- 1Global Geophysics Research Group, Faculty of Mining and Petroleum Engineering, Bandung Institute of Technology, Bandung, Indonesia
- 2Research School of Earth Sciences, Australian National University, Canberra, ACT, Australia
- 3Center for Volcanology and Geological Hazards Mitigation, Geological Agency, Ministry of Energy and Mineral Resources, Bandung, Indonesia
- 4Volcanology and Geothermal Laboratory, Faculty of Mining and Petroleum Engineering, Bandung Institute of Technology, Bandung, Indonesia
The Agung-Batur Volcanic Complex (ABVC), part of the Sunda volcanic arc, is the source of some of the most hazardous volcanic activity in Indonesia. The ABVC has undergone many small (VEI 1-2) eruptions since historical records began in the early 19th century, but Mt. Agung has experienced much larger (VEI 5) eruptions, both in the modern (1963) and historical (1843) eras, as well as several times during the past 2000–3000 years. The 1963 eruption caused more than 1000 deaths, and a more recent eruption in 2017 caused the evacuation of 140,000 people. Delineating the magma structure beneath ABVC is an important first step in understanding the physics of these eruptions. This paper presents the first local-scale study of Rayleigh wave group velocity structure and the seismic velocity structure beneath the ABVC using ambient seismic noise tomography. Seismic data were collected using 25 seismometers deployed across the ABVC during early January to March 2019. The local seismic network provides good resolution beneath both Mt. Agung and Mt. Batur. We obtained 158 Rayleigh Green’s Functions, extracted by cross correlating noise simultaneously recorded at available station pairs. We used sub-space inversion to calculate group velocity at different periods and to estimate the lateral variations in group velocity for given periods. 2-D tomographic maps obtained from the inversion of the group velocity of Rayleigh waves clearly showed some pronounced velocity anomalies beneath the ABVC. We applied the Neighbourhood Algorithm (NA) technique to invert the Rayleigh wave dispersion curves to obtain shear wave velocity (Vs) vs. depth profiles. These profiles indicate a low Vs of about 1 km/s underlying the volcanic complex between Mt. Agung and Mt. Batur at depths up to 2 km, which we suggest is due to a combination of low-Vs volcanic deposits as well as a shallow hydrothermal fluids system associated with magma fluids and/or gases produced by magma intrusion at depths >7 km.
Introduction
Knowledge of the structure and dimensions of a volcano’s plumbing system is important for understanding its eruptive behavior (Pinel and Jaupart, 2003). Local-scale seismic arrival time tomography is an effective way to obtain such knowledge, as shown by Monteiller et al. (2005) and Widiyantoro et al. (2018) for Kilauea, Hawaii and Merapi, Indonesia, respectively. Seismic ambient noise tomography (ANT) (see, e.g., Shapiro and Campillo, 2004; Shapiro et al., 2005) has also been successfully applied to obtain the 3D upper crustal structure in many volcanic regions around the world, such as Toba in Sumatra, Indonesia (Stankiewicz et al., 2010), Asama in Japan (Nagaoka et al., 2012) and Colima in Mexico (Escudero and Bandy, 2017). This study aims to use ANT to delineate upper crustal structure beneath Agung Batur Volcanic Complex (ABVC), Bali, Indonesia using a local seismic network.
Understanding the eruptive behavior of the ABVC is important because of the threat it poses to populations in Bali and beyond. The ABVC has experienced at least 29 historic eruptions since the early 19th century (Global Volcanism Program, 2013). Almost all of the eruptions have Volcanic Explosivity Index (VEI) 1–2. The VEI 5 eruption of Mt. Agung in 1963, however, is regarded as one of the most significant eruptions in the 20th century, having a global climate impact and causing over 1000 fatalities, making it one of Indonesia’s most deadly eruptions (Self and Rampino, 2012). Fontijn et al. (2015) used tephrostratigraphic analysis to show that the 1843 eruption of Mt. Agung, as well as at least 4 other events 2000–3000 BP, were likely as big as the 1963 eruption. The composition of eruptive products ranges is from basaltic andesite to andesite. The latter characterized the large 1963 and 1843 eruptions.
Since December 2018, a temporary seismographic network has been deployed around the ABVC that we refer to as the Agung Seismic Experiment. The Agung Seismic Experiment aims to monitor the heightened seismicity around the ABVC after the eruption in November 2017, and to use ANT to determine seismic velocity structure that can improve our understanding of the ABVC’s magmatic plumbing and hydrothermal systems.
Geological Setting and Recent Eruption Record
Bali, an island in the Indonesian archipelago, is part of the Sunda arc (Figure 1A), which stretches from Sumatra to Nusa Tenggara through Bali. The Agung Batur Volcanic Complex lies above the subduction zone where the Indo-Australian plate dives below the Sunda block, resulting in the production of melt that drives volcanic activity. Chaussard and Amelung (2012) conducted deformation analyses of active volcanoes in the west Sunda arc using 2006–2009 Alos InSAR time series, at Mt. Sinabung, Mt. Kerinci, Mt. Slamet, Mt. Lawu, Mt. Lamongan, Mt. Agung, and Mt. Anak Krakatau. The evidence of inflation can be seen in this study, which showed that these volcanoes have shallow magma reservoirs at depths 1 – 3 km, and significant vertical deformation rates of 3–8 cm/yr were detected, indicating in particular inflation of Mt Agung during 2006–2009.
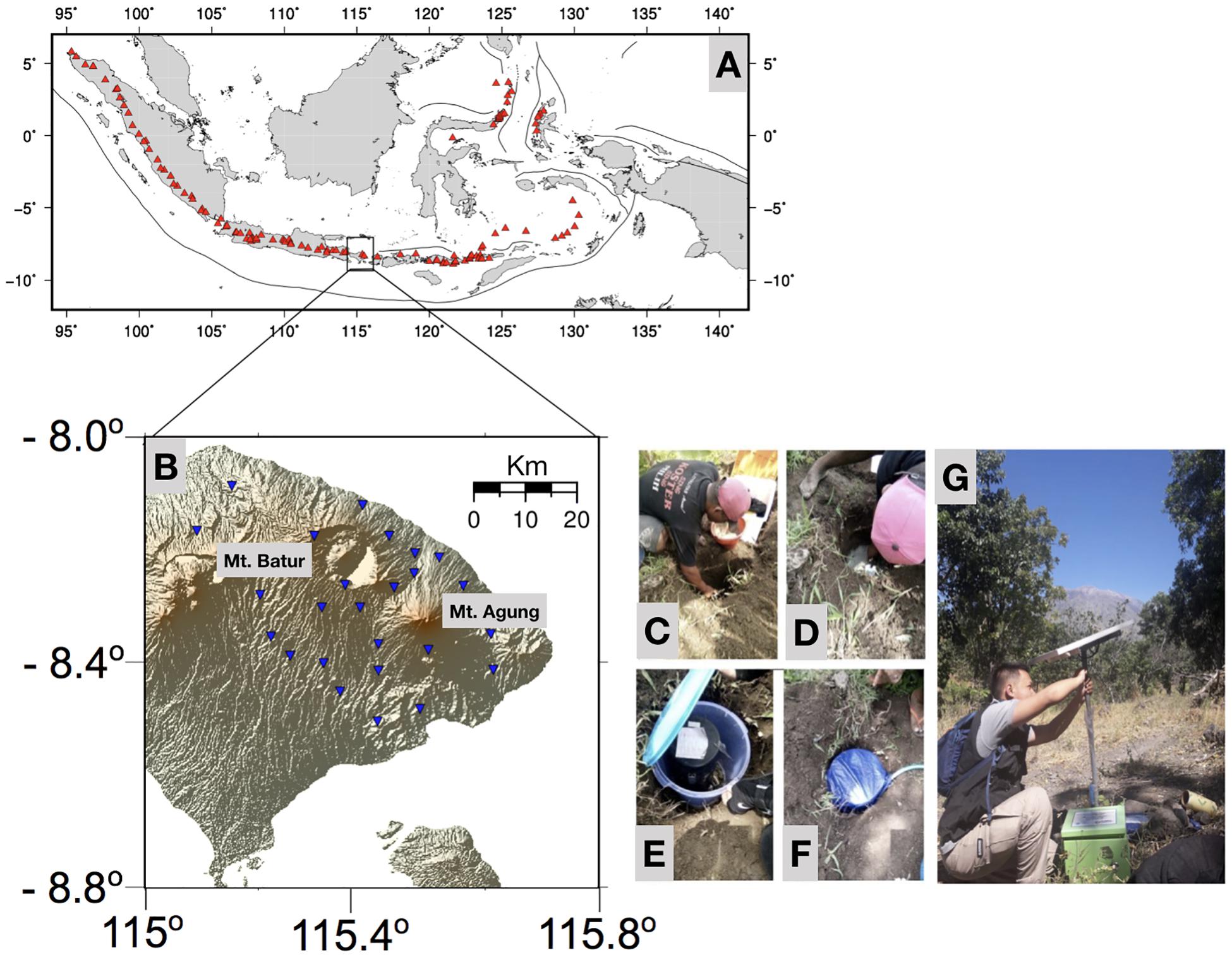
Figure 1. (A) Map of Indonesia with the 127 active volcanoes marked by red triangles, and the black square identifying the study area. (B) Seismic station deployed across Mt. Batur and Mt. Agung, Bali, Indonesia marked by the inverted blue triangles. (C–G) some photographs of instrument installation.
