- 1Chemical, Food and Environmental Engineering Department, Universidad de las Américas Puebla, Puebla, Mexico
- 2Departamento de Bioquímica-Alimentos, Facultad de Ciencias Químicas, Benemérita Universidad Autónoma de Puebla, Puebla, Mexico
- 3Facultad de Ciencias Químicas, Universidad Autónoma de Chihuahua, Chihuahua, Mexico
This study evaluates the antimicrobial potential of thyme essential oil (EO) against fungal growth in high-risk conditions. It uses an integrative modeling approach, including logistic regression, survival analysis, and kinetic modeling. The goal is to understand the conditions that promote fungal growth and the growth rate over time, including pH, water activity (aw), and thyme EO concentration in solid-model systems. The antimicrobial activity was tested against Aspergillus flavus and Penicillium citrinum, varying pH levels (3, 4, and 5), aw (0.90, 0.95, and 0.99), and EO concentrations (0, 25, 50, 100, and 500 ppm) in potato-dextrose agar. The mold growth responses under the different tested conditions were evaluated using complementary modeling techniques, binary logistic regression, regression with life data, and kinetic model using the Gompertz equation. Results showed that reducing aw or pH alone was insufficient to inhibit mold growth without thyme EO. Each tested model offers unique insights into mold growth. The binary logistic model assesses growth versus no-growth conditions and identifies threshold values. Time-to-growth regression analysis with failure data helps understand the delay in mold growth and evaluate the combined preservation factors’ efficacy. When combining stress factors, the kinetic model provides detailed insights into growth rates, maximum growth, and lag phases. Analyzing the data with the three models allows a comprehensive understanding of how the studied factors influence mold growth, which is crucial for food safety and shelf-life evaluation.
1 Introduction
Fungal spoilage of food represents a significant challenge in the food industry despite centuries of preservation methods, as it adversely affects quality and safety. It still causes considerable economic losses today, with the food and beverage industries facing up to 50% annual losses (Houbraken and Samson, 2017; Rico-Munoz et al., 2019). Fungi like Aspergillus and Penicillium are concerning due to their presence in food and ability to produce mycotoxins. Penicillium citrinum is a widespread mold present in almost every kind of food or feed, capable of growing between 5°C and 40°C (30°C as the optimum) and above aw 0.80, it produces citrinin, a mycotoxin of the group polyketide with nephrotoxic activity (Pitt, 2006; Pleadin et al., 2019). P. citrinum typically occurs in small-grained cereals, maize and wheat flour, nuts (peanuts, pecans, pistachios, and hazelnuts), fermented and cured meats (hams), soybeans, cocoa, and coffee beans (Pitt, 2006). On the other hand, Aspergillus flavus is a saprophytic soil fungus naturally occurring as a parasite contaminating food crops before and after harvest (Abd El-Hack et al., 2023). A. flavus is one of the dominant species found on stored products (primary grains) and possesses the capability to thrive in low- aw (0.78–0.84), high temperatures (up to 55°C) and produce aflatoxin B (carcinogenic mycotoxin) under optimal conditions of 16–31°C and 0.95–0.99 aw whereas is almost unaffected by pH (2.1–11.2), its growth is slowed down at pH < 3.5 (Dobson, 2011; Pitt and Hocking, 2022).
Factors such as aw, pH, and the presence of antimicrobial agents like EOs play a crucial role in fungal growth and mycotoxin production (Battilani et al., 2016; Pitt and Hocking, 2022). Thus, understanding and controlling these factors is essential for minimizing fungal growth in foods (Odeyemi et al., 2020; Rico-Munoz et al., 2019). The antimicrobial effect of reduced aw primarily stems from its impact on cellular turgor pressure. Lowering aw induces plasmolysis, reducing cytoplasmic volume and causing cellular stress (Wijnker et al., 2006). For instance, salting effectively slows food deterioration in meat, fish, and seafood (Amit et al., 2017; Wijnker et al., 2006). Similarly, the combination of aw reduction with other factors such as food composition, pH, and the presence of antimicrobials influences fungal survival (Tapia et al., 2020). pH is the main environmental factor that directly influences cell development, morphogenesis, cell membrane and cell wall stabilization, protein stability and function, and secondary metabolism; or indirectly through its effect on nutrient availability (Abubakar et al., 2013; Wang et al., 2013). Fungal growth is quite broad at acid/alkaline pH from 3.0 to >8.0 with an optimum pH of around 5.0 if nutrient requirements are satisfied (Pardo et al., 2006). Penicillium is recognized as more acidic pH tolerant than Aspergillus (Wheeler et al., 1991; Pitt and Hocking, 2022). Antimicrobials, including EOs, are gaining interest as natural preservation agents due to consumer demand for minimally processed foods without chemical additives (Carocho et al., 2014; Leistner and Gould, 2002; Suhr and Nielsen, 2003; Leyva Salas et al., 2017; Reyes-Jurado et al., 2015). EOs derived from plants, such as thyme EO, are particularly promising due to their antifungal properties. Thyme EO efficacy in extending shelf life and enhancing food safety has been well-documented (Aljabeili et al., 2018; Alagawany et al., 2021; Escobar et al., 2020; Reyes-Jurado et al., 2015). Bioactive compounds like thymol and carvacrol in thyme EO disrupt microbial cell membranes, increasing permeability and causing cell death (Mandal and DebMandal, 2016).
On the other hand, predictive microbiology is now a remarkable tool to evaluate fungal responses in food systems, improving the assessment of microbial spoilage risks and food safety (Baert et al., 2007; Tarlak, 2023). Models like the Gompertz equation estimate growth rate and lag time, while logistic regression models predict the likelihood of fungal growth under specific conditions (Zwietering et al., 1990; Kosegarten et al., 2017). Survival analysis provides additional insights by evaluating the time delay until fungal growth occurs, offering strategies to extend shelf life by suppressing fungal development (Tarlak, 2023; Taiwo et al., 2024). Therefore, logistic regression addresses growth probability, survival analysis evaluates the time to growth onset, and kinetic modeling using the Gompertz equation captures the rate and progression of fungal development. Combining these modeling approaches offers a multidimensional understanding of fungal growth. The integrated approach may enhance the understanding of fungal spoilage and support the design of effective control strategies to improve food preservation and safety (Taiwo et al., 2024). This approach has been previously reported for Aspergillus niger growth on yogurt (Gougouli and Koutsoumanis, 2017) and A. flavus growth in model systems formulated with different levels of fat, protein, pH, aw, and cinnamon EO incubated at three temperatures (Kosegarten et al., 2017). Based on previous information, the objective of this study is to comprehensively evaluate the growth dynamics of A. flavus and P. citrinum using an integrative modeling approach that combines logistic regression, survival analysis, and the Gompertz kinetic model in solid model systems. The research aims to describe and predict fungal growth responses to key variables, aw, pH, and thyme EO concentration, in potato-dextrose agar, evaluating the effects on a factorial design response surface framework. This approach enables the assessment of these factors’ individual and interaction effects on growth probability, lag time, and growth rate. Furthermore, the study explores the antifungal efficacy of thyme EO as a natural preservation agent, providing critical insights into its potential to inhibit fungal growth under varying environmental conditions. These findings aim to inform the development of effective food preservation strategies.
2 Materials and methods
2.1 Essential oil extraction
EO was extracted from thyme (Thymus vulgaris L.) leaves obtained from Condimentos Naturales Tres Villas, Puebla, Mexico. Microwave-assisted hydro-distillation was conducted using a NEOS microwave extraction system (800 W, 60 Hz) from Milestone, Sorisole, Italy. For the extraction process, a sample of 200 g of thyme was mixed with distilled water in a beaker, maintaining a solid-to-water ratio of 1:3 (Lainez-Cerón et al., 2022). The beaker was secured to the microwave-assisted extraction system, and the extraction process was carried out for 40 min at a stirring speed of 400 rpm.
EO was analyzed using the methodology described by Paris et al. (2020) with a gas chromatograph (Agilent 6850 N, Agilent Technologies, Santa Clara, CA, USA) coupled with a mass spectrometer (Agilent 5975 C). A 30 m × 0.25 mm column with a 0.25-μm film thickness (Agilent HP-5) was employed, using helium as the carrier gas at a 1.1 mL/min flow rate. The injector temperature was set to 250°C. The oven temperature started at 60°C for 2 min, then increased gradually to 10°C per minute until it reached 250°C. This temperature was maintained for an additional 10 min before rising to 300°C. Thyme EO volatile compounds were identified using the National Institute of Standards and Technology (NIST) library.
2.2 Determination of antifungal activity
Aspergillus flavus and Penicillium citrinum were obtained from the collection of the Food Microbiology laboratory of the University of the Americas Puebla. Mold spores were harvested from 7-day slants using 5 mL of aqueous Tween 80 solution (0.1% v/v). The concentration of spores in the suspension was determined using a Neubauer chamber, and the spore suspension was adjusted to 1 × 106 spores/mL.
