- Department of Geriatrics, The Second Xiangya Hospital of Central South University, Changsha, Hunan, China
Background: Frailty, characterized by functional decline and disability, is an emerging public health concern in aging populations. Chronic inflammation and low high-density lipoprotein cholesterol (HDL-C) levels are key contributors to the progression of frailty. This study aims to examine the association between the ratio of high-sensitivity C-reactive protein (hs-CRP) to HDL-C and frailty among middle-aged and older adults in the United States.
Methods: Our study included participants aged 45 years and older from the 2015–2020 National Health and Nutrition Examination Survey (NHANES). Logistic regression and restricted cubic spline (RCS) analysis were utilized to assess the relationship between the hs-CRP/HDL-C ratio and frailty, adjusting for potential confounding covariates. Mediation analysis was performed to determine whether plasma proteins mediated this association. Least absolute shrinkage and selection operator (LASSO) regression was employed to identify variables strongly correlated with frailty, and a nomogram was subsequently developed based on these variables.
Results: Our study included 3,626 middle-aged and older participants, among whom 787(21.7%) were identified as frailty. After adjusting for all covariates, a high hs-CRP/HDL-C ratio was identified as a significant risk factor for frailty (OR = 1.736, 95% CI: 1.009–2.988). RCS analysis disclosed a nonlinear correlation between the hs-CRP/HDL-C ratio and frailty incidence. Furthermore, mediation analysis suggested that albumin and globulin partially mediated this association, accounting for 37.82% and 11.23% of the indirect effect, respectively. A nomogram, constructed using variables selected via LASSO regression, exhibited promising discriminative ability, with an area under the curve (AUC) of 79.7% (95% CI: 77.7–81.75%).
Conclusion: Our findings suggest that a higher hs-CRP/HDL-C ratio is associated with an increased risk of frailty among middle-aged and older adults. Albumin and globulin partially mediate this relationship. Additionally, the nomogram developed in our study shows strong predictive ability for identifying individuals at high risk of frailty in this population.
1 Introduction
The global aging population is growing rapidly (1). Frailty, an age-associated clinical syndrome, serves as an important indicator for identifying individuals at high risk of adverse outcomes, including falls, hospitalization, disability, and mortality (2, 3). It is a multifactorial condition influenced by various biological mechanisms such as chronic inflammation, oxidative stress, nutritional status(e.g., albumin and diet), aging, and physical activity (4). The frailty index (FI) is a widely accepted tool for assessing frailty across populations (5). Given the wide range of complications associated with frailty, it is crucial to identify reliable biomarkers to predict and slow the progression of frailty.
Inflammation plays a crucial role in the development of frailty (6, 7). C-reactive protein (CRP), a well-established marker of inflammation, has been linked to reduced muscle mass and strength (8). However, the relationship between CRP and frailty remains inconclusive. In an observational study by Kamil R. J. et al., no significant association was found between CRP levels and frailty risk (9). Conversely, Luo et al. reported elevated CRP levels among individuals with frailty (10). High-sensitivity CRP (hs-CRP) assays, commonly used in clinical practice, enable precise detection of CRP levels and serve as valuable predictors of cardiovascular disease and stroke (11, 12). In addition to inflammatory markers, lipid metabolism also plays a role in frailty. Reduced high-density lipoprotein cholesterol (HDL-C) levels are frequently observed in individuals with chronic conditions (13). HDL-C, known for its anti-inflammatory properties, has been inversely associated with frailty in older adults (14, 15). Both hs-CRP and HDL-C are common biomarkers for assessing inflammation and lipid status in clinical settings. Previous research has established that the hs-CRP/HDL-C ratio may serve as a risk indicator for new stroke and cardiovascular issues (16). However, few studies have examined the relationship between the hs-CRP/HDL-C ratio and frailty in large, population-based cohorts. Our research aims to explore whether the hs-CRP/HDL-C ratio can serve as a useful biomarker for identifying frailty among middle-aged and older adult individuals. To achieve this, we will analyze extensive data from the National Health and Nutrition Examination Survey (NHANES) spanning from 2015 to 2020. This study presents an opportunity to identify a more reliable biomarker for frailty assessment in middle-aged and older populations.
2 Methods
2.1 Data collection and study population
The data for this study were obtained from the 2015–2020 cycles of the NHANES, a publicly available cross-sectional survey designed to evaluate the health and nutritional status of the U.S. population. Because of the COVID-19 pandemic, data obtained from 2019 to March 2020 were combined with data from the 2017–2018 cycle to form a nationally representative pre-pandemic dataset covering the period from 2017 to March 2020. A total of 3,626 participants aged 45 years and older were included in the final analysis. Individuals with incomplete data required to construct the frailty index, or with missing data for hs-CRP, HDL-C, or other selected covariates, were excluded. The final cohort is representative of approximately 7.05 million non-institutionalized residents of the U.S. A flowchart detailing participant recruitment is provided in Figure 1.
2.2 The hs-CRP/HDL-C ratio
During all three cycles of the NHANES, serum samples were obtained for analysis. Serum levels of hs-CRP (mg/L) and HDL-C (mg/dL) were analyzed at the University of Minnesota, Minneapolis, MN. The hs-CRP/HDL-C ratio was determined by dividing the concentration of hs-CRP by the concentration of HDL-C. The hs-CRP/HDL-C ratio was categorized into quartiles (Q1–Q4) based on its distribution.
2.3 Frailty index
Frailty was evaluated using the frailty index (FI), constructed from 49 health deficits as presented in Supplementary Table S1 (17, 18). The health deficits encompassed various aspects such as cognition, dependency, depressive symptoms, comorbidities, hospital utilization and access to care, physical performance, and laboratory values. The FI was computed by dividing the total number of deficits identified in each participant by the overall number of deficits considered. This index yields a score ranging from 0 to 1, with a higher score indicating a higher number of deficits and a frailer condition. In accordance with previous research (19, 20), frailty status was categorized based on the FI score: an FI score of <0.25 denoting non-frailty, and an FI score of ≥0.25 denoting frailty.
2.4 Covariates
Multiple sociodemographic factors including age, gender (male, female), marital status (non-single and single), ethnicity (Non-Hispanic White, Non-Hispanic Black, Mexican American, and Other Race), and educational level (> High school, High school, <High school) were collected as covariates. Additionally, the family income-to-poverty ratio (FITPR) was classified as < 1.3, 1.3–3.5, and > 3.5 based on the household income to poverty guidelines ratio (21). Lifestyle factors such as physical activity and smoking status were included. Physical activity was assessed using metabolic equivalent minutes per week, calculated by multiplying the total time spent in transportation, work, and recreational activity. Physical activity was categorized as <500 and ≥500 metabolic equivalent minutes per week, based on the World Health Organization’s recommended physical activity level (22). Smoking status was classified into two categories: “no” (individuals who smoked fewer than 100 cigarettes in their lifetime, or previously smoked more than 100 cigarettes but quit and are currently nonsmokers) and “yes” (individuals who smoked more than 100 cigarettes in their lifetime and are currently smokers) (23).