The Agung Batur Volcanic Complex is a stratovolcano formed by volcanic deposits left by past eruptions that produced andesite lava, volcanic breccia, volcanic ash, and pyroclastic debris. Previous studies have inferred the geological structure of the volcanic layers and depths of magma chambers beneath the ABVC. Geiger et al. (2018) described a multi-level magma plumbing based on mineral-melt equilibrium thermobarometry of lavas produced by the 1963 eruption of Agung and eruptions of Batur in 1963 and 1974. They established that, for both Agung and Batur, magmas were sourced from both an upper crustal reservoir at 3–5 km depth and a lower crustal reservoir near the Moho at about 20 km depth (just above the Moho at 18 km depth for Batur, and right at the Moho for Agung). Using satellite InSAR estimates of surface deformation and 3D numerical modeling, Albino et al. (2019) suggested that a sub-vertical magma intrusion midway between Agung and Batur, at 7–13 km depth, might play an important role in connecting these magmatic systems.
Zen and Hadikusumo (1964) showed that the 1963 Mt. Agung eruption had a major impact on residential and agricultural areas in Bali. Monitoring of volcanic activity in Mt. Agung at that time did not use the type of sophisticated instrumentation that would be used today, so no one knows in detail the changes in seismic activity, inflation and heat flow. The increased volcanic activity at that time was known only through the tremors that were felt by people living within 6 km from the peak of Mt. Agung. A gust of dust billowed and formed a column of hot clouds from March 17 to May 16 at an altitude of >20 km above the crater peak (Self and Rampino, 2012). About 7 days after the earthquake tremors commenced, a thick lava flow emerged from the crater and flowed northward. Increased activity continued until mid-March 1963 (Surjo, 1981).
Self and Rampino (2012) explain the chronology of the eruption of Mt. Agung in 1963–1964 as a continuous eruption. The lava flow produced in the eruption of Mt. Agung has some unusual attributes. The total volume of basaltic andesite material erupted is estimated to be <0.5 km3 (0.1 km3 lava; <0.4 km3 pyroclastic fall deposits; 0.05 km3 pyroclastic flow deposits – all dense rock equivalent volumes).
The Mt. Agung crisis in 2017 began with an increase in earthquakes swarm in mid-May 2017, this time recorded by the PVMBG seismometer network (Syahbana et al., 2019). On September 22, 2017 there was an increase in seismicity around Mt. Agung with the number of detected earthquakes reaching more than 800. On the same date, PVMBG gave a warning about the danger of eruption of Mt. Agung and raised the alert to its highest level, 4 (“Awas”). The announced danger zone was within 9–12 km from the top of the crater and about 140,000 people evacuated, including about 70,000 who self-evacuated from outside the exclusion zone (Syahbana et al., 2019). On November 21 2017, a phreatic eruption produced a hot cloud that rose up to 700 m above the crater. This condition resulted in major disruption of flight schedules in Bali.
Syahbana et al. (2019) chronology of the 2017 eruption includes analysis of seismic activity and geodetic measurements that indicate a cluster of earthquakes as well as inflation centered in an area between Mt. Agung and Batur Cladera. From this evidence Syahbana et al. (2019) inferred that magma intruded into a mid-crustal dike between Mount Agung and Batur Caldera, and that this initiated the earthquake swarm in late September.
Materials and Methods
Seismic Data Acquisition
The temporary local seismograph network at ABVC was installed in mid-December 2018 and has been recording up to August, 2019. We deployed 25 seismographs, covering the area around the ABVC (Figure 1) with minimum and maximum inter-station distances of 5 km and 7 km, respectively. There are 300 potential station pairs for cross correlation. We deployed 3 Geobit, 2 Guralp, and 18 Nanometrics Compact Trillium broadband seismometers, and 2 Lennartz short-period seismometers (Supplementary Table S1). The 3 Geobit sensors are in boreholes and the remaining sensors are surface deployments. Geobit and Guralp instruments are integrated sensor-recorder packages, while data from the remaining sensors are recorded by low-power dataloggers manufactured by the Australian National University. All waveform data are recorded with a 100 Hz sampling rate. We dug pits to keep the seismometer under the ground surface for thermal stability and use solar panels to recharge the battery voltage so that the device can record continuously. A photograph of one of these seismic recorders is shown in Figures 1C–G.
The Geobit and Nanometrics Trillium seismometers are broadband sensors, both with response flat to velocity in the period range 0.1–20 s. The response of the Lennartz short-period sensor, on the other hand, is flat only over the range 0.03–1 s. The manufacturer of the Lennartz claims that the instrument response is sufficiently precise to be reliably deconvolved to periods as long as 20 s (Lennartz Electronic, 2017), longer than the maximum period used here. An example of a cross correlogram between short-period seismogram and broad-band seismogram is shown in Supplementary Figure S1. If some correlogams using data from the Lennartz seismometers are contaminated with instrument noise for the longer periods used in our study (up to 12 s), we expect they will be rejected when we screen the correlograms to keep only those with high signal-to-noise ratio (see Section “Ambient Seismic Noise Cross Correlation”).
Although each station records 3-components of motion – vertical, North and East – for the analysis discussed here we utilized only the vertical component data. Because several different instrument types were used in the Agung Seismic Experiment, we first corrected the instrument response before calculating cross correlograms between each station pair to be used in our ANT analysis. We used a pole-and-zero representation of the frequency response of each different instrument to obtain the corresponding displacement.
Ambient Seismic Noise Cross Correlation
Ambient noise cross correlation was introduced by Shapiro et al. (2005) as a way of extracting from seismic “background” noise signals representing the elastic impulse response of the subsurface. This technique is now widely used to image subsurface structure using group velocity inversion. ANT used to delineate the subsurface structure in Australia (Saygin and Kennett, 2010, 2012), Netherlands (Yudistira et al., 2017). Furthermore, in Indonesian region the ANT used to understand upper crustal structure beneath central Java (Zulfakriza et al., 2014), Jakarta the capital city of Indonesia (Saygin et al., 2016), eastern Java (Martha et al., 2017), western Java (Rosalia et al., 2020), and Bandung Basin (Pranata et al., 2019). On the other hand, ANT also used to delineate structures within volcano bodies, such as Lake Toba, Indonesia (Stankiewicz et al., 2010), Mt. Asama in Japan (Nagaoka et al., 2012) and Colima volcano complex, Mexico (Escudero and Bandy, 2017). In this study, we applied the ANT technique to delineate the Rayleigh wave group velocity structure of the ABVC.
