- School of Economics and Management, Ningbo University of Technology, Ningbo, China
Currently, many cities in China are facing the problem of difficult medical care and expensive medical care, while at the same time a large number of hospitals are on the verge of closing. However, existing literature is unable to adequately explain these phenomena. To fill this gap, this study focuses on the causes of the structural imbalance of medical resources in China from the perspective of population mobility and the governance pathways of digital empowerment. By analyzing the entire country and the port-developed provinces with advanced medical conditions, several key findings are revealed: (1) Different types of medical resources in cities exert differentiated attraction effects on population mobility. High-tier hospitals exert a strong pull on population mobility, while the impact of primary care facilities is not evident; (2) Continuous population movements lead to a structural imbalance of medical resource, characterized by overburdened high-tier hospitals coexisting with underutilized primary care facilities; (3) Economic development has increased people’s emphasis on health and prevention of critical illnesses, making the aforementioned structural imbalance more pronounced in the port-developed provinces of China; (4) Digital empowerment offers a new perspective for addressing these issues. By introducing digital diagnostic devices in primary care facilities, the likelihood of major diseases can be swiftly assessed. This technology not only enables intelligent triage but also effectively mitigates the structural imbalance of medical resources.
1 Introduction
Medical resources are essential for urban and regional development. They not only improve people’s physical health but also boost the economy by creating a healthier population (1–3). Studies show that hospitals are at the core of medical resources and can be divided into primary care facilities and high-tier hospitals (4–6). Recently, both types of hospitals in China have seen rapid growth. According to data from the National Bureau of Statistics of China, the total number of healthcare institutions in China was 990,248 in 2015, and by 2024 this number had increased to 1,092,000, which reflects China’s robust expansion in healthcare infrastructure and its emerging prominence on the global stage.
In China, medical resources have been facing serious structural imbalances in recent years. On the one hand, there are a huge number of high-tier hospitals being overcrowded in many cities, making it difficult for patients to get timely appointments (7). On the other hand, primary care facilities often see very few patients, which lead to doctors and medical equipment being underutilized (8, 9). For example, many people have never sought treatment at a primary care facilities throughout their entire lives in some international cities such as Shanghai. To put it more dramatically, there are many children living in cities who cannot even imagine what a primary care facilities looks like (10, 11). As a result, this structure imbalance of medical resource will not only negatively impact the physical well-being of the population, but also hinder the development of the healthcare industry. In order to explore the causes of this phenomenon, researchers have found several factors: First, the rapidly aging population has led to an increase in the number of consultations at high-end hospitals (12, 13). This is mainly because the immunity of the older adult is much worse than that of the young. Second, as the public awareness of health issues rises, more people are seeking high-quality, personalized medical services (14). This shift adds even more pressure on these hospitals (15, 16). Third, cultivating medical professionals takes a considerable amount of time, which means that the supply of qualified professionals often lags behind the expanding market demand (17, 18). In China, the duration of study for an undergraduate medical degree is 5 years, and for a master’s degree graduate student, it reaches 4 years, which is far longer than students of other majors. Fourth, the construction of modern advanced hospitals requires substantial investment, but due to the sluggish real estate market and the continuous decline in government land transfer revenues, persistent fiscal deficits have limited government’s spending on medical infrastructure (19–21).
While previous studies have looked into the reasons behind the shortage of high-tier hospitals in cities from various angles, there are still some important gaps need to be filled. First, many studies overlooked why primary care facilities are underutilized. Second, most scholars neglect that factors such as population aging often lead to a gradual shortage of high-tier hospitals (22–24), while the shortage in China occurred at an alarming rate. This discrepancy suggests that there may be other reasons behind these issues.
With the acceleration of China’s urbanization, population mobility can be one possible driver. According to the 7th National Census of China, the migrant population accounts for 26.6% of the total population. This phenomenon has been a notable part of China’s growth over the last 40 to 50 years since the reforms began in 1978 (25, 26). Early on in China’s economic boom, people primarily moved from inland areas to the coastal areas, attracted by the prospect of higher salaries (27, 28). In the 1980s and early 1990s, the Pearl River Delta region experienced a remarkable influx of people from all over the country. Today, Guangdong Province still leads China in population growth, with cities like Dongguan, Shenzhen, and Zhongshan hosting over half of the area’s permanent residents. However, this pattern have changed notably after the per capita GDP exceeded CNY 70,000 (approximately $9,589, based on an exchange rate of 7.3 CNY/USD). Currently, people’s desire to boost their life expectancy is motivating them to move to cities that provide improved transportation, healthcare, and educational resources (29), which may help explain the sudden and unexpected shortage of high-tier hospitals in China.
In view of this gap, this paper attempts to construct an ERGM model under a systematic analysis framework focused on do people’s preferences for different types of hospitals lead to population mobility and then identifies the impact of population mobility on the structure imbalance of medical resources in China, analyzing the methods for solving the above problem. Compared with the existing literature, this paper made the following contributions. First, it constructed ERGM models to identify the effects of different types of hospitals on population mobility. Second, it illustrated how population mobility has led to a structural imbalance in medical resources over time. Third, it proposed a digital empowerment approach to address these challenges.
2 Concept definition and study hypothesis
2.1 Medical resources
When discussing medical resources, we generally refer to an interconnected system including hospitals, doctors, technology, and more (30, 31). Narrowing the focus, we can primarily define medical resources as hospitals, which are the most critical component (32). This study mainly refers to medical resources in a narrower view, which specifically means hospitals.
Based on the different populations served, hospitals can be classified into two categories: primary care facilities and high-tier hospitals (33). Specifically, primary care facilities focus on delivering basic medical services for common illnesses, while high-tier hospitals mainly focus on handle more complex illnesses. In most cases, the medical equipment at primary care facilities is more basic (like X-ray machines), and high-tier hospitals mainly apply cutting-edge technology (like CT scanners and MRI machines). In recent years, some high-tier hospitals in major cities in China, like Shanghai, have even started using robotic-assisted surgical devices to enhance the efficiency of surgeries (34, 35). In this study, primary care facilities refer to community health service centers, township hospitals, and first-level hospitals in Chinese cities, while high-tier hospitals include second-and third-level hospitals, specialized hospitals, as well as some international or high-end private hospitals.
2.2 Medical resource allocation and population mobility
Whether in developed or developing countries, population mobility is a widespread phenomenon. Unlike natural population growth, population mobility tends to exert sudden shocks on the preexisting structural imbalance of medical resource. Accordingly, this paper adopts a population mobility perspective to investigate the primary causes of structural imbalances of medical resource in Chinese cities.
Recent studies show that population mobility patterns in China have changed significantly (36, 37). Back in the 1980s and early 1990s, as outlined by Lewis’s theory of a dual urban–rural economic structure, most people moved from rural areas to cities, primarily driven by income differences. Many individuals sought better wages and often relocated from inland regions to coastal areas, where thriving exports usually meant higher salaries (38). Since China’s reform and opening up in 1978, coastal regions have gradually developed a labor-intensive, export-oriented industrial structure, thanks in part to their geographical advantages and favorable policies compared to inland areas. However, as economic development continues and living standards improve, the traditional pattern of rural-to-urban and inland-to-coastal population mobility has gradually slowed (39). It has been replaced by a trend in which populations concentrate in large cities offering superior infrastructure, education, and medical resource (40, 41).