2.3 Experimental design
A full-factorial experimental design was utilized to evaluate the effect of selected factors on the lag time, radial growth, and the growth or no-growth response of A. flavus and P. citrinum. Studied factors were aw, thyme EO concentration, and pH. Solid model systems were prepared using potato-dextrose agar (PDA) as a base for mold growth. In each case, the culture medium was adjusted to different aw values (0.99, 0.95, or 0.90) using sodium chloride. The amount of sodium chloride was calculated using the Favetto and Chirife (1985) Equation 1 as follows:
Where: K* = 0.03710, constant for sodium chloride, and m = solute molality.
After aw of PDA was adjusted, it was sterilized at 121°C for 15 min, cooled, and pH adjusted (5.0, 4.0, and 3.0) with filter-sterilized citric acid solution (10%). The acid amounts required to achieve each pH value were determined through titration curves. The PDA prepared for each condition was divided to add the quantities of thyme EO to reach the concentrations to be evaluated in each case (0, 25, 50, and 500 ppm), mixed, and poured into 60 × 15 mm Petri dishes until solidified. 5 μL of spore suspension was inoculated in the center of the agar and allowed to dry for 10 min under aseptic conditions. Inoculated plates and corresponding controls were incubated at 25°C within airtight plastic chambers to prevent anoxic conditions and were regularly inspected for fungal growth for 90 days (2,160 h). Colony diameters were frequently quantified using a digital caliper (Mitutoyo Corp., Kawasaki, Japan), with measurements taken along two orthogonal axes to ensure accuracy. Negative controls without EO were included in the experimental design. All systems were evaluated in triplicate.
In addition, each medium formulation’s pH and aw, plates without fungal inoculation, were measured initially and after 90 days of incubation to verify that the pH and aw conditions were maintained throughout the incubation period, ensuring that the observed delay in growth rate was not attributable to changes in these two factors. pH was measured using a potentiometer (model 50, Beckman, Brea, CA, USA) that had been previously calibrated. Water activity was assessed with a hygrometer (AQUALAB, series 3B, v.3.0, Decagon, Pullman, WA, USA) that had also been calibrated. All measurements were taken in triplicate.
2.4 Modeling growth response
2.4.1 Modified Gompertz equation
The modified Gompertz equation is commonly used to describe mold growth because of its versatility and ability to represent sigmoidal curves (Zwietering et al., 1990; Kosegarten et al., 2017). P. citrinum and A. flavus growth curves were described using Equation 2, fitting the model parameters by non-linear regression.
Where: μ is the maximum growth rate (1/h), A is the maximum diameter observed, λ is the delay phase or lag time (h), and D is the colony diameter (mm) at time t (h).
A response surface polynomial model (Equation 3) was utilized to assess the dependence of Gompertz parameters on aw, pH, and thyme EO concentration. Stepwise backward elimination was applied to identify significant variables and interactions (p < 0.10), and the analysis was conducted in Minitab 21 software (Minitab Inc., State College, PA, USA).
Where f(x) is the parameter (A, μ, or λ), xi are the independent variables (aw, pH, and EO concentration), and βi are the coefficients of the polynomial model. The R2 and residual analysis values were calculated to assess the goodness of fit of the models.
2.4.2 Probabilistic (binary-logistic regression) model
After the 90-day incubation period, mold responses were classified as 1 if growth was observed and 0 if no growth was detected. Then, a probabilistic model using logistic regression (Kosegarten et al., 2017; Hernández-Figueroa et al., 2024), as described in Equations 4–6, was applied to the data utilizing Minitab 21 software for the analysis.
Where p(x) represents the probability of growth, g(x) is the polynomial model, xi are the independent variables (aw, pH, and thyme EO concentration), and βi are the coefficients of the model. Only those variables and interactions that were statistically significant (p < 0.10) after stepwise backward elimination were utilized to construct the model. Hosmer-Lemeshow and Pearson’s tests were conducted to assess the model’s goodness of fit.
2.4.3 Time-to-growth (survival analysis) model
Based on the time at which the growth of P. citrinum and A. flavus became visible, a polynomial model was applied to describe the time-to-growth (TTG) (Equation 7) using survival analysis (Hernández-Figueroa et al., 2024) in Minitab 21 statistical software. Fungal colonies become visible to the naked eye when they reach a diameter of 2 mm or greater (Marin et al., 2021). The mold growth data was classified as F if the failure (growth) was detected or C (censored) if the mold showed no growth after 90 days. The variables for this model were normalized (normalized value = (actual value − average)/standard deviation).
Where TTG(X) is the time when visible mold growth is detected, βi are the polynomial model coefficients, and Xi are the normalized values of the studied factors (pH, aw, and EO concentration). Only those significant (p < 0.10) variables and interactions were utilized to construct the TTG model using Minitab 21 software.
3 Results and discussion
3.1 Thyme essential oil composition
The major compounds in thyme EO were p-cymene, thymol, carvacrol, caryophyllene, and γ-terpinene (Table 1), representing 90.4% of the total. Etri and Pluhár (2024) and Pluhár et al. (2024) noted that thymol in thyme EO could vary considerably, influenced by chemotypes, variety, region, and environmental factors. Najar et al. (2021) analyzed different chemotypes of thyme, the ‘thymol’ chemotype EO showed thymol as the major constituent (51.26–49.87%) followed by γ-terpinene and p-cymene, and in the ‘linalool’ chemotype, the EO contains oxygenated monoterpenes such as linalool (75%), linalyl acetate (8.15%), and b-caryophyllene (3.2%) as main constituents. The studied thyme variety contains trace amounts of copaene and camphene, each around 1.0%.
3.2 Effect of water activity, pH, and thyme EO concentration on fungal growth
The Gompertz model is the sigmoidal representation of microbial growth kinetics, capturing key parameters such as maximum growth (A), specific growth rate (μ), and lag phase duration (λ). The Gompertz model is often used to analyze mold growth kinetics under varying environmental conditions (Figure 1); it has been employed to evaluate how changes in moisture and temperature affect mold growth; also, the model’s parameters offer valuable insights into mold growth rates and inhibition, necessary for assessing spoilage risk (Pitt and Hocking, 2022). Nielsen and Rios (2000) applied this model to study the effects of EOs on mold inhibition in bakery products, demonstrating how different EO concentrations influenced growth rates and lag phase periods. This highlights the model’s effectiveness in evaluating natural antifungal agents.
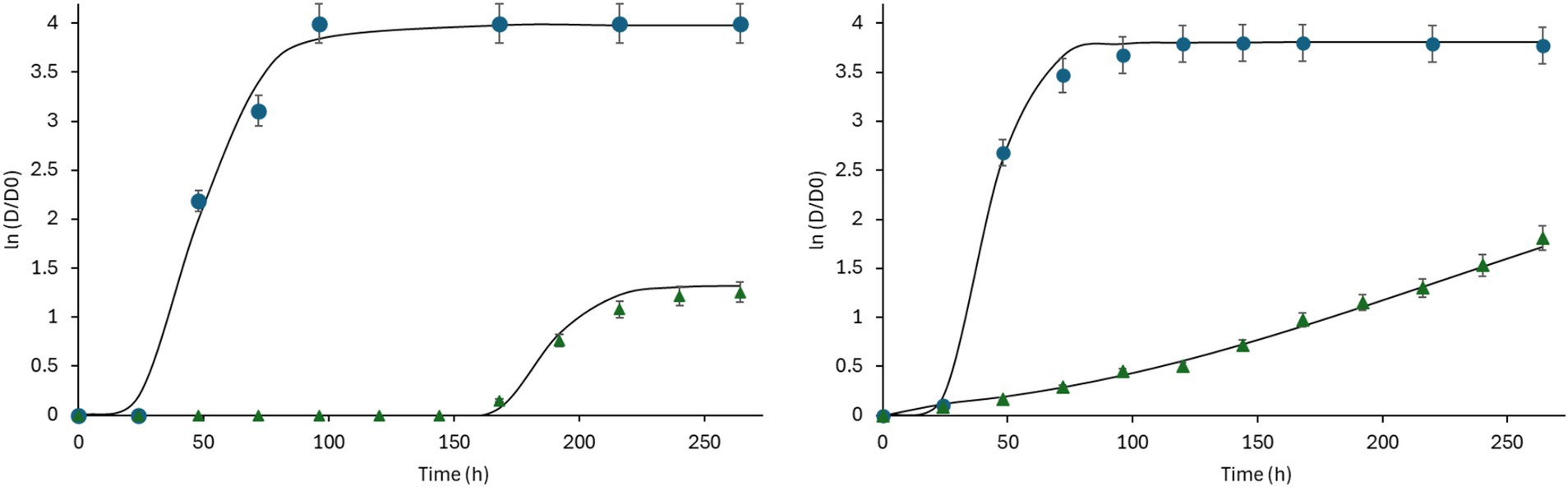
Figure 1. Growth response of Aspergillus flavus (left) in systems formulated with water activity 0.99, pH 3.0, ● control (without EO), or ∆ 25 ppm thyme EO, and Penicillium citrinum (right) in media formulated with water activity 0.99, pH 4.0, ● control (without EO), or ∆ 25 ppm thyme EO. Lines Gompertz model fit.
