- 1Soil Biogeochemistry, Martin Luther University Halle-Wittenberg, Halle, Germany
- 2Department of Computational Landscape Ecology, Helmholtz Centre for Environmental Research UFZ, Leipzig, Germany
Given the multiple challenges agriculture faces today, approaches that ensure both food security and the sustainable use of agroecosystems are urgently needed. The concept of community supported agriculture (CSA) is a promising attempt to address all three sustainability dimensions, but empirical research is still limited. Energy efficiency of farming systems is one important aspect when describing their ecological sustainability. This case study compares three CSA farms with three conventional farms, all focusing on vegetable production. Life cycle assessment (LCA) methodology was used to incorporate all relevant energy flows related to vegetable production, including all upstream activities from cradle to farmgate. CSA-farms showed energy return of investment (EROI) factors of 0.13–0.44, while EROI of conventional farms was between 0.02 and 0.69. Energy inputs, particularly fuels, electricity and fertilizer were major determinants, and related to size and structure of farms, while high yield could partially compensate for high energy inputs. CSA farms thereby tended to show relatively low consumption of fossil energy sources, partly due to on-farm electricity production by photovoltaic. Therefore, the performance of CSA regarding EROI of non-renewable energy sources (NRE) was relatively higher (0.17–0.76 compared to 0.05–0.78 for conventional farms). To further improve the energy efficiency, CSA farms need to improve their balance of inputs and outputs (e. g. reduced consumption of fossil fuels without compromising yields). However, CSA reached high energy efficiency if lifecycle costs of distribution were included (EROI = 0.6–3.1), which is likely to be lower in conventional farms with long supply chains and heavy processing. Moreover, CSA also provides additional ecological (e.g., fostering biodiversity, reduction of food loss and waste), social (e.g., education, transparency) and economic benefits (e.g., guaranteed sales). These benefits, as well as a more comprehensive assessment of energy efficiency of different production systems including more farms, need to be considered to better understand the potential contribution of CSA to a transformation toward sustainable food systems.
1 Introduction
Agriculture, responsible for emitting between 20 and 31% of all anthropogenic emissions, is a key driver of climate change (Tubiello et al., 2022). Agriculture is also relevant in the context of planetary boundaries, a concept used to measure the resilience of the Earth System. Several impact processes have already exceeded their limits, with agriculture being a major driver of this development (Caesar et al., 2024; Campbell et al., 2017). Agriculture plays a critical role in the processes of “land-system change” and “freshwater use” and has been particularly influential in the transgression of “biosphere integrity” and “biogeochemical flows” (Campbell et al., 2017). Therefore, it is needed that agricultural politics balance food security and the sustainability of agroecosystems. European politics emphasize organic agriculture (OA) as a crucial component for promoting a more sustainable agricultural sector. Aligned with the common agricultural policy (CAP) and the European green deal, EU officials decided to increase the share of arable land under organic production to 25% by the year 2030 (European Commission, 2023).
Meanwhile, scientific research is multifarious when it comes to the question of how to balance production and ecology. Expressions like “ecological, sustainable, and agroecological intensification” are extensively discussed and the land sharing versus land sparing controversy is apparent (Loconto et al., 2020; Wezel et al., 2015). Some would promote intensive conventional farming that leaves separated areas to biodiversity conservation, while others would encourage the less naturalistic approach of more extensive farming which tries to preserve non-agricultural flora and fauna while sustaining a certain level of production. The conversion to OA under constant demand is seen as a threat toward food security that would increase land consumption (Meemken and Qaim, 2018). Indeed, the average yield gap between conventional and organic farming is reported to be about 20%, while being less high for vegetables (Ponisio et al., 2015; De Ponti et al., 2012). But the relation of yield to arable land is only one aspect to be considered. To reveal more about the efficiency of farming, the required inputs of different production systems are assessed in this study. By doing so, the strengths and weaknesses as well as the potentials and limits of the respective approaches to farming are pointed out. This includes the approach of community supported agriculture. CSA is a promising concept to address ecological, social and economic sustainability aspects (Egli et al., 2023). While a fair amount of research has already been done on the sociopolitical dimensions of CSA, the level of its ecological and economic sustainability is still uncertain (Egli et al., 2023). In CSA, a group of people cover the entire production costs of a farm by upfront payments. The so-called “prosumers” thereby split both risks and harvest (Flieger, 2016; Saltmarsh et al., 2011). While CSA is still in a niche, the number of CSA farms has increased rapidly in many regions of the world. Agroecological approaches and voluntary manual labor, both widespread in CSA, offer the potential to reduce yield gap and increase energy efficiency.
This case study contributes to this sparsely explored research field. To date, several studies used the life cycle assessment (LCA) methodology to document the environmental impacts of on-farm vegetable production (Kashyap et al., 2023; Stone et al., 2021; Habibi et al., 2019; Jianyi et al., 2015; Markussen et al., 2014). Some LCAs are specifically focused on the comparison of organic versus conventional farming, predominantly indicating OA to have lower impacts on human health and the environment (Kumar et al., 2023; Foppa Pedretti et al., 2021; Kowalczyk and Cupiał, 2020; Tasca et al., 2017). Further research has been done to compare the energy ratio of vegetable production in organic and conventional cropping systems (Ronga et al., 2019; He et al., 2016; Brodt et al., 2013; Bos et al., 2007; Williams et al., 2006; Röver et al., 2000). However, only two studies focused on CSA farming in comparison to other approaches. Pérez-Neira and Grollmus-Venegas (2018) compared the energy efficiency of CSAs and conventional farms in Andalucía, describing CSA farming as an economically and energetically competitive alternative to conventional farming. Within the cradle-to-farm gate approach they detected an energy return of investment (EROI) of 0.57 for the CSA farm, which lies between 0.45 and 0.66 of the conventional farms. Second, Zhen et al. (2020) analyzed greenhouse vegetable production of conventional, organic and CSA farms in the Beijing area. They found CSA farms not only being more profitable and eco-efficient than organic and conventional farms, but also having the lowest environmental impact of the three systems. So far, no study has conducted a comparative energy efficiency analysis based on detailed CSA data and various conventional farms, each with its unique specialization in vegetable production. Moreover, to the best of our knowledge, no study has applied the LCA methodology to CSA farms in Germany, despite an exponential growth of CSA organizations in the last years (Schmidt et al., 2025). This study addressed this research gap and aimed at investigating the energy efficiency of CSA farms compared to conventional farms, practicing vegetable production with different intensity and in different regions. The goal thereby was to describe the energy and resource use efficiency of these different farming systems, to identify key factors determining the ecological sustainability potential, but also to identify major sources of energy consumption and trade-offs. Therefore, (i) detailed data from farms of different sizes and agricultural systems, covering all relevant on-farm activities, was collected. This included all production inputs as well as the production outputs, i.e., marketed products leaving the farm gate. This data was used to (ii) build an energy balance model using the LCA methodology to identify all energy flows embodied in the different farms and (iii) to compare CSA and conventional systems. The farms were located in Central East Germany. Three out of six farms follow the CSA concept. The remaining farms were conventional farms specialized on vegetable production. Furthermore, one out of these farms implemented so-called “regenerative agriculture,” representing a conventional and ecologically sustainable approach to farming, most notably in respect to soil conservation (Schreefel et al., 2020).
2 Materials and methods
2.1 Collection of data
In the first step, all farmers (CSA and conventional) have been interviewed in person to understand the scope of relevant inputs and outputs. Based on this, input categories were defined and a consistent questionnaire for all the farms was developed and filled in by farmers (for a detailed overview of collected data see Supplementary Appendixes 1–7). CSA farmers collected data based on a previous collaboration with Egli (2024). Conventional farmers only accounted for inputs and outputs related to vegetable production (including green manure, if it was related to vegetable production) and neglected other forms of production, such as grain production.
2.2 Study farms
Six farms were included in this study (Table 1). Three of them followed the CSA approach, the other three produced conventionally. All the CSA farms produced vegetables organically, but only two of them were officially certified by the time of the study. The other three farms used conventional means of farming, although there were substantial differences observable between them. Farm 1 (F1) had a mixed production output and used only about 10% of their arable land for vegetables. Farm 2 (F2) also produced a mix of crops and used almost half of its land for vegetable production. Farm 3 (F3) produced vegetables as well as a few seedlings and horticultural products on a family property located in a residential neighborhood. In terms of size the CSA-farms were rather small, with an arable land between 3.5 ha and 10.5 ha. In contrast, the sizes of the conventional farm were wide-ranging. Except F3 with less than 1 ha of arable land, the conventional farms were larger on average compared to CSA farms.
The farms were located in the central east of Germany (Figure 1). CSA1 was located in the urban area of Dresden, right at the floodplain area of the river Elbe (Figure 1). Like all CSAs portrayed here, CSA1 produced vegetables and fruits exclusively for their registered prosumers. CSA2 was located in the north east of Chemnitz, Saxony. Their farm combined arable land for vegetable production, grasslands and forests. In contrast to the other farms, a lot of pre-production steps were done by the farmers themselves. This included the production of seedlings, organic fertilizers and pesticides. CSA3 was located in the outskirts of Leipzig. At the time of the study, four other CSAs were located within 3 km of this CSA. F1 was located in East-Saxony, close to the town of Görlitz and the border to Poland. The farm management described their farming activity as regenerative agriculture, which mainly focuses on sustainable soil-management, but also on further elements like the use of organic amendments instead of synthetic fertilizers and limited use of pesticides (Schreefel et al., 2020). F2 was by far the largest farm included in the study, representing a higher scaled and professionalized production of a few selected types of vegetables. F3, a rather small farm located in the Saxon-Thuringian border region, was maintained part time by mainly one person, who used the old infrastructure of the family property to produce fine vegetables, which were sold to nearby restaurants and more distant farmer markets.
