- 1Earth and Life Institute, Université Catholique de Louvain, Louvain-la-Neuve, Belgium
- 2Permafrost Research Section, Alfred Wegener Institute Helmholtz Centre for Polar and Marine Research, Potsdam, Germany
- 3Institute of Geosciences, University of Potsdam, Potsdam, Germany
- 4Department of Physical Geography, Stockholm University, Stockholm, Sweden
With permafrost thaw, significant amounts of organic carbon (OC) previously stored in frozen deposits are unlocked and become potentially available for microbial mineralization. This is particularly the case in ice-rich regions such as the Yedoma domain. Excess ground ice degradation exposes deep sediments and their OC stocks, but also mineral elements, to biogeochemical processes. Interactions of mineral elements and OC play a crucial role for OC stabilization and the fate of OC upon thaw, and thus regulate carbon dioxide and methane emissions. In addition, some mineral elements are limiting nutrients for plant growth or microbial metabolic activity. A large ongoing effort is to quantify OC stocks and their lability in permafrost regions, but the influence of mineral elements on the fate of OC or on biogeochemical nutrient cycles has received less attention and there is an overall lack of mineral element content analyses for permafrost sediments. Here, we combine portable X-ray fluorescence (pXRF) with a bootstrapping technique to provide i) the first large-scale Yedoma domain Mineral Concentrations Assessment (YMCA) dataset, and ii) estimates of mineral element stocks in never thawed (since deposition) ice-rich Yedoma permafrost and previously thawed and partly refrozen Alas deposits. The pXRF method for mineral element quantification is non-destructive and offers a complement to the classical dissolution and measurement by optical emission spectrometry (ICP-OES) in solution. Using this method, mineral element concentrations (Si, Al, Fe, Ca, K, Ti, Mn, Zn, Sr and Zr) were assessed on 1,292 sediment samples from the Yedoma domain with lower analytical effort and lower costs relative to the ICP-OES method. The pXRF measured concentrations were calibrated using alkaline fusion and ICP-OES measurements on a subset of 144 samples (R2 from 0.725 to 0.996). The results highlight that i) the mineral element stock in sediments of the Yedoma domain (1,387,000 km2) is higher for Si, followed by Al, Fe, K, Ca, Ti, Mn, Zr, Sr, and Zn, and that ii) the stock in Al and Fe (598 ± 213 and 288 ± 104 Gt) is in the same order of magnitude as the OC stock (327–466 Gt).
Introduction
The ice-rich deposits of the Yedoma domain hold more than 25% (213 Gt) of the frozen organic carbon (OC) of the northern circumpolar permafrost region, while covering only about 8% of its total soil area (c. 1.4 of 17.8 million km2; Schirrmeister et al., 2013; Hugelius et al., 2014; Strauss et al., 2017). This carbon- and ice-rich region is particularly vulnerable to abrupt thawing processes (Nitzbon et al., 2020; Turetsky et al., 2020). This is the reason why Yedoma deposits are considered as a potential “tipping element” for future climate warming (Lenton, 2012). The key features of Yedoma deposits relative to other permafrost deposits are i) their ground ice properties, with 50–90% volume percent ice (Schirrmeister et al., 2013) and ii) their large spatial extent and thickness, resulting in a large total volume (Strauss et al., 2017). Thawing of ice-rich sediments has severe geomorphological consequences for the landscape (Kokelj and Jorgenson, 2013). Striking examples are collapsing river and coastal bluffs (Günther et al., 2015; Kanevskiy et al., 2016; Fuchs et al., 2020). But a spatially more important process is thermokarst lake formation, caused by surface subsidence due to excess ground ice melting in Yedoma deposits and leading to the formation of vast thermokarst depressions. The process not only affects the superficial soil horizons but also deep mineral horizons (Strauss et al., 2013; Walter Anthony et al., 2018). Thermokarst processes were highly active during the Deglacial and early Holocene period (Walter et al., 2007; Brosius et al., 2021) and shaped the Yedoma domain region with numerous lakes. Following drainage of lakes, a widespread process across lake-rich lowlands (Grosse et al., 2013), renewed permafrost aggradation and new soil development could occur in the drained thermokarst lake basins also called Alas. The Yedoma domain therefore includes Yedoma deposits never affected by thaw and frozen deposits that accumulated after Yedoma degradation in Alas landforms (Olefeldt et al., 2016; Strauss et al., 2017).
In permafrost soils, between ∼30% (Mueller et al., 2015) and ∼80% (Dutta et al., 2006) of the total soil OC is associated to minerals through various mechanisms, as detailed below. It is well known that interactions between minerals and OC can have a stabilizing effect on OC (Kaiser and Guggenberger, 2003; Lutzow et al., 2006; Kögel-Knabner et al., 2008; Gentsch et al., 2018; Wang et al., 2019). Mineral-protected OC is less bioavailable than mineral free OC (Schmidt et al., 2011; Kleber et al., 2015), thereby contributing to the long term carbon storage (Hemingway et al., 2019). Mineral protection of OC includes aggregation, adsorption and/or complexation or co-precipitation processes (Kaiser and Guggenberger, 2003). These protection mechanisms involve i) clay minerals, Fe-, Al-, Mn-oxy-(hydr)oxides, or carbonates (aggregation); ii) clay minerals and Fe-, Al-, Mn-oxy-(hydr)oxides using polyvalent cation bridges such as Fe3+, Al3+, Ca2+ or Sr2+ (adsorption); or iii) Fe3+, Fe2+ and Al3+ ions (complexation). In addition, mineral elements (e.g., Si, Al, Fe, Ca, K, Mn, Zn, Sr) drive nutrient supply to plants or microorganisms, including algae such as diatoms. Nutrient supply is essential for plants growth and/or microbes metabolic activity and therefore indirectly influences C storage or degradation. It remains however unclear how mineral-OC interactions and nutrient supply will evolve upon permafrost thaw (Opfergelt, 2020), especially in ice-rich permafrost regions. This is mainly due to a lack of knowledge about the mineral element content in permafrost regions, relative to the well-known OC stocks in permafrost soils (Harden et al., 2012; Hugelius et al., 2014; Strauss et al., 2017; Hugelius et al., 2020; Kuhry et al., 2020) and the increasing knowledge on N stocks (Fuchs et al., 2018; Fuchs et al., 2019; Fouché et al., 2020; Hugelius et al., 2020).
To improve our understanding on the potential effects mineral elements can have on OC from permafrost regions, it is essential to have better knowledge on mineral element stocks in these regions, and on the changes in mineral element stocks upon thawing with changing conditions for mineral weathering (Zolkos and Tank, 2020). Ice-rich permafrost regions are particularly well suited areas to study the impact of thawing processes on the mineral elemental stocks in deposits. Indeed, “never” (since deposition) thawed deposits can be compared with previously thawed deposits resulting from thermokarst processes during warmer and wetter periods in the Deglacial and early Holocene periods (13–9 ka BP; Morgenstern et al., 2013; Walter et al., 2007). Assessing the evolution of the mineral element stocks between never thawed (since deposition) and previously thawed, refrozen as well as newly formed deposits will contribute to a better understanding of the impact of past thawing processes on the evolution of the pool of mineral elements available for mineral-OC interactions. It also provides insights into how ongoing permafrost thaw and thermokarst processes may impact mineral elements and what the potential implications are for the fate of OC in ice-rich deposits (Strauss et al., 2017). This is particularly relevant given that thermokarst processes are projected to spread across the Arctic and will potentially unlock additional OC stocks (Lacelle et al., 2010; Abbott and Jones, 2015; Schneider von Deimling et al., 2015; Nitzbon et al., 2020; Turetsky et al., 2020).
The objective of this study is to provide a method to assess the mineral element content on a large sample set in order to cover the different types of deposits from the Yedoma domain. Using this method, we provide a Yedoma domain Mineral Concentrations Assessment (YMCA) dataset for ten mineral elements (Si, Al, Fe, Ca, K, Ti, Mn, Zn, Sr, and Zr) and the stocks for these mineral elements in deposits from ice-rich permafrost regions. This assessment comprises “never” thawed since syngenetic freezing Yedoma Ice Complex deposits (in the following referred as Yedoma) and at least once previously thawed and then refrozen drained thermokarst lake basin deposits (in the following referred as Alas).
Materials
Environmental Settings
The Yedoma domain is part of the permafrost region characterized by organic- and ice-rich deposits as well as thermokarst features. Today, the Yedoma domain predominantly covers areas in Siberia and Alaska (Figure 1) which were not covered with ice sheets during last glacial period (110 ka – 10 ka BP; Schirrmeister et al., 2013).
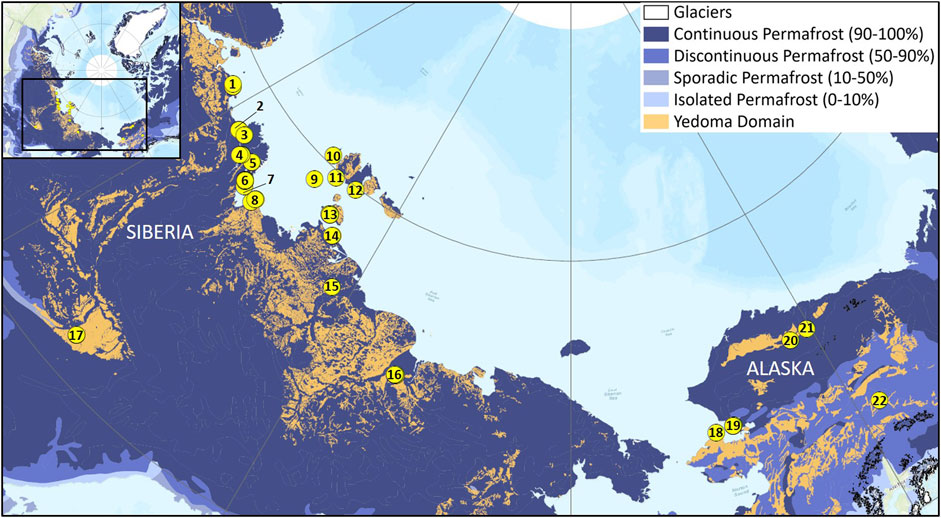
FIGURE 1. Studied permafrost sites from the Yedoma domain. 1) Cape Mamontov Klyk; 2) Nagym (Ebe Sise Island); 3) Khardang Island; 4) Kurungnakh Island; 5) Sobo Sise Island; 6) Bykovsky Peninsula; 7) Muostakh Island; 8) Buor Khaya Peninsula; 9) Stolbovoy Island; 10) Belkovsky Island; 11) Kotel’ny Island; 12) Bunge Land; 13) Bol’shoy Lyakhovsky Island; 14) Oyogos Yar coast; 15) Kytalyk; 16) Duvanny Yar; 17) Yukechi; 18) Kitluk; 19) Baldwin Peninsula; 20) Colville; 21) Itkillik; 22) Vault Creek tunnel. Permafrost coverage layer is derived from the Circum-Arctic map of permafrost and ground-ice conditions shapefile (Brown et al., 1997). Yedoma domain coverage from Strauss et al. (2016) (Database of Ice-Rich Yedoma Permafrost - IRYP).
Recent estimates indicate that the current Yedoma domain has an extent of ∼1.4 million km2 and contains between 327 and 466 Gt OC (Strauss et al., 2017). Frozen deposits from the Yedoma domain alone store probably at least as much carbon as the tropical forest biomass (Lai, 2004). These deposits were formed by long-term continuous sedimentation and syngenetic freezing. Yedoma deposits were first described as homogeneous silty fine, ice-, and organic-rich sediments derived from aeolian processes (i.e., loess or loess-related deposits) (Pewe and Journaux, 1983; Tomirdiaro and Chernen’kiy, 1987; Murton et al., 2015). Current evidence indicates that depositional processes are polygenetic including alluvial and aeolian deposition and re-deposition, as well as in situ weathering during the late Pleistocene cold stages (Konishchev and Rogov, 1993; Schirrmeister et al., 2013). Despite evidence of homogeneity of Yedoma deposit aggradation, grain-size analyses show the large diversity of Yedoma deposits, pointing at multiple origins and transport pathways of sediments as well as a diverse interplay of (post)depositional sedimentary processes (Strauss et al., 2012; Schirrmeister et al., 2020; Sizov et al., 2020). For tens of millennia, continuous sedimentation led to the accumulation of several tens of meters thick permafrost deposits with characteristic syngenetic ice-wedge formation. Harsh, cold late Pleistocene winter climate triggered the formation of vertical frost cracks within surface deposits in which snow melt water percolated during spring and refroze into vertical ice veins in the permafrost. Over time and with annual repetition of this process, many meters wide and up to 40 m high ice-wedges were formed. Those large volumes of icy sediments together with the rise in temperature during the Holocene Thermal Maximum (HTM between 11 and 5 ka BP depending on the region; HTM reached at 7.6–6.6 ka BP in North Alaska and variable with time in Siberian regions) (Velichko et al., 2002; Kaufman et al., 2004; Porter and Opel, 2020) lead to a vast reshaping of the landscape with formation of thermokarst lakes and drained Alas basins (Grosse et al., 2013). Alas deposits in drained thermokarst lake basins are composed of reworked Yedoma deposits as well as Holocene accumulation during sub-aquatic and sub-aerial phases (Strauss et al., 2017; Windirsch et al., 2020). The Yedoma domain area includes 30% of unthawed Yedoma deposits composed of homogeneous silty deposits with polygenetic origins (eolian, alluvial or colluvial deposition) between large ice-wedges. Alas deposits have experienced thawing processes and drainage during early Holocene until more recently and account for 56% of the Yedoma domain area. Deltaic deposits (4%) as well as lakes and rivers (10%) complete the Yedoma domain deposits area distribution (Figure 2).