Table 2 presents the parameters of the Gompertz model, where the maximum growth, the growth rate, and the lag phase are observed. The control systems without EO showed that for aw 0.99 at pH levels of 3, 4, or 5, mold began to grow after 25–27 h. P. citrinum and A. flavus exhibited higher growth rates at the highest aw (0.99). In cultured media formulated with aw 0.99 and pH 5, the growth rate is higher than at pH 3. In contrast, mold growth occurs later at aw 0.95 and pH of 3, starting after 140 h, compared to pH levels of 4 or 5, where growth begins after 26 or 62 h, respectively. Growth decreased significantly as aw was reduced, especially at aw = 0.90, where both molds showed minimal or no growth across pH levels and EO concentrations tested (Table 2). At aw of 0.90, mold growth occurs significantly later at a pH = 3, with a lag phase of more than 1,000 h. Reducing aw to 0.99, 0.95, or 0.90 at pH levels 3, 4, or 5 does not effectively inhibit mold growth without thyme EO. Adding 25 ppm of thyme EO influences the mold response (Figure 1). The lag phase is higher at pH 3 than at pH 5 for concentrations of 0, 25, and 50 ppm of EO. For concentrations of 100 ppm, the growth rate is lower at pH 3 than at pH 5. Figure 1 shows that the Gompertz model fits the experimental data well; the R2 was >0.90 in the analyzed cases.
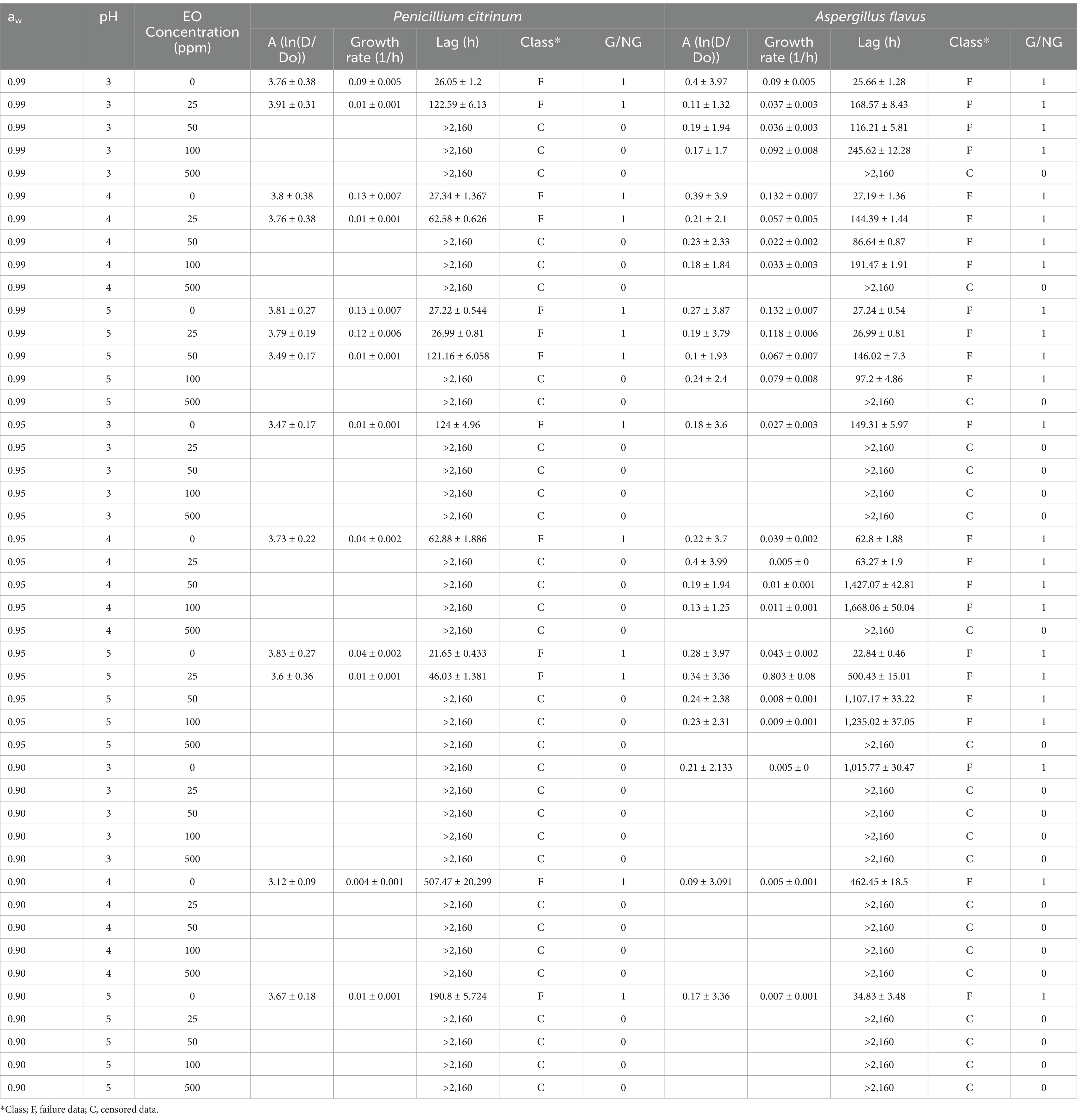
Table 2. Gompertz model parameters, growth (G) or No-Growth (NG) mold response, and class data (F, failure or C, censored) for time-to-growth modeling.
Polynomial models (Table 3) may be used to predict the mold’s behavior under different factor combinations. Figure 2 shows the surface response graphs for the studied molds’ lag time (h) under different combinations of aw and thyme EO in media formulated at pH 4.0. P. citrinum growth remained relatively stable across pH levels when other conditions favored growth, while A. flavus showed variable growth rates and lag phases with changing pH. Acidic pH (around pH 3) tended to reduce growth rates and increase lag phases in both molds, indicating pH as a limiting factor for mold proliferation. Similar findings have been reported previously for Penicillium expansum (Tannous et al., 2016) and A. flavus (Kosegarten et al., 2017). The lag phase typically increases as aw decreases, showing a delay in mold growth (Table 2; Figure 2). Dagnas et al. (2014) also recorded increasing lag times at decreasing aw for A. niger and Penicillium corylophilum in malt extract agar. Growth rates were generally higher at pH 5 for both molds, although pH sensitivity was more pronounced in A. flavus than in P. citrinum as previously mentioned. Both molds demonstrated considerable sensitivity to increasing thyme EO concentrations (Figure 2). At ≥50 ppm, P. citrinum growth was almost completely inhibited (indicating it is more sensitive to this factor), while A. flavus could still grow but with a substantially longer lag phase and reduced growth rate. EO concentrations of 100 or 500 ppm inhibited the growth of both molds entirely (Table 2). This indicates a concentration-dependent inhibition for both molds, but A. flavus seems to tolerate slightly higher concentrations than P. citrinum before complete growth inhibition occurs. A. flavus showed slightly higher resistance to lower EO concentrations than P. citrinum, maintaining growth at 25 and 50 ppm in certain conditions (Figure 2). Under subinhibitory conditions (low pH, high EO concentration), A. flavus generally displayed longer lag phases than P. citrinum, suggesting it may take longer to adapt to challenging environments.
