPFC), anterior cingulate cortex (ACC), and inferior parietal lobule (IPL), leading to cognitive impairment, reduced performance, and increased error rates in work contexts (31–33). If this neural overload remains unaddressed, it can contribute to long-term health issues like chronic stress, fatigue, and burnout (34, 35). However, while effective for neural insights, EEG and fMRI are often unsuitable for dynamic work environments due to their susceptibility to movement artifacts from head and body movements and the requirement for participant immobility (36–38). Continuous monitoring of neural activity in real-world occupational settings is essential for understanding how workload accumulates and impacts cognitive performance, enabling interventions to prevent cognitive overload (39).
Functional near-infrared spectroscopy (fNIRS) is considered a promising tool for measuring occupational workload, as it provides robust data under ecologically realistic conditions (40–45). In brief, fNIRS is an imaging technique indirectly measuring brain activity by using near-infrared light (650–1,000 nm) absorbed by hemoglobin in the brain to measure changes in the concentrations of oxygenated hemoglobin (HbO) and deoxygenated hemoglobin (HbR) (46). Compared to other imaging techniques such as fMRI and EEG, fNIRS offers moderate spatial resolution and potentially better temporal resolution but is limited to neocortical regions (47), and exhibits a lower signal-to-noise ratio than fMRI (48). FNIRS advantages include tolerance for motion artifacts, ease of use, portability, and low costs, making it suitable for studies in natural settings and promoting its use in real work environments (47, 49).
Due to its practical feasibility and advantages over other imaging techniques, fNIRS has increasingly been employed to assess occupational workload in real-world settings such as piloting aircraft (40), operating urban rail transport systems (50), or performing office work (51). These applications demonstrate the potential of fNIRS to provide valuable neurophysiological insights in occupational environments. By identifying neural markers under everyday conditions, fNIRS offers objective data crucial for the early detection and prevention of occupational overload and long-term health issues like chronic stress, fatigue, and burnout. This technology bridges the gap between basic neuroscience research and occupational health promotion, providing a practical tool for implementing evidence-based interventions in the workplace. Therefore, this systematic review addresses the following research questions: How is fNIRS utilized to investigate occupational workload?
2 Materials and methods
This systematic review is conducted according to the Preferred Reporting Items for Systematic Reviews and Meta-Analysis Extension for Systematic Reviews PRISMA-2020 guidelines (52, 53).
2.1 Search strategy
A systematic literature search was initially conducted from November 15, 2023, to December 01, 2023, in the electronic databases PubMed, Web of Science, and Scopus. To ensure that the review reflected the most recent state of research, an updated search was performed from December 1, 2023, to March 20, 2025. Eight additional studies identified during this second screening were assessed for eligibility and fully integrated into the review. These studies are also listed in Supplementary Table S2. Search queries systematically integrated pertinent keywords using Boolean operators (‘OR’, ‘AND’) to optimize precision. A detailed description of these queries is provided in Table 1.

Table 1. Search terms utilized in the databases PubMed, Web of Science, Scopus for the measurement of health hazards at the workplace using fNIRS Boolean operator.
2.2 Inclusion criteria
The eligibility criteria were guided by the PICOS guidelines (54, 55), as shown in Table 2. We used a combined keyword strategy instead of separate terms for each PICO component to ensure a broad capture of studies using fNIRS in occupational contexts. This approach helped identify a wide range of articles without excluding those that might use different terminology for similar research questions. The inclusion criteria included articles that were: (a) written in English and / or German, (b) based on measurements of cortical activity using functional Near-Infrared Spectroscopy (fNIRS), (c) involving healthy working adults studied within their professional milieu, and (d) designed with a workplace-oriented experimental focus, specifically addressing professional and occupational contexts.
2.3 Exclusion criteria
Studies that did not fulfill one of the following criteria were excluded from the literature search: (a) fNIRS was not used as the main modality for cerebral examination techniques; (b) lack of a clear occupational context, meaning that there was no direct association with job affiliation or occupational activity; and (c) study population of professional athletes and professional musicians. Professional athletes and musicians were excluded due to their unique performance contexts and specialized training demands, which differ substantially from conventional workplace tasks and could introduce confounding factors when interpreting fNIRS data in an occupational setting.
2.4 Study selection
The screening procedure utilized Rayyan software (56) to automatically eliminate duplicates from the collected literature. Subsequently, the titles and abstracts were evaluated based on the predefined inclusion and exclusion criteria and classified as relevant, irrelevant, or indeterminate. Records deemed irrelevant were discarded. The remaining articles underwent a full-text review for further evaluation and potential exclusion.
This entire process was executed in a double-blinded manner with two independent reviewers (RG, OM), which aimed to minimize the risk of biased outcomes and proactively address any methodological issues. Disagreements between the reviewers were resolved either by reaching a consensus or consulting a third reviewer (AS).
2.5 Data extraction
During the data extraction process, relevant study characteristics were collected with a meticulously designed data extraction sheet. These characteristics comprised the name of the first author and the publication year, the study sample, including occupation description, years of professional experience, sample size, sex, and age (as illustrated in Table 3). Additionally, aspects of the study design were examined using a second structured form, which encompassed specifications of the control group, procedures for randomization, protocols for follow-up, descriptions of conflict tasks, and types of workplace environments, as presented in Table 4.
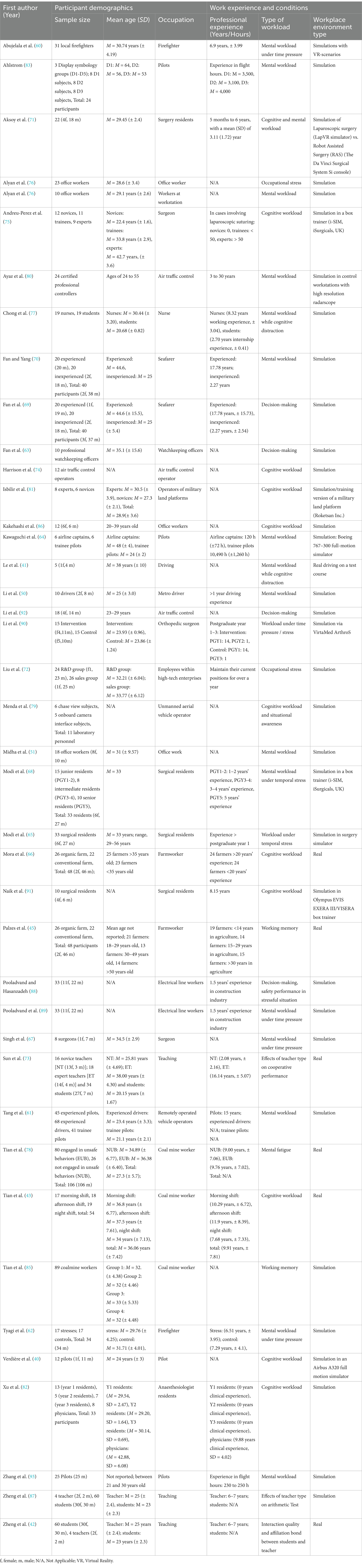
Table 3. Study characteristics, participant demographics and occupational workload information of included studies.
Subsequently, a third structured approach was employed to extract signal-processing components specific to fNIRS from the methods sections of the included articles. These components were categorized based on the framework established by Kohl et al. (57) and were further adapted to address the specific focus of the current research. This involved meticulous examination of fNIRS device specifications, identification of brain regions-of-interest, delineation of target regions (including channels of interest and positioning systems), preprocessing techniques, artifact control strategies [including additional measurements such as short-distance measurements, Electromyography (EMG), Electroencephalography (EEG), electrocardiogram (ECG)], selection of chromophore used for feedback (oxygenated hemoglobin – HbO, deoxygenated hemoglobin – HbR, or total hemoglobin – HbT), and the methodology employed for calculating the feedback signal. These details are comprehensively presented in Table 5.
Both extraction and assessment of all method-specific information were conducted by RG, with validation of accuracy by OM.
2.6 Study quality
To evaluate the methodological quality of the included studies, we used the checklist from the Joanna Briggs Institute (JBI) Critical Appraisal Tool for quasi-experimental studies (58). Although the PICOS framework was considered, the JBI tool was deemed most appropriate due to its stringent criteria tailored to quasi-experimental designs, which are commonly used in systematic reviews when randomized controlled trials (RCTs) are unavailable or scarce. The revised JBI tool integrates recent advancements in bias assessment, making it an optimal choice for this review. Despite recognizing some limitations, we believe this approach achieves the best balance between thorough assessment and methodological rigor. Two of the authors (RG, OM) independently assessed the studies based on the nine criteria of the checklist. These criteria encompass clarity of cause and effect (temporal relationship between variables), similar participants, similar treatment in compared groups, the existence of a control group/condition, multiple measurement points of the outcome, completion of follow-up, similar outcome measurements in compared groups, reliability of outcome measurements and appropriate statistical methods.
Points were allocated to each study based on the number of criteria fulfilled. While we set a criterion of 7 or more ‘yes’ responses to assess the methodological quality, no studies were excluded from the synthesis based on a lower score. This approach aligns with the general recommendation from JBI to include all eligible studies in the synthesis, ensuring a comprehensive analysis of the available evidence (58, 59). Disagreements between the reviewers were resolved either by reaching a consensus or consulting a third reviewer (AS). For further details about rating criteria, see Supplementary Table S1.
3 Results
3.1 Identification of studies
The systematic search resulted in a total of 4,258 articles. After the automated removal of duplicates from the record list using the data management software Rayyan and the subsequent title and abstract screening, a total of 201 articles were identified as potentially relevant, and eligibility was assessed. In the conclusive stage of the full-text screening, 33 articles were deemed pertinent for subsequent data extraction. To ensure that the review reflected the most recent state of research, an additional screening step was conducted, resulting in the identification and inclusion of 8 further eligible studies. These were identified after the initial submission, during the peer-review process, and were fully integrated into the synthesis. In total, 41 studies were included in the final review. A schematic overview of the study selection process is presented in Figure 1.