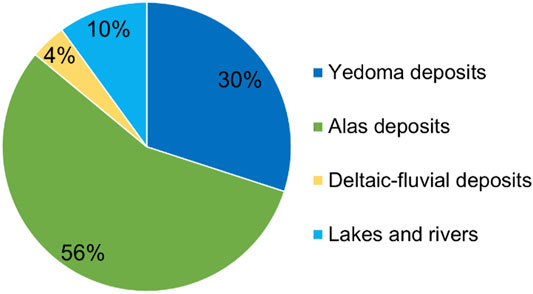
FIGURE 2. Distribution of the area coverage (percentage) in the Yedoma domain region. The total Yedoma domain area is estimated at 1,387,000 km2 (based on Strauss et al. (2017)).
In this study, mineral element stocks are evaluated in Yedoma and Alas deposits (for a mean deposit thickness of 19.6 and 5.5 m, respectively; Figure 3A), but the evaluation does not include sediments underlying extant thermokarst lakes, active layer sediments or fluvial sediments (Figure 3B).
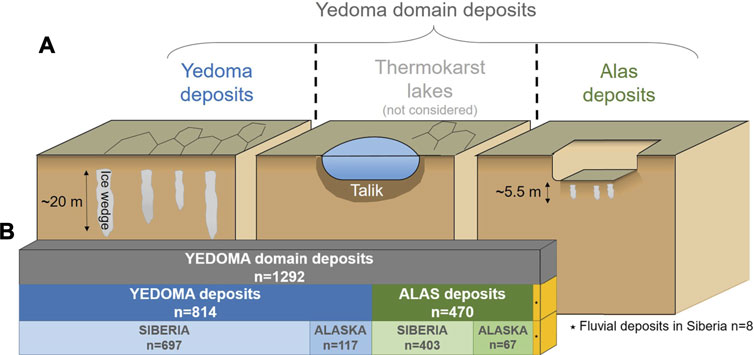
FIGURE 3. (A) Schematic view of Yedoma and Alas (drained thermokarst lake basins) deposits considered for mineral element stock calculations (modified from Nitzbon et al. (2020)); sediments underlying thermokarst lakes or river floodplains are excluded. (B) Number of samples from Yedoma domain deposits included in the Yedoma domain Mineral Concentrations Assessment (YMCA) dataset (see Yedoma Domain Mineral Concentrations Assessment Dataset).
Sample Collection
This large-scale mineral element concentration assessment from Yedoma and Alas deposits is based on samples collected in two major Arctic regions, Alaska and Siberia. More specifically, the dataset includes 22 locations and compiles 75 different profiles from West, North and Interior Alaska, the Kolyma region, the Indigirka region, the New Siberian Archipelago, the Laptev Sea coastal regions, and Central Yakutia (Figure 1). About 64% of the profiles are from Yedoma deposits, 33% from Alas deposits and 3% from deltaic deposits, and the sites cover a range of geomorphological settings (Supplementary Table S1). For each location, Yedoma and Alas deposits profiles were sampled if both types of deposit were present. In total, 1,292 different samples were analyzed for their mineral element concentration. Sampling strategies were as follows: during the cold season, frozen samples were collected by drilling from the surface down with drilling rigs, whereas during the mid-summer season, drilling was performed in frozen ground below the existing thawed active layer. Cliffs or headwall exposures along coasts, rivers, or lakes were cleaned and frozen in situ sediments sampled with hammer and ax. For headwall or cliff sampling, sub-profiles from different vertical exposures were included when needed to reconstruct a complete stratigraphical composite profile. Additional information on specific site sampling techniques can be found in the reference papers cited in Table 1. Recovered samples were air- or freeze-dried before being stored and archived. The number of samples collected in each location and the number of samples analyzed with the different methods presented in Methods are provided in Supplementary Table S1.
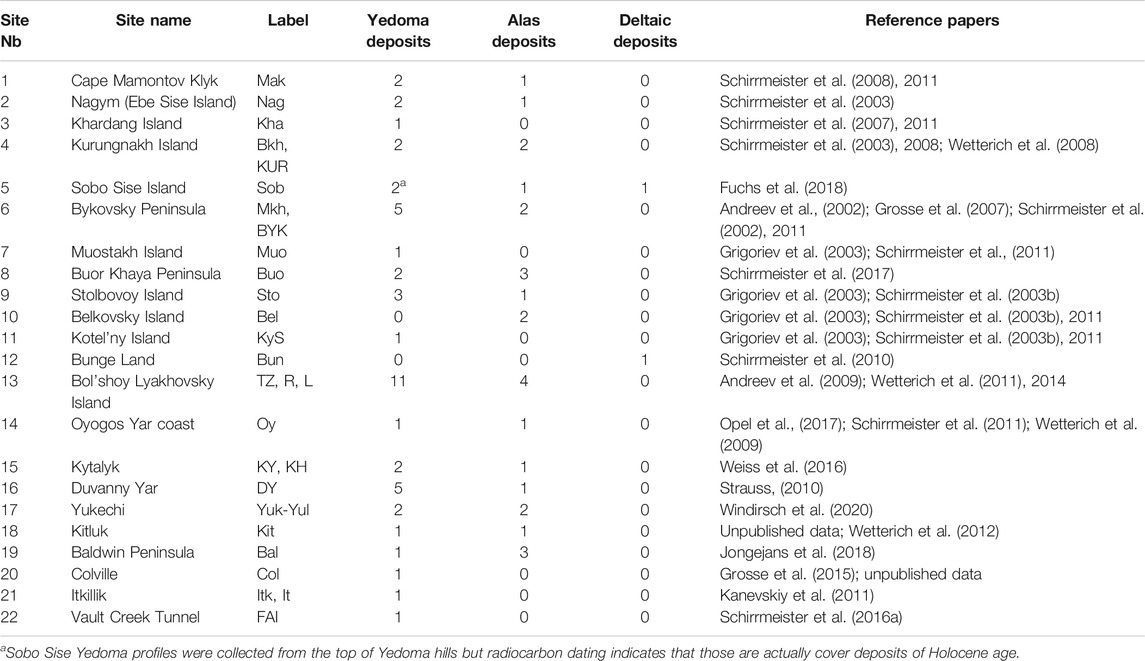
TABLE 1. Studied locations from the Yedoma domain with associated labels, the number of profiles assessed for each type of deposits and the associated reference papers for site description. The site numbers (Site Nb) 1–17 are from Siberia, and 18–22 are from Alaska (located on the map in Figure 1). The labels are used in the Yedoma domain Mineral Concentrations Assessment (YMCA) dataset (doi:10.1594/PANGAEA.922724) (see Yedoma Domain Mineral Concentrations Assessment Dataset for details). Information on site geomorphology, number of samples collected in each location, and samples selected for specific analyses (Methods) are given in Supplementary Table S1
Methods
Total Elemental Analysis by ICP-OES Measurement After Alkaline Fusion
Inductively coupled plasma optical-emission spectrometry (ICP-OES) is a classical method to assess mineral element concentrations in solutions from the environment accurately. Solid phases such as soil samples should be first digested prior to ICP-OES analysis. Here, soils were digested by alkaline fusion. Briefly, air- or freeze-dried soil samples were carefully milled for homogenization (agate mill). We mixed a portion of the milled sample (80 mg) with Lithium metaborate and Lithium tetraborate and heated it up to 1,000°C for 10 min. Then we dissolved the fusion bead in HNO3 2.2N at 80°C and stirred until complete dissolution (Chao and Sanzolone, 1992). We measured the mineral element concentrations in that solution by ICP-OES (iCAP 6500 Thermo Fisher Scientific). We assessed the loss on ignition at 1,000°C, the sum of oxides was between 98 and 102%, and the total element content in soils is expressed in reference to the soil dry weight at 105°C. To assess the accuracy (i.e., trueness and precision) of the method, the analytical measurement was validated by repeated measurements on three certified materials; i) USGS basalt reference material BHVO-2 (Wilson, 1997), ii) GBW07401 podzolitic soil, and iii) GBW07404 limy-yellow soil (National Research Center for CRM, 1986; Supplementary Table S2). To assess the precision of the method on a matrix similar to samples from the Yedoma domain, we conducted three repetitions on three individual samples from Yedoma and Alas deposits. For each mineral element, standard deviations on the repetitions, expressed in mg kg−1, are available in Table 2.

TABLE 2. Precision of the element concentrations expressed as pooled standard deviations (i.e., two pooled standard deviations, expressed in mg kg−1) for the 10 elements considered. The values are based on three repetitions of three identical samples for both ICP-OES and raw concentrations from pXRF method. The coefficient of variation (CV; expressed in %), defined as the ratio of the standard deviation to the mean is also provided.
Out of the 1,292 deposit samples retrieved from the Yedoma domain (Sample Collection), a subset of 144 samples was analyzed by ICP-OES after alkaline fusion to determine their mineral element concentrations (sample list is provided in Supplementary Table S3). We used this subset of analysis to calibrate element concentrations measured by portable X-ray fluorescence (Total Elemental Analysis by ex situ Direct Measurement by Portable X-Ray Fluorescenc) for accurate determination of concentration values. For this first assessment, we measured the following elements on the subset of samples (except for Zn, which was measured on 119 out of the 144 samples): Si, Al, Fe, Ca, Mg, Cr, Ba, K, Ti, P, Cu, Mn, Ni, Zn, Sr, and Zr.
Total Elemental Analysis by ex situ Direct Measurement by Portable X-Ray Fluorescence
X-ray fluorescence (XRF) spectrometry is an elemental analysis technique with broad environmental and geologic applications, from pollution assessments to mining industries (Weindorf et al., 2014a; Rouillon and Taylor, 2016; Young et al., 2016; Ravansari et al., 2020). In addition, there is a growing use of portable XRF for soil science (McLaren et al., 2012; Ravansari and Lemke, 2018). Portable XRF is used primarily for solid elemental analysis (soils, sediments, rocks or even plastics) but can also deal with oil chemical characterization (Weindorf et al., 2014a). XRF is based on the principle that individual atoms emit photons of a characteristic energy or wavelength upon excitation by an external X-ray energy source. By counting the number of photons of each energy emitted from a sample, the elements present may be identified and quantified (Anzelmo and Lindsay, 1987; Supplementary Figure S1). XRF-scanning results represent elements intensities in “counts per second” (cps) which are proportional to chemical concentrations in the sample but depend also on sample properties (Röhl and Abrams, 2000), ice and water content (Tjallingii et al., 2007; Weindorf et al., 2014b) and interactions between elements called “matrix effect” (Weltje and Tjallingii, 2008; Fritz et al., 2018).
In situ pXRF measurement often involves variability from uncontrolled environmental factors, such as water content, organic matter content or sample heterogeneity (Shand and Wendler, 2014; Weindorf et al., 2014b; Ravansari et al., 2020). To avoid such variability in water content, measurements were performed on dried samples in laboratory (ex situ) conditions with the handheld device in the laboratory. The particle size distribution of these Yedoma domain deposits (described in reference papers from Table 1) is below 2 mm: therefore no sieving was necessary. For the pXRF measurement, the dried sample is placed on a circular plastic cap (2.5 cm diameter) provided at its base with a transparent thin film (prolene 4 µm). To avoid the underestimation of the detected intensities, sample thickness in the cap needs to be higher than 5 mm to 2 cm, depending on the element of interest (Ravansari et al., 2020). For a precise measurement, the sample thickness in the cap is set to >2 cm. Above 2 cm, the width is considered as “infinitely thick” for all elements. Measurements on the 1,292 samples from the Yedoma domain were performed using the pXRF device Niton xl3t Goldd+ (Thermo Fisher Scientific), which has two specific internal calibration modes called mining and soil. Each internal calibration is dealing with different energy range and filters to scan the complete energetic spectrum from low to high-energetic fluorescence values. Both modes were used on each sample and depending on the element, the calibration with the best correlation with the ICP-OES method (Total Elemental Analysis by ICP-OES Measurement After Alkaline Fusion) was kept for further calculations (Mineralogy by X-Ray Diffraction). To standardize the analysis, total time of measurement was set to 90 s. We conducted the analysis in laboratory conditions, using a lead stand to protect the operator from X-rays.
