- Department of Gastroenterology, Ningbo Hospital of Traditional Chinese Medicine, Affiliated With Zhejiang Chinese Medical University, Ningbo, China
Background: Recent studies have highlighted an association between lipid disorders and sarcopenia. The role of the non-high-density lipoprotein cholesterol to high-density lipoprotein cholesterol ratio (NHHR) has not been explored among Chinese adults. This study aimed to investigate the association between the NHHR and incident sarcopenia in the Chinese population.
Methods: The study included a total of 4,046 participants aged 50 years and older without a history of sarcopenia, from the China Health and Retirement Longitudinal Study (CHARLS). A multivariate logistic regression model and a restricted cubic spline model were used to investigate the association between NHHR and sarcopenia. Subgroup and sensitivity analyses were conducted to assess the robustness of the findings.
Results: A total of 309 (7.6%) participants were newly diagnosed with sarcopenia in the 2015 wave. Participants in the highest NHHR quartile (≥3.99) had a significantly lower adjusted odds ratio for sarcopenia (OR = 0.40; 95% CI, 0.280.58; p < 0.001) compared with those in the lowest quartile (<2.24). Restricted cubic spline analysis revealed a nonlinear relationship between NHHR and sarcopenia risk (p for nonlinearity <0.05). In piecewise regression models, the adjusted OR for sarcopenia was 0.65 (95% CI, 0.550.78, p < 0.001) among participants with NHHR <4.4, whereas no significant correlation was observed among those with NHHR ≥ 4.4. No significant interactions were found between NHHR and age, sex, hypertension, or diabetes in stratified analysis (p for interaction >0.05).
Conclusion: There is an inverse relationship between NHHR and sarcopenia risk in the Chinese population. A higher NHHR is associated with a lower risk of sarcopenia below the inflection point, beyond which NHHR is no longer significantly associated with sarcopenia risk.
1 Introduction
Sarcopenia is a condition characterized by an age-related loss of muscle strength, which is often paired with a decrease in skeletal muscle mass and/or impaired physical performance (1). The condition is strongly linked to adverse outcomes, including diminished physical function, increased risk of falls, metabolic problems, cognitive decline, and higher mortality rates (2–4). Globally, sarcopenia affects 5–10% of the general population and 10–27% of individuals aged 60 years and above (5, 6). In China, the prevalence of sarcopenia among older adults ranges from 11 to 18% (7).Sarcopenia is an emerging public health concern, due to rising healthcare costs worldwide.
The non-high-density lipoprotein cholesterol to high-density lipoprotein cholesterol ratio (NHHR) is an emerging lipid marker with significant potential for predicting the risk of various diseases, including coronary artery disease, depression, diabetes, and obstructive sleep apnea (8–11). There is evidence suggesting that NHHR is superior to traditional lipid markers in assessing the severity of atherosclerosis in middle-aged and older individuals in China (12). In addition, NHHR has been linked to both all-cause and cardiovascular-related mortality in individuals with diabetes or prediabetes (13).
Several studies have investigated the relationships between common lipoproteins, such as the TG/HDL-C ratio (14) and HDL-C (15, 16), and sarcopenia. Recent research has revealed a positive association between NHHR and sarcopenia in American adults (17–19). However, studies examining this relationship in other populations are limited. From the China Health and Retirement Longitudinal Study (CHARLS), we collected data on sarcopenia, NHHR, and related factors. We then analyzed the incidence of sarcopenia via the 2015 wave of data and performed a stratified analysis.
2 Methods
2.1 Study population
CHARLS is a large prospective cohort study that provides high-quality microdata in China, with a response rate of 80.5%. The baseline survey was conducted in 2011 and covered 28 provinces (autonomous regions and municipalities) nationwide. A multistage sampling method was used, with probability proportional sampling applied at both the county and village levels. The health status of the participants was followed up every 2 to3 years. More comprehensive information about CHARLS has been reported elsewhere (20). The Biomedical Ethics Committee at Peking University approved the trial, and written consent was obtained from all participants.
In total, 17,705 participants were recruited in the CHARLS 2011 wave. A total of 13,659 participants were excluded for the following reasons: (1) age <50 years in 2011 (n = 4,359); (2) missing data on sarcopenia in 2011 (n = 3,411), and 2015 (n = 2,843); (3) presence of sarcopenia in 2011 (n = 1711); (4) missing data on NHHR (n = 1,201); and (5) lack of data on other covariates (n = 134). Finally, 4,046 participants were classified into the nonsarcopenia group and the sarcopenia group based on their sarcopenia diagnosis in 2015. A detailed flowchart is presented in Figure 1.