For energy intake, a 2-day dietary value obtained from Day 1 and Day 2 or using data from Day 1 when Day 2 data were missing. Alcohol consumption was categorized into five groups: former drinkers, nondrinkers, mild drinkers, moderate drinkers, heavy drinkers, as indicated in previous studies (24). Medical condition data encompassed BMI (weight in kg divided by height in meters squared), serum creatinine concentration measured in mg/dL using the Jaffe rate method, and various health statuses. Diabetes mellitus status was classified into four categories: Diabetes mellitus (DM, defined as fasting glucose ≥ 7.0 mmol/L, random plasma glucose ≥ 11.1 mmol/L, two-hour OGTT blood glucose ≥ 11.1 mmoL/L; glycohemoglobin HbA1c ≥ 6.5%, self-reported physician-diagnosed diabetes, or current use of antidiabetic drugs), pre-DM (fasting glucose level should be between 6.11 mmol/L and 7.0 mmol/L, or two-hour OGTT blood glucose range from 7.7 mmol/L to less than 11.1 mmol/L), or ‘no’ (normal glucose levels). Hypertension status was determined as ‘yes’ for elevated blood pressure or medication use, and ‘no’ for normal blood pressure. Similarly, stroke status was classified as either “yes” or “no” based on participants’ self-reported history of physician-diagnosed stroke.
2.5 Statistical analysis
Data analysis was conducted using R software version 4.4.0, incorporating the nhanesR (0.9.5.0), ggplot2, rms, and mediation packages (23). In alignment with analytic guidelines, sample weights were incorporated to ensure the national representativeness of the survey. Baseline characteristics of participants were stratified by frailty status, with survey-weighted mean ± standard error [SE] for continuous variables and survey-weighted proportions for categorical variables. Group comparisons were conducted utilizing survey-weighted t-test for continuous variables and survey-weighted Rao-Scott chi-square test for categorical variables. The association between the hs-CRP/HDL-C ratio and frailty status was examined through univariate and multivariate logistic regression models. Covariates were selected based on prior literature and clinical relevance. The crude model was unadjusted for any covariates. Model I adjusted for age, gender, ethnicity, and education status. Model II further included age, gender, marital status, ethnicity, education status, BMI, energy intake, FITPR, physical activity, smoking status, alcohol user status, creatinine levels, and comorbidities (hypertension, DM, and stroke). Results of the logistic regression were presented as odds ratios (ORs) with 95% confidence intervals (CIs). Restricted cubic splines (RCS) regression were used to evaluate potential non-linear relationships between the hs-CRP/HDL-C ratio and FI score. Additionally, multivariable linear regression was used to explore the associations between the hs-CRP/HDL-C ratio and plasma proteins (albumin and globulin). Causal mediation analysis was conducted to investigate if the association between the hs-CRP/HDL-C ratio and frailty status was mediated by plasma proteins. The mediation effect was estimated using bootstrapping with 1,000 resamples, and indirect, direct, and total effects were reported.
To develop and validate a predictive nomogram, the dataset was randomly divided into a training set (70% of the data) and a validation set (30% of the data), stratified by frailty status. Least absolute shrinkage and selection operator (LASSO) regression, implemented using the glmnet package, was applied to identify the key predictors in the training set. The largest penalty parameter lambda (λ) within one standard error of the minimum binomial deviation, was selected based on tenfold cross-validation with 1,000 iterations. Variables with non-zero coefficients were retained for the final model. A nomogram was then constructed using multivariate logistic regression, and its discriminatory performance was evaluated using the receiver operating characteristic (ROC) curve. Statistical significance was defined as a two-sided p value of less than 0.05.
3 Results
3.1 Baseline characteristics
Baseline characteristics stratified by frailty status are presented in Table 1. The study comprised 3,626 participants (2,839 non-frailty and 787 frailty), comprising 1935 males and 1,691 females, with a mean age of 59.67 ± 0.34 years. The frailty group had a significantly higher mean age (63.59 ± 0.55 years) compared to the non-frailty group (58.97 ± 0.36 years, p < 0.0001). Additionally, the proportion of older individuals (≥75 years) was significantly higher in the frail group (17.07%) compared to the non-frail group (7.58%). Notably, the frailty group displayed higher values in hs-CRP/HDL-C ratios, creatinine levels, and a greater incidence of single status, diabetes mellitus(DM), hypertension, and stroke than the non-frailty group. Moreover, the frailty group displayed less values in energy intake than the non-frailty group. Significant intergroup differences were identified across a range of variables, including age, marital status, ethnicity, education level, BMI, energy intake, FITPR, physical activity, creatinine levels, smoking status, alcohol consumption, hs-CRP/HDL-C ratio, diabetes mellitus status, hypertension status, and stroke status, as delineated in Table 1.
3.2 Association between the hs-CRP/HDL-C ratio and frailty status
Table 2 illustrates the associations between the quartiles of the hs-CRP/HDL-C ratio and frailty status. After adjusting for all confounding covariates, individuals in the highest quartile of the hs-CRP/HDL-C ratio displayed a significantly higher odds ratio (OR) for frailty compared to those in the lowest quartile (OR = 1.736, 95% CI: 1.009, 2.988). The multivariable-adjusted RCS analysis revealed a non-linear relationship between the hs-CRP/HDL-C ratio and frailty, observed in the overall population as well as in subgroups of frailty and non-frailty individuals (P for nonlinear < 0.05, Figures 2A–C).