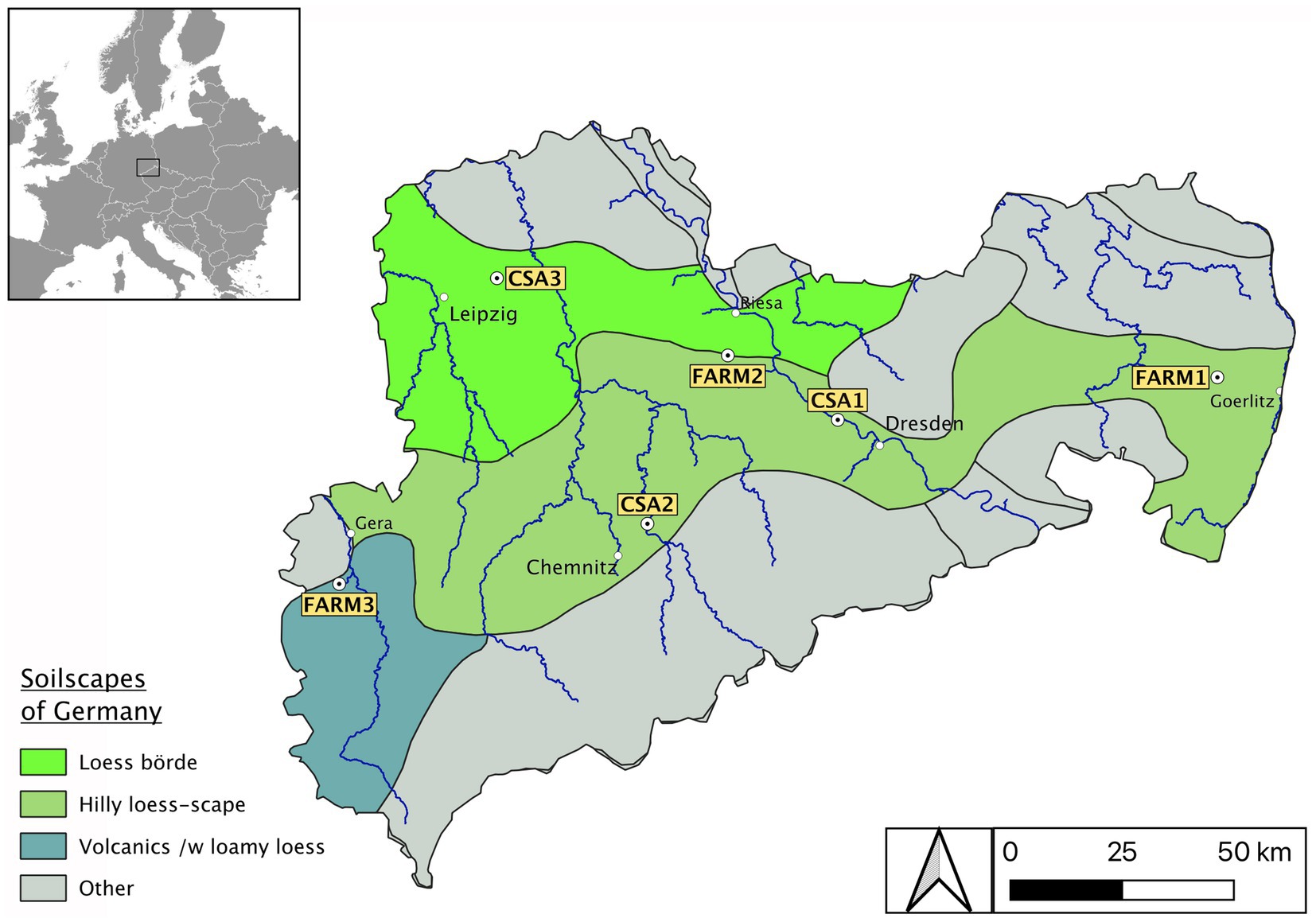
Figure 1. Study area, including relevant soilscapes and rivers. Background data retrieved from ESTAT, 2024b; BGR, 2023; BKG, 2023; BGR, 2013b; Mueller et al., 2007.
2.3 Study area
The study area represents a region with predominantly temperate sub-oceanic to temperate sub continental climate (BGR, 2005). Weather conditions in 2022 were hotter, drier and sunnier compared to the annual average between 1991 and 2020 (Table 2). The farms were affected relatively similarly, as temperature, precipitation and radiation deviated along the same lines from the multi-annual average. Average radiation was similar, while temperature was lower and precipitation higher in the cases of CSA2, F1 and F3.
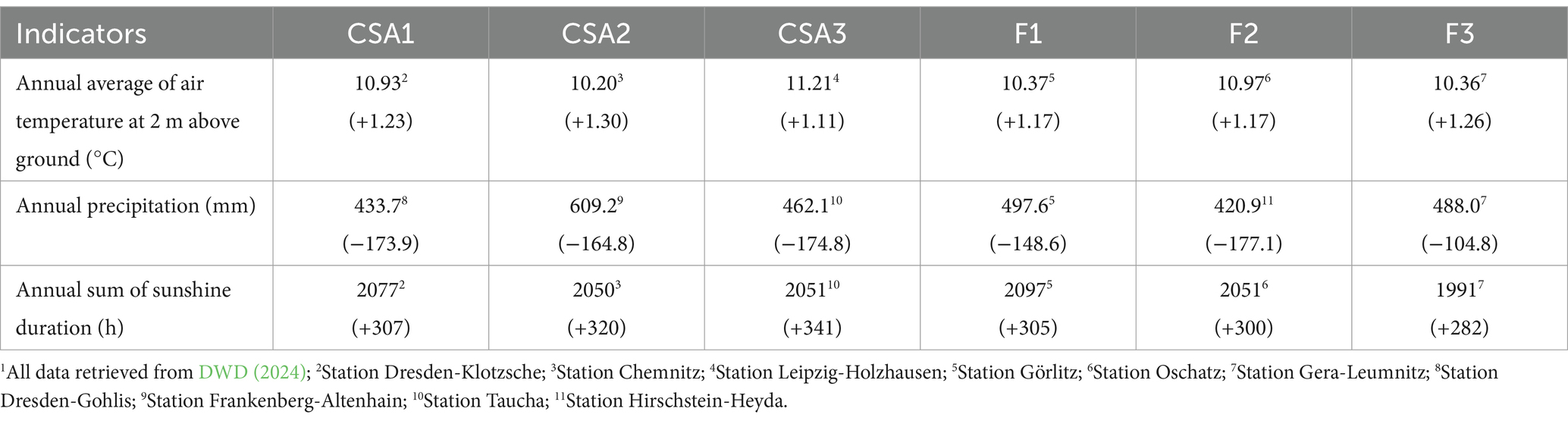
Table 2. Climate data for farm locations in 2022 (and deviation from multi-annual average of 1991–2020)1.
The farms were located in the area of a loess belt, which marks the transition zone from lowlands to the low mountain ranges of the region (ESBN, 2005). According to the world reference base for soil resources (WRB), this zone is characterized by luvisols, albeluvisols, and gleysols (ESBN, 2005; BGR, 2005). It consequently belongs to one of the most fertile and productive cropland regions in Germany and Europe (ESTAT, 2024a). The study farms differed in their predominant soil textures and substrate (Table 2). While CSA3, F1, and F2 were located on a loess substrate with silty clay texture and CSA1 on loamy soil, CSA2 and F3 were located on a coarser sandy soil, and thereby less fertile location (Heinrich and Hergt, 2006).
2.4 Life cycle assessment
To analyze the energy flows that correspond with the input data, we used the LCA methodology. This framework provides a holistic approach to cover all environmental burdens of a product system (Guinée, 2006). LCAs are regulated under ISO 14040 and ISO 14044 including the four stages (1) goal and scope definition, (2) life cycle inventory (LCI), (3) life cycle impact assessment (LCIA) and (4) interpretation (ISO, 2006a,b). The approach is well suited for studying food systems, even though they differ from classical industrial production systems when it comes to the definition of the functional unit, the system boundaries, and the environmental impact categories (Cucurachi et al., 2019; Audsley, 1997).
2.4.1 Goal and scope definition
An LCA of vegetable production on multi-structured farms over an entire year and in one specific region was conducted. The main goal was to establish a comparative energy balance. Therefore, values were harmonized by area (ha) of farmland and mass (kg) of farm produce as two functional units. The assessment was realized within the system boundary, cradle to farm gate (Figure 2). By allocating the processes relevant to the systems of production, an attributional approach was applied. The allocation factor of every product was determined at the point of its substitution (APOS), that is the moment where a product emerges for the first time in the respective supply chain. Farm energy output and farm energy input were defined as impact categories, the latter featuring 12 sub-categories (see Inventory analysis).
All inputs were classified under the single issue LCIA method of the cumulative energy demand (CED), in accordance with the specific focus on energy (Chen et al., 2021). As there are limitations to other environmental impact categories when it comes to comparing different farming systems (especially tracing N-emissions), CED appears to be the most solid tool in this context (Meier et al., 2015).
2.4.2 Inventory analysis
The inventory of energy inputs in this study is composed of 12 categories: seeds and seedlings, fertilizers, crop protection, fuels, lubricants, electricity, irrigation, labor, vehicles and machinery, greenhouses, services, and further inventory (Figure 2). Energy inputs of these categories were calculated based on energy equivalents (EEQ). Farm buildings, except for greenhouse buildings, were not considered. Nevertheless, the inventory of this study is more comprehensive than it is in similar studies investigating the ecological potential of CSA farming (Zhen et al., 2020; Pérez-Neira and Grollmus-Venegas, 2018).
Regarding non-synthetic fertilizers uncontested data was not available (Montemayor et al., 2022). As the actual NPK-contents were available for the majority of applied organic fertilizers, the approach by Hülsbergen et al. (2001) was used to calculate EEQs. Accordingly, the mineral equivalent to the available NPK was ascertained. Equalizing organic and synthetic NPK can be problematic (Meier et al., 2015). However, it was preferred to use precise NPK-contents instead of even more uncertain proxies. Where possible, results still compared to proxies from Ecoinvent 3.3 (Ecoinvent, 2016) and values were similar.
Regarding electricity, consumption was leveled as medium voltage electricity for Germany. Consumption and production were directly balanced. In the case of surplus energy from electricity, 3.6 MJ were credited per extra kWh. The energy required for the production, installation, maintenance, and operation of PV-panels was added separately. Due to a lack of information on the specifics of the PV infrastructure, a scenario of single-crystalline silicon wafers with an estimated life span of 30 years as flat roof installation was adopted (Raugei et al., 2012).