2.2 Measurements
2.2.1 Diagnosis of sarcopenia
On the basis of the Asian Working Group for Sarcopenia (AWGS) 2019, sarcopenia was defined through muscle strength, muscle mass, and physical performance (1). Muscle strength was measured via handgrip strength. It was considered reduced if handgrip strength was <28 kg for men or <18 kg for women. CHARLS did not measure muscle mass in the participants. Appendicular skeletal muscle mass (ASM) was calculated by a validated anthropometric equation in the Chinese population, which has shown a high agreement with dual-energy X-ray absorptiometry (DXA) measured ASM, and has been widely used in large-scale epidemiological studies (21–23), as follows:
Sex was set to 1 for males and 0 for females. For muscle mass assessment, ASM was adjusted for height (ASM/Ht2) where the lowest 20% ASM/Ht2 was the cut-ff value for low muscle mass (22). Accordingly, individuals with ASM/Ht2 values <5.69 kg/m2 for women and <6.88 kg/m2 for men were classified as having low muscle mass.
Physical performance was considered impaired if the walking speed was < 1 m/s, the time to complete the five-time chair stand test was >12 s, or if the participant was unable to complete the test. Participants with low muscle mass and low muscle strength or impaired physical performance were classified as having sarcopenia.
2.2.2 Assessment of NHHR
NHHR was calculated as the ratio of non-HDL-C (mmol/L) to HDL-C (mmol/L), where non-HDL-C was determined by subtracting HDL-C (mmol/L) from total cholesterol (TC, mmol/L) (24).
2.2.3 Assessment of covariates
The covariates used in this study included age, sex, education level, marital status, location of residence, smoking status, drinking status, and chronic diseases (hypertension, diabetes, heart disease, and kidney disease). The covariates were defined in line with a previous study (25).
2.3 Statistical analysis
Continuous variables were expressed as the mean values and standard deviations (SDs), and categorical variables were expressed as percentages. One-way analysis of variance, Kruskal–Wallis test, and chi-square test were used to analyze the baseline characteristics and incidence of sarcopenia. Logistic regression analysis was employed to calculate the odds ratios (ORs) with 95% confidence intervals (CIs) for the association between NHHR and sarcopenia.
In addition, restricted cubic spline (RCS) regression with three knots was conducted to assess the potential nonlinear relationship and to explore the dose–response curve between NHHR and sarcopenia. A two–piece logistic regression model with smoothing was used to investigate the threshold between NHHR and sarcopenia. The inflection points were identified by the likelihood ratio test and the bootstrap resampling method.
Interaction and subgroup analyses were performed to investigate the possible influence of sex, age (50–65 vs. ≥65 years), hypertension, and diabetes on the association between NHHR and sarcopenia. We used multivariate logistic regression to investigate the heterogeneity between subgroups.
To address potential confounding factors, we employed propensity score matching (PSM) to minimize the influence of sex differences between the sarcopenia and non-sarcopenia groups. The PSM analysis was performed using a logistic regression model, where sex was the covariate to estimate the propensity scores.
Matching was conducted using a 1:1 nearest–neighbor matching algorithm without replacement, with a caliper of 0.2 to minimize bias. To assess the balance between the matched groups, standardized mean differences (SMDs) were calculated, and a threshold of SMD < 0.1 was considered an acceptable threshold.
In addition to sex-based PSM, we performed multivariate logistic regression analyses to adjust for other potential confounders, including age and smoking habits. The robustness of the non-linear association between NHHR and sarcopenia was evaluated through unmatched data.
The analyses were carried out using R Statistical Software (Version 4.4.2, http://www.R-project.org, The R Foundation) and the Free Statistics Analysis Platform (Version 2.1, Beijing, China). Two-sided values of p < 0.05 were considered significant.
3 Results
3.1 Baseline characteristics
Table 1 presents the baseline characteristics of the 4,046 participants in this study, 309 of whom developed sarcopenia. The mean age was 60.4 (6.9) years, and 1732 participants were female. Compared with those without sarcopenia, the individuals with sarcopenia were more likely to be older, and female, have lower levels of education, be unmarried, live in nonrural areas, have higher HDL-C levels, and have lower NHHR values. Before matching, the sarcopenia and non-sarcopenia groups differed in sex, age, and smoking habits. After sex-based PSM, the SMDs for all covariates were below 0.1, indicating an adequate balance between the groups (Supplementary Table S1).
3.2 Association between NHHR and sarcopenia
After adjusting for possible confounders, a high NHHR was associated with a decreased likelihood of sarcopenia (Table 2). The association remained stable when NHHR was categorized into quartiles. Compared with the participants in quartile 1 (Q1:≤2.24), those in Q2 (2.25–3.03), Q3 (3.04–3.99), and Q4 (≥4.00) had adjusted ORs for sarcopenia of 0.51 (95% CI, 0.37–0.71; p < 0.001), 0.51 (95% CI, 0.37–0.71, p < 0.001), and 0.40 (95% CI, 0.28–0.58, p < 0.001), respectively.
Furthermore, the association between NHHR and sarcopenia remained stable after PSM, indicating the robustness of our findings (Supplementary Table S2).