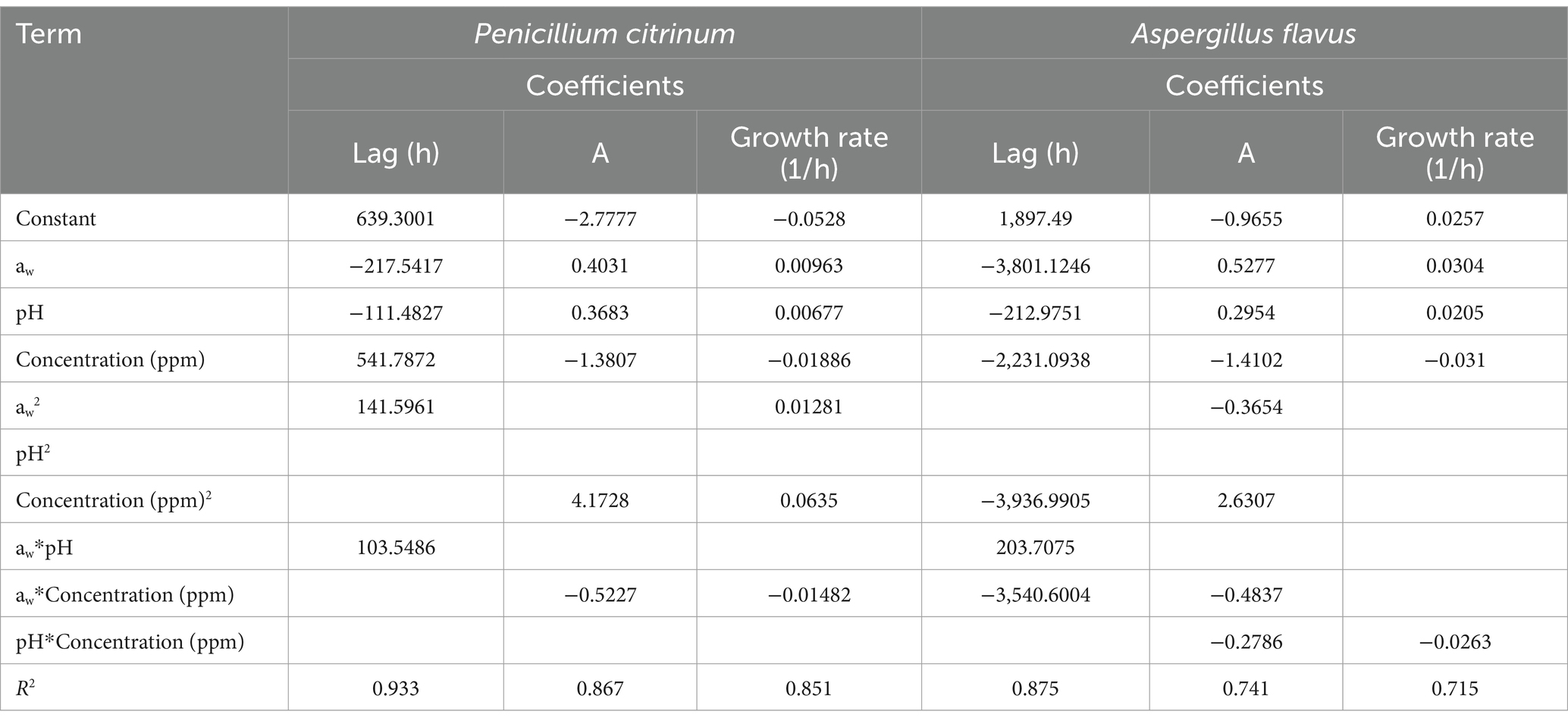
Table 3. Coefficients of the polynomial models based on the parameters of the Gompertz model for each evaluated mold.
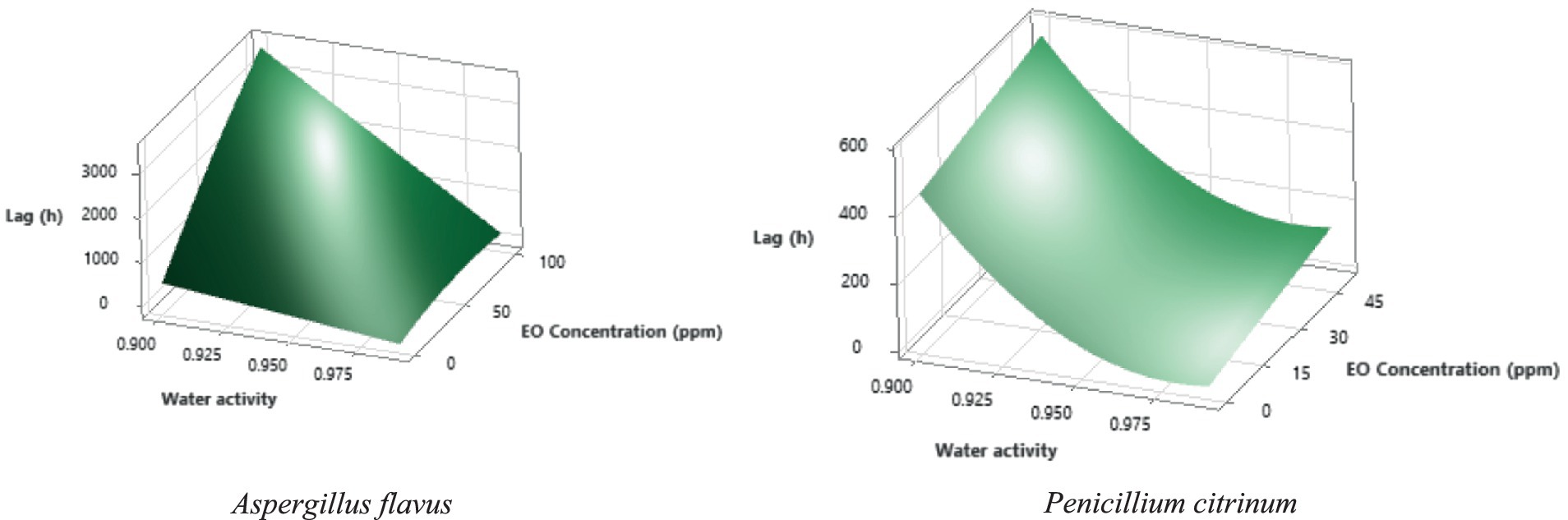
Figure 2. Lag time (h) response surface for the studied molds under different water activity and thyme EO combinations, maintaining pH at 4.0.
The findings reported align with those from authors who evaluated the antifungal effects of thyme EO or its components in various combinations with other factors. Suhr and Nielsen (2003) demonstrated that thymol exhibits greater inhibitory effects at pH 5.5 compared to pH 6.5. At acidic pH, thyme EO or thymol molecules become less dissociated and more hydrophobic, enhancing their ability to bind to hydrophobic regions of proteins and dissolve in the lipid phase (Oliveira et al., 2020; Ranjbar et al., 2022). This possibly explains the disruption of membrane permeability (Oliveira et al., 2020; Ranjbar et al., 2022), confirmed by the efflux of intracellular K+ ions (Hu et al., 2018). Similarly, Thompson (1990) observed that 1.0 mM carvacrol completely inhibited the growth of A. flavus at pH 4 and 8. Mycelial growth was partially inhibited at lower concentrations (0.1 and 0.5 mM), with the most significant reductions at these pH levels. Schlösser and Prange (2019) evaluated the impact of carvacrol on the mycelial growth of Penicillium verrucosum and Aspergillus westerdijkiae. Their findings revealed that supplementation with ½ the minimum inhibitory concentration (MIC) of carvacrol significantly reduced the mycelial dry weight of both molds throughout a 21-day incubation period. Debonne et al. (2019) reported that the growth of Penicillium paneum was significantly reduced at aw 0.88 compared to higher aw levels (0.93–0.97). Additionally, the lowest concentration of thyme EO required to inhibit growth over 144 h was 1 μL/mL. The evaluation of the antifungal activity of thyme EO in vitro against A. niger and P. paneum confirmed that thyme EO at concentrations between 0 and 0.5 μL/mL significantly (p < 0.05) influenced both λ and μmax under various temperature conditions (Debonne et al., 2018).
3.3 Logistic model
Over the last four decades, predictive models originally developed for bacteria have been adapted to describe mold growth, including both primary and secondary models (Dagnas and Membré, 2013). These models allow food manufacturers to design product formulations that achieve a targeted shelf life or, conversely, to adjust shelf life based on the formulation. The binary logistic regression model has proven effective in determining the conditions under which mold growth occurs or is inhibited (Hernández-Figueroa et al., 2024; Kosegarten et al., 2017). The binary logistic regression model is a widely used statistical tool for predicting the probability of a binary outcome, such as the presence or absence of mold growth under specific environmental conditions. This model categorizes conditions as favorable or inhibitory to microbial growth, thereby allowing the assessment of key environmental factors such as pH, aw, and the antimicrobial agents concentration (Henley-Smith et al., 2014; Possas et al., 2021). However, the binary logistic model does not quantify the extent or rate of mold growth; it merely identifies whether conditions favor growth.
This study employed binary logistic regression to predict the probability of A. flavus and P. citrinum growth as a function of aw, pH, and EO concentration. The results indicated that aw and pH were significant predictors, with higher aw levels positively correlated with fungal growth, consistent with the established role of water availability in supporting microbial activity. Similarly, more neutral pH values (closer to pH 5) were associated with increased growth probability. Conversely, higher EO concentrations exhibited a significant inhibitory effect on fungal growth. These findings align with prior research demonstrating that antimicrobial compounds, such as EOs, can effectively suppress fungal activity (Al-Maqtari et al., 2021).
