- School of Biological Sciences, University of Bristol, Bristol, United Kingdom
Static electric fields in the atmosphere are increasingly recognized as interacting with various organisms over several levels of biological organization. Recently, a link between atmospheric electrical variations and biogeochemical processes has been established in the context of open fields, yet biological structures like trees produce substantial alterations in atmospheric electric properties. Here, we assess whether these structural changes affect the dynamics of the electrical landscape and its relation to geochemical processes. To this end, we theoretically assess how trees alter their surrounding electric fields and empirically compare the temporal dynamics of atmospheric potential gradients, positive ions in the near-ground level atmosphere and soil electrochemical properties in an open field and under a tree. The developed model of electric fields around trees provides insight into the extent to which trees shield the underlying electric landscape, revealing that a substantial increase in atmospheric potential gradient only marginally affects the electric field under the canopy. We further show that soil electrochemical properties are tied to the temporal dynamics of positive ion in the near-ground level atmosphere, and that the presence of a tree reduces the temporal variability in both ground level positive ion concentrations and soil redox potential. This suggests that a tree can alter the temporal variability in atmospheric electricity and soil electro-chemistry, thereby likely indirectly influencing soil microorganisms and processes as well as electro-sensitive organisms that perceive and utilize atmospheric electric fields.
Introduction
The ground level atmosphere is host to various distributions of electrical charges and associated static electric fields (Rycroft et al., 2008) and it becomes increasingly apparent that electrostatic interactions form the basis of the electric ecology of various organisms over several levels of biological organization (e.g., molecules, cells, organisms, communities) (Clarke et al., 2013; Greggers et al., 2013; Morley and Robert, 2018; Hunting et al., 2021). Atmospheric charge distributions and electric fields are dynamic and can show variations over hours to seasons which are mainly driven by two sources. First, there is a build-up of mainly positive ions near the surface of the ground (Adkins, 1959; Crozier, 1965; Reiter, 1985) that results from a complex interplay of soil radon exhalation and decay, the charging of aerosols and atmospheric pollution (Kubicki et al., 2016). These largely elusive sources of charge result in hourly and daily variations in ground level (<2 m) atmospheric charges that are important at near surface layers of continental soils. Second, thunderstorm regions around the globe drive positive charges at higher altitudes (approx. 8–13 km) towards fair weather regions as part of the global electric circuit (Wilson, 1903; Rycroft et al., 2008; Fdez-Arroyabe et al., 2021). This results in diel and seasonal variations in atmospheric potential gradients that, although sometimes measureable over continental soils, are far more prevalent in clean oceanic air. Consequently, atmospheric charge distributions and associated electrical fields and charge flow operate at local and global spatial scales and various temporal (hours to seasons) scales.
Atmospheric electric fields—as well as their dynamics—have recently been linked to biogeochemical processes below the Earth’s surface by means of a charge separation between relatively negative charges in the Earth’s interior and positive charge sources in the atmosphere (Hunting et al., 2019). Changes in atmospheric charges were observed to drive a subsurface migration of nutrients that are essential to microbial metabolism, thereby affecting efficiency and dynamics of microbial processes (Hunting et al., 2019). The conceptual and empirical foundation underlying the link between atmospheric electrical variations and biogeochemical processes has been established in the context of open fields (e.g., grasslands) and freshwater systems. However, both empirical data and mathematical models have shown that trees produce substantial alterations in the electric landscape, in particular the electric field strength in the surrounding air (Arnold et al., 1965; Borra et al., 1997; Bowker and Crenshaw 2007; Williams and Mareev, 2014; Clarke et al., 2017; Morley and Robert, 2018). In this, positive charges in the atmosphere draw a negative mirror charge to surfaces of trees with high curvatures (e.g., branches, needles) and thereby produce larger fields compared to surfaces that have a smaller degree of curvature or are flat (Feynman et al., 1964; Rowland et al., 2015; Clarke et al., 2017). Thus, as plants display some mobility in their surface electric charge carriers (electrons, charged molecules and ions), the electric field surrounding them will take up geometries that are influenced by the strength of atmospheric potential gradients as well as the plants’ height and morphology. Likewise, large plants, such as trees, have been reported to contribute substantially to local variations in ground level atmospheric ions (e.g., Jayaratne et al., 2011) that are generated largely by transpiration. It thus becomes apparent that trees and their canopies present both an abiotic modifier as well as biogenic source of atmospheric electricity (See Figure 1 for a conceptual and mathematical representation of electric fields and ions around trees).
In light of the largely uncharacterised and empirically elusive near-ground (0–2 m) electric landscape and its unsuspected roles in geochemical changes in both near-ground and subsurface environments, questions are thus arising whether structural changes within the landscape such as trees also affect the dynamics of atmospheric electricity and its relation to geochemical processes. Here, we theoretically assess how the structural and physical properties of trees are relevant in shaping their surrounding electric fields, and empirically compare the temporal dynamics of atmospheric potential gradients, positive ions in the near-ground level atmosphere and soil electrochemical properties in an open field and under a tree.