In theory, the pXRF device used to generate this dataset can measure simultaneously elements of atomic mass from Mg to U. Because ambient air annihilates fluorescence photons that do not have enough energy, low atomic mass elements from Na and lighter cannot be quantified by pXRF. Note that Na quantification would be possible in controlled void conditions during analysis (Weindorf et al., 2014a). In this study, we focussed on the concentrations in 16 elements (Si, Al, Fe, Ca, Mg, Cr, Ba, K, Ti, P, Cu, Mn, Ni, Zn, Sr, Zr) by pXRF and by the ICP-OES method (Total Elemental Analysis by ICP-OES Measurement After Alkaline Fusion) to allow for quality check, calibration and correction. Some elements are at the limit of detection (LOD) for pXRF device (e.g., Cu, Ni). The LOD was reached when the sample was lacking a specific mineral element. LOD concentrations were set to 0.7 times the minimal concentration measured for this element, which is an arbitrary number but conventionally used for data statistical treatment (Reimann et al., 2008). Depending on the considered element, pXRF measured concentrations were highly precise but not always accurate (far from the true value) (Figure 4). Trueness was achieved after correction using a regression with concentration values measured by the ICP-OES method to avoid systematic bias (Figure 4; Linear Regression for Accurate Mineral Element Concentration Measurements). Using a well-defined regression to correct pXRF measurements for trueness allowed using the pXRF method to measure mineral element concentrations on a large number of Yedoma and Alas sample (n = 1,292), i.e., providing a valuable method to assess the mineral element content on a large sample set (Figure 4). Raw pXRF concentrations cannot be used for absolute quantification if not corrected with a reliable and accurate method. Here, only pXRF concentration values corrected using a well-defined regression were used (Linear Regression for Accurate Mineral Element Concentration Measurements). Because of poor correlation (R2 < 0.5) between pXRF and accurate ICP-OES method for Mg, Cr, Ba, P, Cu and Ni, as discussed in Linear Regression for Accurate Mineral Element Concentration Measurements, these elements were not considered further in this study.
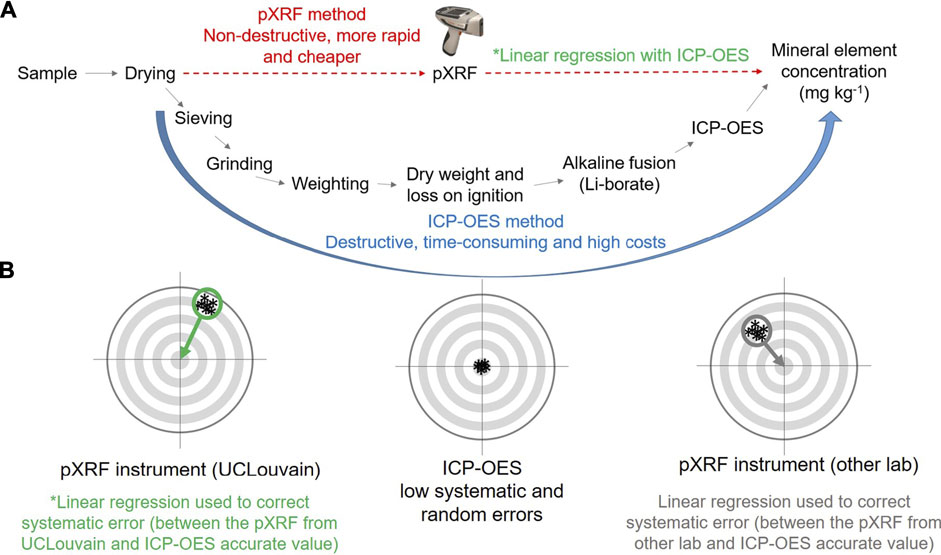
FIGURE 4. (A) Comparison between two methods to assess mineral element concentrations in deposit samples: the inductively coupled plasma optical-emission spectrometry (ICP-OES) method (large blue arrow) is destructive and time-consuming (involving several steps), whereas the portable X-ray fluorescence (pXRF) method (dotted red arrows) is non-destructive and allows a fast and reliable determination of element concentrations on a large set of samples in a cost-effective way when a correction with a linear regression is applied. (B) The pXRF bias (i.e., systematic error) is corrected with a linear regression specific to each pXRF device. Portable XRF devices from each lab require their own linear regression to correct for accurate value. The linear regression is obtained from a selection of samples (here 11 % of the samples) analysed by both methods, pXRF and ICP-OES.
To assess the precision (i.e., random errors) of the pXRF method, three repetitions were conducted on three individual samples from different locations. Between each repetition, instrument/sample repositioning is used to mitigate the “nugget effect” due to heterogeneity in the sample (Ravansari and Lemke, 2018). Pooled standard deviations (SDpooled, weighted average of standard deviations), expressed in mg kg−1, of the repetitions for the ten elements used for stock calculation (Linear Regression for Accurate Mineral Element Concentration Measurements) are available in Table 2. Given the influence of sample matrix on pXRF measurements, the precision of the pXRF method was also evaluated based on three to five repetitions on 20 individual samples and on average 2.6 times larger than based on three repetitions on three samples (Supplementary Table S4). The coefficient of variation was 20% smaller for Al but 6.5 times larger for Ca based on 20 samples. To ensure a cautious evaluation of the dataset, we decided to report the precision on the data in Supplementary Presentation S1 based on the precisions from Table 2 for ICP-OES measurements, and from Supplementary Table S4 for pXRF measurements to use the largest set of sample with precision data available.
Mineralogy by X-Ray Diffraction
The X-ray diffraction (XRD) method allows the characterization of the presence of crystalline mineral phases. We assessed the mineralogy of 39 finely ground bulk samples and six clay fraction samples (samples selected in Siberia and Alaska detailed in Supplementary Table S1). The mineralogy of bulk samples was determined on powder (Cu Kα, Bruker Advance D8). Clay fraction mineralogy was assessed after K+ and Mg2+ saturation, ethylene glycol (eg) solvation and thermal treatments at 300 and 550°C (Robert and Tessier, 1974). The clay size fraction (<2 µm) was recovered after sonication, sieving at 50 µm to remove the sand fraction, and dispersion with Na+-resins to separate silt (2–50 µm) and clay fractions (Rouiller et al., 1972).
Mean-Bootstrapping Technique for Mineral Element Stocks Calculations
Calculations of mineral element stocks are based on a mean-bootstrapping technique. From the corrected mineral element concentrations obtained via linear regression of pXRF measurements (YMCA dataset; Yedoma Domain Mineral Concentrations Assessment (YMCA) Dataset), the aim is to calculate the mineral element stock in Yedoma and Alas deposits individually, in order to estimate total mineral element budget at the Yedoma domain scale. This technique has been used to estimate OC budget from Yedoma and Alas deposits (Strauss et al., 2013) and was further improved by Jongejans and Strauss (2020). Because Yedoma domain deposits contain large ice volumes, stock calculations need to take into account not only the bulk density (BD) of the samples but also total thickness and total wedge-ice volume (WIV) of the deposits. In order to avoid overestimation of the mineral stocks, WIV was subtracted from the total Yedoma domain deposit volume, since the proportion of mineral elements locked inside ice-wedges is negligible. Indeed, Opel et al. (2018) indicate the minor contribution of mineral particles during ice-wedge formation. Fritz et al. (2011) further indicate that ion concentrations in ice is generally low, dominated by HCO3− (55%) and Cl− (37%) for anions and Na+ (58%) and Ca2+ (30%) for cations. Note that a flush of highly labile mineral elements (e.g., Na+, Ca2+, Cl−) locked inside ice-wedges may increase nutrient supply for a short time scale upon Yedoma deposits degradation compared to long-term solid-liquid interactions upon thaw. Assumptions and calculations for BD determination, WIV estimations, deposits thickness, and coverage are fully explained in Strauss et al. (2013) and are summarized here. The bootstrapping statistical method use resampled (10,000 times) observed values (mineral element concentrations, BD, WIV and deposits thickness (Supplementary Figure S2)) and derive the mean afterward. The BD determination for each sample was obtained by using an inverse relationship with porosity (
whereas
If neither BD or TOC is available, BD is fixed to 0.88 103 and 0.93 103 kg m−3 for Yedoma and Alas deposits, respectively, i.e., the mean BD measured in such deposits (Strauss et al., 2013). These values are comparable with other studies on BD of Yedoma deposits (0.98 103 kg m−3; Dutta et al., 2006). The WIV estimations were performed using ice-wedge width from field measurements (Strauss et al., 2013), ice-wedge polygon size determinations from high-resolution satellite, and additional geometrical tools (assuming ice-wedges have an isosceles triangular or rectangular shape depending on the type of ice-wedge; see Strauss et al. (2013), Ulrich et al. (2014)). Thickness used for mineral element stock estimations in Yedoma domain deposits are based on mean profile depths of the sampled Yedoma (n = 19) and Alas (n = 10) deposits (Table 3; Strauss et al., 2013). Since the 10,000 step bootstrapping technique randomly picks one thickness at a time with replacement, we evaluated the stock estimation for a mean thickness of 19.6 m deep in Yedoma deposits and 5.5 m deep in Alas deposits.
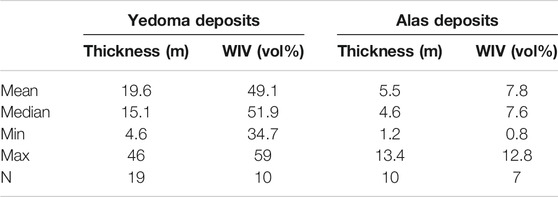
TABLE 3. Summary of key parameters for Yedoma deposits and Alas deposits. Parameters include thicknesses (in meters) and wedge ice volume (WIV, in %) from Strauss et al. (2013). Mineral element stock estimations are based on a mean thickness of 19.6 m for Yedoma and 5.5 m for Alas deposits.
The core Yedoma domain extent was estimated to ∼1,387,000 km2, based on digital Siberian Yedoma region map (Romanovskii, 1993) and the distribution of Alaskan ice-rich silt deposits equivalent to Yedoma (Jorgenson et al., 2008). The Yedoma deposit extent is estimated to 410,000 km2, i.e., 30% of the Yedoma domain, based on the fact that 70% of the Yedoma domain area is affected by degradation (Strauss et al., 2013). Considering 10% of the area of the Yedoma domain covered with lakes and rivers, 4% covered with other deposits including deltaic and fluvial unfrozen sediments, this leaves 56% (780,000 km2) of the Yedoma domain covered by frozen thermokarst deposits in drained thermokarst lakes (Figure 2). Using these parameters, the overall mineral element stock is determined with Eq. 3 included into the bootstrapping calculation:
Because Yedoma and Alas deposits have different properties for BD, ice content, and deposit thickness, mineral element stocks were estimated for each deposit type individually. For each bootstrapping step, the sample mineral element concentration and specific BD were paired (as they are not independent) and a specific stock was estimated based on one value for WIV and thickness. The stock was then multiplied by total coverage for total mineral stock estimation to the Yedoma domain scale. From these input parameters, multiple mineral element stocks were computed, one for each bootstrapping step. Eventually, from those multiple steps (n = 10,000), a mineral element stock distribution was estimated from which the mean represents the best stock estimation of the considered mineral element. The error estimates in this study represent 2 standard deviations (mean ±2σ), with the assumption of a normal distribution. Computations were performed using R software (boot package; R Core Team, 2018). Supplementary information on input parameters (deposit thickness, WIV) are available in Supplementary Figure S2.
Data Processing and Results
Linear Regression for Accurate Mineral Element Concentration Measurements
Mineral element concentrations were measured with both the ICP-OES method (Total Elemental Analysis by ICP-OES Measurement After Alkaline Fusion) and the pXRF method (Total Elemental Analysis by ICP-OES Measurement After Alkaline Fusion) on a subset of samples (n = 144) from Yedoma domain deposits (Supplementary Tables S1, S3). Linear regressions can be computed from this subset of data and parameters of the regression are estimated and based on a robust linear regression (alpha = 0.95; Table 4). Among the 16 mineral elements measured, 10 elements (Si, Al, Fe, Ca, K, Ti, Mn, Zn, Sr and Zr) display high correlations between pXRF and ICP-OES method with R2 ranging from 0.725 (Al) to 0.996 (Ca; Table 4). Linear regression plots for these elements are presented in Supplementary Presentation S1. The other six elements (Mg, Ba, Cr, Cu, Ni and P) present weak (R2 < 0.5) or no correlations between the two methods. According to these correlations, the mineral element stock quantification was performed on the 10 elements reliably measured by pXRF (Si, Al, Fe, Ca, K, Ti, Mn, Zn, Sr and Zr), and the other six elements are not discussed further in this study.