As people increasingly prioritize quality of life, different types of hospitals are becoming more distinct in their appeal, which is influencing where people choose to live. This growing focus on quality of life has made higher-tier hospitals quite rare, which in turn makes them more valuable (42). In Chinese port-developed provinces, many city has only a few top-tier hospitals, which makes them more attractive to people. Thus, this study proposed the following hypotheses:
H1a: More primary care facilities in a city will not really boost its appeal for attracting people.
H1b: Conversely, more high-tier hospitals are likely to make a city much more appealing to potential newcomers.
2.3 Structural imbalance of medical resources
From our earlier discussion, in some cities, the need for high-tier hospitals often grows much faster than what you’d expect from just the natural increase in population. However, training a medical professional takes a long time, and building hospital facilities often requires a lot of money, which makes it tough to quickly scale up. When demand spikes but supply cannot keep up, we would facing serious shortages of high-tier hospitals. In China, if you want to register at a high-end hospital, you typically need to wait several hours at least. Moreover, with more and more people using apps to make appointments, there is an increasing phenomenon of “traffic jam” in high-tier hospitals (43, 44). Meanwhile, primary care facilities are still not being used to their full potential in various cities throughout China. And as more people flock to areas with plentiful high-quality medical resources, this structure imbalance will only worsen, thus creating a self-perpetuating cycle. This is why, when we pass by a primary care facility on the street in Chinese cities, we might notice that there are hardly any patients inside, and doctors and nurses might even be dozing off at desks or playing on their phones.
This issue is not only widespread across the country but is especially noticeable in economically developed regions. Much of this is tied to the rapid growth of China’s economy, which has significantly improved overall living standards and raised public awareness about health issues. Research has shown a strong positive relationship between levels of economic development and health concerns (45, 46). According to the latest data from the National Bureau of Statistics of China, the per capita GDP in 2024 reached 95,700 RMB, approximately 13,700 USD (based on an assumed exchange rate of 1:7). In the port-developed provinces along the coast, the level of economic development is leading, and residents’ attention to health issues is expected to be even more pronounced. Zhejiang Province, recognized as the most economically advanced port province, reported a per capita GDP of 135,600 RMB in 2024, far exceeding the national average. Meanwhile, Zhejiang Province has become a pilot province for “common prosperity” due to its port advantages. Therefore, the phenomenon of structural imbalance of medical resources will be more pronounced in these port-developed provinces.
Given this reasoning, this study proposed the following hypotheses:
H2a: The population mobility driven by high-tier hospitals will result in a structural imbalance of medical resources in China.
H2b: The structural imbalance mentioned above will be more evident in port-developed provinces.
2.4 Digital empowerment
Although most people choose to receive treatments at high-tier hospitals, the majority of patients visiting these facilities in China are diagnosed with routine illnesses (47), which creates a paradox: even though primary care facilities can effectively treat routine illnesses, patients are willing to spend excess time and money at high-tier hospitals. To explain the above phenomenon, some studies have suggested that people are often willing to take measures to reduce their risk of critical illnesses when faced with health concerns (48–50).
Therefore, considering the above viewpoints, this study held that primary care facilities need digital tools using machine learning to diagnose diseases more accurately. By this method, those with routine issues will be able to receive care at local health service centers while significant disease risk cases can be referred to higher-tier hospitals (51, 52). Thus, we proposed the following research hypothesis:
H3: Digital empowerment of primary care facilities can help balance out the different kinds of medical resources in China.
To sum up, the complete research framework is shown in Figure 1. In this framework, “+” represents a promoting effect, “++” represents an even stronger promoting effect, and “−” represents an inhibitory effect.
3 Research methodology
3.1 Data collection
In this study, we examine how the structural imbalance of medical resources in China occurs from the perspective of population mobility. The data on population mobility comes from two sources. The data for 2023 comes from the population mobility data provided by Amap, and the data for 2017 was collected from the large-scale national migration sample survey (CMDS) carried out by the National Health Commission of the People’s Republic of China. To further obtain the data on medical resources, we download a large amount of data from the websites of the Health Committees of each sample cities in China. At the same time, we also search a great amount of data from the China Health Statistics Yearbook. Recognizing that population mobility will be influenced by other various factors in reality, we further collect data from the China Urban Statistical Yearbook and the Zhejiang Statistical Yearbook.
Specifically, the population mobility data from Gaode Map in 2023 mainly reflects the population movement between prefecture-level cities, which covers the movement of people between 288 prefecture-level cities across China. It should be noted that in China, a prefecture-level city (like Hangzhou City in Zhejiang Province) is a type of city whose scale lies between provinces (like Zhejiang Province) and county-level cities (like Chunan County in Hangzhou city, Zhejiang Province).
In order to figure out whether the structural imbalance of medical resources caused by population mobility is more severe in port-developed provinces with relatively higher levels of economic development and health awareness (the hypothesis H2b), we choose Zhejiang Province as our another research sample. This is because Zhejiang province is renowned for its developed port trade and is one of the most economically developed provinces in China. In this part, population mobility primarily occurs between the 88 county-level cities in Zhejiang Province. It is important to note that in China, a province consists of multiple prefecture-level cities, and each prefecture-level city consists of several county-level cities. Therefore, county-level cities can be regarded as the smallest city units.
Figure 2 demonstrates the transformation process from micro-level data at the individual level in CMDS survey to macro-level data at the city level. In this study, all population mobility data from the CMDS survey were organized into an edge list format, arranged according to the movement of individual i from city x to city y. As shown in Figure 2, the data at the city level is an aggregation of the individual data. For example, the population mobility from city A to city B is measured by counting how many individuals in the edge list moved from city A to city B.
3.2 Method selection
The Exponential Random Graph Model (ERGM) is the core analytical method of this study, which is used to examine hypotheses H1 and H2. In academia, some scholars have used this model to analyze interpersonal relationship networks, such as research collaboration among the scholars (53). Besides, some researchers have also used this method to analyze trade networks between countries across the World (54–56). The reason the ERGM model is widely used in network analysis is that it offers a robust way to analyze how multiple factors shape network formation (57, 58). It generally describes the probability distribution of the network based on p influencing factors, where the normalizing constant “k(θ)” makes sure that the probability mass function “pθ(x)” sums to 1 across the entire network.
The ERGM model constructed in this study is formulated as follows. In this formulation, z₁(x), z₂(x), and z₃(x) represent the covariates for the network’s endogenous structure, individual member attributes, and exogenous networks, respectively (59, 60). First, endogenous structural variables are mainly used to test the regular structural patterns that emerge during network formation. Second, individual attribute variables measure how node characteristics shape network formation and are divided into sender and receiver effects. The former explores whether a stronger attribute makes a node more inclined to form connections with others, while the latter evaluates whether a salient attribute increases the likelihood that a node will be connected by others. Third, exogenous network variables are aimed to assess whether other external networks influence the formation of the focal network.
Therefore, θ₁, θ₂, and θ₃ are used to measure which kind of variable will significantly influence the network formation (Table 1). In our study, the number of primary care facilities and the number of top-tier hospitals in a city are individual attribute variables. When the coefficient of the variable in Table 1 is statistically significant, the factor corresponding to which would thus be conducive to attracting population to the city.