For calculating the energy demand of labor, data from Pimentel et al. (1973) was updated by the average energy intake of a German citizen in 2018, including an appropriate holiday (FAO, 2024). Consequently, an EEQ of 2.8 MJ per working hour was used.
On the output side, the amount of each vegetable produced in 2022 was set off against its energy-equivalent (EEQ) from the BLS database (Hartmann et al., 2014). As already mentioned before, the energy output by electricity production using photovoltaic (PV) was directly set off against the energy input from electricity consumption and therefore appears on the input side.
2.4.3 Impact assessment
The CED measured as higher heating value (HHV) was used as the LCIA method. It represents the primary energy consumption within the life cycle of a product (Frischknecht et al., 2015). The main dataset for measuring the CED of farm inputs was Ecoinvent 3.3 (Ecoinvent, 2016). Additionally, few CED values were obtained from Aguilera et al. (2019). For all system processes, according to their availability, German data (e.g., electricity) was preferred over pan-European or single-European-country data (e.g., diesel and coal production, also polyethylene and polypropylene production) and over “rest of the world” data (e.g., some fertilizer production). For measuring the embodied energy of the output of farms, namely vegetables, the latest available version 3.02 of the German nutrient database (BLS) was used (Hartmann et al., 2014).
CED was measured in two ways, as total embedded energy and as NRE. NRE includes fossil, nuclear and nonrenewable biomass, while the total embedded energy also encompasses wind, solar, geothermal, water, renewable biomass and labor. For the specific case of electricity generation using photovoltaics, the solar energy that flows into the process was omitted, as the farmer has no control over the use or non-use of solar energy. Solar energy was therefore considered as an externality outside the system boundary.
We applied specific EEQs on both output and input factors, in accordance with the work of Pérez-Neira and Grollmus-Venegas (2018). For characterizing the output, the sum of the energy contents of all vegetables sold in 2022 was multiplied with EEQs per farm, respectively, (Equation 1).
with the EEQs being related to raw vegetables and including fibers and cu representing individual cultures.
The input was analyzed by applying EEQs on all the energy inputs, whereby the overall input per farm was measured as CED and CED (NRE) (Equations 2 and 3).
with i representing the individual input categories and f. u. the functional unit ha of farmland or kg of farm produce.
For the interpretation of CED, we offset output and input in multiple ways, such as EROI, NRE EROI, EROI without electricity production by PV and net energy. Regarding the functional unit of ha, areas used for green manure were included. Similarly, areas with crop failures have been included (Equations 4 and 5).
2.5 Sensitivity analysis
We tested the sensitivity of the results by applying a local sensitivity analysis. This was useful in identifying the most volatile parameters and therefore the greatest sources of uncertainty (Markwardt and Wellenreuther, 2016; Saltelli et al., 2004).
The influence on the EROI by modifying the EEQs of both the output and the different input categories were measured per farm. For screening the output, the EEQ of any relevant type of vegetable with a share of at least 2% of sales was assumed to be 0, 50, 150, or 200% of its actual value. This same procedure was applied to the input EEQs to analyze their effect on the total CED (Figure 3). As the most crucial categories were identified, the procedure was repeated with the single input factors of those categories. Consequently, the most sensitive impacts toward the EROI were identified.
3 Results
3.1 Output of farms
In contrast to the conventional farms, CSA farms produced a diverse range of vegetables (Table 3). As all farms cultivated different sets of vegetables, single yields could not be compared in every case (Table 4). In cases which allowed comparison, broad ranges between the farms became visible. CSA3 and F1 showed largest yields for all crops compared, except rhubarb. Yields of CSA1 were high for tomatoes and average for potatoes and carrots. CSA2 typically showed lower yields, except for rhubarb. F2 and F3 had the lowest revenues per ha. In sum, there was no consistent difference between CSA and conventional farms regarding yields.
3.2 Input of farms
The categories fuels, electricity and fertilizers showed the highest impacts on the energy input of farms (Figure 4). The category of fuels thereby had the highest impact among all study farms. This included fuels for vehicles and machinery, as well as fuels for greenhouse heating. The exceptional share of fuels to the CED of F3 is noteworthy (Figure 4). Likewise, electricity consumption showed large effects on the six farms. The volume was partially reduced by PV electricity production, which in those cases (CSA1, CSA2, F2), was directly allocated to the input side. Simultaneously, fertilizers proved to be an important impact category, regardless of the farm’s preferences for synthetic or organic fertilizers. Aside from that, the categories of labor, vehicles and machinery and also inventory showed large impacts on the total energy input of different farms. While labor was mainly an important input for CSA-farms, inventory appeared to be the most crucial category for F2. Within inventory, boxes for storage and transport had by far the highest impact, as they consist of energy intensive materials, such as polyethylene and polypropylene. The categories of crop protection, lubricants and greenhouses had a minor impact. Within crop protection, CSA1 and CSA3 showed the lowest results, while CSA2 needed almost twice the energy intake of CSA3.
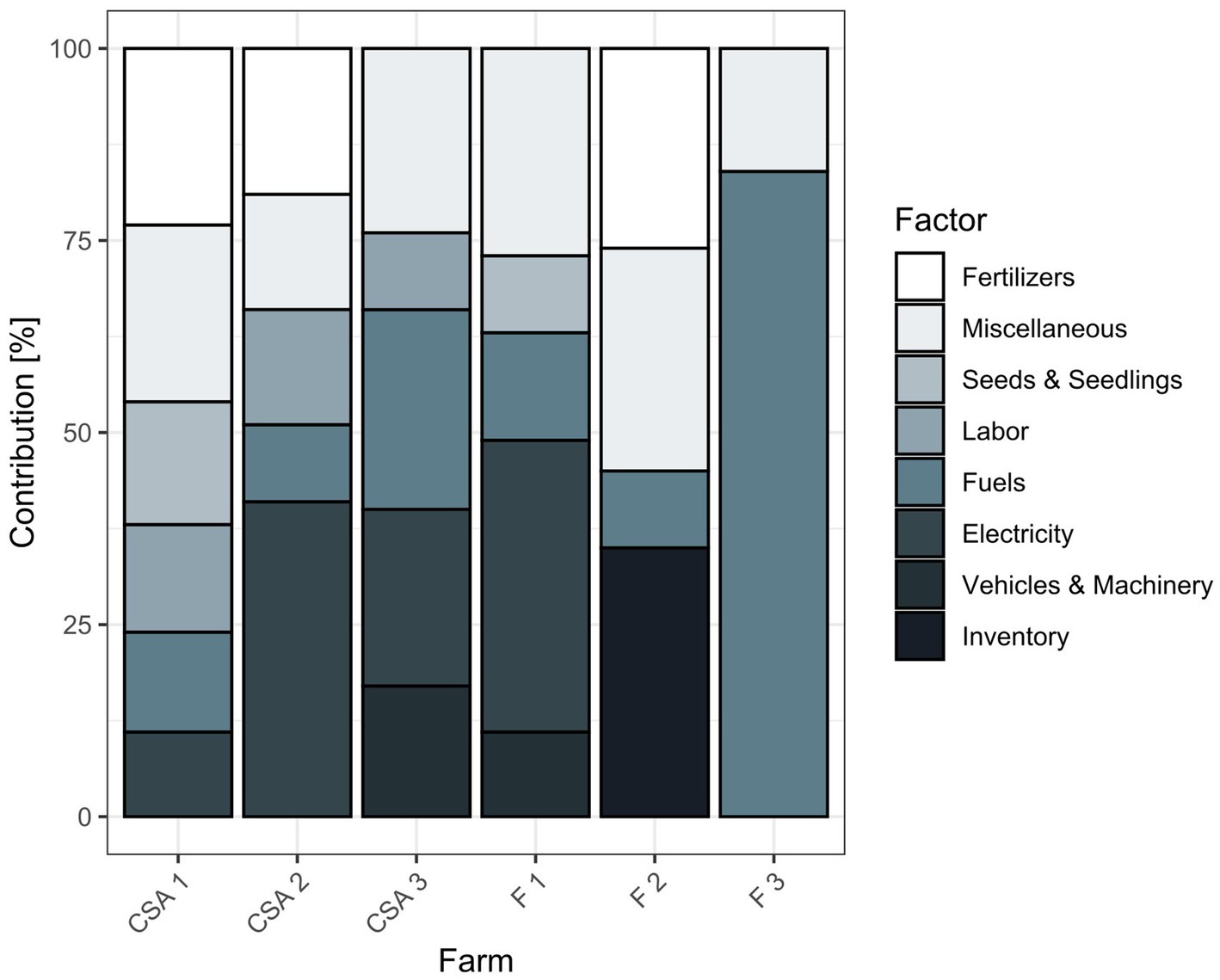
Figure 4. Share of input factors (%), per farm, with miscellaneous describing all input categories of a farm with a share of less than 10 % as listed in Table 5.
3.3 Indicators of energy balance
The most energy efficient farm was F2, with an EROI clearly ahead of any other farm (Table 5). F1, CSA3, and CSA1 followed, while CSA2 and F3 showed relatively low values. CSA3 got the highest EROI of all CSAs. F2 and CSA1 obviously benefited from low CED per ha, while very high CEDs strongly affected the EROI of CSA2 and F3. EROI without PV indicated the importance of electricity production to achieve a low CED for F2 and CSA1. In contrast, high energy outputs compensated moderate CED for F1 and CSA3. F2 instead showed the lowest of all energy outputs per ha, which nevertheless did not compromise the high EROI. F1 and F2 produced the crops with the highest energy contents (Supplementary Appendix 1).
Regarding NRE, F2 and CSA1 again showed the lowest CEDs and also the largest net energy ratios, even though F2 proved to be the farm with the highest share of fossil energies. This is due to F2’s high inputs from storage and transport boxes as well as from synthetic fertilizers. Consequently, CSA1 almost leveled with F2 regarding NRE EROI. In contrast, the high share in NRE and the relatively low results in NRE EROI of CSA2 and CSA3 illustrates the elevated amount of fossil energies they invested into vegetable production. This is mainly related to an extended usage of vehicles and machinery and the corresponding diesel. The differences between NRE EROI and NRE EROI without PV again underlines the importance of PV production for the energy efficiency.