TABLE 4. Robust linear regression adjusted R2 (alpha = 0.95) between concentrations measured from pXRF and ICP-OES method in Yedoma domain deposits (n = 144 for all elements, except for Zn where n = 119).
The uncertainty based on three identical samples on the ICP-OES measurement after alkaline fusion was lower than pXRF measurements, except for Ca, K, and Zr (Table 2). Nonetheless, the advantages of a non-destructive, rapid and cheaper method predominate for a large-scale mineral concentration assessment given that the coefficient of variation (2.1–8.7%) on the pXRF measurement was satisfying to differentiate between high and low concentrations values measured in these deposits (i.e., comparing the horizontal error bar with the total range of values represented on the X-axis in Supplementary Presentation S1).
In this dataset, the risk of overestimating the element concentration by pXRF in organic-rich samples (Shand and Wendler, 2014) is limited. Among the 1,292 Yedoma and Alas samples used in this study to build the YMCA dataset (Yedoma Domain Mineral Concentrations Assessment Dataset), less than 0.5% of the samples were characterized by TOC content similar to or higher than 40 wt% (Table 5). Overestimation of the concentration was only observed for a single sample for Fe (Supplementary Presentation S1C) and for three samples for Ca (Supplementary Presentation S1D) out of the 144 samples from the subset (for samples with TOC content >40 wt%), and not observed for Si, Al, K, Ti, Mn, Zn, Sr and Zr.
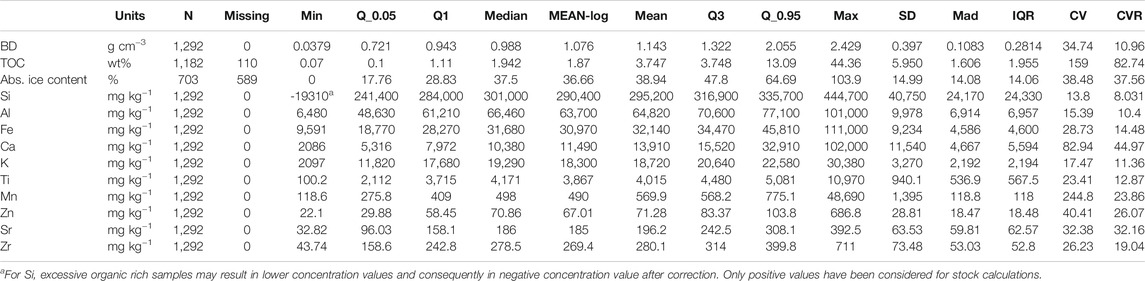
TABLE 5. Statistical summary of YMCA dataset (n = 1,292) for bulk density (BD), total organic carbon content (TOC), absolute ice content, and corrected mineral element concentrations (Si, Al, Fe, Ca, K, Ti, Mn, Zn, Sr and Zr) in Yedoma domain deposit samples (grouping Yedoma and Alas deposits together). Columns stand for N (number of data); Missing (missing data); MIN (minimum value reported); Q (quantiles); Q1 (first quantile); Q3 (third quantile); MEDIAN; MEAN; MAX (maximum value reported); SD (standard deviation); MAD (median absolute deviation standardised to conform with the normal distribution); IQR (interquartile range standardised to conform with the normal distribution); CV (coefficient of variation (%)); CVR (robust coefficient of variation (%)).
Since the points corresponding to these organic-rich samples were excluded from the robust linear regressions (Supplementary Presentation S1), we assume that the matrix effect is not a source of significant bias to correct pXRF measurements of element concentrations using these regressions. Thus, the pXRF method can be applied for this first large-scale mineral element assessment of Yedoma domain deposits.
Yedoma Domain Mineral Concentrations Assessment Dataset
Robust linear regression equations with reliable R2 (Table 4) were applied for each pXRF-measured concentration to correct concentrations values for trueness (Figure 4 and Supplementary Presentation S1). The resulting YMCA dataset, freely available under doi:10.1594/PANGAEA.922724 (Monhonval et al., 2020), compiles 1,292 samples for the following elements: Si, Al, Fe, Ca, K, Ti, Mn, Zn, Sr and Zr.
In addition to the corrected mineral element concentrations, the YMCA dataset combines a wide range of relevant existing information on these 1,292 samples. Site and samples properties are integrated from AWI (Alfred Wegener Institute) Potsdam and Stockholm University data. We associated the lithology of the underlying bedrock, and the type of unconsolidated sediments at the surface characterizing each site from a spatial join using Arc Map 10.4. Specifically, coordinates from each site are joined based on their spatial location to Global Lithology Map (GLiM; Hartmann and Moosdorf, 2012) and Global Unconsolidated Sediments Map (GUM; Börker et al., 2018). Typically, we included the lithology of the underlying bedrock to evaluate its potential control on the mineral element concentrations in the deposits.
The attribute columns of this dataset are organized as follows: i) all site and sample properties (sample ID, description (identifying active layer (AL)), type of deposit, site location, number of profile, GPS coordinates, country, GLiM-lithology, GUM-unconsolidated sediment type, GUM-age, sample depth below surface level (b.s.l) or height above sea/river level (a.s.l), sediment characteristics, BD, gravimetric and absolute ice content, TOC values); and ii) corrected pXRF concentrations based on linear regressions from Linear Regression for Accurate Mineral Element Concentration Measurements. Table 5 summarizes corrected mineral element concentration values as well as BD, TOC and ice content from the whole Yedoma domain region, with no differentiation between Yedoma and Alas deposits samples (n = 1,292).
Yedoma Domain Deposits Mineralogy
The main mineral phases (i.e., primary and secondary minerals) identified in selected Yedoma, Alas and fluvial deposits are presented in Table 6. The following minerals were identified in all bulk samples: quartz, feldspar plagioclase, micas and kaolinite. Chlorite was identified in Siberian deposits from Sobo Sise, Buor Khaya and Kytalyk. Calcite and dolomite were only detected in Alaskan Yedoma deposits from Colville and Itkillik. The mineralogy is generally similar along the profile depth for each location (Supplementary Figure S3). In these silty-fine sediments, the clay content ranges between 6 and 18% (silt content 67–79%; sand content 3–55%), i.e., well in line with Strauss et al. (2012). The diffractograms on clay fractions from Buor Khaya highlighted the presence of kaolinite, illite, and smectite (Supplementary Figure S3D–I). These data highlight the co-existence in these deposits of highly stable minerals such as quartz with more weatherable minerals such as dolomite, calcite, or feldspar plagioclase (Goldich, 1938; Wilson, 2004; Cornelis et al., 2011), and the presence of clay minerals characterized by higher specific surface area relative to the other mineral constituents (i.e., smectite, kaolinite, illite) (Kahle et al., 2004; Saidy et al., 2012).
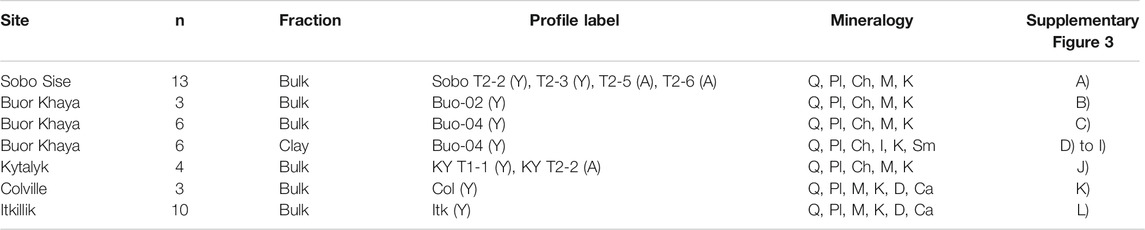
TABLE 6. Mineralogical composition in Yedoma (Y) and Alas and fluvial (A) deposits of Siberia and Alaska (Q, Quartz; Pl, Plagioclase; Ch, Chlorite; M, Mica; I, Illite; K, Kaolinite; D, Dolomite; Ca, Calcite; Sm, Smectites). The diffractograms for each profile (n = number of diffractogram per profile) are presented in Supplementary Figure S3.
Mineral Element Stocks in the Yedoma Domain
The quantification of mineral element stocks is a major step to assess the distribution of mineral elements in the Yedoma domain. We performed mineral elements stock estimations with the bootstrapping technique (Mineralogy by X-Ray Diffraction). For example, the mean Fe stock from Yedoma deposits (n = 814) is 147 ± 43 Gt (±2σ) using the theoretical normal distribution (mean-bootstrapping technique; Figure 5).
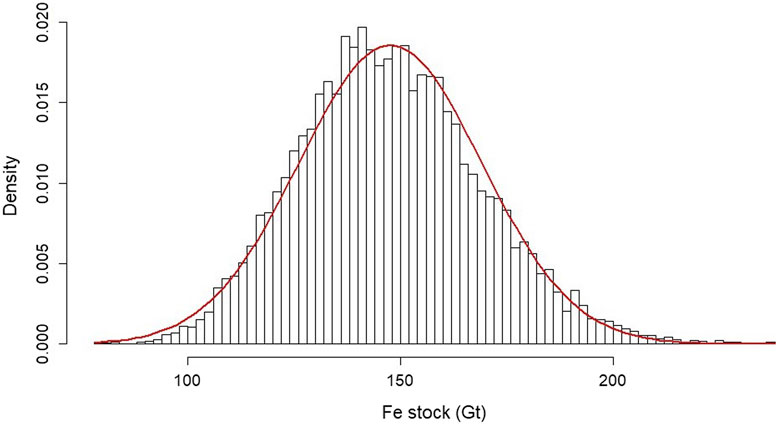
FIGURE 5. Distribution of the iron stock (Gt) in Yedoma deposits (n = 814). The histogram results from the bootstrapping technique (n = 10,000; Supplementary Figure S2). The theoretical normal distribution is shown in red.
Based on the YMCA dataset, the bootstrapping technique was applied to calculate the stocks in Yedoma deposits for the 10 elements (Si, Al, Fe, Ca, K, Ti, Mn, Zn, Sr, and Zr), basing on the same input parameters for BD, thickness, WIV and coverage. The estimate mean and standard deviation derived the best estimation of the Yedoma deposit stocks for each specific mineral element. With a similar approach, mineral element stocks (Si, Al, Fe, Ca, K, Ti, Mn, Zn, Sr, and Zr) were estimated in Alas deposits. The element stocks in the Yedoma domain were obtained by adding element stocks in Yedoma and Alas deposits (Table 7). The results highlight that the mineral element with the largest stock in the Yedoma domain is Si (2,739 ± 986 Gt) followed by Al, Fe, K, Ca, Ti, Mn, Zr, Sr, and Zn (Figure 6). In comparison, the estimated OC stock in the Yedoma domain reaches around 324–466 Gt (Strauss et al., 2017; Figure 6).
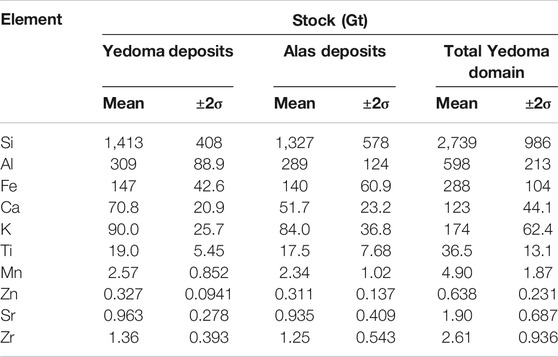
TABLE 7. Mineral elements stock (Gt) summary in Yedoma, Alas and Yedoma domain deposits (n = 814, 470 and 1,284, respectively). Mean and associated 2 standard deviations (σ; absolute value) are provided. Total stocks are the addition from the stocks in Yedoma and Alas deposits. Note, fluvial/deltaic deposits stocks were not estimated because of lack of data and spatial coverage (n = 8).
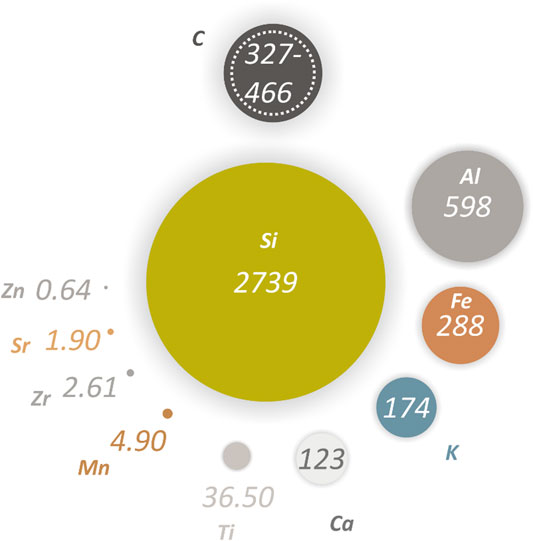
FIGURE 6. Mineral element stocks (in Gt) in the Yedoma domain deposits (including Yedoma and Alas deposits) for Si, Al, Fe, K, Ca, Ti, Mn, Zr, Sr, and Zn. The surface area of the circles is proportional to the stock of the element considered. The organic carbon stock in the Yedoma domain (Strauss et al., 2017) is provided for comparison (the dotted line represents the lower range of the estimate, i.e., 327 Gt).
