- 1Dipartimento di Scienze della Terra, Università di Torino, Turin, Italy
- 2Centro Interdipartimentale sui Rischi Naturali in Ambiente Montano e Collinare, Università di Torino, Turin, Italy
- 3Istituto Nazionale di Geofisica e Vulcanologia, Osservatorio Vesuviano, Naples, Italy
- 4Dipartimento Scienze della Terra, Università degli Studi di Firenze, Florence, Italy
- 5Engineering Ingegneria Informatica S.p.A., Rome, Italy
- 6Icelandic Meteorological Office, Reykjavík, Iceland
- 7Servicio Nacional de Geología y Minería, Santiago, Chile
- 8Instituto GeofÍsico del Perú, Lima, Peru
- 9Vanuatu Meteorology and Geohazards Department, Port Vila, Vanuatu
- 10Instituto Nacional de Sismologia, Vulcanologia, Meteorologia e Hidrologia, Guatemala City, Guatemala
- 11U.S. Geological Survey, Volcano Disaster Assistance Program, Reston, VA, United States
- 12Servicio Geológico Colombiano, Bogota, Colombia
- 13Facultad de Geología, Geofísica y Minas, Universidad Nacional de San Agustin de Arequipa, Arequipa, Peru
- 14Goma Volcano Observatory, Goma, Democratic Republic of Congo
- 15Center for Volcanology and Geological Hazard Mitigation, Bandung, Indonesia
- 16Instituto Geofísico, Escuela Politécnica Nacional, Quito, Ecuador
- 17Instituto Geologico Minero y Metalurgico, Lima, Peru
- 18Université de Paris, Institut de Physique du Globe de Paris, CNRS, Paris, France
- 19Observatoire Volcanologique du Piton de la Fournaise, Institut de Physique du Globe de Paris, La Plaine des Cafres, France
- 20Department of Mineral Policy and Geohazards Management, Port Moresby, Papua New Guinea
- 21Royal Netherlands Meteorological Institute, De Bilt, Netherlands
- 22Colima Intercambio e Investigación en Vulcanología, Universidad de Colima, Colima, Mexico
Volcanic activity is always accompanied by the transfer of heat from the Earth’s crust to the atmosphere. This heat can be measured from space and its measurement is a very useful tool for detecting volcanic activity on a global scale. MIROVA (Middle Infrared Observation of Volcanic Activity) is an automatic volcano hot spot detection system, based on the analysis of MODIS data (Moderate Resolution Imaging Spectroradiometer). The system is able to detect, locate and quantify thermal anomalies in near real-time, by providing, on a dedicated website (www.mirovaweb.it), infrared images and thermal flux time-series on over 200 volcanoes worldwide. Thanks to its simple interface and intuitive representation of the data, MIROVA is currently used by several volcano observatories for daily monitoring activities and reporting. In this paper, we present the architecture of the system and we provide a state of the art on satellite thermal data usage for operational volcano monitoring and research. In particular, we describe the contribution that the thermal data have provided in order to detect volcanic unrest, to forecast eruptions and to depict trends and patterns during eruptive crisis. The current limits and requirements to improve the quality of the data, their distribution and interpretation are also discussed, in the light of the experience gained in recent years within the volcanological community. The results presented clearly demonstrate how the open access of satellite thermal data and the sharing of derived products allow a better understanding of ongoing volcanic phenomena, and therefore constitute an essential requirement for the assessment of volcanic hazards.
Introduction
Volcano monitoring consists of continuous, real-time, acquisition and processing of numerous data that describe the behavior of a volcano (e.g., earthquakes, ground movement, gas emissions, remote-sensed data) in order to detect any sign of change that may precede an eruption (Sparks et al., 2012; Pallister and McNutt, 2015; Newhall et al., 2017). It likely represents the only scientifically valid approach for short-term forecasts of a future eruption, or possible changes during an ongoing eruption (Tilling, 2008).
Volcanic monitoring can be viewed as a form of continuous learning by the scientific community, in which the greater the amount of information available, the greater the understanding of the volcanic phenomena in progress, and the better the ability to forecast future eruptive scenarios (Winson et al., 2014). In this context, satellite data are a reliable source of information, especially for monitoring the large number (∼50%) of potentially active volcanoes (∼1400) still lacking conventional ground-based instruments (Brown et al., 2015; Pritchard et al., 2018; Delgado et al., 2019). Nonetheless, even at well-monitored volcanoes, satellite data offer a spatio-temporal view of eruptive phenomena that may fill gaps left by ground based instruments (Ebmeier et al., 2018).
The acquisition of satellite images and data for volcanological applications is continuously and rapidly growing (Ramsey and Harris, 2013; Furtney et al., 2018; Pritchard et al., unpublished), so that big data analysis techniques (i.e., artificial intelligence and machine learning) are progressively used for research purposes and for monitoring activity (Piscini and Lombardo, 2014; Anantrasirichai et al., 2018; Valade et al., 2019). In particular, with the advent of the new millenium, and with the development of internet, the dissemination and sharing of satellite data/products can be considered a pillar of open science in volcanology, also thanks to the growing availability of open data by space agencies (Delgado et al., 2019).
At present, four main types of Remote Sensing Observations (RSO) provide different insights into the key processes occurring within a volcanic system. These are: (i) ground deformation, from microwaves; (ii) SO2 degassing, from ultraviolet and infrared; (iii) ash emission, from infrared; and (iv) heat flux, from infrared.
Recent scientific pilot projects, as the European Volcano Observatory Space Services, EVOSS (Tait and Ferrucci, 2013) and the Committee on Earth Observation Satellite (CEOS) Volcano Pilot Project (Delgado et al., 2019), demonstrated the potential of integrating these space-based data for forecasting eruptions (Furtney et al., 2018), stressing the need to develop a volcanic monitoring system to support volcano observatories (Pritchard et al., unpublished). The Monitoring Unrest From Space (MOUNTS) project1, although in an embryonic stage, can be considered a first prototype of such integrated system, since it includes near-real time multi-parametric analysis (UV, IR and microwaves) derived from the ESA Sentinel constellation, at several volcanoes (Valade et al., 2019). However, apart from this example, a comprehensive integration of space-based datasets into an operational system for global volcano monitoring is at this time only envisioned, with several distinct groups working on a single RSO (deformation, degassing, ash, thermal; Reath et al., 2019a).
During the past two decades, numerous volcanic hot-spot detection systems have been developed in order to detect, localize and quantify the presence of thermal anomalies produced by volcanic activity (Harris, 2013; Ramsey and Harris, 2013). In Supplementary Table S1, we have compiled a list of all the systems currently operating in real time of which we are aware, although other systems may be operated locally. These systems are based on different sensors having distinct spatial, temporal and radiometric resolution so that each of them is different from the others in terms of performance and usability for research and volcano monitoring (Steffke and Harris, 2011). The scientific results achieved thanks to the open data elaborated by these systems are remarkable and in continuous growth (Harris, 2013; Ramsey and Harris, 2013). However, their use from an operational point of view, that is, during the daily monitoring or during an eruptive crisis, remains little known and poorly described. In particular, what information is most useful to the observatories and how this information should be communicated/interpreted in (near) real time remains a topic of broad interest, especially for the future development of these systems and their integration with other satellite- or ground-based monitoring networks (Pritchard et al., unpublished).
In this work, we describe the architecture of the system named Middle Infrared Observations of Volcanic Activity (MIROVA) and its use by more than 17 volcano observatories/Institutions in charge of monitoring more than 650 volcanoes around the globe (Supplementary Table S2). Based on the experiences of the individual observatories (summarized in the Supplementary Appendix), we then deduced what is the contribution of the satellite thermal data to the daily monitoring of volcanoes in extremely different geological and socio-economic contexts. The use of the results provided by MIROVA makes it possible to understand the current limits of thermal remote sensing systems and the requirements to further develop an open science tool to support the assessment and mitigation of volcanic risk at global scale.
The Mirova System
Architecture of the System
Middle Infrared Observations of Volcanic Activity is an automatic system for detecting thermal anomalies at high temperatures (>500K), based on the analysis of MODIS (Moderate Resolution Imaging Spectroradiometer) data. MODIS is a sensor mounted on board two NASA satellites, called Terra and Aqua, in sun-synchronous polar orbit since March 2000 and May 2002, respectively. The main features of MODIS, useful for volcano thermal monitoring, consist of its global coverage with spatial resolution of 1 km, temporal resolution of about four images/day (at the equator) and the presence of a dual channel in the mid-infrared (centered at 3.959 microm) with low/high gain settings (providing an extended range of unsaturated data). The general architecture of the MIROVA system can be divided into two main parts: (a) data download and processing (developed on a local computer) and (b) data dissemination (developed on a website: www.mirovaweb.it).
Unlike other systems that use MODIS data (e.g., MODVOLC; FIRMS; Supplementary Table S1) MIROVA is not strictly a global monitoring system. In fact, although the algorithm can be applied in any environmental context (Coppola et al., 2016a), the data processing chain actually operates only for a list of selected target volcanoes for which the near real time observation is requested. This list of selected targets is compiled from the list of the Holocene volcanoes (more than 1400) of the Global Volcanism Program (2013) by setting an “operational flag” to all the volcanoes that need to be monitored. Volcanoes are also given an identification number in accordance with the Global Volcanism Program. The number of monitored volcanoes has gradually increased since the start of the operational phase (in 2014) and has now reached a number equal to 216 units. These targets were gradually added to the MIROVA list based on a scientific interest, or following specific requests from the observatories. They now cover the most active volcanoes on the globe (Global Volcanism Program, 2013) with the current number actually limited by the hardware resources of the system and by the amount of data to be downloaded and processed daily (about 25 Gb per day). Despite this limitation, this architecture makes it possible to add other specific targets in case of need (i.e., unrest of a new volcano) without compromising the total system efficiency in terms of processing time, memory, storage capacity, etc. Access to archived MODIS data (available since 2000 and 2002 for Terra and Aqua, respectively) also allows analysis and reconstruction of 20-year time series for any volcano. However, building a complete thermal database for all the volcanoes currently monitored by MIROVA takes time and is in the development phase.
Download, Data Processing, and Sensibility
The original MODIS data (Level 1b – calibrated radiances) are downloaded from the LANCE system, which provides them with a latency of less than 3 h2. The continuous screening of the LANCE daily remote folders (every 5 min), allows timely downloading in the local computer of any newly acquired MODIS granule imaging at least one target volcano. The downloaded granules are then processed following several steps fully described in Coppola et al. (2016a).
For each analyzed volcano, the original spectral radiance data (recorded by MODIS in the Middle Infrared [MIR] at 3.959 μm and Thermal Infrared [TIR] at 12.02 μm) are resampled in regular grids of 50 × 50 km (in UTM coordinates) and processed in order to identify the pixels containing thermal anomalies. This step, or rather the hotspot detection algorithm, comprehends the application of spectral and spatial principles that, combined together, increase the ability to automatically detect hotspots. In particular, the MIROVA algorithm uses the middle infrared MIR bands at 3.959 μm and thermal TIR at 12.02 μm to calculate different spectral indices (such as the Normalized Thermal Index – NTI, Wright et al., 2004, and the Enhanced Thermal Index – ETI, Coppola et al., 2016a) that enhance the presence of hot objects inside the pixels. In addition, a series of spatial operations allow us to highlight the pixels having these indices in excess with respect to their surroundings, thus constituting a hybrid and contextual approach for any environmental condition.
Once the contaminated pixels have been identified, the volcanic radiative power (VRP) is calculated using the MIR-method (Wooster et al., 2003). Specifically, the VRP is calculated as:
where npix is the number of alerted pixels, LMIR,alert is the pixel integrated MIR radiance of the ith alerted pixel, LMIR,bk is the MIR radiance of the background (average radiance of pixels surrounding the anomaly), Apixel is the pixel size (1 km2 for the resampled MODIS pixels), and 18.9 is a constant of proportionality (see Wooster et al., 2003).
The VRP is the main parameter calculated by the MIROVA system and represents the combined effect of the hotspot area (Ahot) and its integrated temperature (Thot) according to Stephan Boltzmann’s law (VRP = σεAhotThot4; being σ and ε the Boltzmann constant and emissivity, respectively).
It is important to underline that the application of the MIR method on hotspots of volcanic origin represents the thermal flux radiated exclusively from the surfaces with T > 500 K (only the portion of the warmer surface produces sufficient MIR radiances to be detected by MODIS) and returns the VRP with an error of ± 30%.
As a whole MIROVA may detect thermal anomalies with VRP spanning from less than ∼1 MW to about ∼50 GW. According to the Stephan-Boltzmann’s law, the lower detection limit (1 MW) would correspond to two end-member cases: (i) a hot case characterized by a vent of ∼7 m2 and a temperature of 1000°C, or (ii) a cold case characterized by a fumarole field having an area of ∼143 m2 and a temperature of 300°C.
Website and Data Dissemination
In order to easily and quickly share the real-time observations of the system, a dedicated website3 summarizes, and continuously updates, a series of tables and graphical outputs for any monitored volcano.
The home page (Figure 1) of this website is constituted by a world map showing all the monitored volcanoes, and by a table summarizing the latest thermal anomalies detected by the system (in chronological order). In order to quickly identify the “thermal magnitude” of the hotspot listed in this table, we represent each detection through a color scale, proportional to the logarithm of the detected VRP. This scale is divided into five distinct levels and each thermal detection is classified into these subdivisions, based on the recorded VRP (Figure 1).
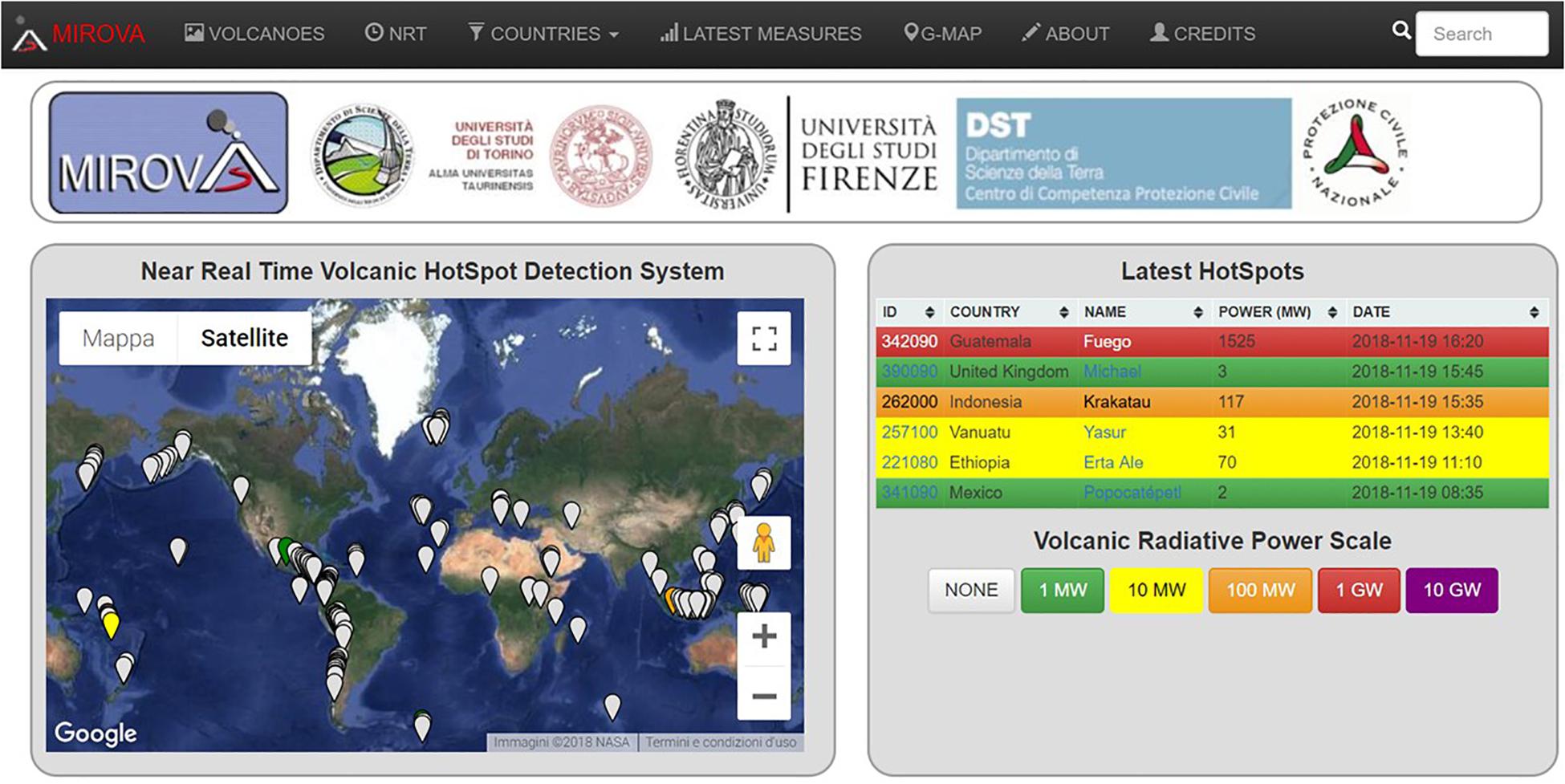
Figure 1. Snapshot of the home page of the MIROVA website (www.mirovaweb.it; accessed on 19 November 2018).