3.4 Comparison of CSA and conventional farms
Focusing on NRE, CSA farms are capable of achieving a similar energy efficiency as conventional enterprises. This is mainly due to high energy outputs and the implementation of PV energy production. Two of three CSA farms showed high inputs in the fields of fertilizers, fuels and labor. On the contrary, the larger and more specialized F2 achieved clearly lower energy inputs per ha of farmland in those categories.
Looking at the three CSA farms in greater detail, their large crop diversity becomes apparent. About half of the respective produces showed an elevated energy content, with potatoes as the vegetable produced in the greatest amount. Yields of single crops did fluctuate between the CSA-farms, with CSA1 and CSA3 showing higher values than CSA2 in most cases (Table 4). On the input side, CSA3 had an increased consumption of fuels. Electricity appeared to be the most important impact category for CSA2 and the second most important for CSA3. Conversely, electricity production at CSA1 exceeded its consumption and therefore remarkably diminished the acquired input energy. Organic fertilizers generally appeared to have a high impact. All CSA farms showed higher values than F1 and F2. Similar results were observed in the category of labor.
Production of F1 was characterized by a less complex and more energy-rich mix of crops. About 80% of their produce showed an elevated energy content. Therefore, it is not surprising that F1 showed the highest output by ha and by kg. Electricity had the main impact on their CED. The effect of fertilizers was instead very low, as this farm showed the lowest of all demands for fertilizers per ha. At the same time their results were above average toward crop protection and quite low toward labor.
F2’s vegetable production encompassed only three different types of vegetables, two of which showed high energy contents, while the third was a perennial. Even though F2 got comparatively low yields and therefore low output per ha, they showed the second highest value at energy output per kg. On the other side, their energy inputs per ha were always among the lowest values of all farms. The most relevant input factor for F2 was its inventory of storage and transport boxes. Input by electricity was also notably low. A reduction of about 50% was achieved through PV electricity production. Synthetic fertilizers were also a relevant impact factor for F2, even though the respective energy input per ha was relatively low. Furthermore, F2 also showed the lowest of all results in the input categories of fuels, vehicles and machinery, labor and lubricants.
Compared to the other farms, F3 showed a high share of fine vegetables, such as tomato, cucumber and paprika. Indeed, less than 10% of their produce showed an elevated energy content. Despite the farm’s high average yield per ha, its output per kg was remarkably low. In combination with an extraordinarily high energy input caused by greenhouse heating, the energy efficiency of F3 turned out to be close to zero. But even without greenhouse heating the results would not improve significantly, as their energy inputs are among the highest of any category (Table 6).
3.5 Sensitivity analysis
The sensitivity analysis was used to identify the most important influencing factors per farm. On the output side, potatoes, peas and carrots were the crops with the greatest overall impact (Figure 3a). These crops were cultivated in large quantities and hold an elevated EEQ of 3,170, 3,820, and 1,630 MJ t−1, respectively.
On the input side, manipulating the EEQs of fertilizers would mainly affect F2 and CSA1 (Figure 3b). Regarding electricity, F1 showed volatility when changes were made in the respective EEQ (Figure 3c). Farms producing electricity by PV generally appeared less susceptible to changes in EEQs. For F2, the EROI would increase significantly more by reducing the EEQ than it would decrease by elevating it. The categories fuels and vehicles and machinery were grouped together (Figure 3d). Changes in EEQs would affect CSA3 and F3 the most, as a 25% reduction would already increase the EROI of CSA3 to 0.50. The exclusion of greenhouse heating would have a notable influence on the EROIs of F1 and F3 (Figure 3e). Finally, only CSA farms were sensitive to changes in the category of labor (Figure 3f). Increasing the EEQ of labor would thereby have slightly less influence than decreasing it.
4 Discussion
In our case study approach, we found that the CSA farms considered tendentially showed a lower performance than the conventional farms, if mass was the functional unit (Table 5). While this has also been observed in studies comparing organic and conventional production of single vegetable crops (Hashemi et al., 2024; Ronga et al., 2019; He et al., 2016; Bos et al., 2007; Williams et al., 2006; Röver et al., 2000), it is in contrast with the study of Pérez-Neira and Grollmus-Venegas (2018), who found CSA farming more energy efficient regarding both area and mass. Also, Zhen et al. (2020) measured the lowest energy depletion for CSA farming compared with organic and conventional cropping systems. However, in our study as well as in Pérez-Neira and Grollmus-Venegas (2018) CSA farming seems to more competitive when NRE is considered.
To sufficiently explore the specific and detailed setup of this research, farm characteristics and CSA performance will be discussed here in depth. To unravel the ecological sustainability potentials and the performance of different agricultural production systems, key factors influencing the balance between inputs and outputs will be highlighted.
4.1 Farm characteristics
Energy input had the greatest impact on the energy efficiency of farms. F2, for example, showed rather low output per ha of farmland, but achieved the highest EROI because of its low energy input. On the contrary, F3 showed a decent output per ha of farmland, which was compromised by a very high energy input. Thereby, fuels, electricity and fertilizers were the most influential impact categories (Figure 3). While fuels for running vehicles and machinery affected especially CSA farms, materials for greenhouse heating had a serious impact on two of the conventional farms. Heating strategies including regional accessible forest waste as burning material and up to date heating infrastructure were thereby energetically most efficient. Electricity proved to be a major input to all vegetable production systems. This is mainly related to irrigation (Temizyurek-Arslan and Karacetin, 2022). According to own calculations, electric power supply refers to 82.1–97.7% of the energy that farms spend on irrigation. With minor effects on efficiency, electric power was also used for cooling and young plant nurseries. More importantly, the on-farm production of electricity from solar radiation proved to be a crucial element for achieving higher energy efficiency. Since PV electricity production notably reduces the demand for fossil energies, the integration of solar power as an agricultural standard will help to reduce its global warming potential and therefore to respect the planetary boundaries (Stranddorf et al., 2023).
The farms also showed considerable differences among their cropping systems. This had notable influences on their outputs. First, the selection of crops had a relevant impact on energy efficiency, as farms largely producing fine vegetables like tomatoes and cucumbers were less energy-efficient compared with those producing legumes or potatoes. This indicates that energy efficiency tends to increase with the selection of vegetables that are rich in calories. Secondly, the farms differed remarkably in the range of cultivated vegetables. The CSA farms engaged in “multi-cropping,” with a total of between 46 and 63 different production goods, while F1 cultivated only 14, F2 three and F3 at least 10 different kinds of vegetables (Petrie and Bates, 2017). Interestingly, extreme multi-cropping as practiced by the CSA farms did not negatively affect yields (Table 4). On the contrary, several positive effects have been reported elsewhere. For example, Li et al. (2018) reported lower global warming, eutrophication, and acidification potentials of such multi-cropping systems, while Trinchera et al. (2022) found various positive effects on soil parameters. Furthermore, a wide variety of crops is likely to increase the resilience of the respective farms against single crop failures (Egli et al., 2021; Renard and Tilman, 2019).
On the contrary, a partially strong do it yourself ethos in CSA proved to be rather energetically inefficient. The approach of being self-sufficient by nursing young plants or producing their own pesticides and fertilizers tie up precious capacities, while the energetical impact of buying seedlings or pesticides from the market is relatively low (Table 6).
Furthermore, scaling effects were observable for the input categories fuels, vehicles and machinery, labor, and lubricants. Thereby, the biggest farm (F2) exhibited low input values, while the smallest farm overall (F3) and the farm with the second smallest area under cultivation (CSA2) showed predominantly high numbers. Larger farms are likely to have specialized machinery that will also gain the efficiency of planting and applying fertilizers and pesticides at scale. In this study, the order of farm sizes was identical with the order of their respective EROI. This finding affirms a correlation between scale and the energy efficiency of farms, as already pointed out by Schlich and Fleissner (2005). Hence, upscaling must be considered as a means to elevate the energy efficiency of farms. In this context, investigating the energy efficiency of larger CSA farms focusing on vegetables (>30 ha) would provide additional insights. Scaling effects could also be achieved by collaboration of several farms that are all specialized on the production of certain groups of vegetables.
Soil and climate conditions also play an important role for farms’ energy efficiency. In this study, farms on loess soils showed the best results, while those on less favorable sandy soils were less energy efficient. Productivity is also influenced by the local weather. As Rempelos et al. (2023) showed radiation and temperature to be the most important impact factors for the yield of cabbage crops, output variations between CSA2 and CSA3 might also be related to differing climate conditions (Tables 2, 4). As energy efficiency also relates to resource efficiency, the placement of agricultural ventures must always recognize the impending threats of water scarcity and soil degradation. To respect the planetary boundaries of freshwater use and land-system change, competing forms of land use need to be mitigated, e.g., by policies (Campbell et al., 2017; Pfister et al., 2011).
4.2 Energetic performance of CSA farms
By comparing the energy balances of the six farms, the CSA farms showed the tendency to be slightly less energy efficient compared to the conventional farms considering a cradle to farm gate approach (see EROI’s at Table 5). The categories of fuels, electricity, fertilizers, and labor had the most impact on these results. This precisely confirms the results of several reference studies (Cristiano, 2021; Pérez-Neira and Grollmus-Venegas, 2018; Christensen et al., 2017). To meet the characteristics of the CSA farms within the scope of our case study approach and to learn about potential trade-off effects, these four major impact categories need to be unraveled in more detail. Energy efficiency of CSA farms increased when focusing on non-renewable energy. CSA1 was even able to achieve almost the same NRE EROI as F2, although CSA1 is 40 times smaller in terms of farm size. This is certainly related to the high amount of electricity produced by PV, as CSA1 got only the fourth highest NRE EROI without PV considered. Moreover, CSA farms showed a tendency to consume fewer fossil fuels (Table 5).
The extended machinery use of CSA farms is related to the replacement of chemical weed control by mechanical weed control in OA. Nevertheless, all three CSAs showed notable differences in their consumption of fuels to run vehicles and machinery (Table 6). However, the farm with the highest input related to machinery usage (CSA3) also achieved higher outputs than the others, indicating trade-off effects.