Besides, to validate hypotheses H2 and H3, this study further apply network centrality analysis by using a mix of tools, including UCINET, ArcGIS, and R.
3.3 Variable measurement
In our ERGM model, the dependent variable is the population mobility network, while the independent variables are categorized into three types (Table 2).
This study selected Edges as its indicator. Specifically, if the coefficient of Edges is significantly negative, the formation of the population mobility network can be considered non-random.
In terms of Individual Attribute Variables, we chose the number of primary care facilities (Nodeicov.PCF) and high-tier hospitals (Nodeicov.HTH) in cities to evaluate their reception effects. At the same time, population mobility can be affected by a range of factors, including economic development level (Nodeicov.GDP) (61), urbanization rate (Nodeicov.UR), environmental quality (Nodeicov.ENV), internet infrastructure development (Nodeicov.INT), and education level (Nodeicov.EDU). The reason for selecting the above control variables is that studies have shown that factors such as environmental quality, internet connectivity, and educational resources will significantly affect population mobility as the living standards improve (62, 63).
In terms of network covariates, we selected geographic proximity effects (Edgecov.GeoProx) and cultural proximity effects (Edgecov.CulProx) as control variables. First, geographic proximity is measured by the inverse of the Euclidean distance between cities. Second, cultural proximity is assessed based on whether the dialects spoken between cities are the same. If two cities share the same dialect, they are marked as having a strong cultural connection. In contrast, if they reside in different dialect regions, they are marked as having a weaker cultural relationship. Typically, people tend to move between areas that share cultural similarities.
4 Data analysis and results
4.1 ERGM analysis at the national level
In the ERGM analysis at the national level, three different models were constructed in Table 3. The results from the analysis indicated that Model 3 had the lowest scores for both the Akaike Information Criterion (AIC) and Bayesian Information Criterion (BIC), which suggests that Model 3 demonstrates the highest goodness of fit.
In Model 3, the coefficient of primary care facilities is not significant (Nodeicov.PCF = 0.0001, p > 0.05). The result has validated Hypothesis H1a, which suggests that cities with a greater number of primary care facilities do not exhibit a noticeable attraction for population mobility. In contrast, the coefficient of high-tier hospitals is significantly positive (Nodeicov.HTH = 0.0122, p < 0.001), indicating that cities with a larger number of high-tier hospitals have a notable appeal for population inflow, thus supporting hypothesis H1b. High-tier hospitals can effectively cater to the people’s demand for increasingly higher quality of life. Because of this, they play a vital role in drawing people to a city.
The result of the control variables are consistent with the findings from previous studies. First, the coefficient of Edges is significantly negative (Edges = −5.0613, p < 0.001), indicating that the formation of the population mobility network is not random. Second, the coefficient of Nodeicov.GDP is significantly positive (Nodeicov.GDP = 0.0000, p < 0.001), indicating that economically developed cities tend to attract more people compared to underdeveloped areas. Third, the coefficient of Nodeicov.UR is significantly negative (Nodeicov.UR = −0.0001, p < 0.01), indicating that cities with higher urbanization rates have a lower attraction for population inflow. This may be because these cities often have higher population densities, which will lead to an increased level of congestion. Fourth, the coefficient of Nodeicov.ENV is also significantly negative (Nodeicov.ENV = −0.0132, p < 0.001), which implies that cities suffering from poor air quality will see a notable decline in their attractiveness for new residents. This is because the incidence and mortality rates of lung cancer are the highest among all cancers in China, and air quality is an important factor influencing the incidence of lung cancer. Besides, both of Nodeicov.INT and Nodeicov.EDU have significantly positive coefficients (Nodeicov.INT = 0.0049, p < 0.001; Nodeicov.EDU = 0.0503, p < 0.001), which suggests that cities with rich internet infrastructure and educational resources are much more appealing to populations.
As population mobility continues, the demand for high-tier medical resources is rising rapidly, far exceeding the growth rate of demand brought about by the natural population growth rate of within 3% per year. This is mainly because population mobility between cities in China is unrestricted, meaning that a city could see an influx of over a hundred thousand or even a million people in a single year. However, because the supply of high-tier hospitals is rigid, it’s not feasible to quickly build a large number of them within a short period. As a result, it will become difficult to respond quickly to the surge in demand, which leads to a supply shortage of high-tier hospitals. Meanwhile, cities with a large amount of primary care facilities fail to attract population mobility. Even if the population increases for some reason, people will seek high-tier medical resources and are unlikely to visit primary care facilities, which would ultimately lead to a surplus in the supply of primary care facilities.
Figure 3 clearly illustrates the structural imbalance of medical resources mentioned above. In 2021, there were a total of 34.86 million patients who had received diagnosis and treatment in high-tier hospitals in China, while only 3.978 million patients received diagnosis and treatment in primary care facilities. Interestingly, the bed occupancy rate in high-tier hospitals sits at 78.2%, while primary care facilities lag behind at just 52.1%. In Chinese cities, many primary care hospitals have opted to close temporarily during the day due to a lack of patients, which leaves many healthcare workers with no choice but to seek other ways to earn a living, such as doing delivery jobs or providing domestic help. Consequently, hypothesis H2a has been validated.
4.2 ERGM analysis at the provincial level
In this part, we chose Zhejiang Province as our research sample. The main reason is that Zhejiang Province is one of the regions in China that has achieved economic prosperity and even become a first pilot area for common prosperity across China due to the development of its ports and international trade. This case primarily aims to test hypothesis H2b, which examine whether the structural imbalance of medical resources is more apparent in port-developed provinces.
The analysis at the provincial level includes three models, but it should be noted that due to the less comprehensive statistical data at the provincial level compared to the national level, the number of control variables in Table 4 is lower than that in Table 3. Model 1 includes most of the variables other than geographic proximity (Edgecov.GeoProx) and cultural proximity (Edgecov.CulProx). Model 2 introduces geographical proximity and Model 3 further adds cultural proximity as an control variable. The empirical results show that Model 3 achieves the lowest AIC and BIC values, which indicates that it provides the best fit for the data. According to the results of Model 3, the coefficient of primary care facilities remains insignificant (Nodeicov.PCF = 0.0004, p > 0.05), while the coefficient of high-tier hospitals remains significantly positive (Nodeicov.HTH = 0.0513, p < 0.001), consistent with the estimates presented in Table 3. This further validates hypotheses H1a and H1b, which state that different types of medical resources exert differentiated attraction effects on population mobility.
The signs of the other control variables align with those in Table 3. Notably, the coefficient of Nodeicov.GDP has lost its significance (Nodeicov.GDP = 0.0001, p > 0.05), which may be due to the balanced economic development across cities in Zhejiang Province. Generally speaking, population mobility tends to occur between regions with significant economic disparities, rather than between areas that are relatively similar. In other words, just as sufficient force is required to overcome static friction and initiate the motion of an object, two cities must exhibit significant economic differences to drive population mobility. Besides, the coefficient of Edgecov.CulProx is significantly negative (Edgecov.CulProx = −0.2533, p < 0.05). This may be due to the higher education level and the widespread use of Mandarin in Zhejiang Province.
