- Michigan Institute for Data and AI in Society (MIDAS), University of Michigan, Ann Arbor, MI, United States
Floods are prevalent disasters in the United States (U.S.), with escalating risks due to climate change-induced factors like rising sea levels and erratic rainfall patterns. While organizational efforts to mitigate the social and economic impacts of floods exist, there is a continued need for innovative approaches to flood management, particularly in flood risk communication. Currently, flood risk communication remains largely one-directional (i.e., communication comes from organizational entities such as emergency services or the media and is not necessarily informed or updated by community need, which can limit preparedness and response capabilities). Prior studies have found that social media platforms offer valuable opportunities for more interactive and real-time dialogue during disasters, including flood events. Additionally, there is an established body of research exploring the communication relationships between communities and organizational entities, respectively; highlighting opportunities to better develop pathways for two-way communications. Our study builds upon this literature by examining the alignment between community and organizational messages on social media during a series of flooding events affecting nine U.S. states from July 2022 to August 2022. Specifically, the study uses Natural Language Processing (NLP) to compare the content, sentiment, and emotion of messages from each entity to assess whether organizational communications reflect public needs and effectively foster two-way communication. Results reveal key temporal and spatial trends in message frequency and topic focus across communities and organizational entities, from which we develop five targeted recommendations designed to support the execution of more effective two-way flood communication. Future work will provide further insights into tailoring communication strategies to more diverse populations and circumstances.
1 Introduction
Floods are one of the most common weather-related natural disasters in the United States (NOAA National Severe Storms Laboratory, 2024). Seventy-five percent of all Presidential Disaster Declarations in the United States can be attributed to flooding (National Weather Service, 2018). As climate change continues to contribute to rising sea levels and unstable rainfalls, the risk of floods is increasing (Tabari, 2020). The U.S. National Flood Insurance Program experienced a 660% increase in dollars paid out for flood insurance claims from 2000 to 2020 compared to the claims from 1980 to 2000 (FEMA, 2021).
Beyond flood damage to physical systems, floods have been documented to substantially impact communities’ physical, mental, and financial health, causing long-term changes to the quality of life that can persist for years after the flood event (Van Ootegem and Verhofstadt, 2016). For example, studies have shown increased instances of anxiety, depression, and post-traumatic stress disorder in people who have experienced a flood compared to a control group (Van Ootegem and Verhofstadt, 2016). Additionally, those affected by floods have been reported to experience long- or short-term injuries, such as respiratory and gastrointestinal illness due to exposure to pathogens via contaminated drinking water, unsafe conditions for food preparation, or lack of access to hygienic practices, and, in some cases, carbon monoxide poisoning (Burger and Gochfeld, 2014; Mulder et al., 2019; Sampson et al., 2019; Van Ootegem and Verhofstadt, 2016; Waite et al., 2014). Moreover, these effects are not experienced independently of one another; a systematic review of factors related to one’s vulnerability before, during, and after a flood event determined that the effects of floods can be compounded by other risk factors, such as the frequency and severity of floods experienced or health, socioeconomic, and educational status (Lowe et al., 2013; Sampson et al., 2019).
Given the ongoing, multifaceted nature of floods, it is crucial to examine the current state of flood communications to better protect society, explore communication mediums that can provide real-time insights into unfolding events, and apply emerging technological advances to analyze this data effectively.
2 Background
2.1 State of flood communications
The magnitude and widespread effects of floods, as well as their increasing risk of occurrence, have motivated studies to examine the information available to those experiencing a flood before, during, and after the event. The primary means through which communities receive information about a flood is via flood risk messages from an organizational entity, such as public health, weather service, or emergency management agencies on a federal, state, or local level. However, studies investigating the effectiveness of flood risk messages from these sources have found that they are inadequate for improving individual self-efficacy, adaptive capacity, and flood preparedness (Forsyth et al., 2023; Haer et al., 2016; Scott and Errett, 2018; Rollason et al., 2018). For example, a study examining the information available on social media via the government during the 2016 Louisiana floods found that, while most messages had information about recovery resources and updates about the status of the disaster and response, few contained “actionable requests” or requests that were substantial and relevant enough to those experiencing the flood that they increased self-efficacy (Cooper et al., 2022; Mostafiz et al., 2022; Scott and Errett, 2018). Furthermore, while some agencies release “Get Ready” checklists as a tool for preparedness before a flood, they do not adequately cover the long-term impacts on mental health and overall well-being that may be experienced (Forsyth et al., 2023). Current flood risk communication practices are still primarily based on a knowledge- or information-deficit model, which perpetuates one-sided communication from an organizational entity to the community (Maidl and Buchecker, 2015). These models are based on the assumption that the public does not understand the risk and that simply providing more information will result in “better” decision-making (Maidl and Buchecker, 2015; O’Sullivan et al., 2012). This assumption emphasizes the need for using a platform that facilitates two-way communication for risk messages, enabling organizational entities to share actionable and relevant flood risk information while actively engaging with community-driven discussions.
2.2 Social media and flood communications
Flood research highlights two primary channels for disseminating risk information: traditional media and emerging social media platforms (Feldman et al., 2016). Social media has increasingly been recognized as a valuable source of information during disasters (Houston et al., 2015; Intrieri et al., 2020; Reuter et al., 2018; Wukich, 2016), including floods. Indeed, to improve flood mitigation and management practices, several studies have demonstrated the effectiveness of community-based social media messages as early warning indicators of floods (Cools et al., 2016; Villagrán De León et al., 2013). For example, Starbird et al. (2010) published a study examining online interactions on Twitter/X during a flood in 2009, just 3 years after Twitter/X was launched. A study analyzing the content of 60,000 tweets over a 5-day flood period found evidence of early and local detection of multiple flood events (Shoyama et al., 2021). Additionally, a method for identifying flood events globally using social media messages to build a real-time and historic database has been created (de Bruijn et al., 2019), advancing the use of social media as a reliable source of critical information related to floods. Research has also illustrated that social media can significantly reduce flood-related losses (in U.S. dollars) for households that use such platforms (Allaire, 2016), by simply having access to additional information not typically provided by traditional outlets.
Common concerns for decision-makers regarding the use of social media as a credible communication channel include the spread of misinformation and disinformation and the prevalence of fake news, among other challenges (Aïmeur et al., 2023). However, social media has long been recognized by the public as a reliable source of information, often considered on par with traditional media outlets, and has become a societal norm for staying informed and accessing news, even amid concerns about potential biases or inaccuracies (Flanagin and Metzger, 2000). Furthermore, studies have found that social media users often actively addressed these challenges, such as misinformation, during past disaster events (Zubiaga et al., 2018). Typically, social media users are more likely to critically evaluate and question false information than to accept it (Mendoza et al., 2010), thus challenging the common narrative about the unreliability of social media and providing more confidence in its usability in risk communication opportunities. Research examining messages disseminated by organizational entities on social media has identified the tendency for such entities to favor a one-sided communication strategy, which may not effectively address the needs of the public during disaster (Graham et al., 2015; Lovari and Bowen, 2020; Lovari and Parisi, 2015; Pourebrahim et al., 2019; Scott and Errett, 2018; Salley et al., 2024a; Waters and Williams, 2011). The use of social media as a channel for risk communication provides the opportunity to critically examine messages from both the community and organizational entities to better understand if these messages align for productive risk communication. Natural Language Processing (NLP) provides an effective means to examine such messages efficiently.
2.3 Natural language processing techniques for analyzing social media
NLP techniques like Named Entity Recognition (NER), topic modeling, and sentiment and emotion analysis offer powerful tools for automating the analysis of social media responses to flood events. NER is a method used to automatically identify and classify key information in text, such as names of people, locations, organizations, or other relevant categories (Li et al., 2020). This helps extract structured data from unstructured social media posts, allowing researchers to pinpoint areas affected by flooding, identify organizations involved in relief efforts, or highlight key individuals mentioned in discussions.
Topic modeling is an NLP technique that generates structured text data from unstructured text data inputs, and in doing so, allows for the discovery of latent topics in the text. Analyzing social media messages for content using topic modeling has been well-established in literature. In the disaster space, for example, topic modeling has been used to identify the sentiments and thoughts of populations during flood events (Choirul Rahmadan et al., 2020), hurricanes (Mihunov et al., 2022; Zhou et al., 2023), and earthquakes (Vo and Collier, 2013). A recent study applied BERTopic modeling to draw information from German tweets about floods between 2014 and 2022, tracking topic frequency over time (Veigel et al., 2024). The advent of Artificial Intelligence (AI) NLP models, such as transformer-based models that use attention mechanisms to improve language understanding, allows researchers to harness AI to more efficiently and more accurately analyze and interpret large text corpora for flood-related events (Devlin et al., 2019). Social media data and AI NLP models can aid in, for example, assessing flood severity (Kanth et al., 2022), gauging public sentiments (Li et al., 2023), and informing rescue and search activities (Wang et al., 2020).
Lastly, the analysis of sentiment and emotion (i.e., what we deem as overall disposition in this paper) provides more information about the opinion or affect within the message. In the analysis of risk messages, it is important to distinguish between sentiment and emotion. In this study, sentiments are defined as indicators of opinion that express a judgment about something. Sentiment is important to consider in the context of risk messages. For example, the sentiment (negative, positive, or neutral) of messages from organizational channels can both communicate opinions to message receivers and potentially influence their perspectives, regardless of whether this influence is intentional. Furthermore, the sentiment of messages from the public can help evaluate how well disaster response and recovery efforts were received (Ragini et al., 2018) or provide a sense of the societal impacts of the disaster itself (Fan et al., 2020). Emotions, on the other hand, are affective states. Emotional appeals have been used in risk communication to persuade the public to alter their behavior or take a specific action (Ho et al., 2024). For example, risk messages during the COVID-19 pandemic contained emotional appeals related to hope, fear, anger, humor, among others (Ho et al., 2024). There is evidence that risk communication that is too neutral or lacking empathy hinders the efficacy of the risk message (Campbell and Babrow, 2004). By utilizing a combination of NLP techniques, such as NER, topic modeling, and sentiment and emotion analysis, it is possible to efficiently analyze large volumes of social media data to understand entity reactions, identify hotspots of concern, and gauge the overall disposition toward emergency efforts.
The primary purpose of this study is to conduct a comparative analysis of social media messages from community members and organizational entities during a major flooding event between July 2022 to August 2022 that impacted multiple areas to (1) understand similarities and differences in their content and disposition and (2) inform recommendations for best practices. This work is motivated by literature identifying the tendency for risk communication to be one-sided, which can result in the failure to actively engage with community knowledge, needs, and concerns (Demeritt and Nobert, 2014; Lovari and Bowen, 2020). Advanced NLP techniques such as NER, topic modeling, and sentiment and emotion analysis offer the opportunity to study this phenomenon by enabling the rapid analysis and comparison of social media messages between communities and organizational entities. This approach can uncover insights into how flood communication strategies can better align with the lived experiences and priorities of affected communities, fostering more effective, inclusive, and responsive flood management. To that end, we aim to answer the following two-part research question: How do social media messages from community members and organizational entities during a flood event differ in content and disposition, and how can these insights inform recommendations to bridge gaps between organizational messaging and community needs?