Methods
Model of Atmospheric Electric Fields
Since modelling approaches with two-dimensional geometries published to date are potentially prone to exaggerating the electrical shielding effect of most structures, we modelled a three-dimensional geometry to assess the effect of a tree on local atmospheric electric fields. Modelling was performed using finite element analysis within COMSOL Multiphysics® v. 5.4 (COMSOL AB, Stockholm, Sweden) utilising the “Electric Currents” interface within the “AC/DC” module. The three-dimensional geometry consisted of a 300 m × 300 m x 300 m cube within which the model operated. The model ground level was occupied by a 300 m × 300 m x 2 m block of soil. At its centre, an approximate representation of a 30 m tall deciduous tree, with a 2 m wide trunk, and a canopy spanning roughly 30 m was positioned. This tree could be regarded as a model of an oak or an alder tree. The remainder of the geometry was assigned as air. The upper surface of this air column was given an electrical potential typical of a 300 m altitude in various meteorological conditions, representative of the atmospheric potential gradient (Wilson 1903; Bennett and Harrison 2007). The electrical ground was defined 2 m below the upper surface of the soil (a typical groundwater table depth). Meshing of this geometry was physics-controlled, set to “extremely fine” (Supplementary Figure S1). Electrical properties of all materials were obtained from measurements or estimates from primary literature. The electrical conductivity, σ, and relative permittivity, εr, were defined as: for soil, σ = 0.05 S/m, εr = 15 (Rhebergen et al., 2002; Brovelli and Cassiani, 2011); for living trees, σ = 2 × 10−4 S/m, εr = 12 (Suojanen et al., 2001; Gora and Yanoviak, 2015); and for air, σ = 1 × 10−14 S/m, εr = 1 (Hogg 1939; Higazi and Chalmers, 1966). Model outputs presented for this study were produced by plotting data from two-dimensional slices or one-dimensional cut lines through areas of interest within the three-dimensional geometry. An identical model, except with no tree present, was constructed as a reference. To test for the sensitivity of the results to parameter value selection, parametric sweeps were performed across a wide range of possible values for all of the included electrical material properties except for the relative permittivity of air as this is a well-known quantity that is unlikely to vary substantially.
Experimental Approach
Atmospheric Drivers of Soil Redox Potential
Soil redox potential was previously linked to ground level ion concentrations, yet it is necessary to assess whether local soil redox potential is potentially coupled to atmospheric potential gradients as well as other meteorological variables. Due to technical issues it was impossible to measure atmospheric potential gradients, atmospheric ions and other meteorological variables simultaneously in relation to soil redox potential. Two separate time-series where therefore measured to assess the relative importance of both atmospheric potential gradients and ions as a driver of soil redox potential. These time series measurements and subsequent experiments were performed at the University of Bristol, School of Veterinary Sciences, Langford United Kingdom, between October 2018 and February 2019, in which measurements took place during fair weather with occassional cloud cover (fair weather conditions as defined in Harrison and Nicoll, 2018).
The first time-series was recorded between 24-10-2018 and 31-10-2018 in open field conditions to assess whether local soil redox potential is potentially coupled to atmospheric potential gradients as well as other meteorological variables. To this end, the atmospheric potential gradients was continuously measured on site using a field mill (Boltek EFM 100 Field Mill) that consists of a horizontal electrode connected to an electrometer that is alternately exposed and shielded from the atmospheric electric field in which a charge is induced on the electrode that is proportional to the field. The field mill was positioned with its rotor facing up on top of a single vertical aluminium pole at 1.8 m above the top layer of the soil to assess variations in the atmospheric potential gradient above the first 2 m of the ground level atmosphere. Meteorological parameters where continuously measured on site using a Maximet (GMX501) weather station, and included temperature (°C), humidity (RH), air pressure (mB), solar radiation (W/m2), windspeed (m/s) and precipitation (mm/min). Redox potentials (Eh) in soils were measured simultaneously every 20 min using a permanently installed platinum electrode at 10 cm depth connected to a Hypnos three data logger and corrected for a calomel reference electrode and measured pH. Details on the Eh monitoring devices and electrodes are described elsewhere (Vorenhout et al., 2011).
A second time-series was measured between 13-11-2018 and 17-11-2018 to assess the relation between soil redox potential and concentration of positive ions in the atmosphere just above (<0.2 m) the soil. This was simultaneously measured in an open field and next to the trunk of a grey alder (Alnus incana) in which the tree did not carry leaves and the top soil layer was covered with short (<10 cm) grass during the measurement period. Atmospheric cation concentrations were measured every second using two cylindrical capacitor-based ion-counters operating on the “Gerdien-tube” principle (Alpha-lab Inc., Salt Lake City, UT, United States), calibrated in open field conditions and recorded onto a PC using a National Instruments (Austin, Texas) DAQ system. Redox potentials (Eh) in soils were measured as described above.