Overall, Yedoma and Alas deposits (Table 7) represent 86% of the total Yedoma domain area (Figure 2): this means that mineral element stocks are based on 86% of the deposits. The remaining surfaces of the Yedoma domain correspond to deltaic deposits (4%) or lakes and rivers (10%). Element stocks in deltaic deposits have not been estimated in this study due to the scarce number of available mineral concentration data in deltaic deposits (0.6% of the YMCA dataset) and the lack of empirical estimations of their thickness, or their ice volume (even if ice volume is probably negligible in these deposits). Waterbodies, lakes and rivers are not considered in this assessment focusing on the mineral element content in surface solid materials vulnerable to thaw or already thawed in the past. Sediments underlying lakes and rivers are therefore outside the scope of this evaluation and would only become relevant if drainage occurs. Absolute stock estimates (in Gt) allow direct comparison between Yedoma and Alas deposits, despite their different thickness (19.6 and 5.5 m, respectively), WIV and coverage. Mineral element density estimations (kg m−3) are available (Supplementary Table S5) in which thickness and WIV have been neglected: this estimation is the product of mineral element concentrations and BD using bootstrapping technique.
Advantages and Limitations of the Methodological Approach
Portable X-Ray Fluorescence
The main advantage of the pXRF method is to ensure a non-destructive, more rapid (less steps involved; Figure 4) and cheaper method for mineral element concentration measurement compared to the ICP-OES measurements after alkaline fusion. This is beneficial when dealing with large-scale assessments involving thousands of archive samples from the Yedoma domain. The concentrations of 10 elements could be reliably measured in these deposits (i.e., Si, Al, Fe, K, Ca, Ti, Mn, Zr, Sr, and Zn). The main limitation of the pXRF method is that raw concentration data cannot be used before applying a linear regression to correct for systematic error. This drawback related to inaccurate raw pXRF measurements can be rectified by correcting the raw pXRF values with accurate values obtained with the ICP-OES method after alkaline fusion (calibration based on 144 samples in this study). This correction can only be applied when the correlation between pXRF and ICP-OES concentrations is satisfactory (here, R2 > 0.7). In these deposits, the concentrations of some elements (Mg, P, Cu, Ni, Ba) could not be assessed due to poor pXRF-ICP-OES correlation (R2 < 0.5). Linear regressions used to correct raw pXRF concentrations depend on internal geometry of the pXRF device used. This means that each pXRF device needs its own linear regression and that a single linear regression equation cannot be used with different pXRF devices. Moreover, pXRF measurements are also matrix-dependent. The matrix (i.e., organic content, bulk density) of a sample can affect pXRF-measured concentrations and therefore linear regressions must be calibrated with samples from the same matrix (here, we used a subset of 11% of samples from the Yedoma domain). For some other elements, pXRF measurements are not possible due to the low atomic mass of these elements (N, Na).
Mean-Bootstrapping Technique
The main advantage of the mean-bootstrapping technique is to ensure a reliable calculation of non-parametric uncertainty estimates. The bootstrapping technique allows to include variability in mineral element concentrations and BD, WIV and thickness of the deposits. However, standard deviations from stock estimations (Table 7) are markedly large because of the conservative (observation-based) approach using all measurement for bootstrapping and deriving the mean afterwards. The only fixed parameter included in mineral element stock calculation (Eq. 3) is coverage. The coverage is set to 410,000 and 780,000 km2 for Yedoma and Alas deposits, respectively. These estimations are subject to uncertainty but are the best current estimates, identical to the ones used in Strauss et al. (2013) to allow direct comparison with OC stocks. However, with the ongoing Arctic warming, these coverage estimations are dedicated to change with time. Because mineral element stocks are based on different WIV, thickness and coverage, the mineral element density expressed in kg m−3 allows to compare Yedoma and Alas deposits for an identical one cubic meter of sediments (without ice-wedges volume) (Supplementary Table S5).
Implications Upon Permafrost Thaw
Implications for the Evolution of Mineral-Organic Carbon Interactions
The YMCA dataset allows investigating the evolution of selected element to OC ratio upon thermokarst processes resulting from permafrost thaw, i.e., between Yedoma and Alas deposits. Organo-mineral associations with Fe-, Al-, Mn-oxides or clay minerals using polyvalent cations bridging between OC and mineral surfaces (e.g., Ca2+, Sr2+ in neutral and alkaline soils or Fe3+ and Al3+ in acid soils), or organo-metallic complexes involving Fe3+, Fe2+ and Al3+ ions are well known to contribute to OC stabilization (Lutzow et al., 2006). Some mineral elements such as Fe or Mn are known for an inhibition effect on methane production (Lovley and Phillips, 1987; Beal et al., 2009; Herndon et al., 2015; Sowers et al., 2018), despite microbial function being considered as a larger part of the limitation for methane production (Monteux et al., 2020). The present dataset highlights a decrease of the Fe/TOC ratio and the Al/TOC ratio from Yedoma to Alas in Alaska, and virtually no change in these ratios in the Siberian Yedoma domain (Figures 7A,B). Providing evidence for changes in Fe/TOC or Al/TOC ratios upon thermokarst processes in a portion of the Yedoma domain highlights the need for further investigations regarding the evolution of interactions between OC and Fe or Al upon thawing.
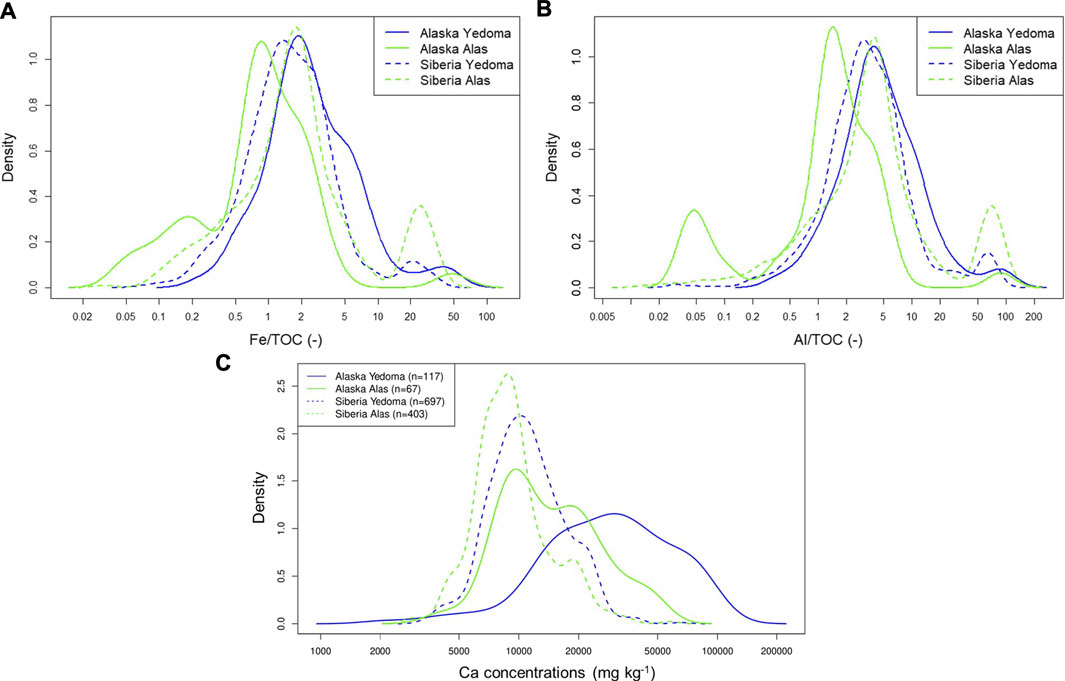
FIGURE 7. Empirical density histogram of (A) Fe/total organic carbon (TOC) ratio, (B) Al/TOC ratio and (C) Ca concentrations (mg kg−1) in Yedoma domain deposits in Alaska (full line) and Siberia (dashed line) for Yedoma (blue line) and Alas (green line) deposits. The X-axis is a log scale.
In addition, the clay minerals present in these Yedoma domain deposits (confirmed by XRD; Table 6) can be associated to the formation of aggregates, thereby contributing to spatial inaccessibility of OC for decomposer organisms due to occlusion in aggregates (Lutzow et al., 2006). Iron oxides are also known to contribute to the formation of aggregates (Eusterhues et al., 2005; Kleber et al., 2015). Changing Fe/TOC ratio between Yedoma and Alas in Alaska (Figures 7A,B) reinforces the need to further investigate the potential role of the evolution of aggregates for OC availability upon thawing (Monhonval et al., 2021). Changing conditions for mineral protection of OC upon thawing is likely to influence OC microbial degradation (Gentsch et al., 2015; Herndon et al., 2017; Kögel-Knabner et al., 2010; Opfergelt, 2020), thereby contributing to modulate the permafrost carbon feedback (Schuur et al., 2015).
Implications for Mineral Weathering and Nutrient Supply to Ecosystems
The YMCA dataset allows investigating the change in concentration of soluble elements such as Ca upon thermokarst processes resulting from permafrost thaw, i.e., between Yedoma and Alas deposits. As shown on a density plot (Figure 7C), sediments from Alas deposits are characterized by lower Ca concentrations compared to Yedoma deposits. This was observed between Yedoma and Alas deposits in Alaska, and in Siberia (Figure 7C).
Higher Ca concentrations in Yedoma and Alas deposits from Alaska relative to the deposits from Siberia can be explained by the local lithology, i.e., carbonate rocks from the northern Brooks Range contributing to the deposits in Alaska (Till et al., 2008). The lithology of the bedrock, beneath Quaternary deposits, inferred from the GLiM map, is similar between Yedoma and Alas deposits (Supplementary Figure S4). This can likely be explained by the fact that the Yedoma and Alas deposits from the Yedoma domain mainly originate from the same source material on local to regional scales (Grosse et al., 2007; Strauss et al., 2017). Since most of the studied regions are covered by thick Quaternary deposits, mineral element concentrations are likely more influenced by the mixing of the unconsolidated sediments contributing to the Quaternary deposits rather than by the lithology of the underlying bedrock. The lithological similarity between Yedoma and Alas deposits can also be explained by the fact that Alas deposits are dominated by reworked sediments from former Yedoma deposits (Schirrmeister et al., 2020). Therefore, Ca depletion in Alas deposits relative to Yedoma deposits from one region is not lithology dependent but potentially results from mineral weathering and leaching processes of soluble elements such as Ca during former thawing periods. Indeed, Alas formation history includes lake formation and drainage, and the dynamism of such formation over the past thousands of years may lead to leaching processes of soluble elements, a commonly observed process in non-cryogenic soils (Stumm and Morgan, 1995) and cryogenic soils (Ping et al., 2005).
The co-existence of more weatherable (dolomite, calcite, or feldspar plagioclase) and less weatherable (quartz) mineral constituents in Yedoma deposits (Table 6) is likely to lead to an increasing contribution from mineral weathering to the inorganic carbon budget (Zolkos and Tank, 2020) upon thermokarst processes. The balance between carbonate or silicate mineral weathering is known to influence the short-term and long-term atmospheric CO2 consumption (Berner et al., 1983). Locally, the oxidation of sulfides such as pyrite present in low amount, not detected here but reported in beach deposits (Schirrmeister et al., 2010), can lead to the formation of sulfuric acid and the subsequent weathering of carbonate mineral without involving atmospheric CO2 consumption (Zolkos and Tank, 2020).