The interaction between factors provided additional insights (Table 4; Figure 3). For instance, aw and thyme EO concentration interaction affects the probability of mold growth (Figure 3), and the inhibitory effect of EO was more pronounced under conditions of lower aw, suggesting a synergistic potential for combining these variables in mold control strategies (Figure 3). Likewise, binary interactions of factors aw-cinnamon EO, aw-pH, and other combinations of fat content, protein content, temperature, and pH impacted A. flavus growth probability in PDA systems (Kosegarten et al., 2017). In another study, binary interactions of time, type of edible film application, and EO concentration determined the growth probability of Penicillium commune, Cladosporium herbarum, and Fusarium spp. on tomatoes (Hernández-Figueroa et al., 2024). According to the predictive model (Table 4), several conditions decrease the growth probability of P. citrinum to values below 0.05. These conditions include an aw of 0.95 at a pH of 3, combined with 20–25 ppm of EO, or an aw of 0.94 at a pH of 5 and 35 ppm, as shown in Figure 3. For aw lower than 0.93 combined with a pH of 3.0, EO at <10 ppm achieves this growth probability. These conditions balance the need for a low probability of fungal growth with practical constraints in food formulation (lower EO concentration). Based on the binary logistic regression, the developed predictive framework was designed to understand the conditions that favor or inhibit A. flavus and P. citrinum growth under varying aw, pH, and EO concentration levels (Figure 3). The binary logistic regression model revealed important findings: higher aw increases fungal growth, while higher concentrations of EO inhibit it.
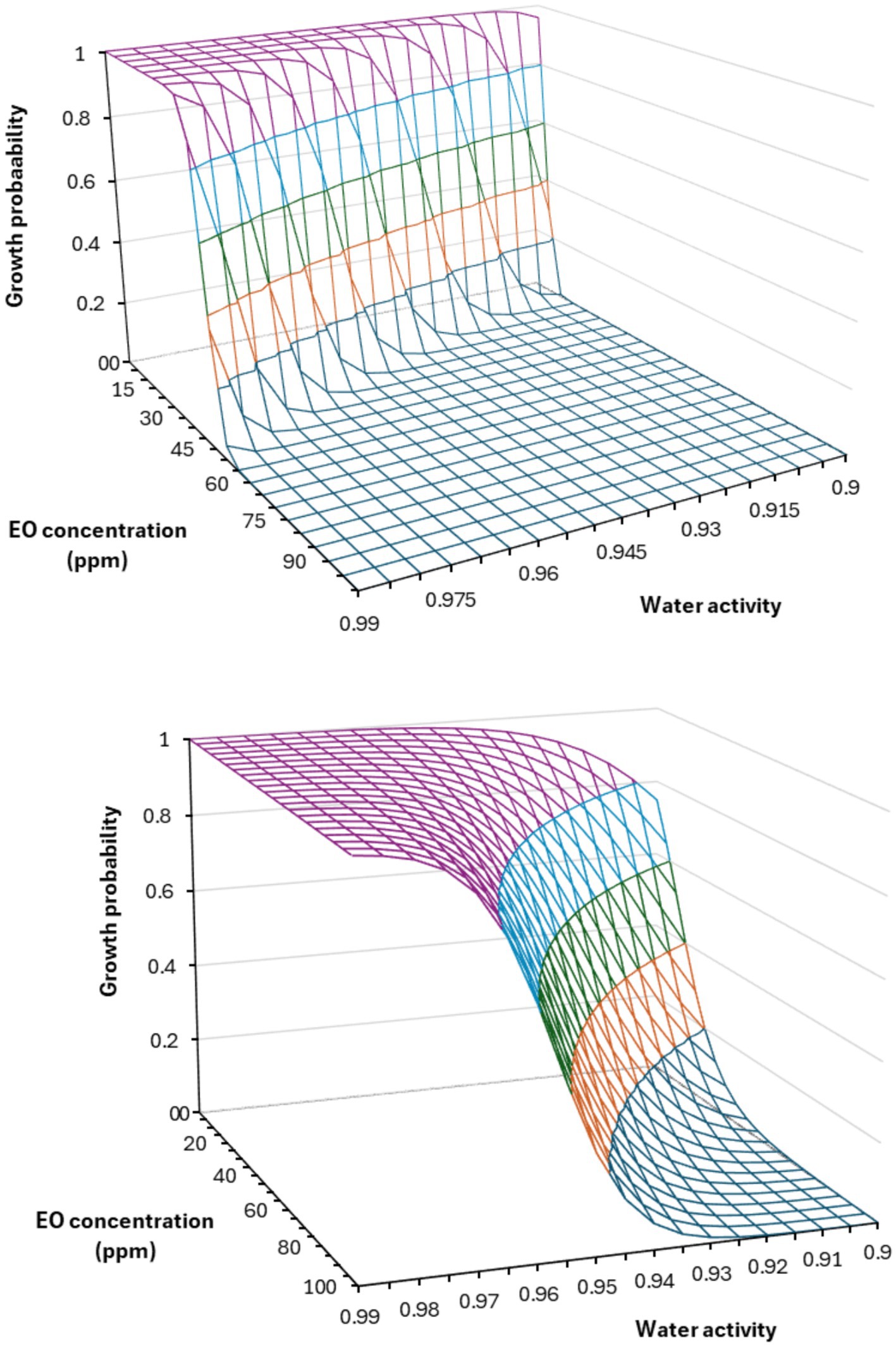
Figure 3. Probability of growth after 90 days of incubation of Penicillium citrinum (top) and Aspergillus flavus (bottom) in systems formulated at pH 4.0 and different water activities and thyme EO concentrations.
3.4 Survival model
Time-to-growth regression is an important method for analyzing time-to-event data, commonly used in survival analysis and shelf-life estimation for food products (Bandyopadhaya et al., 2024; González-Pérez et al., 2024). In food preservation, this method helps determine the length of the lag phase—the period before mold growth begins—under different environmental conditions. By modeling the effects of factors such as aw, pH, and EO concentrations, this procedure provides insights into how these variables can delay the onset of mold growth. However, it is important to note that while this method effectively quantifies growth delays, it does not describe the subsequent growth rate or the extent of mold proliferation after the lag phase (Zaffora et al., 2024; Dantigny et al., 2005; Gougouli et al., 2011). Survival analysis is valuable for examining the lag phase before mold growth, including under antifungal agents. This method helps researchers measure the time it takes for molds like Aspergillus to grow, providing insights for shelf-life extension strategies (Gómez-Ramírez et al., 2013; Kosegarten et al., 2017). Suhr and Nielsen (2004) evaluated weak acid preservatives’ effect on the growth of spoilage fungi at different aw and pH values. The obtained data was modeled using survival analysis to determine ‘spoilage-free time’ for the fungi. At the low aw level (0.80), only Eurotium species grew within the test period of 30 days. Higher aw levels and higher pH values decreased the spoilage-free times.
The time-to-growth regression method effectively quantifies conditions that delay the growth of A. flavus and P. citrinum (Figure 4). As can be seen, depending on the selected time (100, 500, or 1,500 h) to delay the growth of each mold studied, different aw and EO concentrations at pH 5 are required. In the same way, predictions of the time to growth can be made at other pHs (Table 4). In this study, aw exhibited a negative coefficient (Table 4), indicating that higher aw levels reduce the time required for growth to begin. Similarly, pH showed a negative effect, suggesting that shifting toward neutral pH accelerates mold growth. Conversely, the thyme EO concentration had a strong positive impact, significantly delaying mold growth at higher concentrations. Interaction terms showed the combined effects of the studied variables (Table 4). The interactions aw-pH, pH-EO concentration, and aw-EO concentration revealed synergistic or antagonistic effects on the mold’s time to growth. The regression model also offers practical recommendations for maximizing the mold’s lag phase under specific constraints. These findings emphasize the importance of considering combined factors rather than individual variables in preservation strategies (López-Malo et al., 2005; Mannaa and Kim, 2017; Samapundo et al., 2007). The regression model also offers practical recommendations for maximizing the lag phase of P. citrinum under specific constraints. For conditions with aw 0.95 and minimal thyme EO concentration (25 ppm), pH 5 (Figure 4) can extend the lag phase and delay growth detection. Predictions indicate that these conditions could inhibit P. citrinum growth for over 1,000 h (~42 days). A shorter lag time (20 days) had been reported for A. niger growth at aw 0.90 and <50 ppm of Mexican oregano EO in dried tomatoes (Gómez-Ramírez et al., 2013).