Cross-correlating the ambient seismic noise recorded simultaneously at a pair of stations over many days (∼60 days in our study) makes use of the coherent noise produced by the interaction of the ground surface with the atmosphere and ocean wave activity, to produce a signal that approximates the impulse response of the medium, or Green’s Function, between the two stations (Bensen et al., 2007). This estimate of the Green’s Function obtained from cross correlation of interstation noise is referred to here as the Time Domain Empirical Green’s Function, or TDEGF (Snieder, 2004). When the vertical component of motion at the two sites is cross correlated, the resulting TDEGF can be used to measure Rayleigh wave group velocities at different periods (Shapiro and Campillo, 2004; Sabra et al., 2005; Shapiro et al., 2005).
The procedure used to estimate Rayleigh-wave TDEGFs follows the steps carried out by Saygin and Kennett (2010). The cross correlation between two stations A and B can be written as follows,
Where V(XA, t) and V(XB, t) are signals recorded continuously by two different stations at positions XA and XB, respectively.
We used 60 days of observed data and divided each into several segments. Each segment has a duration of 3,600 s with overlaps of 1,200 s for each segment. After that, we stacked the result to get an estimate of the TDEGF for each day of observation, so that 1-day TDEGFs were finally obtained for all observation days, and these were finally stacked to get a single TDEGF for each interstation pair.
Although our dataset was of only 60 days duration, we found that a collection of TDEGFs associated with a particular station, when sorted according to inter-station distance, displays a clearly identifiable arrival of energy with an average moveout of about 2 km/s, which we assume to be the fundamental-mode Rayleigh wave (Figure 2A). Following Saygin and Kennett (2010) and Zulfakriza et al. (2014), for each correlogram we selected a window that encompassed this energy, while rejecting correlograms that were too noisy to unambiguously identify the onset and duration of the Rayleigh wave. We note that more than half (158) of the 300 available correlograms were rejected as too noisy, including some with data at long period from the short-period Lennartz seismometers. A narrow-band Gaussian is applied to each these TDEGFs, and group velocity arrivals for each period are manually picked from time-frequency plots over periods of 0.5–15 s, as indicated with white dots in Figures 2B,C.
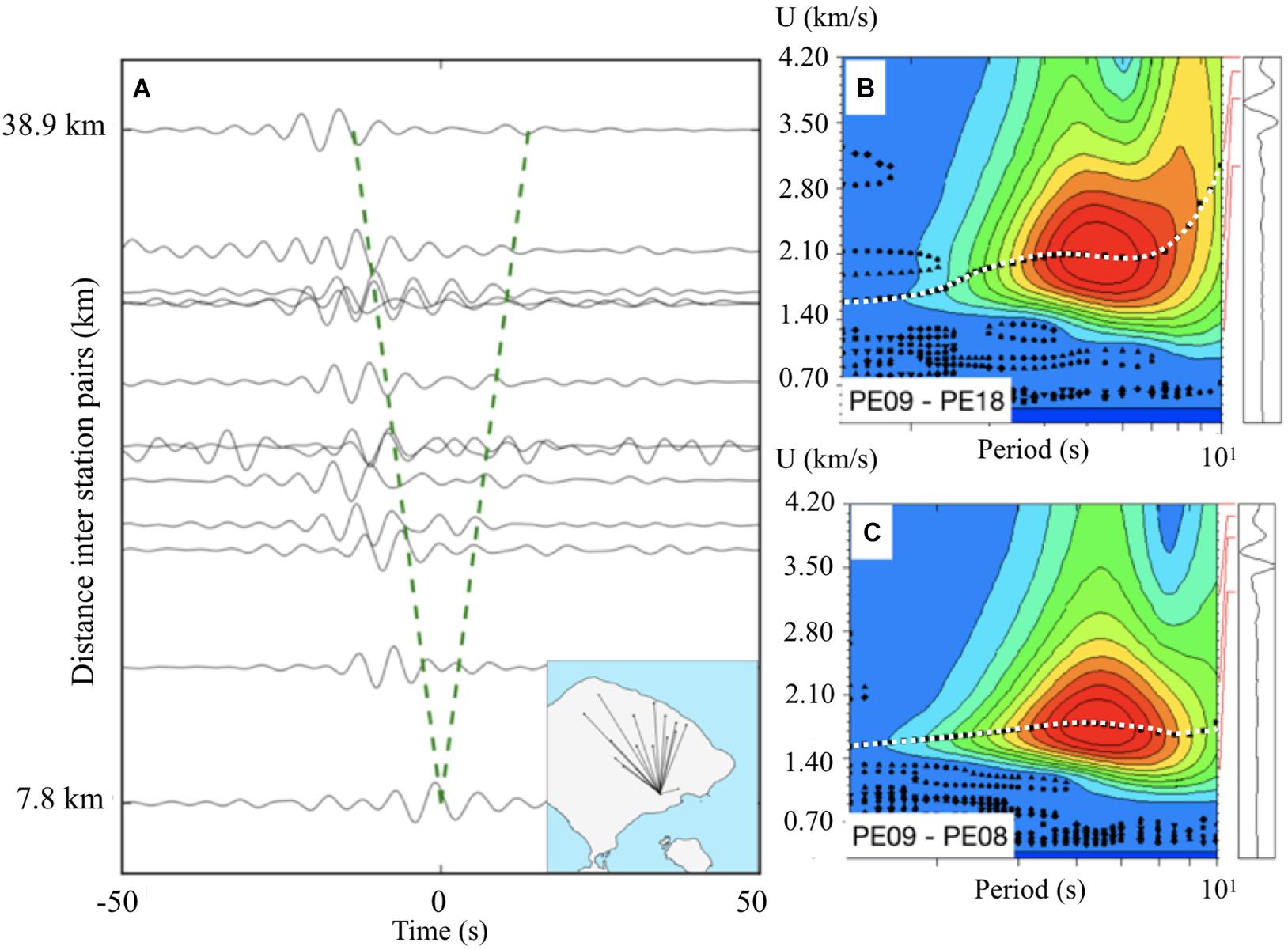
Figure 2. (A) Rayleigh wave Green’s functions estimated for the path between station PE09 other stations (see insert map). Waveforms were filtered between 0.05 and 0.2 Hz, and the time lag of cross-correlation ranges from –50 to 50s, with the dash green line indicating the approximate group velocity of 2.2 km/s. (B,C) Example time-frequency plots and extracted dispersion curves between PE09-PE18 and PE09-PE08, where red colors indicate larger amplitudes, with white dots indicating value of dispersion curve picked.
Tomographic Inversion
Sub-Space Inversion of Group Velocity
The TDEGF for each station pair can provide measurements of group velocity for different periods by applying the Multiple Filter Technique (MFT), popularized by Dziewonski et al. (1969). This technique has been widely applied in previous studies such as Saygin and Kennett (2010), Zulfakriza et al. (2014), and Martha et al. (2017). In this way group velocity dispersion curves are obtained for each interstation pair in the network of seismographs, giving rise to a web of intersecting inter-station paths along which dispersion is measured. Tomographic techniques can then be applied to provide an image of group velocity for select periods. In many cases these maps of group velocity anomalies can be directly interpreted in terms of low-velocity sedimentary basins or high-velocity metamorphic rock formations.
In this study, we used the surface wave tomography approach developed by Rawlinson (2005), which uses a subspace inversion method to obtain the optimal group velocity map along with the Fast Marching Method (FMM) to trace surface wave raypaths in heterogeneous media. Subspace inversion is an iterative method in which an objective functional is minimized by using successive quadratic approximations of the function in an n-dimensional subspace (Kennett et al., 1988). Once the minimum of the quadratic approximation is found in the model subspace, a new quadratic approximation is made, either in the same or a different model subspace, and the process repeated. At each step the rays are re-traced using FMM, so the non-linear relationship between velocity and travel time is accounted for. The method has been successfully applied to obtain the group velocity structure in various regional studies (Saygin and Kennett, 2012; Martha et al., 2017). According to Rawlinson et al. (2010) the incorporation of FMM and subspace inversion can provide stable inversion results even for strongly heterogeneous media.
The inversion problem is solved by optimization the objective function S (m), which includes misfit, damping and smoothing terms as indicated in equation (2) below.
where m is a vector of model parameters (i.e., the group velocity map), g(m) are the predicted group traveltimes from the model, d are the observed group traveltimes, Ce is the data error covariance matrix, m0 is the reference model, Cm is the model parameter covariance matrix, D is a flatness/smoothness matrix, ε is the damping parameter, and η is the smoothing parameter.