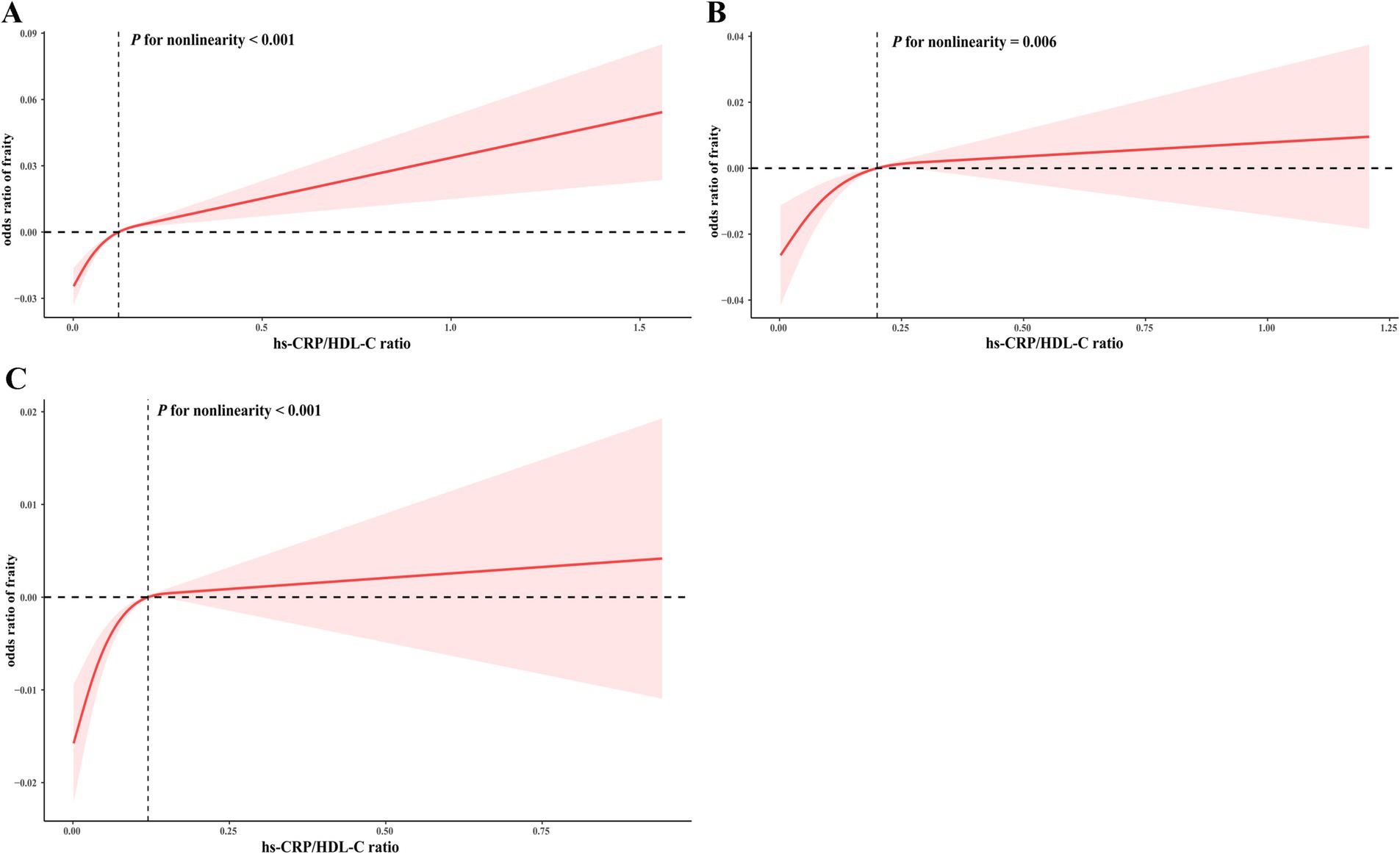
Figure 2. RCS for the correlation between the hs-CRP/HDL-C ratio and frailty index. The RCS analysis was performed in the overall population (A), as well as in subgroups specifically categorized as frailty (B) or non-frailty (C) individuals.
3.3 Associations between the hs-CRP/HDL-C ratio and plasma proteins
Plasma proteins, particularly albumin and globulin, have been associated with nutrition and inflammation (25). In our study, we employed a multivariate linear regression model to explore the connections between the hs-CRP/HDL-C ratio and these plasma proteins. Notably, as depicted in Table 3, the hs-CRP/HDL-C ratio displayed a significantly negative correlation with albumin (β = −0.011; 95% CI –0.013, –0.010, p < 0.0001), while showing a positive association with globulin (β = 0.007; 95% CI 0.006, 0.008, p < 0.0001).
3.4 The mediating role of plasma proteins in the association between the hs-CRP/HDL-C ratio and frailty
As illustrated in Figure 3, both albumin and globulin displayed significant mediation effects on the association between the hs-CRP/HDL-C ratio and frailty, with mediated proportions of 37.82% (p < 0.001, Figure 3A) and 11.23% (p = 0.016, Figure 3B), respectively.
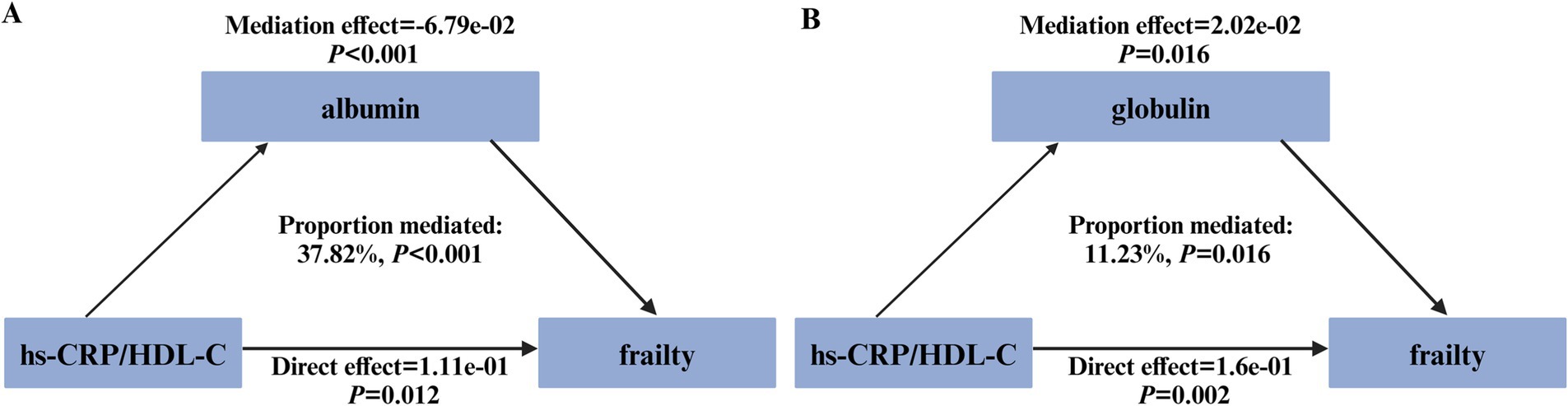
Figure 3. Path diagram illustrating the mediating role of plasma proteins. The graphs in (A,B) showed the mediating role of albumin and globulin, respectively.
3.5 LASSO regression and nomogram model
LASSO regression was employed to identify the relevant factors most associated with frailty. The LASSO regression model selected nine factors with non-zero coefficients in the training dataset, including the hs-CRP/HDL-C ratio, age, BMI, gender, FITPR, smoke, DM status, hypertension status, and stroke status (Figure 4) in the final model. These nine independent predictors were subsequently included in the nomogram construction (Figure 5). The ROC analysis demonstrated that the nomogram exhibited strong discriminatory power, with an AUC of 79.7% (95% CI 77.7–81.75%) in the training set and an AUC of 78.2% (95% CI 75.0–81.4%) in the validation set (Figure 6).