In the case of electricity, CSA farms producing power from solar radiation performed better. Moreover, all CSAs saved electric energy by using simple underground storage pits instead of modern cooling rooms. CSA farms dropped their electricity consumption, but simultaneously compromised the quality and durability of their produce. However, this trade-off is reduced by a short and immediate supply chain that reduces the need for cooling and the reduction of food losses due to no standards regarding size and shape of the produce (Voge et al., 2023).
The relatively high impact through usage of organic fertilizers on CSA farms was surprising, as mineral fertilizers are usually reported to be very energy-intensive (Aguilera et al., 2019). The share of fertilizers from animal origin was 36% for CSA1, 82% for CSA2 and 98% for CSA3, and the main source of the respective amounts was horse manure from neighboring farms. Eventually, CSA3 was the farm with the highest total demand of external fertilizers from animal origin and with the highest EROI. However, these outcomes might be compromised by the approach of applying mineral fertilizer equivalents to calculate the impact of organic compounds. With no primary data at hand the precise impact of those fertilizers remains uncertain (Montemayor et al., 2022). Consequently, the EROIs of the CSA farms are likely to change, if more accurate information on organic fertilizers were provided. In contrast, no tremendous changes should be expected, if green manure were to be considered. As all farms but F3 applied relevant amounts of green manure, the relative deviation is not expected to be extremely high. The same was the case with potting soil, which was predominantly used by CSA farms. Own estimations did not indicate substantial effects on the results of this study, if potting soil would have been included, although its energetic importance should not be underestimated (Dorr et al., 2017; Reyhani et al., 2022). This differs from the self-production of fertilizers by on-farm materials, as done by CSA2. Without this practice, the farm’s EROI would have dropped to 0.10.
In terms of labor, CSA farms showed notably high impacts compared to the conventional farms. Organic agriculture practices clearly increase labor inputs (Zhen et al., 2020; Pimentel et al., 1983). This is mainly due to the abandonment of chemical pesticides and therefore higher demands of labor-intensive mechanical weed control. In addition, managing large varieties of crops is likely to be more time-consuming. Therefore, the high proportion of voluntary work needs to be considered. CSAs ask their prosumers to support the working processes of the farm and furthermore offer internships. This additional workforce is obviously less efficient compared to professional farm workers, but it is still an important feature not only to connect city dwellers with farming practices, but also to reduce labor costs and to increase vegetable output. If the voluntary working hours would have been reduced by half to simulate the replacement of unskilled work, CSAs would still show high labor costs. On the contrary, an increase in working hours would have little effect on the energy efficiency indicators compared to other relevant impact categories. Consequently, if CSAs could find the capacity to employ a well-trained workforce to enhance the quality and quantity of the output, energy efficiency would likely improve. This also corresponds to the notion that the success of organic agriculture depends on farmers’ talents in applying the necessary techniques (Meemken and Qaim, 2018).
To sum up, CSA farms show a number of special features, such as low-tech infrastructure, an unconventional mix of organic fertilizers and a high amount of voluntary workforce. Although low input energy was stated as the determinant factor for high energy efficiency, increasing or reducing specific inputs can cause trade-off effects and are not necessarily advantageous.
4.3 Methodological reflection
Due to the small sample size, this study does not allow any general conclusions about the performance of different production systems and CSA in particular. However, given the limited research regarding the energy efficiency of CSA, this case study offers additional important insights regarding potential benefits (e.g., related to yields per area) and challenges (e.g., related to high labor requirements), as well as leverage points to improve performance (e.g., renewable energy sources). Due to the detailed understanding of the farms considered, the study also allows to derive practical implications (e.g., collaboration or outsourcing of seed and seedling production). Finally, it also allows to refine hypotheses and to derive research priorities.
Furthermore, the significance of the system boundaries needs to be considered. If they would be modified, the performance of CSA farms compared to conventional farms is likely to change, for example by using a cradle to consumer approach (e.g., in Bosona and Gebrensenbet, 2018; Pérez-Neira and Grollmus-Venegas, 2018; Tasca et al., 2017). A fair share of the vegetables produced by the CSAs, F1 and F3 were not stored for long and did not pass-through long distribution channels. In contrast, all vegetables of F2 underwent processing or traveled long distances before reaching consumers. A farmgate to consumer approach was not applied because the distribution channels of the conventional farms could not be adequately depicted. For CSA farms, the energy ratio from farm gate to supply station could be calculated. The EROI was 1.8 for CSA1, 0.6 for CSA3 and 3.1 for CSA3. All these values exceed the reference EROI for an Andalusian CSA of 0.54 to 0.47 calculated by Pérez-Neira and Grollmus-Venegas (2018). Even though the reference study additionally included the last kilometer from distribution point to consumer, values of CSA1 and CSA3 are likely to be comparatively high, as most people pick up their vegetables by bike or foot (Egli, 2024). In contrast, the reference EROI for conventional farms is 0.35–0.23 (Pérez-Neira and Grollmus-Venegas, 2018). This indicates how results would have been affected, if the system boundaries were expanded beyond the farm gates.
While assessing the sustainability of agroecosystems holistically, one also needs to consider ecological and social indicators. This includes soil degradation, water scarcity, biodiversity loss, and health impacts from pesticide application (Naeem et al., 2021; van der Werf et al., 2020). For example, the average biodiversity gap between organic and conventional agriculture is reported to be about 34% (Tuck et al., 2014). Consequently, CSA farms again have great potential to compensate for energy- and yield efficiency gaps with other ecosystem services.
Likewise, the recognition of social and cultural embeddedness of farms would probably favor the CSA methodology. Accordingly, CSA was described as a resilient food system, where new spaces of trust can manifest (Zoll et al., 2022; Gugerell et al., 2021; White, 2021; Menzel, 2017). Furthermore, CSA reportedly strengthens food sovereignty and food security, even though the access for lower classes remains limited (Parot et al., 2023; Verfuerth et al., 2023; Naylor, 2019). Notions of trust and security are hard to quantify, but must be considered to gain a more holistic understanding of farming systems in the future.
4.4 Further outlook
Several aspects of the environmental potential of CSA farming have been explored, but its capacity to make agriculture more sustainable remains uncertain. A widely discussed framework for assessing the resilience of the planet is the concept of planetary boundaries (Rockström et al., 2009). Several impact processes are directly or indirectly affected by agriculture. Many of them have already exceeded the “safe operating space” or are nearing the limit (Caesar et al., 2024; Campbell et al., 2017). The alteration of biogeochemical flows, particularly nitrogen and phosphorus cycles, has significantly impacted freshwater reservoirs. Livestock farming contributes to aerosol emissions and exacerbates climate change. Additionally, the use of pesticides and ongoing soil degradation threaten biosphere integrity. Agriculture also drives land-system changes and is recognized as a primary cause of deforestation.
In general, OA appears to show lower environmental impacts per area unit than conventional farming (Hashemi et al., 2024). Nevertheless, CSA should be compared with regular OA in different environmental impact categories to learn about their differences. This is the case with the eutrophication potential of nitrogen and phosphorus flows. Therefore, better ways must be found, to compare different cropping systems on that level (Meier et al., 2015). The same applies to the assessment of biodiversity, where CSAs could play an important role due to a high crop diversity and the provision of natural habitats (Egli, 2024). In contrast, conventional farming is often reported to consume less land than OA overall, because of generally lower yields (Hashemi et al., 2024). However, reducing the consumption of animal products and waste could help to feed the world organically (Muller et al., 2017). In the context of food production, the agency of the consumers is also relevant. CSAs have the potential to integrate consumers into the farming business and raise awareness for the environmental challenges agriculture faces today. One of today’s main challenges is to balance the social, economic, and ecological needs of our planet and our societies and transmit this knowledge into smart ways of production management and transparency (Latino et al., 2024; Almasi et al., 2021). So far, consumers are only receiving limited information regarding the sustainability of the foods they are buying. The transparency in CSA farming may work as a great example for the food industry. Furthermore, LCA studies on innovative food systems like CSA farming can help to inform customer friendly product declarations that go beyond the simple dichotomy of organic versus conventional (Latino et al., 2022; Schau and Fet, 2008). Comprehensive assessments including a wide range of ecological, social, and economic aspects are needed to better understand the potential of CSA to support a transformation toward sustainable agri-food systems.
5 Conclusion
In this case study approach CSA farms needed more input energy than a range of different sized and specialized conventional farms to produce an equivalent amount of vegetable incorporated energy within the system boundaries from cradle to farm gate. Results deviated, however, if only fossil fuel resources were considered, where one CSA farm even reached the second highest energy efficiency. However, these differences did not systematically differ between CSA and conventional farms. Moreover, CSA reached high energy efficiency when system boundaries were extended from the farmgate to the consumer, which is likely to be higher compared to farms with long supply chains and heavy processing. Cumulative energy demand was shown to be the dominant impact factor, with fuels, electricity and fertilizers being the most crucial inputs across all farms. Farm size was also an important factor, with larger farms performing better by decreasing inputs per area. Electricity production by PV and the optimization of greenhouse heating showed great extra potential for improving energy efficiency and for reducing the consumption of fossil fuels. This partly helped CSA production to achieve remarkable energy efficiency in terms of NRE. Moreover, increasing outputs efficiently, for example by adapted management (e.g., adjustments regarding selection of crops, sowing dates, and the management of soil organic matter), can also be a way to enhance the energetic performance of farms. This study also reveals trade-offs between different input categories. Replacing fertilizers of animal origin with plant-based compounds does not necessarily increase the energy efficiency, if the output is simultaneously compromised. Lower usage of machinery and fuels might cause similar results, and a self-sufficient nursery for young plants can elevate the energy input from electricity. Accordingly, it is worth the effort to consider possible trade-offs and to optimize them.