Shear Wave Velocity Inversion
There are several ways to estimate velocity-depth profiles from surface wave dispersion curves. Shapiro et al. (2000) estimated the model uncertainty using a Monte Carlo scheme, which performs non-linear inversion of dispersion curves using a random search of the model space. Herrmann (2013) used a linearized method to find the stack of horizontally homogeneous layers that best fit dispersion curve. Throughout the inversion, the thickness and Vp/Vs for each layer remained fixed, and the density was estimated from the Vp.
We applied the scheme of neighborhood algorithm introduced by Sambridge (1999a, b) to address the inversion problem. This algorithm uses a random search that exploits information accumulated over the history of the model space search to adapt the sampling, concentrating it in parts of the model space surrounding the lower misfit models. We used the AK135 model developed by Kennett et al. (1995) as an initial upper crustal mode; over depths 500 – 15000 m, with maximum Vp 5.8 km/s, and maximum Vs 3.46 km/s.
Results
Here we consider the application of tomographic inversion to the fundamental-mode Rayleigh wave TDEGFs estimated from inter-station cross correlograms of ambient seismic noise on the ABVC, as well as Neighbourhood Algorithm (NA) inversion of the resulting dispersion curves. We first consider an analysis of the lateral resolution achievable with our experimental configuration, followed by a discussion of the images of Rayleigh wave group velocity obtained by tomographic inversion of the observed travel times at each period. Finally, we apply NA inversion to the dispersion curves obtained from the tomographic images, to obtain 1D Vs to depth profiles at each point of a regular grid covering the study area, and discuss the implications for 3D Vs structure of the ABVC.
Checkerboard Resolution Test
We conducted tests using “checkerboard” group velocity patterns of alternating low- and high-velocity cells to generate synthetic Rayleigh wave travel times for the inter-station paths of our dataset, which we then invert to assess how well the original velocity pattern is recovered. From such tests we determine the optimum parameterization and achievable resolution of the inversion. In this paper, the checkerboard resolution tests were used with noiseless data.
We conducted three checkerboard resolution tests, having cells of dimension ∼5 km × 5 km (0.05° × 0.05°), ∼10 km × 10 km (0.1° × 0.1°), and ∼15 km × 15 km (0.15° × 0.15°), as shown in Figures 3A–C. The results of tomographic inversion obtained with the same configuration of station pairs as that of the observed data are shown in Figures 3D–F. These indicate that, for cell size 0.1° × 0.1° and greater, the original checkerboard pattern is recovered well within the area covered by inter-station paths, particularly between Mt. Agung and Mt. Batur. “Smeared” patterns are only seen at the edges where there is poor raypath coverage, such as the eastern part of Mt. Agung where the original pattern is not recovered well. The extent to which the original pattern is recovered depends on the ray-path coverage for each period, which is shown in the insets of Figures 4A–F.
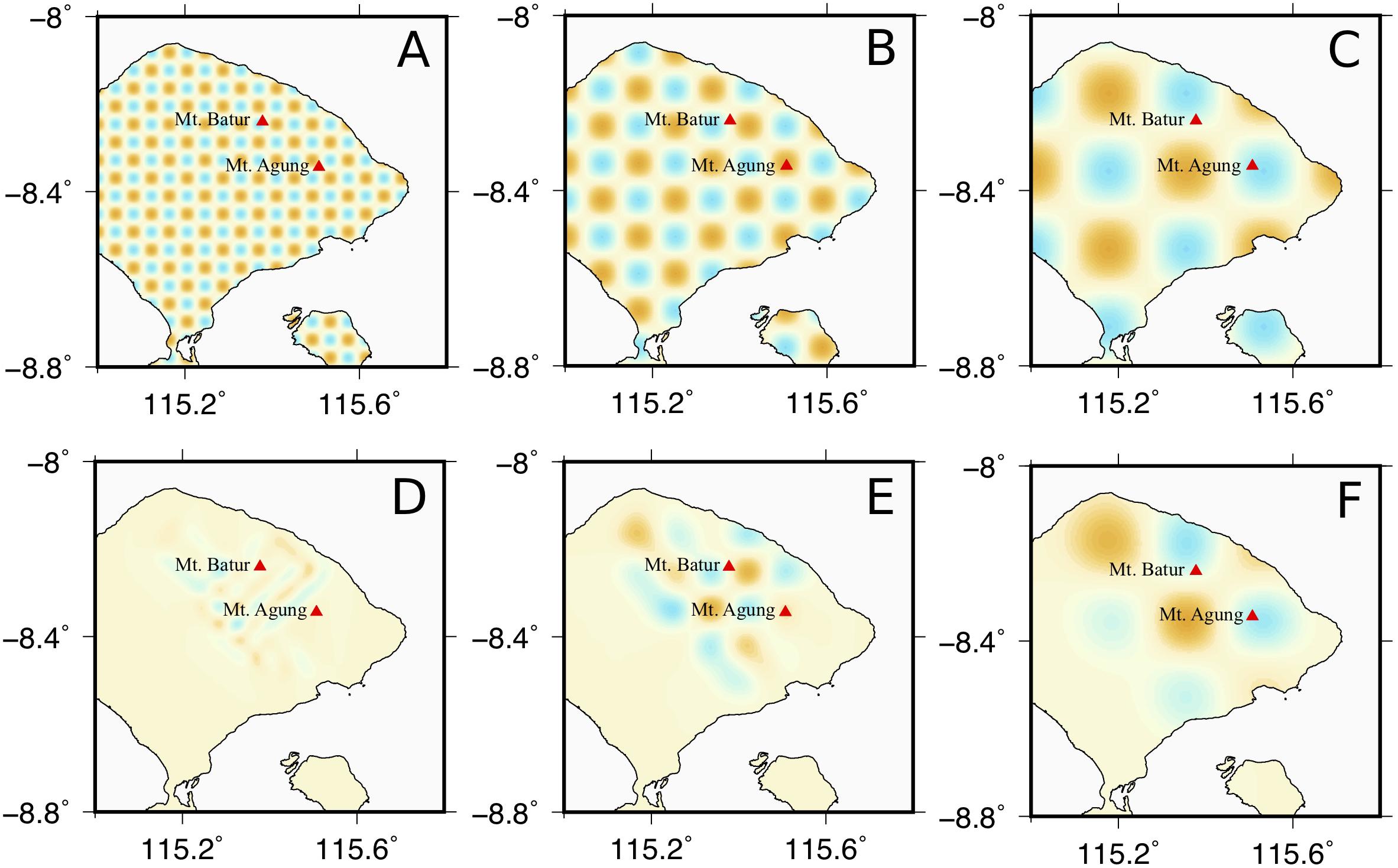
Figure 3. (A–C): Initial Rayleigh-wave group velocity models used for checkerboard resolution tests with cell sizes of ∼5 km × 5 km (0.05° × 0.05°), ∼10 km × 10 km (0.1° × 0.1°), and (∼15 km × 15 km (0.15° × 0.15°), respectively. (D–F): Final models obtained after tomographic inversion is applied to the travel times generated from the initial models in (A–C), respectively. Positions of volcanoes are marked by red triangles.