The non–linear association between NHHR and sarcopenia was observed both before (Figure 2) and after (Supplementary Figure S1) PSM in the RCS. Before PSM, in the two-piecewise regression models, the OR for developing sarcopenia was 0.65 (95% CI, 0.55–0.78, p < 0.001) among the participants with an NHHR <4.4, whereas no significant association was found between NHHR and sarcopenia in those with an NHHR ≥4.4 (Table 3). Supplementary Figure S1 illustrates the results of the RCS analysis, demonstrating a stable non–linear trend between NHHR and sarcopenia regardless of sex matching (p for non-linearity <0.001).
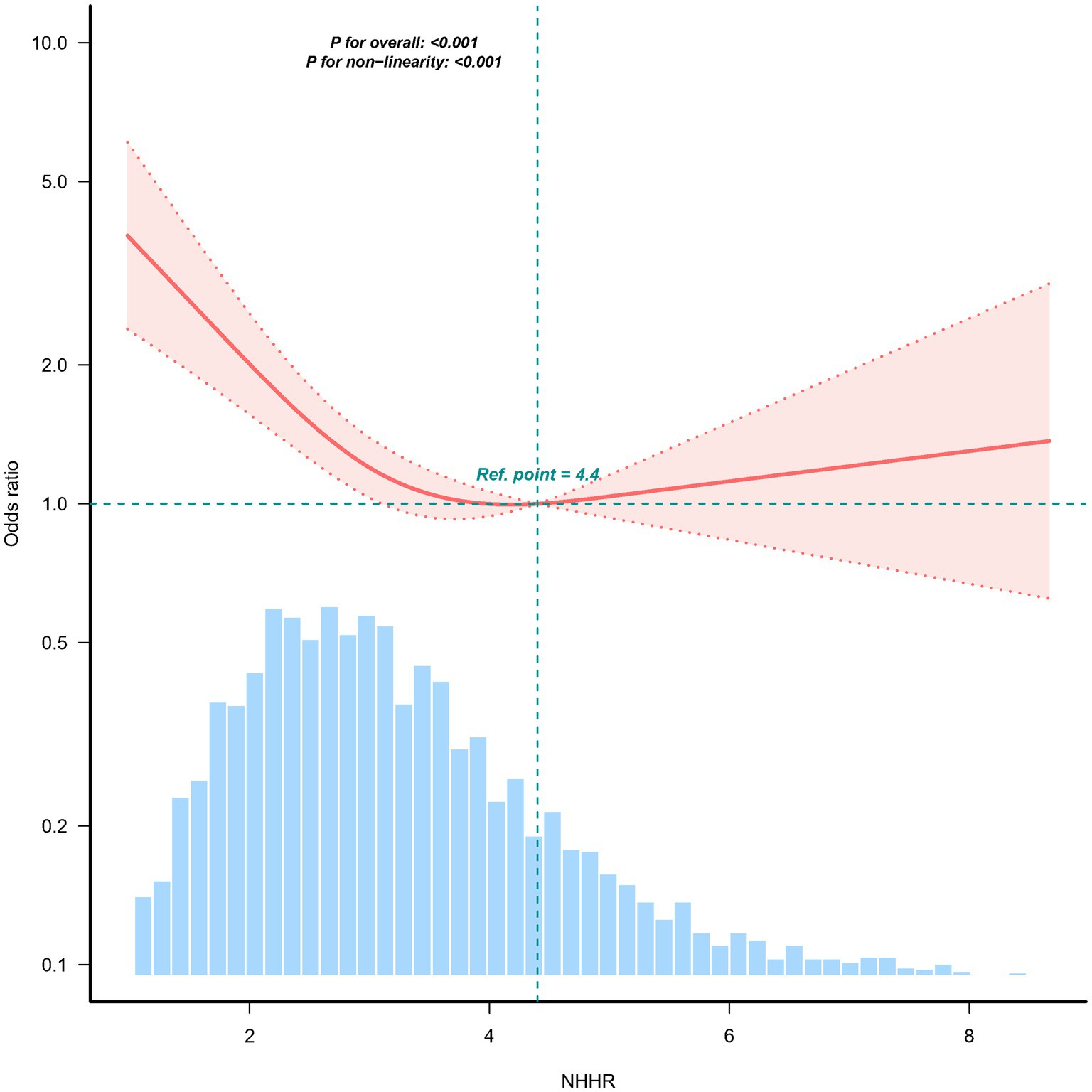
Figure 2. Association between the NHHR and the sarcopenia odds ratio. The solid and dashed lines indicate the predicted values and 95% confidence intervals, respectively. The restricted cubic spine model was adjusted for age, sex, education level, location, marital status, smoking status, drinking status, diabetes, hypertension, heart disease, and kidney disease. Only 98.5% of the data are shown.