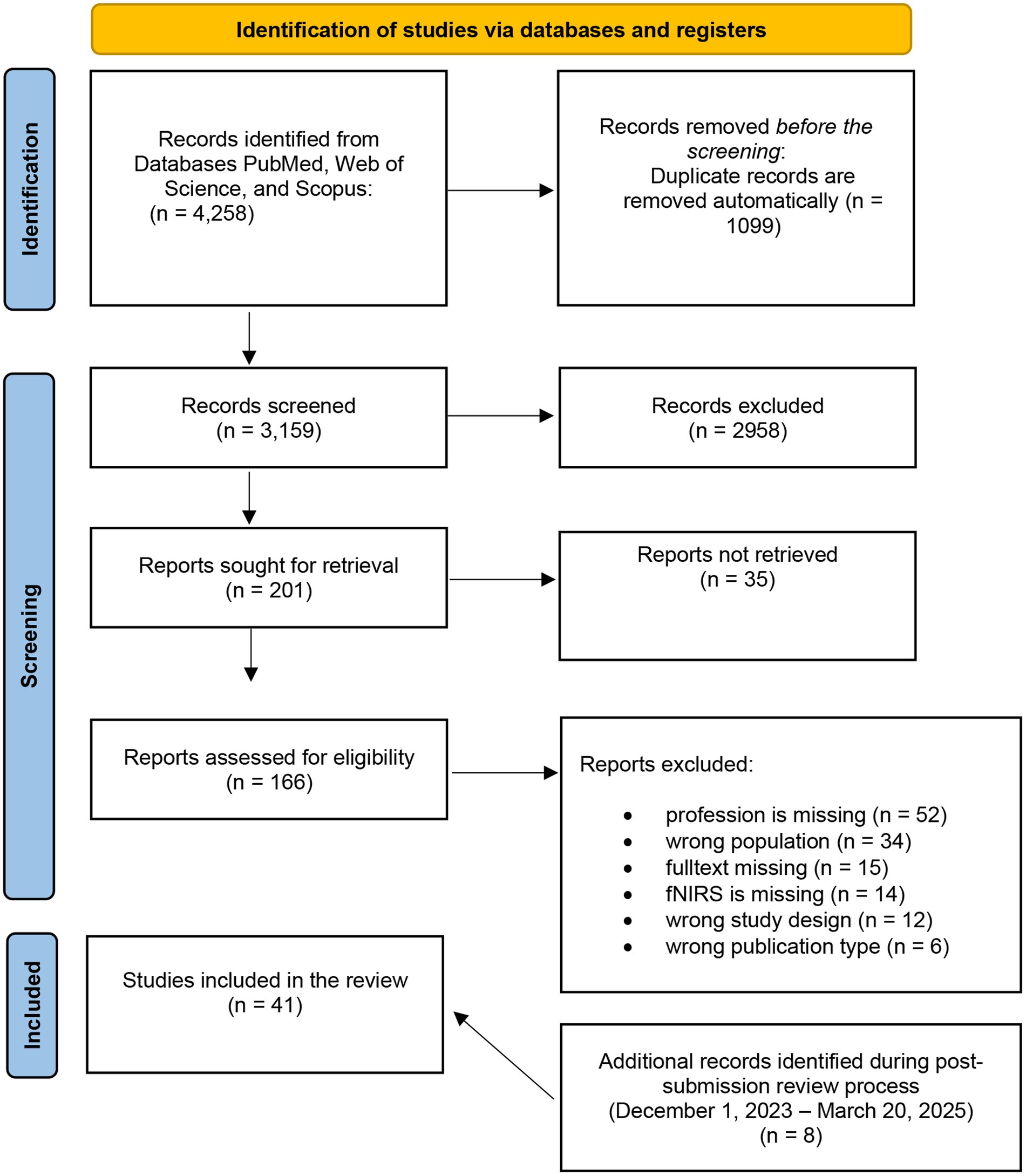
Figure 1. PRISMA 2020 flow diagram for new systematic reviews which included searches of databases, and registers.
3.2 Study characteristics
Table 3 summarizes the characteristics of the 41 included studies. Sample sizes ranged from 5 participants (41, 60) to 154 participants (61). 15 studies included exclusively (62–64) or predominantly male participants (>80%) (40, 41, 45, 50, 65–72). Only one study (73) focused primarily on female participants, while four reported a balanced gender distribution. 15 studies did not report gender information (43, 60, 61, 74–85). The participants’ age range varied widely across studies. For example, trainee pilots had an average age of 21.1 (± 2.1) (61) and experienced seafarers averaging M = 44.6 years (± 15.5) (69, 70).
3.3 Occupation and professional experience in years
The studies covered a variety of occupations and work environments. Sedentary work environments were represented in studies on office work (51, 72, 76, 84, 86), and teachers (42, 73, 87). Physically active occupations included firefighters (60, 62), miners (43, 78, 85), agricultural workers (45, 66), military personnel (81), seafarer (63, 69, 70), and electrical line workers (88, 89). Medical professions were represented by surgeons (65, 67, 68, 71, 75, 90, 91), anesthesiologists (82), and nurses (77). The aviation and transportation sector was represented by drivers (41) air traffic controllers (50, 74, 80, 92), pilots (40, 64, 80, 83, 93), drone pilots (61, 79), and subway drivers (50).
Participants’ professional experience varied widely, ranging from 1 year (68, 71, 82, 88–90) to over 30 years (45, 80). Ten studies compared different levels of professional experience, for example between experts and novices, about job-specific tasks (61, 64, 68–70, 73, 75, 81–83). In 13 studies, the participants’ professional experience was not specified (40, 41, 51, 63, 67, 72, 74, 76, 79, 81, 84, 86, 92).
3.4 Types of workloads
The focus of the investigated occupational workload was primarily on mental workload (7, 41, 50, 51, 60–65, 67, 68, 70, 71, 76, 77, 80, 83, 89, 93) and cognitive workload (40, 43, 66, 71, 74, 75, 79, 81, 82, 86, 91). Mental workload refers to the cognitive demands of processing, storing, and managing information during task performance, influenced by task complexity, environment, and individual capabilities (4–6). Cognitive workload, based on Cognitive Load Theory (2, 3) describes the mental effort needed to process and integrate information, shaped by task complexity, instructional design, and cognitive resources. Although we distinguished between mental and cognitive workload based on each publication’s terminology, both terms refer to the cognitive demands and effort exerted during occupational tasks. For our purposes, we treated them as functionally equivalent under the broader concept of occupational workload. Among the studies included, six studies also investigated temporary stress (65, 68), or time pressure (60, 62, 67, 90) in addition to mental or cognitive workload. In total, 29 studies examined the effects of occupational activities on cognitive and mental workload, occasionally under additional stress while performing or learning job-specific tasks (67, 72).
Five studies investigated decision-making in job-specific contexts (63, 69, 88, 89, 92). Another three studies focused on teacher-student interactions, including cooperative performance, quality of interaction, and affective commitment (42, 73, 87). Two studies analyzed the effects of pesticide exposure on the cognitive functioning of agricultural workers (45, 66). One study investigated mental fatigue and functional brain connectivity in miners (78).
3.5 Design aspects
3.5.1 Methodological quality (JBI critical appraisal tool)
Supplementary Table S1 summarizes the assessment results for each study. On average, the studies met 3.6 (SD = 2.09) of the 9 essential quality criteria, thus receiving a ‘yes’ rating. Notably, only seven studies were deemed to employ appropriate statistical methodologies. Many studies were considered deficient in statistical rigor due to inadequate justification of their sampling plans or failure to classify their research as pilot, feasibility, or concept studies. Additionally, the lack of control groups further contributed to the methodological shortcomings of these studies, significantly diminishing their scientific robustness. Importantly, question 9, which concerns statistical conclusion validity, was not included in the overall risk of bias assessment, aligning with the guidance of the JBI tool to focus solely on internal validity (59).
3.5.2 Randomization
Of the 41 included studies, four randomized the assignment of participants to different groups (62, 73, 85, 90). Eight studies randomized the sequence of conditions within the utilized conflict tasks (63, 65, 67, 68, 71, 82, 83, 93), such as the randomization of condition employed in various flight scenarios (69, 82, 93). Additionally, five other studies used pseudo-randomization for group assignment (42, 87) the order of conditions (40, 80), or the order of participants (74). One study used quasi-randomized group for distraction task (69, 70).
3.5.3 Control conditions
Only six of the 41 studies included a control group in their study design. Two studies divided firefighters into stress and control groups to compare prefrontal cortex brain activity during occupational workload under time pressure in a between-subjects design (60, 62). Additionally, two other studies compared the effects of pesticides in agriculture on working memory performance and prefrontal cortex activity between organic and conventional farmers in a between-subjects design (45, 66). Menda et al. (79) divided unmanned aerial vehicle (UAV) pilots into two groups: Group 1 followed tasks from a chase view, while Group 2, serving as the control group, used the onboard camera interface to investigate the use of fNIRS for monitoring cognitive workload and situational awareness of UAV operators during simulated missions in a between-subjects design. Li et al. (90) examined whether a two-week mindfulness training program could enhance performance under time pressure in a simulated knee arthroscopy task. The intervention group received structured mindfulness training, while the control group underwent no additional training.
3.5.4 Follow-up measures
Six studies assessed the effects of occupational workload with follow-up periods measurements ranging from several days to multiple weeks. Two studies focused on air traffic controllers: one examined the stability of cognitive performance across repeated sessions (92), while the other investigated the influence of conflict resolution advisories on workload during a multi-day training course (74). Other follow-up designs included the assessment of sustained functional connectivity in nurses (77), the consistency of neurophysiological responses in urban rail drivers (50), and the long-term effects of pesticide exposure in farmworkers (66). Additionally, one study conducted follow-up measurements to evaluate the effects of a two-week mindfulness intervention on surgical performance under time pressure in orthopedic training (90).