To improve the quality of output data, more precise and detailed (e.g., variety) EEQs in the context of small-scale and organic agriculture are urgently needed. Also including the energy demand of farm buildings would foster a more comprehensive assessment. To gain a more complete understanding of the potentials of different approaches, sample sizes for both CSA and conventional farms, the evaluation period and the system boundaries should be extended. Further research should also focus on the potential benefits of farm collaboration to achieve scaling effects in smallholder agriculture, as well as aspects that go beyond energy, including global warming potential, water footprint, soil degradation and biodiversity loss. Likewise, the capability of conventional systems to become more sustainable, e.g., through implementation of regenerative agriculture approaches should be further investigated.
Data availability statement
The raw data supporting the conclusions of this article will be made available by the authors, without undue reservation.
Author contributions
AM: Conceptualization, Data curation, Formal analysis, Visualization, Writing – original draft. LE: Data curation, Supervision, Validation, Writing – review & editing. AG: Conceptualization, Supervision, Validation, Writing – review & editing.
Funding
The author(s) declare that financial support was received for the research and/or publication of this article. This research was part of the project InnoLand-Sachsen (Innovative Modelle für eine nachhaltige und regionale Wertschöpfung: Synergien und Potenziale der Solidarischen Landwirtschaft in Sachsen). This measure was co-financed with tax funds on the basis of the budget passed by the Saxon State Parliament (FKZ:100595134).
Acknowledgments
The authors acknowledge the cooperation of farmers collecting and sharing their precious data. Special thanks are due to Jonathan Steffens for his graphical work on Figure 2. The authors acknowledge the financial support of the Open Access Publication Fund of the Martin Luther University Halle-Wittenberg.
Conflict of interest
The authors declare that the research was conducted in the absence of any commercial or financial relationships that could be construed as a potential conflict of interest.
Publisher’s note
All claims expressed in this article are solely those of the authors and do not necessarily represent those of their affiliated organizations, or those of the publisher, the editors and the reviewers. Any product that may be evaluated in this article, or claim that may be made by its manufacturer, is not guaranteed or endorsed by the publisher.
Supplementary material
The Supplementary material for this article can be found online at: https://www.frontiersin.org/articles/10.3389/fsufs.2025.1490652/full#supplementary-material
References
Aguilera, E., Guzmán Casado, G. I., Infante, J., Soto, D., and de Molina, M. G. (2019). The input side: calculating the embodied energy of agricultural inputs. In: G. I. Guzmán Casado and M. G. MolinaDe (Ed.), Energy in agroecosystems: a tool for assessing sustainability (pp. 79–108). Boca Raton, FL: CRC Press.
Almasi, M., Khoshfetrat, S., and Rahiminezhad Galankashi, M. (2021). Sustainable supplier selection and order allocation under risk and inflation condition. IEEE Trans. Eng. Manag. 68, 823–837. doi: 10.1109/TEM.2019.2903176
Audsley, E. (1997). Harmonisation of environmental life cycle assessment for agriculture. Final report, concerted action AIR3-CT94-2028. Brussels: European Commision.
Audsley, E., Stacey, K., Parsons, D. J., and Williams, A. G. (2009). Estimation of the greenhouse gas emissions from agricultural pesticide manufacture and use. Cranfield: Cranfield University.
BGR (2005). Soil regions map of the European Union and adjacent countries 1:5,000,000 (version 2.0). Special publication, Ispra. EU catalogue number S.P.I.05.134. Hanover: Federal Institute for Geosciences and Natural Resources.
BGR (2013a). Bodenübersichtskarte der Bundesrepublik Deutschland 1:1,000,000. Hanover: Federal Institute for Geosciences and Natural Resources.
BGR (2013b). Ackerbauliches Ertragspotential der Böden in Deutschland 1:1,000,000. Hanover: Federal Institute for Geosciences and Natural Resources.
BGR (2023). Karte der Bodenregionen und Bodengroßlandschaften der Bundesrepublik Deutschland 1:5,000,000. Hanover: Federal Institute for Geosciences and Natural Resources.
BKG (2023). Karte der Verwaltungsgebiete der Bundesrepublik Deutschland 1:2,500,000. Leipzig: Federal Agency for Cartography and Geodesy.
Bos, J. F. F. P., de Haan, J. J., Sukkel, W., and Schils, R. L. M. (2007). Comparing energy use and greenhouse gas emissions in organic and conventional farming systems in the Netherlands. Lecture at: 3rd QLIF Congress: Improving Sustainability in Organic and Low Input Food Production Systems, University of Hohenheim, Germany.
Bosona, T., and Gebrensenbet, G. (2018). Life cycle analysis of organic tomato production and supply in Sweden. J. Clean. Prod. 196, 635–643. doi: 10.1016/j.jclepro.2018.06.087
Breuer, J., König, V., Merkel, D., Olfs, H.-W., Steingrobe, B., Stimpfl, E., et al. (2014). Humusbilanzierung: Eine Methode zur Analyse und Bewertung der Humusversorgung von Ackerland. VDLUFA (Verband Deutscher Landwirtschaftlicher Untersuchungs- und Forschungsanstalten), Speyer. Available online at: https://www.vdlufa.de/wp-content/uploads/2021/05/11-Humusbilanzierung.pdf (Accessed April 26, 2025).
Brodt, S., Kramer, K. J., Kendall, A., and Feenstra, G. (2013). Comparing environmental impacts of regional and national-scale food supply chains: a case study of processed tomatoes. Food Policy 42, 106–114. doi: 10.1016/j.foodpol.2013.07.004
Bundesverband der deutschen Bioethanolwirtschaft e.V. (2024). Fakten & Anwendungen. Available online at: https://www.bdbe.de/bioethanol/bioethanol-fakten (Accessed 11, April 2024).
Caesar, L., Sakschewski, B., Andersen, L. S., Beringer, T., Braun, J., Dennis, D., et al. (2024). Planetary health check report 2024. Potsdam, Germany: Potsdam Institute for Climate Impact Research.
Campbell, B. M., Beare, D. J., Bennett, E. M., Hall-Spencer, J. M., Ingram, J. S. I., Jaramillo, F., et al. (2017). Agriculture production as a major driver of the earth system exceeding planetary boundaries. Ecol. Soc. 22, 1–4. doi: 10.5751/ES-09595-220408
Chen, X., Matthews, H. S., and Griffin, W. M. (2021). Uncertainty caused by life cycle impact assessment methods: case studies in process-based LCI databases. Resour. Conserv. Recycl. 172:105678. doi: 10.1016/j.resconrec.2021.105678
Christensen, L. O., Galt, R. E., and Kendall, A. (2017). Life-cycle greenhouse gas assessment of community supported agriculture in California’s Central Valley. Renew. Agric. Food Syst. 33, 393–405. doi: 10.1017/s1742170517000254
Cristiano, S. (2021). Organic vegetables from community-supported agriculture in Italy: energy assessment and potential for sustainable, just, and resilient urban-rural local food production. J. Clean. Prod. 292:126015. doi: 10.1016/j.jclepro.2021.126015
Cucurachi, S., Scherer, L., Guinée, J., and Tukker, A. (2019). Life cycle assessment of food systems. One Earth 1, 292–297. doi: 10.1016/j.oneear.2019.10.014
de Backer, E., Aertsens, J., Vergucht, S., and Steurbaut, W. (2009). Assessing the ecological soundness of organic and conventional agriculture by means of life cycle assessment (LCA): a case study of leek production. Br. Food J. 111, 1028–1061. doi: 10.1108/00070700910992916
De Ponti, T., Rijk, B., and van Ittersum, M. K. (2012). The crop yield gap between organic and conventional agriculture. Agric. Syst. 108, 1–9. doi: 10.1016/j.agsy.2011.12.004
Dorr, E., Sanyé-Mengual, E., Gabrielle, B., Grard, B. J.-P., and Aubry, C. (2017). Proper selection of substrates and crops enhances the sustainability of Paris rooftop garden. Agron. Sustain. Dev. 37. doi: 10.1007/s13593-017-0459-1
Düwel, O., Siebner, C. S., Utermann, J., and Krone, F. (2007). Bodenarten der Böden Deutschlands: Bericht über länderübergreifende Auswertungen von Punktinformationen im FISBo BGR. Federal Institute for Geosciences and Natural Resources, Hanover. Available online at: https://www.bgr.bund.de/DE/Themen/Boden/Produkte/Schriften/Downloads/Bodenarten_Bericht.pdf;jsessionid=630C4A5E6A282022704E1E5CA860C170.internet971?__blob=publicationFile&v=2 (Accessed March 21, 2024).
Egli, L. (2024). Innovative Modelle für eine nachhaltige und regionale Wertschöpfung: Synergien und Potenziale der Solidarischen Landwirtschaft in Sachsen (InnoLand-Sachsen) – Final report. Zenodo. doi: 10.5281/zenodo.10986415
Egli, L., Rüschhoff, J., and Priess, J. (2023). A systematic review of the ecological, social and economic sustainability effects of community-supported agriculture. Front. Sustain. Food Syst. 7:1136866. doi: 10.3389/fsufs.2023.1136866
Egli, L., Schröter, M., Scherber, C., Tscharntke, T., and Seppelt, R. (2021). Crop diversity effects on temporal agricultural production stability across European regions. Reg. Environ. Chang. 21:96 (2021). doi: 10.1007/s10113-021-01832-9
ESBN (2005). Soil atlas of Europe. Luxembourg: European Commission, Office for Official Publications of the European Communities.
ESTAT. (2024a). Crop production in EU standard humidity by NUTS 2 regions. European Commission, Brussels. Available online at: https://ec.europa.eu/eurostat/databrowser/view/APRO_CPSHR__custom_192348/default/table?lang=en (Accessed March 21, 2024).
ESTAT. (2024b). Digital elevation model over Europe (hydrography, ETRS1989). European Commission, Brussels. Available online at: https://ec.europa.eu/eurostat/web/gisco/geodata/reference-data/elevation/eu-dem/hydrography-etrs1989 (Accessed March 21, 2024).
European Commission (2023). Organic farming in the EU - a decade of organic growth. Brussels: European Commission, DG Agriculture and Rural Development.
FAO. (2024). Daily supply of calories per person, 1800 to 2018. Food and agriculture Organization of the United Nation & historical sources, processed by our world in data. Available online at: https://ourworldindata.org/grapher/daily-per-capita-caloric-supply?tab=chart&country=~DEU (Accessed April 10, 2024).