3 Methods
3.1 Case study
To investigate the research question and address the aforementioned gap, an illustrative case study analysis of nine states (Arizona, California, Illinois, Kentucky, Mississippi, Missouri, Nevada, Virginia, and West Virginia) that were affected by flash floods occurring between July 2022 and August 2022 was performed (FEMA, 2022a). This cluster of flash flood events was chosen because it provides a unique opportunity to analyze multiple instances of risk communication (1) within a well-defined and cohesive timeframe of significant flash flood occurrences, and (2) across similar or, in some cases, interconnected flood events of comparable severity. Three of the included states (Illinois, Missouri, and Kentucky) had declared disasters listed by the Federal Emergency Management Agency (FEMA) during the selected period (FEMA, 2022a). Additionally, a state of emergency was declared in Missouri, Kentucky, West Virginia, Arizona, Nevada, and Illinois (AFSPA, 2022; FEMA, 2024). The first flood event started on July 24, in Illinois and Missouri, and resulted in a new record 24-hour rainfall in St. Louis, Missouri, beating the previous record set by the 1915 Galveston hurricane (National Weather Service, 2022). Historic flooding in the Appalachia area, including Kentucky, West Virginia, and Virginia, began on July 26, resulting in 38 deaths directly related to the flooding (Associated Press, 2022a). In Las Vegas, Nevada, an unprecedented flash flood began on July 28, with more flooding affecting Arizona, California, and Kentucky between July 30 and August 1 (Gilbert, 2022). For many of these events, there were record rainfalls, widespread power outages, significant damage to buildings and homes, road closures, and at least one injury or fatality, which may have prompted discussion by those throughout the affected states on social media. In the following section, we detail our approach for analyzing these series of severe flooding events, providing a step-by-step walkthrough of our methodology, as depicted in Figure 1.
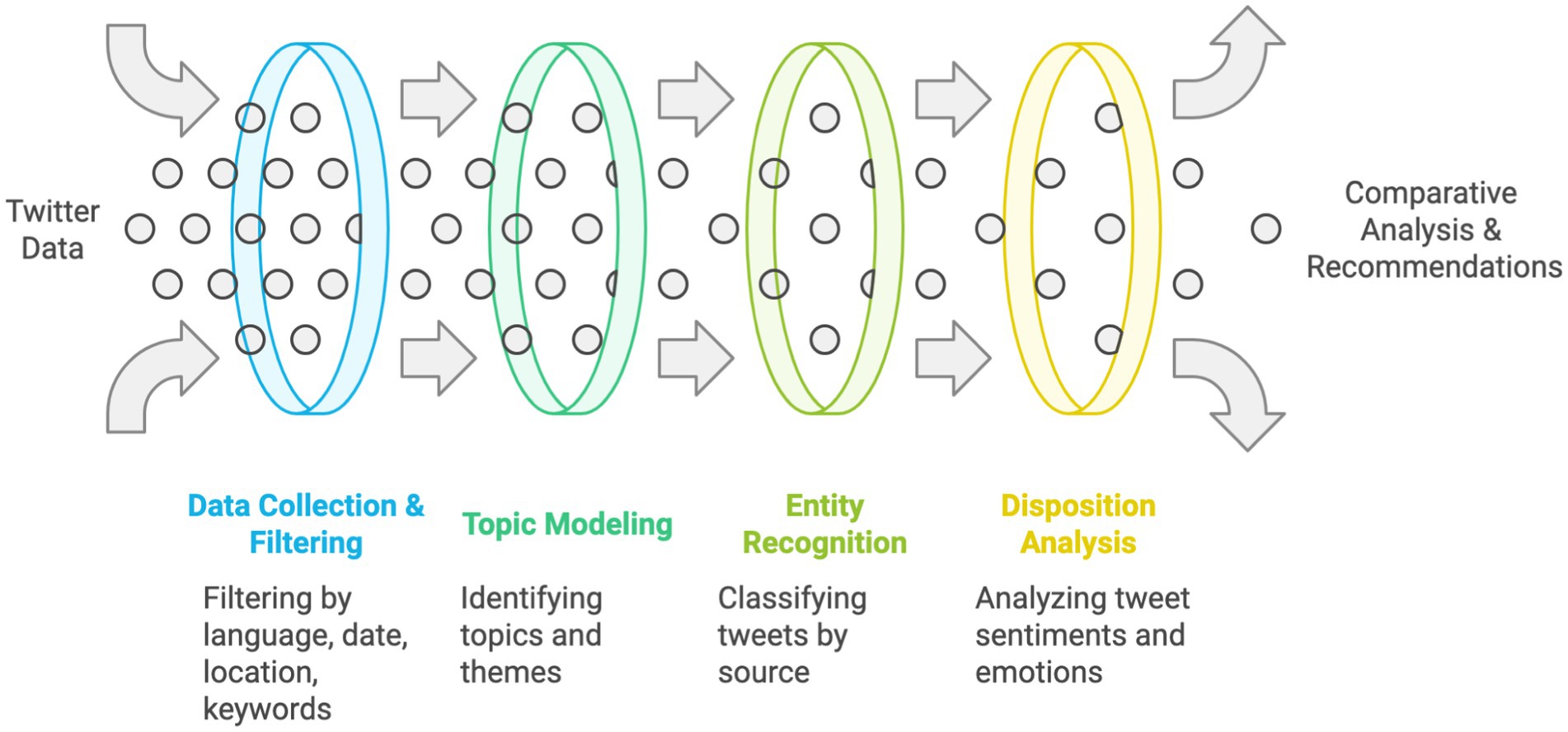
Figure 1. Research workflow outlining the sequential steps from data gathering via Twitter/X Streaming API (archived data) to advanced NLP analyses to transform data, culminating in comparative insights and actionable recommendations.
3.2 Data collection and filtering
Data were gathered through a multi-step filtering process. First, data were filtered based on language (English) and geographic location (the nine selected states). Then, data were filtered to include only the specified dates encompassing the flood events included in the case study. The first flood event began on July 24, marking the starting point of active floods occurring in this study. Notably, the last incident to be included in this analysis was the July 26 to August 11 series of severe storms, floods, landslides, and mudslides in the state of Kentucky. This series triggered a FEMA Disaster Declaration (FEMA, 2022b). In previous disaster studies, flooding is a prime example of what is known as a short-notice disaster (Chiu and Zheng, 2007). This means agencies typically have only 24–72 hours to send out important risk communication messages, such as advisories or evacuation notices (Wolshon et al., 2001). Therefore, the date range was selected to capture the average time frame typically associated with a short-notice disaster response period (i.e., 48 hours), and also 7 days after the end of the last recorded incident similar to a previous study (Salley et al., 2024b). Thus, Twitter/X data (i.e., tweets) were collected for each of the nine affected states between July 22 (48 hours before the start of the first flood event) and August 18 (7 days post the end of the last flood event) using archived data from a Twitter/X streaming API built in the Network Dynamics Lab (Wang and Taylor, 2016). After filtering for language, location, and date, the final step in the multi-step filtering process was to identify tweets directly related to flooding. To achieve this, we constructed and then applied a glossary of 70 flood-related terms, consistent with methodologies used in previous research (Salley et al., 2024b) (i.e., based on common words found within local to federal agencies and documents related to floods) (Supplementary Table S1). After all filtering steps were completed, 118,146 tweets from between July 22 to August 18 were captured for further analysis.
3.3 Topic modeling
To explore the thematic content of tweets related to flooding events, the BERTopic framework, which combines transformer-based embeddings with clustering techniques to extract coherent topics from textual data, was employed. Given the scale and complexity of the dataset, we opted for dimensionality reduction and clustering techniques to efficiently group tweets into meaningful topics. The BERTopic workflow involves embedding documents, reducing their dimensionality with Uniform Manifold Approximation and Projection (UMAP), clustering the embeddings using HDBSCAN, and creating topic representations from those clusters (Wang et al., 2023). For dimensionality reduction, we utilized the UMAP algorithm. The choice of 10 neighbors ensured that each point is influenced by its local context, capturing the nuanced relationships between semantically similar tweets. Reducing to 5 components allowed us to retain the most critical dimensions while filtering out noise, which is particularly important when dealing with high-dimensional embeddings generated by transformer models (Dalmia and Sia, 2021). Additionally, setting the minimum distance to 0.0 helped maximize cluster density, ensuring that similar tweets are tightly grouped. Using the cosine metric enabled the model to focus on the angular distance between data points, which is well-suited for high-dimensional text embeddings. Following dimensionality reduction, we applied Hierarchical Density-Based Spatial Clustering of Applications with Noise (HDBSCAN) to identify clusters within the reduced data space. We set the minimum cluster size to 295 (0.25% of all Tweets) to ensure that only robust, meaningful clusters were retained, reducing the impact of noise and small, spurious groupings (Campello et al., 2015). The minimum sample parameter of 20 controlled the sensitivity of the clustering process, determining how conservative the algorithm is in classifying points as outliers.
These choices were guided by the need to balance computational efficiency with the interpretability of the resulting topics (Wang et al., 2023). By optimizing UMAP and HDBSCAN parameters, we ensured that the model could identify well-defined topics that captured the diverse ways entities responded to flooding events on Twitter/X. The resulting 64 topics were further refined by merging semantically similar clusters or excluding topics that were deemed to be unrelated to floods (e.g., “Lakers,” which appeared due to its similarity to the keyword “lake,” was excluded as it pertained to a basketball team rather than flood-related content), enhancing the coherence and relevance of the final topic set (Supplementary Figure S1). We excluded 42 topics from the initial 64 and clustered all of the remaining 22 topics into five topics related to “Traffic,” “Weather,” “Operations,” “Society,” and “Power.” The categorization process involved manually analyzing the words and phrases associated with each topic. First, individual labels were assigned based on the most prominent terms within each topic. Then, commonalities across topics were identified, and broader labels were applied to form the five final classifications. Joining topics in topic modeling is useful when closely related topics are fragmented, which makes interpretation and thematic continuity (e.g., two topics on power outage blackouts/brownouts) difficult. This helps create more coherent and semantically meaningful topics while preserving distinctions in other topics (Hu et al., 2014). While automatic topic naming can be carried out through methods such as generative AI, these labels are often less cohesive than manual labels from experts (Porturas and Taylor, 2021). Therefore, the three authors collectively clustered the 22 initial topics based on the given words into 5 representative topics, following established approaches utilized in literature (Fox et al., 2021; Rahmadan et al., 2020). A complete list of each of the sub-topics assigned to one of the five manually labeled topics is available in Supplementary Table S2. After these steps, a total of 17,214 tweets were available for analysis.