3.6 Signal processing aspects
3.6.1 Devices
Fifteen different devices were used across the studies: ETG-4000, OT-R40, NIRSport/NIRSport 2, NIR Smart fNIRS, NIRSIT, ASTEM’s fNIRS, LABNIRS, fNIRS PioneerTM, fNIRS Imager 1,002, Brite MKIII, Artinis Brite24, BS-3000, fNIR Devices LLC, NIRSport 88, LIGHTNIRS, WOT-100 System, and Octamon. Additionally, one study employed a custom-developed portable fNIRS system (61), and another study did not specify the device manufacturer (79), as shown in Table 5. The frequency of use of certain devices does not imply qualitative superiority. The primary differences between the devices include the wavelengths used and the number of wavelengths supported by the systems. Some devices use two wavelengths (e.g., ETG-4000, NIRSport/NIRSport 2, Brite MKIII, Brite24), while others support three wavelengths (e.g., OT-R40, LABNIRS). Each device utilizes different wavelengths within the range of 690 to 860 nm, and they exhibit varying degrees of accuracy (e.g., dynamic range or sensitivity), depending on the hardware and its quality.
3.6.2 Target brain regions: selection of channels of interest
The majority of studies focused on investigating cortical activity in the prefrontal cortex (PFC), particularly the dorsolateral prefrontal cortex (dlPFC), the frontopolar cortex (FPC), and the orbitofrontal cortex (OFC). The motor cortex (MC), including the premotor cortex, the supplementary motor area (SMA), and the primary motor cortex, was also a frequently studied area. Cortical activities in the temporal cortex (TC), parietal cortex (PC), and occipital cortex (OC) were only analyzed in a few studies. One study additionally focused on Broca’s area in the left hemisphere (66).
To identify specific brain regions, the studies employed various methods for optode placement on participants’ heads. All studies relied on a priori knowledge of brain region locations. Among the 41 studies, 20 used the EEG 10–20 system (94) as a reference for optode placement (42, 45, 50, 63, 65–70, 73–77, 82, 87, 91–93), while six used the EEG 10–10 system (60, 62, 75, 84) or the 5–10 system (65, 85). Additionally, seven studies utilized the MNI space registration methods outlined by Okamoto et al. (95), Singh et al. (96) and Tsuzuki et al. (42, 64, 72, 75, 77, 84, 87, 97). Additionally, six studies performed 3D digitizer measurements to verify optode positions (42, 43, 64, 72, 78, 92). Conversely, 13 studies provided no specific information on additional systems or optode registration methods (40, 41, 51, 61, 70, 71, 79–81, 83, 86, 88, 90). The number of channels used varied between 1 and 54.
3.6.3 Chromophores and visualization of cortical activity
To visualize cortical activity using fNIRS, 26 of the 41 studies used HbO as the preferred chromophore (42, 43, 50, 51, 60, 62–65, 69–73, 76–78, 85–93). Eleven studies used both HbO and HbR (40, 45, 50, 66–68, 74, 75, 80, 82–84). Three studies used all three chromophores: HbO, HbR, and HbT (10, 60, 79). Only one study used HbT to derive cortical activity (81) (see Table 5).
3.6.4 Signal pre-processing and artifact control
The fNIRS signal is subject to various sources of noise, particularly physiological noise such as low-frequency blood pressure oscillations (Mayer waves) (98, 99), vasomotor interference (100), and movement artifacts (e.g., head movements) (101). These interferences can overlap with the task frequency. Various preprocessing methods were used to reduce the noise. In 22 studies, the signal was filtered using low-pass filters (50, 60, 61, 64, 65, 67–71, 74–77, 79–81, 84, 86, 88, 89, 91). 17 studies used band-pass filters, which included both low-pass and high-pass filters (40, 41, 43, 45, 51, 60, 62, 63, 66, 73, 76, 78, 82, 84, 88, 92, 93). Six studies did not report or use a filtering method (42, 73, 76, 83, 87, 90).
Four studies that applied artifact control used either reference channels (43, 78) or ECG measures for post-hoc artifact control to detect changes in heart rate (77, 91). Two study used post-hoc EEG measurement for artifact control and compared neuronal activity in synchronization measurements of brain connectivity with EEG and fNIRS (84, 91). 15 of 41 studies did not use or report any explicit artifact control on top of the filtering methods (42, 61, 64, 69, 72, 73, 79, 80, 83, 84, 86–90). Three studies used a sliding-window motion artifact rejection (SMAR) procedure which rejected motion-affected periods in the fNIRS signal (72, 81, 92). 14 studies used wavelet filters for real-time spike artifact removal (40, 43, 45, 50, 51, 60, 62, 63, 66, 71, 76–78, 82). Three studies removed motion artifacts through visual inspection (67, 70, 74). Eight studies applied a channel rejection based on amplitude thresholding and a signal-to-noise ratio from 1 to >2 (63, 67, 68, 72, 80) or applied channel rejection without any further information (75, 80, 88). Two study implemented short-distance channels for artifact control and applied short-channel regression within a general linear model (GLM) framework (63, 85).
3.6.5 Data processing and calculation of workload information
In almost all of the studies reviewed, baseline correction and averaging (e.g., across channels, trials, and/or distinct time periods) were conducted. The average cortical activity measurements for the chromophores used (HbO, HbR, and HbT) during the task block were generally used and compared either to a pre-recorded baseline (41, 43, 51, 61, 64, 65, 67, 68, 71, 72, 75, 76, 78, 79, 82, 84), the fNIRS-system baseline (42, 73, 81, 87), or a GLM baseline (45, 66, 88, 89). The length of the baselines varied as follows: Four studies used a baseline of ≤5 s after the trial onset (41, 43, 61, 78), four studies used a baseline of 10 s after the trial onset (51, 65, 72, 75), and seven studies used a baseline of ≥10 s after the trial onset (68, 71, 76, 79, 82, 84, 90). 13 studies did not provide length specifications for the baseline comparison (40, 42, 50, 60, 63, 64, 69, 70, 74, 80, 81, 86, 93). In other studies, the peak value of cortical activity proxies over a specific time period was used for statistical analysis (76, 78).
3.7 Cortical hemodynamics during occupational workload
Most studies assessed cortical activity, (HbO and HbT), during occupational workload. A total of 16 studies integrated standardized neurobehavioral tasks alongside cortical measurements. Of these, seven employed the n-back task to assess working memory or cognitive workload (41, 50, 80, 88, 89, 91, 92), or a short-term visual memory task (85). Three studies used the Montreal Imaging Stress Task (MIST) to induce cognitive stress (76, 84, 86). Two applied a modified Sternberg letter-retrieval task to evaluate short-term memory (45, 66). One study combined a Go/No-Go task with the Wisconsin Card Sorting Test to assess executive functions (66). Only two studies investigated the effects of occupational workload on cortical activity during subsequent rest phases (43, 78).
Twenty three of the 41 studies reported increased HbO or HbT levels in the PFC during occupational workload. These studies spanned various professional sectors. Five studies focused on office work settings (51, 72, 76, 84, 86). Seven studies involved physically active occupations, such as maritime navigation and manual labor (45, 63, 69, 70, 81, 88, 89). Four studies examined medical professionals performing tasks such as surgery (65, 67, 82, 91). Additionally, five studies investigated individuals in the aviation and transportation sectors (64, 74, 80, 92, 93). Four studies also reported increased activity in the SMA, motor cortex, and premotor cortex during occupational tasks (76, 84, 88, 93). Functional connectivity (FC), reflecting interactions between brain regions, was analyzed in five studies. Increased FC between the PFC and FPA was observed across professions, including seafarers (63, 69), air traffic controllers (92), drivers (41), firefighters (62), and student nurses (77). Two studies reported increased FC between the FPA and dlPFC during rest in miners (43, 78). Additionally, two studies found increased brain synchronization during teacher-student interactions in both interactive and lecture settings (42, 87).
Conversely, several studies reported significant decreases in HbO concentrations during occupational workload. Participants using an ergonomic workstation exhibited decreased HbO levels in the medial, right, and left dlPFC during the MIST (86). HbO decreased over time in the right PFC during maritime watchkeeping tasks (70). Under additional time pressure, decreased HbO in the dlPFC was observed in firefighters (62), drivers (41), and junior surgical residents (65, 68, 71). High workload during flight simulator tasks led to decreases in HbO in the PFC and motor cortex (93). Additionally, morning and afternoon shifts reduced FC in the dlPFC during rest among coal miners (43).
Ten studies examined cortical activity differences between experts and novices during occupational workload. Experts generally exhibited increased HbO in the PFC compared to novices. This was evident in seafarers during decision-making tasks involving spotting distant targets (69, 70), surgeons performing laparoscopic suturing under time pressure (65, 68), and operators of military land platforms during advanced tasks (81). Experts also showed increased FC between the PFC and motor areas during laparoscopic suturing under time pressure (75). Teacher-student cooperative tasks revealed increased brain synchronization in the left dlPFC in expert dyads compared to novice dyads (42, 87). Conversely, experienced drone pilots (61) and surgeons (65) exhibited decreased HbO levels in the PFC over the task duration compared to novices.
4 Discussion
In this systematic review, we analyzed 41 studies that utilized fNIRS to measure cortical activity in healthy adult workers during occupational tasks. We extracted methodological details such as study characteristics, design aspects, and signal processing methods. Additionally, we collected information on occupational workload and the main findings related to cortical hemodynamics during work-related tasks. The primary goal was to determine how fNIRS is employed to investigate occupational workload.
The results demonstrate that fNIRS is effectively used to assess workload in various professions. Different occupational tasks are associated with specific changes in cortical hemodynamics, particularly in the PFC. In safety-critical and cognitively demanding fields such as aviation, maritime navigation, and medicine, a significant increase in HbO and HbT concentrations within the PFC was frequently observed during occupational workload (45, 63–65, 67, 69, 70, 74, 80–82, 88, 89, 91–93). The PFC is crucial for executive functions such as working memory, attention regulation, and decision-making (27, 102). The observed hemodynamic responses suggest heightened neural activation due to increased cognitive demands and complex decision-making inherent in these professions. This finding aligns with cognitive load theory and mental workload models, which propose that higher task demands require more cognitive resources, leading to increased neural activity in executive brain regions (4, 6). For example, experienced seafarers showed increased PFC activation during navigation-related decision-making tasks, which correlated with better task performance. This suggests efficient use of executive functions to manage complex operational environments (63, 70). These results are consistent with neuroimaging studies associating increased PFC activation with higher cognitive load and engagement of executive control networks (103–106). However, these cortical activations should not be considered as linear indicators of task difficulty. From the perspective of neural efficiency, HbO and HbT patterns instead reflect how optimally cognitive resources are allocated. Experts may display lower PFC activation while achieving high performance, suggesting more efficient neural processing, whereas novices often recruit larger cortical regions to perform at a similar level. Over time, training can restructure these activation patterns, with reduced HbO marking an improved cognitive strategy rather than diminished engagement. Consequently, both increases and decreases in fNIRS signals should be interpreted as markers of adaptive cortical regulation, rather than direct proxies for occupational workload.