Flieger, B. (2016). Prosumentenkooperation: Geschichte, Struktur und Entwicklungschancen gemeinschaftsorientierten Wirtschaftens in der Ernährungswirtschaft am Beispiel der Erzeuger-Verbraucher-Genossenschaften. Marburg: Metropolis-Verlag.
Foppa Pedretti, E., Boakye-Yiadom, K. A., Valentini, E., Ilari, A., and Duca, D. (2021). Life cycle assessment of spinach produced in central and southern Italy. Sustain. For. 13:10001. doi: 10.3390/su131810001
Frischknecht, R., Wyss, F., Büsser Knöpfel, S., Lützkendorf, T., and Balouktsi, M. (2015). Cumulative energy demand in LCA: the energy harvested approach. Int. J. Life Cycle Assess. 20, 957–969. doi: 10.1007/s11367-015-0897-4
Fritsche, U. R. (2000). Xtra-Abbau\Kali-DE-2000. UBA (German Environment Agency), Dessau. Available online at: https://www.probas.umweltbundesamt.de/datenbank/#/ (Accessed April 10, 2024).
Green, M. (1987). “Energy in pesticide manufacture, distribution, and use” in Energy in plant nutrition and pest control. ed. Z. R. Helsel (Amsterdam: Elsevier), 165–178.
Gugerell, C., Sato, T., Hvitsand, C., Toriyama, D., Suzuki, N., and Penker, M. (2021). Know the farmer that feeds you: a cross-country analysis of spatial-relational proximities and the attractiveness of community supported agriculture. Agriculture 11:1006. doi: 10.3390/agriculture11101006
Guinée, J. B. (2006). Handbook on life cycle assessment operational guide to the ISO standards: operational guide to the ISO standards. London: Springer Limited.
Habibi, E., Niknejad, Y., Fallah, H., Dastan, S., and Tari, D. B. (2019). Life cycle assessment of rice production systems in different paddy field size levels in north of Iran. Environ. Monit. Assess. 191:202. doi: 10.1007/s10661-019-7344-0
Hartmann, B. M., Schmidt, C., and Sandfuchs, K. (2014). Bundeslebensmittelschlüssel (BLS). Version 3.02. Max Rubner-Institut, Karlsruhe.
Hashemi, F., Mogensen, L., van der Werf, H. M. G., Cederberg, C., and Knudsen, M. T. (2024). Organic food has lower environmental impacts per area unit and similar climate impacts per mass unit compared to conventional. Commun. Earth Environ. 5:250. doi: 10.1038/s43247-024-01415-6
He, X., Qiao, Y., Liu, Y., Dendler, L., Yin, C., and Martin, F. (2016). Environmental impact assessment of organic and conventional tomato production in urban greenhouses of Beijing city, China. J. Clean. Prod. 134, 251–258. doi: 10.1016/j.jclepro.2015.12.004
Hülsbergen, K.-J., Feil, B., Biermann, S., Rathke, G.-W., Kalk, W.-D., and Diepenbrock, W. (2001). A method of energy balancing in crop production andits application in a long-term fertilizer trial. Agric. Ecosyst. Environ. 86, 303–321. doi: 10.1016/S0167-8809(00)00286-3
ISO (2006a). ISO 14040. Environmental management – life cycle assessment – requirements and guidelines. Geneva: ISO.
ISO (2006b). ISO 14044. Environmental management – life cycle assessment – principles and framework. Geneva: ISO.
Jianyi, L., Yuanchao, H., Shenghui, C., Jiefeng, K., and Lilai, X. (2015). Carbon footprints of food production in China (1979–2009). J. Clean. Prod. 90, 97–103. doi: 10.1016/j.jclepro.2014.11.072
Kashyap, D., de Vries, M., Pronk, A., and Adiyoga, W. (2023). Environmental impact assessment of vegetable production in West Java, Indonesia. Sci. Total Environ. 864:160999. doi: 10.1016/j.scitotenv.2022.160999
Kowalczyk, Z., and Cupiał, M. (2020). Environmental analysis of the conventional and organic production of carrot in Poland. J. Clean. Prod. 269:122169. doi: 10.1016/j.jclepro.2020.122169
Kumar, R., Bhardwaj, A., Singh, L. P., and Singh, G. (2023). Quantifying ecological impacts: a comparative life cycle assessment of conventional and organic potato cultivation. Ecol. Model. 486:110510. doi: 10.1016/j.ecolmodel.2023.110510
Latino, M. E., Corallo, A., Menegoli, M., and Nuzzo, B. (2022). An integrative conceptual framework of food certifications: systematic review, research agenda, and macromarketing implications. J. Macromark. 42, 71–99. doi: 10.1177/02761467211049071
Latino, M. E., Menegoli, M., and De Lorenzi, M. C. (2024). A pattern-based tool to support companies in sustainability-oriented business strategies: grounded theory research in agribusiness. Corp. Soc. Responsib. Environ. Manag. 31, 3348–3367. doi: 10.1002/csr.2730
Li, L., Wu, W., Giller, P., O’Halloran, J., Liang, L., Peng, P., et al. (2018). Life cycle assessment of a highly diverse vegetable multi-cropping system in Fengqiu County, China. Sustainability 10:983. doi: 10.3390/su10040983
Loconto, A., Desquilbet, M., Moreau, T., Couvet, D., and Dorin, B. (2020). The land sparing – land sharing controversy: tracing the politics of knowledge. Land Use Policy 96:103610. doi: 10.1016/j.landusepol.2018.09.014
Markussen, M., Kulak, M., Smith, L., Nemecek, T., and Østergård, H. (2014). Evaluating the sustainability of a small-scale low-input organic vegetable supply system in the United Kingdom. Sustain. For. 6, 1913–1945. doi: 10.3390/su6041913
Markwardt, S., and Wellenreuther, F. (2016). Sensitivity analysis as a tool to extend the applicability of LCA findings. Int. J. Life Cycle Assess. 21, 1148–1158. doi: 10.1007/s11367-016-1094-9
Meemken, E.-M., and Qaim, M. (2018). Organic agriculture, food security, and the environment. Ann. Rev. Resour. Econ. 10, 39–63. doi: 10.1146/annurev-resource-100517-023252
Meier, M. S., Stoessel, F., Jungbluth, N., Juraske, R., Schader, C., and Stolze, M. (2015). Environmental impacts of organic and conventional agricultural products: are the differences captured by life cycle assessment? J. Environ. Manag. 149, 193–208. doi: 10.1016/j.jenvman.2014.10.006
Menzel, A. (2017). Vertrauen als Systemkomponente der Solidarischen Landwirtschaft: Befunde einer Einzelfallstudie aus Ostdeutschland. (Unpublished bachelor thesis). Halle (Saale): Martin-Luther-Universität Halle-Wittenberg.
Montemayor, E., Andrade, E. P., Bonmatí, A., and Antón, A. (2022). Critical analysis of life cycle inventory datasets for organic crop production systems. Int. J. Life Cycle Assess. 27, 543–563. doi: 10.1007/s11367-022-02044-x
Mueller, L., Schindler, U., Behrendt, A., Eulenstein, F., and Dannowski, R. (2007). The Muencheberg soil quality rating (SQR): Field manual for detecting and assessing properties and limitations of soils for cropping and grazing. Draft. ZALF (Leibniz Centre for Agricultural Landscape Research), Muencheberg. Available online at: https://www.zalf.de/de/forschung_lehre/publikationen/Documents/Publikation_Mueller_L/field_mueller.pdf (Accessed February 29, 2024).
Muller, A., Schader, C., El-Hage Scialabba, N., Brüggemann, J., Isensee, A., Erb, K.-H., et al. (2017). Strategies for feeding the world more sustainably with organic agriculture. Nat. Commun. 8:1290. doi: 10.1038/s41467-017-01410-w
Naeem, S., Lipton, S., and van Huysen, T. (2021). Sustainable food production: An earth institute sustainability primer. New York, NY: Columbia University Press.