The YMCA dataset provides total mineral element concentrations, including i) a mineral element reservoir known to be rapidly available to vegetation and microorganisms in permafrost soils (Ping et al., 1998, 2005), and ii) a mineral element reservoir providing a longer term supply of nutrients through mineral weathering (Zolkos and Tank, 2020). Indeed, the weathering of minerals such as dolomite, calcite, or feldspar plagioclase is expected to release soluble cations such as Ca available to supply nutrients to terrestrial and aquatic Arctic ecosystems, or to interact with OC (Implications for the Evolution of Mineral-Organic Carbon Interactions). Among the 10 elements included in the YMCA dataset, macro- (e.g., K, Ca) and micro-nutrients (e.g., Fe, Mn, Zn) are required for plant nutrition and also regulate other vital processes for plants and microorganisms growth and metabolic activity (DalCorso et al., 2014). Briefly, K regulates vital processes, such as photosynthesis, water and nutrient transportation, or protein synthesis (Marschner, 2012). Ca is a major second messenger in plant signal transduction, mediating stress- and developmental processes (Liese and Romeis, 2013). Micro-elements are required as cofactor for some essential proteins (Fe; Morgan and Connolly, 2013), play an essential role as a photosynthetic function or in metallo-protein conformation (Mn; Yang et al., 2008) or can have enzymatic functions (Zn; Lindsay, 1972). Some non-essential elements, such as Si and Al, can stimulate plant growth by playing with abiotic-biotic stress resistance and symbiosis (Richmond and Sussman, 2003; DalCorso et al., 2014). In aquatic ecosystems, silicon is a limiting nutrient for diatoms and other siliceous organisms, thereby controlling diatom abundance and community structure in the ocean, and as a result, food web and CO2 uptake by photosynthesis (Smetacek, 1999; Yool and Tyrrell, 2003). The leaching of the more mobile cations such as Ca or K can be estimated by comparing ratios between mobile and immobile elements: this is why assessing the concentration of elements considered as immobile such as Ti or Zr can be useful to evaluate the advance of weathering in a soil relative to its parent material, and thereby the soil mineral reserve (Kurtz et al., 2000; Hodson, 2002; Jiang et al., 2018). However, the reserve in important nutrients such as P, N, S, and Mg in the Yedoma domain could not be estimated with the method used in this study (Sect. 5). The YMCA dataset is a first step needed in order to evaluate the impact of widespread rapid permafrost thaw through thermokarst processes (Turetsky et al., 2020) on the mineral element concentrations in the deposits and the potential implications for OC and mineral nutrient supply.
Conclusion
This study presents a method combining portable X-ray fluorescence with a bootstrapping technique to generate the first mineral element inventory of permafrost deposits from the ice-rich Yedoma region, i.e., never thawed Yedoma deposits and previously thawed Alas deposits for a mean thickness of 19.6 and 5.5 m, respectively. In the resulting Yedoma domain Mineral Concentrations Assessment (YMCA) dataset, the total concentrations of 10 mineral elements (Si, Al, Fe, Ca, K, Ti, Mn, Zn, Sr, Zr) in Yedoma domain deposits have been quantified in 75 different profiles. The associated mineral elements stocks are shown to be in the same order of magnitude for Al and Fe than for OC, and to decrease from Si, Al, Fe, K, Ca, Ti, Mn, Zr, Sr, to Zn. This dataset allows tracking dynamic processes controlling mineral element concentrations in thawing environments, as illustrated by lower Ca concentration in Alas deposits relative to Yedoma deposits highlighting potential Ca leaching upon thawing. Providing the YMCA dataset is contributing to improve the knowledge on the mineral constituents in ice-rich permafrost, i.e., a necessary step to better understand the evolution of mineral-OC interactions, mineral weathering and mineral nutrient supply to ecosystems upon permafrost thaw. The YMCA dataset is particularly relevant given the increasing occurrence of abrupt thaw in ice-rich permafrost regions.
Data Availability Statement
The datasets presented in this study can be found in online repositories. The names of the repository/repositories and accession number(s) can be found below: https://doi.pangaea.de/10.1594/PANGAEA.922724.
Authors Contribution
AM, SO, and JS conceived the project. JS, GG, LS, MF, and PK retrieved samples from Alaska and Siberia from many different field expeditions. AM did the pXRF measurements with the help of EM. AM, SO, EM, BP, and AV contributed to set up the YMCA database. AM analyzed the data and calculated to the stocks based on the code developed by JS for carbon stock estimation using mean-bootstrapping. AM wrote the manuscript with contributions from all co-authors.
Funding
This project received funding from the European Union’s Horizon 2020 research and innovation program under grant agreement No. 714617 to SO, and SO acknowledges funding from the Fund for Scientific Research FNRS in Belgium (FC69480). This work was embedded into the Action Group “The Yedoma Region” funded by the International Permafrost Association. Field sample collection was funded by a European Research Council (ERC) Starting Grant (338335) to GG as well as Alfred Wegener Institute basic funding. Kytalyk samples were collected with support of the European Union FP7—ENVIRONMENT project PAGE21 (grant agreement 282700) and the EU FP7 INTERACT Integrating Activity.
Conflict of Interest
The authors declare that the research was conducted in the absence of any commercial or financial relationships that could be construed as a potential conflict of interest.
Publisher’s Note
All claims expressed in this article are solely those of the authors and do not necessarily represent those of their affiliated organizations, or those of the publisher, the editors and the reviewers. Any product that may be evaluated in this article, or claim that may be made by its manufacturer, is not guaranteed or endorsed by the publisher.
Acknowledgments
The authors acknowledge Hélène Dailly, Anne Iserentant, Elodie Devos and Laurence Monin from the MOCA analytical platform at UCLouvain for mineral elemental analysis. We thank Waldemar Schneider (AWI logistics) and Dmitry Melnichenko (Hydrobase Tiksi) for many years of logistical support, and Catherine Hirst, Maxime Thomas, and Pierre Delmelle for fruitful scientific discussions. We thank the two reviewers for their constructive comments.
Supplementary Material
The Supplementary Material for this article can be found online at: https://www.frontiersin.org/articles/10.3389/feart.2021.703304/full#supplementary-material
Supplementary Presentation S1 | Robust linear regressions (blue line) between element concentrations obtained by the ICP-OES method as a function of the raw concentrations obtained by the pXRF method. The data are presented for the 10 elements considered: Si (A), Al (B), Fe (C), Ca (D), K (E), Ti (F), Mn (G), Zn (H), Sr (I), and Zr (J) with n = 144 for all elements, except for Zn where n = 119. Grey points (which include organic-rich samples; TOC >40 wt%) were excluded from the robust linear regression. Errors bar (±2 pooled σ) were calculated based on three repetitions of three samples for ICP-OES method and on three to five repetitions of 20 samples for pXRFmethod. Robust linear regression R2 and equation are provided for each element.
References
Abbott, B. W., and Jones, J. B. (2015). Permafrost Collapse Alters Soil Carbon Stocks, Respiration, CH4, and N2O in upland Tundra. Glob. Change Biol. 21, 4570–4587. doi:10.1111/gcb.13069
Andreev, A. A., Grosse, G., Schirrmeister, L., Kuznetsova, T. V., Kuzmina, S. A., Bobrov, A. A., et al. (2009). Weichselian and Holocene Palaeoenvironmental History of the Bol'shoy Lyakhovsky Island, New Siberian Archipelago, Arctic Siberia. Boreas 38, 72–110. doi:10.1111/j.1502-3885.2008.00039.x
Andreev, A. A., Schirrmeister, L., Siegert, C., Bobrov, A. A., Demske, D., Seiffert, M., et al. (2002). Paleoenvironmental Changes in Northeastern Siberia during the Late Quaternary - Evidence from Pollen Records of the Bykovsky Peninsula. Polarforschung 70, 13–25. doi:10.2312/polarforschung.70.13
Anzelmo, J. A., and Lindsay, J. R. (1987). X-ray Fluorescence Spectrometric Analysis of Geologic Materials Part 1. Principles and Instrumentation. J. Chem. Educ. 64, A181. doi:10.1021/ed064pA181
Börker, J., Hartmann, J., Amann, T., and Romero-Mujalli, G. (2018). Global Unconsolidated Sediments Map Database v1.0 (Shapefile and Gridded to 0.5° Spatial Resolution). doi:10.1594/PANGAEA.884822
Beal, E. J., House, C. H., and Orphan, V. J. (2009). Manganese- and Iron-dependent Marine Methane Oxidation. Science 325, 184–187. doi:10.1126/science.1169984
Berner, R. A., Lasaga, A. C., and Garrels, R. M. (1983). The Carbonate-Silicate Geochemical Cycle and its Effect on Atmospheric Carbon Dioxide over the Past 100 Million Years. Am. J. Sci. 283, 641–683. doi:10.2475/ajs.283.7.641
Brosius, L. S., Anthony, K. M. W., Treat, C. C., Lenz, J., Jones, M. C., Bret-Harte, M. S., et al. (2021). Spatiotemporal Patterns of Northern lake Formation since the Last Glacial Maximum. Quat. Sci. Rev. 253, 106773. doi:10.1016/j.quascirev.2020.106773
Brown, J., Ferrians, O. J., Heginbottom, J. A., Melnikov, E. S., and Akerman, J. (1997). Circum-Arctic Map of Permafrost and Ground-Ice conditionsCircum-Pacific Map Series CP-45, Scale 1:10,000,000, Tech. Rep. (Washington, DC: U.S.Geological Survey in Cooperation with the Circum-Pacific Council for Energy and Mineral Resources). doi:10.13140/RG.2.1.2994.9040
Chao, T. T., and Sanzolone, R. F. (1992). Decomposition Techniques. J. Geochemical Exploration 44, 65–106. doi:10.1016/0375-6742(92)90048-d
Cornelis, J.-T., Delvaux, B., Georg, R. B., Lucas, Y., Ranger, J., and Opfergelt, S. (2011). Tracing the Origin of Dissolved Silicon Transferred from Various Soil-Plant Systems towards Rivers: a Review. Biogeosciences 8, 89–112. doi:10.5194/bg-8-89-2011
DalCorso, G., Manara, A., Piasentin, S., and Furini, A. (2014). Nutrient Metal Elements in Plants. Metallomics 6, 1770–1788. doi:10.1039/c4mt00173g
Dutta, K., Schuur, E. A. G., Neff, J. C., and Zimov, S. A. (2006). Potential Carbon Release from Permafrost Soils of Northeastern Siberia. Glob. Change Biol. 12, 2336–2351. doi:10.1111/j.1365-2486.2006.01259.x
Eusterhues, K., Rumpel, C., and Kogel-Knabner, I. (2005). Organo-mineral Associations in sandy Acid forest Soils: Importance of Specific Surface Area, Iron Oxides and Micropores. Eur. J. Soil Sci. 56, 050912034650049–050912034650763. doi:10.1111/j.1365-2389.2005.00710.x
Fouché, J., Christiansen, C. T., Lafrenière, M. J., Grogan, P., and Lamoureux, S. F. (2020). Canadian Permafrost Stores Large Pools of Ammonium and Optically Distinct Dissolved Organic Matter. Nat. Commun. 11, 4500. doi:10.1038/s41467-020-18331-w
Fritz, M., Unkel, I., Lenz, J., Gajewski, K., Frenzel, P., Paquette, N., et al. (2018). Regional Environmental Change versus Local Signal Preservation in Holocene Thermokarst lake Sediments: A Case Study from Herschel Island, Yukon (Canada). J. Paleolimnol. 60, 77–96. doi:10.1007/s10933-018-0025-0
Fritz, M., Wetterich, S., Meyer, H., Schirrmeister, L., Lantuit, H., and Pollard, W. H. (2011). Origin and Characteristics of Massive Ground Ice on Herschel Island (Western Canadian Arctic) as Revealed by Stable Water Isotope and Hydrochemical Signatures. Permafrost Periglac. Process. 22, 26–38. doi:10.1002/ppp.714
Fuchs, M., Grosse, G., Strauss, J., Günther, F., Grigoriev, M., Maximov, G. M., et al. (2018). Carbon and Nitrogen Pools in Thermokarst-Affected Permafrost Landscapes in Arctic Siberia. Biogeosciences 15, 953–971. doi:10.5194/bg-15-953-2018
Fuchs, M., Lenz, J., Jock, S., Nitze, I., Jones, B. M., Strauss, J., et al. (2019). Organic Carbon and Nitrogen Stocks along a Thermokarst Lake Sequence in Arctic Alaska. J. Geophys. Res. Biogeosci. 124, 1230–1247. doi:10.1029/2018JG004591
Fuchs, M., Nitze, I., Strauss, J., Günther, F., Wetterich, S., Kizyakov, A., et al. (2020). Rapid Fluvio-thermal Erosion of a Yedoma Permafrost Cliff in the Lena River Delta (Accepted). Front. Earth Sci.