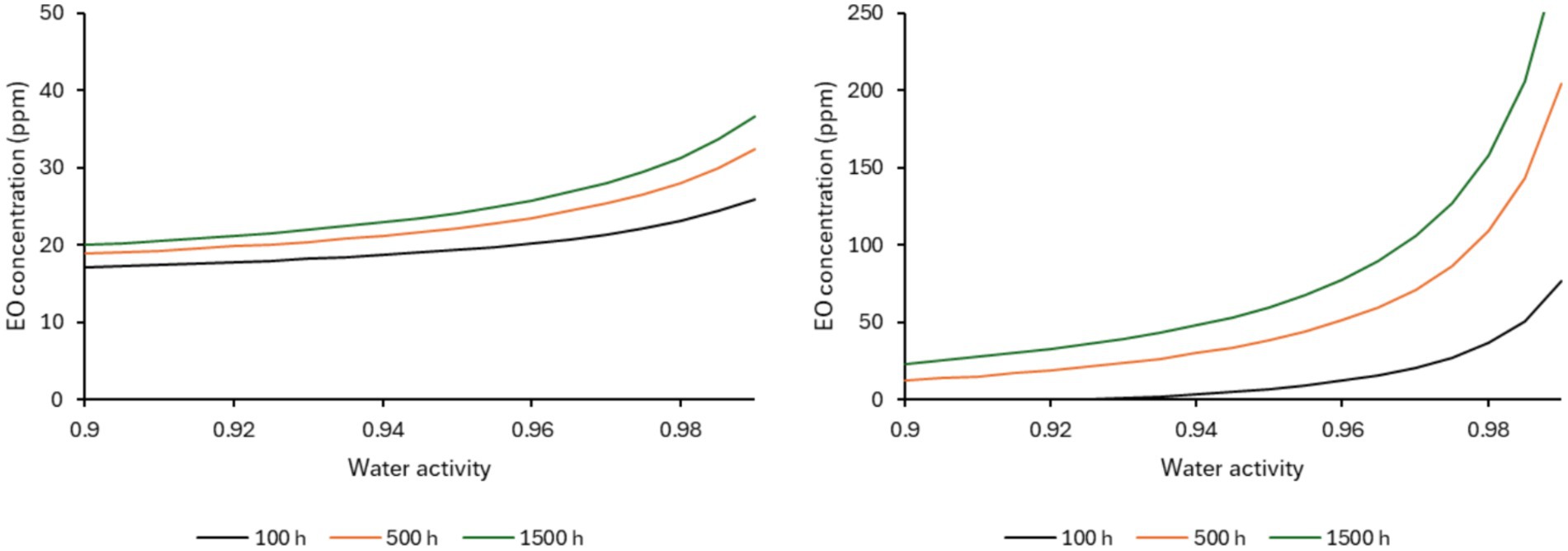
Figure 4. Essential oil (EO) concentrations required to delay the growth of Penicillium citrinum (left) and Aspergillus flavus (right) for 100, 500, or 1,500 h (solid lines), at different water activity levels and a constant pH of 5.0, predicted with the time-to-growth models (Table 4).
Based on the results of the binary logistic regression and regression with life data analyses, the growth responses of A. flavus and P. citrinum can be compared under varying aw, pH, and EO concentration conditions. For A. flavus, the logistic regression analysis indicated a strong positive effect of aw on fungal growth probability, with high aw levels significantly increasing the probability of growth. In the regression with the life data model, higher aw led to faster growth onset. In contrast, P. citrinum was slightly less sensitive to changes in aw. Although increased aw did raise the probability of growth for P. citrinum, the magnitude of the effect was less pronounced than for A. flavus. For A. flavus, pH showed only a marginal impact on both models. A lower pH (around 3–4) slightly reduced the growth probability and delayed the growth onset, but this effect was not as pronounced as the effects of aw and EO concentration. In the case of P. citrinum, pH had a more substantial influence on growth probability and time-to-growth. Marin et al. (2021) highlighted that numerous studies have been published in recent years focusing on applying predictive mycology for the early prediction of fungal spoilage. Examining the lag time before fungal colonies appear is crucial, as molds are undesirable in several food products. Many studies use a growth/no-growth approach to define the boundaries influenced by various factors. This shift in predictive mycology—focusing on lag times and the probability of colony appearance—represents a significant advancement. While the kinetic model can fully describe growth dynamics. Together, these models would give a holistic understanding of how environmental factors impact mold growth, particularly useful in food safety and shelf-life studies. Dantigny (2021) highlights that fundamental principles from process control can be applicable to process modeling, emphasizing that the simplest model that effectively represents the data is the most desirable. A thorough understanding of the process is essential since no model can encompass all the complexities of a system. Identifying key biological responses and the significant factors influencing them is important since each model provides unique insights into mold growth.
4 Conclusion
The comparison shows that each model brings unique advantages to mold inhibition studies. The binary logistic model is helpful for quick assessment of growth vs. no-growth conditions and determining threshold values. The time-to-growth regression is valuable for understanding the delay in mold growth and evaluating preservation efficacy. The kinetic model is the most comprehensive for studying mold growth dynamics, providing a complete picture of growth rates, maximum growth, and lag phases. Given each model’s distinct advantages, analyzing the data with all three approaches would be beneficial. Combining three approaches offers a clear understanding of mold inhibition by identifying threshold conditions, analyzing time delays, and assessing growth rates. This integrated method is valuable for evaluating antifungal strategies in food preservation. The study shows that a holistic modeling approach using kinetic analysis, logistic regression, and survival analysis reveals how formulation factors affect fungal growth. By adjusting aw, pH, and thyme EO concentration, food scientists can create strategies that reduce or delay fungal growth (A. flavus and P. citrinum). This is crucial for high-risk foods vulnerable to spoilage and mycotoxin contamination, ensuring microbial safety and extending shelf life.
Data availability statement
The original contributions presented in the study are included in the article/supplementary material, further inquiries can be directed to the corresponding author.
Author contributions
RH-F: Data curation, Formal analysis, Investigation, Methodology, Writing – original draft, Writing – review & editing. EM-L: Data curation, Formal analysis, Investigation, Methodology, Writing – original draft, Writing – review & editing. AL-M: Formal analysis, Investigation, Resources, Supervision, Validation, Writing – original draft, Writing – review & editing. EP: Formal analysis, Investigation, Resources, Supervision, Validation, Writing – original draft, Writing – review & editing. TC-P: Formal analysis, Investigation, Resources, Supervision, Validation, Writing – original draft, Writing – review & editing. GN-M: Formal analysis, Investigation, Resources, Supervision, Validation, Writing – original draft, Writing – review & editing. RA-S: Formal analysis, Funding acquisition, Investigation, Resources, Supervision, Validation, Writing – original draft, Writing – review & editing.
Funding
The author(s) declare that financial support was received for the research and/or publication of this article. This paper was funded by VIEP-BUAP, grant number 100377166-VIEP2024.
Acknowledgments
The authors thank the University of the Americas Puebla (UDLAP) and Benemérita Universidad Autónoma de Puebla for supporting this work.
Conflict of interest
The authors declare that the research was conducted in the absence of any commercial or financial relationships that could be construed as a potential conflict of interest.
The author(s) declared that they were an editorial board member of Frontiers, at the time of submission. This had no impact on the peer review process and the final decision.
Generative AI statement
The authors declare that no Gen AI was used in the creation of this manuscript.
Publisher’s note
All claims expressed in this article are solely those of the authors and do not necessarily represent those of their affiliated organizations, or those of the publisher, the editors and the reviewers. Any product that may be evaluated in this article, or claim that may be made by its manufacturer, is not guaranteed or endorsed by the publisher.
References
Abd El-Hack, M. E., Kamal, M., Altaie, H. A. A., Youssef, I. M., Algarni, E. H., Almohmadi, N. H., et al. (2023). Peppermint essential oil and its nano-emulsion: potential against aflatoxigenic fungus Aspergillus flavus in food and feed. Toxicon 234:107309. doi: 10.1016/j.toxicon.2023.107309
Abubakar, A., Suberu, H. A., Bello, I. M., Abdulkadir, R., Daudu, O. A., and Lateef, A. A. (2013). Effect of pH on mycelial growth and sporulation of Aspergillus parasiticus. J. Plant Sci. 1, 64–67. doi: 10.11648/j.jps.20130104.13
Alagawany, M., Farag, M. R., Abdelnour, S. A., and Elnesr, S. S. (2021). A review on the beneficial effect of thymol on health and production of fish. Rev. Aquac. 13, 632–641. doi: 10.1111/raq.12490
Aljabeili, H. S., Barakat, H., and Abdel-Rahman, H. A. (2018). Chemical composition, antibacterial and antioxidant activities of thyme essential oil (Thymus vulgaris). Food Nutr. Sci. 9, 433–446. doi: 10.4236/fns.2018.95034
Al-Maqtari, Q. A., Rehman, A., Mahdi, A. A., Al-Ansi, W., Wei, M., Yanyu, Z., et al. (2021). Application of essential oils as preservatives in food systems: challenges and future prospectives–a review. Phytochem. Rev. 21, 1209–1246. doi: 10.1007/s11101-021-09776-y
Amit, S. K., Uddin, M. M., Rahman, R., Islam, S. R., and Khan, M. S. (2017). A review on mechanisms and commercial aspects of food preservation and processing. Agric. Food Secur. 6, 1–22. doi: 10.1186/s40066-017-0130-8
Baert, K., Valero, A., De Meulenaer, B., Samapundo, S., Ahmed, M. M., Bo, L., et al. (2007). Modeling the effect of temperature on the growth rate and lag phase of Penicillium expansum in apples. Int. J. Food Microbiol. 118, 139–150. doi: 10.1016/j.ijfoodmicro.2007.07.006
Bandyopadhaya, S., Sarkar, U., and Bera, D. (2024). “Food stability simulation: accelerated shelf-life mechanism” in Food coatings and preservation technologies. ed. M. Sen (Hoboken, NJ, USA: John Wiley & Sons), 259–300.