4.3 Mechanism analysis in port-developed provinces
Further analysis is shown in Figure 4. In the Yangtze River Delta urban agglomeration in China, there are three provinces and one province-level municipality, namely Shanghai, Jiangsu, Zhejiang, and Anhui. Among these areas, Shanghai, Jiangsu, and Zhejiang are coastal regions with ports and relatively developed economies, while Anhui is an inland region without a port and exhibits slower economic development. It is clear that people in port-developed areas pay much more attention to health compared to those in inland areas. For example, in terms of individual commercial medical insurance purchases, the level in port-developed areas exceeds 200 individuals per 10,000 people. However, the rate is only 133 per 10,000 in Anhui Province. Similarly, the number of critical illness medical insurance purchases per 10,000 unemployed people is far higher in port regions than in the inland areas. In short, economic development has made people pay more attention to disease prevention, which will results in a more pronounced structural imbalance of medical resources in port-developed provinces.
As shown in Figure 5, the bed occupancy rates of community health service centers and clinics in 2021 in China were 42.69 and 37.86%, which are far below the provincial average of 76.3% and significantly lower than the 52.1% occupancy rate of primary care facilities shown in Figure 3. This indicates that the structural imbalance of medical resources is even more pronounced in the economically and port-developed Zhejiang Province compared to other regions across the country. Therefore, hypothesis H2b is validated, which indicates that economic development has intensified people’s focus on health and their preference for high-tier hospitals and further amplified the structural imbalance in the port-developed provinces of China.
To illustrate the mechanisms mentioned above dynamically, this study further conducted the following analysis.
As shown in Figure 6, the left side shows the amount of primary care facilities of each county-level city in Zhejiang Province, while the right side shows the amount of high-tier hospitals. It’s clear that the southwestern part of Zhejiang has a higher concentration of primary care facilities, as shown by the overall reddish tone on the map, which stands out in comparison to the predominantly orange areas in the north. However, when we look at the right chart, it reveals that the southwestern region has significantly fewer high-tier hospitals, represented by a yellow hue on the map, which is dramatically less than the red areas in the northern part of the province.
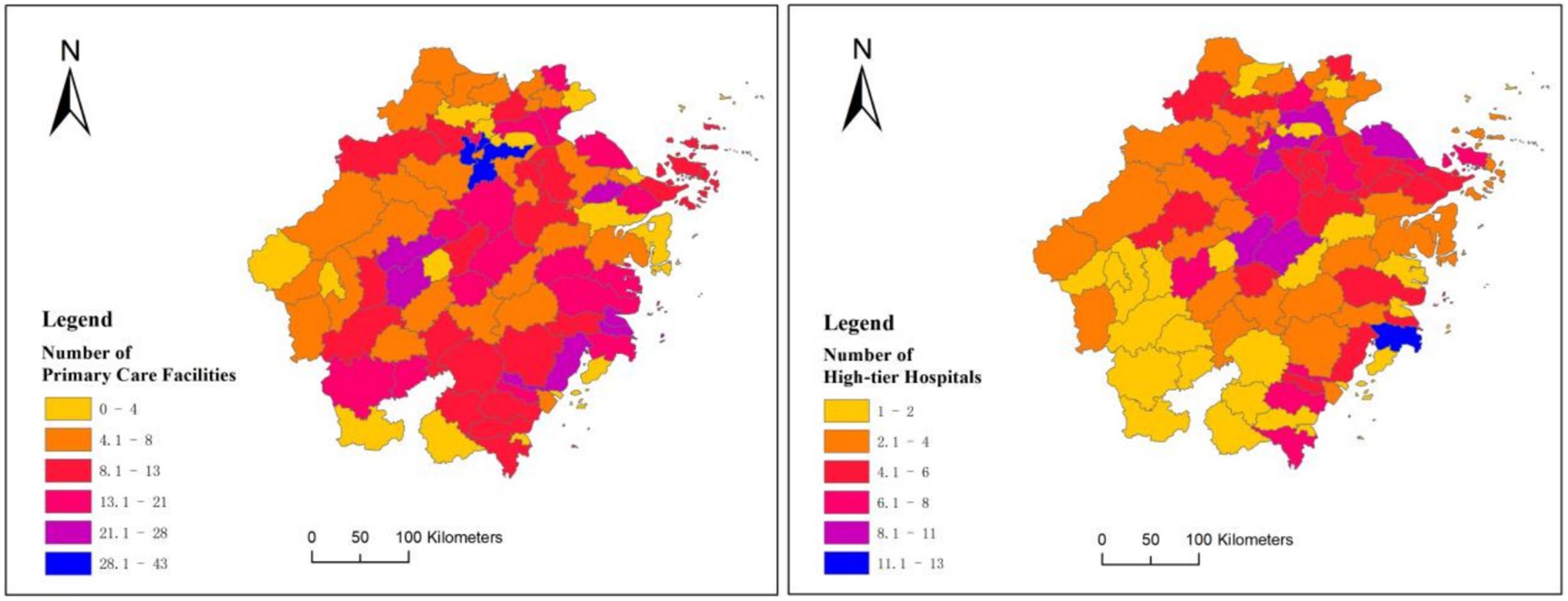
Figure 6. Distribution of primary care facilities and high-tier hospitals in cities of Zhejiang Province.
Figure 7 further displays the standardized in-degree centrality of population movement across various cities in Zhejiang Province. It’s apparent that the northern region shows higher standardized in-degree centrality compared to the southwestern area. For example, it has reached above 0.068 in regions such as Ningbo and Hangzhou. More importantly, the color distribution pattern we have seen in Figure 7 aligns well with the color distribution pattern seen on the right side of Figure 6, which further validate hypotheses H1 and H2.
At the same time, the population mobility shown in Figure 7 will further exacerbate the structural imbalance of medical resources in Zhejiang Province. As more people move into the northern part of Zhejiang Province, the southwestern region is experiencing population outflow, exacerbating the structural imbalances in medical resources across the province. In some county-level cities in Zhejiang Province, high-tier hospitals have captured almost 80% of patients, leaving only a handful for primary care facilities.
4.4 The role of digital empowerment
In this section, Table 5 showcases data conducted by researchers at Zhongnan University of Economics and Law from 2016 to 2022 (64). Notably, about 76.4% among the respondents believe that the quality of medical equipment is lacking. Figure 8 further illustrates the distribution of medical equipment valued over 10,000 yuan in primary care facilities and high-tier hospitals in China in 2022. It is clear that high-tier hospitals possess significantly more medical devices valued at this amount compared to primary care facilities. At the same time, as the value of the equipment increases, we can see a noticeable decline in the proportion of devices found in primary care facilities. Specifically, among equipment valued at less than 500,000 yuan, the share of equipment owned by primary care facilities accounts for 18% of the total. This share decreases to 14% for equipment valued between 500,000 and 990,000 yuan, and further drops to just 6% for equipment over 1 million yuan. As a result, primary care facilities face a significant service capacity gap. Consequently, it is no surprise that many people hesitate to seek diagnoses and treatments there.