The interactive map and table take the user to the page dedicated to each individual volcano, where specific graphical outputs are updated approximately four times per day (according to the number of MODIS overpasses) and available online from 1 to 4 h after image acquisition. These outputs consist of the “Latest IR Images,” “VRP time series,” “Distance from the summit,” and a “Google Earth overlap” which are described in more detail below.
Latest IR Images
The latest IR Images screen summarizes the latest 10 MODIS acquisitions over the target volcano (Figure 2). This display provides an intuitive and fast overview of the thermal state of the volcano during the past 48 h (assuming four images per day). Each image displays a grayscale map according to the NTI relative to each pixel. When a hotspot is detected, the VRP (in MW) is reported below each image, and the frame colored according to the VRP Scale. The satellite zenith and azimuth are also displayed at the bottom of each image in order to permit a quick evaluation of the viewing geometry conditions. This information allows the user, who knows the topography of the volcano, to assess whether the observation conditions are favorable or unfavorable to the detection of a hotspot. For example, if a lava dome emerges at the bottom of a deep crater, the observation conditions are quite restricted, since in this case (high zenith angle over a deep crater) the crater’s rim could block the line of sight of the sensor and, therefore, the sensor would not detect the hot dome. Similarly, a lava flow descending on a steep flank of a volcano will be poorly imaged by a satellite looking from the opposite side and with a high zenith angle. In this case the VRP could be underestimated and would not correspond to a real lowering of the thermal activity.
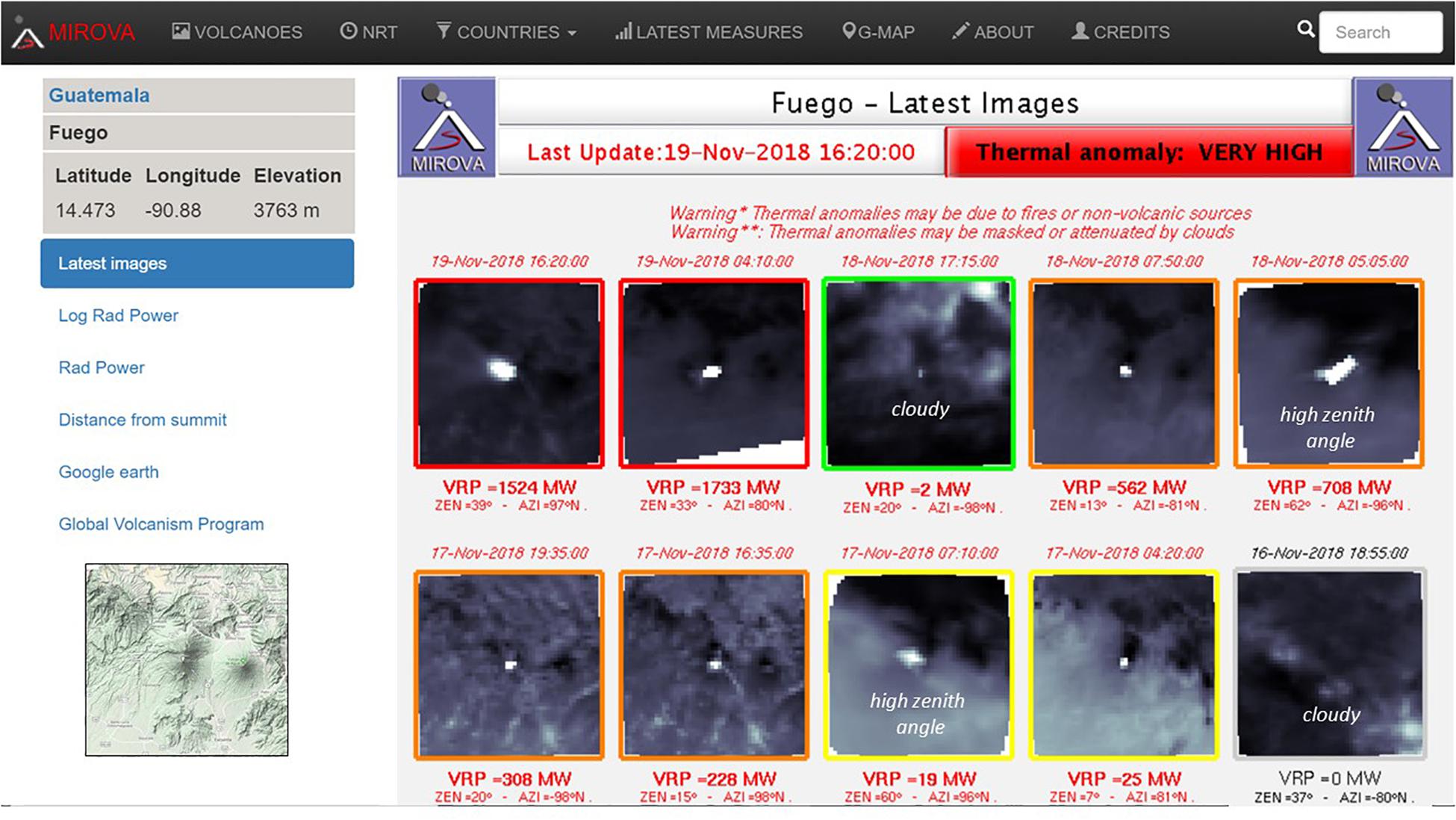
Figure 2. Snapshot of the Latest IR Images display posted on MIROVA website for the Fuego volcano, Guatemala (accessed on 19 November 2018).
The visualization of the last 10 NTI maps allows also a first-order evaluation of the cloud fraction, essential for a correct interpretation of the data (e.g., presence/absence of hotspots, intensity and location of the thermal anomaly). In a general way, during the course of an eruption user can refer to the latest images (Figure 2) to follow the detection of thermal activity level and evaluate the quality of each VRP related to the weather conditions and the geometry of the satellite acquisition. It is however important to emphasize that the VRP and the color code provided by MIROVA are not corrected automatically for the acquisition conditions (i.e., clouds/geometry) but they simply represent a measurement of the thermal radiation reaching the sensor.
VRP Time Series
The VRP time series can be displayed in both Log and Linear scale, which summarizes the detected VRP values within the previous month and year of the MODIS observation (upper and lower panel, respectively in Figure 3). Each stem represents a single detection (one MODIS passage) with a distinction between proximal (hotspots located within 5 km from the summit are represented by blue stems) and distal anomalies (far hotspots located at more than 5 km from the summit, are represented by black stems).
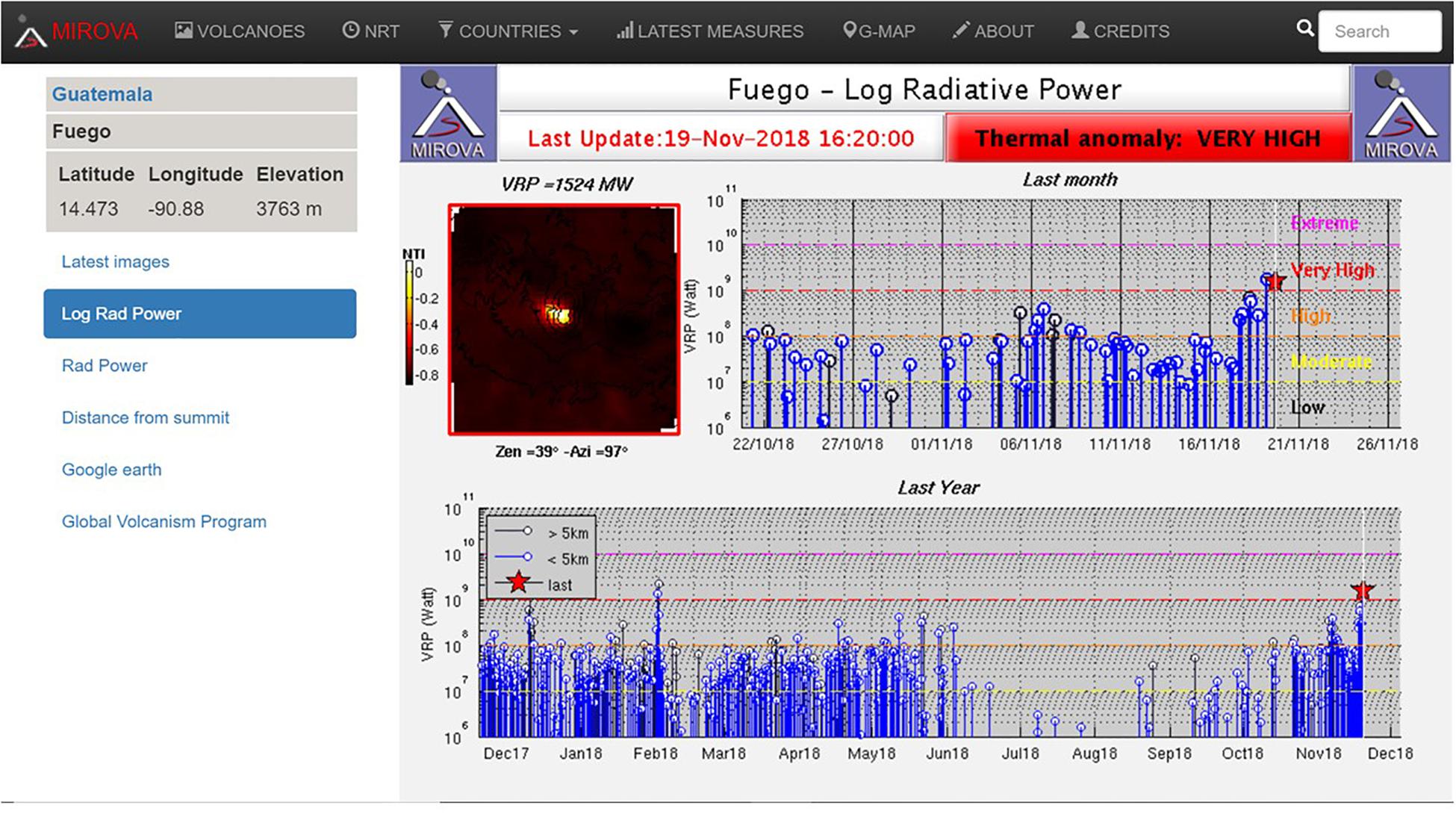
Figure 3. Snapshot of the “Log Radiative Power” screen posted on the MIROVA website for the Fuego volcano, Guatemala (accessed on 19 November 2018). Note the fluctuations in thermal activity in the last month (top right panel), with an evident increase in VRP (almost 2 orders of magnitude) recorded from 14 to 19 November 2018. The trend of thermal anomalies recorded during the past year (lower panel) shows that after the eruption of the June 3, 2018 the thermal activity of Fuego was very low for at least 3 months. However, since September 2018 the radiate heat flux gradually intensified by means of repeated pulses culminating with the high VRP recorded on November 19, 2018.
The time series are displayed in a logarithmic scale (Figure 3) to allow the visualization of the large variations in the thermal intensity that may accompany a single eruption (up to five orders of magnitude). Also, the logarithmic scale permits to recognize subtle long-term trends and patterns such as the exponential decays (appearing linear in the log scale plot) that often accompany the waning phase of an eruption (Wadge, 1981). The extrapolation of these trends offers a rapid method to qualitatively detect a change from the expected values, possibly associated with a variation of the behavior of the ongoing volcanic activity (see section “Eruptive Evolution, Trends and Patterns”). The same VRP time series are also shown with a linear scale (“Rad Power” button on the left menu; Figure 3) in order to better visualize real intensity changes in thermal activity.
Distance From the Summit
This screen named “Distance from summit” shows the distance from the farthest hot pixel to the summit of the volcano, during the last month and last year of activity (upper and lower panel, respectively in Figure 4). This graph has the dual purpose of (i) identifying gradual displacements of the thermal anomaly, for example during the advancement of a lava front, or (ii) detecting sudden changes in the hot spot location, likely associated with the occurrence of forest fires or false alerts (in distal areas of the volcano).
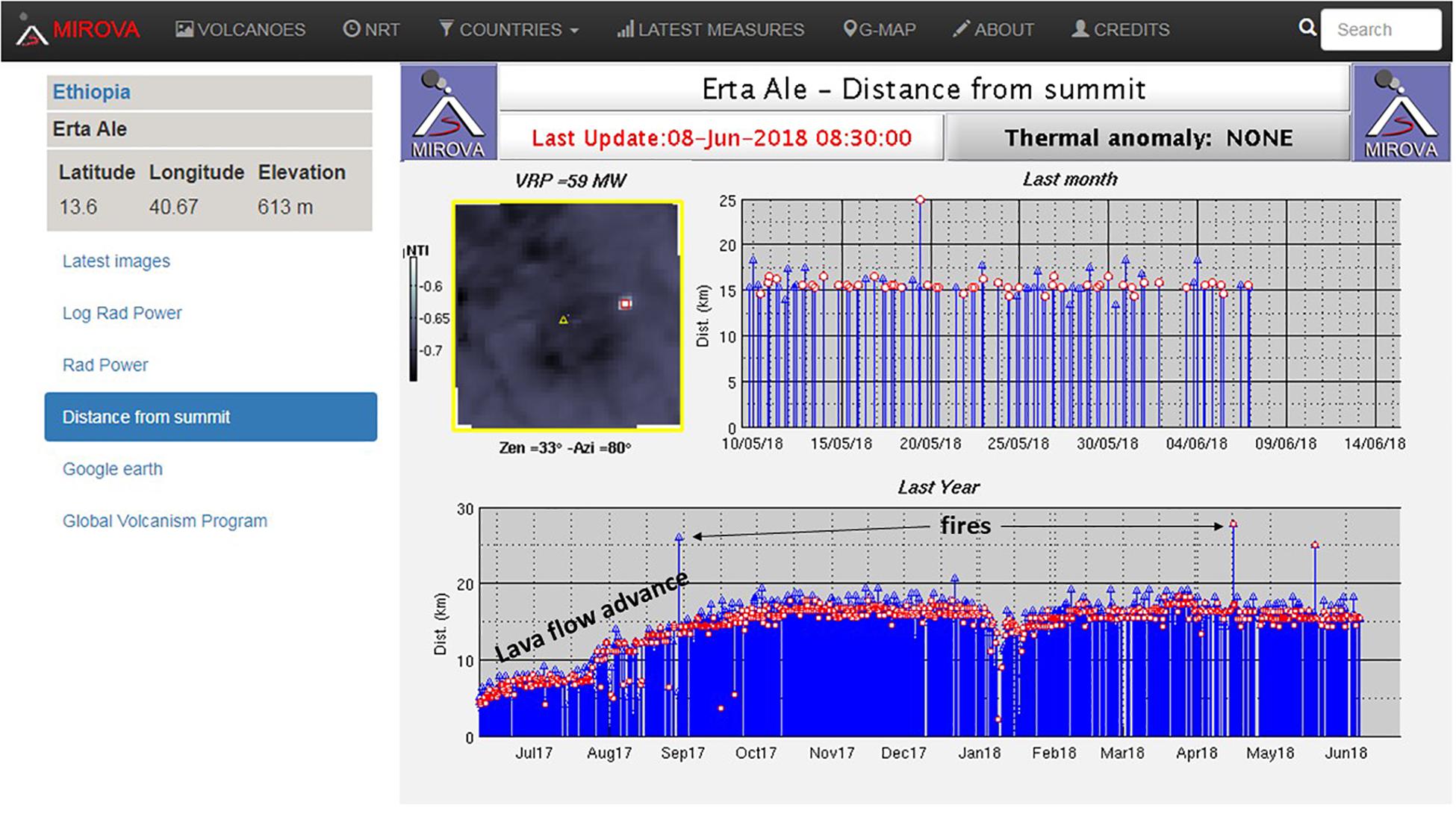
Figure 4. Snapshot of the “Distance from summit” figure posted on MIROVA website for the Erta Ale volcano (accessed on 08 June 2018). Note the lengthening phase of the lava flow occurred between June and October 2017 with an average flow advancement velocity of 100 m day–1.