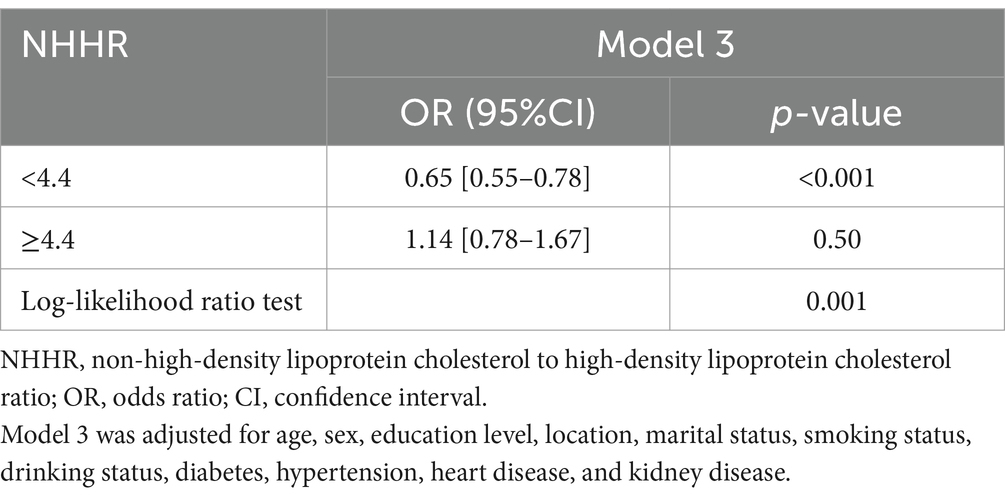
Table 3. The relationship between NHHR and the risk of sarcopenia using two-piecewise regression models.
3.3 Stratified analyses
Analyses were stratified to evaluate possible modifications in the effect of NHHR on sarcopenia across different subgroups. There were no significant interactions in any of the subgroups after stratification by age, sex, hypertension, and diabetes (Figure 3). However, a statistically significant interaction was observed for sex (p for interaction = 0.031), indicating that the association between NHHR and sarcopenia may differ by sex. The stratified analysis after PSM showed that although higher NHHR levels (such as in the age group ≥65 years, and in the non-hypertension group) could reduce the risk of sarcopenia, the statistical significance was relatively weak. Overall, the heterogeneity of the association between NHHR and sarcopenia across different subgroups was minimal, suggesting that the impact of NHHR on sarcopenia risk was relatively consistent among various subgroups, consistent with the results before PSM (Supplementary Figure S2). After PSM, the interaction between sex and NHHR was no longer statistically significant (P for interaction >0.05), suggesting that the previously observed sex-specific heterogeneity may have been influenced by confounding factors or an imbalance in baseline characteristics.
3.4 Sensitivity analysis
Sensitivity analysis was performed to verify the robustness of the study findings. Individuals with extreme NHHR values (<0 or >6.61) were excluded, and 3,922 participants remained. The association between NHHR and the risk of sarcopenia remained consistent. Compared with the participants in the lowest quartile (Q1: ≤2.24), the adjusted ORs for NHHR in Q3 (3.04–3.99) and Q4 (≥4.00) were 0.52 (95% CI, 0.37–0.73, p < 0.001) and 0.39 (95% CI, 0.27–0.57, p < 0.001), respectively (Supplementary Table S1).
4 Discussion
Baseline data from CHARLS indicated that participants with sarcopenia were more likely to be older, female, and have higher HDL-C levels, and have lower NHHRs. Follow-up data revealed an inverse relationship between NHHR and sarcopenia risk. Each unit increase in NHHR was associated with a 16% reduction in sarcopenia risk (OR, 0.84; 95% CI, 0.76–0.92). Dose–response analysis further confirmed a nonlinear (L-shaped) association between NHHR and sarcopenia. Notably, the sex interaction that had been observed before matching disappeared after PSM, which may indicate that the initial interaction effect was partly attributable to residual confounding factors such as age and comorbidities. The consistency of the association after matching further supports the robustness of the relationship between NHHR and sarcopenia.
The results revealed a consistent and stable association between higher NHHR levels and reduced sarcopenia risk both before and after PSM. Before PSM, we identified a significant threshold at an NHHR of 4.4 in the nonlinear relationship with sarcopenia. Specifically, NHHR was negatively associated with the incidence of sarcopenia when it was lower than 4.4. Although NHHR has traditionally been considered a risk factor for cardiovascular diseases, our findings offer new insights into the potential positive impact of NHHR on sarcopenia in older adults. These results underscore the importance of screening for sarcopenia in older adults with very low NHHR levels in clinical practice.
Lipid profiles provide essential insights into lipid metabolism and overall health status and are commonly used to predict the risk of various diseases (26). Several studies have suggested a potential association between lipid profiles and sarcopenia. Notably, high HDL-C levels traditionally considered protective against cardiovascular disease have been linked to an increased risk of sarcopenia among middle-aged and older adults in China (15, 16). A study by Wang et al. (27) with 2,613 participants revealed that the TG/HDL-C ratio was inversely associated with sarcopenia (OR, 0.63; 95% CI, 0.49–0.81), which aligns with our findings. Similarly, Lin et al. (14) reported that the TG/HDL-C ratio inversely correlated with sarcopenia, noting that individuals in the highest quartile of TG/HDL-C had a lower risk of severe sarcopenia among patients with diabetes in China.