Additionally, functional connectivity serves as a robust indicator of occupational workload. Studies have observed increased functional connectivity between the PFC, FPA and motor areas across diverse professions, including seafarers (63, 69), air traffic controllers (92), drivers (41), firefighters (62), and student nurses (77). This heightened connectivity reflects the enhanced integration of cognitive and motor processes essential for executing complex tasks that require simultaneous attention, decision-making, and motor responses (27, 107). These findings are corroborated by neuroimaging studies utilizing fNIRS and EEG, which have demonstrated that increased workload is associated with increased connectivity within executive and attentional networks (22, 108, 109). Thus, it appears that improved functional connectivity underlies the effective integration of cognitive processes that enables adaptive responses to complex task demands and the maintenance of performance under pressure.
Despite the effective use of fNIRS in assessing occupational workload in safety-critical and cognitively demanding professions, research in typical office settings remains scarce (51, 72, 76, 84, 86). This gap is significant, given that office work constitutes a large portion of employment and involves specific cognitive demands. Office tasks often require sustained attention, multitasking, and managing information overload, leading to cognitive fatigue and potential neural overload (110, 111). The included studies demonstrate that even routine office tasks can impose substantial cognitive load, reflected in increased HbO concentrations in the prefrontal cortex (PFC). Midha et al. (51) found elevated HbO in the left PFC during more difficult reading tasks with interruptions. Similarly, Kakehashi et al. (86) reported increased HbO in the PFC during challenging arithmetic and copying tasks. These findings indicate that typical office activities engage executive functions mediated by the PFC. Prolonged high cognitive load can lead to neural overload in the PFC, resulting in cognitive impairment, reduced performance, and increased error rates (31–33). According to Cognitive Load Theory (2, 3), when task demands exceed an individual’s cognitive resources, executive functions such as decision-making and attentional control are impaired.
Understanding the neural correlates of occupational workload in office environments is crucial for developing interventions to prevent cognitive overload and its adverse effects on employee well-being and performance (15, 88). Utilizing fNIRS in these settings can provide valuable insights into how everyday work demands affect cortical activity, informing evidence-based strategies for workload management. Therefore, future research should employ fNIRS to investigate occupational workload in office settings. Such studies are essential to bridge the gap between neuroscience and occupational health promotion, ultimately enhancing employee well-being and productivity.
Our analysis revealed that 26 of the 41 studies used standardized optode placement based on the EEG 10–20 system or its extensions (10-10 and 10-5). Standardized EEG positions facilitate virtual registration of fNIRS optodes and probabilistic estimation of Montreal Neurological Institute (MNI) coordinates (112–114). Combining this method with functional localizers accounts for individual variability and allows for a more detailed determination of optimal placement (115). These practices align with the work of Yücel et al. (116), which emphasizes the importance of standardized placement protocols to increase accuracy and reproducibility. Menant et al. (117) and Pinti et al. (47) also underscore the necessity of precise placement methods to ensure the quality of fNIRS data, highlighting the 10–20 EEG system as the predominant placement strategy. Our findings indicate a common approach among the reviewed studies regarding optode placement strategies, primarily favoring the EEG 10–20 system. However, we observed considerable diversity in the signal processing methods employed. While standardized optode placement contributes to comparability and reproducibility, variations in signal processing techniques may affect the consistency of results across studies. It is crucial to consider the methodological rigor necessary to ensure the accuracy, comparability, and reproducibility of fNIRS research findings. Precise localization of functionally active brain regions remains a central concern in neuroscience research (118, 119). Although fNIRS does not provide direct anatomical information, standardized optode placement is essential for ensuring comparability of results (120). Co-registration with fMRI is considered the gold standard for anatomical localization but is often impractical due to resource constraints (120, 121). Alternatively, researchers can use a digitizer to map the three-dimensional coordinates of the fNIRS channels onto an anatomical atlas (112, 117).
While most studies adopted standardized optode placement, only 17 explicitly implemented methods to correct systemic and extracerebral artifacts. Techniques used included wavelet filters, visual inspections to remove motion artifacts, and the use of reference channels. It is important to note that reference channels must be independent of the target region to avoid influencing the feedback signal (122). Wavelet filters are particularly effective in removing motion artifacts and are considered promising for correcting fNIRS data (120, 121). The use of additional measurements to control subtle movements and systemic factors like heart rate, respiration, or mean arterial pressure was largely neglected, appearing in only one study (77). Future research could incorporate electromyography (EMG) signals as control variables to minimize motion artifacts by including them in the general linear model used for signal calculation (57, 101). Monitoring heart rate through heart rate monitors or electrocardiography can further support the interpretation of cortical hemodynamic changes, as these are associated with systemic blood flow variations (98, 123, 124), cognitive performance, and mental workload (21, 125).
An efficient method for correcting extracerebral physiological signals is the use of short-separation channels combined with the GLM (126–128). Only two (63, 85) of the included studies employed this technique, possibly due to hardware limitations in most fNIRS systems (124, 129, 130). Implementing advanced artifact correction methods is essential to enhance data quality and validity. Careful selection and application of signal processing methods are necessary to avoid distorted or misleading information, especially since there are no established standards for processing fNIRS data (47, 98, 131). Future studies should explore various methods and provide detailed information to facilitate successful replication.
We also observed inconsistencies in sample sizes, with many studies using inadequate participant numbers, limiting the reliability of their findings. Only two study (77, 90) conducted a sensitivity power analysis using G*Power 3 to determine sample size (132). This aligns with trends in neuroimaging research, where statistical evaluations of sample size are rare, leading to low statistical power and higher variability in effect size estimates (133, 134). Small samples tend to overestimate reported effects (135). To address this, researchers advocate for sample size planning based on adequate power analyses to make findings more meaningful (136, 137). Defining the “Smallest Effect Size of Interest” (SESOI) is a potential solution, allowing studies to detect effects that are practically or clinically relevant and enhancing resource efficiency (138, 139). Doing so makes it possible to detect effects that are practically or clinically relevant, thus ensuring more efficient use of resources and strengthening the robustness of the results. Achieving an accurate power analysis also depends on clearly defined hardware configurations, optode placement, and data preprocessing methods (138, 139). Variations in these parameters can alter signal quality and effect sizes, which in turn affects the assumptions underpinning sample-size calculations. Adopting standardized protocols and transparent reporting of these parameters, as recommended in best-practice guidelines (47), will help researchers more reliably estimate the sample size needed for their particular fNIRS setup. Ideally, these details should be established and preregistered before data collection, ensuring methodological consistency and allowing future studies to compare their results across different contexts (136, 137).
A significant issue is the widespread use of quasi-experimental designs, which offer limited insights into intervention effectiveness and measurement validity (140). Transitioning to RCTs is essential to strengthen the evidence base of fNIRS methods. RCTs, regarded as the gold standard in clinical research, provide a robust assessment of causal effects (141). Implementing RCTs would enhance methodological rigor, leading to more reliable and generalizable results (142). The lack of standardized protocols and methodological consensus in fNIRS research hampers comparability and reproducibility (47). RCTs could mitigate these issues by promoting systematic and controlled approaches to investigating intervention effects (24). Standardized protocols would significantly improve data quality and consistency, crucial for establishing fNIRS as a reliable neuroimaging tool in studying occupational workload (143). For example, Yücel et al. (116) emphasized the importance of standardized procedures for enhancing study quality and comparability.
4.1 Limitations and strengths
First, the publication bias cannot be entirely excluded, as variations in terminology across studies may have led to the omission of relevant research. Secondly, the review was limited to studies published in English and German up to March 2025, potentially overlooking significant research in other languages. Thirdly, the terminology for the concept of occupational workload is not consistently defined and is used differently across various fields. This lack of terminological consistency complicates the systematic identification and analysis of the phenomenon in the existing literature.
Despite the limitations mentioned, this systematic review provides valuable insights into the application of fNIRS in occupational contexts. The study shows considerable methodological variability, particularly in the choice of signal processing techniques and the use of standardized protocols. The comprehensive data extraction methods enabled detailed analyses of various study characteristics, including occupation type, professional experience, and specific workload types, illustrating the diverse approaches to using fNIRS across different work environments. A unique strength of this review is its focus on methodological approaches for signal processing with fNIRS, specifically in the context of occupational workload. By systematically examining the signal processing techniques used across studies, this review highlights current practices and identifies areas for methodological improvement. This emphasis on signal processing is crucial, as it directly impacts the quality and reliability of fNIRS data. Furthermore, the review underscores the need for standardized protocols and methodological consistency to improve the comparability and reproducibility of fNIRS studies in occupational health research.
5 Conclusion
This systematic review demonstrates that fNIRS is an effective tool for assessing occupational workload across various professions. The consistent observation of increased HbO concentrations in the PFC during work-related tasks highlights fNIRS’s capability to detect neural correlates of cognitive demands in real-world settings. In safety-critical and cognitively demanding occupations, such as aviation and maritime navigation, fNIRS has provided valuable insights into the neural mechanisms underlying task performance and workload management. The application of fNIRS in typical office environments remains limited, despite a large portion of employment inherent in office work. The existing studies indicate that even routine office tasks can impose substantial cognitive load, leading to increased PFC activation. Expanding fNIRS research into these settings is crucial for understanding and mitigating cognitive fatigue and neural overload among office workers. This could inform the development of evidence-based interventions aimed at enhancing employee well-being and productivity. To advance the field, methodological improvements are necessary. Standardizing optode placement, employing consistent signal processing techniques, and increasing sample sizes will enhance the validity and comparability of findings. Incorporating randomized controlled trials will further strengthen the evidence base, enabling robust conclusions about fNIRS’s effectiveness in different occupational contexts.
In summary, fNIRS holds significant promise as a non-invasive method for evaluating occupational workload. By bridging neuroscience and occupational health promotion, it can contribute to strategies that promote employee well-being across diverse work environments.