Influence of Tree Canopy on Soil Redox Potential and Atmospheric Ions
Initial time-series measurements revealed that local soil redox potential was primarily governed by atmospheric cation concentrations (see results section for details). Therefore, to further quantify the influence of canopies on ground level variations of both redox potential and atmospheric ions, a series of measurements in time were performed during the day in an open field and next to the trunk of a grey alder (Alnus incana) in February 2019. This focussed on the period between 10:00 am and 20:00 pm to avoid large influences of diel fluctuations typically observed in ground level atmospheric ion concentrations (e.g. Jayaratne et al., 2011). We used standardized soil microcosms to assure soils were comparable between both tree and open field conditions (i.e., sufficient water content, exclusion of ground water flow). To this end, 400 ml glass beakers were filled with a pre-wetted standardized soil mixture (quartz and turf, 3:1). Positive ground level atmospheric ions and soil redox potential were measured simultaneously in soil microcosms at 1 cm depth in which the microcosms were positioned at the base of a stand-alone tree and an open field, 5 m away from the margin of the canopy of the tree. This was repeated six times (n = 6) at six different fair weather days with freshly prepared microcosms. In this, soil redox potentials (Eh) were measured as described above, yet using two permanently installed gold-plated PCB electrodes (Vorenhout et al., 2011). Temporal variability of the measurements of the six individual days were subsequently assessed with a Wavelet transform spectral analysis in PAST (Hammer et al., 2001). This form of spectral analysis proved a valuable approach in evaluating spatial and temporal patterns in ecological research by creating a composite measure of temporal variance for each treatment or spatially separated samples over time (Bradshaw and Spies, 1992; Ibarra-Junquera et al., 2006; Escalante-Minakata et al., 2009). As applied here, we followed the procedures described in detail by Hunting et al. (2015). Amplitude of temporal variation were thus characterised, allowing the comparison between simultaneous or spatially separated measurements by quantifying the variance of the wavelet transform for each day and individual microcosms. Finally, differences between trees and open-field wavelet variances were assessed using a small sample-corrected Epps-Singleton test for equal distributions (Epps and Singleton, 1986).
Results
Modelling using finite element analysis demonstrates that the presence of a tree has a marked impact on the surrounding electric field (Figures 2A,B,C). Beneath the tree, electric field strengths are reduced by more than an order of magnitude, supporting the hypothesis that trees act as electrical shields, greatly diminishing variability in electrical conditions beneath their canopies. A horizontal transect taken through the model (Figure 2B) shows that for a large tree in an open field, this shielding effect persists beyond the immediate vicinity of the tree, with the electric field strength continuing to be reduced in excess of 100 m away from the trunk in comparison to no tree being present. In contrast to this arboreal suppression of electric fields beneath the canopy, we conversely find that the electric field strength immediately above the tree is amplified above levels expected if no tree were present. Examining the vertical transect through the model, positioned 5 m away from the trunk (Figure 2C), emphasises this effect, whereby significant amplification takes place near the treetop, persisting over significant distances, with some visible elevation of electric field strength even at 100 m above the tree canopy. Under a fair-weather atmospheric potential gradient of 100 V/m, or unstable conditions presenting 1 kV/m, the electric field in the immediate vicinity of the treetop can reach 450 V/m and 4.5 kV/m, respectively.
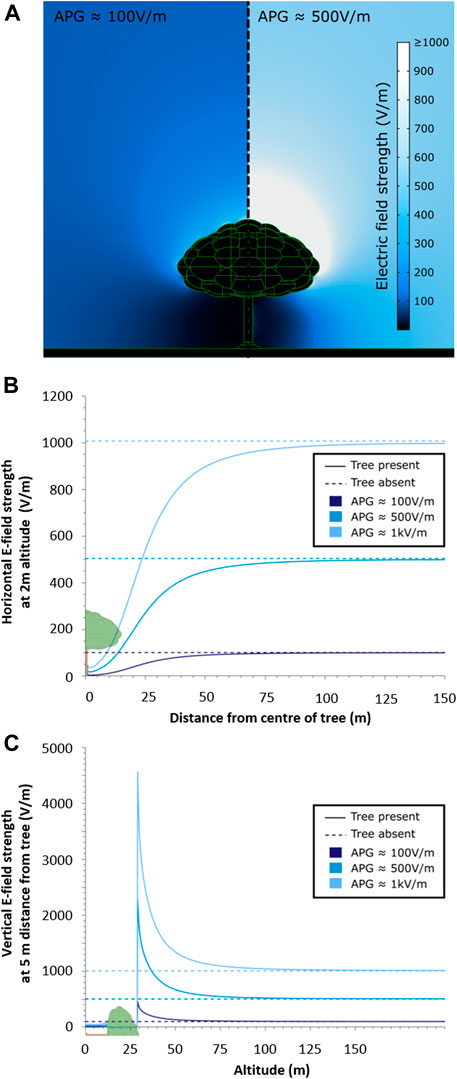
FIGURE 2. Outputs from a three-dimensional finite element analysis model of the electric fields surrounding a tree stood in open ground, exposed to various vertical atmospheric potential gradients (APGs). (A) two-dimensional slice through the centre of the tree in two different APG strengths, 100 V/m (left) and 500 V/m (right), showing the resultant electric landscape. (B) cutline horizontal transects taken through the model travelling from the centre of the tree outwards for 150 m, at an altitude of 2 m, for various APG strengths, exemplifying the electrical shielding effect of the tree both immediately underneath the tree and for large distances away from the canopy. Model outputs with a tree present (solid) are compared with identical models with no tree present (dashed) (C) cutline vertical transects taken through the model, travelling from the soil surface upwards for 200 m, 5 m horizontally from the centre of the tree, for various APG strengths, showing both the shielding effect beneath the canopy and the amplification of the APG above the tree. Again, model outputs with a tree present (solid) are compared with identical models with no tree present (dashed).