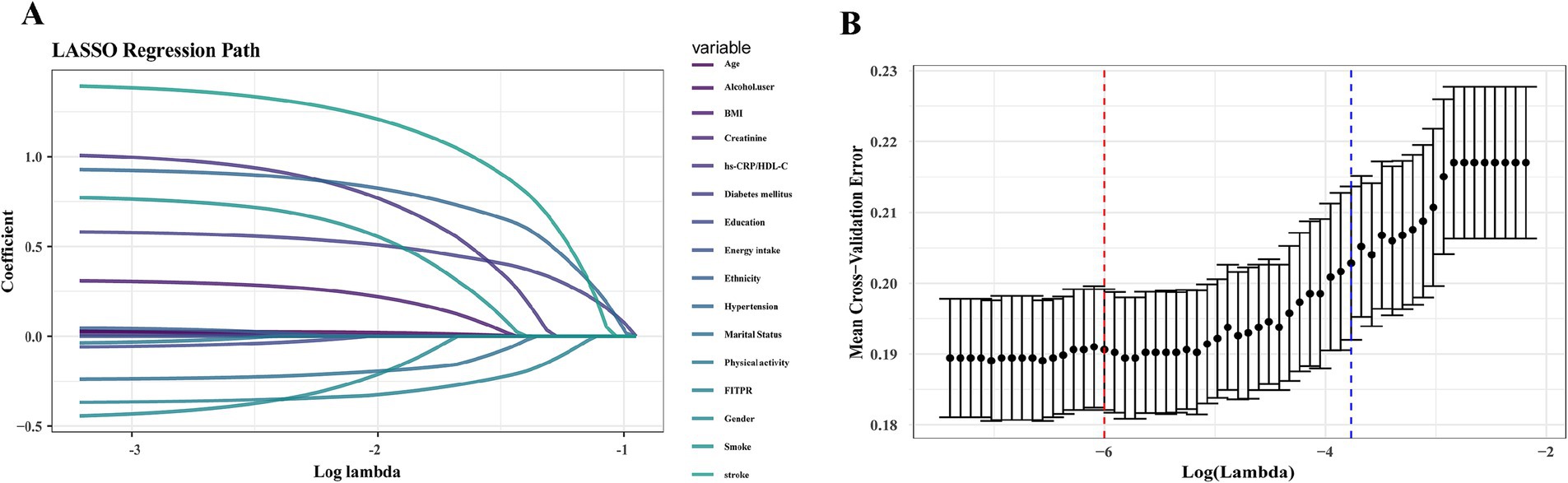
Figure 4. LASSO regression analysis to identify key variables most correlated with frailty. (A) A LASSO regression coefficient plot was constructed. Each curve represents the trajectory of an individual feature coefficient. (B) Cross-validation plot.
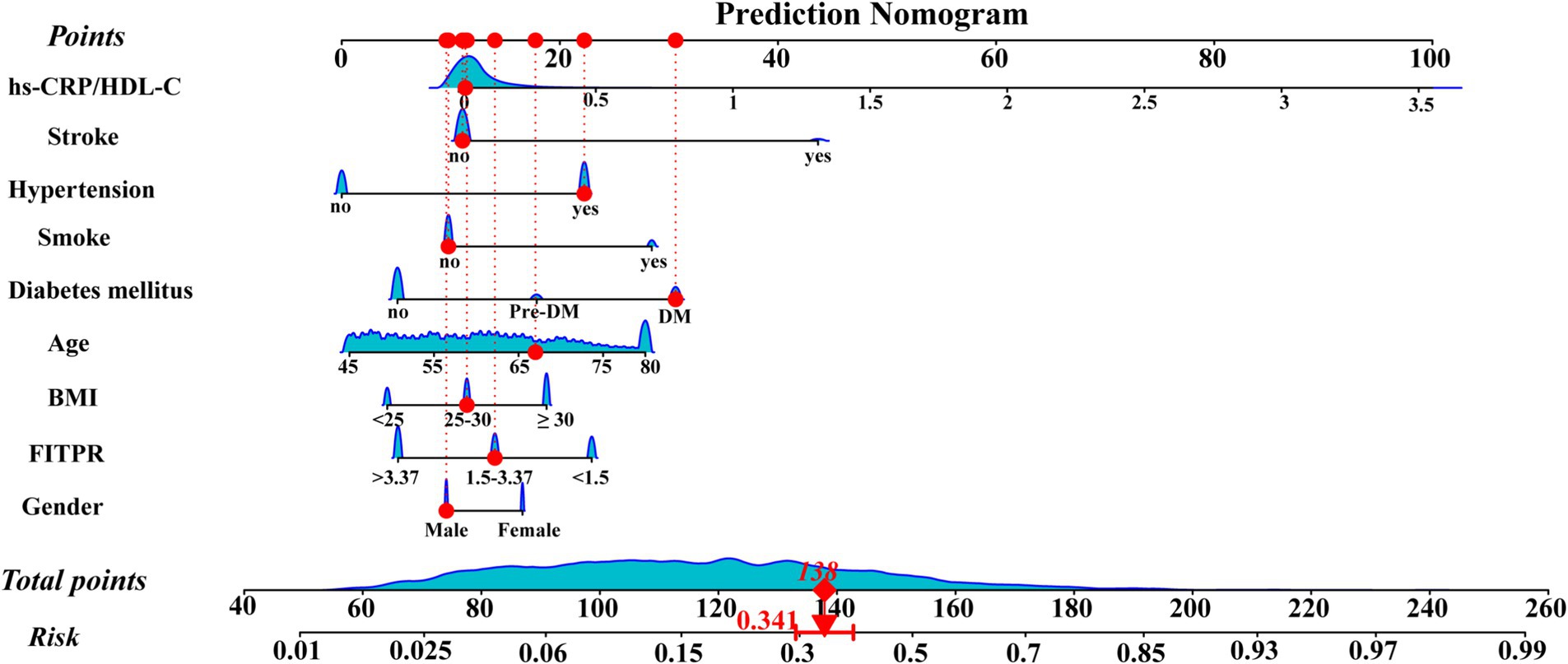
Figure 5. Nomogram for predicting the risk of frailty. The points corresponding to each feature were summed to obtain a total score, and a vertical line was drawn from the total score to determine the ‘frailty risk’. The red points illustrate a specific example: for a 67-year-old male participant with DM and hypertension, but a low hs-CRP/HDL-C ratio, the predicted probability of frailty is 34.1%.