As an accessible and low-cost lipid marker, NHHR could serve as a useful tool in primary care settings, especially in low-resource environments where more expensive diagnostic tests may not be feasible. Its potential application in sarcopenia screening could offer a cost-effective alternative for early identification of participants who may be at risk for sarcopenia. Our findings revealed an inverse relationship between low NHHR and sarcopenia, consistent with recent epidemiological studies examining other health outcomes. Yu et al. (13) found that when NHHR values were below 2.72 and 2.83, each unit increase in NHHR corresponded to a 24% decrease in overall mortality and a 30% decrease in cardiovascular mortality. However, NHHR positively correlated with both overall and cardiovascular mortality when values exceeded the inflection point. Similarly, Liu et al. (28) reported a U-shaped relationship between NHHR and the incidence of major adverse cardiovascular and cerebrovascular events(MACCEs) in Chinese patients with coronary artery disease who underwent percutaneous coronary intervention (PCI), indicating that both low and high NHHR values were associated with an increased risk of MACCEs.
The findings of these studies highlight the importance of maintaining an appropriate NHHR, and our study also suggests that the lowest NHHR does not necessarily indicate the best health state. The possible reasons why lower NHHR values are linked to a higher risk of sarcopenia remain unclear. Excessively low NHHR values may result from high levels of HDL-C. Although HDL-C has traditionally been considered “good cholesterol” due to its protective effects against cardiovascular diseases and reduces all-cause mortality (29), recent studies have demonstrated that elevated HDL-C levels may paradoxically increase the risk of adverse cardiovascular outcomes and mortality (30). Differences in HDL particle composition between individuals with low and high HDL-C levels have been reported (31), which may potentially lead to altered HDL functions (32). Excessively elevated HDL-C levels have been shown to induce cellular senescence and become pro–inflammatory (33, 34). Increased levels of reactive oxygen species are induced, leading to the activation of the ubiquitin–proteasome cascade reaction, which subsequently promotes muscle proteolysis and results in reduced muscle mass (35, 36). Thus, the inverse association between NHHR and sarcopenia observed in our study may reflect complex interactions between lipid metabolism and inflammation. Further studies are warranted to elucidate these mechanisms.
In contrast to our findings, previous research has reported a positive association between NHHR and sarcopenia in American adults (17, 19). Our findings differ from those of previous studies, possibly due to variations in the study populations and the diagnostic criteria used for diagnosing sarcopenia. However, further studies are needed to clarify the mechanisms linking NHHR to sarcopenia risk.
Emerging evidence suggests that both lipid and glucose metabolism are influenced by genetic factors, contributing to atherosclerotic burden and metabolic fragility, which is particularly relevant in the aging population (37). These findings support the notion that composite lipid indexes, such as NHHR, may impact broader age-related outcomes such as sarcopenia. In addition, subclinical organ damage, especially kidney dysfunction, can often be detected at an early stage using sensitive biomarkers, even in the absence of overt clinical symptoms (38). These studies are relevant to understanding how metabolic imbalances may drive early changes in sarcopenia.
4.1 Strengths and limitations
As a nationwide and prospective cohort study, our research included a diverse representative sample. We employed three models to adjust for potential confounders, enhancing the robustness and validity of our findings. We also performed stratified analyses to explore this association across multiple subgroups.
However, this study has some limitations. First, although we employed multivariate logistic regression models, along with subgroup and sensitivity analyses, residual confounding effects cannot be entirely ruled out, particularly due to the self-reported nature of comorbidities and lifestyle factors. The reliance on self-reported data introduced potential biases such as misclassification or recall bias, which could have affected the accuracy of the estimates. Second, we exclusively recruited participants aged 50 years and above, so additional studies are needed to extend our findings to younger populations. Third, certain variables, such as chronic disease status, smoking, and alcohol consumption, were self-reported through questionnaires, which could have introduced recall bias. Fourth, this study used an equation to calculate muscle mass rather than direct techniques such as DXA. Although the equation has been validated and shows high agreement with DXA-measured ASM, potential misclassification exists, particularly in aging populations. Finally, as this study focused on Chinese older individuals, caution should be exercised when generalizing the findings to other populations.
5 Conclusion
In this study, we observed an inverse association between NHHR and sarcopenia. In older adults with a very low NHHR, screening for sarcopenia in clinical practice is crucial.
Data availability statement
The original contributions presented in the study are included in the article/Supplementary material, further inquiries can be directed to the corresponding author.
Ethics statement
The studies involving humans were approved by Peking University in Beijing, China. The studies were conducted in accordance with the local legislation and institutional requirements. The participants provided their written informed consent to participate in this study. Written informed consent was obtained from the individual(s) for the publication of any potentially identifiable images or data included in this article.