3.4 Named entity recognition and disposition analysis
After collecting and filtering the data and identifying the thematic content of discussions surrounding the flood event (i.e., the tweets), the next step was to perform the comparison of the content and disposition (i.e., sentiment and emotion) of tweets from the public and organizational entities. To accomplish this, NER was employed to classify tweets into entities. Two entities were of primary interest: those originating from community members and those from organizations, including federal, state, and local agencies responsible for emergency services, natural disaster response, weather or environmental data, news outlets, and other channels. To carry out NER, we utilized the pre-trained bert-large-cased-finetuned-conll03-english model from the Hugging Face transformers library, optimized for recognizing grouped entities (Staatsbibliothek, 2020). We extracted entity information from usernames associated with tweets within each identified topic. The NER process was applied to categorize entities into four groups: organizations (ORG), persons (PER), locations (LOC), or miscellaneous (MISC). Tweets could be double counted in this process; for example, if a username included “California” and “Environmental Protection Agency,” the tweet would be assigned to both LOC and ORG. This step provided insight into how various entity types contributed to the online discourse surrounding the flooding events.
To assess the sentiment expressed in tweets, we employed a fine-tuned Bertweet-base-sentiment-analysis model from the Transformers library, specifically designed for sentiment analysis of social media content (Pérez, 2021). The model classified tweets into positive, negative, or neutral sentiments. The sentiment classifier accounts for emojis and emoticons (e.g., the smiley face emoticon “:)” is acknowledged as a positive sentiment). We applied this model to the text content of tweets, capturing both the sentiment label and the associated confidence score. This approach allowed us to quantify the sentiment of tweets linked to specific entities and topics. To explore how sentiment varied across entity types, we aggregated the NER and sentiment analysis results. We explored the dataset to associate each targeted entity type (e.g., organizations, individuals) with the corresponding tweet sentiment. This enabled a comparative analysis of how different entities expressed positive, negative, or neutral sentiments. The results were normalized to present the distribution of sentiments as percentages, highlighting distinct patterns in responses. To analyze emotional responses to the Weather topic, we used the cardiffnlp/twitter-roberta-base-emotion model (Cardiff NLP, 2020), which categorizes text into four emotions: anger, joy, optimism, and sadness. Compared to assessing sentiment polarity of positive to negative, this approach can provide a more nuanced understanding of public reactions in response to the flood events.
4 Results and discussion
Table 1 contains the five manually clustered topics. The Traffic topic encompassed discussions about road incidents and conditions. The Weather topic was the most directly related to the flood events themselves, including representative words such as “warning,” “thunderstorm,” and “severe.” The Operations topic contained tweets related to flights and helicopters. For context, the flood event in Nevada resulted in delayed or canceled flights (KNTV Las Vegas, 2022), while residents in Kentucky were rescued from flooded homes via helicopters (National Guard Bureau, 2022). The Society topic included tweets related to homes and gas, which were likely related to damaged or destroyed homes, or issues obtaining gasoline as a result of the floods (Associated Press, 2022b; Belleville News-Democrat, 2022; Jenkins and Bivens, 2022). The Power topic focused on outages resulting from the floods.
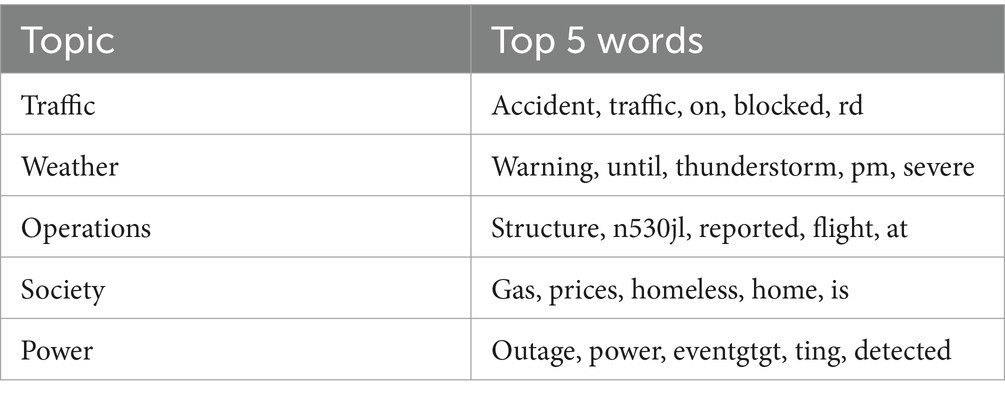
Table 1. Topic word scores for the five manually labeled topics produced from BERTopic modeling of all tweets containing at least one of the 70 keywords.
Importantly, none of the five topics, nor their sub-topics (Supplementary Table S2), were labeled with words indicative of directives for action or behaviors that community members should take. For example, the sub-topics did not prominently feature representative words related to containing information about evacuation, sheltering, resilience, or how to stay safe before, during, or after the flood. A 2018 study examining the content and dissemination of risk messages via Facebook and Twitter/X during a series of floods found that most of the analyzed posts from organizational channels contained information about situational awareness and recovery resources, but there was a lack of messages with actionable requests or behavioral resources (Scott and Errett, 2018). Our analysis is in agreement with these findings.
These 5 topics (“Traffic,” “Weather,” “Operations,” “Society,” and “Power”), were further analyzed for spatial and temporal trends for both (1) all data together and (2) a comparative analysis between the community and organizational entities (i.e., PER and ORG in the NER model described in the Methods section, respectively). For the Weather topic, tweet disposition over time for the PER and ORG entities was also analyzed. Figure 2 below shows the comparative analysis process and outputs produced, which we will describe in the coming sections.
4.1 Spatial density of posts
Figures 3a–c illustrate the geographical variation in the location and density of tweets regarding the analyzed flood events, where areas that are warmer toned (i.e., yellow hues) have a higher density of tweets than those that are cooler toned (i.e., blue hues). The nine states included in this study are outlined in black and are primarily clustered in the West, Midwest, and Southeast portions of the United States. Figure 3a shows tweets from all entities, Figure 3b shows tweets from the ORG entity, and Figure 3c shows tweets from the PER entity. Unsurprisingly, the locations with the highest density of tweets are primarily the urban areas that were most affected by the flooding, such as Los Angeles, California, and Las Vegas, Nevada, and this trend holds independent of entity type. There are fewer regions of high density in states that have more rural populations, such as Missouri, Mississippi, and Kentucky. Despite the relatively centralized locations of the flood events within each affected state, most states also had tweets originating from regions not directly impacted (Figure 3a). These posts, distributed widely across the state, suggest that severe flooding events can resonate, even on a small scale, beyond the immediately affected locations, garnering attention and concern from neighboring areas. This broader engagement may stem from ties or connections to the affected areas, or from genuine concern for fellow residents within or around the state.
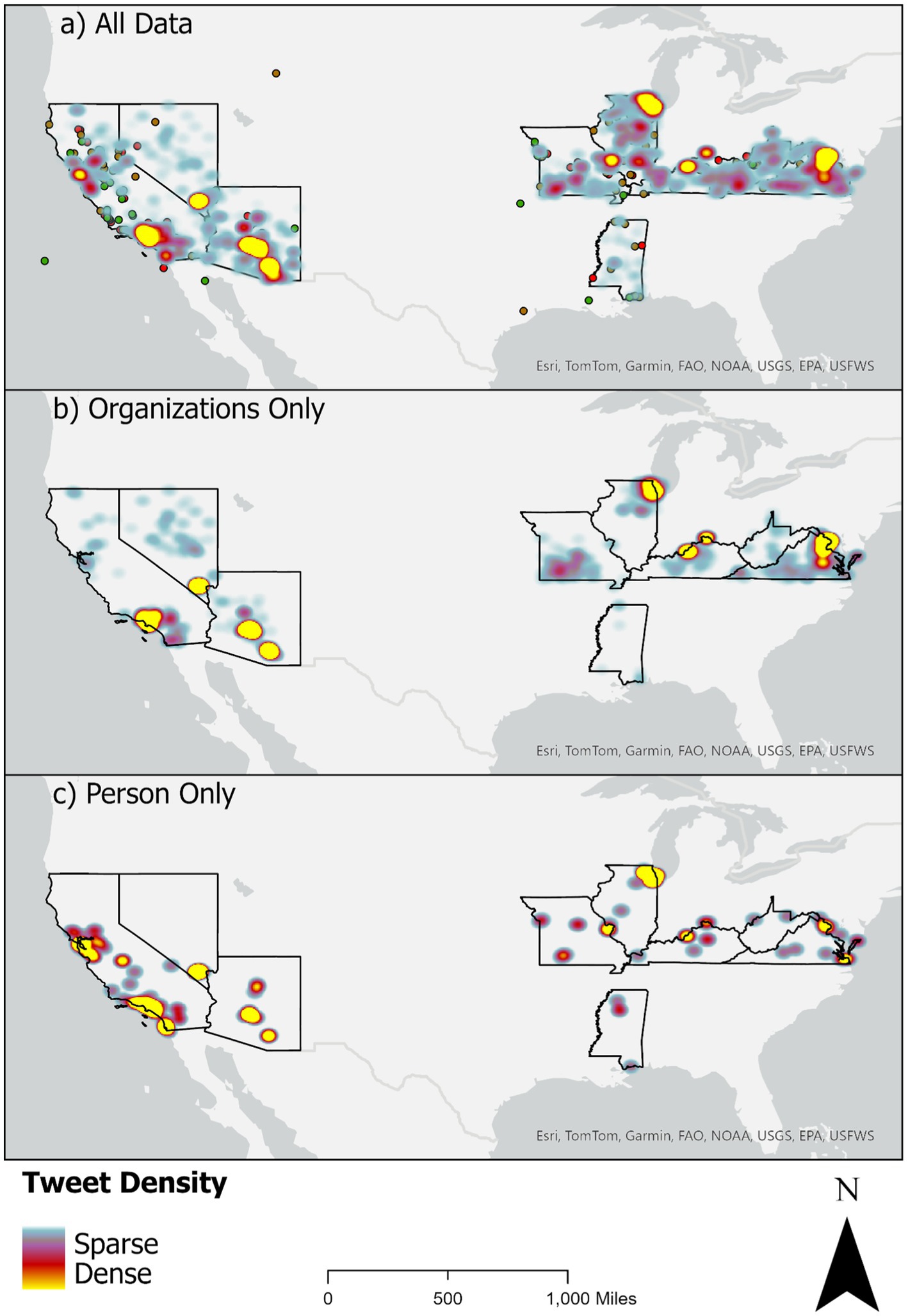
Figure 3. Heatmap of tweets, associated with “Traffic,” “Weather,” “Operations,” “Society,” and “Power,” distributed across the affected states during the July to August 2022 floods. (A) Tweets from the PER and ORG entities combined. (B) Tweets from the ORG entity only. (C) Tweets from the PER entity only. Warmer-toned areas (i.e., yellow hues) had a higher density of tweets. Cooler-toned areas (i.e., blue hues) had fewer tweets.
An examination of tweet density between the PER and ORG entities suggests that tweets from the ORG entity (Figure 3b) covered more areas outside of the directly impacted locales than the PER entity (Figure 3c). This suggests that there is likely interdepartmental communication from several local or state organizational entities across an impacted state, and organizations from different regional areas within a state may be providing updates to their local constituents. While tweets from organizational channels appear to be more widely distributed in general, there do not appear to be as many hotspots as there are for the PER entity. Tweets from the PER entity are much more clustered around the specific areas in each state that are directly affected by the flood.