Battilani, P., Toscano, P., Van der Fels-Klerx, H. J., Moretti, A., Camardo Leggieri, M., Brera, C., et al. (2016). Aflatoxin B1 contamination in maize in Europe increases due to climate change. Sci. Rep. 6:24328. doi: 10.1038/srep24328
Carocho, M., Barreiro, M. F., Morales, P., and Ferreira, I. C. (2014). Adding molecules to food, pros and cons: a review on synthetic and natural food additives. Compr. Rev. Food Sci. Food Saf. 13, 377–399. doi: 10.1111/1541-4337.12065
Dagnas, S., and Membré, J. M. (2013). Predicting and preventing mold spoilage of food products. J. Food Prot. 76, 538–551. doi: 10.4315/0362-028X.JFP-12-349
Dagnas, S., Onno, B., and Membré, J.-M. (2014). Modeling growth of three bakery product spoilage molds as a function of water activity, temperature and pH. Int. J. Food Microbiol. 186, 95–104. doi: 10.1016/j.ijfoodmicro.2014.06.022
Dantigny, P. (2021). Applications of predictive modeling techniques to fungal growth in foods. Curr. Opin. Food Sci. 38, 86–90. doi: 10.1016/j.cofs.2020.10.028
Dantigny, P., Guilmart, A., and Bensoussan, M. (2005). Basis of predictive mycology. Int. J. Food Microbiol. 100, 187–196. doi: 10.1016/j.ijfoodmicro.2004.10.013
Debonne, E., Van Bockstaele, F., De Leyn, I., Devlieghere, F., and Eeckhout, M. (2018). Validation of in-vitro antifungal activity of thyme essential oil on Aspergillus Niger and Penicillium paneum through application in par-baked wheat and sourdough bread. LWT 87, 368–378. doi: 10.1016/j.lwt.2017.09.007
Debonne, E., Vermeulen, A., Van Bockstaele, F., Soljic, I., Eeckhout, M., and Devlieghere, F. (2019). Growth/no-growth models of in-vitro growth of Penicillium paneum as a function of thyme essential oil, pH, aw, temperature. Food Microbiol. 83, 9–17. doi: 10.1016/j.fm.2019.04.003
Dobson, A. D. W. (2011). “Yeasts and molds: Aspergillus flavus” in Encyclopedia of dairy sciences. ed. J. W. Fuquay. 2nd ed (San Diego, CA, USA: Academic Press), 785–791.
Escobar, A., Perez, M., Romanelli, G., and Blustein, G. (2020). Thymol bioactivity: a review focusing on practical applications. Arab. J. Chem. 13, 9243–9269. doi: 10.1016/j.arabjc.2020.11.009
Etri, K., and Pluhár, Z. (2024). Exploring chemical variability in the essential oils of the thymus genus. Plan. Theory 13:1375. doi: 10.3390/plants13101375
Favetto, G. J., and Chirife, J. (1985). Simplified method for the prediction of water activity in binary aqueous solutions. Int. J. Food Sci. Technol. 20, 631–636. doi: 10.1111/j.1365-2621.1985.tb01822.x
Gómez-Ramírez, C., Sosa-Morales, M. E., Palou, E., and López-Malo, A. (2013). Aspergillus niger time to growth in dried tomatoes. Int. J. Food Microbiol. 164, 23–25. doi: 10.1016/j.ijfoodmicro.2013.03.017
González-Pérez, J. E., Jiménez-González, O., Romo-Hernández, A., López-Malo, A., and Ramírez-Corona, N. (2024). Time-to-failure approach for estimating the shelf life of freeze-dried carotenoid-enriched apples: forecasting the deterioration of quality properties for different packaging types and storage conditions. ACS Agric. Sci. Technol. 4, 1241–1249. doi: 10.1021/acsagscitech.4c00465
Gougouli, M., Kalantzi, K., Beletsiotis, E., and Koutsoumanis, K. P. (2011). Development and application of predictive models for fungal growth as tools to improve quality control in yogurt production. Food Microbiol. 28, 1453–1462. doi: 10.1016/j.fm.2011.07.006
Gougouli, M., and Koutsoumanis, K. P. (2017). Risk assessment of fungal spoilage: a case study of Aspergillus niger on yogurt. Food Microbiol. 65, 264–273. doi: 10.1016/j.fm.2017.03.009
Henley-Smith, C. J., Steffens, F. E., Botha, F. S., and Lall, N. (2014). Predicting the influence of multiple components on microbial inhibition using a logistic response model-a novel approach. BMC Complementary Altern. Med. 14, 1–10. doi: 10.1186/1472-6882-14-190
Hernández-Figueroa, R. H., Mani-López, E., and López-Malo, A. (2024). Antifungal activity of alginate coatings with essential oil of Mexican oregano incorporated in the stem of tomatoes. Int. J. Food Sci. Technol. 59, 4774–4783. doi: 10.1111/ijfs.17207
Houbraken, J., and Samson, R. A. (2017). Current taxonomy and identification of foodborne fungi. Curr. Opin. Food Sci. 17, 84–88. doi: 10.1016/j.cofs.2017.10.010
Hu, L.-B., Ban, F.-F., Li, H.-B., Qian, P.-P., Shen, Q.-S., Zhao, Y.-Y., et al. (2018). Thymol induces conidial apoptosis in Aspergillus flavus via stimulating K+ eruption. J. Agric. Food Chem. 66, 8530–8536. doi: 10.1021/acs.jafc.8b02117
Kosegarten, C. E., Ramírez-Corona, N., Mani-López, E., Palou, E., and López-Malo, A. (2017). Description of Aspergillus flavus growth under the influence of different factors (water activity, incubation temperature, protein and fat concentration, pH, and cinnamon essential oil concentration) by kinetic, probability of growth, and time-to-detection models. Int. J. Food Microbiol. 240, 115–123. doi: 10.1016/j.ijfoodmicro.2016.04.024
Lainez-Cerón, E., López-Malo, A., Palou, E., and Ramírez-Corona, N. (2022). Dynamic performance of optimized microwave assisted extraction to obtain Eucalyptus essential oil: energy requirements and environmental impact. Int. J. Food Eng. 18, 129–142. doi: 10.1515/ijfe-2021-0102
Leistner, L., and Gould, G. W. (2002). “Hurdles in foods” in Hurdle technologies: combination treatments for food stability, safety, and quality. eds. L. Leistner and G. W. Gould (Boston, MA: Springer), 47–50.
Leyva Salas, M., Mounier, J., Valence, F., Coton, M., Thierry, A., and Coton, E. (2017). Antifungal microbial agents for food biopreservation—a review. Microorganisms 5:37. doi: 10.3390/microorganisms5030037
López-Malo, A., Alzamora, S. M., and Palou, E. (2005). Aspergillus flavus growth in the presence of chemical preservatives and naturally occurring antimicrobial compounds. Int. J. Food Microbiol. 99, 119–128. doi: 10.1016/j.ijfoodmicro.2004.08.010
Mandal, S., and DebMandal, M. (2016). “Thyme (Thymus vulgaris L.) oils” in Essential oils in food preservation, flavor and safety (San Diego, CA, USA: Academic Press), 825–834.