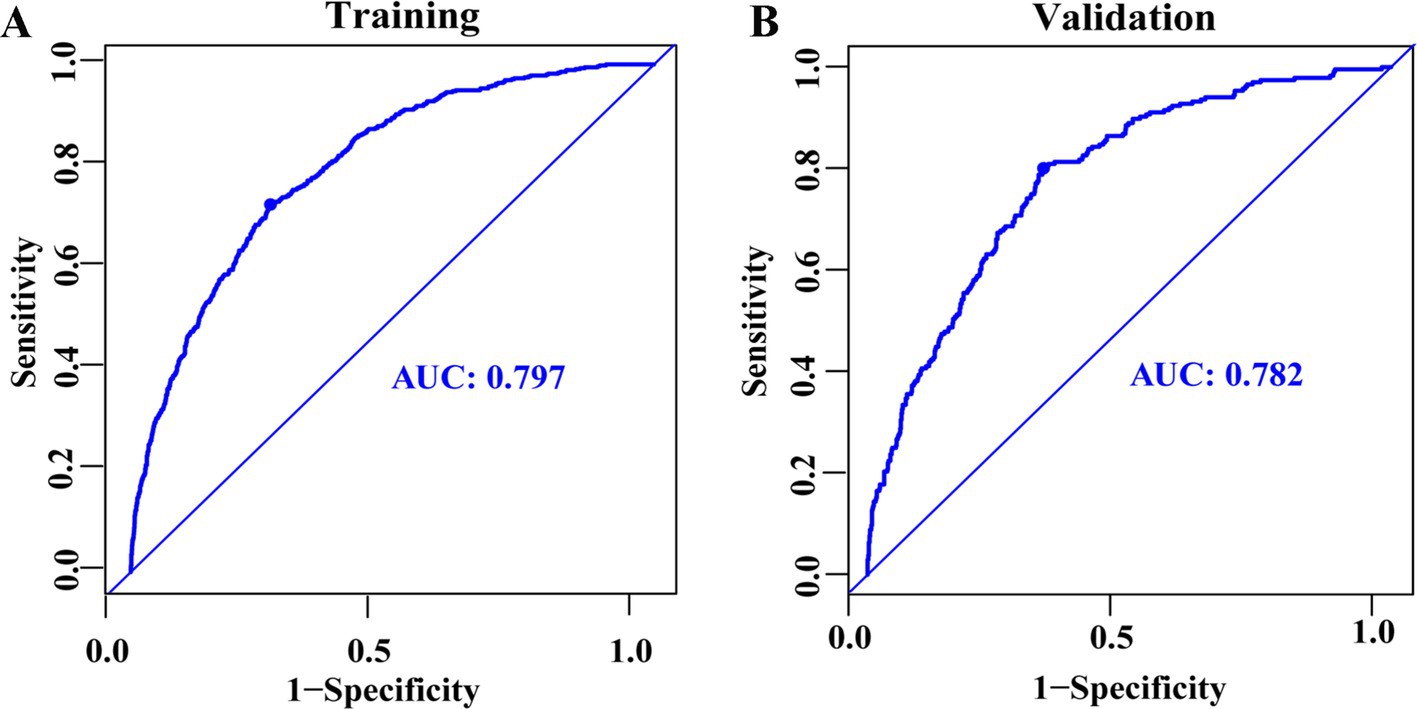
Figure 6. ROC curves for predicting frailty among middle-aged and older adults, presented for the training dataset (A) and the validation dataset (B).
4 Discussion
The prevalence of frailty is increasing with the aging population. Frailty, characterized by functional decline and increased risk of adverse outcomes such as falls, hospitalizations, and complications (26), arises from multisystem dysregulation and metabolic imbalances (4). Its diagnosis remains clinically challenging, and delayed recognition may lead to increased healthcare costs. Systemic inflammation has been linked to frailty risk (18). A 3-year longitudinal study in China revealed that hs-CRP contributes to frailty development (10). Besides, higher HDL-C levels have been associated with improved well-being and physical health (15, 27). HDL-C, with its roles in reverse cholesterol transport, anti-inflammatory, and antioxidant functions, may exert protective effects against age-related diseases (28). The hs-CRP/HDL-C ratio, a composite marker of inflammation and lipid status, reflects the balance between pro- and anti-inflammatory processes. This ratio has been recognized as a clinically relevant biomarker for cardiovascular diseases(CVD), metabolic dysfunction-associated steatotic liver disease (MASLD), advanced liver fibrosis, and poor outcomes in acute ischemic stroke (16, 29, 30), due to its simplicity and clinical relevance. These conditions, in turn, are known contributors to frailty. CVD may promote frailty by reducing physical activity and functional capacity (31). Similarly, the progression of MASLD is frequently accompanied by sarcopenia, insulin resistance, and chronic inflammation, all of which are implicated in frailty development (32). In older stroke patients, diminished multi-organ function and physiological reserve may reduce the ability to respond to external stressors, thereby increasing frailty risk (33). Therefore, an elevated hs-CRP/HDL-C ratio may contribute to frailty development through its associations with chronic comorbidities and systemic dysfunction. In our nationally representative U.S. sample, we observed a significant and non-linear association between the hs-CRP/HDL-C ratio and frailty risk among middle-aged and older adults. Collectively, these findings suggest that the hs-CRP/HDL-C holds promise as a potential biomarker for frailty assessment in this population.
The precise mechanisms underlying the association between the hs-CRP/HDL-C ratio and frailty remain elusive. Albumin, a vital serum protein component, exerts nutritional and antioxidant functions, whereas globulin, an immunoglobulin type, is indicative of systemic inflammatory activity (34, 35). Hypoalbuminemia, which worsens with age, is associated with both malnutrion and inflammation (36), and prior studies have identified albumin as a potential biomarker for frailty (37, 38). In our analysis, the hs-CRP/HDL-C ratio was negatively associated with albumin and positively related to globulin. These plasma proteins partially mediated the relationship between the hs-CRP/HDL-C ratio and frailty, suggesting their roles in inflammation-related frailty pathogenesis.
Identifying critical predictors is essential for the prevention and early management of frailty. In this study, LASSO regression was employed for variable selection. Our analysis identified the hs-CRP/HDL-C ratio, age, BMI, gender, FITPR, smoke, DM status, hypertension status, and stroke status as key predictors of frailty. Frailty, a clinical syndrome characterized by diminished muscle mass and physical activity, is closely linked to aging (39). Sex differences also influence frailty risk; men generally have greater muscle mass than women due to higher androgen levels (40). Elevated BMI is linked to increased comorbidities, such as diabetes and cardiovascular disease, and has been related to higher frailty risk in older adults (41). Beyond physiological factors, social health factors such as poverty is associated with frailty by reflecting cumulative health risks over time (42). Besides, tobacco use has been associated with telomere shortening, sarcopenia, reduced gait speed, and physical inactivity, indicating its potential role in age-related frailty (43). Hypertension may elevate frailty risk through mechanisms involving polypharmacy, physical and cognitive decline, and fall susceptibility in older adults (44). Similarly, DM promotes frailty via dysglycemia, sarcopenia, decreased muscle strength, and multimorbidity (45). In older patients, stroke may contribute to frailty through neurological dysfunction, sarcopenia and malnutrition (46). Our study supports a positive association between the hs-CRP/HDL-C ratio and frailty. Based on the identified predictors, we constructed a nomogram to serve as a predictive tool for frailty risk. This model may assist clinicians to efficiently identify individuals at high risk of frailty and initiate appropriate interventions in a timely manner.