4.2 Temporality of posts
To understand the temporal trends of tweet content over time, we first examined the frequency of tweeted topics over time across all entities. Then, we repeated the analysis by separating the topics by the PER and ORG entities to better understand similarities and differences in the content tweeted over time by each entity.
4.2.1 Topics/content pertaining to flood over time
Figure 4 illustrates the trends in how frequently each topic was tweeted over time for all entities. From this figure, it is clear that the Weather topic was most frequently tweeted about for almost the entire duration of all flood events, followed by Traffic, with Society, Power, and Operations closely tied. Weather being the dominant topic is not unexpected and indicates that weather-related information draws the most attention of Twitter/X users during this time frame. Additionally, of all the topics, Weather appears to be most aligned with the dates on which the flood events began. For example, local maxima of Weather tweets occurred on July 24 (the start date of floods in Missouri), July 27 (the start date of historic flooding in Kentucky), August 2 (one to two days after floods began in Arizona and California), and August 10th. The presence of local maxima supports findings from prior scholarship around social media as an early warning or communication network during disasters (de Bruijn et al., 2019; Shoyama et al., 2021). There is a noticeable decline in the frequency of tweets about Weather after August 9th. The last included flood event in this analysis has an end date of August 11th; this suggests that conversation around Weather was largely contained to the pre- and during-flood time periods, with less discussion in the post-flood phase.
Although tweets about Traffic were less frequent, there is good alignment between the local maxima and minima of the Weather topic with the Traffic topic, which suggests that the tweets labeled with Traffic, such as traffic accidents or road closures, are related to the floods. This is also true for the Society topic, although the peaks and troughs are slightly time-lagged. In contrast, we see a lasting increase in tweets related to Operations approximately halfway through our chosen time frame, suggesting that there may be a time delay between the start of flood events and the impact of Operations-related tweets. Overall, the presence of distinct patterns in discussed topics over time aligns with prior work using BERTopic modeling for tweets about floods in Germany, which found that it was possible to map trends in topic progression and shifts in the discussed topics over time (Veigel et al., 2024).
Figure 5 illustrates the differences in absolute count of tweets over time for the PER (dashed lines) and ORG (solid line) entities per topic. Overall, there were consistently more tweets over time from the PER entity for the Society and Weather topics, whereas the ORG entity tweeted more frequently for the Operations and Traffic topics. Both PER and ORG entities tweeted relatively similarly about Power over time, with a peak from PER occurring after August 14. Notably, the absolute number of tweets related to Power were less than any other topic; this was surprising, as we expected tweets about power outages or restoration services to be frequent in the post-flood periods. Because the number of tweets about Power are relatively low for both the PER and ORG entities, there does not appear to be a gap in communication by ORG about power. However, it is possible that discussion about Power is occurring more frequently on social media platforms other than Twitter/X.
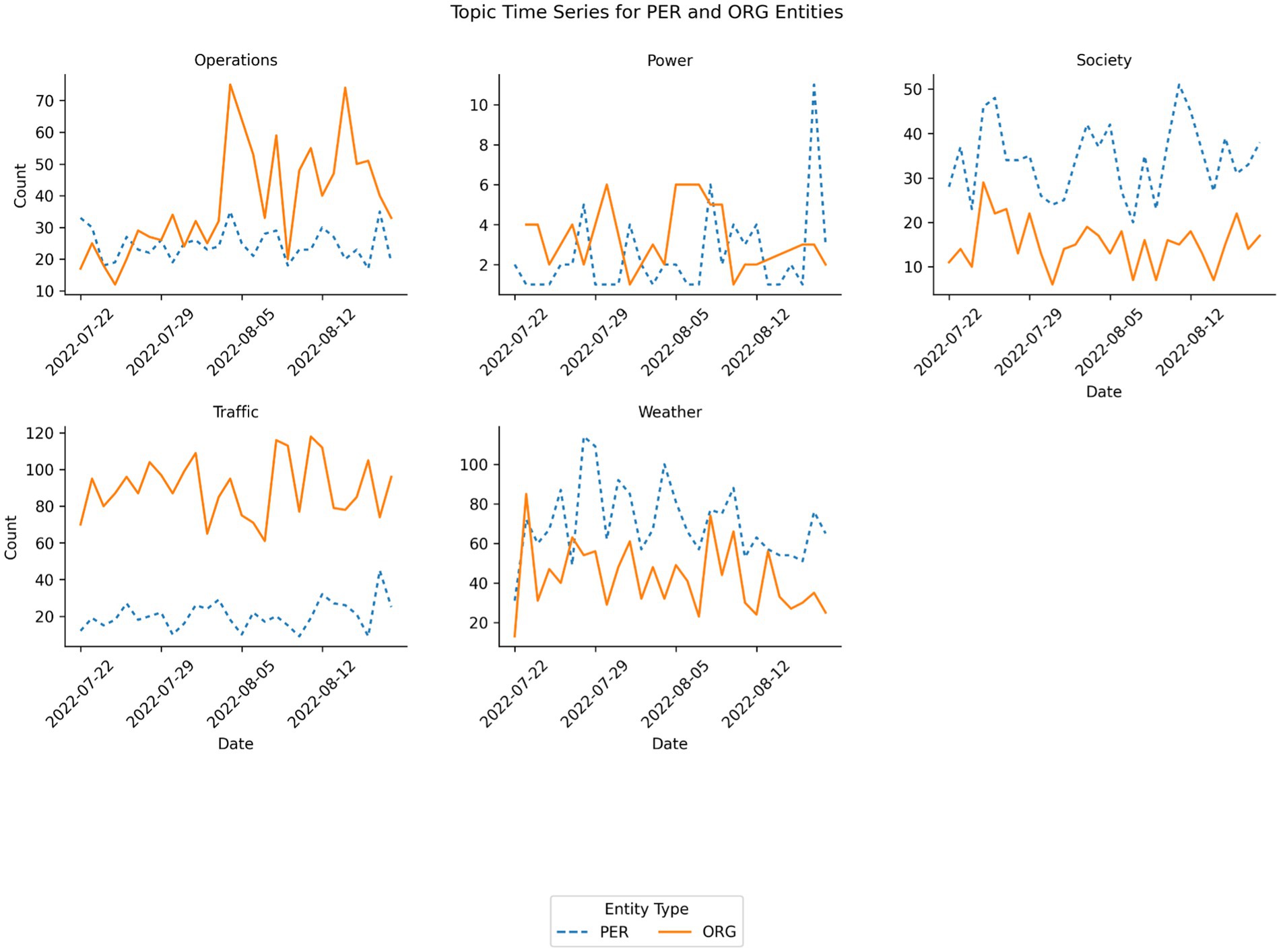
Figure 5. Temporal trends in tweet frequency per topic. Tweets from the PER entity are mapped by a blue dashed line. Tweets from the ORG entity are mapped by an orange solid line.
The relative abundance of tweets from the PER entity about the Society and Weather topics indicates that the public are focusing more on human-centered topics and immediate impact. For example, the emphasis on Weather reflects public concerns and discussion around the floods and storms. Furthermore, the Society topic contains tweets about survival and safety needs, such as gasoline prices and homelessness. Thus, the data suggest that the public are focused on the personal and societal impacts of the flood events. In contrast, tweets from the ORG entity are more frequent for the Operations and Traffic topics, which are more centered around logistics, such as mobility, transportation, and infrastructure. These topics indicate a focus on coordination efforts around the flood impacts. For instance, there was a significant increase in the number of tweets related to Operations by the ORG entity starting on August 1. The flash floods in Eastern Kentucky occurred from July 26 to August 11, which brought extreme flooding with up to 10.4 inches of rain in parts of the state (Finch, 2022), driving widespread road closures. The Operations topic includes tweets related to flights and helicopters; recovery efforts in Kentucky included rescue operations from flooded areas via helicopter (U.S. Department of Commerce et al., 2023). Additionally, the Traffic topic contained tweets related to traffic stops, traffic accidents, road closures, and injuries. The majority of tweets in Traffic originated from an organization, with a critical mass issuing from “Los Angeles Incident Tracker” and “LA Structure Fire Alerts.” Thus, the large number of tweets in Traffic are likely influenced by the inclusion of large urban areas, such as Los Angeles, in the tweet dataset.
The differences in tweet frequency between topics for the PER and ORG entities indicates a potential mismatch between risk messages from organizational channels and public concerns. For example, the limited focus on the Society topic by the ORG entity suggests a relative lack of communications about public needs or societal impact not related to infrastructural issues covered by the Operations topic. The gap between tweet frequency for the PER and ORG entities is less pronounced for the Weather topic as local maxima and minima follow similar trends between the two entities. This alignment highlights the potential of the Weather topic as a strong candidate for targeted content analysis to determine whether differences in tweet frequency are indicative of a communication gap.
4.2.2 Disposition pertaining to flood over time (Weather topic only)
Figure 6 contains time series results for the emotions of Weather tweets over time for the ORG entity (left panel) and PER entity (right panel).