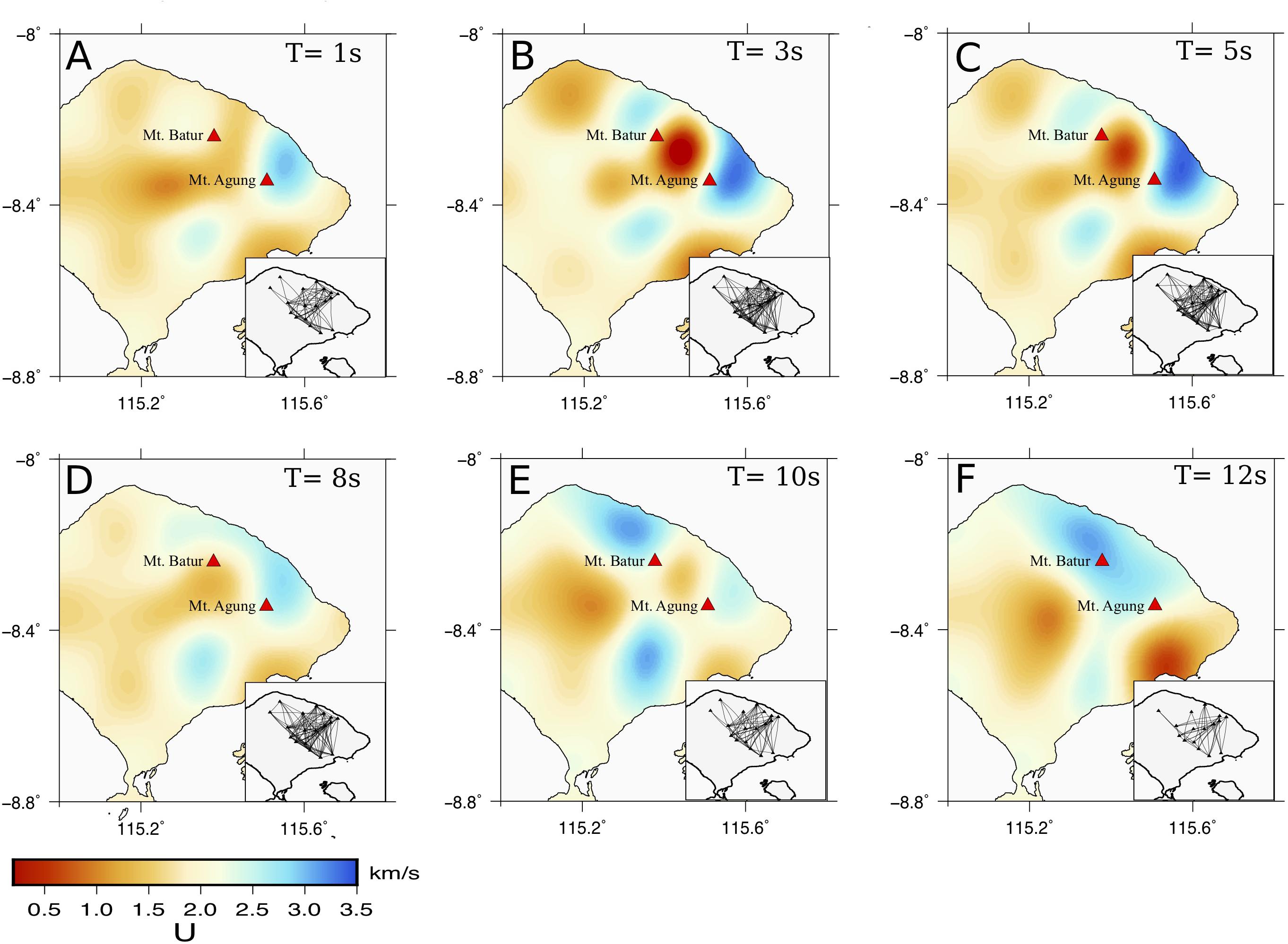
Figure 4. Results of group velocity tomography of ABVC using Rayleigh wave data extracted from ambient noise for different period ranges 1 s, 3 s, 5 s, 8 s, 10 s, and 12 s in (A–F), respectively. The Positions of volcanoes are marked by red triangles and inserted maps show the ray-path coverage at different periods.
Tomographic Group Velocity Model
Following the inversion procedure described above, Rayleigh wave group velocity tomograms at different periods were obtained. The damping and smoothing hyperparamters – and η, respectively, in Eq. 2 – were each assigned a value of 50, which we found provided acceptable trade-offs between the final model’s deviation from its initial value and its smoothness, and the data misfit. Figure 4 shows 2-D maps of tomographic group velocity models obtained for periods of 1, 3, 5, 8, 10, and 12 s, with insets depicting the ray-path coverage. In this study, longer periods (more than 10 s) have poor resolution due to sparse ray-path coverage.
The most prominent feature of the tomograms at different periods plotted in Figure 4 is the low velocity anomaly between Mt. Agung and Mt. Batur. This is a significant low velocity anomaly, with group velocity about 500 m/s at period 3 s (Figure 5). The position of this anomaly coincides with the cluster of earthquake epicenters between Mt. Agung and the Batur Caldera at depths less than 10 km estimated by Syahbana et al. (2019).
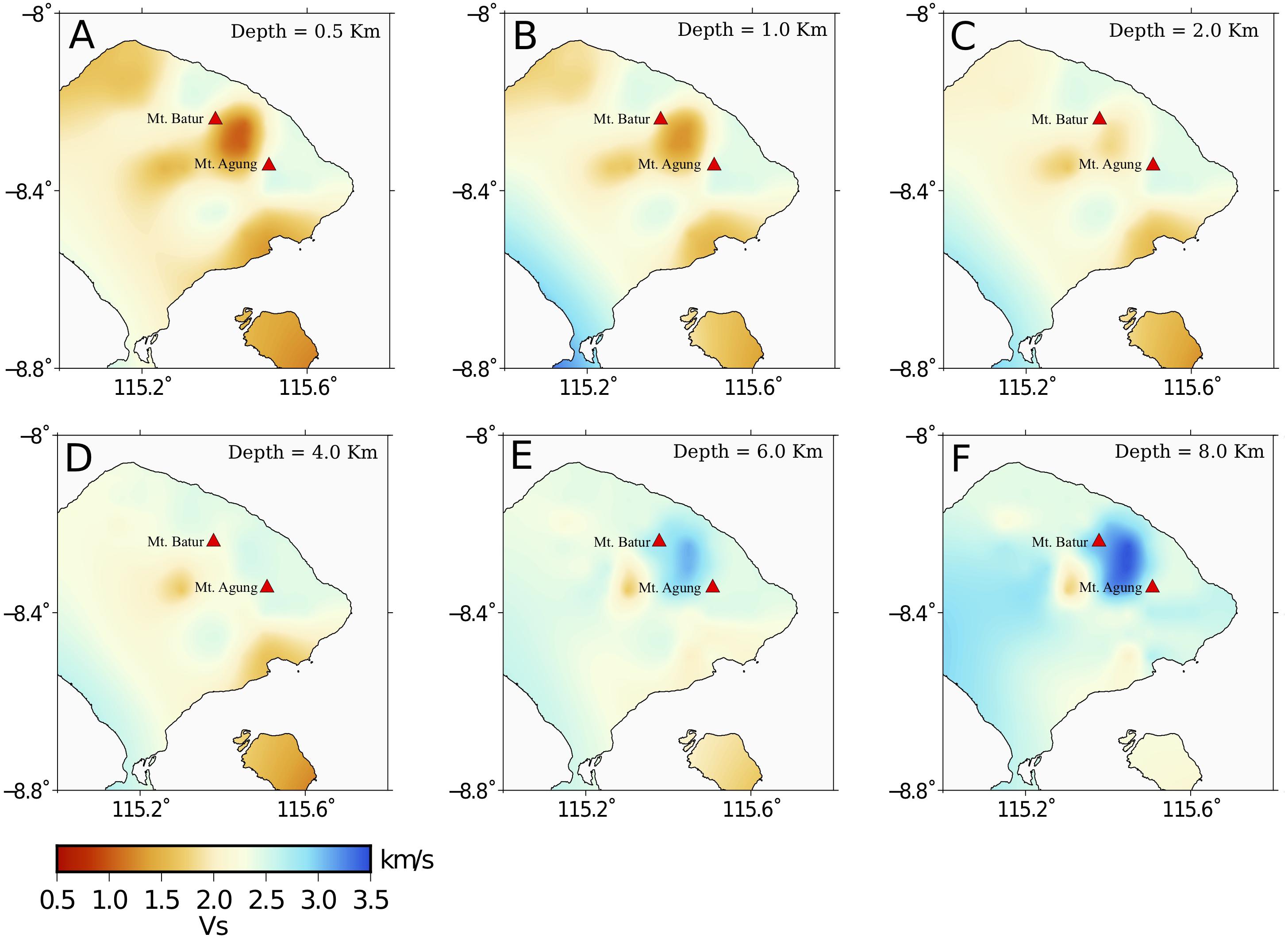
Figure 5. Results of shear wave velocity (Vs) of ABVC of group velocity ambient noise tomography for different depth ranges 0.5 km, 1.0 km, 2.0 km, 4.0 km, 6.0 km, and 8.0 km (A–F). The positions of volcanoes are marked by red triangles.