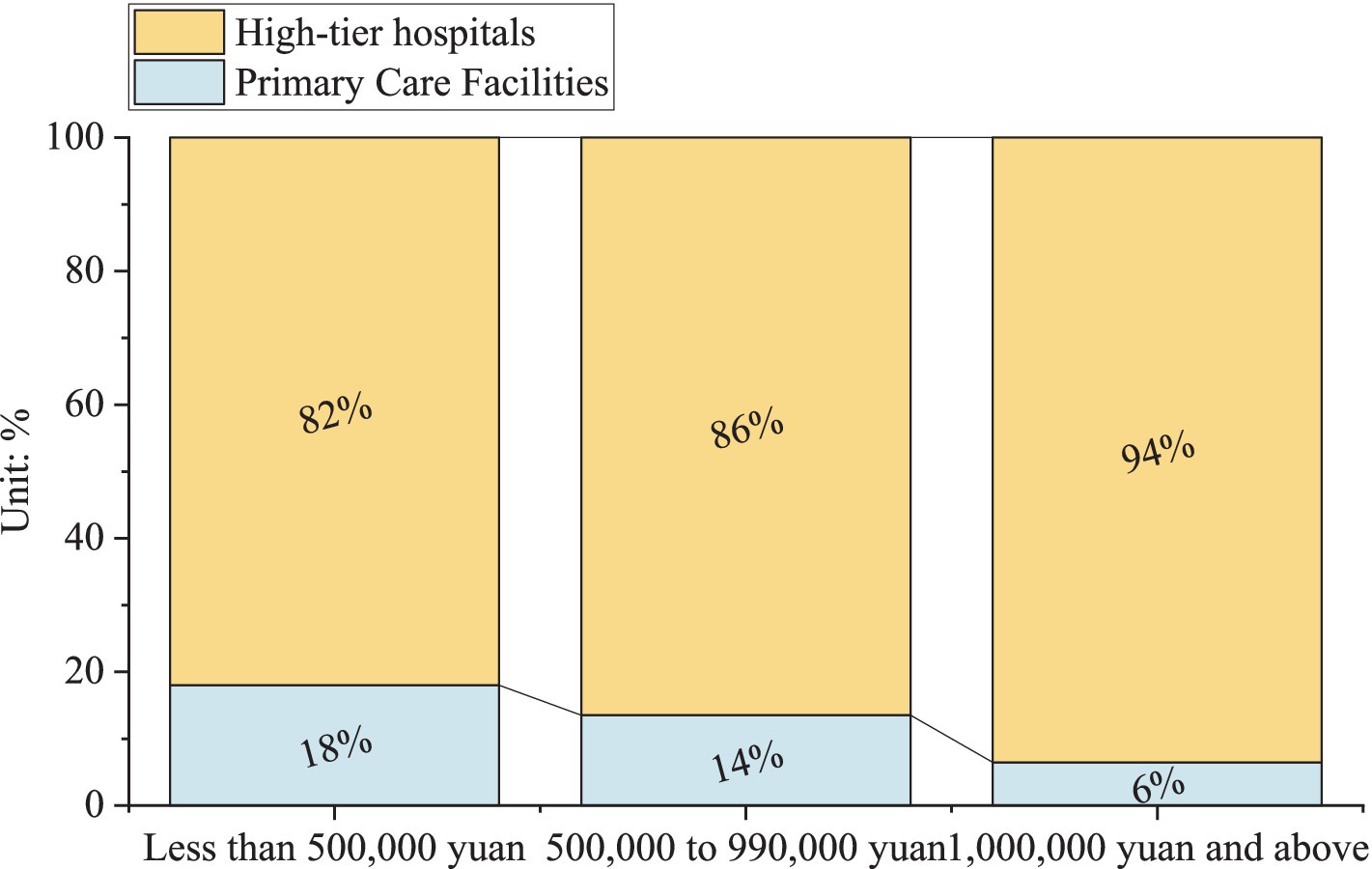
Figure 8. Distribution of medical equipment valued over 10,000 yuan in primary care facilities and high-tier hospitals in China in 2022.
Therefore, digital empowerment may be at work. This is because digital devices employed in machine learning-based risk probability models can meet patients’ needs for managing health risks (65–67). As primary care facilities can provide diagnostic services comparable to those of high-tier hospitals, this will ultimately help to correct the imbalance in the structure of medical resources in China.
For instance, at the Fengxian Community Health Service Center in Shanghai, an AI-assisted imaging ultrasound device was introduced in 2025. Using this machine for examinations is like having an doctor nearby constantly giving audio and visual alerts when abnormalities are detected. Since this device was introduced, over 1,000 patients have undergone ultrasound screenings for breast cancer as of April. Through this approach, this center is able to generate substantial income and makes healthcare more convenient for local residents.
Another example is the Pozi Street Community Health Service Center in Changsha. Powered by DeepSeek’s large-model computing and deep-learning capabilities, the system performs real-time data analysis to track patients’ health metrics, promptly flag abnormal readings early warnings about potential major disease. So far, the center has handled a total of 29,048 face-to-face consultations, which has raised patient satisfaction to 97% and greatly enhanced the appeal of primary care facilities. Thus, hypothesis H3 is validated.
5 Conclusions and policy implications
In China and its port-developed provinces, a large number of high-tier hospitals are experiencing severe shortages, while primary care facilities remain underutilized. To explore its underlying causes, this paper presented a research framework to examine how population mobility contributes to this phenomenon and propose strategies to address the issue.
Research have shown that high-tier hospitals play a vital role in meeting people’s needs for health investments and risk management, thereby can help a region attract a large inflow of population. In comparison, the role of primary care facilities is not as remarkable. The ERGM models conducted on 288 prefecture-level cities in China and 88 county-level cities in Zhejiang Province have both confirmed this mechanism. More crucially, unlike natural population growth, population mobility will create a sudden and explosive impact on the existing supply and demand market for medical resources. Consequently, high-tier hospitals will face severe supply shortages, while primary care facilities often experience apparent supply surpluses. In addition, since higher economic growth often signifies greater public health awareness, this structural imbalance of medical resources is often more pronounced in port-developed provinces in China (68, 69). This is because port areas often have well-established medical and transportation facilities (7, 70–72). Therefore, people are more inclined to seek the best medical conditions, which exacerbates the differences in the attraction of various types of medical resources to the population.
Further empirical analysis have indicated that the aforementioned phenomenon mainly stems from primary-level hospitals’ inability to provide advanced diagnostic and treatment equipment. As a result, most individuals end up seeking treatment for even minor health issues (like colds) at more advanced hospitals (73, 74). To tackle this problem, digital empowerment could be the key. Through AI-assisted diagnosis and remote expert consultations, primary care hospitals can compensate for their technological shortcomings and rebuild patient trust. This, in turn, will enable patients with common ailments to receive treatment on-site while reducing the long waiting times and costs typically associated with high-tier hospitals. In addition, for more serious condition, the patient can be quickly referred to higher-tier hospitals for specialized care.
In China, the closure of numerous primary care facilities has forced society to reevaluate the logic of medical resource distribution, prompting people to question: Is primary healthcare doomed to become a victim? It should be noted that the essence of any hospital is to safeguard lives, not to engage in cold market competition (75, 76). After all, the older adult and chronic disease patients who remain in remote areas also need accessible medical services. Nowadays, many developing countries around the world are also confronting these issues, especially in the context of accelerating population aging (77–79). Therefore, it is crucial to harness the latest advancements in artificial intelligence and introduce digital tools in primary care facilities, which can enhance patient triage and improve the overall efficiency of healthcare services.
Data availability statement
The data analyzed in this study is subject to the following licenses/restrictions: the raw data supporting the conclusions of this article will be made available by the authors, without undue reservation. Requests to access these datasets should be directed to bGpqXzIwMThhdXR1bW5AMTYzLmNvbQ==.