Author contributions
CS: Conceptualization, Data curation, Writing – original draft, Writing – review & editing. HJ: Conceptualization, Methodology, Writing – original draft, Writing – review & editing. HZ: Conceptualization, Methodology, Writing – original draft, Writing – review & editing. ZL: Formal analysis, Investigation, Software, Writing – original draft, Writing – review & editing. SW: Project administration, Supervision, Visualization, Writing – original draft, Writing – review & editing.
Funding
The author(s) declare that no financial support was received for the research and/or publication of this article.
Acknowledgments
We appreciate CHARLS for making their data available and thank all of the CHARLS personnel for their contributions to this study. We thank LetPub (www.letpub.com.cn) for its linguistic assistance during the preparation of this manuscript.
Conflict of interest
The authors declare that the research was conducted in the absence of any commercial or financial relationships that could be construed as a potential conflict of interest.
Generative AI statement
The authors declare that no Gen AI was used in the creation of this manuscript.
Publisher’s note
All claims expressed in this article are solely those of the authors and do not necessarily represent those of their affiliated organizations, or those of the publisher, the editors and the reviewers. Any product that may be evaluated in this article, or claim that may be made by its manufacturer, is not guaranteed or endorsed by the publisher.
Supplementary material
The Supplementary material for this article can be found online at:https://www.frontiersin.org/articles/10.3389/fpubh.2025.1585986/full#supplementary-material
SUPPLEMENTARY FIGURE S1 | Association between NHHR and the sarcopenia odds ratio after PSM. The solid and dashed lines indicate the predicted values and 95% confidence intervals, respectively. The restricted cubic spline model was adjusted for age, sex, education level, location, marital status, smoking status, drinking status, diabetes, hypertension, heart disease, and kidney disease. Only 98.5% of the data are shown.
References
1. Chen, LK, Woo, J, Assantachai, P, Auyeung, TW, Chou, MY, Iijima, K, et al. Asian working Group for Sarcopenia: 2019 consensus update on sarcopenia diagnosis and treatment. J Am Med Dir Assoc. (2020) 21:300–307.e2. doi: 10.1016/j.jamda.2019.12.012
2. Yuan, S, and Larsson, SC. Epidemiology of sarcopenia: prevalence, risk factors, and consequences. Metabolism. (2023) 144:155533. doi: 10.1016/j.metabol.2023.155533
3. Hu, Y, Peng, W, Ren, R, Wang, Y, and Wang, G. Sarcopenia and mild cognitive impairment among elderly adults: the first longitudinal evidence from CHARLS. J Cachexia Sarcopenia Muscle. (2022) 13:2944–52. doi: 10.1002/jcsm.13081
4. Vicedomini, ACC, Waitzberg, DL, Lopes, NC, Magalhães, N, Prudêncio, APA, Jacob Filho, W, et al. Prognostic value of new sarcopenia screening tool in the elderly-SARC-GLOBAL. Nutrients. (2024) 16:1717. doi: 10.3390/nu16111717
5. Huang, Q, Wan, J, Nan, W, Li, S, He, B, and Peng, Z. Association between manganese exposure in heavy metals mixtures and the prevalence of sarcopenia in US adults from NHANES 2011-2018. J Hazard Mater. (2024) 464:133005. doi: 10.1016/j.jhazmat.2023.133005
6. Petermann-Rocha, F, Balntzi, V, Gray, SR, Lara, J, Ho, FK, Pell, JP, et al. Global prevalence of sarcopenia and severe sarcopenia: a systematic review and meta-analysis. J Cachexia Sarcopenia Muscle. (2022) 13:86–99. doi: 10.1002/jcsm.12783
7. Xin, C, Sun, X, Lu, L, and Shan, L. Prevalence of sarcopenia in older Chinese adults: a systematic review and meta-analysis. BMJ Open. (2021) 11:e041879. doi: 10.1136/bmjopen-2020-041879