Data availability statement
The original contributions presented in the study are included in the article/Supplementary material, further inquiries can be directed to the corresponding author.
Author contributions
RG: Conceptualization, Formal analysis, Investigation, Methodology, Visualization, Writing – original draft, Writing – review & editing, Data curation, Project administration, Validation. OM: Investigation, Methodology, Writing – review & editing. AS: Conceptualization, Supervision, Writing – review & editing, Methodology, Project administration.
Funding
The author(s) declare that no financial support was received for the research and/or publication of this article.
Conflict of interest
OM was employed by Agito Gesundheit GmbH.
The remaining authors declare that the research was conducted in the absence of any commercial or financial relationships that could be construed as a potential conflict of interest.
Generative AI statement
The authors declare that no Gen AI was used in the creation of this manuscript.
Publisher’s note
All claims expressed in this article are solely those of the authors and do not necessarily represent those of their affiliated organizations, or those of the publisher, the editors and the reviewers. Any product that may be evaluated in this article, or claim that may be made by its manufacturer, is not guaranteed or endorsed by the publisher.
Supplementary material
The Supplementary material for this article can be found online at: https://www.frontiersin.org/articles/10.3389/fpubh.2025.1560605/full#supplementary-material
References
1. Quick, JC, and Henderson, DF. Occupational stress: preventing suffering, enhancing wellbeing. Int J Environ Res Public Health. (2016) 13:459. doi: 10.3390/ijerph13050459
2. Paas, F, Renkl, A, and Sweller, J. Cognitive load theory and instructional design: recent developments. Educ Psychol. (2003) 38:1–4. doi: 10.1207/S15326985EP3801_1
3. Sweller, J. Cognitive load during problem solving: effects on learning. Cogn Sci. (1988) 12:257–85. doi: 10.1207/s15516709cog1202_4
4. Baddeley, A. The episodic buffer: a new component of working memory? Trends Cogn Sci. (2000) 4:417–23. doi: 10.1016/S1364-6613(00)01538-2
5. Sharples, S Workload II: A future paradigm for analysis and measurement. In: Proceedings of the 20th congress of the international ergonomics association (IEA 2018): Volume VII: Ergonomics in design, Design for all, activity theories for work analysis and design, affective design. Cham: Springer International Publishing; (2019). p. 489–498.
6. Wickens, CD. Multiple resources and mental workload. Hum Factors. (2008) 50:449–55. doi: 10.1518/001872008X288394
7. Karasek, RA. Job demands, job decision latitude, and mental strain: implications for job redesign. Adm Sci Q. (1979) 24:285. doi: 10.2307/2392498
8. Siegrist, J. Adverse health effects of high-effort/low-reward conditions. J Occup Health Psychol. (1996) 1:27–41. doi: 10.1037/1076-8998.1.1.27
9. Longo, L, Wickens, CD, Hancock, G, and Hancock, PA. Human mental workload: a survey and a novel inclusive definition. Front Psychol. (2022) 13:883321. doi: 10.3389/fpsyg.2022.883321
10. Lean, Y, and Shan, F. Brief review on physiological and biochemical evaluations of human mental workload. Hum Ftrs Erg Mfg Svc. (2012) 22:177–87. doi: 10.1002/hfm.20269
11. Rump, J, and Eilers, S. Auf dem Weg zur Arbeit 4.0. Springer Berlin Heidelberg: Berlin, Heidelberg (2017).
12. Körner, U, Müller-Thur, K, Lunau, T, Dragano, N, Angerer, P, and Buchner, A. Perceived stress in human-machine interaction in modern manufacturing environments-results of a qualitative interview study. Stress Health. (2019) 35:187–99. doi: 10.1002/smi.2853
13. Du, Y, Zhang, L, and Tekleab, AG. Job strains, job control, and POS on employee performance: an interactionist perspective. J Bus Res. (2018) 82:213–9. doi: 10.1016/j.jbusres.2017.09.040
14. Meyer, S-C, and Hünefeld, L. Challenging cognitive demands at work, related working conditions, and employee well-being. Int J Environ Res Public Health. (2018) 15:2911. doi: 10.3390/ijerph15122911
15. Salvagioni, DAJ, Melanda, FN, Mesas, AE, González, AD, Gabani, FL, and De, ASM. Physical, psychological and occupational consequences of job burnout: a systematic review of prospective studies. PLoS One. (2017) 12:e0185781. doi: 10.1371/journal.pone.0185781
16. Cardás, T. International Comparison of Costs of Work-Related Accidents and Illnesses. European Agency for Safety and Health at Work, Spain. (2017) Available at: https://coilink.org/20.500.12592/b1mm99
17. Centers for Disease Control and Prevention. Workplace health promotion: Using the workplace to improve the nation’s health: At a glance 2016. Atlanta, GA: Centers for Disease Control and Prevention (CDC). (2020).
18. Pega, F, Momen, NC, Ujita, Y, Driscoll, T, and Whaley, P. Systematic reviews and meta-analyses for the WHO/ILO joint estimates of the work-related burden of disease and injury. Environ Int. (2021) 155:106605. doi: 10.1016/j.envint.2021.106605
19. Tompa, E, Mofidi, A, van den Heuvel, S, van Bree, T, Michaelsen, F, Jung, Y, et al. Economic burden of work injuries and diseases: a framework and application in five European Union countries. BMC Public Health. (2021) 21:49. doi: 10.1186/s12889-020-10050-7
20. Hart, SG, and Staveland, LE. Development of NASA-TLX (task load index): results of empirical and theoretical research In: PA Hancock and N Meshkati, editors. Human mental workload. New York, NY: Elsevier Science Pub. Co (1988). 139–83.
21. Tao, D, Tan, H, Wang, H, Zhang, X, Qu, X, and Zhang, T. A systematic review of physiological measures of mental workload. Int J Environ Res Public Health. (2019) 16:716. doi: 10.3390/ijerph16152716
22. Causse, M, Lepron, E, Mandrick, K, Peysakhovich, V, Berry, I, Callan, D, et al. Facing successfully high mental workload and stressors: an fMRI study. Hum Brain Mapp. (2022) 43:1011–31. doi: 10.1002/hbm.25703
23. Charles, RL, and Nixon, J. Measuring mental workload using physiological measures: a systematic review. Appl Ergon. (2019) 74:221–32. doi: 10.1016/j.apergo.2018.08.028
24. Aghajani, H, Garbey, M, and Omurtag, A. Measuring mental workload with EEG+fNIRS. Front Hum Neurosci. (2017) 11:359. doi: 10.3389/fnhum.2017.00359
25. Young, MS, Brookhuis, KA, Wickens, CD, and Hancock, PA. State of science: mental workload in ergonomics. Ergonomics. (2015) 58:1–17. doi: 10.1080/00140139.2014.956151
26. Loosen, AM, Kato, A, and Gu, X. Revisiting the role of computational neuroimaging in the era of integrative neuroscience. Neuropsychopharmacology. 50:103–13. doi: 10.1038/s41386-024-01946-8
27. Friedman, NP, and Robbins, TW. The role of prefrontal cortex in cognitive control and executive function. Neuropsychopharmacology. (2022) 47:72–89. doi: 10.1038/s41386-021-01132-0
28. Hopfinger, JB, and Slotnick, SD. Attentional control and executive function. Cogn Neurosci. (2020) 11:1–4. doi: 10.1080/17588928.2019.1682985
29. Bernadine, C, Nanda, N, Vijayalakshmi, R, Thilaga, M, Naga, D, and Nabaraj, D. Breaking the Camel's Back: can cognitive overload be quantified in the human brain? Procedia Soc Behav Sci. (2013) 97:21–9. doi: 10.1016/j.sbspro.2013.10.200
30. Chikhi, S, Matton, N, and Blanchet, S. EEG power spectral measures of cognitive workload: a meta-analysis. Psychophysiology. (2022) 59:e14009. doi: 10.1111/psyp.14009
31. Panikratova, YR, Vlasova, RM, Akhutina, TV, Korneev, AA, Sinitsyn, VE, and Pechenkova, EV. Functional connectivity of the dorsolateral prefrontal cortex contributes to different components of executive functions. Int J Psychophysiol. (2020) 151:70–9. doi: 10.1016/j.ijpsycho.2020.02.013
32. Duncan, J. The structure of cognition: attentional episodes in mind and brain. Neuron. (2013) 80:35–50. doi: 10.1016/j.neuron.2013.09.015
33. Brosnan, MB, and Wiegand, I. The dorsolateral prefrontal cortex, a dynamic cortical area to enhance top-down attentional control. J Neurosci. (2017) 37:3445–6. doi: 10.1523/JNEUROSCI.0136-17.2017
34. Khammissa, RAG, Nemutandani, S, Feller, G, Lemmer, J, and Feller, L. Burnout phenomenon: neurophysiological factors, clinical features, and aspects of management. J Int Med Res. (2022) 50:3000605221106428. doi: 10.1177/03000605221106428
35. Cunningham, TR, Guerin, RJ, Ferguson, J, and Cavallari, J. Work-related fatigue: a hazard for workers experiencing disproportionate occupational risks. Am J Ind Med. (2022) 65:913–25. doi: 10.1002/ajim.23325