Several limitations were identified in our study. First, we did not evaluate the cumulative impact of the hs-CRP/HDL-C ratio on frailty status. Second, the retrospective nature of self-reported data, such as smoking and alcohol use, may lead to recall bias, potentially leading to misclassification. Third, due to the observational design, causal relationships between hs-CRP/HDL-C and frailty cannot be determined. Although we adjusted for a wide range of potential confounders, there may be residual confounding from unmeasured factors that influence the association. While NHANES provides a nationally representative sample, our findings may not be applicable to individuals with specific health conditions-such as rare or severe conditions. Therefore, future study in specific populations is needed to validate our results and improve clinical applicability. Moreover, multiple biomarkers reflecting oxidative stress, nutrition, and aging—such as oxidative balance score (OBS), composite dietary antioxidant index (CDAI), albumin, globulin, and klotho—have been proposed in relation to frailty (4). Previous studies showed that OBS or CDAI has significant associations with frailty (47, 48). Although our primary focus was the hs-CRP/HDL-C ratio, we also evaluated additional markers (e.g., albumin, globulin) and demonstrated their partial mediating roles in the association between hs-CRP/HDL-C ratio and frailty. Nonetheless, some relevant biomarkers were not included in our analysis, limiting our ability to capture the full biological complexity of frailty. Future prospective studies incorporating more comprehensive data, as well as longitudinal or interventional designs, are needed to validate these findings and further explore the underlying mechanisms.
5 Conclusion
In summary, this cross-sectional study of middle-aged and older participants demonstrated a positive association between frailty and the hs-CRP/HDL-C ratio. Plasma proteins, including albumin and globulin, were identified as mediators in this correlation. Through LASSO regression, the key factors correlated with frailty were identified, and a nomogram was subsequently developed to estimate frailty risk. These findings emphasize the critical role of the hs-CRP/HDL-C ratio in predicting frailty risk, highlighting its potential clinical utility.
Data availability statement
The datasets presented in this study can be found in online repositories: https://wwwn.cdc.gov/nchs/nhanes/. Further inquiries can be directed to the corresponding author.
Ethics statement
Ethical approval for NHANES was obtained from the National Center for Health Statistics (NCHS) Institutional Review Board (Protocol #2011–17), and informed consent was obtained from all participants prior to data collection. As this study involved secondary analysis of publicly available data, additional ethical approval was not required. All procedures were conducted in accordance with local legislation and institutional requirements.
Author contributions
YiW: Funding acquisition, Methodology, Writing – review & editing. JS: Methodology, Writing – review & editing. YaW: Conceptualization, Investigation, Methodology, Supervision, Writing – original draft, Writing – review & editing.
Funding
The author(s) declare that financial support was received for the research and/or publication of this article. This work was supported by the National Natural Scientific Foundation (81100059), the Natural Scientific Fund of Hunan province (2020JJ4812,2021JJ31013,2023JJ30786,2025JJ50713, 2025JJ60578), Health and Health Project of Hunan Provincial Department of Finance (Xiang Cai She Zhi [2023] No. 79,Xiang Cai She Zhi [2024] No.0031), and Degree & Postgraduate Education Reform Project of Central South University(2022JGB075, 2023YJSKS035), China. The funding source had no role in the design, methods, subject recruitment, data collections, analysis, and preparation of this paper.
Acknowledgments
We would like to thank the NHANES team for providing the data.
Conflict of interest
The authors declare that the research was conducted in the absence of any commercial or financial relationships that could be construed as a potential conflict of interest.
Generative AI statement
The authors declare that no Gen AI was used in the creation of this manuscript.
Publisher’s note
All claims expressed in this article are solely those of the authors and do not necessarily represent those of their affiliated organizations, or those of the publisher, the editors and the reviewers. Any product that may be evaluated in this article, or claim that may be made by its manufacturer, is not guaranteed or endorsed by the publisher.
Supplementary material
The Supplementary material for this article can be found online at: https://www.frontiersin.org/articles/10.3389/fpubh.2025.1564206/full#supplementary-material
Abbreviations
BMI, Body mass index; CRP, C-reactive protein; hs-CRP, high-sensitivity C-reactive protein; HDL-C, high-density lipoprotein cholesterol; FITPR, family income-to-poverty ratio; NHANES, National Health and Nutrition Examination Survey; OR, Odds ratio; CI, Confidence interval; DM, Diabetes mellitus; IFG, impaired fasting glycaemia; IGT, impaired glucose tolerance.