Although the location accuracy remains significant (± 1 km), in the case of km-long lava flows, this automatic analysis provides a useful estimate of lava front advancement. As an example, in Figure 4 we show the case of the eruption of Erta Ale (Figure 4) during which the flow front advanced by 12 ± 1 km in 4 months (June–September 2017), with an average speed of 100 m day–1. Isolated spikes showing thermal anomalies more than 25 km away from the summit (Figure 4), can be easily identified and associated with possible fires.
Google Earth Overlap
The Google Earth overlap screen shows the last processed image superimposed on google map which allows to locate the thermal anomaly and estimate its dimensions (Figure 5). In many cases, the overlap of the thermal images on google map can be used to verify if the hotspot is located inside or outside a summit crater, for hazard evaluation of the ongoing activity. The estimation of hotspot location is also very useful to verify if the thermal anomaly is possibly associated to a forest fire (e.g., located over a vegetated area), anthropogenic source (e.g., located over an urban/industrial area), or it is a false alert (e.g., located above a body of water).
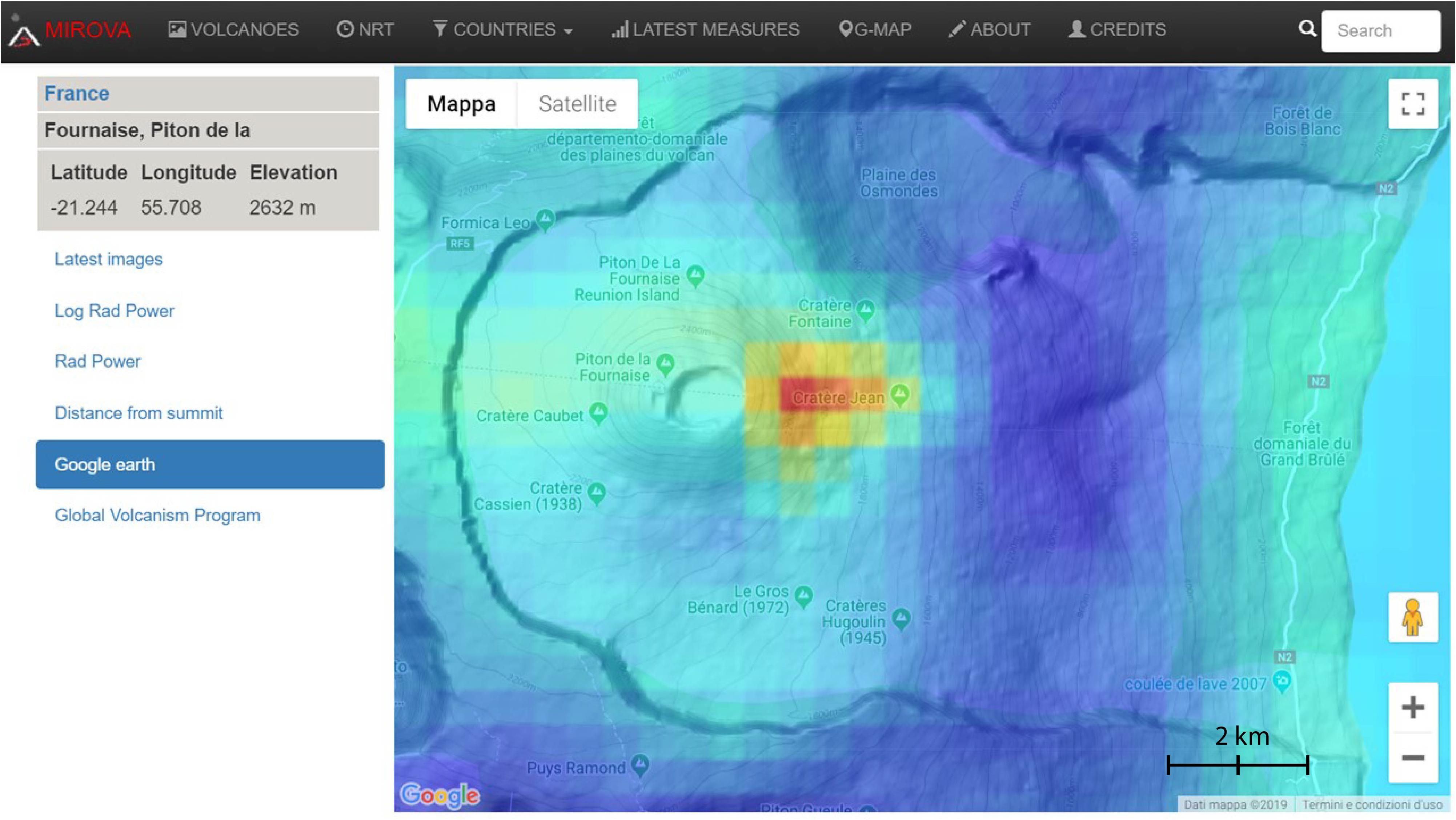
Figure 5. Snapshot of the Google Map’s overlay posted on MIROVA website for the Piton de la Fournaise volcano, La Réunion Island (accessed on 14 June 2019). The overlay image represents the Brightness Temperature (BT) recorded by MODIS in the MIR channel, resampled to a spatial resolution of 500 m. Yellow to red pixels indicate higher BT values associated to the presence of a cooling lava flow on the upper eastern flank of the volcano.
All the outputs described in the previous section are exclusively made up of static graphs that are continuously overwritten as newer data are processed. The original images (i.e., 50 × 50 km maps) and time series (numerical tables) are not archived on a web database and cannot be query and downloaded. Although this limitation prevents any direct data download, the management of the complete MIROVA database (that currently include a total of more than 2 million images) would require a large bandwidth and greatly slow down the use of the website.
Data sharing is currently done by request through email by the observatories. This practice, although slow and impractical from an operational point of view, allows a continuous exchange of information and discussions between the system developers and the end users, not necessarily experts in the interpretation of remote-sensed thermal data.
Operational Use of Mirova for Volcano Monitoring: Experiences From the Observatories
The MIROVA system was developed in 2013 in collaboration with the University of Firenze (Italy) for monitoring Italian volcanoes on behalf of the Italian Department of Civil Protection (DPC). Since then, the satellite thermal data have been integrated with the ground-based data of the Laboratorio di Geofisica Sperimentale (LGS) of the University of Firenze to provide to the DPC daily/weekly and monthly reports regarding the activity of Stromboli and Etna (Italy) volcanoes4. During major eruptive crises of these volcanoes, the effusion rate and erupted volume calculated from MIROVA were presented and discussed at the daily briefing organized by the Volcano Risk management of the Italian Civil Protection Department (DPC) together with the scientific staff of the Istituto Nazionale di Geofisica e Vulcanologia (INGV).
In recent years, and thanks to its easy application on diverse volcanic environments, the MIROVA system has gradually turned into a small pilot project testing the capabilities for global volcano monitoring, with the observations now covering the full range of volcanic activities in near real time at 216 volcanoes (Coppola et al., 2016a). Currently, the website is routinely used by 17 volcanological observatories or other institutions in charge of volcano monitoring (Figure 6 and Supplementary Table S2), supporting their daily monitoring duties and management during eruptive crises. Since 2016, MIROVA is included in the list of websites consulted by the ARISTOTLE2 program (All Risk Integrated System TOward Trans-boundary hoListic Early-warning – European Natural Hazards Scientific Partnership) for volcanic surveillance on a global scale by the Emergency Response Coordination Centre (ERCC5). Together with other remote sensing systems, MIROVA is currently used by the Volcano Disaster Assistance Program (VDAP), formed in 1986 to assist foreign partners in mitigating hazards at their country’s threatening volcanoes (Lowenstern and Ramsey, 2017).
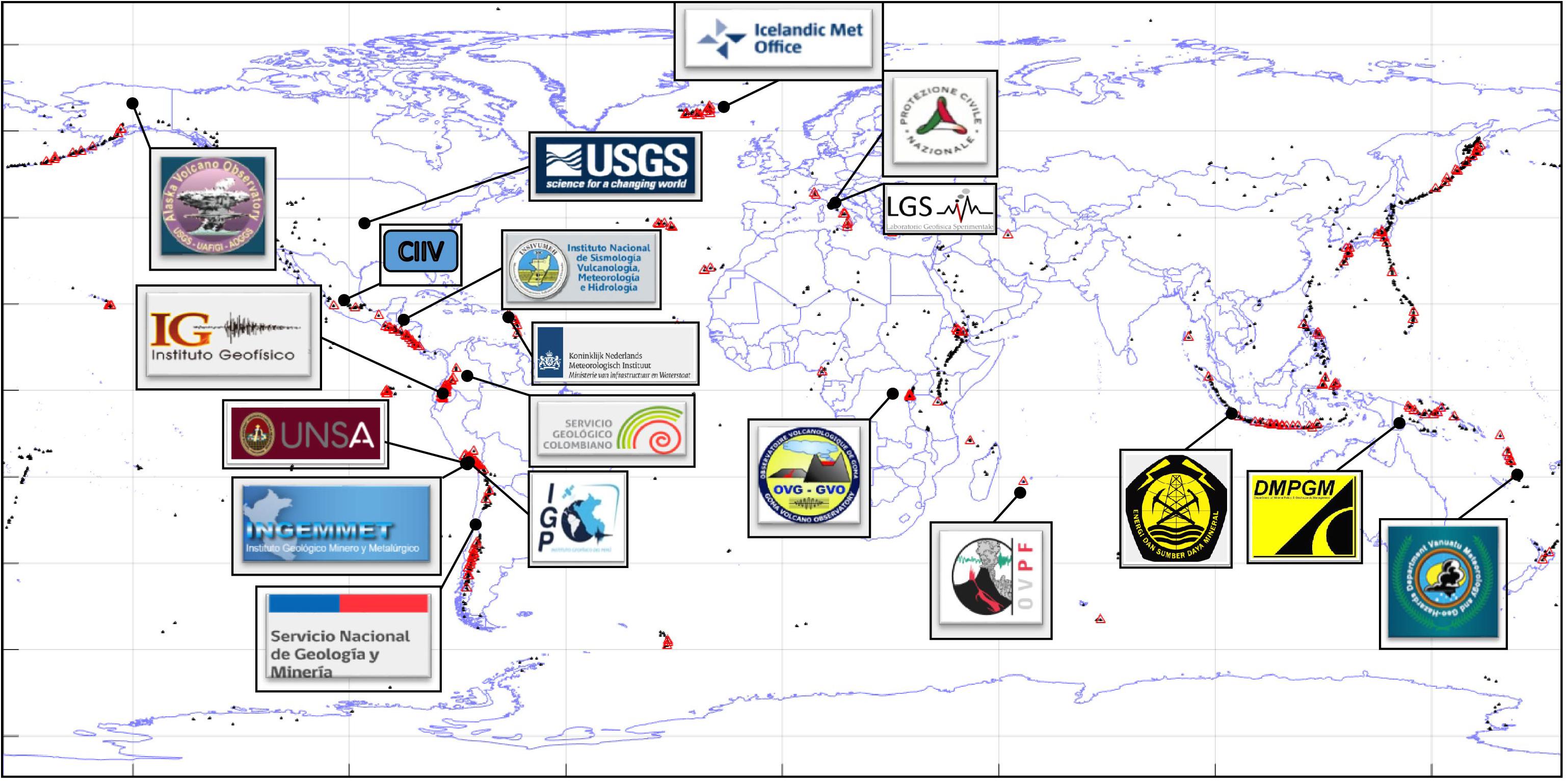
Figure 6. Volcanoes currently observed by MIROVA (red triangles) with distribution of observatories (logos within insets) that use MIROVA in support of monitoring activities (see Supplementary Table S2 for details).
In order to understand user’ experiences and motivation in using the MIROVA system, a survey (see Supplementary Appendix) was recently conducted, whose results can be summarized in the following four main points.
State of the Art of Operational Thermal Remote Sensing Systems Currently Used by Volcano Observatories
None of the participating observatories has its own automatic detection/quantification hot spot system, but they rely on systems developed and maintained by external groups. Although there are many thermal remote sensing data available online (see Supplementary Table S1), most of them are not operational in near real time and in the area of interest. The most used remote sensing thermal monitoring systems are those based on moderate resolution sensors, such as MODIS data (MIROVA, MODVOLC, REALVOLC) or VIIRS (FIRMS), which provide approximately 2/4 images daily, at a resolution of 1 km. Some observatories also use automatic systems based on higher sampling rate data (e.g., HOTVOLC, HOTSAT based on SEVIRI data), which are particularly useful for tracking volcanic plumes or for detecting high-radiating effusive eruptions. Others use the available ASTER data (i.e., ASTER Image database for Volcanoes), based on TIR observations, which enable to detect low-level thermal activity (such as fumarole fields) but with longer revisit time (e.g., >15 days; Reath et al., 2019a). The lack of a near-real time data dissemination system makes this resource less used for monitoring purposes despite the fact that since 2011 the ASTER Urgent Request Protocol (URP) system has allowed the continuous increase of acquisitions of new volcanic activity as quickly as possible (Ramsey, 2016). Due to the lack of operational monitoring systems to analyze high-spatial resolution Short-Wave Infrared (SWIR) images, no observatory uses automatic systems focused on Sentinel 2 or Landsat 8, which have a typical revisit time of 5 to 16 days (see Supplementary Table S1). Only recently this gap has started to be covered by the MOUNTS pilot project that provides automatic Sentinel 2 hotspot detections alerts (together with other remote sensed parameters) on specific volcanic targets (Valade et al., 2019).
Frequency of Use of MIROVA Website and Volcano Alert Levels
The experiences reported by the observatories during the past 5 years allow us to state that the frequency of use of a satellite thermal monitoring system such as MIROVA strongly depends on the specific alert level of each monitored volcano. Many alert level systems exist in the world, with the majority based on four levels, e.g., the US system developed by the USGS (Normal, Advisory, Watch, and Warning). Each country (or volcano) has a specific scale which is adapted to the particular volcanological, environmental, social, political and economic context (Fearnley et al., 2012). For each level there is therefore a different need/urgency to have up-to-date data on the thermal state of the volcano which can be summarized as follows:
Normal
Volcano is in typical background, non-eruptive state or, after a change from a higher level, volcanic activity has ceased and volcano has returned to non-eruptive background state. The thermal state of volcanoes at this level is typically checked on a monthly basis (e.g., Vesuvius, Italy) or occasionally, for example to verify reports or rumors (e.g., Cumbal, Colombia; Mt. Scenery, Saba Island – Netherlands). Automatic alerting systems (for example, by sending emails) can facilitate the immediate detection of hotspots. This is especially useful in remote volcanoes in a quiescent state (Normal alert level), when thermal state is irregularly controlled and for which there are no other operational monitoring systems.
Advisory
Volcano is exhibiting signs of elevated unrest above known background level or, after a change from a higher level, volcanic activity has decreased significantly but continues to be closely monitored for possible renewed increase. Several volcanoes may persist at this levels for several months or years showing persistent thermal anomalies (e.g., Stromboli, Italy; Villarrica, Chile). The typical frequency of use in this case is one to several times per week, in accordance with the reporting tasks of each observatory. In these cases, automatic alert systems, by setting up specific thresholds, can be used to warn the end-user(s) of a sudden increase in thermal activity that may lead to a higher alert level.
Watch
Volcano is exhibiting heightened or escalating unrest with increased potential of eruption, timeframe uncertain, or eruption is underway but poses limited hazards. In this case, the thermal data are typically checked on a daily basis, just before briefing or to write the daily reports (e.g., Etna, Italy, during major lava flows or paroxysms; Piton de la Fournaise, Réunion Island, during eruptions). At this alert level, the daily comparison of thermal data with other parameters (seismic, outgassing, deformation) is usually an integral part of the discussions within the observatory, and for analysis and evaluation of the ongoing volcanic activity.