36. Causse, M, Chua, Z, Peysakhovich, V, Del Campo, N, and Matton, N. Mental workload and neural efficiency quantified in the prefrontal cortex using fNIRS. Sci Rep. (2017) 7:5222. doi: 10.1038/s41598-017-05378-x
37. Oliveira, AS, Schlink, BR, Hairston, WD, König, P, and Ferris, DP. A channel rejection method for attenuating motion-related artifacts in EEG recordings during walking. Front Neurosci. (2017) 11:225. doi: 10.3389/fnins.2017.00225
38. Urigüen, JA, and Garcia-Zapirain, B. EEG artifact removal-state-of-the-art and guidelines. J Neural Eng. (2015) 12:31001. doi: 10.1088/1741-2560/12/3/031001
39. Mehta, RK, and Parasuraman, R. Neuroergonomics: a review of applications to physical and cognitive work. Front Hum Neurosci. (2013) 7:889. doi: 10.3389/fnhum.2013.00889
40. Verdière, KJ, Roy, RN, and Dehais, F. Detecting Pilot's engagement using fNIRS connectivity features in an automated vs manual landing scenario. Front Hum Neurosci. (2018) 12:6. doi: 10.3389/fnhum.2018.00006
41. Le, AS, Aoki, H, Murase, F, and Ishida, K. A novel method for classifying driver mental workload under naturalistic conditions with information from near-infrared spectroscopy. Front Hum Neurosci. (2018) 12:431. doi: 10.3389/fnhum.2018.00431
42. Zheng, L, Liu, W, Long, Y, Zhai, Y, Zhao, H, Bai, X, et al. Affiliative bonding between teachers and students through interpersonal synchronisation in brain activity. Soc Cogn Affect Neurosci. (2020) 15:97–109. doi: 10.1093/scan/nsaa016
43. Tian, F, Li, H, Tian, S, Shao, J, and Tian, C. Effect of shift work on cognitive function in Chinese coal mine workers: a resting-state fNIRS study. Int J Environ Res Public Health. (2022) 19:4217. doi: 10.3390/ijerph19074217
44. Helmich, I, Chang, YY, Gemmerich, R, Rodrigo, L, Funken, J, Arun, KM, et al. Neurobehavioral consequences of repetitive head impacts in Para swimming: a case report. J Sci Med Sport. (2024) 27:16–9. doi: 10.1016/j.jsams.2023.10.015
45. Palzes, VA, Sagiv, SK, Baker, JM, Rojas-Valverde, D, Gutiérrez-Vargas, R, Winkler, MS, et al. Manganese exposure and working memory-related brain activity in smallholder farmworkers in Costa Rica: results from a pilot study. Environ Res. (2019) 173:539–48. doi: 10.1016/j.envres.2019.04.006
46. Jöbsis, FF. Noninvasive, infrared monitoring of cerebral and myocardial oxygen sufficiency and circulatory parameters. Science. (1977) 198:1264–7. doi: 10.1126/science.929199
47. Pinti, P, Tachtsidis, I, Hamilton, A, Hirsch, J, Aichelburg, C, Gilbert, S, et al. The present and future use of functional near-infrared spectroscopy (fNIRS) for cognitive neuroscience. Ann N Y Acad Sci. (2020) 1464:5–29. doi: 10.1111/nyas.13948
48. Cui, X, Bray, S, Bryant, DM, Glover, GH, and Reiss, AL. A quantitative comparison of NIRS and fMRI across multiple cognitive tasks. NeuroImage. (2011) 54:2808–21. doi: 10.1016/j.neuroimage.2010.10.069
49. Quaresima, F. A Mini-review on functional near-infrared spectroscopy (fNIRS): where do we stand, and where should we go? Photo Dermatol. (2019) 6:87. doi: 10.3390/photonics6030087
50. Li, L-P, Liu, Z-G, Zhu, H-Y, Zhu, L, and Huang, Y-C. Functional near-infrared spectroscopy in the evaluation of urban rail transit drivers' mental workload under simulated driving conditions. Ergonomics. (2019) 62:406–19. doi: 10.1080/00140139.2018.1535093
51. Midha, S, Maior, HA, Wilson, ML, and Sharples, S. Measuring mental workload variations in office work tasks using fNIRS. Int J Hum Comput Stud. (2021) 147:102580. doi: 10.1016/j.ijhcs.2020.102580
52. Peters, MDJ, Marnie, C, Tricco, AC, Pollock, D, Munn, Z, Alexander, L, et al. Updated methodological guidance for the conduct of scoping reviews. JBI Evid Synth. (2020) 18:2119–26. doi: 10.11124/JBIES-20-00167
53. Tricco, AC, Lillie, E, Zarin, W, O'Brien, KK, Colquhoun, H, Levac, D, et al. PRISMA extension for scoping reviews (PRISMA-ScR): checklist and explanation. Ann Intern Med. (2018) 169:467–73. doi: 10.7326/M18-0850
54. Methley, AM, Campbell, S, Chew-Graham, C, McNally, R, and Cheraghi-Sohi, S. PICO, PICOS and SPIDER: a comparison study of specificity and sensitivity in three search tools for qualitative systematic reviews. BMC Health Serv Res. (2014) 14:579. doi: 10.1186/s12913-014-0579-0
55. Eriksen, MB, and Frandsen, TF. The impact of patient, intervention, comparison, outcome (PICO) as a search strategy tool on literature search quality: a systematic review. J Med Libr Assoc. (2018) 106:420–31. doi: 10.5195/jmla.2018.345
56. Ouzzani, M, Hammady, H, Fedorowicz, Z, and Elmagarmid, A. Rayyan-a web and mobile app for systematic reviews. Syst Rev. (2016) 5:210. doi: 10.1186/s13643-016-0384-4
57. Kohl, SH, Mehler, DMA, Lührs, M, Thibault, RT, Konrad, K, and Sorger, B. The potential of functional near-infrared spectroscopy-based neurofeedback-a systematic review and recommendations for best practice. Front Neurosci. (2020) 14:594. doi: 10.3389/fnins.2020.00594
58. Barker, TH, Habibi, N, Aromataris, E, Stone, JC, Leonardi-Bee, J, Sears, K, et al. The revised JBI critical appraisal tool for the assessment of risk of bias for quasi-experimental studies. JBI Evid Synth. (2024) 22:378–88. doi: 10.11124/JBIES-23-00268
59. Barker, SJC, Sears, K, Klugar, M, Leonardi-Bee, J, Tufanaru, C, Aromataris, E, et al. Revising the JBI quantitative critical appraisal tools to improve their applicability: an overview of methods and the development process. JBI Evid Synth. (2023) 21:478–93. doi: 10.11124/JBIES-22-00125
60. Abujelala, M, Karthikeyan, R, Tyagi, O, Du, J, and Mehta, RK. Brain activity-based metrics for assessing learning states in VR under stress among firefighters: an explorative machine learning approach in Neuroergonomics. Brain Sci. (2021):11. doi: 10.3390/brainsci11070885
61. Tang, L, Si, J, Sun, L, Mao, G, and Yu, S. Assessment of the mental workload of trainee pilots of remotely operated aircraft using functional near-infrared spectroscopy. BMC Neurol. (2022) 22:160. doi: 10.1186/s12883-022-02683-5
62. Tyagi, O, Hopko, S, Kang, J, Shi, Y, Du, J, and Mehta, RK. Modeling brain dynamics during virtual reality-based emergency response learning under stress. Hum Factors. (2021) 65:1804–20. doi: 10.1177/00187208211054894
63. Fan, S, Fairclough, S, Khalique, A, Bury, A, and Yang, Z. Towards advanced decision-making support for shipping safety: a functional connectivity analysis. Transp Res Part E. (2025) 193:103861. doi: 10.1016/j.tre.2024.103861
64. Kawaguchi, K, Nikai, Y, Yomota, S, Kawashima, A, Inoue, Y, and Takahashi, M. Effects of age and flight experience on prefrontal cortex activity in airline pilots: an fNIRS study. Heliyon. (2024) 10:e30242. doi: 10.1016/j.heliyon.2024.e30242
65. Modi, SH, Fiorentino, F, Orihuela-Espina, F, Athanasiou, T, Yang, G-Z, Darzi, A, et al. Association of Residents' neural signatures with stress resilience during surgery. JAMA Surg. (2019) 154:e192552. doi: 10.1001/jamasurg.2019.2552
66. Mora, AM, Baker, JM, Hyland, C, Rodríguez-Zamora, MG, Rojas-Valverde, D, Winkler, MS, et al. Pesticide exposure and cortical brain activation among farmworkers in Costa Rica. Neurotoxicology. (2022) 93:200–10. doi: 10.1016/j.neuro.2022.10.004
67. Singh, A, Modi, HN, Ranjan, S, Dilley, JWR, Airantzis, D, Yang, G-Z, et al. Robotic surgery improves technical performance and enhances prefrontal activation during high temporal demand. Ann Biomed Eng. (2018) 46:1621–36. doi: 10.1007/s10439-018-2049-z
68. Modi, HN, Singh, H, Orihuela-Espina, F, Athanasiou, T, Fiorentino, F, Yang, G-Z, et al. Temporal stress in the operating room: brain engagement promotes "coping" and disengagement prompts "choking". Ann Surg. (2018) 267:683–91. doi: 10.1097/SLA.0000000000002289
69. Fan, S, Blanco-Davis, E, Zhang, J, Bury, A, Warren, J, Yang, Z, et al. The role of the prefrontal cortex and functional connectivity during maritime operations: an fNIRS study. Brain Behav. (2021) 11:e01910. doi: 10.1002/brb3.1910
70. Fan, S, and Yang, Z. Towards objective human performance measurement for maritime safety: a new psychophysiological data-driven machine learning method. Reliab Eng Syst Safety. (2023) 233:109103. doi: 10.1016/j.ress.2023.109103
71. Aksoy, ME, Izzetoglu, K, Utkan, NZ, Agrali, A, Yoner, SI, Bishop, A, et al. Comparing behavioral and neural activity changes during laparoscopic and robotic surgery trainings. J Surg Educ. (2025) 82:103486. doi: 10.1016/j.jsurg.2025.103486
72. Liu, Q, Cui, H, Yuan, P, Liu, J, Jin, Y, Xia, L, et al. Distinction of mental health between salesman and R&D in high-tech enterprise: a fNIRS study. Sci Rep. (2024) 14:22371. doi: 10.1038/s41598-024-74216-8