The results of the model were found to be insensitive to parameter value selection in most cases (Supplementary Figure S3), suggesting the results of this model hold for all realistic values of the included parameters. Specifically, within the wide-ranging but still potentially possible bounds tested, the permittivity of the soil and tree had no marked effect at all on the shielding ability of the tree. Likewise, within realistic ranges of air conductivity, the tree continues to act as an electrical shield. Some variation in the results is seen when the conductivity of the tree approaches that of the air. This is to be expected because it is largely the elevated conductivity of the tree, relative to the air, that allows it to act as an electrical shield. However, it is highly unlikely that a living tree would have a conductivity this low, and it can be seen that within a more realistic range of tree conductivities, the tree continues to be an effective electrical shield. Similarly, some increase in electric field strength is seen as the soil conductivity approaches that of air. This is again a very unlikely physical situation, but even at these extreme values, the tree continues to reduce the APG by an order of magnitude.
While differences is sampling frequencies prevented a statistical analysis of correlation between the measured variables, it becomes apparent that soil redox potential did not visibly co-vary with any of the measured atmospheric meteorological variables (Supplementary Figure S1). An increase in redox potential and subsequent emergence of a diel cycle coincided with a period of prolonged precipitation on the 27th of October, suggesting the soil was not necessarily saturated with water throughout the measurement period (e.g., Cusell et al., 2015). Diel cycles in soil redox potential between 29th and October 31, 2018 did not seem to be directly governed by changes in the atmospheric potential gradient.
The 5 days measurement period between 13th and November 17, 2018 suggests that ground-level positive atmospheric ions and soil redox potential in soil microcosms in the open field have distinct diel cycles (Figures 3A,B) that seem to co-vary. In contrast, near the trunk of a tree, soil redox potential revealed substantially (∼80%) lower amplitude in diel variation, and ground-level positive atmospheric ions did not appear to have any diel rhythm (Figures 3A,B).
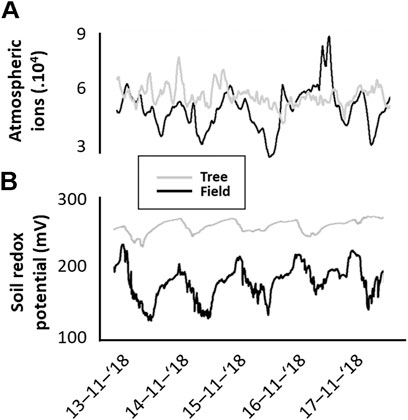
FIGURE 3. Co-variability of positive atmospheric ions and soils redox potential as revealed by a time-series (13-11-2018 untill 17-11-2018) measurements of (A) concentration of positive ions in the atmosphere just above (<0.2 m) and (B) soil redox potential, Eh. This was simultaneously measured in an open field and next to the trunk of a grey alder (Alnus incana).
A representative example of the measured time-series similarly reveals that the moving averages of positive atmospheric ions and soils redox potential in soil microcosms covary during the day (Figures 4A,B). Comparison of Wavelet variances throughout the entire measurement period across the replicated time-series (Figures 4C,D) suggests that temporal variability was lower for both ground level atmospheric ion concentrations and soil redox potential near a tree compared to Wavelet variances in the open field (Epps-Singleton-derived error: W2 = 63.94; p < 0.001 and W2 = 1,439.1; p < 0.001, respectively).
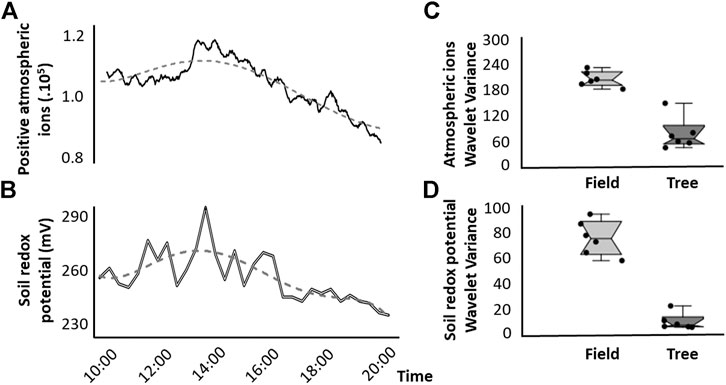
FIGURE 4. Representative example of the measured time-series revealing co-variability of (A) positive atmospheric ions and (B) soils redox potential in soil microcosms, in which dashed line represents the hourly moving average. (C,D) Comparison of temporal variability across the replicated time-series for each day and individual microcosms for repeated measurements during fair weather days in February 2019. Temporal variability is expressed as Wavelet variances between near a tree and in an open field for (C) near ground-level atmospheric positive ions and (D) soil redox potential.