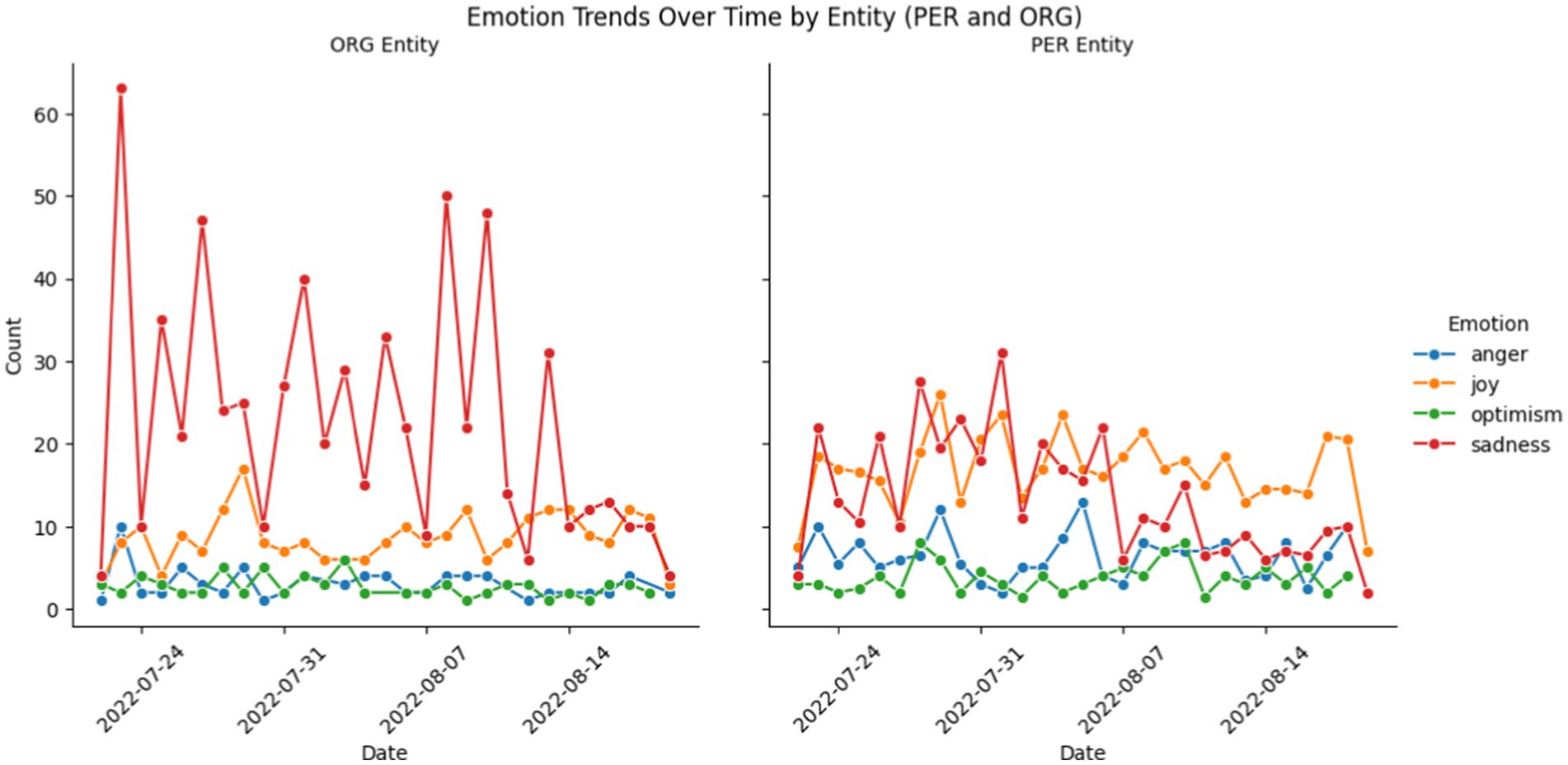
Figure 6. Temporal trends in emotion for the Weather topic for the ORG entity (left panel) and PER entity (right panel).
The left panel in Figure 6 contains the emotions over time in the Weather topic for the ORG entity. Notably, sadness is the primary and most frequently detected emotion, followed by joy, optimism, and anger. Sadness as the primary emotion suggests that messages from organizational channels may be acknowledging loss or the impact of flood events. While emotion classifications are limited by the constraints of the model, it is possible that some of the messages classified as sadness could be expressing sympathy, concern, or empathy. Empathy is an important concept in risk communication. A paper articulating five themes around studies of empathy defines them as an identification with another person, an understanding of the context or situation, a sharing of emotions with another person, an expression of concern for another person, and a belief that the information or situation is true or real (Campbell and Babrow, 2004). Studies suggest that empathy can improve the efficacy or persuasion of risk messages by bolstering credibility and fostering a positive relationship with the message receivers (Seeger and Schwarz, 2024). Therefore, some frequency of sadness is expected and likely useful in messages coming from the ORG entity during the flood events in this study. However, sadness as the primary emotion is somewhat surprising. An overemphasis of sadness could result in conveying hopelessness or amplifying public distress. As organizational entities, risk messages during disaster should ideally strike a balance between acknowledging the severity of the flood events without excessively conveying sadness, such that public panic or worry is minimized.
Emotions over time for the PER entity are in the right panel of Figure 6. In similarity to the ORG entity, the primary emotion of messages from the public is sadness, but joy is also expressed at a similar frequency. In fact, there is a sharp decline in the number of tweets classified with sadness on August 6, after which joy is the primary emotion. The majority of the flood events in this analysis had occurred and were in the post-disaster phase by August 6. The trends in emotions over time, therefore, suggest two key findings. First, a decrease of tweets labeled with the sadness emotion occurred as most of the flood events were moving into the post-disaster phase, which suggests that the number of tweets expressing sadness may be a useful barometer for the severity of the flood events, and may correlate with the success of post-disaster recovery efforts. A recent study (Kim, 2021) examining the relationship between emotions and perception of risk related to fine dust pollution found that feelings of sadness, anxiety, and anger were positively correlated with the perception of risk; in other words, study participants who expressed these feelings perceived the risk of fine dust pollution as higher than those who did not. Second, in this analysis, messages expressing joy actually remain relatively stable over time and do not increase, even as sadness decreases. Therefore, joy is not as effective of an indicator of public reaction to the floods in this study as are the more “negative” emotions, such as sadness. Overall, the identifiable trends in emotion from the public over time suggest that real-time or near-real-time analysis of public emotion may be useful for organizational entities to tailor their risk messages and address waves of emotion more effectively and proactively as a disaster progresses.
Figure 7 contains time series results for the sentiments of Weather tweets over time. The left panel contains results for the ORG entity and the right panel contains results for the PER entity. Overall, the neutral (NEU) sentiment is most prominent for both the PER and ORG entities. The prevalence of the neutral sentiment in tweets from organizational channels is not unexpected and suggests that the tweets generally maintain a neutral tone with little expression of judgment or opinion. On the other hand, there are more tweets from the public that have a negative (NEG) or positive (POS) sentiment than there are from organizational entities. The higher variation in sentiments in tweets from the public indicate a broader expression of judgment about the topics at hand. Interestingly, for the PER entity, the frequency of negative sentiments is greater than those that are positive in roughly the middle of the analyzed time frame, from around August 1 to August 9. The subsequent decline in negative sentiment occurs approximately around the same time as does the decline in frequency of tweets about the Weather topic, suggesting that the public are generally discussing the floods less in the period immediately after the end of the last flood event. This is not surprising, given that for the majority of the public included in this analysis, the flood events they experienced have either finished or are entering the post-flood phase. This suggests that sentiment is somewhat related to time, and tracking sentiment over time before, during, and after a flood event can be a useful data for tracking the impact of disasters and disaster recovery efforts (Neppalli et al., 2017).
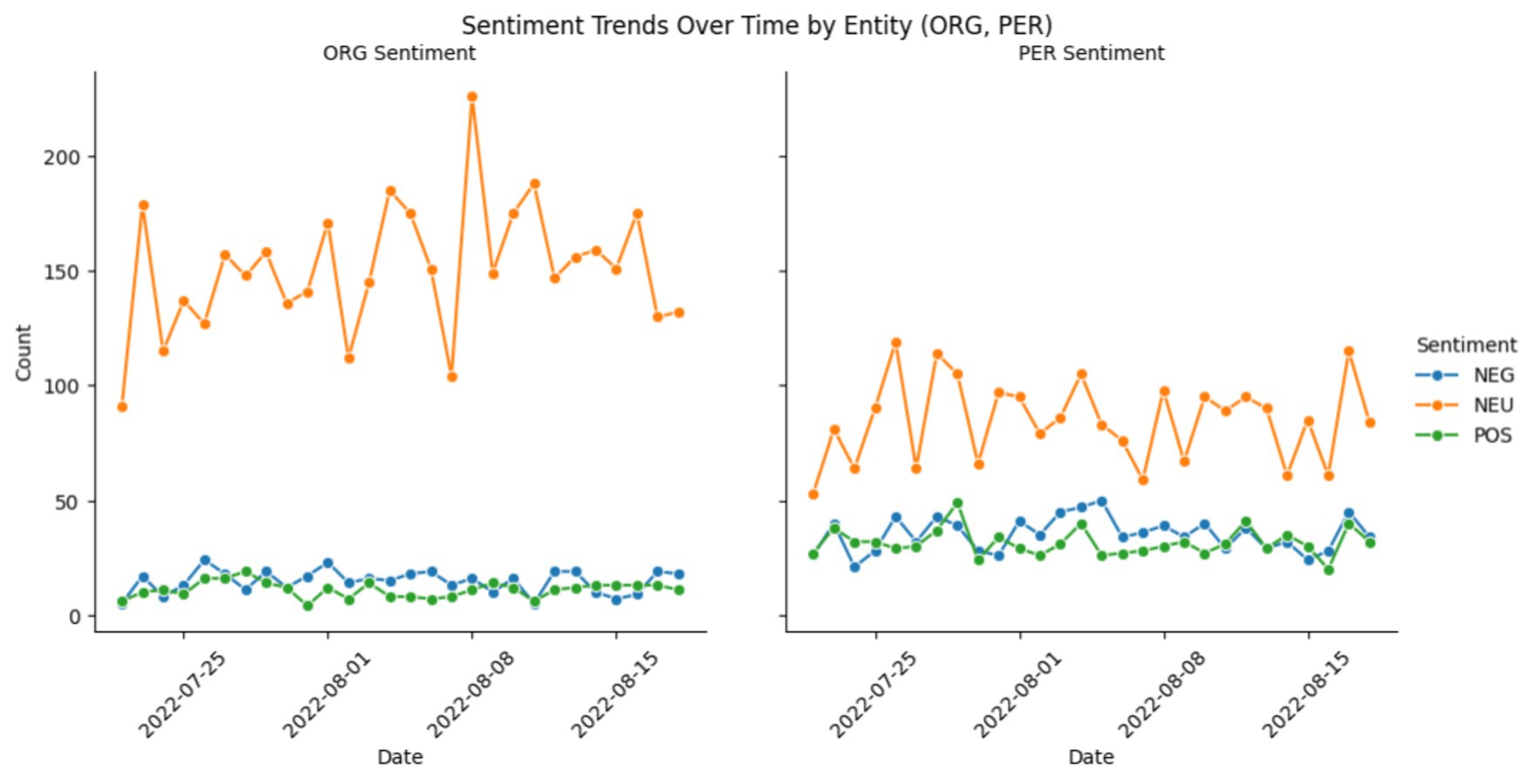
Figure 7. Temporal trends in sentiment for the Weather topic for the ORG entity (left panel) and PER entity (right panel).
4.3 Recommendations
Based on our comparative analysis and relevant literature, we propose a range of actionable steps for risk communicators to enhance information dissemination and community engagement during disaster events. These strategies, grounded in the demonstrated potential of social media and NLP from our study, can be customized to align with the unique goals and capabilities of a stakeholder. Table 2 presents our recommendations.
Our results reinforce the relevance of themes identified in previous studies on flood risk communication, while contributing to a more comprehensive understanding of the alignment of messaging between the public and organizational entities. For example, our recommendation to provide actionable content aligns with prior findings, such as a study examining perceived flood map usability and accuracy, which found that maps with a clear legend using general risk language were rated as most useful (Stephens et al., 2024). Furthermore, our results support existing calls in the literature for investigating baseline engagement metrics (Salley et al., 2024b), and exploring the role of emerging influential users (e.g., professionals, popular users, etc.) on social media during disasters (Li et al., 2022; Yang et al., 2019). Given the use of social media as early warning networks during disasters (Cools et al., 2016; Shoyama et al., 2021; Villagrán De León et al., 2013), risk communicators may also consider working with community members that have or are gaining a high visibility platform and influence during such events to aid in engagement efforts. Additionally, this second outlined recommendation aligns with standardized practices to better facilitate communication online, such as those outlined in Intrieri et al. (2020), who recommend standardizing the use of hashtags as part of an operational framework for flood risk communication.