73. Sun, B, Xiao, W, Feng, X, Shao, Y, Zhang, W, and Li, W. Behavioral and brain synchronization differences between expert and novice teachers when collaborating with students. Brain Cogn. (2020) 139:105513. doi: 10.1016/j.bandc.2019.105513
74. Harrison, J, Izzetoglu, K, Ayaz, H, Willems, B, Hah, S, Ahlstrom, U, et al. Cognitive workload and learning assessment during the implementation of a next-generation air traffic control technology using functional near-infrared spectroscopy. IEEE Trans Human Mach Syst. (2014) 44:429–40. doi: 10.1109/THMS.2014.2319822
75. Andreu-Perez, J, Leff, DR, Shetty, K, Darzi, A, and Yang, G-Z. Disparity in frontal lobe connectivity on a complex bimanual motor task aids in classification of operator skill level. Brain Connect. (2016) 6:375–88. doi: 10.1089/brain.2015.0350
76. Alyan, E, Saad, NM, and Kamel, N. Effects of workstation type on mental stress: FNIRS study. Hum Factors. (2021) 63:1230–55. doi: 10.1177/0018720820913173
77. Chong, JS, Chan, YL, Ebenezer, EGM, Chen, HY, Kiguchi, M, Lu, C-K, et al. fNIRS-based functional connectivity estimation using semi-metric analysis to study decision making by nursing students and registered nurses. Sci Rep. (2020) 10:22041. doi: 10.1038/s41598-020-79053-z
78. Tian, F, Li, H, Tian, S, Tian, C, and Shao, J. Is there a difference in brain functional connectivity between Chinese coal mine workers who have engaged in unsafe behavior and those who have not? Int J Environ Res Public Health. (2019):19. doi: 10.3390/ijerph19010509
79. Menda, J, Hing, JT, Ayaz, H, Shewokis, PA, Izzetoglu, K, Onaral, B, et al. Optical brain imaging to enhance UAV operator training, evaluation, and Interface development. J Intell Robot Syst. (2011) 61:423–43. doi: 10.1007/s10846-010-9507-7
80. Ayaz, H, Shewokis, PA, Bunce, S, Izzetoglu, K, Willems, B, and Onaral, B. Optical brain monitoring for operator training and mental workload assessment. NeuroImage. (2012) 59:36–47. doi: 10.1016/j.neuroimage.2011.06.023
81. Isbilir, E, Çakır, MP, Acartürk, C, and Tekerek, AŞ. Towards a multimodal model of cognitive workload through synchronous optical brain imaging and eye tracking measures. Front Hum Neurosci. (2019) 13:375. doi: 10.3389/fnhum.2019.00375
82. Xu, J, Slagle, JM, Banerjee, A, Bracken, B, and Weinger, MB. Use of a portable functional near-infrared spectroscopy (fNIRS) system to examine team experience during crisis event Management in Clinical Simulations. Front Hum Neurosci. (2019) 13:85. doi: 10.3389/fnhum.2019.00085
83. Ahlstrom, U. Weather display symbology affects pilot behavior and decision-making. Int J Ind Ergon. (2015) 50:73–96. doi: 10.1016/j.ergon.2015.09.008
84. Alyan, E, Saad, NM, Kamel, N, and Rahman, MA. Workplace design-related stress effects on prefrontal cortex connectivity and neurovascular coupling. Appl Ergon. (2021) 96:103497. doi: 10.1016/j.apergo.2021.103497
85. Tian, C, Li, H, Tian, S, Tian, F, and Yang, H. The neurocognitive mechanism linking temperature and humidity with miners' working memory: an fNIRS study. Front Hum Neurosci. (2024) 18:1414679. doi: 10.3389/fnhum.2024.1414679
86. Kakehashi, E, Choi, JS, Takekawa, S, Akira, S, Kimura, M, and Hibino, H. Physiological and psychological effects of OLED lighting location on office work efficiency. IJAE. (2018) 17:99–107. doi: 10.5057/ijae.IJAE-D-17-00019
87. Zheng, L, Chen, C, Liu, W, Long, Y, Zhao, H, Bai, X, et al. Enhancement of teaching outcome through neural prediction of the students' knowledge state. Hum Brain Mapp. (2018) 39:3046–57. doi: 10.1002/hbm.24059
88. Pooladvand, S, and Hasanzadeh, S. Neurophysiological evaluation of workers' decision dynamics under time pressure and increased mental demand. Autom Constr. (2022) 141:104437. doi: 10.1016/j.autcon.2022.104437
89. Pooladvand, S, Chang, W-C, and Hasanzadeh, S. Identifying at-risk workers using fNIRS-based mental load classification: a mixed reality study. Autom Constr. (2024) 164:105453. doi: 10.1016/j.autcon.2024.105453
90. Li, W, Wu, S, Xie, X, Yang, W, Feng, L, Yang, Z, et al. Effect of mindfulness training on skill performance in simulator-based knee arthroscopy training for novice residents-a randomized controlled study. J Surg Educ. (2024) 81:103306. doi: 10.1016/j.jsurg.2024.103306
91. Naik, R, Rubio-Solis, A, Jin, K, and Mylonas, G. Novel multimodal sensing and machine learning strategies to classify cognitive workload in laparoscopic surgery. Eur J Surg Oncol. (2024):108735. doi: 10.1016/j.ejso.2024.108735
92. Li, NKK, Fan, Z, Yuan, X, Liu, H, and Bu, L. A human-centred approach based on functional near-infrared spectroscopy for adaptive decision-making in the air traffic control environment: a case study. Adv Eng Inform. (2021) 49:101325. doi: 10.1016/j.aei.2021.101325
93. Zhang, C, Yuan, J, Jiao, Y, Liu, H, Fu, L, Jiang, C, et al. Variation of pilots’ mental workload under emergency flight conditions induced by different equipment failures: a flight simulator study. Trans Res Rec. (2023) 2678:365–77. doi: 10.1177/03611981231184188
94. Jasper, HH. Report of the committee on methods of clinical examination in electroencephalography. Electroencephalogr Clin Neurophysiol. (1958) 10:370–5. doi: 10.1016/0013-4694(58)90053-1
95. Okamoto, M, Dan, H, Sakamoto, K, Takeo, K, Shimizu, K, Kohno, S, et al. Three-dimensional probabilistic anatomical cranio-cerebral correlation via the international 10-20 system oriented for transcranial functional brain mapping. NeuroImage. (2004) 21:99–111. doi: 10.1016/j.neuroimage.2003.08.026
96. Singh, OM, Dan, H, Jurcak, V, and Dan, I. Spatial registration of multichannel multi-subject fNIRS data to MNI space without MRI. NeuroImage. (2005) 27:842–51. doi: 10.1016/j.neuroimage.2005.05.019
97. Tsuzuki, D, Jurcak, V, Singh, AK, Okamoto, M, Watanabe, E, and Dan, I. Virtual spatial registration of stand-alone fNIRS data to MNI space. NeuroImage. (2007) 34:1506–18. doi: 10.1016/j.neuroimage.2006.10.043
98. Kamran, MA, Mannan, MMN, and Jeong, MY. Cortical signal analysis and advances in functional near-infrared spectroscopy signal: a review. Front Hum Neurosci. (2016) 10:261. doi: 10.3389/fnhum.2016.00261
99. Luke, R, Shader, MJ, and McAlpine, D. Characterization of Mayer-wave oscillations in functional near-infrared spectroscopy using a physiologically informed model of the neural power spectra. Neurophotonics. (2021) 8:41001. doi: 10.1117/1.NPh.8.4.041001
100. Ghouse, A, Candia-Rivera, D, and Valenza, G. Nonlinear neural patterns are revealed in high frequency functional near infrared spectroscopy analysis. Brain Res Bull. (2023) 203:110759. doi: 10.1016/j.brainresbull.2023.110759
101. Huang, R, Hong, K-S, Yang, D, and Huang, G. Motion artifacts removal and evaluation techniques for functional near-infrared spectroscopy signals: a review. Front Neurosci. (2022) 16:878750. doi: 10.3389/fnins.2022.878750
102. Miller, EK, and Cohen, JD. An integrative theory of prefrontal cortex function. Annu Rev Neurosci. (2001) 24:167–202. doi: 10.1146/annurev.neuro.24.1.167
103. Qi, P, Ru, H, Gao, L, Zhang, X, Zhou, T, Tian, Y, et al. Neural mechanisms of mental fatigue revisited: new insights from the brain connectome. Engineering. (2019) 5:276–86. doi: 10.1016/j.eng.2018.11.025
104. Ishii, A, Tanaka, M, and Watanabe, Y. Neural mechanisms of mental fatigue. Rev Neurosci. (2014) 25:469–79. doi: 10.1515/revneuro-2014-0028
105. Herff, C, Heger, D, Fortmann, O, Hennrich, J, Putze, F, and Schultz, T. Mental workload during n-back task-quantified in the prefrontal cortex using fNIRS. Front Hum Neurosci. (2013) 7:935. doi: 10.3389/fnhum.2013.00935
106. Causse, M, Mouratille, D, Rouillard, Y, El Yagoubi, R, Matton, N, and Hidalgo-Muñoz, A. How a pilot's brain copes with stress and mental load? Insights from the executive control network. Behav Brain Res. (2024) 456:114698. doi: 10.1016/j.bbr.2023.114698
107. Biswal, BB, Mennes, M, Zuo, X-N, Gohel, S, Kelly, C, Smith, SM, et al. Toward discovery science of human brain function. Proc Natl Acad Sci USA. (2010) 107:4734–9. doi: 10.1073/pnas.0911855107
108. Ghasimi, A, and Shamekhi, S. Assessment of cognitive workload using simultaneous EEG and fNIRS: a comparison of feature combinations. Comput Electr Eng. (2024) 119:109619. doi: 10.1016/j.compeleceng.2024.109619
109. Lim, J, Wu, W-C, Wang, J, Detre, JA, Dinges, DF, and Rao, H. Imaging brain fatigue from sustained mental workload: an ASL perfusion study of the time-on-task effect. NeuroImage. (2010) 49:3426–35. doi: 10.1016/j.neuroimage.2009.11.020