8. Qi, X, Wang, S, Huang, Q, Chen, X, Qiu, L, Ouyang, K, et al. The association between non-high-density lipoprotein cholesterol to high-density lipoprotein cholesterol ratio (NHHR) and risk of depression among US adults: a cross-sectional NHANES study. J Affect Disord. (2024) 344:451–7. doi: 10.1016/j.jad.2023.10.064
9. Sheng, G, Liu, D, Kuang, M, Zhong, Y, Zhang, S, and Zou, Y. Utility of non-high-density lipoprotein cholesterol to high-density lipoprotein cholesterol ratio in evaluating incident diabetes risk. Diabetes Metab Syndr Obe. (2022) 15:1677–86. doi: 10.2147/DMSO.S355980
10. Pan, X, Zhang, X, Wu, X, Zhao, Y, Li, Y, Chen, Z, et al. Association between non-high-density lipoprotein cholesterol to high-density lipoprotein cholesterol ratio and obstructive sleep apnea: A cross-sectional study from NHANES. Lipids Health Dis. (2024) 23:209. doi: 10.1186/s12944-024-02195-w
11. You, J, Wang, Z, Lu, G, and Chen, Z. Association between the non-high-density lipoprotein cholesterol to high-density lipoprotein cholesterol ratio and the risk of coronary artery disease. Biomed Res Int. (2020) 2020:7146028. doi: 10.1155/2020/7146028
12. Zhao, W, Gong, W, Wu, N, Li, Y, Ye, K, Lu, B, et al. Association of lipid profiles and the ratios with arterial stiffness in middle-aged and elderly Chinese. Lipids Health Dis. (2014) 13:37. doi: 10.1186/1476-511X-13-37
13. Yu, B, Li, M, Yu, Z, Zheng, T, Feng, X, Gao, A, et al. The non-high-density lipoprotein cholesterol to high-density lipoprotein cholesterol ratio (NHHR) as a predictor of all-cause and cardiovascular mortality in US adults with diabetes or prediabetes: NHANES 1999–2018. BMC Med. (2024) 22:317. doi: 10.1186/s12916-024-03536-3
14. Lin, Y, Zhong, S, and Sun, Z. Association between serum triglyceride to high-density lipoprotein cholesterol ratio and sarcopenia among elderly patients with diabetes: a secondary data analysis of the China health and retirement longitudinal study. BMJ Open. (2023) 13:e075311. doi: 10.1136/bmjopen-2023-075311
15. Wang, M, Yang, Z, and Zhai, H. Association of High-Density Lipoprotein Cholesterol with sarcopenia in Chinese community-dwelling middle-aged and older adults: evidence from 4-year longitudinal study. Gerontology. (2024) 70:812–22. doi: 10.1159/000538980
16. Hua, N, Qin, C, Wu, F, Wang, A, Chen, J, and Zhang, Q. High-density lipoprotein cholesterol level and risk of muscle strength decline and sarcopenia in older adults. Clin Nutr. (2024) 43:2289–95. doi: 10.1016/j.clnu.2024.08.017
17. He, R, Ye, Y, Zhu, Q, and Xie, C. Association between non-high-density lipoprotein cholesterol to high-density lipoprotein cholesterol ratio and sarcopenia in individuals with cancer: a cross-sectional study. Lipids Health Dis. (2024) 23:217. doi: 10.1186/s12944-024-02205-x
18. Yang, X, and Zhong, Z. The association between non-high-density lipoprotein cholesterol to high-density lipoprotein cholesterol ratio (NHHR) and sarcopenia: a cross-sectional study. Exp Gerontol. (2025) 200:112680. doi: 10.1016/j.exger.2025.112680
19. Yanming, M, Lingjiang, L, Renji, W, and Xiaojun, Y. Yinguang W, Ruoyu L, Huimin H, Xiaodong W, Dingjun H: association between non-high-density lipoprotein cholesterol to high-density lipoprotein cholesterol ratio and sarcopenia based on NHANES. Sci Rep. (2024) 14:30166. doi: 10.1038/s41598-024-81830-z
20. Zhao, Y, Hu, Y, Smith, JP, Strauss, J, and Yang, G. Cohort profile: The China health and retirement longitudinal study (CHARLS). Int J Epidemiol. (2014) 43:61–8. doi: 10.1093/ije/dys203
21. Wen, X, Wang, M, Jiang, CM, and Zhang, YM. Anthropometric equation for estimation of appendicular skeletal muscle mass in Chinese adults. Asia Pac J Clin Nutr. (2011) 20:551–6.