Warning
Hazardous eruption is imminent, underway, or suspected. During imminent eruptions or ongoing eruptions, the thermal data are checked daily or hourly during crisis (e.g., Bardarbunga, Iceland [August 2014]; Agung, Indonesia [August 2017]; Kilauea, United States [May 2018]). In these warning conditions, each dataset must be more frequently updated. A screen grab of the MIROVA website is often displayed in the monitoring acquisition rooms during eruptive crisis (e.g., Nevado del Ruiz, Colombia; Manam, Papua New Guinea).
What Kind of Information Is Retrieved From MIROVA
The most useful information to get from a hotspot detection system such as MIROVA, according to volcano observatories, is summarized in the following four parameters:
Presence or Absence of Thermal Anomalies
The first and most direct information obtainable from a hotspot detection system is the presence/absence of thermal anomalies. During volcanic unrest, the appearance of a hotspot is indicative of the breaking of the magmatic system through the surface. In the case of MIROVA, which only detects high-temperature features, such appearance is commonly ascribed to the presence of magma at the surface, or at very shallow depths (Laiolo et al., 2019). For example, during the eruptive crisis of Ubinas in 2014, the detection of a small thermal anomaly inside the deep crater signaled the first appearance of a lava dome (confirmed only later by observations on the ground) after several days of seismic unrest. The opening of a magma path marked the beginning of an intense explosive activity that culminated few weeks later in a series of major explosions (Coppola et al., 2015). The fast detection of thermal anomalies is therefore very important to indicate the opening of the system, and coupled with other geophysical parameters often leads the rise of the alert level, as recently occurred at Sabancaya (Perù) (Reath et al., 2019b). On the other hand, the absence of a thermal anomaly is also important, and may help to discard any possible surface activity associated with deep seismic activity. This occurred for example at the Galeras and Chiles-Cerro Negro (Colombia) volcanoes, which experienced some seismic swarms in the last 4 years (2015–2019) without showing any kind of surface activity (Ebmeier et al., 2016). In other cases, and especially during clear waning phase, the persistence of thermal anomalies is useful to determine the thermal state of the associated lava bodies. At Momotombo (Nicaragua), where lava flowed down the flank during the 2015 eruption, from visual observation it appeared like the eruption had ceased and that the optically black lava had cooled. However, thermal data showed that the lava near the summit and along the flow was still hot. Thermal anomalies, combined with seismic activity, suggested that the lava extrusion was still feeding the flow and that the potential advancement for lava flow front/perimeter was still present. Generally, the presence or absence of thermal anomalies is often used to depict the beginning and the end of eruptive episodes, especially for which direct or indirect observations from other monitoring systems are not available.
Intensity
The intensity of the thermal anomaly, referred to as VRP, is the fundamental parameter of MIROVA and constitutes the main added value compared to other systems able to detect the presence/absence of hotspots, without quantifying their intensity in terms of radiated energy. Besides being a direct measurement of radiated thermal energy, the VRP can be used to calculate the area or the temperature of the lava body using Stephan-Boltzmann law (see details in section “Download, Data Processing, and Sensibility”), if at least one of the two variables can be estimated (or assumed) independently. The area or temperature of a lava dome is a parameter that can be easily understandable even by non-experts, and provides a clear idea of the size of the phenomenon underway. Moreover, during effusive eruptions, the VRP can be used to calculate the Time Averaged lava Discharge Rate (TADR) and the volume of erupted lava, subject to a calibration of the conversion factors or a basic knowledge of the type of erupted lava (Coppola et al., 2016a; Bernard et al., 2019). These second level products (Area, Temperature, TADR, Volume) are important for hazard evaluation and communication, and require data independent of the satellite monitoring system as well as interpretation skills by the end-user. Finally, the quantification of the thermal activity by means of a parameter that compute in a standardized and consistent way (such as the VRP), allows for the comparison between different volcanoes or between different eruptions of the same volcano. Statistical analysis of the VRP allows identification of distinct thermal regimes (Coppola and Cigolini, 2013) and may be used to detect change of activity, such as from Strombolian activity to effusive activity (Coppola et al., 2013, 2019a; Naismith et al., 2019; see section “Forecasting Eruptive Trends”), or the occurrence of episodes of dome growth (Werner et al., 2017). During the growth of a new spatter cone on February 29, 2016 inside the Nyiragongo Crater, MIROVA data were used to eventually advise about anomalous large thermal emissions within the lava lake and/or the presence of active lava flows in areas around the volcano (see details in the following section).
Location/Dimension of the Hotspot and Its Distance From the Volcanic Summit
Locating the thermal anomaly with precision is fundamental to recognize the type of activity in progress, to assess the areas at risk and possibly to be able to run forecast models (for example, forecasting flooding of lava flows; Harris et al., 2016). The ability to promptly locate these anomalies is closely linked to the spatial and temporal resolution of the sensor used. With its 1 km pixel, the MIROVA system does not have the spatial resolution sufficient to locate the eruptive vents precisely. However, in most cases, this moderate resolution was found to be sufficient to discriminate between intra-crater anomalies (produced, for example, by the fissure opening, lava dome extrusion, development of high-temperature fumarole fields, appearance of lava lakes) and lateral anomalies (associated with lava flows or large pyroclastic flows descending the flanks of the volcano). This information, supplied in a relatively short time (e.g., few hours), was fundamental for hazard evaluation and to timely forecast the eruptive scenarios. It is also useful tool to detect lava flows generated from more distal flank fissures, although naturally, there are many false positives (i.e., fires). At effusive eruptions, thermal data can be used to map (at low resolution, e.g., 1 km) lava flow emplacement in order to detect the position of the flow front and track the formation of lava tubes (Coppola et al., 2019a). At dome-forming volcanoes, such as Sinabung (Indonesia), the spatial distribution/extension of the thermal anomalies allows for an assessment of stable dome growth, indicated by cluster of hot pixels at the summit, while for collapsing dome materials (large rock fall or pyroclastic density currents) and viscous lava flow, the hot pixels will be aligned with a channel down the flank. In the case of hot pixels down a channel, the sector and distance of furthest pixels provides a rapid estimate of hazardous areas that ought to be maintained as part of an exclusion zone. This becomes critical as eruption duration spans years and societal pressure on local officials to re-open evacuated areas increases (see Supplementary Appendix – VDAP Survey).
Eruptive Evolution, Trends, and Patterns
In conjunction with seismic, gas geochemistry, visual change and deformation data, MIROVA provides an initial check for level and type of activity at volcanoes, e.g., the occurrence of lava flows, domes or simply hot, degassing open vents. Being able to track relative changes in thermal output (i.e., VRP) as a time series, provides a useful assessment of any changes in activity, although a lack of thermal data does not necessarily means a volcano is cooling off (it can be due to cloud or thick ash cloud cover). Stationary, waning or intensifying trends obviously have different implications on the evaluation of the hazards and can be used (modeled) to define future eruptive scenarios, and possibly to make forecasts. At Piton de la Fournaise (La Réunion Island), the knowledge of the effusion rate trend (derived from thermal data), in combination with previously generated models of the likely lava flow path, has been useful to predict whether the flow will tend to lengthen or shorten, and whether the lava flows will reach the sea, cutting the national road that goes around La Réunion Island (Harris et al., 2017, 2019). Moreover, by comparing this variable with the gas flux and deformation rate, it is possible to analyze the balance of the erupted/degassed material and eventually to quantify magmatic intrusions or endogenous growth (Coppola et al., 2017, 2019a). During the Holuhraun (Iceland) eruption 2014–2015, MIROVA was regularly checked to monitor the level of activity at the eruption site (Barsotti et al., 2019), to estimate of lava discharge rate and, eventually, to declare the end of the eruption based on the observed eruptive trend (Coppola et al., 2017). In the case of Nevado del Ruiz (Colombia), MIROVA’s thermal anomaly data matched very well with shallow seismicity increments, ash emissions (seismically associated to volcanic tremor), large SO2 fluxes, tiltmeters inflationary trends, etc. The largest VRP values have been clearly associated with magma ascent to shallower levels of the volcanic conduit and surface magma extrusion process (lava dome emplacement within the inner part of crater). During the 2018–2019 Manam (Papua New Guinea) eruptions (Global Volcanism Program, 2019), the thermal trends provided confirmation that increased RSAM was due to renewed activity and that the activity involved the effusion of lava flows, and in what direction the flows were moving. After reaching the climax, the thermal data also indicated the waning of the activity, suggesting that the effusive event was coming to an end. Similarly, during the 2014 eruption of Ubinas (Peru), the increasing VRP values were compared with the state of seismicity, as well as with other parameters (such as ash or SO1 missions, etc.; Coppola et al., 2015) to provide, eventually, recommendations to the civil protection authorities for the evacuation of the population.
How Thermal Data Are Used
The use of the observations/images/data obtained from MIROVA is highly varied and depends fundamentally on four factors: (i) the presence and (quality) of a ground-based monitoring network; (ii) the accessibility of the volcano; (iii) the alert level; and (iv) the type of activity in progress.
Remote-sensed thermal observations clearly provide invaluable information for volcanoes not monitored in other ways, and may represent the only evidence of ongoing volcanic activity (Coppola et al., 2016a). These data may provide fundamental indications on the evolution of the unrest/eruption (e.g., stationary, increasing, decreasing), although incomplete.
When an eruption begins, remote sensing methods allow the surveillance of volcanic phenomena, and help minimizing the risks associated with direct exposure of observers to the activity. Often, it is very difficult, impossible, or too expensive to make frequent observations of the volcanoes’ summit, as well as to measure directly lava flows, domes or fumarole temperatures. It is usually impossible to conduct frequent airborne observations within the crater, so MIROVA data are useful to get a longer time series for routine monitoring, to verify reports, rumors, or provide observations for internal and wider discussions before making a decision to assess the level of activity at the volcano.
At basaltic volcanoes equipped with a large ground-based monitoring network, such as Stromboli, Etna, Piton de la Fournaise, etc., MIROVA is commonly used to estimate time-averaged lava discharge rates (TADR) and erupted volumes in near real time (Coppola et al., 2019a; Laiolo et al., 2019), to define imminent eruptive scenarios (Ripepe et al., 2017) and to model the path and length of lava flows (Harris et al., 2017, 2019).
In summary, the processed data provided by MIROVA are used by the observatories in different ways, but essential for monitoring activities, internal discussions on the volcano’s activity level and for real-time calculations of the erupted volume and eruptive trend. This information actually converges to activity reports (daily, weekly, monthly or extraordinary reports), in which the thermal data are integrated with other monitoring parameters in order to evaluate the state of activity and to maintain situational awareness.
The Volcano Disaster Assistance Program (VDAP) also includes the use of the website at trainings offered at volcano observatories where they are just starting to use remote sensing as part of their volcano monitoring. The MIROVA website is freely accessible to observers who do not necessarily have the background knowledge or funding support for more elaborate remote sensing analysis. Furthermore, the website is reliable, immediately accessible and requires no maintenance on their part beyond reliable internet connectivity.
Key-Case Studies
Below we summarize some case studies for which MIROVA satellite thermal data proved to be an essential element in the detection and characterization of volcanic (thermal) unrest, in the forecast of eruptions and in the recognition of particular trends and patterns.
Thermal Unrest
Volcanic unrest is commonly defined as a change from “normal state” at a volcano, or deviation from its background behavior (Gottsmann et al., 2017). Seismic activity, surface deformation, heat or gas emissions can be used as unrest indicators for a certain volcano and need to be interpreted to be able to implement timely mitigation actions (Phillipson et al., 2013). The appearance of thermal anomalies before an eruption is often considered as an important precursor and a clear symptom of volcanic unrest (Reath et al., 2016). A recent review (Furtney et al., 2018) suggests that about 19% of major eruptions are anticipated by thermal precursors detected by satellites, although the mere presence of thermal activity to a volcano does not necessarily constitute a change from its background level.
The MIROVA archive now embeds 65 of 72 volcanoes that after 2002 produced at least one VEI 3 eruption (Global Volcanism Program database, accessed on 26 June 2019). A preliminary analysis suggests that only 4 to 5 volcanoes of these 65 MIROVA targets, displays anomalies that can be considered as a thermal precursor of the upcoming VEI 3 eruption (Figure 7). This number possibly increases if we consider less explosive eruptions and changes from background activity at persistently active volcanoes. However, from our analysis, only 6–8% of the volcanoes seem to develop thermal unrest before a VEI 3 eruption detectable by MIROVA. It is interesting to note that the thermal unrest observed at these volcanoes shows unique behavior in terms of duration, pre- and post-eruption trends, as described below (Figures 7a–e):
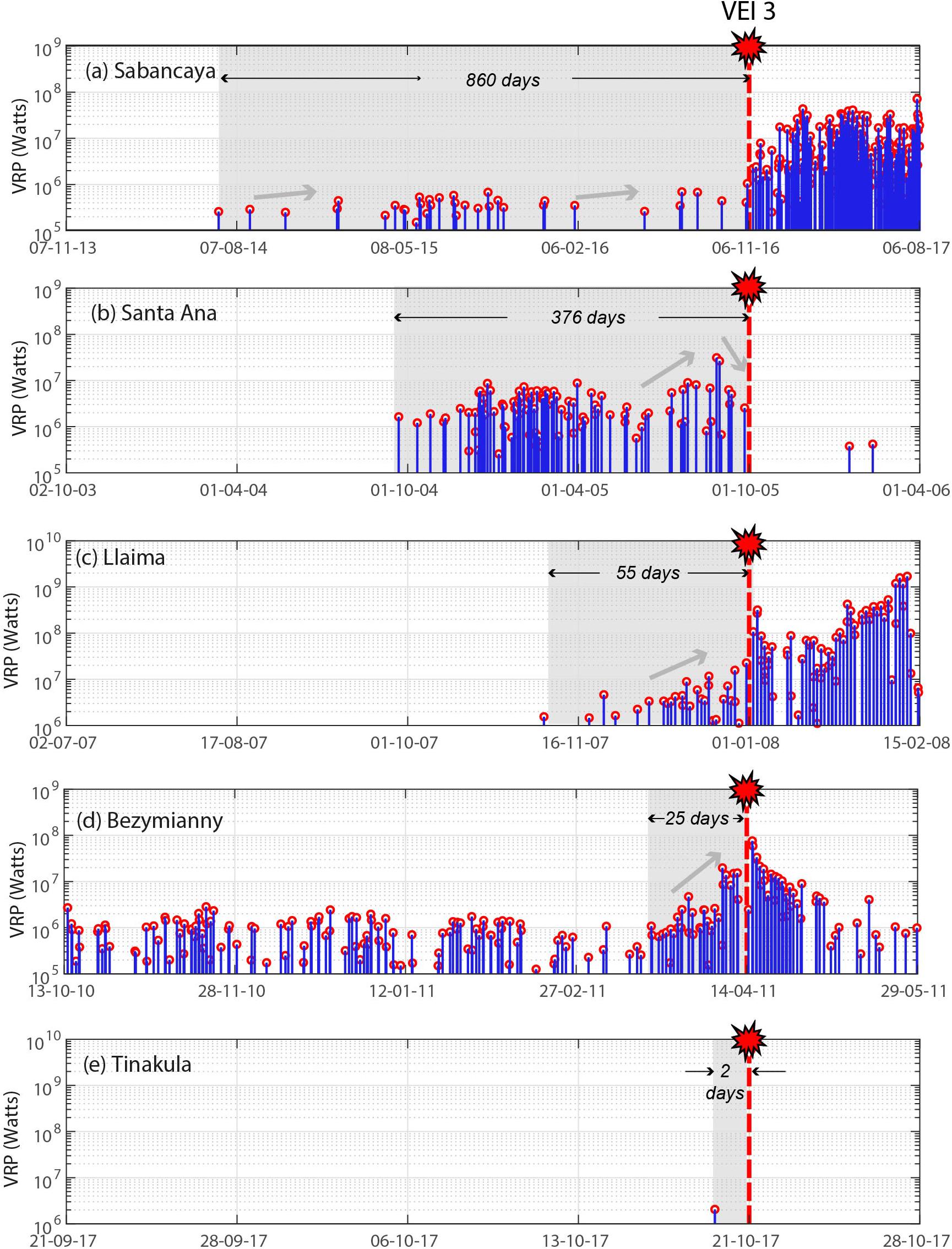
Figure 7. Five cases of thermal unrest detected by MIROVA: (a) Sabancaya, (b) Santa Ana, (c) Llaima, (d) Bezymianny, (e) Tinakula. The red dashed line indicates the occurrence of a large explosion (VEI 3). The gray fields indicate the periods showing precursory thermal activity. The duration of each thermal unrest phase is indicated with arrows. Note how each volcano shows different behavior of thermal unrest in terms of duration, pre-eruptive level and trend, as well as post-eruptive pattern. See the text for more details.