Discussion
The developed model of the electric fields around trees exemplifies the drastic influence of trees and other large plants on their electrical environment, even when only considering passive electrical interactions. Undoubtedly, an even more marked effect can be expected in tree lines or forests due to a cumulative effect of collections of plants and trees (see also Williams et al., 2005). Importantly, the extension of previous two-dimensional models on electrical shielding by trees (Clarke et al., 2017; Morley and Robert, 2018) into three dimensions corroborates the influence of vegetation on its local electrical environment. The presented three-dimensional model confirms that prior findings on the shielding ability of trees using a two-dimensional approach were qualitatively valid yet may not have been entirely numerically accurate. Previous studies demonstrated that tree canopies can alter atmospheric electric fields, almost nullifying them near the base of the tree trunk, while the reach of this shielding effect extended beyond the canopy cover by 5–20 m with increasing distance from the tree (Märcz and Harrison, 2003; Williams et al., 2005; Clarke et al., 2017; Morley and Robert, 2018). Our three-dimensional model confirms that the electric field strength is reduced to near zero beneath a tree canopy, and reveals that a substantial (5–10 fold) increase in atmospheric potential gradient only marginally affects electric fields under the canopy, articulating the extent to which a tree canopy shields its underlying electric landscape and distorts the atmospheric potential gradient beyond its own span. Remarkably, above the canopy the local electric field can be greatly enhanced, generating features in the electric landscape otherwise absent in open fields.
Local sources of atmospheric electricity in continental environments often remain ambiguous, relying on both globally driven atmospheric potential gradients and local sources of ionization (e.g., Wright et al., 2020). The absence of correlation between atmospheric potential gradients and other metereological parameters in our study strongly supports the notion that ground level atmospheric ion concentrations above soils are driven by local concentrations of atmospheric ions, charged aerosols and radionuclides (Kubicki et al., 2016). These ground level ion concentrations can be substantially higher around trees, observable especially in forests, compared to open fields due to transpiration of ions and radon by vegetation (e.g. Ling, et al., 2010; Jayaratne et al., 2011). Importantly, since transpiration is drastically reduced in winter, atmospheric ions between tree canopy cover and open fields are not expected to markedly differ in magnitude, suggesting that, in the present study, local sources of atmospheric ions such as soil radon exhalation and air pollution (e.g. fuel exhausts) made up the electric charges at near-ground level atmosphere in both open fields and near vegetation.
While several studies have focussed on how trees affect the local electric landscape, it remains largey unknown how trees, as large partially conductive and dielectric structures, affect the temporal dynamics of atmospheric potential gradients, and whether and how this affects multiscale chemical and biological processes. Using microcosms in fair weather conditions to exclude other major drivers of variability (e.g., shower clouds, soil water content), we observed clear differences in the dynamics between both ground level atmospheric ions and soil redox potential between the open field and below a tree canopy over a 5 days measuring period. Although clear diel cycles were visible in both ions and redox potential in open fields, diel cycles were not observed in atmospheric ions near the trunk of the tree. Soil redox potential was observed to have diel cycles, yet their magnitude was substantially lower (∼10%) compared to open field conditions. A closer assessment of the temporal variability near a tree and the open field revealed that soil electrochemical properties are tied to temporal dynamics of positive ions in the near-ground level atmosphere, and that the presence of a tree reduced the temporal variability in both ground level positive ions concentrations and soil redox potential. This shows that, while ground level atmospheric ions were of primarily local origin, a tree can have a stabilizing effect on drivers of temporal variability. It is important to consider that various sources are known to contribute to ground-level electric variability, including windspeed, soil radon exhalation, tree transpiration and air-earth currents driven by atmospheric potential gradients (Jayaratne et al., 2011; Kubicki et al., 2016). Since our measurements were obtained in winter and near a single tree, it is unlikely that wind speed and tree transpiration were the source of variability. Likewise, soil radon exhalation is expected to add variability near trees provided tree soils are substantially more permeable compared to grasslands (Alaoui et al., 2011; Holthusen et al., 2018). Therefore, the most likely source of variability in this study is of atmospheric origin, in which a tree and its canopy appear to shield ground-level electric dynamics from atmospheric influences. This offers a plausible explanation to the frequently observed differences in the temporal characteristics of soil and sediment electrochemical signatures between open fields and near vegetation.
The observed interplay between vegetation, atmospheric electrical variations and soil redox potential is biologically relevant since redox potential is an important driver of bacterial community structure and metabolism in both soils and aquatic sediments (Newman and Banfield, 2002; Bertics and Ziebis, 2009; Hunting and van der Geest, 2011; Hunting et al., 2012), and oscillations in redox conditions are known to change microbial community composition (Pett-Ridge and Firestone, 2005) and promote bacterial metabolic processes (Aller, 1994). These changes in microbial metabolism can be attributed to the mobilization of microbial nutrients due to changes in the physico-chemical environment (Aller, 1994) as well as the migration of respiratory ions along electrochemical gradients and an electric field (Hunting et al., 2019). Bacteria themselves have been observed to occupy an apparent “redox niche” in which they actively migrate towards the most favourable electrochemical conditions (Bespalov et al., 1996) or actively control the redox conditions in their immediate surroundings by membrane bound and secreted redox mediators (Hunting and Kampfraath, 2013). The observed influence of local dynamics in atmospheric electricity governed by vegetation may thus affect microbial processes in soils and aquatic sediments in vegetated areas, and likely carries wider implications for electro-sensitive organisms (e.g., pollinators, ballooning spiders, and perhaps other arthropod species) that perceive and utilize atmospheric electric fields.