The evidence of varied emotion and sentiment in messages from both entities suggest the need for careful consideration of tone during disaster (Visschers et al., 2012), which can aid balance in messaging. Neppalli et al. (2017) and Kim (2021) find that the sentiment and emotion, respectively, of risk communications can be important and useful indicators of public experiences and perceptions during a disaster. The temporal trends we found in our disposition analysis align with these prior works and motivate our recommendation to adapt messaging in real-time. Lastly, barriers to practical implementation should be considered, such as a shortage of time or resources available to engage in meaningful conversation (Demeritt and Nobert, 2014; Hinata et al., 2024; Lovari and Bowen, 2020) and the heterogeneous needs of the community (Haer et al., 2016; Martens et al., 2009).
When studying flood risk communication, there are different models that one can follow (Demeritt and Nobert, 2014). The recommendations we outline most closely align with following the risk instrument (RI) or risk dialogue (RD) models. The RI model prioritizes interactions between attitudes, behavior, and information, redefining successful communication away from just the transfer of information and instead toward changing the attitudes or behaviors of those receiving the communication. However, the RI model still implies a one-way exchange of information with a specific distinction between “senders” and “receivers.” The RD model moves away from this approach, instead emphasizing two-way exchanges of information. Tensions involved in the RD model include questions around whose voices should be included and what kind of dialogue should occur. Our recommendations, bolstered by the cited literature, provide suggestions that can move the needle toward more practical and feasible implementation of the RD flood risk communication model.
5 Conclusion
While our analysis using topic modeling, NER, and sentiment and emotion analysis provided valuable insights, limitations should be noted. The BERTopic model, despite its effectiveness, is highly sensitive to parameter settings. The unsupervised nature of topic modeling can result in topics that are overly broad or fragmented, with interpretations that may not always align with human intuition. Also, while pre-trained transformer models like those used in our analysis are powerful, they do not always generalize well to other contexts without additional fine-tuning, which could affect their applicability to different events or domains. Therefore, if used for other analyses, each flood event would require a tailored approach to ensure the analysis is both accurate and contextually relevant. For NER, the pre-trained model we used was developed for general text and can struggle with the informal, context-specific language commonly found on social media. This is why incorporating human interpretation, as we did in this study, is a valuable and necessary step to ensure contextual understanding. Additionally, it’s important to note that extracting entities from usernames rather than tweet content can sometimes lead to inaccuracies, as usernames may not always correspond to verified organizations, individuals, or places. Recognizing and addressing these nuances helps enhance the reliability and applicability of NER in social media analyses.
The study also reflected certain biases. The reliance on English-language tweets introduces a linguistic bias, limiting the generalizability of our findings to non-English-speaking communities. Furthermore, social media data itself is inherently biased toward more active user groups, which can disproportionately amplify certain voices or perspectives. As such, it should not be relied upon as the sole metric for representing community voices as it reflects only a subset of the population. While the data are still valuable, this limitation highlights the importance of complementing social media analyses with other data sources for a more comprehensive understanding. Researchers should diversify their sources of data to ensure its continued use in addressing environmental challenges (Ghermandi et al., 2023).
Future work should, therefore, analyze data from multiple social media platforms and aim to understand if, and how, the needs of different populations during floods can be understood from social media messages. Additionally, we did not include information about viewership, comments, likes, or retweets in our analysis. A next step in future work should include this information to draw conclusions about how frequently or effectively the intended audience is engaging with the risk messages. Our analysis was performed using Twitter/X data, and does not include any messages related to floods that may have been communicated on other social media platforms. Studies on population-specific use of social media platforms find that certain populations have preferences for which social media platforms they use; for example, a Pew Research study found that 70% of adults aged 50–64 years old reported using Facebook, whereas only 15% reported using Twitter/X (Pew Research Center, 2024). Therefore, future work should aim to replicate our approach across different or multiple social media platforms to enhance the applicability and scope of the findings. Lastly, future research should incorporate advanced models for fake news detection (Chauhan, 2024; Fu, 2022; Konet, 2024) to further enhance the reliability of messages collected and analyzed within such a system, benefiting both community and organizational messaging.
In conclusion, our comparative study of community and organizational social media posts contributes to the growing body of literature examining the relationship between social media, risk messages, and the sending/receiving entities. The comparative analysis completed in this study emphasizes the potential of social media platforms to enhance real-time information dissemination and community engagement and provides insight into temporal and spatial trends in messages from the public and organizational entities. Throughout the time frame for our analysis, hotspots of Twitter/X activity were centralized primarily around cities that were directly affected by the floods; however, a spatial density analysis revealed that tweets from organizational entities were occurring across affected states, suggesting interstate coordination or updates. Overall, Weather was the most frequently tweeted topic over time. Temporal trends in tweet frequency for Weather aligned well with the start of each flood event included in this analysis, and Traffic tweets demonstrated time-lagged alignment with peaks in tweets about Weather.
In general, there was more discussion about the Weather and Society topics from the public than from organizational entities; whereas the ORG entity tweeted more about Traffic and Operations. While this is not an unexpected result, further analysis should be performed to closely examine the content of the tweets within these topics to better understand if these differences are indicative of more risk communication gaps. For example, are the public not tweeting as much about Traffic because they are experiencing more immediate or pressing flood-related challenges related to Weather and Society? If so, then the frequency of tweets about Traffic from the ORG entity may be disproportionate and indicative of a communication misalignment. Temporal trends in sentiment and emotion provided insight around the dispositional responses by both entities to the flood events. Analyses for both entities indicate changes in emotions and sentiment over time, both of which align with a shift from active floods to the post-flood phase. Other literature suggests that trends in emotion and sentiment over time can be useful data for targeting recovery efforts, crafting more effective risk messages, and learning about public concerns (Neppalli et al., 2017). The results from our analyses support these findings. Further analysis will be performed to examine the emotion and sentiment of tweets from both entities over time separated either by flood severity or by state. In this way, the dispositional analysis can be better understood in the context of different population densities, state politics, agency mobilization efforts, and flood impacts.
Lastly, we presented five targeted recommendations to foster more actionable, two-way risk communication. In doing so, we effectively addressed our two-part research question: critically comparing how social media messages from community members and organizational entities differ in content and disposition during a flood event, and exploring how these insights can inform strategies to foster more effective risk communication. Overall, the findings from this study highlight the need for a paradigm shift toward more collaborative and responsive flood communication strategies, designed to better address community needs and strengthen the interactions between organizational entities and the public during flood events.
Data availability statement
The data analyzed in this study is subject to the following licenses/restrictions: The data supporting the conclusions of this article can be made available by the authors, upon reasonable request. Requests to access these datasets should be directed to Dr. Nathan Fox, Zm94bmF0QHVtaWNoLmVkdQ==.
Ethics statement
Ethical approval was not required for the study involving human data in accordance with the local legislation and institutional requirements. Written informed consent was not required, for either participation in the study or for the publication of potentially/indirectly identifying information, in accordance with the local legislation and institutional requirements. The social media data was accessed and analyzed in accordance with the platform's terms of use and all relevant institutional/national regulations.
Author contributions
CS: Conceptualization, Investigation, Methodology, Project administration, Resources, Visualization, Writing – original draft, Writing – review & editing, Data curation, Formal analysis, Software, Validation. NF: Conceptualization, Data curation, Formal analysis, Investigation, Methodology, Resources, Validation, Visualization, Writing – original draft, Writing – review & editing, Software. AS: Conceptualization, Formal analysis, Investigation, Methodology, Project administration, Resources, Writing – original draft, Writing – review & editing, Validation.
Funding
The author(s) declare that financial support was received for the research and/or publication of this article. This research received support through Schmidt Sciences, LLC. Any opinions, findings, and conclusions or recommendations expressed in this material are those of the authors.
Acknowledgments
We would like to extend a special thank you to the Network Dynamics Lab at the Georgia Institute of Technology, for providing their archived Twitter/X postings for us to utilize in this research. Additionally, we would like to thank Matthew Seeger and David Herman for their thoughtful comments during the ideation phase of this work.
Conflict of interest
The authors declare that the research was conducted in the absence of any commercial or financial relationships that could be construed as a potential conflict of interest.
Generative AI statement
ChatGPT 4 was used to assist with minor editing of the manuscript only (e.g., sentence restructuring). Napkin AI was used to create Figures 1, 2.
Publisher’s note
All claims expressed in this article are solely those of the authors and do not necessarily represent those of their affiliated organizations, or those of the publisher, the editors and the reviewers. Any product that may be evaluated in this article, or claim that may be made by its manufacturer, is not guaranteed or endorsed by the publisher.
Supplementary material
The Supplementary material for this article can be found online at: https://www.frontiersin.org/articles/10.3389/fcomm.2025.1553746/full#supplementary-material
References
AFSPA. (2022). State of emergency issued in Nevada due to flooding. AFSPA: State of Emergency Notification. Available online at: https://www.afspa.org/soeitems/state-of-emergency-issued-in-nevada-due-to-flooding/.
Aïmeur, E., Amri, S., and Brassard, G. (2023). Fake news, disinformation and misinformation in social media: a review. Soc. Netw. Anal. Min. 13:30. doi: 10.1007/s13278-023-01028-5
Allaire, M. C. (2016). Disaster loss and social media: can online information increase flood resilience? Water Resour. Res. 52, 7408–7423. doi: 10.1002/2016WR019243
Associated Press. (2022a). Death toll from Kentucky flood reaches 40, latest victim died during cleanup effort. Fox News. Available online at: https://www.foxnews.com/us/death-toll-kentucky-flood-reaches-40-latest-victim-died-cleanup-effort.
Associated Press. (2022b). Heavy rain causes flash flooding in Central Mississippi. U.S. News. Available online at: https://www.usnews.com/news/best-states/mississippi/articles/2022-08-02/heavy-rain-causes-flash-flooding-in-central-mississippi.
Belleville News-Democrat. (2022). How much damage did flooding cause in southwest IL? Here’s a town-by-town assessment so far. Belleville News-Democrat. Available online at: https://www.bnd.com/news/local/article263875972.html.
Burger, J., and Gochfeld, M. (2014). Health concerns and perceptions of central and coastal New Jersey residents in the 100 days following Superstorm Sandy. Sci. Total Environ. 481, 611–618. doi: 10.1016/j.scitotenv.2014.02.048
Campbell, R. G., and Babrow, A. S. (2004). The role of empathy in responses to persuasive risk communication: overcoming resistance to HIV prevention messages. Health Commun. 16, 159–182. doi: 10.1207/S15327027HC1602_2
Campello, R. J., Moulavi, D., Zimek, A., and Sander, J. (2015). Hierarchical density estimates for data clustering, visualization, and outlier detection. ACM Trans. Knowl. Discov. Data 10, 1–51. doi: 10.1145/2733381
Cardiff NLP. (2020). Cardiffnlp/twitter-roberta-base-emotion. Hugging Face. Available online at: https://huggingface.co/cardiffnlp/twitter-roberta-base-emotion.