Sabancaya, Perú (Development of Fumarole Fields – Figure 7a)
This is the archetype of thermal unrest consisting of the gradual appearance of very low-level thermal anomalies (< 1MW) localized at the bottom of a summit crater. It is associated with the slow (∼860 days for Sabancaya) development of a fumarole field within the crater area, showing a slight increase in the area and temperatures of fumaroles and almost constant water-vapor and SO2 plumes rising from the crater (Global Volcanism Program, 2016). The explosive VEI 3 eruption definitely opened the magma path and allowed the underlying magma to come to the surface producing evident and more persistent thermal anomalies (Figure 7a).
Santa Ana, El Salvador (Rupture of Hydrothermal System – Figure 7b)
In this case the thermal unrest has a pre-eruptive phase characterized by a long (∼376 days) and gradual development of a high-temperature fumarole field developing on the border of an acidic crater lake. Possibly, this type of thermal precursor develops in response to a magmatic injection at very superficial levels, which causes the rupture of the hydrothermal system feeding the acidic lake (Laiolo et al., 2017). Notably, the interaction between the fumarolic field and the crater lake may eventually cause a “decrease” in thermal activity due to self-sealing phenomena that may precede a major explosion (Laiolo et al., 2017). Unlike the previous case, the explosive event is not followed by a magmatic eruption (with lava dome extrusion and/or recurrent ash-explosions), but it is characterized by the almost total, definitive absence of a thermal anomaly (Figure 7b).
Llaima, Chile (Rise of Magmatic Column – Figure 7c)
This type of thermal precursor may occur in basaltic and basaltic-andesitic volcanoes characterized by the reactivation of the central vent after a period of quiescence. The unrest phase is typically characterized by an evident increase in thermal anomalies inside the summit crater, corresponding to the opening of the system, followed by the ascent of the magmatic column and by increasing explosive activity (Franco et al., 2019), that culminates with a paroxysmal phase (Figure 7c). The explosive activity is followed by a further intensification of the effusive activity with the consequent emission of lava flows along the flanks of the volcano (Global Volcanism Program, 2008; Bouvet de Maisonneuve et al., 2012).
Bezymianny, Kamchatka (Increase in Dome Extrusion Rate – Figure 7d)
Activity of Bezymianny consists of ongoing lava-dome growth inside a large horseshoe-shaped crater, accompanied by intermittent explosive activity and pyroclastic flows. As already advised by previous works (van Manen et al., 2010, 2013), some major explosions of Bezymianny are preceded by weeks of gradual increase of the thermal anomaly, thus constituting one of the most robust precursors for this remote volcano. In this case the thermal precursor occurred at a volcano characterized by a persistent, low level thermal activity, associated with the presence of degassing hot cracks and minor explosions. The increase of VRP prior to large explosions (Figure 7d) has been attributed to an increase in the dome extrusion rate (van Manen et al., 2010, 2013).
Tinakula, Solomon Islands (Sudden Opening of Magma Path – Figure 7e)
After more than 5 years of quietness, on 19 October 2017, a low thermal anomaly was detected by MIROVA at the Tinakula volcano. This small thermal precursor was the unique sign of renewed eruptive activity at this remote volcano and preceded by only 2 days the large VEI 3 explosion which occurred on 21 October (Global Volcanism Program, 2018; Laiolo et al., 2018). This short unrest is probably a limit case for MIROVA-type systems (i.e., based on Moderate Resolution data) and suggests that the first thermal anomaly, detected after a several year-long period of quiescence, must be seriously evaluated and can represent a solitary short-term precursor of an incoming major eruption. On the other hand, it also outlines the need to have thermal satellite data with a high temporal resolution by keeping a high efficiency and a very limited number of false alerts. In the specific case of Tinakula it was necessary the post-event supervision (e.g., after the VEI 3 explosion) of the image of October 19, in order to exclude that it was a false alert. Actually, one real problem is how to assess the accuracy of each thermal alert, since there will always be a possibility of false or non-volcanic alerts. Fires constitute non-volcanic heat sources frequently detected in volcanic areas, but their distinction from volcanic hotspots cannot currently be done on a spectral basis. In addition, a smaller but variable percentage of false alerts, generally comprised between 0 and 3% (number of false alerts/number of MODIS overpasses), is detected by the MIROVA system at different volcanoes (Coppola et al., 2016b). These false alerts depend on the regional and local environmental conditions as climate, elevation, topography and land cover type.
With the exception of these five cases, our data suggest that most of VEI 3 eruptions are not preceded by thermal anomalies detectable by monitoring systems such as MIROVA. This is possibly due to the sensitivity of this type of systems that fail to detect small, low-intensity thermal anomalies. Indeed, the percentage of thermal unrest rises considerably if thermal data with higher spatial resolution are used as suggested by Furtney et al. (2018) and Reath et al. (2019a). On the other hand, it cannot be excluded that the appearance of thermal precursors and the explosivity index of the subsequent eruption are somehow related to the degree of openness of the shallow magmatic system. A more detailed analysis, which also considers the possible appearance/variation of thermal anomalies before the numerous VEI 1-2 eruptions that have occurred in the MODIS era, will surely help to clarify whether there is a correlation between the timing and intensity of thermal unrest and the magnitude of the explosive eruption.
Forecasting Eruptive Trends
Forecasting the time and scale of a volcano eruption is the goal of several monitoring and research efforts (see Marzocchi and Bebbington, 2012 for a review). Generally, with eruption forecasting we mean the ability to evaluate the occurrence of a volcanic eruption on the basis of a probabilistic approach. Forecasting what happens after eruption onset, or when an eruption will come to an end is also critical for the decision-making procedure and for updating eruptive scenarios (Bebbington and Jenkins, 2019).
As illustrated in the previous paragraph, in most cases, the MIROVA data do not record thermal precursors before > VEI 3 eruptions and therefore in many cases do not allow to make forecasts of imminent major explosive eruptions (with some important exceptions; Figure 7). However, thermal data show their strength during the course of eruptions, allowing, in some cases, the generation of forecasts of future activity based on the analysis of eruptive trends (Bonny and Wright, 2017).
Many effusive eruptions show a characteristic exponential decrease of effusion rates over time, ascribed to elastic or inelastic processes occurring within the decompressing magmatic system (Wadge, 1981; Coppola et al., 2017). In Figure 8a we show the TADR and erupted volumes, reconstructed through the MIROVA data, for the April 2018 eruption of Piton de la Fournaise (Harris et al., 2019) that can be considered as archetypal for this type of trend. Taking into account the uncertainty in the TADR estimates (typically between 30 and 50%; Coppola et al., 2019b), several types of predictions are possible. For example, the initial flow rate (Q0) can be used (as soon as the MODIS data has been processed) within an effusive response protocol (Harris et al., 2017, 2019), to timely provide the observatory flow path projections and maximum run-out of the emplacing lava flow (Figure 8b).
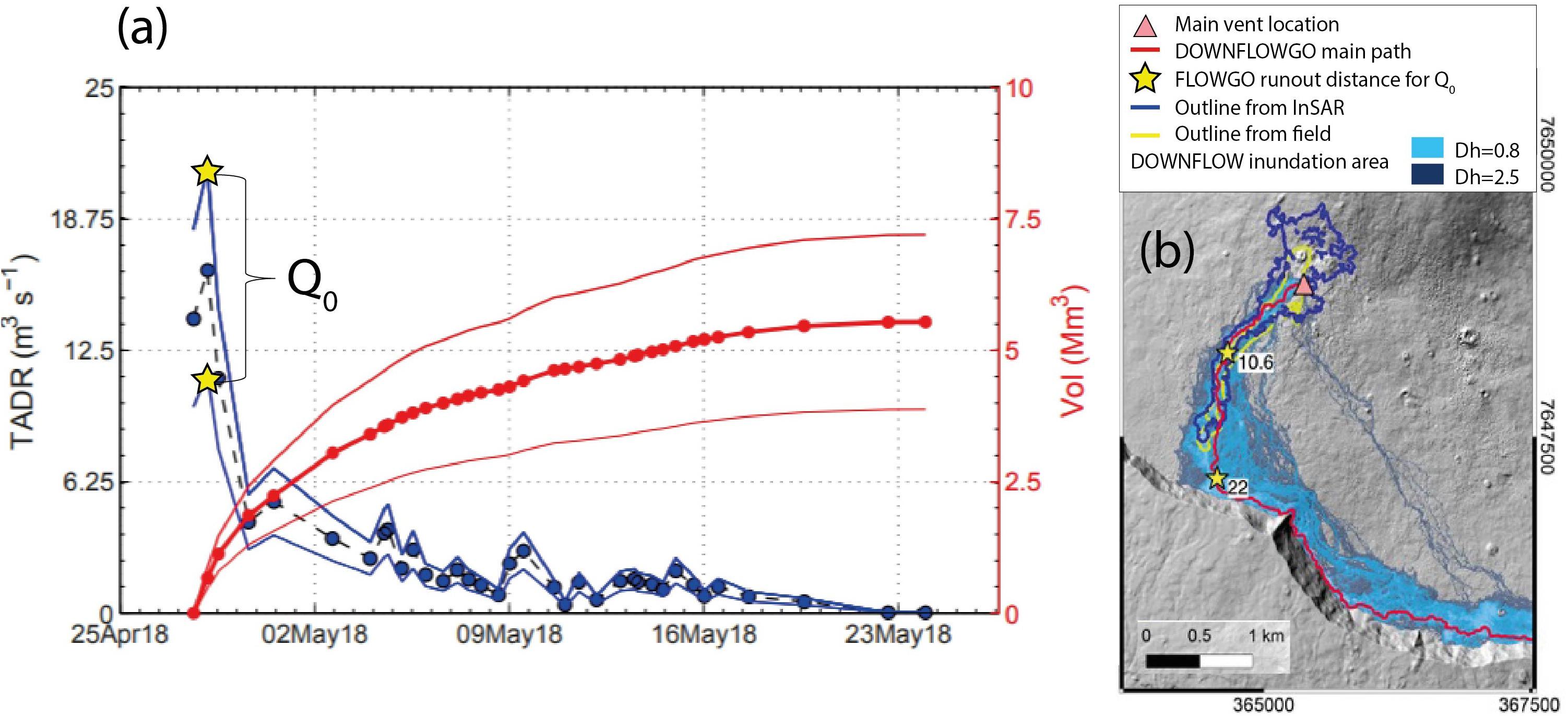
Figure 8. (a) Middle Infrared Observations of Volcanic Activity-derived TADR (blue circles) and cumulative volume (red circles) provided to the Observatoire Volcanologique du Piton de la Fournaise (OVPF) during the April 2018 eruption. Q0 is the initial flow rate, used to model the maximum runout of the emplacing lava flow (modified from Harris et al., 2019). (b) Lava flow inundation map derived from ingestion of MIROVA-derived TADR into DOWNFLOW-PyFLOWGO models. The numbers (in m3 s–1) next to each star correspond to maximum and minimum estimate of Q0 measured on 28 April 2018. Overlain are the limits of the flow field defined from InSAR incoherence (blue outline) and field mapping (yellow outline) on the same dates. Background shows the DOWNFLOW inundation area (modified from Harris et al., 2019).
Once the exponential trend has become recognizable, as in the case of the Bárðarbunga-Holuhraun (Iceland) 2014–2015 eruption, extrapolation from initial data can be used to evaluate final (or intermediate) erupted volumes (Figure 9a), useful, for example, to run simulation codes for lava flow invasion maps (Tarquini et al., 2019).
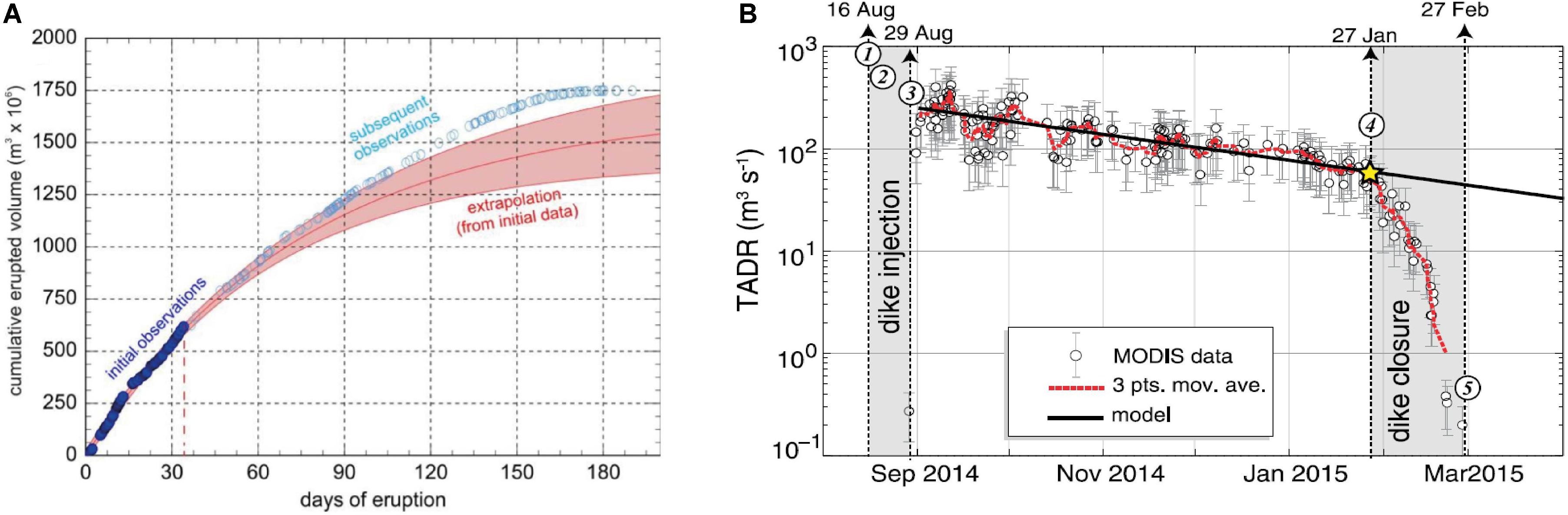
Figure 9. (A) Extrapolation of the cumulative erupted volume during the Holuhraun 2014–2015 eruption, based on the initial 5 weeks of observations (modified from Tarquini et al., 2019), (B) Time-averaged lava discharge rate (TADR) measured during Holuhraun eruption (Iceland) (black circles; modified from Coppola et al., 2017) shows exponential decay of effusive activity. Departure from exponential trend began on 27 January 2015 and preluded the end of the eruption on 27 February. The yellow star indicates the critical flow rate Qc of ∼50 m3 s–1 when dike started to close. Numbered circles indicate main steps of eruption: (1) start of rifting episode and lateral magmatic injection; (2) start of caldera subsidence; (3) start of effusive eruption; (4) closure of lateral dike; and (5) end of eruption (modified from Coppola et al., 2017).
Projection of effusion rate trends to the future (black line Figure 9b) may also be used to forecast/predict the end of this type of eruptions (Coppola et al., 2017). In fact, before the end of the Bárðarbunga-Holuhraun eruption there was a drastic decrease in the effusion rates with respect to the model based upon the exponential curve. In this case, the phenomenon was attributed to the gradual closure of the dike, once the effusion rates (or driving pressure) dropped below a critical value (yellow star in Figure 9b). The detection in real-time of a change from the expected trend can therefore be useful information to estimate when the eruption end (Coppola et al., 2019a).
At persistently active volcanoes, such as Etna or Stromboli, the long-term analysis of MIROVA data has allowed the determination of specific thresholds (Figure 10) that separates the typical open-vent activity (with continuous degassing and intermittent strombolian activity), from more intense phases characterized by effusive activity and/or fountaining episodes (Coppola et al., 2013, 2019a). According to the authors, these thresholds represent a critical magma flux (about 0.1–0.3 m3 s–1) above which the ascending magma can no longer be recycled in the conduit, and must be partially or completely extruded through effusive activity. The definition of these thresholds (blue dashed lines in Figure 10), allows the separation of the different regimes of activity, making it possible to track the rise of magma within the conduit and to advise about potential transitions toward magma effusion (Valade et al., 2016; Ripepe et al., 2017; Laiolo et al., 2019).