Gentsch, N., Mikutta, R., Shibistova, O., Wild, B., Schnecker, J., Richter, A., et al. (2015). Properties and Bioavailability of Particulate and Mineral-Associated Organic Matter in Arctic Permafrost Soils, Lower Kolyma Region, Russia: Organic Matter Stabilization in Permafrost Soils. Eur. J. Soil Sci. 66, 722–734. doi:10.1111/ejss.12269
Gentsch, N., Wild, B., Mikutta, R., Čapek, P., Diáková, K., Schrumpf, M., et al. (2018). Temperature Response of Permafrost Soil Carbon Is Attenuated by mineral protection. Glob. Change Biol. 24, 3401–3415. doi:10.1111/gcb.14316
Grigoriev, M. N., Rachold, V., and Bolshiyanov, D. (2003). Russian-German cooperation System Laptev Sea : the expedition LENA 2002 , Berichte zur Polar- und Meeresforschung (Reports on Polar and Marine Research), Alfred Wegener Inst. Polar Mar. Res. 466, 341. doi:10.2312/BzPM_0466_2003
Grosse, G., Jones, B., and Arp, C. (2013). Treatise on Geomorphology. Elsevier, 325–353. doi:10.1016/B978-0-12-374739-6.00216-58.21 Thermokarst Lakes, Drainage, and Drained Basins
Grosse, G., Jones, B. M., Schirrmeister, L., Meyer, H., Wetterich, S., Strauss, J., et al. (2015). Late Pleistocene and Holocene Ice-Rich Permafrost in the Colville River valley, Northern Alaska. EPIC3PAST Gateways 2015, Potsdam, 18, Geophysical Research Abstracts (Potsdam: EGU2015-10607). Available at: http://www.awi.de/pastgateways2015 (Accessed October 12, 2020).
Grosse, G., Schirrmeister, L., Siegert, C., Kunitsky, V. V., Slagoda, E. A., Andreev, A. A., et al. (2007). Geological and Geomorphological Evolution of a Sedimentary Periglacial Landscape in Northeast Siberia during the Late Quaternary. Geomorphology 86, 25–51. doi:10.1016/j.geomorph.2006.08.005
Günther, F., Overduin, P. P., Yakshina, I. A., Opel, T., Baranskaya, A. V., and Grigoriev, M. N. (2015). Observing Muostakh Disappear: Permafrost Thaw Subsidence and Erosion of a Ground-Ice-Rich Island in Response to Arctic Summer Warming and Sea Ice Reduction. The Cryosphere 9, 151–178. doi:10.5194/tc-9-151-2015
Harden, J. W., Koven, C. D., Ping, C.-L., Hugelius, G., David McGuire, A., Camill, P., et al. (2012). Field Information Links Permafrost Carbon to Physical Vulnerabilities of Thawing. Geophys. Res. Lett. 39. doi:10.1029/2012GL051958
Hartmann, J., and Moosdorf, N. (2012). Global Lithological Map Database v1.0 (Gridded to 0.5° Spatial Resolution). doi:10.1594/PANGAEA.788537
Hemingway, J. D., Rothman, D. H., Grant, K. E., Rosengard, S. Z., Eglinton, T. I., Derry, L. A., et al. (2019). Mineral protection Regulates Long-Term Global Preservation of Natural Organic Carbon. Nature 570, 228–231. doi:10.1038/s41586-019-1280-6
Herndon, E., AlBashaireh, A., Singer, D., Roy Chowdhury, T., Gu, B., and Graham, D. (2017). Influence of Iron Redox Cycling on Organo-mineral Associations in Arctic Tundra Soil. Geochimica et Cosmochimica Acta 207, 210–231. doi:10.1016/j.gca.2017.02.034
Herndon, E. M., Mann, B. F., Roy Chowdhury, T., Yang, Z., Wullschleger, S. D., Graham, D., et al. (2015). Pathways of Anaerobic Organic Matter Decomposition in Tundra Soils from Barrow, Alaska. J. Geophys. Res. Biogeosci. 120, 2345–2359. doi:10.1002/2015JG003147
Hodson, M. E. (2002). Experimental Evidence for Mobility of Zr and Other Trace Elements in Soils. Geochimica et Cosmochimica Acta 66, 819–828. doi:10.1016/S0016-7037(01)00803-1
Hugelius, G., Loisel, J., Chadburn, S., Jackson, R. B., Jones, M., MacDonald, G., et al. (2020). Large Stocks of Peatland Carbon and Nitrogen Are Vulnerable to Permafrost Thaw. Proc. Natl. Acad. Sci. USA 117, 20438–20446. doi:10.1073/pnas.1916387117
Hugelius, G., Strauss, J., Zubrzycki, S., Harden, J. W., Schuur, E. A. G., Ping, C.-L., et al. (2014). Estimated Stocks of Circumpolar Permafrost Carbon with Quantified Uncertainty Ranges and Identified Data Gaps. Biogeosciences 11, 6573–6593. doi:10.5194/bg-11-6573-2014
Jiang, K., Qi, H.-W., and Hu, R.-Z. (2018). Element Mobilization and Redistribution under Extreme Tropical Weathering of Basalts from the Hainan Island, South China. J. Asian Earth Sci. 158, 80–102. doi:10.1016/j.jseaes.2018.02.008
Jongejans, L. L., and Strauss, J. (2020). Bootstrapping Approach for Permafrost Organic Carbon Pool Estimation. doi:10.5281/zenodo.3734247
Jongejans, L. L., Strauss, J., Lenz, J., Peterse, F., Mangelsdorf, K., Fuchs, M., et al. (2018). Organic Matter Characteristics in Yedoma and Thermokarst Deposits on Baldwin Peninsula, West Alaska. Biogeosciences 15, 6033–6048. doi:10.5194/bg-15-6033-2018
Jorgenson, M. T., Yoshikawa, K., Kanveskiy, M., Shur, Y., Romanovsky, V., Marchenko, S., et al. (2008). Permafrost Characteristics of Alaska.
Kahle, M., Kleber, M., and Jahn, R. (2004). Retention of Dissolved Organic Matter by Phyllosilicate and Soil clay Fractions in Relation to mineral Properties. Org. Geochem. 35, 269–276. doi:10.1016/j.orggeochem.2003.11.008
Kaiser, K., and Guggenberger, G. (2003). Mineral Surfaces and Soil Organic Matter. Eur. J. Soil Sci. 54, 219–236. doi:10.1046/j.1365-2389.2003.00544.x
Kanevskiy, M., Shur, Y., Fortier, D., Jorgenson, M. T., and Stephani, E. (2011). Cryostratigraphy of Late Pleistocene Syngenetic Permafrost (Yedoma) in Northern Alaska, Itkillik River Exposure. Quat. Res. 75, 584–596. doi:10.1016/j.yqres.2010.12.003
Kanevskiy, M., Shur, Y., Strauss, J., Jorgenson, T., Fortier, D., Stephani, E., et al. (2016). Patterns and Rates of riverbank Erosion Involving Ice-Rich Permafrost (Yedoma) in Northern Alaska. Geomorphology 253, 370–384. doi:10.1016/j.geomorph.2015.10.023
Kaufman, D., Ager, T. A., Anderson, N. J., Anderson, P. M., Andrews, J. T., Bartlein, P. J., et al. (2004). Holocene thermal Maximum in the Western Arctic (0-180°W). Quat. Sci. Rev. 23, 529–560. doi:10.1016/j.quascirev.2003.09.007
Kleber, M., Eusterhues, K., Keiluweit, M., Mikutta, C., Mikutta, R., and Nico, P. S. (2015). Mineral-organic Associations: Formation, Properties, and Relevance in Soil Environments. In Advances in Agronomy. Elsevier, 1–140. doi:10.1016/bs.agron.2014.10.005
Kögel-Knabner, I., Amelung, W., Cao, Z., Fiedler, S., Frenzel, P., Jahn, R., et al. (2010). Biogeochemistry of Paddy Soils. Geoderma 157, 1–14. doi:10.1016/j.geoderma.2010.03.009
Kögel-Knabner, I., Guggenberger, G., Kleber, M., Kandeler, E., Kalbitz, K., Scheu, S., et al. (2008). Organo-mineral Associations in Temperate Soils: Integrating Biology, Mineralogy, and Organic Matter Chemistry. J. Plant Nutr. Soil Sci. 171, 61–82. doi:10.1002/jpln.200700048
Kokelj, S. V., and Jorgenson, M. T. (2013). Advances in Thermokarst Research. Permafrost Periglac. Process. 24, 108–119. doi:10.1002/ppp.1779
Konishchev, V. N., and Rogov, V. V. (1993). Investigations of Cryogenic Weathering in Europe and Northern Asia. Permafrost Periglac. Process. 4, 49–64. doi:10.1002/ppp.3430040105
Kuhry, P., Bárta, J., Blok, D., Elberling, B., Faucherre, S., Hugelius, G., et al. (2020). Lability Classification of Soil Organic Matter in the Northern Permafrost Region. Biogeosciences 17, 361–379. doi:10.5194/bg-17-361-2020
Kurtz, A. C., Derry, L. A., Chadwick, O. A., and Jo Alfano, M. (2000). Refractory Element Mobility in Volcanic Soils. Geology 28, 683–686. doi:10.1130/0091-7613(2000)028<0683:remivs>2.3.co;2
Lacelle, D., Bjornson, J., and Lauriol, B. (2010). Climatic and Geomorphic Factors Affecting Contemporary (1950-2004) Activity of Retrogressive Thaw Slumps on the Aklavik Plateau, Richardson Mountains, NWT, Canada. Permafrost Periglac. Process. 21, 1–15. doi:10.1002/ppp.666
Lai, R. (2004). Soil Carbon Sequestration in Natural and Managed Tropical Forest Ecosystems. J. Sust. For. 21, 1–30. doi:10.1300/J091v21n01_01
Liese, A., and Romeis, T. (2013). Biochemical Regulation of In Vivo Function of Plant Calcium-dependent Protein Kinases (CDPK). Biochim. Biophys. Acta (Bba) - Mol. Cel Res. 1833, 1582–1589. doi:10.1016/j.bbamcr.2012.10.024
Lindsay, W. L. (1972). “Zinc in Soils and Plant Nutrition,” in “Zinc in Soils and Plant Nutrition,” in Advances in Agronomy. Editor N. C. Brady (Academic Press), 147–186. doi:10.1016/S0065-2113(08)60635-5
Lovley, D. R., and Phillips, E. J. P. (1987). Competitive Mechanisms for Inhibition of Sulfate Reduction and Methane Production in the Zone of Ferric Iron Reduction in Sediments. Appl. Environ. Microbiol. 53, 2636–2641. doi:10.1128/aem.53.11.2636-2641.1987
Lützow, M. v., Kögel-Knabner, I., Ekschmitt, K., Matzner, E., Guggenberger, G., Marschner, B., et al. (2006). Stabilization of Organic Matter in Temperate Soils: Mechanisms and Their Relevance under Different Soil Conditions - a Review. Eur. J. Soil Sci. 57, 426–445. doi:10.1111/j.1365-2389.2006.00809.x
McLaren, T. I., Guppy, C. N., Tighe, M. K., Forster, N., Grave, P., Lisle, L. M., et al. (2012). Rapid, Nondestructive Total Elemental Analysis of Vertisol Soils Using Portable X-ray Fluorescence. Soil Sci. Soc. America J. 76, 1436–1445. doi:10.2136/sssaj2011.0354
Monteux, S., Keuper, F., Fontaine, S., Gavazov, K., Hallin, S., Juhanson, J., et al. (2020). Carbon and Nitrogen Cycling in Yedoma Permafrost Controlled by Microbial Functional Limitations. Nat. Geosci. 13, 794–798. doi:10.1038/s41561-020-00662-4
Monhonval, A., Opfergelt, S., Mauclet, E., Pereira, B., Vandeuren, A., Grosse, G., et al. (2020). Yedoma Domain Mineral Concentrations Assessment (YMCA). doi:10.1594/PANGAEA.922724
Monhonval, A., Strauss, J., Mauclet, E., Hirst, C., Bemelmans, N., Grosse, G., et al. (2021). Iron Redistribution upon Thermokarst Processes in the Yedoma Domain. Front. Earth Sci. 9, 629. doi:10.3389/feart.2021.703339
Morgan, J. B., and Connolly, E. L. (2013). Plant-Soil Interactions: Nutrient Uptake. Nat. Educ. Knowl. 482. Available at: https://www.nature.com/scitable/knowledge/library/plant-soil-interactions-nutrient-uptake-105289112/ (Accessed November 2, 2020).
Morgenstern, A., Ulrich, M., Günther, F., Roessler, S., Fedorova, I. V., Rudaya, N. A., et al. (2013). Evolution of Thermokarst in East Siberian Ice-Rich Permafrost: A Case Study. Geomorphology 201, 363–379. doi:10.1016/j.geomorph.2013.07.011
Mueller, C. W., Rethemeyer, J., Kao-Kniffin, J., Löppmann, S., Hinkel, K. M., and G. Bockheim, J. (2015). Large Amounts of Labile Organic Carbon in Permafrost Soils of Northern Alaska. Glob. Change Biol. 21, 2804–2817. doi:10.1111/gcb.12876
Murton, J. B., Goslar, T., Edwards, M. E., Bateman, M. D., Danilov, P. P., Savvinov, G. N., et al. (2015). Palaeoenvironmental Interpretation of Yedoma Silt (Ice Complex) Deposition as Cold-Climate Loess, Duvanny Yar, Northeast Siberia. Permafrost Periglac. Process. 26, 208–288. doi:10.1002/ppp.1843
National Research Centre for CRM (1986). Institute of Geophysical and Geochemical Exploration Component (GBW 07401- GBW 07404.