Author contributions
HF: Writing – original draft, Methodology, Conceptualization, Writing – review & editing, Supervision. JL: Visualization, Data curation, Writing – review & editing, Writing – original draft.
Funding
The author(s) declare that financial support was received for the research and/or publication of this article. The work was supported by the Provincial Philosophy and Social Sciences Foundation of Zhejiang Province (under the grant 25NDJC136YB: Mechanisms and Pathways for Enhancing the Resilience of Port Supply Chains through Digital Empowerment), and supported by the Provincial-Municipal Cooperation Project of the Provincial Philosophy and Social Sciences Foundation of Zhejiang Province (under the grant 24SSHZ052YB: Characteristics, Evolution, and Implications of Urban Youth Population Mobility Networks in the Digital Economy Era).
Acknowledgments
The research purpose, plan, methodology, content, and implementation process of this study have complied with all relevant ethical requirements of Ningbo University of Technology. Explicit permission is granted for subsequent studies building on this research, provided that any follow-up research strictly adheres to the original study protocol.
Conflict of interest
The authors declare that the research was conducted in the absence of any commercial or financial relationships that could be construed as a potential conflict of interest.
Generative AI statement
The author(s) declare that no Gen AI was used in the creation of this manuscript.
Publisher’s note
All claims expressed in this article are solely those of the authors and do not necessarily represent those of their affiliated organizations, or those of the publisher, the editors and the reviewers. Any product that may be evaluated in this article, or claim that may be made by its manufacturer, is not guaranteed or endorsed by the publisher.
References
1. Hu, M, Tao, CH, and Zhou, H. The influence of industrial structure upgrade on coupling and coordinated development-empirical analysis from Chinese pharmaceutical manufacturing and pharmaceutical service industries. Front Public Health. (2021) 9:722006. doi: 10.3389/fpubh.2021.722006
2. Tang, ZW, Ye, CX, Fu, Z, Zhang, JR, and Gong, ZP. Primary medical and health preparedness and people's life satisfaction in China: the mediating role of satisfaction with medical and health services. Front Public Health. (2023) 11:1037574. doi: 10.3389/fpubh.2023.1037574
3. Zamzam, AH, Al-Ani, AKI, Wahab, AKA, Lai, KW, Satapathy, SC, Khalil, A, et al. Prioritisation assessment and robust predictive system for medical equipment: a comprehensive strategic maintenance management. Front Public Health. (2021) 9:782203. doi: 10.3389/fpubh.2021.782203
4. Kim, JH, Bell, GA, Bitton, A, Desai, E, Hirschhorn, LR, Makumbi, F, et al. Health facility management and primary health care performance in Uganda. BMC Health Serv Res. (2022) 22:275. doi: 10.1186/s12913-022-07674-3
5. Zhang, AW, Nikoloski, Z, Albala, SA, Yip, WN, Xu, J, and Mossialos, E. Patient choice of health care providers in China: primary care facilities versus hospitals. Health Syst Reform. (2020) 6:e1846844. doi: 10.1080/23288604.2020.1846844
6. Zhang, T, Xu, YJ, Ren, JP, Sun, LQ, and Liu, CJ. Inequality in the distribution of health resources and health services in China: hospitals versus primary care institutions. Int J Equity Health. (2017) 16:42. doi: 10.1186/s12939-017-0543-9
7. Wu, M, and Liu, C. The impact of long-term care insurance on hospital bed utilization. Economics (Quarterly). (2024) 24:1867–82. doi: 10.13821/j.cnki.ceq.2024.06.10
8. Pan, J, Zhang, TF, Zhang, YM, Lin, XJ, Li, WW, Song, C, et al. Intelligent and digital driven high-quality development of medical and health service system: development mechanism and implementation path. J Sichuan Univ (Med Ed). (2024) 55:1055–62. doi: 10.12182/20240960401
9. Zhang, Y, You, YH, and Wang, GL. Model study of the integration of physical education and medicine in the intervention of chronic diseases under the “healthy China” initiative. Health Econ Res. (2021) 38:23-25+29. doi: 10.14055/j.cnki.33-1056/f.2021.10.005
10. Li, CL, Chen, Z, and Khan, MM. Bypassing primary care facilities: health-seeking behavior of middle age and older adults in China. BMC Health Serv Res. (2021) 21:895. doi: 10.1186/s12913-021-06908-0
11. Zhang, WW, Ung, COL, Lin, GH, Liu, J, Li, WJ, Hu, H, et al. Factors contributing to Patients' preferences for primary health care institutions in China: a qualitative study. Front Public Health. (2020) 8:414. doi: 10.3389/fpubh.2020.00414
12. Dall, TM, Gallo, PD, Chakrabarti, R, West, T, Semilla, AP, and Storm, MV. An aging population and growing disease burden will require a large and specialized health care workforce by 2025. Health Aff. (2013) 32:2013–20. doi: 10.1377/hlthaff.2013.0714
13. Lakin, KC, and Burke, MM. Looking forward: research to respond to a rapidly aging population. Res. Pract. Pers. Sev. Disabil. (2019) 44:280–92. doi: 10.1177/1540796919882356
14. Minvielle, E. Toward customized care comment on "(re) making the procrustean bed? Standardization and customization as competing logics in healthcare". Int J Health Policy Manag. (2018) 7:272–4. doi: 10.15171/ijhpm.2017.84
15. Palangkaraya, A, and Yong, J. Population ageing and its implications on aggregate health care demand: empirical evidence from 22 OECD countries. Int J Health Care Finance Econ. (2009) 9:391–402. doi: 10.1007/s10754-009-9057-3
16. Yang, SZ, Qiu, YG, Huang, X, Kang, L, Xie, J, and Chen, LL. Information technology practice and effectiveness evaluation in the outpatient and emergency departments of a certain hospital. China Hospital. (2024) 28:75–7. doi: 10.19660/j.issn.1671-0592.2024.5.18
17. Orton, M, Agarwal, S, Muhoza, P, Vasudevan, L, and Vu, A. Strengthening delivery of health services using digital devices. Global Health-Sci Pract. (2018) 6:S61–71. doi: 10.9745/ghsp-d-18-00229
18. Wu, HB, Xie, A, and Wang, WM. Eight-year medical education program: retrospect and prospect of the high-level medical talent training in China. ECNU Rev Educ. (2021) 4:190–209. doi: 10.1177/2096531120957665