Shear Wave Velocity Model
We applied the NA as described by Sambridge (1999a, b) to invert group velocity dispersion curves. The group velocity curves were extracted for a set of regularly spaced grid points covering the study area, and inverted to create 1-D depth profiles of Vs (Supplementary Figure S2). There are 59 points that covered the study area at 5 km intervals. The group velocity at these spatial grid points retrieved from tomograms at periods between 0.5 and 12 s. The dispersion curve at each grid point was inverted using the program “dinver” in the Geopsy software package developed by Wathelet et al. (2008), to produce Vs profiles having a depth variation of velocity consistent with the observed dispersion curves. We allowed dinver to consider 4-layer model, with each layer of variable thickness and velocity range, and each having five “sublayers” over which the layer’s top and bottom velocities are linearly interpolated. The allowed layer parameters are Vs ranges of 500–2000, 1000–3000, and 1000–3000 m/s and thickness ranges of 500–2000, 2000–5000, and 5000–8000 m, respectively, for the top three layers, and 2000–4000 m/s for the Vs of the underlying half-space.
We interpolated the minimum-misfit 1-D Vs profiles produced by the NA inversion at each grid point to create a 3-D model of the Vs structure of the ABVC. The resulting maps of Vs for several depths (0.5–8 km) are plotted in Figure 5. The spatial pattern of low and high velocity at each depth is roughly consistent with that of the Rayleigh wave group velocity at different periods shown in Figure 4. The low velocity anomaly appears between Mt. Agung and Mt. Batur at depths up to 2 km, as shown in Figures 4A–D.
Discussion
The group velocity and shear velocity model as shown in Figures 4, 5 show the variation of velocity structure beneath the ABVC. A prominent low-velocity anomaly can be seen at periods u to 3 s for the group velocity maps, and depths up to 2 km for Vs, but disappears at greater periods and depth. We did synthetic test using initial model then conducted the inversion based on initial model (Supplementary Figure S3). The low velocity anomaly appears in between Mt Batur and Mt Agung. Vertical cross-sections of velocity structure in W–E and S–N directions can be seen in Figure 6. Both of these vertical cross-sections intersect the low velocity (∼0.5–2.2 km/s) anomaly between Mt. Agung and Mt. Batur that extends from the surface to about 2 km depth. In Figure 6 we overlay the Vs tomographic map with event hypocenters in the interval December 2018 to June 2019, to show the positive correlation between the low velocity anomaly and the hypocenter distribution. The hypocenters were estimated using the Non-Linloc method (Lomax et al., 2014), with manually picked P-and S-wave phases (Supplementary Figure S4) and the 1D seismic velocity model of Ak 135 (Kennett et al., 1995). We estimated the locations of 307 events that occurred during the seismometer deployment, with average location uncertainty: 1.70 km (EW), 1.75 km (NS), and 1.99 km (Z), for which the uncertainty histograms are shown in Supplementary Figure S5. Some of the hypocenters are at shallow depth near the low velocity anomaly, but much of the seismicity extends to greater depth beneath the low-velocity anomaly (Figure 6).
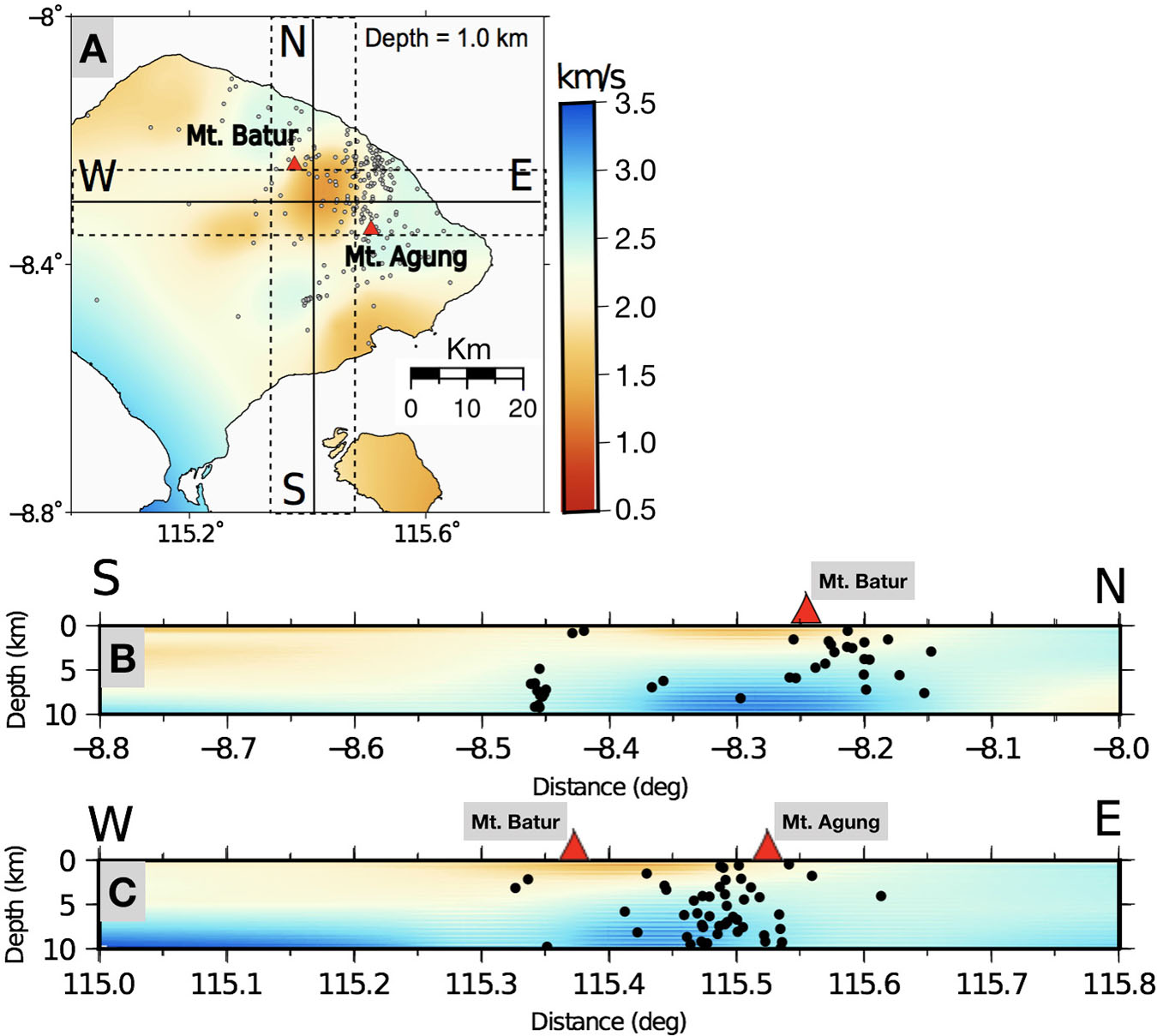
Figure 6. (A) The Vs tomographic map at 1.0 km depth with white circles indicating earthquake hypocenters and red triangles the volcanoes. Two black lines show the SN and WE tracks along which the vertical cross sections displayed in (B,C), respectively, are taken, while the black dash lines indicate the 5 km buffers around these cross-sections within which earthquake hypocenters were projected onto the respective vertical cross-section and displayed in (B) ad (C). (B,C), SN and WE vertical cross sections, respectively, of shear wave velocity (Vs) across Mt. Agung and Mt. Batur with hypocenters marked by filled black circles.
On the one hand, the low velocity observed at such shallow depth between Mt. Agung and Mt. Batur could simply reflect the accumulation of low-Vs volcanic deposits in this topographic “saddle” between the volcanoes. This could explain how the anomalous Vs could be so low, at 1000 m/s. However, this interpretation fails to explain the 2 km thickness of the low Vs anomaly, or the clustering of seismicity that extends from depth to the low Vs anomaly at 2 km depth (Figure 6).