22. Yang, M, Hu, X, Wang, H, Zhang, L, Hao, Q, and Dong, B. Sarcopenia predicts readmission and mortality in elderly patients in acute care wards: a prospective study. J Cachexia Sarcopenia Muscle. (2017) 8:251–8. doi: 10.1002/jcsm.12163
23. Gao, K, Cao, LF, Ma, WZ, Gao, YJ, Luo, MS, Zhu, J, et al. Association between sarcopenia and cardiovascular disease among middle-aged and older adults: findings from the China health and retirement longitudinal study. EClinicalMedicine. (2022) 44:101264. doi: 10.1016/j.eclinm.2021.101264
24. Hu, H, Fukunaga, A, Yokoya, T, Nakagawa, T, Honda, T, Yamamoto, S, et al. Non-high-density lipoprotein cholesterol and risk of cardiovascular disease: the Japan epidemiology collaboration on occupational health study. J Atheroscler Thromb. (2022) 29:1295–306. doi: 10.5551/jat.63118
25. Li, H, Li, C, Wang, A, Qi, Y, Feng, W, Hou, C, et al. Associations between social and intellectual activities with cognitive trajectories in Chinese middle-aged and older adults: a nationally representative cohort study. Alzheimers Res Ther. (2020) 12:115. doi: 10.1186/s13195-020-00691-6
26. Di Angelantonio, E, Gao, P, Pennells, L, Kaptoge, S, Caslake, M, Thompson, A, et al. Lipid-related markers and cardiovascular disease prediction. JAMA. (2012) 307:2499–506. doi: 10.1001/jama.2012.6571
27. Wang, N, Chen, M, and Fang, D. Relationship between serum triglyceride to high-density lipoprotein cholesterol ratio and sarcopenia occurrence rate in community-dwelling Chinese adults. Lipids Health Dis. (2020) 19:248. doi: 10.1186/s12944-020-01422-4
28. Liu, J, Oorloff, MD, Nadella, A, Guo, P, Ye, M, Wang, X, et al. Association between the non-high-density lipoprotein cholesterol to high-density lipoprotein cholesterol ratio (NHHR) and cardiovascular outcomes in patients undergoing percutaneous coronary intervention: a retrospective study. Lipids Health Dis. (2024) 23:324. doi: 10.1186/s12944-024-02309-4
29. Gu, X, Li, Y, Chen, S, Yang, X, Liu, F, Li, Y, et al. Association of Lipids with Ischemic and Hemorrhagic Stroke. Stroke. (2019) 50:3376–84. doi: 10.1161/STROKEAHA.119.026402
30. Li, H, Qian, F, Zuo, Y, Yuan, J, Chen, S, Wu, S, et al. U-shaped relationship of high-density lipoprotein cholesterol and incidence of Total, ischemic and hemorrhagic stroke: a prospective cohort study. Stroke. (2022) 53:1624–32. doi: 10.1161/STROKEAHA.121.034393
31. Yetukuri, L, Söderlund, S, Koivuniemi, A, Seppänen-Laakso, T, Niemelä, PS, Hyvönen, M, et al. Composition and lipid spatial distribution of HDL particles in subjects with low and high HDL-cholesterol. J Lipid Res. (2010) 51:2341–51. doi: 10.1194/jlr.M006494
32. Rohatgi, A, Westerterp, M, von Eckardstein, A, Remaley, A, and Rye, KA. HDL in the 21st century: a multifunctional roadmap for future HDL research. Circulation. (2021) 143:2293–309. doi: 10.1161/CIRCULATIONAHA.120.044221
33. Mazidi, M, Mikhailidis, DP, and Banach, M. Associations between risk of overall mortality, cause-specific mortality and level of inflammatory factors with extremely low and high high-density lipoprotein cholesterol levels among American adults. Int J Cardiol. (2019) 276:242–7. doi: 10.1016/j.ijcard.2018.11.095
34. Xiang, Q, Tian, F, Xu, J, Du, X, Zhang, S, and Liu, L. New insight into dyslipidemia-induced cellular senescence in atherosclerosis. Biol Rev Camb Philos Soc. (2022) 97:1844–67. doi: 10.1111/brv.12866
35. Bilski, J, Pierzchalski, P, Szczepanik, M, Bonior, J, and Zoladz, JA. Multifactorial mechanism of sarcopenia and Sarcopenic obesity. Role of physical exercise, microbiota and Myokines. Cells. (2022) 11:160. doi: 10.3390/cells11010160
36. Nishikawa, H, Fukunishi, S, Asai, A, Yokohama, K, Nishiguchi, S, and Higuchi, K. Pathophysiology and mechanisms of primary sarcopenia (review). Int J Mol Med. (2021) 48:156. doi: 10.3892/ijmm.2021.4989
37. Di Giacomo, BF, Bosco, G, Di Marco, M, Scilletta, S, Miano, N, Musmeci, M, et al. Evaluation of glycemic status and subclinical atherosclerosis in familial hypercholesterolemia subjects with or without LDL receptor mutation. Cardiovasc Diabetol. (2025) 24:126. doi: 10.1186/s12933-025-02683-y
Keywords: NHHR, sarcopenia, lipid metabolism, dyslipidemia, Chinese population
Citation: Sun C, Jiang H, Zhu H, Luo Z and Wang S (2025) Association between the non-high-density lipoprotein cholesterol to high-density lipoprotein cholesterol ratio and sarcopenia: evidence from CHARLS. Front. Public Health. 13:1585986. doi: 10.3389/fpubh.2025.1585986
Edited by:
Lindsay McDermott, University of Bedfordshire, United KingdomReviewed by:
Hengyi Xu, The University of Texas at Austin, United StatesFrancesco Di Giacomo Barbagallo, University of Catania, Italy
Copyright © 2025 Sun, Jiang, Zhu, Luo and Wang. This is an open-access article distributed under the terms of the Creative Commons Attribution License (CC BY). The use, distribution or reproduction in other forums is permitted, provided the original author(s) and the copyright owner(s) are credited and that the original publication in this journal is cited, in accordance with accepted academic practice. No use, distribution or reproduction is permitted which does not comply with these terms.
*Correspondence: Shiyi Wang, c2hpeWl3YW5nMjAyNUAxMjYuY29t