Data Availability Statement
The raw data supporting the conclusion of this article will be made available by the authors, without undue reservation.
Author Contributions
EH conceived the study and collected empirical data and performed statistical analyses. SE developed the model. EH drafted the manuscript. All authors contributed to study design and developing the manuscript.
Funding
EH received financial support from the Swiss National Science Foundation, SNF (CRSK-2 190855). DR is funded by the BBSRC (Grant BB/T003235/1) and the European Research Commission (ERC-ADG 743093), supporting EH and SE.
Conflict of Interest
The authors declare that the research was conducted in the absence of any commercial or financial relationships that could be construed as a potential conflict of interest.
The handling editor declared a past co-authorship with one of the authors EH.
Supplementary Material
The Supplementary Material for this article can be found online at: https://www.frontiersin.org/articles/10.3389/feart.2021.671870/full#supplementary-material
Supplementary Figure 1 | Three-dimensional geometry of the tree used for finite element analysis.
Supplementary Figure 2 | Soil redox potential and meteorological parameters at the field site.
Supplementary Figure 3 | Sensitivity of model results to parametric sweeps across a wide range of possible values for all of the included electrical material properties, except relative permittivity of air.
References
Adkins, C. J. (1959). The Small-Ion Concentration and Space Charge Near the Ground. Q.J R. Met. Soc. 85 (365), 237–252. doi:10.1002/qj.49708536506
Alaoui, A., Caduff, U., Gerke, H. H., and Weingartner, R. (2011). Preferential Flow Effects on Infiltration and Runoff in Grassland and forest Soils. Vadose Zone J. 10 (1), 367–377. doi:10.2136/vzj2010.0076
Aller, R. C. (1994). Bioturbation and Remineralization of Sedimentary Organic Matter: Effects of Redox Oscillation. Chem. Geology. 114 (3-4), 331–345. doi:10.1016/0009-2541(94)90062-0
Arnold, H. R., Pierce, E. T., and Whitson, A. L. (1965). The Effect of a Living Tree upon the Fair Weather Potential Gradient. J. Atmos. Terrestrial Phys. 27, 429–430. doi:10.1016/0021-9169(65)90045-0
Bennett, A. J., and Harrison, R. G. (2007). Atmospheric Electricity in Different Weather Conditions. Weather 62, 277–283. doi:10.1002/wea.97
Bertics, V. J., and Ziebis, W. (2009). Biodiversity of Benthic Microbial Communities in Bioturbated Coastal Sediments is Controlled by Geochemical Microniches. ISME J. 3, 1269–1285. doi:10.1038/ismej.2009.62
Bespalov, V. A., Zhulin, I. B., and Taylor, B. L. (1996). Behavioral Responses of Escherichia coli to Changes in Redox Potential. Proc. Natl. Acad. Sci. 93, 10084–10089. doi:10.1073/pnas.93.19.10084
Borra, J.-P., Roos, R. A., Renard, D., Lazar, H., Goldman, A., and Goldman, M. (1997). Electrical and Chemical Consequences of point Discharges in a forest during a Mist and a Thunderstorm. J. Phys. D: Appl. Phys. 30 (1), 84–93. doi:10.1088/0022-3727/30/1/011
Bowker, G. E., and Crenshaw, H. C. (2007). Electrostatic Forces in Wind-Pollination—Part 2: Simulations of Pollen Capture. Atmos. Environ. 41, 1596–1603. doi:10.1016/j.atmosenv.2006.10.048
Bradshaw, G. A., and Spies, T. A. (1992). Characterizing Canopy Gap Structure in Forests Using Wavelet Analysis. J. Ecol. 80, 205–215. doi:10.2307/2261007
Brovelli, A., and Cassiani, G. (2011). Combined Estimation of Effective Electrical Conductivity and Permittivity for Soil Monitoring. Water Resour. Res. 47, W08510. doi:10.1029/2011WR010487
Clarke, D., Morley, E., and Robert, D. (2017). The Bee, the Flower, and the Electric Field: Electric Ecology and Aerial Electroreception. J. Comp. Physiol. A. 203, 737–748. doi:10.1007/s00359-017-1176-6
Clarke, D., Whitney, H., Sutton, G., and Robert, D. (2013). Detection and Learning of floral Electric fields by Bumblebees. Science. 340, 66–69. doi:10.1126/science.1230883
Crozier, W. D. (1965). Atmospheric Electrical Profiles below Three Meters. J. Geophys. Res. 70 (12), 2785–2792. doi:10.1029/jz070i012p02785
Cusell, C., Mettrop, I. S., Loon, E. E. v., Lamers, L. P. M., Vorenhout, M., and Kooijman, A. M. (2015). Impacts of Short-Term Droughts and Inundations in Species-Rich Fens during Summer and winter: Large-Scale Field Manipulation Experiments. Ecol. Eng. 77, 127–138. doi:10.1016/j.ecoleng.2015.01.025
Epps, T. W., and Singleton, K. J. (1986). An Omnibus Test for the Two-Sample Problem Using the Empirical Characteristic Function. J. Stat. Comput. Simulation. 26, 177–203. doi:10.1080/00949658608810963
Escalante-Minakata, P., Ibarra-Junquera, V., Rosu, H. C., De León-Rodriquez, A., and González-García, R. (2009). Online monitoring of Mezcal Fermentation Based on Redox Potential Measurements. Bioprocess Biosyst. Eng. 32, 47–52. doi:10.1007/s00449-008-0219-3
Fdez-Arroyabe, P., Kourtidis, K., Haldoupis, C., Savoska, S., Matthews, J., Mir, L. M., et al. (2021). Glossary on Atmospheric Electricity and its Effects on Biology. Int. J. Biometeorol. 65 (1), 5–29. doi:10.1007/s00484-020-02013-9
Feynman, R. P., Leighton, R. B., and Sands, M. L. (1964). The Feynman Lectures on Physics: Electromagnetism and Matter (Vol. 2). Addison-Wesley Publishing Company.