References
1. Ofori-Asenso, R, Chin, KL, Mazidi, M, Zomer, E, Ilomaki, J, Zullo, AR, et al. Global incidence of frailty and prefrailty among community-dwelling older adults: a systematic review and meta-analysis. JAMA Netw Open. (2019) 2:e198398. doi: 10.1001/jamanetworkopen.2019.8398
2. El Assar, M, Rodríguez-Sánchez, I, Álvarez-Bustos, A, and Rodríguez-Mañas, L. Biomarkers of frailty. Mol Asp Med. (2024) 97:101271. doi: 10.1016/j.mam.2024.101271
3. Stenholm, S, Ferrucci, L, Vahtera, J, Hoogendijk, EO, Huisman, M, Pentti, J, et al. Natural course of frailty components in people who develop frailty syndrome: evidence from two cohort studies. J Gerontol A Biol Sci Med Sci. (2019) 74:667–74. doi: 10.1093/gerona/gly132
4. Dzięgielewska-Gęsiak, S, and Muc-Wierzgoń, M. Inflammation and oxidative stress in frailty and metabolic syndromes-two sides of the same coin. Meta. (2023) 13:475. doi: 10.3390/metabo13040475
5. Liu, X, Wang, Y, Shen, L, Sun, Y, Zeng, B, Zhu, B, et al. Association between frailty and chronic constipation and chronic diarrhea among American older adults: National Health and nutrition examination survey. BMC Geriatr. (2023) 23:745. doi: 10.1186/s12877-023-04438-4
6. Yin, MJ, Xiong, YZ, Xu, XJ, Huang, LF, Zhang, Y, Wang, XJ, et al. Tfh cell subset biomarkers and inflammatory markers are associated with frailty status and frailty subtypes in the community-dwelling older population: a cross-sectional study. Aging. (2020) 12:2952–73. doi: 10.18632/aging.102789
7. Westbrook, R, Chung, T, Lovett, J, Ward, C, Joca, H, Yang, H, et al. Kynurenines link chronic inflammation to functional decline and physical frailty. JCI Insight. (2020) 5:e136091. doi: 10.1172/jci.insight.136091
8. Tuttle, CSL, Thang, LAN, and Maier, AB. Markers of inflammation and their association with muscle strength and mass: a systematic review and meta-analysis. Ageing Res Rev. (2020) 64:101185. doi: 10.1016/j.arr.2020.101185
9. Kamil, RJ, Betz, J, Powers, BB, Pratt, S, Kritchevsky, S, Ayonayon, HN, et al. Association of hearing impairment with incident frailty and falls in older adults. J Aging Health. (2016) 28:644–60. doi: 10.1177/0898264315608730
10. Luo, YF, Cheng, ZJ, Wang, YF, Jiang, XY, Lei, SF, Deng, FY, et al. Unraveling the relationship between high-sensitivity C-reactive protein and frailty: evidence from longitudinal cohort study and genetic analysis. BMC Geriatr. (2024) 24:222. doi: 10.1186/s12877-024-04836-2
11. Rolver, MG, Emanuelsson, F, Nordestgaard, BG, and Benn, M. Contributions of elevated CRP, hyperglycaemia, and type 2 diabetes to cardiovascular risk in the general population: observational and Mendelian randomization studies. Cardiovasc Diabetol. (2024) 23:165. doi: 10.1186/s12933-024-02207-0
12. Chaturvedi, S, and De Marchis, GM. Inflammatory biomarkers and stroke subtype: an important new frontier. Neurology. (2024) 102:e208098. doi: 10.1212/wnl.0000000000208098
13. Milman, S, Atzmon, G, Crandall, J, and Barzilai, N. Phenotypes and genotypes of high density lipoprotein cholesterol in exceptional longevity. Curr Vasc Pharmacol. (2014) 12:690–7. doi: 10.2174/1570161111666131219101551
14. Kosmas, CE, Sourlas, A, Guzman, E, and Kostara, CE. Environmental factors modifying HDL functionality. Curr Med Chem. (2022) 29:1687–701. doi: 10.2174/0929867328666210714155422
15. Yuan, Y, Lin, S, Huang, X, Li, N, Zheng, J, Huang, F, et al. The identification and prediction of frailty based on Bayesian network analysis in a community-dwelling older population. BMC Geriatr. (2022) 22:847. doi: 10.1186/s12877-022-03520-7
16. Gao, Y, Wang, M, Wang, R, Jiang, J, Hu, Y, Wang, W, et al. The predictive value of the hs-CRP/HDL-C ratio, an inflammation-lipid composite marker, for cardiovascular disease in middle-aged and elderly people: evidence from a large national cohort study. Lipids Health Dis. (2024) 23:66. doi: 10.1186/s12944-024-02055-7
17. Hakeem, FF, Bernabé, E, and Sabbah, W. Association between oral health and frailty among American older adults. J Am Med Dir Assoc. (2021) 22:559–563.e2. doi: 10.1016/j.jamda.2020.07.023
18. Zhang, H, Liu, X, Wang, X, and Jiang, Y. Association of two novel systemic inflammatory biomarkers and frailty based on NHANES 2007-2018. Front Public Health. (2024) 12:1377408. doi: 10.3389/fpubh.2024.1377408
19. Fan, J, Yu, C, Guo, Y, Bian, Z, Sun, Z, Yang, L, et al. Frailty index and all-cause and cause-specific mortality in Chinese adults: a prospective cohort study. Lancet Public Health. (2020) 5:e650–60. doi: 10.1016/s2468-2667(20)30113-4
20. Guan, Z, Ma, L, and Wu, C. Association between serum klotho and physical frailty in middle-aged and older adults: finding from the National Health and nutrition examination survey. J Am Med Dir Assoc. (2023) 24:1173–1178.e2. doi: 10.1016/j.jamda.2023.02.103
21. Bao, W, Liu, B, Simonsen, DW, and Lehmler, HJ. Association between exposure to pyrethroid insecticides and risk of all-cause and cause-specific mortality in the general US adult population. JAMA Intern Med. (2020) 180:367–74. doi: 10.1001/jamainternmed.2019.6019
22. Hupin, D, Roche, F, Gremeaux, V, Chatard, JC, Oriol, M, Gaspoz, JM, et al. Even a low-dose of moderate-to-vigorous physical activity reduces mortality by 22% in adults aged ≥60 years: a systematic review and meta-analysis. Br J Sports Med. (2015) 49:1262–7. doi: 10.1136/bjsports-2014-094306
23. Bao, W, Liu, B, Rong, S, Dai, SY, Trasande, L, and Lehmler, HJ. Association between bisphenol a exposure and risk of all-cause and cause-specific mortality in US adults. JAMA Netw Open. (2020) 3:e2011620. doi: 10.1001/jamanetworkopen.2020.11620
24. Rattan, P, Penrice, DD, Ahn, JC, Ferrer, A, Patnaik, M, Shah, VH, et al. Inverse association of telomere length with liver disease and mortality in the US population. Hepatol Commun. (2022) 6:399–410. doi: 10.1002/hep4.1803
25. Chen, Z, Song, C, Yao, Z, Sun, J, and Liu, W. Associations between albumin, globulin, albumin to globulin ratio and muscle mass in adults: results from the national health and nutrition examination survey 2011-2014. BMC Geriatr. (2022) 22:383. doi: 10.1186/s12877-022-03094-4
26. Dent, E, Martin, FC, Bergman, H, Woo, J, Romero-Ortuno, R, and Walston, JD. Management of frailty: opportunities, challenges, and future directions. Lancet (London, England). (2019) 394:1376–86. doi: 10.1016/s0140-6736(19)31785-4