19. Chen, MK, Chen, T, Ruan, DB, and Wang, XW. Land finance, real estate market, and local government debt risk: evidence from China. Land. (2023) 12:1597. doi: 10.3390/land12081597
20. Coccia, M, and Benati, I. Negative effects of high public debt on health systems facing pandemic crisis: lessons from COVID-19 in Europe to prepare for future emergencies. Aims Public Health. (2024) 11:477–98. doi: 10.3934/publichealth.2024024
21. Federspiel, F, Borghi, J, and Martinez-Alvarez, M. Growing debt burden in low-and middle-income countries during COVID-19 may constrain health financing. Glob Health Action. (2022) 15:2072461. doi: 10.1080/16549716.2022.2072461
22. Jakovljevic, M. Population ageing alongside health care spending growth. Srp Arh Celok Lek. (2017) 145:534–9. doi: 10.2298/sarh160906113j
23. Mai, YH, Peng, XJ, and Chen, W. How fast is the population aging in China? Asian Popul Stud. (2013) 9:216–39. doi: 10.1080/17441730.2013.797295
24. Vogelsang, EM, Raymo, JM, Liang, J, Kobayashi, E, and Fukaya, T. Population aging and health trajectories at older ages. J Gerontol B Psychol Sci Soc Sci. (2019) 74:1245–55. doi: 10.1093/geronb/gbx071
25. Shen, JF. Increasing internal migration in China from 1985 to 2005: institutional versus economic drivers. Habitat Int. (2013) 39:1–7. doi: 10.1016/j.habitatint.2012.10.004
26. Wei, YD, Wu, YY, Liao, FH, and Zhang, L. Regional inequality, spatial polarization and place mobility in provincial China: a case study of Jiangsu province. Appl Geogr. (2020) 124:102296. doi: 10.1016/j.apgeog.2020.102296
27. Qi, W, Abel, GJ, and Liu, SH. Geographic transformation of China's internal population migration from 1995 to 2015: insights from the migration centerline. Appl Geogr. (2021) 135:102564. doi: 10.1016/j.apgeog.2021.102564
28. Wu, LL. Correlation between population migration and regional planning based on urbanization of coastal cities. J Coast Res. (2020) 110: 50–53. doi: 10.2112/jcr-si110-012.1
29. Weili, Z, Zhijuan, H, Yibin, W, and Ruibo, W. Spatial network and influencing factors of population flow in urban agglomeration. Geogr Sci. (2023) 43:72–81. doi: 10.13249/j.cnki.sgs.2023.01.008
30. Frenk, J, Chen, L, Bhutta, ZA, Cohen, J, Crisp, N, Evans, T, et al. Health professionals for a new century: transforming education to strengthen health systems in an interdependent world. Lancet. (2010) 376:1923–58. doi: 10.1016/s0140-6736(10)61854-5
31. Kruk, ME, Gage, AD, Arsenault, C, Jordan, K, Leslie, HH, Roder-DeWan, S, et al. High-quality health systems in the sustainable development goals era: time for a revolution. Lancet Global Health. (2018) 6:E1196–252. doi: 10.1016/s2214-109x(18)30386-3
32. Xing, W. Practical exploration and policy reflections on the development of the health services industry. Macroecon Manage. (2014) 6:29–31. doi: 10.19709/j.cnki.11-3199/f.2014.06.012
33. Wang, TY, Wen, K, Gao, QM, and Sun, RC. Small money, big change: the distributional impact of differentiated doctor's visit fee on healthcare utilization. Soc Sci Med. (2023) 339:116355. doi: 10.1016/j.socscimed.2023.116355
34. Li, L, Lin, L, Xu, HS, Zhang, Y, and Chai, G. New productive force: the preliminary report of first craniofacial surgical robot IST multicenter clinical trial in China. J Craniofac Surg. (2025) 36:21–5. doi: 10.1097/scs.0000000000010992
35. Xie, FF, Zhang, QC, Mu, CY, Zhang, Q, Yang, HZ, Mao, JY, et al. Shape-sensing robotic-assisted bronchoscopy (SS-RAB) in sampling peripheral pulmonary nodules. J Bronchology Interv Pulmonol. (2024) 31:e0981. doi: 10.1097/lbr.0000000000000981
36. Xu, HG, and Wu, YF. Lifestyle mobility in China: context, perspective and prospects. Mobilities. (2016) 11:509–20. doi: 10.1080/17450101.2016.1221027
37. Zhang, XY, Zheng, MR, Wang, PP, Ma, Y, and Zheng, XQ. Analyzing disruptions in post-pandemic population return patterns: a network perspective on Chinese cities. Appl Geogr. (2024) 173:103448. doi: 10.1016/j.apgeog.2024.103448
38. Chan, KW, and Wei, Y. Two systems in one country: the origin, functions, and mechanisms of the rural-urban dual system in China. Eurasian Geogr Econ. (2019) 60:422–54. doi: 10.1080/15387216.2019.1669203
39. Guan, WH, Wu, XN, Li, HL, Zhang, H, Wu, W, and Wu, LX. Regional urbanization patterns and evolution mechanisms in China since the reform and opening-up. Geogr Sci. (2025) 45:265–77. doi: 10.13249/j.cnki.sgs.20230576
40. Cheng, M, Yin, Z, and Westlund, H. Counterurbanization in China? A case study of counties in Huang-Huai-Hai area from the perspective of urban-rural relations. J Rural Stud. (2024) 110:103386. doi: 10.1016/j.jrurstud.2024.103386
41. Peng, BL, and Ling, L. Health service behaviors of migrants: a conceptual framework. Front Public Health. (2023) 11:1043135. doi: 10.3389/fpubh.2023.1043135
42. Shah, AK, Shafir, E, and Mullainathan, S. Scarcity frames value. Psychol Sci. (2015) 26:402–12. doi: 10.1177/0956797614563958
43. Bo, Y, Ben Liu, Q, and Tong, Y. The effects of adopting Mobile health and fitness apps on hospital visits: quasi-experimental study. J Med Internet Res. (2023) 25:e45681. doi: 10.2023/1/e45681
44. Zhao, PJ, Li, SX, and Liu, D. Unequable spatial accessibility to hospitals in developing megacities: new evidence from Beijing. Health Place. (2020) 65:102406. doi: 10.1016/j.healthplace.2020.102406
45. Li, SH, and Zong, J. Economic development, social security fiscal expenditure and Residents' health: an examination of adverse selection behavior. Macroecon Res. (2018) 11:26–43. doi: 10.16304/j.cnki.11-3952/f.2018.11.003
46. Zhang, XC, Wang, GY, Ma, JW, and Bai, HJ. The impact of income level on skeletal muscle health in rural Chinese older residents: a study of mediating effects based on dietary knowledge. Front Public Health. (2024) 12:1329234. doi: 10.3389/fpubh.2024.1329234
47. Yao, M, Qi, JG, Yan, H, Jiang, YW, and Zheng, JQ. Investigation and analysis of outpatient visits at the general pediatrics Department of a Tertiary Hospital in Beijing. Chin Gen Pract. (2015) 18:1288–92.