Another explanation for the low velocity anomaly between Mt. Agung and Mt. Batur is that it could reflect magmatic processes. From an InSAR analysis of Sentinal-1 SAR data prior to the September 2017 eruption, Albino et al. (2019) inferred magma intrusion into a subvertical dike aligned with and located about midway along the axis connecting Mt. Batur and Mt. Agung – i.e., directly below the Vs anomaly in Figure 5, but at 7–13 km depth. Syahbana et al. (2019) found that both pre-eruptive seismicity and displacements at GNSS stations on the ABVC are consistent with this interpretation, and also note the potential for pressurization of groundwater above the dike. The shallow, low-Vs anomaly we observed is much shallower than the depth of the dike, so it is unlikely to be caused by magma intrusion, but it may be explained by overpressurization of groundwater. As described by Syahbana et al. (2019), meteoric water seeping down from the summit of Mt. Agung might interact with magmatic fluid and/or gases rising from the deep magmatic intrusion, leading to over-pressurization. Such overpressurization is consistent with both reduced Vs (Vanorio et al., 2005; Brenguier et al., 2008) and the occurrence of earthquakes (Coulon et al., 2017).
Conclusion
The evolution of our knowledge of the ABVC has involved several studies to understand volcanic processes beneath Mt. Agung and Mt. Batur. This paper presents the first local-scale study of Rayleigh wave group velocity structure of the ABVC, delineating the structure using ambient seismic noise tomography, which provides good resolution beneath and between Mt. Agung and Mt. Batur.
We estimated inter-station Rayleigh wave Green’s functions using ambient seismic noise cross correlation from available station pairs across the ABVC. Observed Rayleigh waves contain energy in the period range of 1–10 s, and arrival times of these surface waves were picked for available station pairs (see Figure 3).
2-D tomographic maps obtained from the inversion of the group velocity of Rayleigh waves and the Vs inverted from the resulting dispersion curves clearly delineate a strong low velocity anomaly as deep as 2 km depth between Mt. Agung and the Batur Caldera, and this coincides with a clustering of seismicity observed during the experiment. We suggest this low Vs anomaly can be explained by a combination of: (1) the accumulation of low-Vs volcanic deposits in the topographic saddle between Mt. Batur and Mt. Agung, and (b) the presence of over-pressurized hydrothermal fluids, possibly sourced from magma intrusion into a dike or system of dikes at depth >7 km inferred by Albino et al. (2019) and Syahbana et al. (2019). Of these explanations, only the presence of over-pressurized fluids provides a mechanism for generating the seismicity near the low Vs anomaly we imaged at <2 km depth.
Data Availability Statement
The datasets generated for this study are available on request to the corresponding author.
Author Contributions
ZZ, AN, SW, PC, and DPS conceived the survey and seismic study on ABVC. ZZ, DPS, and SR contributed to the writing of the manuscript. All authors contributed to the preparation of the manuscript, and read and approved the final manuscript.
Funding
This work was supported by the USAID’s PEER (Partnership for Enhance Engagement in Research) program with agreement number AID-OAA-A-11-00012, partially supported by P3MI of Global Geophysics Research Group, Faculty of Mining and Petroleum Engineering, Bandung Institute of Technology 2019 awarded to ZZ. 20 of the instrument sets used in the Agung Seismic Experiment were from the Australia-Indonesia Tectonics Observatory, funded by the Australian National University Major Equipment Committee Grant 17MEC33.
Conflict of Interest
The authors declare that the research was conducted in the absence of any commercial or financial relationships that could be construed as a potential conflict of interest.
Acknowledgments
This experiment was a collaboration between Bandung Institute of Technology (ITB), the Indonesian Geological Agency’s Center for Volcanology and Geological Hazard Mitigation (PVMBG), the Australian National University and the United States Geological Survey’s Volcano Disaster Assistance Program (VDAP). We used Generic Mapping Tools (Wessel and Smith, 1998) for producing the figures. We thank the two reviewers and JV as editor who gave constructive review and suggestions.
Supplementary Material
The Supplementary Material for this article can be found online at: https://www.frontiersin.org/articles/10.3389/feart.2020.00043/full#supplementary-material
References
Albino, F., Biggs, J., and Syahbana, D. K. (2019). Dyke intrusion between neighbouring arc volcanoes responsible for 2017 pre-eruptive seismic swarm at Agung. Nat. Commun. 10:748. doi: 10.1038/s41467-019-08564-9
Bensen, G. D., Ritzwoller, M. H., Barmin, M. P., Levshin, A. L., Lin, F., Moschetti, M. P., et al. (2007). Processing seismic ambient noise data to obtain reliable broad-band surface wave dispersion measurements. Geophys. J. Int. 169, 1239–1260. doi: 10.1111/j.1365-246X.2007.03374.x
Brenguier, F., Shapiro, N. M., Campillo, M., Ferrazzini, V., Duputel, Z., Coutant, O., et al. (2008). Towards forecasting volcanic eruptions using seismic noise. Nat. Geosci. 1, 126–130. doi: 10.1038/ngeo104
Chaussard, E., and Amelung, F. (2012). Precursory inflation of shallow magma reservoirs at west Sunda volcanoes detected by InSAR. Geophys. Res. Lett. 39:21311.
Coulon, C. A., Hsieh, P. A., White, R., Lowenstern, J. B., and Ingebritsen, S. E. (2017). Causes of distal volcano-tectonic seismicity inferred from hydrothermal modeling. J. Volcanol. Geotherm. Res. 345, 98–108. doi: 10.1016/j.jvolgeores.2017.07.011
Dziewonski, A. S., Bloch, S., and Landisman, M. (1969). A technique for the analysis of transient seismic signals. Bull. Seismol. Soc. Am. 59, 427–444. doi: 10.1016/j.ultras.2008.12.001
Escudero, C. R., and Bandy, W. L. (2017). Ambient seismic noise tomography of the Colima Volcano complex. Bull. Volcanol. 79:13. doi: 10.1007/s00445-016-1096-2
Fontijn, K., Costa, F., Sutawidjaja, I., Newhall, C. G., and Herrin, J. S. (2015). A 5000-year record of multiple highly explosive mafic eruptions from Mt. Agung (Bali, Indonesia): implications for eruption frequency and volcanic hazards. Bull. Volcanol. 77:59.
Geiger, H., Troll, V. R., Jolis, E. M., Deegan, F. M., Harris, C., Hilton, D. R., et al. (2018). Multi-level magma plumbing at Agung and Batur volcanoes increases risk of hazardous eruptions. Sci. Rep. 8:10547. doi: 10.1038/s41598-018-28125-2
Herrmann, R. B. (2013). Computer programs in seismology: an evolving tool for instruction and research. Siesmol. Res. Lett. 84, 1081–1088. doi: 10.1785/0220110096
Kennett, B. L. N., Engdahl, E. R., and Buland, R. (1995). Constrains on seismic velocities in the earth from travel times. Geophys. J. Int. 122, 108–124.
Kennett, B. L. N., Sambridge, M. S., and Williamson, P. R. (1988). Subspace methods for large scale inverse problems involving multiple parameter classes. Geophys. J. Int. 94, 237–247. doi: 10.1111/j.1365-246x.1988.tb05898.x
Lennartz Electronic (2017). LE-3Dlite MkIII: Compact, High Performance Three-Component 1 Hz Seismometer (Pamphlet). Tübingen: Lennartz Electronic.
Lomax, A., Michelini, A., and Curtis, A. (2014). “Earthquake location, direct, global-search methods,” in Encyclopedia of Complexity and System Science, 2nd Edn, ed. R. A. Meyers (New York, NY: Springer), 1–33. doi: 10.1007/978-3-642-27737-5_150-2
Martha, A. A., Cummins, P. R., Saygin, E., and Widiyantoro, S. (2017). Imaging of upper crustal structure beneath east Java–Bali, Indonesia with ambient noise tomography. Geosci. Lett. 4:14.