Chauhan, P. (2024). Pulk17/fake-news-detection. Hugging Face. Available online at: https://huggingface.co/Pulk17/Fake-News-Detection.
Chiu, Y. C., and Zheng, H. (2007). Real-time mobilization decisions for multi-priority emergency response resources and evacuation groups: model formulation and solution. Trans. Res. E Logis. Trans. Rev. 43, 710–736. doi: 10.1016/j.tre.2006.11.006
Choirul Rahmadan, M., Nizar Hidayanto, A., Swadani Ekasari, D., and Purwandari, B.Theresiawati (2020). “Sentiment analysis and topic modelling using the LDA method related to the flood disaster in Jakarta on twitter” in Proceedings - 2nd international conference on informatics, multimedia, cyber, and information system, ICIMCIS 2020, 126–130.
Cools, J., Innocenti, D., and O’Brien, S. (2016). Lessons from flood early warning systems. Environ. Sci. Pol. 58, 117–122. doi: 10.1016/j.envsci.2016.01.006
Cooper, C. M., Sharma, S., Nicholas, R. E., and Keller, K. (2022). Toward more actionable flood-risk information. Earth’s Future 10:e2022EF003093. doi: 10.1029/2022EF003093
Dalmia, A., and Sia, S. (2021). Clustering with UMAP: why and how connectivity matters. arXiv:2108.05525. doi: 10.48550/arXiv.2108.05525
de Bruijn, J. A., de Moel, H., Jongman, B., de Ruiter, M. C., Wagemaker, J., and Aerts, J. C. J. H. (2019). A global database of historic and real-time flood events based on social media. Sci. Data 6:311. doi: 10.1038/s41597-019-0326-9
Demeritt, D., and Nobert, S. (2014). Models of best practice in flood risk communication and management. Environ. Haz. 13, 313–328. doi: 10.1080/17477891.2014.924897
Devlin, J., Chang, M. W., Lee, K., and Toutanova, K. (2019). “Bert: Pre-training of deep bidirectional transformers for language understanding,” in Proceedings of the 2019 conference of the North American chapter of the association for computational linguistics: human language technologies, volume 1 (long and short papers). 4171–4186.
Fan, C., Farahmend, H., and Mostafavi, A. (2020). Rethinking infrastructure resilience assessment with human sentiment reactions on social media in disasters.
Feldman, D., Contreras, S., Karlin, B., Basolo, V., Matthew, R., Sanders, B., et al. (2016). Communicating flood risk: looking back and forward at traditional and social media outlets. Int. J. Disast. Risk Reduct. 15, 43–51. doi: 10.1016/j.ijdrr.2015.12.004
FEMA. (2021). Climate change increases risk of flooding. FEMA. Available online at: https://www.floodsmart.gov/climate-change#:~:text=The%20risk%20of%20flooding%20is,and%20rising%20risk%20of%20flooding.
FEMA. (2022a). Disasters and other declarations. FEMA. Available online at: https://www.fema.gov/disaster/declarations?field_dv2_declaration_date_value%5Bmin%5D=2024&field_dv2_declaration_date_value%5Bmax%5D=2024&field_dv2_declaration_type_value=All&field_dv2_incident_type_target_id_selective=All.
FEMA. (2022b). Kentucky severe storms, flooding, landslides, and mudslides. FEMA. Available online at: https://www.fema.gov/disaster/4663.
FEMA. (2024). Disaster declarations for states and counties. FEMA. Available online at: https://www.fema.gov/data-visualization/disaster-declarations-states-and-counties.
Finch, A. (2022). Death toll rises following catastrophic Kentucky flooding. AccuWeather. Available online at: https://www.accuweather.com/en/severe-weather/death-toll-rises-following-catastrophic-kentucky-flooding/1223842.
Flanagin, A. J., and Metzger, M. J. (2000). Perceptions of internet information credibility. J. Mass Commun. Q. 77, 515–540. doi: 10.1177/107769900007700304
Forsyth, W., Roberts, T., and Brewer, G. (2023). Conceptualising risk communication barriers to household flood preparedness. Urban Gover. 3, 116–129. doi: 10.1016/j.ugj.2023.02.001
Fox, N., Graham, L. J., Eigenbrod, F., Bullock, J. M., and Parks, K. E. (2021). Enriching social media data allows a more robust representation of cultural ecosystem services. Ecosyst. Serv. 50:101328. doi: 10.1016/j.ecoser.2021.101328
Fu, J. (2022). jy46604790/fake-news-Bert-detect. Hugging Face. Available online at: https://huggingface.co/jy46604790/Fake-News-Bert-Detect/commits/main/.gitattributes.
Ghermandi, A., Langemeyer, J., Van Berkel, D., Calcagni, F., Depietri, Y., Vigl, L. E., et al. (2023). Social media data for environmental sustainability: a critical review of opportunities, threats, and ethical use. One Earth 6, 236–250. doi: 10.1016/j.oneear.2023.02.008
Gilbert, M. (2022). Las Vegas records month’s worth of rain in less than 2 hours as storms drench southwest. AccuWeather. Available online at: https://www.accuweather.com/en/severe-weather/las-vegas-records-months-worth-of-rain-in-less-than-2-hours-as-storms-drench-southwest/1224627#google_vignette.
Graham, M. W., Avery, E. J., and Park, S. (2015). The role of social media in local government crisis communications. Public Relat. Rev. 41, 386–394. doi: 10.1016/j.pubrev.2015.02.001
Haer, T., Botzen, W. J. W., and Aerts, J. C. J. H. (2016). The effectiveness of flood risk communication strategies and the influence of social networks-insights from an agent-based model. Environ Sci Policy 60, 44–52. doi: 10.1016/j.envsci.2016.03.006
Hinata, S., Rohde, H., and Templeton, A. (2024). Communicating with the public in emergencies: a systematic review of communication approaches in emergency response. Int. J. Disast. Risk Reduct. 111:104719. doi: 10.1016/j.ijdrr.2024.104719
Ho, S. S., Chuah, A. S., Ho, V. S., Rosenthal, S., Kim, H. K., and Soh, S. S. (2024). Crisis and emergency risk communication and emotional appeals in COVID-19 public health messaging: quantitative content analysis. J. Med. Internet Res. 26:e56854. doi: 10.2196/56854
Houston, J. B., Hawthorne, J., Perreault, M. F., Park, E. H., Goldstein Hode, M., Halliwell, M. R., et al. (2015). Social media and disasters: a functional framework for social media use in disaster planning, response, and research. Disasters 39, 1–22. doi: 10.1111/disa.12092
Hu, Y., Boyd-Graber, J., Satinoff, B., and Smith, A. (2014). Interactive topic modeling. Mach. Learn. 95, 423–469. doi: 10.1007/s10994-013-5413-0
Intrieri, E., Dotta, G., Fontanelli, K., Bianchini, C., Bardi, F., Campatelli, F., et al. (2020). Operational framework for flood risk communication. Int. J. Disast. Risk Reduct. 46:101510. doi: 10.1016/j.ijdrr.2020.101510
Jenkins, N., and Bivens, E. (2022). UPDATE: Residents around Panbowl dam in Jackson evacuated. ABC 36 News. Available online at: https://www.wtvq.com/state-officials-recommend-evacuation-of-panbowl-lake-floodplain-in-jackson/.
Kanth, A. K., Chitra, P., and Sowmya, G. G. (2022). Deep learning-based assessment of flood severity using social media streams. Stoch. Env. Res. Risk A. 36, 473–493. doi: 10.1007/s00477-021-02161-3
Kim, S. (2021). The role of discrete emotions in risk perception and policy support during public health crises: the moderation effect of SNS dependency. Int. J. Environ. Res. Public Health 18:11654. doi: 10.3390/ijerph182111654
KNTV Las Vegas. (2022). Monsoon weather continues throughout Las Vegas. KNTV Las Vegas. Available online at: https://www.ktnv.com/news/monsoon-weather-continues-throughout-las-vegas.
Konet, A. (2024). Amandakonet/climatebert-fact-checking. Hugging Face. Available online at: https://huggingface.co/amandakonet/climatebert-fact-checking.
Li, W., Haunert, J. H., Knechtel, J., Zhu, J., Zhu, Q., and Dehbi, Y. (2023). Social media insights on public perception and sentiment during and after disasters: the European floods in 2021 as a case study. Trans. GIS 27, 1766–1793. doi: 10.1111/tgis.13097
Li, J., Sun, A., Han, J., and Li, C. (2020). A survey on deep learning for named entity recognition. IEEE Trans. Knowl. Data Eng. 34, 50–70. doi: 10.1109/TKDE.2020.2981314
Li, L., Xie, R., Dong, R., and Wen, H. (2022). Characterizing the role of influencers in disseminating situational information after an earthquake. Int. J. Disast. Risk Reduct. 83:103416. doi: 10.1016/j.ijdrr.2022.103416
Lovari, A., and Bowen, S. A. (2020). Social media in disaster communication: a case study of strategies, barriers, and ethical implications. J. Public Aff. 20:e1967. doi: 10.1002/pa.1967
Lovari, A., and Parisi, L. (2015). Listening to digital publics. Investigating citizens’ voices and engagement within Italian municipalities’ Facebook pages. Public Relat. Rev. 41, 205–213. doi: 10.1016/j.pubrev.2014.11.013
Lowe, D., Ebi, K. L., and Forsberg, B. (2013). Factors increasing vulnerability to health effects before, during and after floods. Int. J. Environ. Res. Public Health 10, 7015–7067. doi: 10.3390/ijerph10127015
Maidl, E., and Buchecker, M. (2015). Raising risk preparedness by flood risk communication. Nat. Hazards Earth Syst. Sci. 15, 1577–1595. doi: 10.5194/nhess-15-1577-2015
Martens, T., Garrelts, H., Grunenberg, H., and Lange, H. (2009). Taking the heterogeneity of citizens into account: flood risk communication in coastal cities–a case study of Bremen. Nat. Hazards Earth Syst. Sci. 9, 1931–1940. doi: 10.5194/nhess-9-1931-2009
Mendoza, M., Poblete, B., and Castillo, C. (2010). “Twitter under crisis: can we trust what we RT?” in Proceedings of the first workshop on social media analytics, 71–79.