27. Wang, S, Liu, M, Yang, S, Wang, J, Jia, W, Cao, W, et al. Higher normal levels of triglyceride and low and high-density lipoprotein cholesterol might have a protective effect against activities of daily living disability within Chinese female centenarians: a cross-sectional, complete sample study. Clin Interv Aging. (2020) 15:225–37. doi: 10.2147/cia.S237505
28. Asztalos, BF, Tani, M, and Schaefer, EJ. Metabolic and functional relevance of HDL subspecies. Curr Opin Lipidol. (2011) 22:176–85. doi: 10.1097/MOL.0b013e3283468061
29. Liang, B, Qiu, X, Huang, J, Lu, Y, Shen, H, Ma, J, et al. Nonlinear associations of the hs-CRP/HDL-C index with metabolic dysfunction-associated steatotic liver disease and advanced liver fibrosis in US adults: insights from NHANES 2017-2018. Sci Rep. (2025) 15:4029. doi: 10.1038/s41598-025-88685-y
30. Luwen, H, Lei, X, Qing-Rong, O, Linlin, L, and Ming, Y. Association between hs-CRP/HDL-C ratio and three-month unfavorable outcomes in patients with acute ischemic stroke: a second analysis based on a prospective cohort study. BMC Neurol. (2024) 24:418. doi: 10.1186/s12883-024-03929-0
31. Takefuji, Y. Exploring the connection between frailty and cardiovascular diseases. Arch Gerontol Geriatr. (2024) 124:105449. doi: 10.1016/j.archger.2024.105449
32. Karakousis, ND, Chrysavgis, L, Chatzigeorgiou, A, Papatheodoridis, G, and Cholongitas, E. Frailty in metabolic syndrome, focusing on nonalcoholic fatty liver disease. Ann Gastroenterol. (2022) 35:234–42. doi: 10.20524/aog.2022.0705
33. Huang, YN, Yan, FH, Wang, XY, Chen, XL, Chong, HY, Su, WL, et al. Prevalence and risk factors of frailty in stroke patients: a meta-analysis and systematic review. J Nutr Health Aging. (2023) 27:96–102. doi: 10.1007/s12603-023-1879-z
34. Lai, KJ, Hsieh, YP, Chiu, PF, and Lin, PR. Association of albumin and globulin with mortality risk in incident peritoneal dialysis patients. Nutrients. (2022) 14:2850. doi: 10.3390/nu14142850
35. Huang, CY, Liou, SY, Kuo, WW, Wu, HC, Chang, YL, and Chen, TS. Chemiluminescence analysis of antioxidant capacity for serum albumin isolated from healthy or uremic volunteers. Luminescence. (2016) 31:1474–8. doi: 10.1002/bio.3132
36. Zhang, L, Yang, P, Yin, F, Zhang, J, Zhao, B, and Zhou, J. Association between frailty and hypoproteinaemia in older patients: meta-analysis and systematic review. BMC Geriatr. (2024) 24:689. doi: 10.1186/s12877-024-05275-9
37. Mailliez, A, Guilbaud, A, Puisieux, F, Dauchet, L, and Boulanger, É. Circulating biomarkers characterizing physical frailty: CRP, hemoglobin, albumin, 25OHD and free testosterone as best biomarkers. Results of a meta-analysis. Exp Gerontol. (2020) 139:111014. doi: 10.1016/j.exger.2020.111014
38. Yamamoto, M, Adachi, H, Enomoto, M, Fukami, A, Nakamura, S, Nohara, Y, et al. Lower albumin levels are associated with frailty measures, trace elements, and an inflammation marker in a cross-sectional study in Tanushimaru. Environ Health Prev Med. (2021) 26:25. doi: 10.1186/s12199-021-00946-0
39. Cruz-Jentoft, AJ, Baeyens, JP, Bauer, JM, Boirie, Y, Cederholm, T, Landi, F, et al. Sarcopenia: European consensus on definition and diagnosis: report of the European working group on sarcopenia in older people. Age Ageing. (2010) 39:412–23. doi: 10.1093/ageing/afq034
40. Conforto, R, Rizzo, V, Russo, R, Mazza, E, Maurotti, S, Pujia, C, et al. Advances in body composition and gender differences in susceptibility to frailty syndrome: role of osteosarcopenic obesity. Metab Clin Exp. (2024) 161:156052. doi: 10.1016/j.metabol.2024.156052
41. Sun, Q, Xia, X, and He, F. Longitudinal association between body mass index (BMI), BMI trajectories and the risk of frailty among older adults: a systematic review and meta-analysis of prospective cohort studies. Arch Gerontol Geriatr. (2024) 124:105467. doi: 10.1016/j.archger.2024.105467
42. Stolz, E, Mayerl, H, Waxenegger, A, and Freidl, W. Explaining the impact of poverty on old-age frailty in Europe: material, psychosocial and behavioural factors. Eur J Pub Health. (2017) 27:1003–9. doi: 10.1093/eurpub/ckx079
43. Park, S, Kim, SG, Lee, S, Kim, Y, Cho, S, Kim, K, et al. Causal linkage of tobacco smoking with ageing: Mendelian randomization analysis towards telomere attrition and sarcopenia. J Cachexia Sarcopenia Muscle. (2023) 14:955–63. doi: 10.1002/jcsm.13174
44. Shi, J, Tao, Y, Chen, S, Zhou, Z, Meng, L, Duan, C, et al. Interaction between hypertension and frailty and their impact on death risk in older adults: a follow-up study. BMC Geriatr. (2024) 24:187. doi: 10.1186/s12877-024-04793-w
45. Chi, CY, Wang, J, Lee, SY, Chao, CT, Hung, KY, and Chien, KL. The impact of glucose-lowering strategy on the risk of increasing frailty severity among 49,519 patients with diabetes mellitus: a longitudinal cohort study. Aging Dis. (2023) 14:1917–26. doi: 10.14336/ad.2023.0225
46. Wei, J, Wang, J, Chen, J, Yang, K, and Liu, N. Stroke and frailty index: a two-sample Mendelian randomisation study. Aging Clin Exp Res. (2024) 36:114. doi: 10.1007/s40520-024-02777-9
47. Liu, Y, Han, Y, Gao, Y, Yao, N, Wang, Y, Wang, F, et al. The association between oxidative balance score and frailty in adults across a wide age spectrum: NHANES 2007-2018. Food Funct. (2024) 15:5041–9. doi: 10.1039/d4fo00870g
Keywords: frailty, hs-CRP, HDL-C, NHANES, plasma proteins
Citation: Wang Y, Su J and Wang Y (2025) Cross-sectional association between hs-CRP/HDL-C ratio and physical frailty among middle-aged and older adults: findings from a population-based study. Front. Public Health. 13:1564206. doi: 10.3389/fpubh.2025.1564206
Edited by:
Alexey Moskalev, Komi Scientific Center (RAS), RussiaReviewed by:
Sylwia Dziegielewska-Gesiak, Medical University of Silesia, PolandLorenzo Blandi, University of Pavia, Italy
Copyright © 2025 Wang, Su and Wang. This is an open-access article distributed under the terms of the Creative Commons Attribution License (CC BY). The use, distribution or reproduction in other forums is permitted, provided the original author(s) and the copyright owner(s) are credited and that the original publication in this journal is cited, in accordance with accepted academic practice. No use, distribution or reproduction is permitted which does not comply with these terms.
*Correspondence: Yang Wang, MTkxMzY1NDUxM0BxcS5jb20=