110. Stajkovic, AD, and Stajkovic, KS. Human sustainability and cognitive overload at work. New York: Routledge (2024).
111. Biondi, FN, Cacanindin, A, Douglas, C, and Cort, J. Overloaded and at work: investigating the effect of cognitive workload on assembly task performance. Hum Fact. (2021) 63:813–20. doi: 10.1177/0018720820929928
112. Tsuzuki, D, and Dan, I. Spatial registration for functional near-infrared spectroscopy: from channel position on the scalp to cortical location in individual and group analyses. NeuroImage. (2014) 1:92–103. doi: 10.1016/j.neuroimage.2013.07.025
113. Jurcak, V, Tsuzuki, D, and Dan, I. 10/20, 10/10, and 10/5 systems revisited: their validity as relative head-surface-based positioning systems. NeuroImage. (2007) 34:1600–11. doi: 10.1016/j.neuroimage.2006.09.024
114. Gallego Martínez, EE, González Mitjans, A, Garea-Llano, E, Bringas-Vega, ML, and Valdes-Sosa, PA. Automatic detection of fiducial landmarks toward the development of an application for digitizing the locations of EEG electrodes: occipital structure sensor-based work. Front Neurosci. (2021) 15:526257. doi: 10.3389/fnins.2021.526257
115. Machado, A, Cai, Z, Pellegrino, G, Marcotte, O, Vincent, T, Lina, J-M, et al. Optimal positioning of optodes on the scalp for personalized functional near-infrared spectroscopy investigations. J Neurosci Methods. (2018) 309:91–108. doi: 10.1016/j.jneumeth.2018.08.006
116. Yücel, MA, Lühmann, AV, Scholkmann, F, Gervain, J, Dan, I, Ayaz, H, et al. Best practices for fNIRS publications. Neurophotonics. (2021) 8:12101. doi: 10.1117/1.NPh.8.1.012101
117. Menant, JC, Maidan, I, Alcock, L, Al-Yahya, E, Cerasa, A, and Clark, DJ, Bruin ED de, Fraser, S, Gramigna, V, Hamacher, D, et al. A consensus guide to using functional near-infrared spectroscopy in posture and gait research. Gait Posture (2020); 82:254–265. doi: 10.1016/j.gaitpost.2020.09.012
118. Dohmatob, E, Richard, H, Pinho, AL, and Thirion, B. Brain topography beyond parcellations: local gradients of functional maps. NeuroImage. (2021) 229:117706. doi: 10.1016/j.neuroimage.2020.117706
119. Brett, M, Johnsrude, IS, and Owen, AM. The problem of functional localization in the human brain. Nat Rev Neurosci. (2002) 3:243–9. doi: 10.1038/nrn756
120. Benitez-Andonegui, A, Lührs, M, Nagels-Coune, L, Ivanov, D, Goebel, R, and Sorger, B. Guiding functional near-infrared spectroscopy optode-layout design using individual (f)MRI data: effects on signal strength. Neurophotonics. (2021) 8:25012. doi: 10.1117/1.NPh.8.2.025012
121. Bonilauri, A, Pirastru, A, Sangiuliano Intra, F, Isernia, S, Cazzoli, M, Blasi, V, et al. Surface-based integration approach for fNIRS-fMRI reliability assessment. J Neurosci Methods. (2023) 398:109952. doi: 10.1016/j.jneumeth.2023.109952
122. Boas, DA, Elwell, CE, Ferrari, M, and Taga, G. Twenty years of functional near-infrared spectroscopy: introduction for the special issue. NeuroImage. (2014) 85:1–5. doi: 10.1016/j.neuroimage.2013.11.033
123. Yücel, MA, Selb, J, Aasted, CM, Lin, P-Y, Borsook, D, Becerra, L, et al. Mayer waves reduce the accuracy of estimated hemodynamic response functions in functional near-infrared spectroscopy. Biomed Opt Express. (2016) 7:3078–88. doi: 10.1364/BOE.7.003078
124. Pfeifer, MD, Scholkmann, F, and Labruyère, R. Signal processing in functional near-infrared spectroscopy (fNIRS): methodological differences Lead to different statistical results. Front Hum Neurosci. (2017) 11:641. doi: 10.3389/fnhum.2017.00641
125. Murray, NP, and Russoniello, C. Acute physical activity on cognitive function: a heart rate variability examination. Appl Psychophysiol Biofeedback. (2012) 37:219–27. doi: 10.1007/s10484-012-9196-z
126. Brigadoi, S, and Cooper, RJ. How short is short? Optimum source-detector distance for short-separation channels in functional near-infrared spectroscopy. Neurophotonics. (2015) 2:25005. doi: 10.1117/1.NPh.2.2.025005
127. Tachtsidis, I, and Scholkmann, F. False positives and false negatives in functional near-infrared spectroscopy: issues, challenges, and the way forward. Neurophotonics. (2016) 3:31405. doi: 10.1117/1.NPh.3.3.031405
128. Von Lühmann, A, Ortega-Martinez, A, Boas, DA, and Yücel, MA. Using the general linear model to improve performance in fNIRS single trial analysis and classification: a perspective. Front Hum Neurosci. (2020) 14:30. doi: 10.3389/fnhum.2020.00030
129. Klein, F, and Kranczioch, C. Signal processing in fNIRS: a case for the removal of systemic activity for single trial data. Front Hum Neurosci. (2019) 13:331. doi: 10.3389/fnhum.2019.00331
130. Nambu, I, Imai, T, Saito, S, Sato, T, and Wada, Y. Detecting motor learning-related fNIRS activity by applying removal of systemic interferences. IEICE Trans Inf Syst. (2017) E100:242–5. doi: 10.1587/transinf.2016EDL8132
131. Pinti, P, Aichelburg, C, Gilbert, S, Hamilton, A, Hirsch, J, Burgess, P, et al. A review on the use of wearable functional near-infrared spectroscopy in naturalistic environments. Jpn Psychol Res. (2018) 60:347–73. doi: 10.1111/jpr.12206
132. Faul, F, Erdfelder, E, Lang, A-G, and Buchner, A. G*power 3: a flexible statistical power analysis program for the social, behavioral, and biomedical sciences. Behav Res Methods. (2007) 39:175–91. doi: 10.3758/bf03193146
133. Button, KS, Ioannidis, JPA, Mokrysz, C, Nosek, BA, Flint, J, Robinson, ESJ, et al. Power failure: why small sample size undermines the reliability of neuroscience. Nat Rev Neurosci. (2013) 14:365–76. doi: 10.1038/nrn3475
134. Poldrack, RA, Baker, CI, Durnez, J, Gorgolewski, KJ, Matthews, PM, Munafò, MR, et al. Scanning the horizon: towards transparent and reproducible neuroimaging research. Nat Rev Neurosci. (2017) 18:115–26. doi: 10.1038/nrn.2016.167
135. Algermissen, J, and Mehler, DMA. May the power be with you: are there highly powered studies in neuroscience, and how can we get more of them? J Neurophysiol. (2018) 119:2114–7. doi: 10.1152/jn.00765.2017
136. Mehler, D. The replication challenge In: A Raz and RT Thibault, editors. Casting light on the dark side of brain imaging. London: Academic Press, an imprint of Elsevier (2019). 67–71.
137. Schroeder, PA, Artemenko, C, Kosie, JE, Cockx, H, Stute, K, Pereira, J, et al. Using preregistration as a tool for transparent fNIRS study design. Neurophotonics. (2023) 10:23515. doi: 10.1117/1.NPh.10.2.023515
138. Lakens, D, Scheel, AM, and Isager, PM. Equivalence testing for psychological research: a tutorial. Adv Methods Pract Psychol Sci. (2018) 1:259–69. doi: 10.1177/2515245918770963
139. Lakens, D. Sample size justification. Collabra Psychol. (2022) 8:33267. doi: 10.1525/collabra.33267
140. Andrade, C. The limitations of quasi-experimental studies, and methods for data analysis when a quasi-experimental research design is unavoidable. Indian J Psychol Med. (2021) 43:451–2. doi: 10.1177/02537176211034707
141. Hariton, E, and Locascio, JJ. Randomised controlled trials - the gold standard for effectiveness research: study design: randomised controlled trials. BJOG. (2018) 125:1716. doi: 10.1111/1471-0528.15199
142. Ioannidis, JPA. Why Most clinical research is not useful. PLoS Med. (2016) 13:e1002049. doi: 10.1371/journal.pmed.1002049
143. Chu, H, Cao, Y, Jiang, J, Yang, J, Huang, M, Li, Q., et al. Optimized EEG–fNIRS Based Mental Workload Detection Method for Practical Applications. (2021).
144. Abdelnour, AF, and Huppert, T. Real-time imaging of human brain function by near-infrared spectroscopy using an adaptive general linear model. NeuroImage, (2009) 46, 133–143. doi: 10.1016/j.neuroimage.2009.01.033
145. Ayaz, H. Functional near infrared spectroscopy based brain computer interface. (2010). Available at: https://patents.google.com/patent/us9946344b2/en
146. Hosseini, SMH, Bruno, JL, Baker, JM, Gundran, A, Harbott, LK, Gerdes, JC., et al. Neural, physiological, and behavioral correlates of visuomotor cognitive load. Sci. Rep. (2017) 7, 8866. doi: 10.1038/s41598-017-07897-z
147. Liu, Y, Piazza, EA, Simony, E, Shewokis, PA, Onaral, B, Hasson, U., et al. Measuring speaker-listener neural coupling with functional near infrared spectroscopy. Scientific Reports, (2017) 7, 43293. doi: 10.1038/srep43293
148. Molavi, B, and Dumont, GA. Wavelet-based motion artifact removal for functional near-infrared spectroscopy. Physiol. Meas. (2012) 33, 259–270. doi: 10.1088/0967-3334/33/2/259
149. Nozawa, T, Sasaki, Y, Sakaki, K, Yokoyama, R, and Kawashima, R. Interpersonal frontopolar neural synchronization in group communication: An exploration toward fNIRS hyperscanning of natural interactions. NeuroImage, (2016) 133, 484–497. doi: 10.1016/j.neuroimage.2016.03.059
Keywords: functional near-infrared spectroscopy, occupational workload, reporting, workplace health promotion, neuroimaging
Citation: Gemmerich R, Müller O and Schaller A (2025) The application of fNIRS in studies on occupational workload: a systematic review. Front. Public Health. 13:1560605. doi: 10.3389/fpubh.2025.1560605
Edited by:
Jodi M. Gilman, Massachusetts General Hospital and Harvard Medical School, United StatesReviewed by:
Jesse Mark, Drexel University, United StatesWilliam Algoner, Technological University of Peru, Peru
Copyright © 2025 Gemmerich, Müller and Schaller. This is an open-access article distributed under the terms of the Creative Commons Attribution License (CC BY). The use, distribution or reproduction in other forums is permitted, provided the original author(s) and the copyright owner(s) are credited and that the original publication in this journal is cited, in accordance with accepted academic practice. No use, distribution or reproduction is permitted which does not comply with these terms.
*Correspondence: Robin Gemmerich, cm9iaW4uZ2VtbWVyaWNoQHVuaWJ3LmRl