Mihunov, V. V., Jafari, N. H., Wang, K., Lam, N. S. N., and Govender, D. (2022). Disaster impacts surveillance from social media with topic modeling and feature extraction: case of hurricane Harvey. Int. J. Disaster Risk Sci. 13, 729–742. doi: 10.1007/s13753-022-00442-1
Mostafiz, R. B., Rohli, R. V., Friedland, C. J., and Lee, Y. C. (2022). Actionable information in flood risk communications and the potential for new web-based tools for long-term planning for individuals and community. Front. Earth Sci. 10:840250. doi: 10.3389/feart.2022.840250
Mulder, A. C., Pijnacker, R., De Man, H., Van De Kassteele, J., Van Pelt, W., Mughini-Gras, L., et al. (2019). “Sickenin’ in the rain” - increased risk of gastrointestinal and respiratory infections after urban pluvial flooding in a population-based cross-sectional study in the Netherlands. BMC Infect. Dis. 19:377. doi: 10.1186/s12879-019-3984-5
National Guard Bureau. (2022). National Guard Assists with flood Rescue in Kentucky. National Guard. Available online at: https://www.nationalguard.mil/News/Article-View/Article/3109036/national-guard-assists-with-flood-rescue-in-kentucky/#:~:text=The%20Tennessee%20National%20Guard%20sent,in%20search%20and%20rescue%20efforts.&text=An%20aerial%20view%20of%20flash,Hawk%20helicopter%20July%2028%2C202022.
National Weather Service. (2018). Flood related hazards. NWS, NOAA. Available online at: https://www.weather.gov/safety/flood-hazards.
National Weather Service. (2022). July 26th, 2022 historic flash flooding in the St. Louis Metro Area. NWS, NOAA. Available online at: https://www.weather.gov/lsx/July262022Flooding.
Neppalli, V. K., Caragea, C., Squicciarini, A., Tapia, A., and Stehle, S. (2017). Sentiment analysis during hurricane Sandy in emergency response. Int. J. Disast. Risk Reduct. 21, 213–222. doi: 10.1016/j.ijdrr.2016.12.011
NOAA National Severe Storms Laboratory. (2024). Severe weather 101. NOAA National Severe Storms Laboratory. Available online at: https://www.nssl.noaa.gov/education/svrwx101/floods/.
O’Sullivan, J. J., Bradford, R. A., Bonaiuto, M., De Dominicis, S., Rotko, P., Aaltonen, J., et al. (2012). Enhancing flood resilience through improved risk communications. Nat. Haz. Earth Syst. Sci. 12, 2271–2282. doi: 10.5194/nhess-12-2271-2012
Pérez, J. M. (2021). Finiteautomata/bertweet-base-sentiment-analysis. Hugging Face. Available online at: https://huggingface.co/finiteautomata/bertweet-base-sentiment-analysis/tree/main.
Pew Research Center. (2024). Social media fact sheet. Pew Research Center. Available online at: https://www.pewresearch.org/internet/fact-sheet/social-media/.
Porturas, T., and Taylor, R. A. (2021). Forty years of emergency medicine research: uncovering research themes and trends through topic modeling. Am. J. Emerg. Med. 45, 213–220. doi: 10.1016/j.ajem.2020.08.036
Pourebrahim, N., Sultana, S., Edwards, J., Gochanour, A., and Mohanty, S. (2019). Understanding communication dynamics on twitter during natural disasters: a case study of hurricane Sandy. Int. J. Disast. Risk Reduct. 37:101176. doi: 10.1016/j.ijdrr.2019.101176
Ragini, J. R., Anand, P. R., and Bhaskar, V. (2018). Big data analytics for disaster response and recovery through sentiment analysis. Int. J. Inf. Manag. 42, 13–24. doi: 10.1016/j.ijinfomgt.2018.05.004
Rahmadan, M. C., Hidayanto, A. N., Ekasari, D. S., and Purwandari, B. (2020). “Sentiment analysis and topic modelling using the lda method related to the flood disaster in Jakarta on twitter” in 2020 international conference on informatics, multimedia, cyber and information system (ICIMCIS) (IEEE), 126–130.
Reuter, C., Hughes, A. L., and Kaufhold, M. A. (2018). Social media in crisis management: an evaluation and analysis of crisis informatics research. Int. J. Hum. Comput. Interact. 34, 280–294. doi: 10.1080/10447318.2018.1427832
Rollason, E., Bracken, L. J., Hardy, R. J., and Large, A. R. (2018). Rethinking flood risk communication. Nat. Hazards 92, 1665–1686. doi: 10.1007/s11069-018-3273-4
Salley, C., Fox, N., and Schubert, A. (2024a). Tweeting through the flood: application of BERT topic modeling for a comparative flood communication analysis. In Proceedings of the International ISCRAM Conference, 21.
Salley, C., Mohammadi, N., Xie, J., Tien, I., and Taylor, J. E. (2024b). Assessing community needs in disasters: transfer learning for fusing limited georeferenced data from crowdsourced applications on the community level. J. Manag. Eng. 40:04024055. doi: 10.1061/JMENEA.MEENG-6208
Sampson, N. R., Price, C. E., Kassem, J., Doan, J., and Hussein, J. (2019). “We’re just sitting ducks”: recurrent household flooding as an underreported environmental health threat in Detroit’s changing climate. Int. J. Environ. Res. Public Health 16:6. doi: 10.3390/ijerph16010006
Scott, K. K., and Errett, N. A. (2018). Content, accessibility, and dissemination of disaster information via social media during the 2016 Louisiana floods. J. Public Health Manag. Pract. 24, 370–379. doi: 10.1097/PHH.0000000000000708
Seeger, M. W., and Schwarz, A. (2024). “Best practices in crisis and disaster communication” in Routledge Handbook of Risk, Crisis, and Disaster Communication, 65–82.
Shoyama, K., Cui, Q., Hanashima, M., Sano, H., and Usuda, Y. (2021). Emergency flood detection using multiple information sources: integrated analysis of natural hazard monitoring and social media data. Sci. Total Environ. 767:144371. doi: 10.1016/j.scitotenv.2020.144371
Staatsbibliothek, B. (2020). Dbmdz/bert-large-cased-finetuned-conll03-english. Hugging Face. Available online at: https://huggingface.co/dbmdz/bert-large-cased-finetuned-conll03-english.
Starbird, K., Palen, L., Hughes, A. L., and Vieweg, S. (2010). Chatter on the red: what hazards threat reveals about the social life of microblogged information. In Proceedings of the 2010 ACM conference on Computer supported cooperative work (pp. 241–250).
Stephens, K. K., Blessing, R., Tasuji, T., McGlone, M. S., Stearns, L. N., Lee, Y., et al. (2024). Investigating ways to better communicate flood risk: the tight coupling of perceived flood map usability and accuracy. Environ. Haz. 23, 92–111. doi: 10.1080/17477891.2023.2224956
Tabari, H. (2020). Climate change impact on flood and extreme precipitation increases with water availability. Sci. Rep. 10:13768. doi: 10.1038/s41598-020-70816-2
Turner, M. M. (2010). “Emotion in persuasion and risk communication,” in The Routledge Handbook of Emotions and Mass Media (New York: Routledge), 251–272.
U.S. Department of Commerce, National Oceanic and Atmospheric Administration, and National Weather Service, Central Region Headquarters. (2023). July 2022 Significant River/Flash Flood in Southeastern Kentucky. Available online at: https://www.weather.gov/media/publications/assessments/July_2022_Significant_River_Flash_Flood_SE_KY.pdf.
Van Ootegem, L., and Verhofstadt, E. (2016). Well-being, life satisfaction and capabilities of flood disaster victims. Environ. Impact Assess. Rev. 57, 134–138. doi: 10.1016/j.eiar.2015.12.001
Veigel, N., Kreibich, H., de Bruijn, J. A., Aerts, J. C., and Cominola, A. (2024). Content analysis of multi-annual time series of flood-related twitter (X) data. EGUsphere 2024, 1–19.
Villagrán de León, J. C., Pruessner, I., and Breedlove, H. (2013). Alert and warning frameworks in the context of early warning systems. Available at: https://digitallibrary.un.org/record/4072023?v=pdf
Visschers, V. H., Wiedemann, P. M., Gutscher, H., Kurzenhäuser, S., Seidl, R., Jardine, C. G., et al. (2012). Affect-inducing risk communication: current knowledge and future directions. J. Risk Res. 15, 257–271. doi: 10.1080/13669877.2011.634521
Vo, B.-K. H., and Collier, N. (2013). Twitter emotion analysis in earthquake situations. Int. J. Comput. Linguist. Appl. 4, 159–173.
Waite, T., Murray, V., and Baker, D. (2014). Carbon monoxide poisoning and flooding: changes in risk before, during and after flooding require appropriate public health interventions. PLoS Curr. 6. doi: 10.1371/currents.dis.2b2eb9e15f9b982784938803584487f1
Wang, Z., Chen, J., Chen, J., and Chen, H. (2023). Identifying interdisciplinary topics and their evolution based on BERTopic. Scientometrics, 1–26.
Wang, R. Q., Hu, Y., Zhou, Z., and Yang, K. (2020). Tracking flooding phase transitions and establishing a passive hotline with AI-enabled social media data. IEEE Access 8, 103395–103404. doi: 10.1109/ACCESS.2020.2994187
Wang, Q., and Taylor, J. E. (2016). Process map for urban-human mobility and civil infrastructure data collection using geosocial networking platforms. J. Comput. Civ. Eng. 30:04015004. doi: 10.1061/(ASCE)CP.1943-5487.0000469
Waters, R. D., and Williams, J. M. (2011). Squawking, tweeting, cooing, and hooting: analyzing the communication patterns of government agencies on twitter. J. Public Aff. 11, 353–363. doi: 10.1002/pa.385
Wolshon, P. B., Urbina, E. A., and Levitan, M. L. (2001). National review of hurricane evacuation plans and policies. Baton Rouge, LA: Louisiana State University Hurricane Center.
Wukich, C. (2016). Government social media messages across disaster phases. J. Contingen. Crisis Manag. 24, 230–243. doi: 10.1111/1468-5973.12119
Yang, Y., Zhang, C., Fan, C., Yao, W., Huang, R., and Mostafavi, A. (2019). Exploring the emergence of influential users on social media during natural disasters. Int. J. Disast. Risk Reduct. 38:101204. doi: 10.1016/j.ijdrr.2019.101204
Zhou, S., Kan, P., Huang, Q., and Silbernagel, J. (2023). A guided latent Dirichlet allocation approach to investigate real-time latent topics of twitter data during hurricane Laura. J. Inf. Sci. 49, 465–479. doi: 10.1177/01655515211007724
Keywords: flooding, natural language processing (NLP), risk communication, social media, Twitter/X
Citation: Salley C, Fox N and Schubert A (2025) Bridging the gap in flood risk communication: a comparative study of community and organizational social media posts using natural language processing. Front. Commun. 10:1553746. doi: 10.3389/fcomm.2025.1553746
Edited by:
Rob Grace, University of Cincinnati, United StatesReviewed by:
Salvatore Micciche’, University of Palermo, ItalyEnzo Loner, University of Trento, Italy
Copyright © 2025 Salley, Fox and Schubert. This is an open-access article distributed under the terms of the Creative Commons Attribution License (CC BY). The use, distribution or reproduction in other forums is permitted, provided the original author(s) and the copyright owner(s) are credited and that the original publication in this journal is cited, in accordance with accepted academic practice. No use, distribution or reproduction is permitted which does not comply with these terms.
*Correspondence: Christin Salley, Y2pzYWxsZXlAdW1pY2guZWR1
†These authors have contributed equally to this work