Gora, E. M., and Yanoviak, S. P. (2015). Electrical Properties of Temperate forest Trees: a Review and Quantitative Comparison with Vines. Can. J. For. Res. 45 (3), 236–245. doi:10.1139/cjfr-2014-0380
Greggers, U., Koch, G., Schmidt, V., Dürr, A., Floriou-Servou, A., Piepenbrock, D., et al. (2013). Reception and Learning of Electric fields in Bees. Proc. R. Soc. B. 280 (1759), 20130528. doi:10.1098/rspb.2013.0528
Hammer, Ø., Harper, D. A., and Ryan, P. D. (2001). PAST: Paleontological Statistics Software Package for Education and Data Analysis. Palaeontol. electronica. 4 (1), 9. Available at: http://palaeo-electronica.org/2001_1/past/issue1_01.htm
Harrison, R. G., and Nicoll, K. A. (2018). Fair Weather Criteria for Atmospheric Electricity Measurements. J. Atmos. Solar-Terrestrial Phys. 179, 239–250. doi:10.1016/j.jastp.2018.07.008
Higazi, K. A., and Chalmers, J. A. (1966). Measurements of Atmospheric Electrical Conductivity Near the Ground. J. Atmos. Terrestrial Phys. 28 (3), 327–330. doi:10.1016/0021-9169(66)90042-0
Hogg, A. R. (1939). The Conduction of Electricity in the Lowest Levels of the Atmosphere. Memoires Commonw. Observatory. 7, 1–24. Available at: http://adsabs.harvard.edu/pdf/1939MmMtS...7....1H
Holthusen, D., Brandt, A. A., Reichert, J. M., and Horn, R. (2018). Soil Porosity, Permeability and Static and Dynamic Strength Parameters under Native forest/grassland Compared to No-Tillage Cropping. Soil Tillage Res. 177, 113–124. doi:10.1016/j.still.2017.12.003
Hunting, E. R., Harrison, R. G., Bruder, A., van Bodegom, P. M., van der Geest, H. G., Kampfraath, A. A., et al. (2019). Atmospheric Electricity Influencing Biogeochemical Processes in Soils and Sediments. Front. Physiol. 10. doi:10.3389/fphys.2019.00378
Hunting, E. R., Matthews, J., de Arróyabe Hernáez, P. F., England, S. J., Kourtidis, K., Koh, K., et al. (2021). Challenges in Coupling Atmospheric Electricity with Biological Systems. Int. J. Biometeorol. 65 (1), 45–58. doi:10.1007/s00484-020-01960-7
Hunting, E. R., Vijver, M. G., van der Geest, H. G., Mulder, C., Kraak, M. H., Breure, A. M., et al. (2015). Resource Niche Overlap Promotes Stability of Bacterial Community Metabolism in Experimental Microcosms. Front. Microbiol. 6, 105. doi:10.3389/fmicb.2015.00105
Hunting, E. R., Whatley, M. H., van der Geest, H. G., Mulder, C., Kraak, M. H. S., Breure, A. M., et al. (2012). Invertebrate Footprints on Detritus Processing, Bacterial Community Structure, and Spatiotemporal Redox Profiles. Freshw. Sci. 31 (3), 724–732. doi:10.1899/11-134.1
Hunting, E. R., and Kampfraath, A. A. (2013). Contribution of Bacteria to Redox Potential (E H) Measurements in Sediments. Int. J. Environ. Sci. Technol. 10, 55–62. doi:10.1007/s13762-012-0080-4
Hunting, E. R., and Van der Geest, H. G. (2011). Predictability of Bacterial Activity and Denitrification in Aquatic Sediments with Continuous Measurements of Redox Potential. Int. J. Environ. Sci. Technol. 8 (3), 553–560. doi:10.1007/bf03326241
Ibarra-Junquera, V., Escalante-Minakata, P., Murguía, J. S., and Rosu, H. C. (2006). Inferring Mixed-Culture Growth From Total Biomass Data in a Wavelet Approach. Phys. A Stat. Mech. Appl. 370, 777–792. doi:10.1016/j.physa.2006.03.015
Jayaratne, E. R., Ling, X., and Morawska, L. (2011). Role of Vegetation in Enhancing Radon Concentration and Ion Production in the Atmosphere. Environ. Sci. Technol. 45 (15), 6350–6355. doi:10.1021/es201152g
Kubicki, M., Odzimek, A., and Neska, M. (2016). Relationship of Ground-Level Aerosol Concentration and Atmospheric Electric Field at Three Observation Sites in the Arctic, Antarctic and Europe. Atmos. Res. 178-179, 329–346. doi:10.1016/j.atmosres.2016.03.029
Ling, X., Jayaratne, R., and Morawska, L. (2010). Air Ion Concentrations in Various Urban Outdoor Environments. Atmos. Environ. 44 (18), 2186–2193. doi:10.1016/j.atmosenv.2010.03.026