Mannaa, M., and Kim, K. D. (2017). Influence of temperature and water activity on deleterious fungi and mycotoxin production during grain storage. Mycobiology 45, 240–254. doi: 10.5941/MYCO.2017.45.4.240
Marin, S., Freire, L., Femenias, A., and Sant’Ana, A. S. (2021). Use of predictive modelling as tool for prevention of fungal spoilage at different points of the food chain. Curr. Opin. Food Sci. 41, 1–7. doi: 10.1016/j.cofs.2021.02.006
Najar, B., Pistelli, L., Ferri, B., Angelini, L. G., and Tavarini, S. (2021). Crop yield and essential oil composition of two Thymus vulgaris chemotypes along three years of organic cultivation in a hilly area of central Italy. Molecules 26:5109. doi: 10.3390/molecules26165109
Nielsen, P. V., and Rios, R. (2000). Inhibition of fungal growth on bread by volatile components from spices and herbs, and the possible application in active packaging, with special emphasis on mustard essential oil. Int. J. Food Microbiol. 60, 219–229. doi: 10.1016/S0168-1605(00)00343-3
Odeyemi, O. A., Alegbeleye, O. O., Strateva, M., and Stratev, D. (2020). Understanding spoilage microbial community and spoilage mechanisms in foods of animal origin. Compr. Rev. Food Sci. Food Saf. 19, 311–331. doi: 10.1111/1541-4337.12526
Oliveira, R. C., Carvajal-Moreno, M., Correa, B., and Rojo-Callejas, F. (2020). Cellular, physiological and molecular approaches to investigate the antifungal and anti-aflatoxigenic effects of thyme essential oil on Aspergillus flavus. Food Chem. 315:126096. doi: 10.1016/j.foodchem.2019.126096
Pardo, E., Marin, S., Ramos, A. J., and Sanchis, V. (2006). Ecophysiology of ochratoxigenic Aspergillus ochraceus and Penicillium verrucosum isolates. Predictive models for fungal spoilage prevention–a review. Food Additives and Contaminants, 23, 398–410. doi: 10.1080/02652030500376102
Paris, M. J., Ramírez-Corona, N., Palou, E., and López-Malo, A. (2020). Modelling release mechanisms of cinnamon (Cinnamomum zeylanicum) essential oil encapsulated in alginate beads during vapor-phase application. J. Food Eng. 282:110024. doi: 10.1016/j.jfoodeng.2020.110024
Pitt, J. I. (2006). “Penicillium and related genera” in Food spoilage microorganisms. ed. C. W. Blackburn (Cambridge, UK: Woodhead Publishing Ltd), 437–450.
Pitt, J. I., and Hocking, A. D. (2022). “Ecology of fungal food spoilage” in Fungi and food spoilage (Cham: Springer International Publishing), 3–12.
Pleadin, J., Frece, J., and Markov, K. (2019). “Mycotoxins in food and feed” in Advances in food and nutrition research. ed. F. Toldrá, vol. 89 (Cambridge, MA, USA: Academic Press), 297–345.
Pluhár, Z., Kun, R., Cservenka, J., Neumayer, É., Tavaszi-Sárosi, S., Radácsi, P., et al. (2024). Variations in essential oil composition and chemotype patterns of wild thyme (Thymus) species in the natural habitats of Hungary. Horticulturae 10:150. doi: 10.3390/horticulturae10020150
Possas, A., Bonilla-Luque, O. M., and Valero, A. (2021). From cheese-making to consumption: exploring the microbial safety of cheeses through predictive microbiology models. Food Secur. 10:355. doi: 10.3390/foods10020355
Ranjbar, A., Ramezanian, A., Shekarforoush, S., Niakousari, M., and Eshghi, S. (2022). Antifungal activity of thymol against the main fungi causing pomegranate fruit rot by suppressing the activity of cell wall degrading enzymes. LWT 161:113303. doi: 10.1016/j.lwt.2022.113303
Reyes-Jurado, F., Franco-Vega, A., Ramírez-Corona, N., Palou, E., and López-Malo, A. (2015). Essential oils: antimicrobial activities, extraction methods, and their modeling. Food Eng. Rev. 7, 275–297. doi: 10.1007/s12393-014-9099-2
Rico-Munoz, E., Samson, R. A., and Houbraken, J. (2019). Mould spoilage of foods and beverages: using the right methodology. Food Microbiol. 81, 51–62. doi: 10.1016/j.fm.2018.03.016
Samapundo, S., Devlieghere, F., Geeraerd, A. H., De Meulenaer, B., Van Impe, J. F., and Debevere, J. (2007). Modelling of the individual and combined effects of water activity and temperature on the radial growth of Aspergillus flavus and A. parasiticus on corn. Food Microbiol. 24, 517–529. doi: 10.1016/j.fm.2006.07.021
Schlösser, I., and Prange, A. (2019). Effects of selected natural preservatives on the mycelial growth and ochratoxin A production of the food-related moulds Aspergillus westerdijkiae and Penicillium verrucosum. Food Addit. Contam. Part A 36, 1411–1418. doi: 10.1080/19440049.2019.1640397
Suhr, K. I., and Nielsen, P. V. (2003). Antifungal activity of essential oils evaluated by two different application techniques against rye bread spoilage fungi. J. Appl. Microbiol. 94, 665–674. doi: 10.1046/j.1365-2672.2003.01896.x
Suhr, K. I., and Nielsen, P. V. (2004). Effect of weak acid preservatives on growth of bakery product spoilage fungi at different water activities and pH values. Int. J. Food Microbiol. 95, 67–78. doi: 10.1016/j.ijfoodmicro.2004.02.004
Taiwo, O. R., Onyeaka, H., Oladipo, E. K., Oloke, J. K., and Chukwugozie, D. C. (2024). Advancements in predictive microbiology: integrating new technologies for efficient food safety models. Int. J. Food Microbiol. 2024:6612162. doi: 10.1155/2024/6612162
Tannous, J., Atoui, A., El Khoury, A., Francis, Z., Oswald, I. P., Puel, O., et al. (2016). A study on the physicochemical parameters for Penicillium expansum growth and patulin production: effect of temperature, pH, and water activity. Food Sci. Nutr. 4, 611–622. doi: 10.1002/fsn3.324
Tapia, M. S., Alzamora, S. M., and Chirife, J. (2020). “Effects of water activity (aw) on microbial stability as a hurdle in food preservation” in Water activity in foods: fundamentals and applications, Hoboken, NJ, USA: John Wiley & Sons, Inc. 323–355.
Tarlak, F. (2023). The use of predictive microbiology for the prediction of the shelf life of food products. Food Secur. 12:4461. doi: 10.3390/foods12244461
Thompson, D. P. (1990). Influence of pH on the fungitoxic activity of naturally occurring compounds. J. Food Prot. 53, 428–429. doi: 10.4315/0362-028X-53.5.428
Wang, Z., Wang, C. Y., Wang, Y. B., Xue, J. J., Li, Z., Li, J. J., et al. (2013). Effect of different pH values on growth and sporulation of Estye vermicola. Afr. J. Microbiol. Res. 7, 3217–3221. doi: 10.5897/AJMR12.783
Wheeler, K. A., Hurdman, B. J., and Pitt, J. I. (1991). Influence of pH on the growth of some toxigenic species of Aspergillus, Penicillium and Fusarium. Int. J. Food Microbiol. 12, 141–149. doi: 10.1016/0168-1605(91)90063-U
Wijnker, J. J., Koop, G., and Lipman, L. J. A. (2006). Antimicrobial properties of salt (NaCl) used for the preservation of natural casings. Food Microbiol. 23, 657–662. doi: 10.1016/j.fm.2005.11.004
Zaffora, B., Coisne, L., and Gérard, C. (2024). Survival models to estimate time to visible mold growth on new paper-based food-contact materials under varying environmental conditions. LWT 193:115767. doi: 10.1016/j.lwt.2024.115767
Keywords: thyme essential oil, fungal growth inhibition, Aspergillus flavus, Penicillium citrinum, predictive modeling
Citation: Hernández-Figueroa RH, Mani-López E, López-Malo A, Palou E, Cid-Pérez TS, Nevárez-Moorillón GV and Avila-Sosa R (2025) Antifungal efficacy of thyme essential oil: a multi-model approach to growth inhibition. Front. Sustain. Food Syst. 9:1535812. doi: 10.3389/fsufs.2025.1535812
Edited by:
Pedro Ferreira Santos, University of Vigo, SpainReviewed by:
Leonardo do Prado-Silva, University of São Paulo, Ribeirão Preto, BrazilHimanshu Arora, Indian Institute of Technology Delhi, India
Copyright © 2025 Hernández-Figueroa, Mani-López, López-Malo, Palou, Cid-Pérez, Nevárez-Moorillón and Avila-Sosa. This is an open-access article distributed under the terms of the Creative Commons Attribution License (CC BY). The use, distribution or reproduction in other forums is permitted, provided the original author(s) and the copyright owner(s) are credited and that the original publication in this journal is cited, in accordance with accepted academic practice. No use, distribution or reproduction is permitted which does not comply with these terms.
*Correspondence: Raúl Avila-Sosa, cmF1bC5hdmlsYUBjb3JyZW8uYnVhcC5teA==