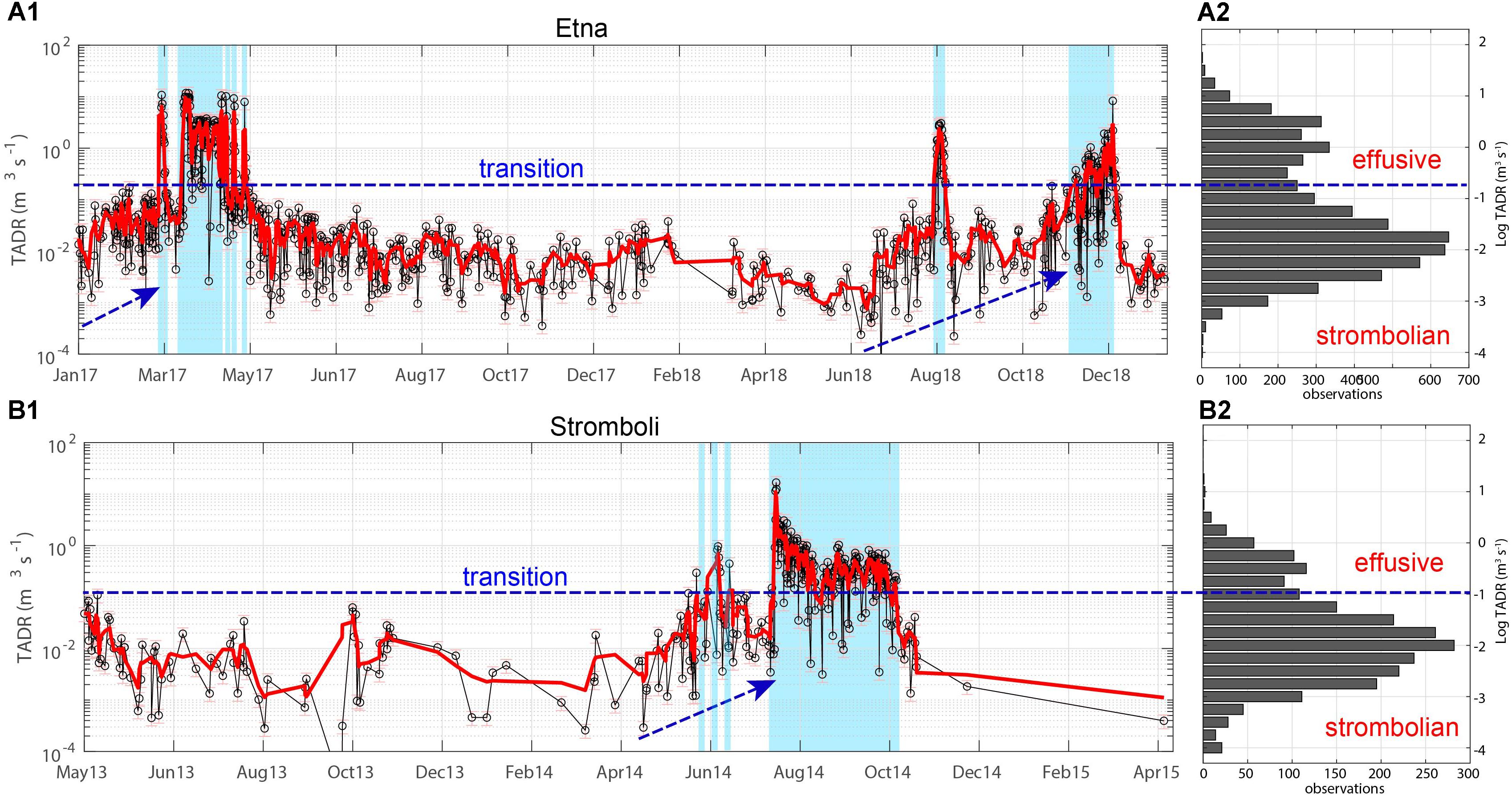
Figure 10. Two-years long time-series of MIROVA-derived TADR for Etna (A1) and Stromboli (B1) volcanoes (modified from Valade et al., 2016 and Laiolo et al., 2019, respectively). A threshold separating the Strombolian and the effusive regime, is calculated from the statistical analysis of log-transformed TADRs (A2,B2) that outline the bimodal behaviors of magma flux.
Limits and Future Developments
The surveys carried out by the observatories (Supplementary Appendix) highlight the current limits of the MIROVA system and provide guidelines for future development of an optimal satellite thermal monitoring system. In particular, the following points were highlighted:
Image Quality Assessment
Providing an evaluation of cloudiness and image/data quality is fundamental. In particular, any hotspot detection system should be able to quantify the effects of clouds and viewing geometry condition, within each acquired image (Coppola et al., 2016a). This fundamental step is currently absent in all the operational systems (Supplementary Table S1) and surely requires further efforts. One particular goal is to be able to determine automatically if the absence of a hotspot is real (no sign of thermal anomaly) or if it is due to the presence of clouds or to unfavorable viewing geometry. Typical estimates of the average cloud fraction within a region of interest may be useful for long-term analysis but not practical for operational monitoring in real time, where every single image must be interpreted. Moreover, when considered over large areas, the quantification of cloud fraction may cause misinterpretation of thermal data. In fact, in many cases thermal anomalies within high-altitude summit craters may be discarded or classified as strongly attenuated, because the surroundings pixels are cloudy (although the crater is actually without cloud cover). Quantification of cloud attenuation on a pixel per pixel basis would be an ideal solution (Koeppen et al., 2011), but this would require the collection and analysis of many more bands, including ancillary metadata and other atmospheric properties for every acquired image. Although this approach may provide excellent results, it remains time- and resource- consuming, especially for real time analysis. Similarly, as several volcanoes have steep slopes, the influence of topography on lava flow quantification from satellite thermal data may be important and require case by case correction models (Zakšek et al., 2017). An apparent decrease in heat flux may be due to unfavorable viewing conditions. This information must be accessible to users in order to correctly interpret each individual image. A promising solution is provided by machine learning (i.e., Valade et al., 2019), where an artificial intelligence is instructed on the basis of a supervised manual selection of cloud free images acquired in optimal viewing conditions.
Improve Spatial Resolution
The spatial resolution of the satellite thermal detection system is fundamental in every aspect of volcanic monitoring. In particular, it has been proved that the integration of high spatial resolution data in the TIR (i.e., ASTER; Landsat8-TIRS) as well as in the NIR/SWIR (i.e., Landsat8-OLI; Sentinel2) greatly improves the ability to detect smaller or low intensity thermal anomalies (Jay et al., 2013; Reath et al., 2019a; Valade et al., 2019). From an operational point of view, this translates into detecting a thermal precursor with respect to days/months earlier than moderate-resolution systems (Reath et al., 2019b). Nevertheless, integration of multisensor thermal data into a single volcanic hotspot detection system is still matter of development (Laiolo et al., 2019), and currently no operative system exists that systematically provide this integrated information on the web (Supplementary Table S2).
Moreover, given the variability of wavelengths at which these sensors operate (from SWIR to MIR and TIR), the thermal information retrieved display different sensitivities to the wide range of temperatures of volcanological interest (i.e., from 50 to 1200°C) and is often inhomogeneous between the different datasets (for example, spectral radiance, hotspot area, temperature, radiative power).
A preliminary comparative study between MODIS-MIROVA and SENTINEL2 (Massimetti et al., 2018; Valade et al., 2019) showed that the two systems provide extremely coherent and complementary information. The example of Figure 11 shows what could be an integrated output of these two datasets (Massimetti et al., 2018) for the case of Agung volcano. The frequent acquisition of SENTINEL2 images (every 5 days) allows the tracking of the evolution of the area of the thermal anomaly inside the Agung crater, with a discrete temporal detail (red circles in Figure 11). The latter is in excellent agreement with the thermal flux measured by the MODIS (blue stem in Figure 11) to which it adds a high spatial resolution (20 m in the NIR/SWIR) that allows the location and mapping of the thermal anomaly inside the crater with great detail. Small changes in the location, area and or temperature of the hot feature are thus easily tracked by SENTINEL 2 data, and are coherent with MIROVA dataset. An additional improvement can be obtained by integrating also the data provided by Landsat8-OLI sensor (30 m), considering the appropriate spectral bands in NIR/SWIR.
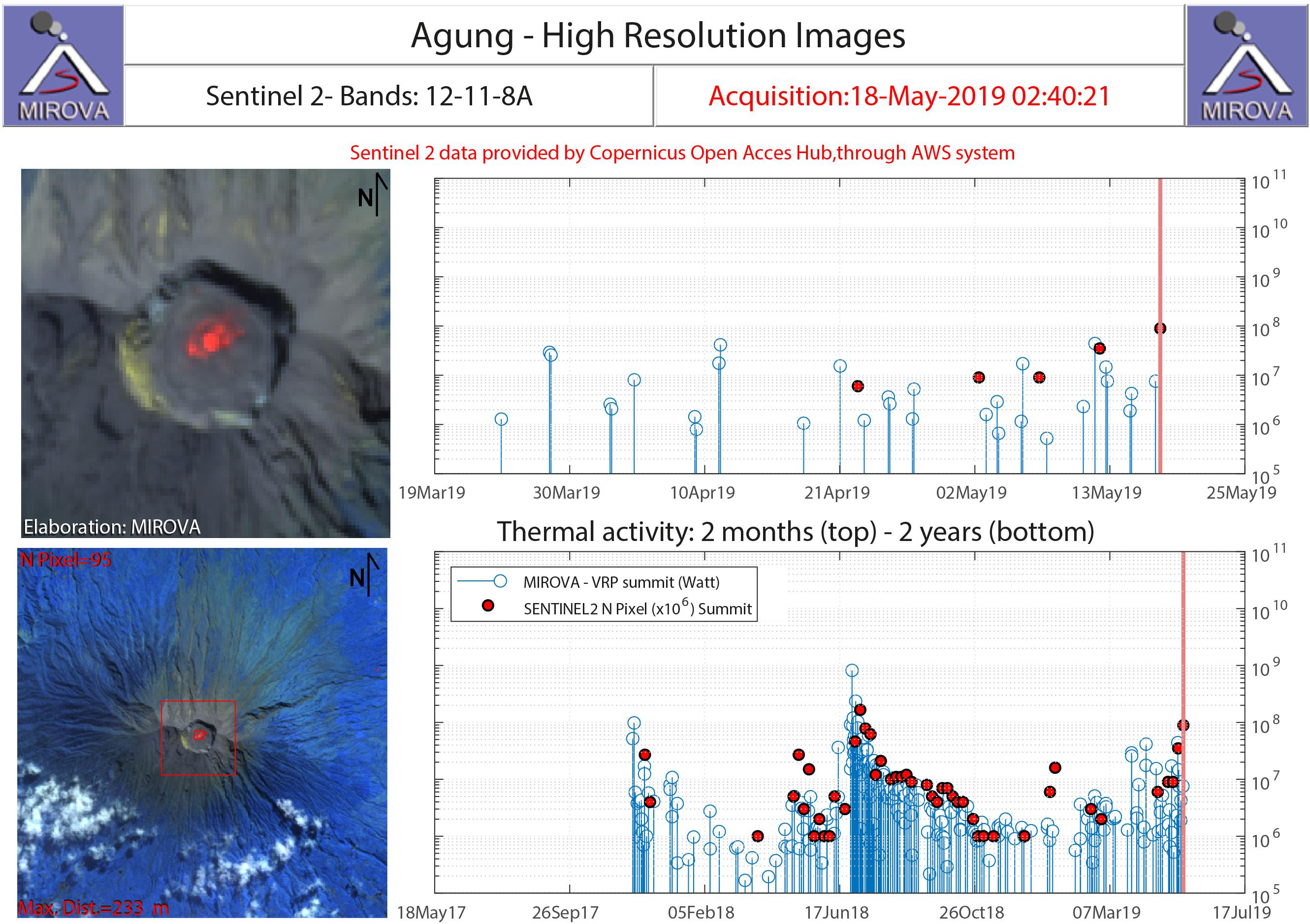
Figure 11. Example of integration of MODIS-MIROVA (1 km) and SENTINEL2 (20 m) thermal data. The panels on the left show the SENTINEL 2 image of 18 May 2019 (combination of bands 12, 11, 8A) acquired over Agung volcano (Indonesia). On the top-left is shown a zoomed view of the crater area (2 km × 2 km) enhancing the presence of a hot lava body. The panels on the right show the MIROVA thermal flow time series (stems) and SENTINEL 2 hot pixel number (circles) of the last 2 months (high) and 2 years (low). The red circles represent the extension of the thermal anomaly within the Agung crater (N Pixel × 106). Note the excellent correlation between the volcanic radiative power, measured by the MODIS, and the extent of the anomaly (number of hot pixels), detected by SENTINEL 2.
Moving toward the TIR-based systems, it has been shown that sensors such as ASTER (Ramsey et al., 2004) and LANDSAT8-TIRS (Blackett, 2014) allow the monitoring of low temperature thermal anomalies, such as fumaroles, hydrothermal systems, temperature of crater lakes, otherwise undetectable via systems using the NIR, SWIR or MIR bands. The integration of these data is certainly an opportunity that has not been currently exploited.
Improve Temporal Resolution of MIR Data
The temporal resolution is also extremely important to promptly detect a thermal anomaly, as well as to follow the course of an eruptive crisis. Increasing the frequency of thermal observations can be achieved through two ways: (i) adding new coherent datasets, from other similar polar sensors, and (ii) processing geostationary data.
The acquisition, processing and integration of thermal data from other sensors having MIR channels, such as the VIIRS or the SENTINEL 3, constitutes a relatively simple step to implement the VRP time series provided by MIROVA. In fact, these sensors have spatial and spectral characteristics very similar to MODIS (Blackett, 2015) that allow the direct application of the algorithms behind MIROVA on the new acquired data. Their processing would therefore allow thermal anomalies to be detected more frequently and with a greater probability of acquisition in optimal conditions. The main obstacle for such implementation remains the quantity of data to be analyzed and the storage capacity of the local server. These problems require the development of a more complex infrastructure than the existing one or, alternatively, the use of cloud computing services such as those provided by Google Earth Engine6 or Amazon Web Services7. However, the products useful for the application of the MIROVA algorithm (geolocated, corrected spectral radiances) are not yet available on these platforms in near real time, making this option currently impossible.
Given the low spatial resolution of geostationary platforms (Supplementary Table S1), the hot spot detection systems based on these sensors are generally not able to detect low intensity thermal precursors. However, once an eruption has begun, it is possible to follow the event with temporal resolutions up to 5–15 min which make them extremely useful during crisis management (i.e., Ganci et al., 2011; Schneider et al., 2014; Gouhier et al., 2016; Lombardo, 2016; Patrick et al., 2016). The analysis of these data at high temporal frequency and their integration with systems based on polar satellites (such as MODIS) is not trivial and requires the processing of data in virtually streaming mode. The recent availability of geolocated and radiometrically pre-processed geostationary image data, provided by systems like EUMETVIEW8 in near real time, is certainly a first fundamental step which needs further efforts and development to integrate these high-frequency datasets into an multiplatform satellite volcanic monitoring systems.
It is hoped that with the continuous technological development, future satellite missions will be specifically dedicated to volcanic monitoring, or at least will be characterized by sensors with appropriate features that would make a breakthrough in volcanic monitoring. In particular, it is absolutely necessary to maintain the continuity of observations in the MIR (such as MODIS and VIIRS), to continue the almost 20-years-long dataset, provided by MIROVA and MODVOLC, possibly improving both spatial and temporal resolution. The presence of SWIR and TIR data, co-registered with MIR and not saturated, would also be a significant improvement for future sensors that would permit the coverage, in a single system, of the wide range of temperature observed on volcanoes. This would allow a detailed characterization of thermal anomalies, potentially useful for real-time determination of the eruption style and composition of terrestrial lavas (Wright et al., 2011).