Märcz, F., and Harrison, R. G. (2003). Long-Term Changes in Atmospheric Electrical Parameters Observed at Nagycenk (Hungary) and the UK Observatories at Eskdalemuir and Kew. Annales Geophysicae 21, 2193–2200. doi:10.5194/angeo-21-2193-2003
Morley, E. L., and Robert, D. (2018). Electric Fields Elicit Ballooning in Spiders. Curr. Biol. 28, 2324–2330. doi:10.1016/j.cub.2018.05.057
Newman, D. K., and Banfield, J. F. (2002). Geomicrobiology: How Molecular-Scale Interactions Underpin Biogeochemical Systems. Science. 296, 1071–1077. doi:10.1126/science.1010716
Pett-Ridge, J., and Firestone, M. K. (2005). Redox Fluctuation Structures Microbial Communities in a Wet Tropical Soil. Appl. Environ. Microbiol. 71 (11), 6998–7007. doi:10.1128/aem.71.11.6998-7007.2005
Reiter, R. (1985). Fields, Currents and Aerosols in the Lower Troposphere; published for the division of Atmospheric Sciences, National Science Foundation, Washington, D.C. New Delhi: Amerind Publishing Co. Pvt. Ltd.
Rhebergen, J. B., Lensen, H. A., Schwering, P. B., Marin, G. R., and Hendrickx, J. M. (2002). “Soil Moisture Distribution Around Land Mines and the Effect on Relative Permittivity,” in Detection and Remediation Technologies for Mines and Minelike Targets VII (Orlando, FL: International Society for Optics and Photonics), Vol. 4742, 269–280
Rowland, S. M., Schurch, R., Pattouras, M., and Li, Q. (2015). Application of FEA to Image-Based Models of Electrical Trees with Uniform Conductivity. IEEE Trans. Dielect. Electr. Insul. 22 (3), 1537–1546. doi:10.1109/tdei.2015.004922
Rycroft, M. J., Harrison, R. G., Nicoll, K. A., and Mareev, E. A. (2008). “An Overview of Earth's Global Electric Circuit and Atmospheric Conductivity,” in Planetary Atmospheric Electricity (New York, NY: Springer), 83–105. doi:10.1007/978-0-387-87664-1_6
Suojanen, M., Lederle, C., Heim, R., Keikko, T., Kuusiluoma, S., and Korpinen, L. (2001). The Effect of Trees on Calculation of Electric fields Near 400 kV Transmission Lines. WIT Trans. Model. Simulation. 30, 935–944. doi:10.2495/CMEM010911
Vorenhout, M., van der Geest, H. G., and Hunting, E. R. (2011). An Improved Datalogger and Novel Probes for Continuous Redox Measurements in Wetlands. Inter. J. Environ. Anal. Chem. 91.7-8, 801–810. doi:10.1080/03067319.2010.535123
Williams, E., and Mareev, E. (2014). Recent Progress on the Global Electrical Circuit. Atmos. Res. 135-136, 208–227. doi:10.1016/j.atmosres.2013.05.015
Williams, E., Markson, R., and Heckman, S. (2005). Shielding Effects of Trees on the Measurement of the Earth's Electric Field: Implications for Secular Variations of the Global Electrical Circuit. Geophys. Res. Lett. 32, a–n. doi:10.1029/2005GL023717
Keywords: atmospheric electricity, ions, wavelet variance, potential gradient, redox potential - Eh
Citation: Hunting ER, England SJ and Robert D (2021) Tree Canopies Influence Ground Level Atmospheric Electrical and Biogeochemical Variability. Front. Earth Sci. 9:671870. doi: 10.3389/feart.2021.671870
Received: 24 February 2021; Accepted: 23 June 2021;
Published: 15 July 2021.
Edited by:
Konstantinos Kourtidis, Democritus University of Thrace, GreeceReviewed by:
Francesca Apollonio, Sapienza University of Rome, ItalyAndrea Schievano, University of Milan, Italy
Copyright © 2021 Hunting, England and Robert. This is an open-access article distributed under the terms of the Creative Commons Attribution License (CC BY). The use, distribution or reproduction in other forums is permitted, provided the original author(s) and the copyright owner(s) are credited and that the original publication in this journal is cited, in accordance with accepted academic practice. No use, distribution or reproduction is permitted which does not comply with these terms.
*Correspondence: Ellard R. Hunting, e.r.hunting@bristol.ac.uk; Daniel Robert, d.robert@bristol.ac.uk