Monteiller, V., Got, J. L., Virieux, J., and Okubo, P. (2005). An efficient algorithm for double-difference tomography and location in heterogeneous media, with an application to the Kilauea volcano. J. Geophys. Res. Solid Earth 110:B12306.
Nagaoka, Y., Nishida, K., Aoki, Y., Aoki, Y., Takeo, M., and Ohminato, T. (2012). Seismic imaging of magma chamber beneath an active volcano. Earth Planet. Sci. Lett. 33, 1–8. doi: 10.1016/j.epsl.2012.03.034
Pinel, V., and Jaupart, C. (2003). Magma chamber behavior beneath a volcanic edifice. J. Geophys. Res. Solid Earth 108:2072. doi: 10.1029/2002JB001751
Pranata, B., Yudistira, T., Widiyantoro, S., Brahmantyo, B., Cummins, P. R., Saygin, E., et al. (2019). Shear wave velocity structure beneath Bandung basin, West Java, Indonesia from ambient noise tomography. Geophys. J. Int. 220, 1045–1054. doi: 10.1093/gji/ggz493
Rawlinson, N. (2005). FMST: Fast Marching Surface Tomography Package. Canberra: Research School of Earth Sciences, Australian National University.
Rawlinson, N., Pozgay, S., and Fishwick, S. (2010). Seismic tomography: a window into deep Earth. Geoscience 178, 101–135. doi: 10.1016/j.pepi.2009.10.002
Rosalia, S., Cummins, P. R., Widiyantoro, S., Yudistira, T., Nugraha, A. D., and Hawkins, R. (2020). Group velocity maps using subspace and transdimensional inversions: ambient noise tomography in the western part of Java, Indonesia. Geophys. J. Int. 220, 1260–1274. doi: 10.1093/gji/ggz498
Sabra, K. G., Gerstoft, P., Roux, P., Kuperman, W. A., and Fehler, M. C. (2005). Surface wave tomography from microseisms in Southern California. Geophys. Res. Lett. 32:L14311. doi: 10.1029/2005GL023155
Sambridge, M. (1999a). Geophysical inversion with a neighborhood algorithm I: searching a parameter space. Geophys. J. Int. 138, 479–494. doi: 10.1046/j.1365-246x.1999.00876.x
Sambridge, M. (1999b). Geophysical inversion with a neighborhood algorithm II: appraising the ensemble. Geophys. J. Int. 138, 727–746. doi: 10.1046/j.1365-246x.1999.00900.x
Saygin, E., Cummins, P. R., Cipta, A., Hawkins, R., Pandhu, R., Murjaya, J., et al. (2016). Imaging architecture of the Jakarta Basin, Indonesia with transdimensional inversion of seismic noise. Geophys. J. Int. 204, 918–931. doi: 10.1093/gji/ggv466
Saygin, E., and Kennett, B. L. N. (2010). Ambient seismic noise tomography of Australian continent. Tectonophysics 481, 116–125. doi: 10.1038/srep08218
Saygin, E., and Kennett, B. L. N. (2012). Crustal structure of Australia from ambient seismic noise tomography. J. Geophys. Res. 117:B01304. doi: 10.1029/2011JB008403
Self, S., and Rampino, M. R. (2012). The 1963–1964 eruption of Agung volcano (Bali, Indonesia). Bull. Volcanol. 74, 1521–1536. doi: 10.1007/s00445-012-0615-z
Shapiro, N. M., and Campillo, M. (2004). Emergence of broadband Rayleigh waves from correlations of the ambient seismic noise. Geophys. Res. Lett. 31:L07614. doi: 10.1029/2004GL019491
Shapiro, N. M., Campillo, M., Stehly, L., and Ritzwoller, M. H. (2005). High- resolution surface-wave tomography from ambient seismic noise. Science 307, 1615–1618. doi: 10.1126/science.1108339
Shapiro, N. M., Gorbatov, A. V., Gordeev, E., and Dominguez, J. (2000). Average shear-wave velocity structure of the Kamchatka peninsula from the dispersion of surface wave. Earth Planets Space 52:573577.
Snieder, R. (2004). Extracting Green’s function from the correlation of coda waves: a derivation based on stationary phase. Phys. Rev. E 69:046610. doi: 10.1103/PhysRevE.69.046610
Stankiewicz, J., Ryberg, T., Haberland, C., and Natawidjaja, D. H. (2010). Lake Toba volcano magma chamber imaged by ambient seismic noise tomography. Geophys. Res. Lett. 37:L17306. doi: 10.1029/2010GL044211
Surjo, I. (1981). Report on the volcanic activity in Indonesia during the period 1961–1963. Bull. Volcanol Surv. Indonesia 104, 58–94.
Syahbana, D. K., Kasbani, K., Suantika, G., Prambada, O., Andreas, A. S., Saing, S. L., et al. (2019). The 2017–19 activity at Mount Agung in Bali (Indonesia): intense unrest, monitoring, crisis response, evacuation, and eruption. Sci. Rep. 9:8848.
Vanorio, T., Virieux, J., Capuano, P., and Russo, G. (2005). Three-dimensional seismic tomography from P wave and S wave microearthquake travel times and rock physics characterization of the Campi Flegrei Caldera. J. Geophys. Res. 110:B03201. doi: 10.1029/2004JB003102
Wathelet, M., Jongmans, D., Ohrnberger, M., and Bonnefoy-Claudet, S. (2008). Array performances for ambient vibrations on a shallow structure and consequences over Vs inversion. J. Seismol. 12, 1–19. doi: 10.1007/s10950-007-9067-x
Wessel, P., and Smith, W. H. F. (1998). New, improved version of the generic mapping tools released. EOS 79:579. doi: 10.1029/98eo00426
Widiyantoro, S., Ramdhan, M., Métaxian, J. P., Cummins, P. R., Martel, C., Erdmann, S., et al. (2018). Seismic imaging and petrology explain highly explosive eruptions of Merapi Volcano, Indonesia. Sci. Rep. 8:13656. doi: 10.1038/s41598-018-31293-w
Yudistira, T., Paulssen, H., and Trampert, J. (2017). The crustal structure beneath The Netherlands derived from ambient seismic noise. Tectonophysics 721, 361–371. doi: 10.1016/j.tecto.2017.09.025
Zen, M. T., and Hadikusumo, D. (1964). Preliminary report on the 1963 eruption of Mt. Agung in Bali (Indonesia). Bull. Volcanol. 27, 269–300.
Keywords: Agung Volcano, Bali-Indonesia, cross correlation, Rayleigh wave, ambient seismic noise tomography
Citation: Zulfakriza Z, Nugraha AD, Widiyantoro S, Cummins PR, Sahara DP, Rosalia S, Priyono A, Kasbani K, Syahbana DK, Priambodo IC, Martanto M, Ardianto A, Husni YM, Lesmana A, Kusumawati D and Prabowo BS (2020) Tomographic Imaging of the Agung-Batur Volcano Complex, Bali, Indonesia, From the Ambient Seismic Noise Field. Front. Earth Sci. 8:43. doi: 10.3389/feart.2020.00043
Received: 30 July 2019; Accepted: 04 February 2020;
Published: 20 February 2020.
Edited by:
Jean Vandemeulebrouck, Université Savoie Mont Blanc, FranceReviewed by:
James Oliver Scott Hammond, Birkbeck, University of London, United KingdomFinnigan Illsley-Kemp, Victoria University of Wellington, New Zealand
Copyright © 2020 Zulfakriza, Nugraha, Widiyantoro, Cummins, Sahara, Rosalia, Priyono, Kasbani, Syahbana, Priambodo, Martanto, Ardianto, Husni, Lesmana, Kusumawati and Prabowo. This is an open-access article distributed under the terms of the Creative Commons Attribution License (CC BY). The use, distribution or reproduction in other forums is permitted, provided the original author(s) and the copyright owner(s) are credited and that the original publication in this journal is cited, in accordance with accepted academic practice. No use, distribution or reproduction is permitted which does not comply with these terms.
*Correspondence: Zulfakriza Zulfakriza, enVsZmFrcml6YUBpdGIuYWMuaWQ=; Andri D. Nugraha, YW5kcmlkbjEwNEBnbWFpbC5jb20=