Data/Image Availability, Archive and Sharing
The availability of data and the possibility of them being searched, displayed and downloaded in real time is another important element to make a volcanic hot spot detection system fully operational. Currently, MIROVA does not allow this possibility, which is compensated by sharing data via email in the case of need. This step requires considerable and continuous efforts by the system developers, but allows the checking of the dataset for the presence of any false alerts, to remove other thermal emitters, such as fires, and to provide support for the interpretation of the data, which in some cases can be ambiguous for non-experts. It can therefore be considered a sort of quality control service of the data that reduces the uncertainty (including cleaning of data sets of unreliable or untrustworthy data points) and any indirect, incidental or consequential damages arising out of any use of, or inability to use, the data.
Nevertheless, the creation of a web-based database for the entire MIROVA archive (time series) remains a task for future development to ensure faster information flow. The development of the apps (for mobiles and desktop pc) is an additional data sharing method, which would allow each individual user to select only the volcanoes of interest observed by MIROVA. Updates could be available through real-time notifications.
The system could be also improved by providing a simple display of daily, monthly and annual values (including cumulative curves) that could be easily accessed by volcano observatories for research/monitoring purposes.
Although the time series plots of VRP and distance are very useful for ongoing situation information, visualization of the image is useful to get the full sense for the spatial pattern of the thermal anomaly, or to verify the presence of clouds or ash plumes above the volcano. MIROVA offers the ability to check the latest 10 images on the web site, but is not possible to go back days, months, years and visualize the archived images, thermal alerts and hotspots. In addition to the time series, a data archive of thermal images, searchable and downloadable on line, is certainly a useful tool for the post-event analysis, but also for real-time assessments. Comparison of older data to the near-real time scenes allows for a quick reality check of the current situation to the last thermal events to see whether this is a repeat of normal behavior of whether this new thermal pattern is anomalous and atypical.
The integration of the thermal dataset with the satellite image viewing capabilities offered by cloud platforms such as WorldView9 could be a particularly interesting solution to greatly simplify the visualization of MODIS images by local systems (such as MIROVA), by maintaining the possibility of linking the VRP time series with an independent image browser. However, building an online archive requires a major upgrade of the system and improved storage capacity (millions of images to manage). MODVOLC (Wright et al., 2004) offers an efficient ability to go back days, months, years and still get a quick plot of the distribution of thermal alerts, but it does not store the images.
True Global Detection Capabilities Keeping High Sensitivity
A final limit that could be overcome is the transformation of a system like MIROVA into a real global volcanic monitoring system. In fact, many observatories have shown interest in extending the satellite observations currently provided by MIROVA to many more volcanoes. However, the current limit of download and storage capacity of the system does not allow for such expansion, making MIROVA a potentially global system only. Hotspot detection systems that are really global, such as MODVOLC or FIRMS, offer effective coverage over the entire earth’s surface, but at the expense of less efficiency in detecting low-intensity thermal anomalies (due to the simplicity of the algorithm). Moreover, they do not allow the display of original images, which we have seen to be fundamental for the correct interpretation of the thermal anomalies in real time. This major limitation, essentially technological, could possibly be overcome by a hardware upgrade of the current system, allowing the inclusion of all the Holocene volcanoes (about 1500) currently present in the Global Volcanism Database. This option would limit the amount of data to be analyzed only to areas of volcanic interest (not to the whole Earth’s surface as it is done for MODVOLC or for FIRMS), retaining the possibility to add, in few hours at most, new targets in areas not yet covered. Alternatively, the development of 5 or 6 local systems (MIROVA clones), hosted by respective volcanological observatories, and focused only on regions (e.g., Asia, Africa, Europe, etc.) or countries can be a technologically easier solution to implement (it simply requires settling MIROVA on different targets in each region), which however involves an adaptation of the system to the specific hardware and networking requirements of each observatory.
Conclusion
Middle Infrared Observations of Volcanic Activity is a system that provides satellite observations, in near real time, regarding the thermal state of more than 200 volcanoes worldwide. The system provides on a specific website10 thermal images and time series of VRP that are examined daily by more than 15 volcanological observatories. This information is being used daily to assess the presence/absence of thermal activity and eventually to locate and quantify these anomalies during phases of unrest or during eruptions. The recognition of particular thermal data trends or patterns, and the integration with other geophysical/geochemical parameters, permits an improved evaluation of the activity of a volcano. In many cases, the thermal data have been fundamental for the detection of the appearance of lava domes at the bottom of deep craters, or to quantify effusion rates and volumes erupted during effusive eruptions. In open vent volcanoes, the thermal data also permit to track the rise of the magmatic column that precedes effusive eruptions, although the detection of thermal precursors before major explosions remain currently a challenge for MIROVA.
All these applications are the result of a long chain of data sharing which, starting from the raw data generated by satellite and delivered by the space agency (NASA) via an open-data policy, finally reaches the observatories, as a useful product for volcanic monitoring.
The current system is, however, limited in a series of aspects that include: (i) the moderate spatial and temporal resolution of the data, (ii) the limited capacity to download and explore the entire archive of time series images, and (iii) the non-global coverage.
Several improvements can be made to partially overcome these limits. However, all these enhancements require human effort, as well as financial and technological resources that can be only partially supported by individual observatories or small research groups. As envisioned by Pritchard et al. (unpublished), the future development of a global volcano remote sensing observatory requires international support at each stage of the processing chain, from the acquisition and elaboration of the data, to their interpretation and dissemination to the volcano observatories or civil protection agencies.
Middle Infrared Observations of Volcanic Activity is a small pilot project, originally created to monitor only a few volcanoes. However, thanks to the sharing of open data and the growing collaboration with volcanological observatories, it has become a useful tool for monitoring hundreds of volcanoes around the world. Thanks to this experience, we are convinced that the role of thermal remote sensing will grow in the coming years, due to the great contribution provided by this open data to the comprehension of volcanic phenomena, and in particular for the hazard evaluation of volcanoes worldwide.
Data Availability Statement
The datasets generated for this study are available on request to the corresponding author.
Author Contributions
DC conceived and wrote the work. DC and ML have developed and analyzed the MIROVA data. FM analyzed the Sentinel2 images. All authors listed have made a substantial, direct and intellectual contribution to the work, and approved it for publication.
Funding
This research has benefited from funding provided by the Italian Presidenza del Consiglio dei Ministri, Dipartimento della Protezione Civile (DPC) as part of the DEVnet Program (2014–2016) that includes a collaborative project between the Earth Sciences departments of the University of Florence and the University of Turin. This research was partially supported by the Italian Ministry for Universities and Research (MIUR).
Conflict of Interest
The authors declare that the research was conducted in the absence of any commercial or financial relationships that could be construed as a potential conflict of interest.
Acknowledgments
We thank the three reviewers for their constructive comments and for the attention paid to revising this work. We acknowledge the LANCE-MODIS system (http://lance-modis.eosdis.nasa.gov/) for providing Level 1B MODIS data in near-real time. We thank many collaborators and students of the University of Turin who have contributed to the development and maintenance of the MIROVA system.
Supplementary Material
The Supplementary Material for this article can be found online at: https://www.frontiersin.org/articles/10.3389/feart.2019.00362/full#supplementary-material
TABLE S1 | Thermal Remote sensing systems available on the web, for operational volcano monitoring.
TABLE S2 | Synthesis of observatories currently using MIROVA in support of monitoring activity. From Global Volcanism Program (2013).
Footnotes
- ^ http://mounts-project.com/
- ^ https://lance-modis.eosdis.nasa.gov/
- ^ www.mirovaweb.it
- ^ http://lgs.geo.unifi.it/
- ^ http://aristotle.ingv.it/tiki-index.php
- ^ https://earthengine.google.com/
- ^ https://aws.amazon.com
- ^ https://eumetview.eumetsat.int/mapviewer/
- ^ https://worldview.earthdata.nasa.gov/
- ^ www.mirovaweb.it
References
Anantrasirichai, N., Biggs, J., Albino, F., Hill, P., and Bull, D. (2018). Application of machine learning to classification of volcanic deformation in routinely generated InSAR data. J. Geophys. Res. Solid Earth 123, 6592–6606.
Barsotti, S., Oddsson, B., Gudmundsson, M. T., Pfeffer, M. A., Parks, M. M., et al. (2019). Operational response and hazards assessment during the 2014–2015 volcanic crisis at Bárðarbunga volcano and associated eruption at Holuhraun, Iceland. J. Volcanol. Geotherm. Res. doi: 10.1016/j.jvolgeores.2019.106753
Bebbington, M. S., and Jenkins, S. F. (2019). Intra-eruption forecasting. Bull. Volcanol. 81:34. doi: 10.1007/s00445-019-1294-9
Bernard, B., Gibson, S., Ramon, P., Gleeson, M., Stock, M., Hernandez, S., et al. (2019). Chronology and phenomenology of the 1982 and 2015 wolf volcano eruptions, Galápagos Archipelago. J. Volcanol. Geotherm. Res. 374, 26–38. doi: 10.1016/j.jvolgeores.2019.02.013
Blackett, M. (2014). Early analysis of landsat-8 thermal infrared sensor imagery of volcanic activity. Remote Sens. 6, 2282–2295. doi: 10.3390/rs6032282
Blackett, M. (2015). An initial comparison of the thermal anomaly detection products of MODIS and VIIRS in their observation of Indonesian volcanic activity. Remote Sens. Environ. 171, 75–82. doi: 10.1016/j.rse.2015.10.002
Bonny, E., and Wright, R. (2017). Predicting the end of lava flow-forming eruptions from space. Bull. Volcanol. 79:52. doi: 10.1007/s00445-017-1134-1138
Bouvet de Maisonneuve, C., Dungan, M. A., Bachmann, O., and Burgisser, A. (2012). Insights into shallow magma storage and crystallization at Volcán Llaima (Andean Southern Volcanic Zone, Chile). J. Volcanol. Geotherm. Res. 211-212, 76–91. doi: 10.1016/j.jvolgeores.2011.09.010
Brown, S. K., Loughlin, S. C., Sparks, R. S. J., Vye-Brown, C., Barclay, J., Calder, E., et al. (2015). “Global volcanic hazard and risk,” in Global Volcanic Hazards and Risk (Cambridge, MA: Cambridge University Press).
Coppola, D., Barsotti, S., Cigolini, C., Laiolo, M., Pfeffer, M. A., and Ripepe, M. (2019a). Monitoring the time-averaged discharge rates, volumes and emplacement style of large lava flows by using MIROVA system: the case of the 2014-2015 eruption at Holuhraun (Iceland). Ann. Geophys. 61:VO221. doi: 10.4401/ag-7812
Coppola, D., Campion, R., Laiolo, M., Cuoco, E., Balagizi, C., Ripepe, M., et al. (2016a). Birth of a lava lake: Nyamulagira volcano 2011-2015. Bull. Volcanol. 78, 1–13. doi: 10.1007/s00445-016-1014-7
Coppola, D., and Cigolini, C. (2013). Thermal regimes and effusive trends at Nyamuragira volcano (DRC) from MODIS infrared data. Bull. Volcanol. 75:744. doi: 10.1007/s00445-013-0744-z
Coppola, D., Laiolo, M., Cigolini, C., Donne, D. D., and Ripepe, M. (2016b). Enhanced volcanic hot-spot detection using MODIS IR data: results from the MIROVA system. Geol. Soc. Lond. Spec. Publ. 426, 181–205. doi: 10.1144/sp426.5
Coppola, D., Laiolo, M., Massimetti, F., and Cigolini, C. (2019b). Monitoring endogenous growth of open-vent volcanoes by balancing thermal and SO2 emissions data derived from space. Sci. Rep. 9:9394. doi: 10.1038/s41598-019-45753-4
Coppola, D., Macedo, O., Ramos, D., Finizola, A., Delle Donne, D., Del Carpio, J., et al. (2015). Magma extrusion during the Ubinas 2013–2014 eruptive crisis based on satellite thermal imaging (MIROVA) and ground-based monitoring. J. Volcanol. Geotherm. Res. 302, 199–210. doi: 10.1016/j.jvolgeores.2015.07.005
Coppola, D., Piscopo, D., Laiolo, M., Cigolini, C., Delle Donne, D., and Ripepe, M. (2013). Radiative heat power at stromboli volcano during 2000–2011: twelve years of MODIS observations. J. Volcanol. Geotherm. Res. 215–216, 48–60. doi: 10.1016/j.jvolgeores.2011.12.001
Coppola, D., Ripepe, M., Laiolo, M., and Cigolini, C. (2017). Modelling satellite-derived magma discharge to explain caldera collapse. Geology 45, 523–526. doi: 10.1130/G38866.1
Davies, D. K., Ilavajhala, S., Wong, M. M., and Justice, C. O. (2009). Fire information for resource management system: archiving and distributing modis active fire data. IEEE Trans. Geosci. Remote Sens. 47, 72–79. doi: 10.1109/tgrs.2008.2002076
Dean, K. G., Jon, D., Kevin, E., Pavel, I., and Ken, P. (2002). “Operational satellite monitoring of volcanoes at the alaska volcano observatory,” in Monitoring Volcanic Hotspots Using Thermal Remote Sensing, Advances in Environmental Monitoring and Modelling, eds A. J. H. Harris, E. Wooster, and D. A. Rothery, (Anchorage: Alaska Volcano Observatory).
Delgado, F., Poland, M., Biggs, J., Ebmeier, S., Sansosti, E., Lundgren, P., et al. (2019). lessons learned from the ceos volcano pilot in latin american and the ongoing volcano demonstrator project. Geophys. Res. Abstr. 21, EGU2019–EGU14981.
Ebmeier, S. K., Andrews, B., Araya, M. C., Arnold, D. W. D., Biggs, J., Cooper, C., et al. (2018). Synthesis of global volcano deformation observations: implications for volcano monitoring and the lateral extent of magmatic systems. J. Appl. Volcanol. 7:2.
Ebmeier, S. K., Elliott, J. R., Nocquet, J.-M., Biggs, J., Mothes, P., Jarriìn, P., et al. (2016). Shallow earthquake inhibits unrest near chiles–cerro negro volcanoes, ecuador–colombian border. Earth Planet Sci. Lett. 450, 283–291. doi: 10.1016/j.epsl.2016.06.046
Elvidge, C. D., Zhizhin, M., Hsu, F., and Baugh, K. E. (2013). VIIRS nightfire: satellite pyrometry at night. Remote Sens. 5, 4423–4449. doi: 10.3390/rs5094423
Fearnley, C. J., McGuire, W. J., Davies, G., and Twigg, J. (2012). Standardisation of the USGS volcano alert level system (VALS): analysis and ramifications. Bull. Volcanol. 74, 2023–2036. doi: 10.1007/s00445-012-0645-6
Franco, L., Palma, J. L., Lara, L. E., Gil-Cruz, F., Cardona, C., Basualto, D., et al. (2019). Eruptive sequence and seismic activity of llaima volcano (Chile) during the 2007–2009 eruptive period: inferences of the magmatic feeding system. J. Volcanol. Geotherm. Res. 379, 90–105. doi: 10.1016/j.jvolgeores.2019.04.014
Furtney, M. A., Pritchard, M. E., Ebmeier, S. K., Jay, J. A., Carn, S. A., McCormick, B. T., et al. (2018). Synthesizing multi-sensor, multi-satellite, multi-decadal data sets for global volcano monitoring. J. Volcanol. Geotherm. Res. 365, 38–56. doi: 10.1016/j.jvolgeores.2018.10.002
Ganci, G., Vicari, A., Fortuna, L., and Del Negro, C. (2011). The HOTSAT volcano monitoring system based on combined use of SEVIRI and MODIS multispectral data. Ann. Geophys. 54, 544–550.
Global Volcanism Program, (2008). “Report on llaima (Chile),” in Bulletin of the Global Volcanism Network, ed. R. Wunderman, (Washington, DC: Smithsonian Institution), BGVN200801–BGVN357110.
Global Volcanism Program, (2013). “East diamante (284201),” in Volcanoes of the World, v. 4.8.0, ed. E. Venzke, (Washington, DC: Smithsonian Institution).
Global Volcanism Program, (2016). “Report on sabancaya (Peru),” in Bulletin of the Global Volcanism Network, 41:5, ed. E. Venzke, (Washington, DC: Smithsonian Institution).
Global Volcanism Program, (2018). “Report on tinakula (Solomon Islands),” in Bulletin of the Global Volcanism Network, 43:2, ed. E. Venzke, (Washington, DC: Smithsonian Institution).