Naylor, L. (2019). Food sovereignty in place: Cuba and Spain. Agric. Hum. Values 36, 705–717. doi: 10.1007/s10460-019-09938-x
Parot, J., Wahlen, S., Schryro, J., and Weckenbrock, P. (2023). Food justice in community supported agriculture – differentiating charitable and emancipatory social support actions. Agric. Hum. Values 41, 685–699. doi: 10.1007/s10460-023-10511-w
Peano, L., Loerincik, Y., Margni, M., and Rossi, V. (2012). Comparative life cycle assessment of horticultural growing media based on peat and other growing media constituents. Version 5. Quantis SA, Lausanne. Available online at: https://erden-substrate.info/wp-content/uploads/2023/02/comparative-life-cycle-assessment-of-horticultural-growing-media-based-on-peat-and-other-growing-media-constituents.pdf
Pérez-Neira, D., and Grollmus-Venegas, A. (2018). Life-cycle energy assessment and carbon footprint of peri-urban horticulture. A comparative case study of local food systems in Spain. Landsc. Urban Plan. 172, 60–68. doi: 10.1016/j.landurbplan.2018.01.001
Petrie, C. A., and Bates, J. (2017). ‘Multi-cropping’, intercropping and adaptation to variable environments in Indus South Asia. J. World Prehist. 30, 81–130. doi: 10.1007/s10963-017-9101-z
Pfister, S., Bayer, P., Koehler, A., and Hellweg, S. (2011). Environmental impacts of water use in global crop production: hotspots and trade-offs with land use. Environ. Sci. Technol. 45, 5761–5768. doi: 10.1021/es1041755
Pimentel, D., Berardi, G., and Fast, S. (1983). Energy efficiency of farming systems: organic and conventional agriculture. Agric. Ecosyst. Environ. 9, 359–372. doi: 10.1016/0167-8809(83)90021-X
Pimentel, D., Hurd, L. E., Bellotti, A. C., Forster, M. J., Oka, I. N., Sholes, O. D., et al. (1973). Food production and the energy crisis. Science 182, 443–449. doi: 10.1126/science.182.4111.443
Ponisio, L. C., M’Gonigle, L. K., Mace, K. C., Palomino, J., de Valpine, P., and Kremen, C. (2015). Diversification practices reduce organic to conventional yield gap. Proc. R. Soc. B 282:20141396. doi: 10.1098/rspb.2014.1396
Raugei, M., Fullana-i-Palmer, P., and Fthenakis, V. (2012). The energy return on energy investment (EROI) of photovoltaics: methodology and comparisons with fossil fuel life cycles. Energy Policy 45, 576–582. doi: 10.1016/j.enpol.2012.03.008
Rempelos, L., Barański, M., Sufar, E. K., Gilroy, J., Shotton, P., Leifert, H., et al. (2023). Effect of climatic conditions, and agronomic practices used in organic and conventional crop production on yield and nutritional composition parameters in potato, cabbage, lettuce and onion: results from the long-term NFSC-trials. Agronomy 13:1225. doi: 10.3390/agronomy13051225
Renard, D., and Tilman, D. (2019). National food production stabilized by crop diversity. Nature 571, 257–260. doi: 10.1038/s41586-019-1316-y
Reyhani, M., Santolini, E., Torreggiani, D., and Tassinari, P. (2022). Assessing the environmental performance of plastic-based and felt-based green wall systems in a life-cycle perspective. Sci. Total Environ. 822:153648. doi: 10.1016/j.scitotenv.2022.153648
Rockström, J., Steffen, W., Noone, K., Persson, Å., Chapin, F. S. III, Lambin, E. F., et al. (2009). A safe operating space for humanity. Nature 461, 472–475. doi: 10.1038/461472a
Ronga, D., Gallingani, T., Zaccardelli, M., Perrone, D., Francia, E., Milc, J., et al. (2019). Carbon footprint and energetic analysis of tomato production in the organic vs the conventional cropping systems in southern Italy. J. Clean. Prod. 220, 836–845. doi: 10.1016/j.jclepro.2019.02.111
Röver, M., Murphy, D. P. L., and Heinemeyer, O. (2000). “Evaluation of conventional and organic agricultural production in relation to primary energy inputs and certain pollution gas emissions” in Landbauforschung Voelkenrode, Sonderheft 211 (Braunschweig: FAL).
Saltelli, A., Tarantola, S., Campolongo, F., and Ratto, M. (2004). Sensitivity analysis in practice: a guide to assessing scientific models, (1). Chichester: Wiley Online Library.
Saltmarsh, N., Meldrum, J., and Longhurst, N. (2011). The impact of community supported agriculture. Soil Association, Bristol. Available online at: https://communitysupportedagriculture.org.uk/wp-content/uploads/2015/03/The-impact-of-community-supported-agriculture.pdf (Accessed April 23, 2025).
Schau, E. M., and Fet, A. M. (2008). LCA studies of food products as background for environmental product declarations. Int. J. Life Cycle Assess. 13, 255–264. doi: 10.1065/lca2007.12.372
Schlich, E., and Fleissner, U. (2005). The ecology of scale: assessment of regional energy turnover and comparison with global food. Int. J. Life Cycle Assess. 10, 219–223. doi: 10.1065/lca2004.09.180.9
Schmidt, J., Egli, L., Gaspers, M., Zech, M., Gastinger, M., and Rommel, M. (2025). Conversion to community-supported agriculture—pathways, motives and barriers for German farmers. Reg. Environ. Change 25:2. doi: 10.1007/s10113-024-02332-2
Schreefel, L., Schulte, R. P. O., de Boer, I. J. M., Schrijver, A. P., and van Zanten, H. H. E. (2020). Regenerative agriculture – the soil is the base. Glob. Food Sec. 26:100404. doi: 10.1016/j.gfs.2020.100404
SFV. (2024). Ertragsübersichten. Solarenergie-Förderverein Deutschland e.V: Aachen. Available online at: https://ertragsdatenbank.de/auswertung/index.html (Accessed April 11, 2024).
Steffen, W., Richardson, K., Rockström, J., Cornell, S. E., Fetzer, I., Bennett, E. M., et al. (2015). Planetary boundaries: guiding human development on a changing planet. Science 347:1259855. doi: 10.1126/science.1259855
Stone, T. F., Thompson, J. R., Rosentrater, K. A., and Nair, A. (2021). A life cycle assessment approach for vegetables in large-, mid-, and small-scale food systems in the Midwest US. Sustain. For. 13:11368. doi: 10.3390/su132011368
Stranddorf, L. K., Clavreul, J., Prieur-Vernat, A., and Ryberg, M. W. (2023). Evaluation of life cycle impacts of European electricity generation in relation to the planetary boundaries. Sustain. Prod. Consumpt. 39, 414–424. doi: 10.1016/j.spc.2023.05.026
Tajane, S., Dadhe, P., Chole, K. K., Mandavgane, S., and Mehetre, S. S. (2017). Material and energy balance calculations for commercial production of whole neem fruit powder using particle-size distribution and energy models. Curr. Sci. 113:911. doi: 10.18520/cs/v113/i05/911-918
Tasca, A. L., Nessi, S., and Rigamonti, L. (2017). Environmental sustainability of Agri-food supply chains: an LCA comparison between two alternative forms of production and distribution of endive in northern Italy. J. Clean. Prod. 140, 725–741. doi: 10.1016/j.jclepro.2016.06.170
Temizyurek-Arslan, M., and Karacetin, E. (2022). Assessing the environmental impacts of organic and conventional mixed vegetable production based on the life cycle assessment approach. Integr. Environ. Assess. Manag. 18, 1733–1746. doi: 10.1002/ieam.4609
Trinchera, A., Migliore, M., Warren Raffa, D., Ommeslag, S., Debode, J., Shanmugam, S., et al. (2022). Can multi-cropping affect soil microbial stoichiometry and functional diversity, decreasing potential soil-borne pathogens? A study on European organic vegetable cropping systems. Front. Plant Sci. 13:952910. doi: 10.3389/fpls.2022.952910
Tubiello, F. N., Karl, K., Flammini, A., Gütschow, J., Obli-Laryea, G., Conchedda, G., et al. (2022). Pre- and post-production processes increasingly dominate greenhouse gas emissions from Agri-food systems. Earth Syst. Sci. Data 14, 1795–1809. doi: 10.5194/essd-14-1795-2022
Tuck, S. L., Winqvist, C., Mota, F., Ahnström, J., Turnbull, L. A., and Bengtsson, J. (2014). Land-use intensity and the effects of organic farming on biodiversity: a hierarchical meta-analysis. J. Appl. Ecol. 51, 746–755. doi: 10.1111/1365-2664.12219
van der Werf, H. M. G., Knudsen, M. T., and Cederberg, C. (2020). Towards better representation of organic agriculture in life cycle assessment. Nat. Sustain. 3, 419–425. doi: 10.1038/s41893-020-0489-6
Verfuerth, C., Bellamy, A. S., Adlerova, B., and Dutton, A. (2023). Building relationships back into the food system: addressing food insecurity and food well-being. Front. Sustain. Food Syst. 7:1218299. doi: 10.3389/fsufs.2023.1218299
Voge, J., Newiger-Dous, T., Ehrlich, E., Ermann, U., Ernst, D., Haase, D., et al. (2023). Food loss and waste in community-supported agriculture in the region of Leipzig, Germany. Int. J. Agric. Sustain. 21, 7–8. doi: 10.1080/14735903.2023.2242181
Wezel, A., Soboksa, G., McClelland, S., Delespesse, F., and Boissau, A. (2015). The blurred boundaries of ecological, sustainable, and agroecological intensification: a review. Agron. Sustain. Dev. 35, 1283–1295. doi: 10.1007/s13593-015-0333-y
White, N. (2021). Farming in the time of pandemic: small farms demonstrate flexibility, innovation, and hope. J. Agric. Food Syst. Commun. Dev. 1–3, 1–3. doi: 10.5304/jafscd.2021.102.008
Williams, A. G., Audsley, E., and Sandars, D. L. (2006). Determining the environmental burdens and resource use in the production of agricultural and horticultural commodities. Main report. Defra research project IS0205. Bedford: Cranfield University and Defra.
Wittkopf, S., Hömer, U., and Feller, S. (2003). Bereitstellungsverfahren für Waldhackschnitzel - Leistungen, Kosten, Rahmenbedingungen. Bayerische Landesanstalt für Wald und Forstwirtschaft (LWF), Freising. Available online at: https://www.lwf.bayern.de/mam/cms04/service/dateien/w38_bereitstellungsverfahren_fuer_waldhackschnitzel_gesamtheft_gesch.pdf (Accessed April 23, 2025).
Zerbe, C. (Ed.) (1952). Mineralöle und verwandte Produkte: Ein Handbuch für das Laboratorium. Berlin: Springer-Verlag.
Zhen, H., Gao, W., Jia, L., Qiao, Y., and Ju, X. (2020). Environmental and economic life cycle assessment of alternative greenhouse vegetable production farms in peri-urban Beijing, China. J. Clean. Prod. 269:122380. doi: 10.1016/j.jclepro.2020.122380
Keywords: agricultural transformation, cumulative energy demand, energy balancing, life cycle assessment, organic agriculture, smallholder agriculture, sustainability, yield gap
Citation: Menzel A, Egli L and Gross A (2025) Energy-efficiency of community supported agriculture farms and conventional vegetable production. Front. Sustain. Food Syst. 9:1490652. doi: 10.3389/fsufs.2025.1490652
Edited by:
Faissal Aziz, Cadi Ayyad University, MoroccoReviewed by:
Maria Elena Latino, University of Salento, ItalyMariany Costa Deprá, Federal University of Santa Maria, Brazil
Copyright © 2025 Menzel, Egli and Gross. This is an open-access article distributed under the terms of the Creative Commons Attribution License (CC BY). The use, distribution or reproduction in other forums is permitted, provided the original author(s) and the copyright owner(s) are credited and that the original publication in this journal is cited, in accordance with accepted academic practice. No use, distribution or reproduction is permitted which does not comply with these terms.
*Correspondence: Arthur Gross, QXJ0aHVyLmdyb3NzQGxhbmR3LnVuaS1oYWxsZS5kZQ==