48. Barsky, A, and Borus, J. Functional somatic syndromes. Ann Intern Med. (1999) 130:910–21. doi: 10.7326/0003-4819-130-11-199906010-00016
49. Chen, LH, Zhang, LY, and Xu, XC. Health behavior and medical insurance under the healthy China strategy: a moral hazard perspective. Front Public Health. (2024) 12:1315153. doi: 10.3389/fpubh.2024.1315153
50. Ferrer, R, and Klein, WM. Risk perceptions and health behavior. Curr Opin Psychol. (2015) 5:85–9. doi: 10.1016/j.copsyc.2015.03.012
51. Chang, HY, Jung, CK, Woo, JI, Lee, S, Cho, J, Kim, SW, et al. Artificial intelligence in pathology. J Pathol Transl Med. (2019) 53:1–12. doi: 10.4132/jptm.2018.12.16
52. Olawade, DB, Wada, OJ, David-Olawade, AC, Kunonga, E, Abaire, O, and Ling, JAT. Using artificial intelligence to improve public health: a narrative review. Front Public Health. (2023) 11:1196397. doi: 10.3389/fpubh.2023.1196397
53. He, CC, Liu, FZ, Dong, K, Wu, J, and Zhang, QP. Research on the formation mechanism of research leadership relations: an exponential random graph model analysis approach. J Informet. (2023) 17:101401. doi: 10.1016/j.joi.2023.101401
54. Gutiérrez-Moya, E, Lozano, S, and Adenso-Díaz, B. Analysing the structure of the global wheat trade network: an ERGM approach. Agronomy-Basel. (2020) 10:1967. doi: 10.3390/agronomy10121967
55. Jang, Y, and Yang, JS. The dynamics of the EU’s nuclear trade network: an ERGM analysis. Struct Chang Econ Dyn. (2022) 63:470–7. doi: 10.1016/j.strueco.2022.07.002
56. Li, J, Liu, KY, Yang, ZX, and Qu, Y. Evolution and impacting factors of global renewable energy products trade network: an empirical investigation based on ERGM model. Sustain For. (2023) 15:8701. doi: 10.3390/su15118701
57. Ghafouri, S, and Khasteh, SH. A survey on exponential random graph models: an application perspective. PeerJ Comput Sci. (2020) 6:e269. doi: 10.7717/peerj-cs.269
58. van der Pol, J. Introduction to network modeling using exponential random graph models (ERGM): theory and an application using R-project. Comput Econ. (2019) 54:845–75. doi: 10.1007/s10614-018-9853-2
59. Krivitsky, PN, Morris, M, Hunter, DR, and Klumb, C. Ergm 4: new features for analyzing exponential-family random graph models. J Stat Softw. (2023) 105:1–44. doi: 10.18637/jss.v105.i06
60. Yaveroglu, Ö, Fitzhugh, SM, Kurant, M, Markopoulou, A, Butts, CT, and Przulj, N. Ergm.Graphlets: a package for ERG modeling based on Graphlet statistics. J Stat Softw. (2015) 65:1–29. doi: 10.18637/jss.v065.i12
61. Moomaw, RL, and Shatter, AM. Urbanization and economic development: a bias toward large cities? J Urban Econ. (1996) 40:13–37. doi: 10.1006/juec.1996.0021
62. Kokubun, K. Factors that attract the population: empirical research by multiple regression analysis using data by prefecture in Japan. Sustain For. (2022) 14:1595. doi: 10.3390/su14031595
63. Zhai, X, and Luo, Y. Can urban internet development attract labor force? Evidence from Chinese cities. Sustainability. (2025) 17:260. doi: 10.3390/su17010260
64. Song, G.H. (2022). The influence of township hospital medical service capacity on rural elderly People's choice of healthcare institutions. Master's Thesis, Central South University of Economics and Law.
65. Li, MF, Jiang, YY, Zhang, YZ, and Zhu, HS. Medical image analysis using deep learning algorithms. Front Public Health. (2023) 11:1273253. doi: 10.3389/fpubh.2023.1273253
66. Villalobos-Quesada, M, Ho, KD, Chavannes, NH, and Talboom-Kamp, E. Direct-to-patient digital diagnostics in primary care: opportunities, challenges, and conditions necessary for responsible digital diagnostics. Eur J Gen Pract. (2023) 29:2273615. doi: 10.1080/13814788.2023.2273615
67. Zaidan, AM. The leading global health challenges in the artificial intelligence era. Front Public Health. (2023) 11:1328918. doi: 10.3389/fpubh.2023.1328918
68. Wang, LY, Hu, ZY, Chen, HX, Zhou, CF, Tang, ML, and Hu, XY. Differences in regional distribution and inequality in health workforce allocation in hospitals and primary health centers in China: a longitudinal study. Int J Nurs Stud. (2024) 157:104816. doi: 10.1016/j.ijnurstu.2024.104816
69. Zhang, ZT. Survey and analysis on the resource situation of primary health care institutions in rural China. Front Public Health. (2024) 12:1394527. doi: 10.3389/fpubh.2024.1394527
70. Li, S, Haralambides, H, and Zeng, QC. Economic forces shaping the evolution of integrated port systems – the case of the container port system of China's Pearl River Delta. Res Transp Econ. (2022) 94:101183. doi: 10.1016/j.retrec.2022.101183
71. Wang, YF, Li, XY, Xu, K, and Zhang, AS. The impact of high-speed rail connectivity on the linkage between investment and innovation elements. Sci Technol Prog Policy. (2025) 1:1–13.
72. Zhang, QQ, Hu, XZ, Li, ZF, Zhou, YT, and Qi, XL. Port's industry ecosystem construction: empirical evidence from China. Transp Policy. (2025) 163:42–60. doi: 10.1016/j.tranpol.2025.01.005
73. Qing, XL, Chen, XY, and Zhang, JJ. Advances in the application of the internet plus medical model in the management of chronic non-communicable diseases. Clin Med Pract. (2025) 34:211–5. doi: 10.16047/j.cnki.cn14-1300/r.2025.03.015
74. Wang, ZM, and Li, CJ. Application of information technology in hospital medical equipment management and maintenance. China Med Device Inf. (2022) 28:166–9. doi: 10.15971/j.cnki.cmdi.2022.22.008
75. Gould, CC. Solidarity and the problem of structural injustice in healthcare. Bioethics. (2018) 32:541–52. doi: 10.1111/bioe.12474
76. Zhou, M, Zhao, SY, and Fu, MW. Public hospital expansion and overtreatment: evidence from changes in hospital ranking. Econ Sci. (2021) 1:109–21. doi: 10.12088/PKU.jjkx.2021.01.09
77. Kyoon-Achan, G, Phillips-Beck, W, Kinew, KA, Lavoie, JG, Sinclair, S, and Katz, A. Our people, our health: envisioning better primary healthcare in Manitoba first nation communities. Int Indig Policy J. (2021) 12:1–22. doi: 10.18584/iipj.2021.12.1.13561
78. Mackey, TK, and Liang, BA. Rebalancing brain drain: exploring resource reallocation to address health worker migration and promote global health. Health Policy. (2012) 107:66–73. doi: 10.1016/j.healthpol.2012.04.006
Keywords: medical resources, structural imbalance, population mobility, digital empowerment, China, port-developed provinces
Citation: Fu H and Lu J (2025) Structural imbalance of medical resources amid population mobility and digital empowerment: a study of national and port-developed provinces in China. Front. Public Health. 13:1613293. doi: 10.3389/fpubh.2025.1613293
Edited by:
Tao Zhang, Macao Polytechnic University, Macao SAR, ChinaReviewed by:
Jiang Ziran, Zhejiang Normal University, ChinaGuiyun Liu, Ningbo University, China
Shuangbo Liang, Chinese Academy of Sciences (CAS), China
Copyright © 2025 Fu and Lu. This is an open-access article distributed under the terms of the Creative Commons Attribution License (CC BY). The use, distribution or reproduction in other forums is permitted, provided the original author(s) and the copyright owner(s) are credited and that the original publication in this journal is cited, in accordance with accepted academic practice. No use, distribution or reproduction is permitted which does not comply with these terms.
*Correspondence: Junjie Lu, bGpqXzIwMThhdXR1bW5AMTYzLmNvbQ==