Global Volcanism Program, (2019). “Report on manam (Papua New Guinea),” in Bulletin of the Global Volcanism Network, 44:2, eds A. E. Crafford and E. Venzke, (Washington, DC: Smithsonian Institution).
Gordeev, E. I., Girina, O. A., Lupyan, E. A., Sorokin, A. A., Kramareva, L. S., Efremov, V., et al. (2016). The volsatview information system for monitoring the volcanic activity in Kamchatka and on the Kuril Islands. J. Volcanol. Seismol. 10, 382–394. doi: 10.1134/s074204631606004x
Gottsmann, J., Komorowski, J.-C., and Barclay, J. (2017). “Volcanic unrest and pre-eruptive processes: a hazard and risk perspective,” in Volcanic Unrest: from Science to Society. Advances in Volcanology, (Berlin: Springer).
Gouhier, M., Guéhenneux, Y., Labazuy, P., Cacault, P., Decriem, J., and Rivet, S. (2016). HOTVOLC: a web-based monitoring system for volcanic hot spots. Geol. Soc. Lond. Spec. Publ. 426, 223–241. doi: 10.1144/sp426.31
Harris, A. (2013). Thermal Remote Sensing of Active Volcanoes: A User’s Manual. Cambridge, MA: Cambridge university press.
Harris, A. J. L., Carn, S., Dehn, J., Del Negro, C., Guðmundsson, G., Cordonnier, B., et al. (2016). Conclusion: recommendations and findings of the red seed working group. detecting, modelling and responding to effusive eruptions. Geol. Soc. Lond. Spec. Publ. 426, 567–648. doi: 10.1144/SP426.11
Harris, A. J. L., Chevrel, M. O., Coppola, D., Ramsey, M., Hrysiewicz, A., Thivet, S., et al. (2019). Validation of an integrated satellite-data-driven response to an effusive crisis: the April-May 2018 eruption of Piton de la fournaise. Ann. Geophys. 61:2018. doi: 10.4401/ag-7972
Harris, A. J. L., Villeneuve, N., Di Muro, A., Ferrazzini, V., Peltier, A., Coppola, D., et al. (2017). - Effusive crises at piton de la fournaise 2014-2015: a multi-national response model. J. Appl. Volcanol. 6:11. doi: 10.1186/s13617-017-0062-9
Jay, J. A., Welch, M., Pritchard, M. E., Mares, P. J., Mnich, M. E., Melkonian, A. K., et al. (2013). Volcanic hotspots of the central and southern andes as seen from space by ASTER and MODVOLC between the years 2000-2010, remote sensing of volcanoes and volcanic processes: integrating observation and modelling. Geol. Soc. Lond. Spec. Publ. 380, 161–185. doi: 10.1144/SP380.1
Kaneko, T., Yasuda, A., Aoki, Y., Kajiwara, K., and Kitagawa, S. (2010). Realtime monitoring of active volcanoes in East Asia using MODIS and MTSAT data its advancement by GCOM-C1 SGLI. Int. Arch. Photogramm. Remote Sens. Spatial Inform. Sci. 38, 209–212.
Koeppen, W. C., Pilger, E., and Wright, R. (2011). Time series analysis of infrared satellite data for detecting thermal anomalies: a hybrid approach. Bull. Volcanol. 73, 577–593. doi: 10.1007/s00445-010-0427-y
Laiolo, M., Coppola, D., Barahona, F., Benítez, J. E., Cigolini, C., Escobar, D., et al. (2017). Evidences of volcanic unrest on high-temperature fumaroles by satellite thermal monitoring: the case of Santa Ana volcano, El Salvador. J. Volcanol. Geotherm. Res. 340, 170–179. doi: 10.1016/j.jvolgeores.2017.04.013
Laiolo, M., Massimetti, F., Cigolini, C., Ripepe, M., and Coppola, D. (2018). Long-term eruptive trends from space-based thermal and SO2 emissions: a comparative analysis of Stromboli, Batu Tara and Tinakula volcanoes. Bull. Volcanol. 80, doi: 10.1007/s00445-018-1242-0
Laiolo, M., Ripepe, M., Cigolini, C., Coppola, D., Della Schiava, M., Genco, R., et al. (2019). Space- and ground-based geophysical data tracking of magma migration in shallow feeding system of mount etna volcano. Remote Sens. 11:1182. doi: 10.3390/rs11101182
Linick, J. P., Pieri, D. C., and Sanchez, R. M. (2014). “The JPL ASTER Volcano Archive: the development and capabilities of a 15 year global high resolution archive of volcano data,” in Proceedings of the Abstract ID. GC51E-0482 Presented at Fall Meeting 2014, (San Francisco, CA: American Geophysical Union).
Lombardo, V. (2016). The AVHotRR: near-real time routine for volcano monitoring using IR satellite data. Geol. Soc. 426, 73–92. doi: 10.1144/sp426.18
Lowenstern, J. B., and Ramsey, D. W. (2017). The volcano disaster assistance program—helping to save lives worldwide for more than 30 years. U.S. Geol. Survey Fact Sheet 6, 2017–3071. doi: 10.3133/fs20173071
Marzocchi, W., and Bebbington, M. S. (2012). Probabilistic eruption forecasting at short and long time scales. Bull. Volcanol. 74, 1777–1805. doi: 10.1007/s00445-012-0633-x
Massimetti, F., Coppola, D., Laiolo, M., Cigolini, C., and Ripepe, M. (2018). “First comparative results from SENTINEL-2 and MODIS-MIROVA volcanic thermal dataseries,” in Proceedings of the CoV10 IAVCEI General Assembly, Naples, Italy, 2–7.
Naismith, A., Watson, I., Quinillo, C. C., Chigna, G., Escobar-Wolf, R., Coppola, D., et al. (2019). Eruption frequency patterns through time for the current (1999 – 2018) activity cycle at Volcán de Fuego derived from remote sensing data: evidence for an accelerating cycle of explosive paroxysms and potential implications of eruptive activity. J. Volcanol. Geotherm. Res. 371, 206–219. doi: 10.1016/j.jvolgeores.2019.01.001
Newhall, C. G., Costa, F., Ratdomopurbo, A., Venezky, D. Y., Widiwijayanti, C., Win, N. T. Z., et al. (2017). WOVOdat – an online, growing library of worldwide volcanic unrest. J. Volcanol. nd Geotherm. Res. 345, 184–199. doi: 10.1016/j.jvolgeores.2017.08.003
Pallister, J., and McNutt, S. R. (2015). “Synthesis of volcano monitoring,” in The Encyclopedia of Volcanoes, 2nd Edn, ed. H. Sigurdsson, (Cambridge, MA: Academic Press), 1151–1171. doi: 10.1016/b978-0-12-385938-9.00066-3
Patrick, M., Kauahikaua, J., Orr, T., Davies, A., and Ramsey, M. (2016). “Operational thermal remote sensing and lava flow monitoring at the hawaiian volcano observatory,” in Detecting, Modelling and Responding to Effusive Eruptions, eds A. J. L. Harris, T. De Groeve, F. Garel, and S. A. Carn, (London: Special Publications), 426.
Pergola, N., Coviello, I., Filizzola, C., Lacava, T., Marchese, F., Paciello, R., et al. (2016). A review of RSTVOLC, an original algorithm for automatic detection and near-real-time monitoring of volcanic hotspots from space. Geol. Soc. Lond. Spec. Publ. 426:55. doi: 10.1144/SP426.1
Phillipson, G., Sobradelo, R., and Gottsmann, J. (2013). Global volcanic unrest in the 21st century: an analysis of the first decade. J. Volcanol. Geotherm. Res. 264, 183–196. doi: 10.1016/j.jvolgeores.2013.08.004
Piscini, A., and Lombardo, V. (2014). Volcanic hot spot detection from optical multispectral remote sensing data using artificial neural networks. Geophys. J. Int. 196, 1525–1535. doi: 10.1093/gji/ggt506
Pritchard, M. E., Biggs, J., Wauthier, C., Sansosti, E., Arnold, W. D., Delgado, F., et al. (2018). Towards coordinated regional multi-satellite InSAR volcano observations: results from the latin America pilot project. J. Appl. Volcanol. 7:5. doi: 10.1186/s13617-018-0074-70
Ramsey, M., Flynn, L., and Wright, R. (2004). Volcanic observations from space: new results from the eos satellite instruments. J. Volcanol. Geotherm. Res. 135, 1–219.
Ramsey, M. S. (2016). Synergistic use of satellite thermal detection and science: a decadal perspective using ASTER. Geol. Soc. Lond. Spec. Publ. 426, 115–136. doi: 10.1144/sp426.23
Ramsey, M. S., and Harris, A. J. L. (2013). Volcanology 2020: how will thermal remote sensing of volcanic surface activity evolve over the next decade? (invited review article). J. Volcanol. Geotherm. Res. 249, 217–233. doi: 10.1016/j.jvolgeores.2012.05.011
Reath, K., Pritchard, M., Moruzzi, S., Alcott, A., Coppola, D., and Pieri, D. (2019a). The. (AVTOD)(ASTER Volcano Thermal Output Database) latin america archive. J. Volcanol. Geotherm. Res. 376, 62–76. doi: 10.1016/j.jvolgeores.2019.03.019
Reath, K., Pritchard, M., Poland, M., Delgado, F., Carn, S., Coppola, D., et al. (2019b). Thermal, deformation, and degassing remote sensing time series (A.D. 2000-2017) at the 47 most active volcanoes in Latin America: implications for volcanic systems. J. Geophys. Res. Solid Earth 124, 195–218. doi: 10.1029/2018JB016199
Reath, K. A., Ramsey, M. S., Dehn, J., and Webley, P. W. (2016). Predicting eruptions from precursory activity using remote sensing data hybridization. J. Volcanol. Geotherm. Res. 321, 18–30. doi: 10.1016/j.jvolgeores.2016.04.027
Ripepe, M., Pistolesi, M., Coppola, D., Delle Donne, D., Genco, R., Lacanna, G., et al. (2017). Forecasting effusive dynamic and decompression rates by magmastatic model at open-vent volcanoes. Sci. Rep. 7:3885. doi: 10.1038/s41598-017-03833-3
Schneider, D. J., Randall, M., and Parker, T. (2014). “Volcview: a web-based platform for satellite monitoring of volcanic activity and eruption response,” in Proceedings of the Abstract ID IN41D-05 Presented at Fall Meeting 2014, (San Francisco, CA: American Geophysical Union).
Sparks, R. S. J., Biggs, J., and Neuberg, J. W. (2012). Monitoring volcanoes. Science 335, 1310–1311.
Steffke, A. M., and Harris, A. J. L. (2011). A review of algorithms for detecting volcanic hot spots in satellite infrared data. Bull. Volcanol. 73, 1109–1137. doi: 10.1007/s00445-011-0487-487
Tait, S., and Ferrucci, F. (2013). “A real-time, space borne volcano observatory to support decision making during eruptive crises: european volcano observatory space services,” in proceedings of the 2013 UKSim 15th International Conference on Computer Modelling and Simulation, (Washington, DC: IEEE Computer Society).
Tarquini, S., de’ Micheli Vitturi, M., Jensen, E., Pedersen, G. M. B., Barsotti, S., Coppola, D., et al. (2019). Modeling lava flow propagation over a flat landscape by using MrLavaLoba: the case of the 2014-2015 eruption at holuhraun, Iceland. Ann. Geophys. 62:228. doi: 10.4401/ag-7812
Tilling, R. I. (2008). The critical role of volcano monitoring in risk reduction. Adv. Geosci. 14, 3–11. doi: 10.5194/adgeo-14-3-2008
Urai, M. (2011). “Volcano observations with aster and ASTER Image Database for Volcanoes,” in Proceedings of the IEEE International Geoscience and Remote Sensing Symposium, (Piscataway, NJ: IEEE).
Valade, S., Lacanna, G., Coppola, D., Laiolo, M., Pistolesi, M., Delle Donne, D., et al. (2016). Tracking dynamics of magma migration in open-conduit systems. Bull. Volcanol. 78:78.
Valade, S., Ley, A., Massimetti, F., D’Hondt, O., Laiolo, M., Coppola, D., et al. (2019). Towards global volcano monitoring using multisensor sentinel missions and artificial intelligence: the MOUNTS monitoring system. Remote Sens. 11:528.
van Manen, S. M., Blake, S., Dehn, J., and Valcic, L. (2013). Forecasting large explosions at Bezymianny Volcano using thermal satellite data. Geol. Soc. Lond. Spec. Publ. 380, 187–201. doi: 10.1144/sp380.3
van Manen, S. M., Dehn, J., and Blake, S. (2010). Satellite thermal observations of the Bezymianny lava dome 1993–2008: precursory activity, large explosions, and dome growth. J. Geophys. Res. 115:B08205.
Wadge, G. (1981). The variation of magma discharge during basaltic eruptions. J. Volcanol. Geotherm. Res. 11, 139–168. doi: 10.1016/0377-0273(81)90020-2
Werner, C., Kern, C., Coppola, D., Lyons, J., Kelly, P., Wallace, K., et al. (2017). Magmatic degassing, lava dome extrusion, and explosions from mount cleveland volcano, alaska, 2011-2015: insight into the continuous nature of volcanic activity over multi-year timescales. J. Volcanol. Geotherm. Res. 337, 98–110. doi: 10.1016/j.jvolgeores.2017.03.001
Winson, A. E. G., Costa, F., Newhall, C. G., and Woo, G. (2014). An analysis of the issuance of volcanic alert levels during volcanic crises. J. Appl. Volcanol. 3:14. doi: 10.1186/s13617-014-0014-6
Wooster, M. J., Zhukov, B., and Oertel, D. (2003). Fire radiative energy for quantitative study of biomass burning: derivation from the BIRD experimental satellite and comparison to modis fire products. Remote Sens. Environ. 86, 83–107. doi: 10.1016/s0034-4257(03)00070-1
Wright, R., Flynn, L. P., Garbeil, H., Harris, A. J. L., and Pilger, E. (2004). MODVOLC: near-real-time thermal monitoring of global volcanism. J. Volc. Geotherm. Res. 135, 29–49. doi: 10.1016/j.jvolgeores.2003.12.008
Wright, R., Glaze, L., and Baloga, S. M. (2011). Constraints on determining the eruption style and composition of terrestrial lavas from space. Geology 39, 1127–1130. doi: 10.1130/g32341.1
Keywords: thermal remote sensing, global volcano monitoring, MIROVA, MODIS, thermal unrest, eruption forecasting
Citation: Coppola D, Laiolo M, Cigolini C, Massimetti F, Delle Donne D, Ripepe M, Arias H, Barsotti S, Parra CB, Centeno RG, Cevuard S, Chigna G, Chun C, Garaebiti E, Gonzales D, Griswold J, Juarez J, Lara LE, López CM, Macedo O, Mahinda C, Ogburn S, Prambada O, Ramon P, Ramos D, Peltier A, Saunders S, de Zeeuw-van Dalfsen E, Varley N and William R (2020) Thermal Remote Sensing for Global Volcano Monitoring: Experiences From the MIROVA System. Front. Earth Sci. 7:362. doi: 10.3389/feart.2019.00362
Received: 17 September 2019; Accepted: 30 December 2019;
Published: 27 January 2020.
Edited by:
Fidel Costa, Nanyang Technological University, SingaporeReviewed by:
Társilo Girona, NASA Jet Propulsion Laboratory (JPL), United StatesYosuke Aoki, The University of Tokyo, Japan
Christina Widiwijayanti, Earth Observatory of Singapore, Singapore
Copyright © 2020 Coppola, Laiolo, Cigolini, Massimetti, Delle Donne, Ripepe, Arias, Barsotti, Parra, Centeno, Cevuard, Chigna, Chun, Garaebiti, Gonzales, Griswold, Juarez, Lara, López, Macedo, Mahinda, Ogburn, Prambada, Ramon, Ramos, Peltier, Saunders, de Zeeuw-van Dalfsen, Varley and William. This is an open-access article distributed under the terms of the Creative Commons Attribution License (CC BY). The use, distribution or reproduction in other forums is permitted, provided the original author(s) and the copyright owner(s) are credited and that the original publication in this journal is cited, in accordance with accepted academic practice. No use, distribution or reproduction is permitted which does not comply with these terms.
*Correspondence: Diego Coppola, ZGllZ28uY29wcG9sYUB1bml0by5pdA==