Nitzbon, J., Westermann, S., Langer, M., Martin, L. C. P., Strauss, J., Laboor, S., et al. (2020). Fast Response of Cold Ice-Rich Permafrost in Northeast Siberia to a Warming Climate. Nat. Commun. 11, 2201. doi:10.1038/s41467-020-15725-8
Olefeldt, D., Goswami, S., Grosse, G., Hayes, D., Hugelius, G., Kuhry, P., et al. (2016). Circumpolar Distribution and Carbon Storage of Thermokarst Landscapes. Nat. Commun. 7, 13043. doi:10.1038/ncomms13043
Opel, T., Meyer, H., Wetterich, S., Laepple, T., Dereviagin, A., and Murton, J. (2018). Ice Wedges as Archives of winter Paleoclimate: A Review. Permafrost and Periglac Process 29, 199–209. doi:10.1002/ppp.1980
Opel, T., Wetterich, S., Meyer, H., Dereviagin, A. Y., Fuchs, M. C., and Schirrmeister, L. (2017). Ground-ice Stable Isotopes and Cryostratigraphy Reflect Late Quaternary Palaeoclimate in the Northeast Siberian Arctic (Oyogos Yar Coast, Dmitry Laptev Strait). Clim. Past 13, 587–611. doi:10.5194/cp-13-587-2017
Opfergelt, S. (2020). The Next Generation of Climate Model Should Account for the Evolution of mineral-organic Interactions with Permafrost Thaw. Environ. Res. Lett. 15, 091003. doi:10.1088/1748-9326/ab9a6d
Pewe, T. L., and Journaux, A. (1983). Origin and Character of Loesslike silt in Unglaciated South-central Yakutia, Siberia, U.S.S.R. US Geol. Surv. Prof. Pap. 1262. doi:10.3133/pp1262
Ping, C.-L., Michaelson, G. J., Kimble, J. M., and Walker, D. A. (2005). Soil Acidity and Exchange Properties of Cryogenic Soils in Arctic Alaska. Soil Sci. Plant Nutr. 51, 649–653. doi:10.1111/j.1747-0765.2005.tb00083.x
Ping, C. L., Bockheim, J. G., Kimble, J. M., Michaelson, G. J., and Walker, D. A. (1998). Characteristics of Cryogenic Soils along a Latitudinal Transect in Arctic Alaska. J. Geophys. Res. 103, 28917–28928. doi:10.1029/98JD02024
Porter, T. J., and Opel, T. (2020). Recent Advances in Paleoclimatological Studies of Arctic Wedge‐ and Pore‐ice Stable‐water Isotope Records. Permafrost and Periglac Process 31, 429–441. doi:10.1002/ppp.2052
R Core Team (2018). R: A Language and Environment for Statistical Computing. Vienna, Austria: R Foundation for Statistical Computing. available at: https://www.R-project.org/.
Ravansari, R., and Lemke, L. D. (2018). Portable X-ray Fluorescence Trace Metal Measurement in Organic Rich Soils: pXRF Response as a Function of Organic Matter Fraction. Geoderma 319, 175–184. doi:10.1016/j.geoderma.2018.01.011
Ravansari, R., Wilson, S. C., and Tighe, M. (2020). Portable X-ray Fluorescence for Environmental Assessment of Soils: Not Just a point and Shoot Method. Environ. Int. 134, 105250. doi:10.1016/j.envint.2019.105250
Reimann, C., Filzmoser, P., Garrett, R., and Dutter, R. (2008). Statistical Data Analysis Explained: Applied Environmental Statistics with R |.
Richmond, K. E., and Sussman, M. (2003). Got Silicon? the Non-essential Beneficial Plant Nutrient. Curr. Opin. Plant Biol. 6, 268–272. doi:10.1016/s1369-5266(03)00041-4
Robert, M., and Tessier, D. (1974). Méthode de préparation des argiles des sols pour des études minéralogiques. Méthode Préparation Argiles Sols Pour Études Minéralogiques 25, 859–882.
Röhl, U., and Abrams, L. J. (2000). High-resolution, Downhole, and Nondestructive Core Measurements from Sites 999 and 1001 in the Caribbean Sea: Application to the Late Pleistocene. Therm. Maximum 165, 191–203.
Romanovskii, N. N. (1993). Fundamentals of Cryogenesis of Lithosphere. Moscow: Moscow University Press.
Rouiller, J., Burtin, G., and Souchier, B. (1972). La dispersion des sols dans l’analyse granulométrique. Méthode utilisant les résines échangeuses d’ions 14, 194–205.
Rouillon, M., and Taylor, M. P. (2016). Can Field Portable X-ray Fluorescence (pXRF) Produce High Quality Data for Application in Environmental Contamination Research?. Environ. Pollut. 214, 255–264. doi:10.1016/j.envpol.2016.03.055
Saidy, A. R., Smernik, R. J., Baldock, J. A., Kaiser, K., Sanderman, J., and Macdonald, L. M. (2012). Effects of clay Mineralogy and Hydrous Iron Oxides on Labile Organic Carbon Stabilisation. Geoderma 173-174 (174), 104–110. doi:10.1016/j.geoderma.2011.12.030
Schirrmeister, L., Dietze, E., Matthes, H., Grosse, G., Strauss, J., Laboor, S., et al. (2020). The Genesis of Yedoma Ice Complex Permafrost - Grain-Size Endmember Modeling Analysis from Siberia and Alaska. E&g Quat. Sci. J. 69, 33–53. doi:10.5194/egqsj-69-33-2020
Schirrmeister, L., Froese, D., Tumskoy, V., Grosse, G., and Wetterich, S. (2013). PERMAFROST and PERIGLACIAL FEATURES | Yedoma: Late Pleistocene Ice-Rich Syngenetic Permafrost of Beringia. in Encyclopedia of Quaternary Science. 2nd edition. Elsevier, 542–552. doi:10.1016/b978-0-444-53643-3.00106-0
Schirrmeister, L., Grosse, G., Kunitsky, V., Magens, D., Meyer, H., Dereviagin, A., et al. (2008). Periglacial Landscape Evolution and Environmental Changes of Arctic lowland Areas for the Last 60 000 Years (Western Laptev Sea Coast, Cape Mamontov Klyk). Polar Res. 27, 249–272. doi:10.1111/j.1751-8369.2008.00067.x
Schirrmeister, L., Grosse, G., Kunitsky, V. V., Fuchs, M. C., Krbetschek, M., Andreev, A. A., et al. (2010). The Mystery of Bunge Land (New Siberian Archipelago): Implications for its Formation Based on Palaeoenvironmental Records, Geomorphology, and Remote Sensing. Quat. Sci. Rev. 29, 3598–3614. doi:10.1016/j.quascirev.2009.11.017
Schirrmeister, L., Grosse, G., Kunitsky, V. V., Meyer, H., Dereviagyn, A. Y., and Kuznetsova, T. V. (2003a). Permafrost, Periglacial and Paleo-Environmental Studies on New Siberian Islands. Rep. Polar Res. 466, 195–314.
Schirrmeister, L., Grosse, G., Schwamborn, G., Andreev, A. A., Meyer, H., Kunitsky, V. V., et al. (2003b). Late Quaternary History of the Accumulation Plain North of the Chekanovsky Ridge (Lena Delta, Russia): A Multidisciplinary Approach. Polar Geogr. 27, 277–319. doi:10.1080/789610225
Schirrmeister, L., Kunitsky, V., Grosse, G., Wetterich, S., Meyer, H., Schwamborn, G., et al. (2011). Sedimentary Characteristics and Origin of the Late Pleistocene Ice Complex on north-east Siberian Arctic Coastal Lowlands and Islands - A Review. Quat. Int. 241, 3–25. doi:10.1016/j.quaint.2010.04.004
Schirrmeister, L., Meyer, H., Andreev, A., Wetterich, S., Kienast, F., Bobrov, A., et al. (2016). Late Quaternary Paleoenvironmental Records from the Chatanika River valley Near Fairbanks (Alaska). Quat. Sci. Rev. 147, 259–278. doi:10.1016/j.quascirev.2016.02.009
Schirrmeister, L., Schwamborn, G., Overduin, P. P., Strauss, J., Fuchs, M. C., Grigoriev, M., et al. (2017). Yedoma Ice Complex of the Buor Khaya Peninsula (Southern Laptev Sea). Biogeosciences 14, 1261–1283. doi:10.5194/bg-14-1261-2017
Schirrmeister, L., Siegert, C., Kuznetsova, T., Kuzmina, S., Andreev, A., Kienast, F., et al. (2002). Paleoenvironmental and Paleoclimatic Records from Permafrost Deposits in the Arctic Region of Northern Siberia. Quat. Int. 89, 97–118. doi:10.1016/S1040-6182(01)00083-0
Schirrmeister, L., Wagner, D., Grigoriev, M., and Bolshiyanov, D. (2007). The Expedition LENA 2005 , Berichte zur Polar- und Meeresforschung (Reports on Polar and Marine Research), Bremerhaven, 550. Bremerhaven: Alfred Wegener Institute for Polar and Marine Research, 289. doi:10.2312/BzPM_0550_2007
Schmidt, M. W. I., Torn, M. S., Abiven, S., Dittmar, T., Guggenberger, G., Janssens, I. A., et al. (2011). Persistence of Soil Organic Matter as an Ecosystem Property. Nature 478, 49–56. doi:10.1038/nature10386
Schneider von Deimling, T., Grosse, G., Strauss, J., Schirrmeister, L., Morgenstern, A., Schaphoff, S., et al. (2015). Observation-based Modelling of Permafrost Carbon Fluxes with Accounting for Deep Carbon Deposits and Thermokarst Activity. Biogeosciences 12, 3469–3488. doi:10.5194/bg-12-3469-2015
Schuur, E. A. G., McGuire, A. D., Schädel, C., Grosse, G., Harden, J. W., Hayes, D. J., et al. (2015). Climate Change and the Permafrost Carbon Feedback. Nature 520, 171–179. doi:10.1038/nature14338
Shand, C. A., and Wendler, R. (2014). Portable X-ray Fluorescence Analysis of mineral and Organic Soils and the Influence of Organic Matter. J. Geochemical Exploration 143, 31–42. doi:10.1016/j.gexplo.2014.03.005
Sizov, O., Konstantinov, A., Volvakh, A., and Molodkov, A. (2020). Timing and Sedimentary Record of Late Quaternary Fluvio-Aeolian Successions of the Tura-Pyshma Interfluve (SW Western Siberia, Russia). Geosciences 10, 396. doi:10.3390/geosciences10100396
Smetacek, V. (1999). Diatoms and the Ocean Carbon Cycle. Protist 150, 25–32. doi:10.1016/S1434-4610(99)70006-4
Sowers, T. D., Stuckey, J. W., and Sparks, D. L. (2018). The Synergistic Effect of Calcium on Organic Carbon Sequestration to Ferrihydrite. Geochem. Trans. 19, 4. doi:10.1186/s12932-018-0049-4
Strauss, J., Laboor, S., Fedorov, A. N., Fortier, D., Froese, D., Fuchs, M., et al. (2016). Database of Ice-Rich Yedoma Permafrost (IRYP). doi:10.1594/PANGAEA.861733
Strauss, J. (2010). Late Quaternary Environmental Dynamics at the Duvanny Yar Key Section, Lower Kolyma, East Siberia Diploma Thesis. Potsdam: Potsdam University.
Strauss, J., Schirrmeister, L., Grosse, G., Fortier, D., Hugelius, G., Knoblauch, C., et al. (2017). Deep Yedoma Permafrost: A Synthesis of Depositional Characteristics and Carbon Vulnerability. Earth-Science Rev. 172, 75–86. doi:10.1016/j.earscirev.2017.07.007
Strauss, J., Schirrmeister, L., Grosse, G., Wetterich, S., Ulrich, M., Herzschuh, U., et al. (2013). The Deep Permafrost Carbon Pool of the Yedoma Region in Siberia and Alaska. Geophys. Res. Lett. 40, 6165–6170. doi:10.1002/2013GL058088
Strauss, J., Schirrmeister, L., Wetterich, S., Borchers, A., and Davydov, S. P. (2012). Grain‐size Properties and Organic‐carbon Stock of Yedoma Ice Complex Permafrost from the Kolyma lowland, Northeastern Siberia. Glob. Biogeochem. Cycles 26 (3). doi:10.1029/2011GB004104
Stumm, W., and Morgan, J. J. (1995). Aquatic Chemistry: Chemical Equilibria and Rates in Natural Waters, 3rd Edition | Wiley.
Till, A. B., Dumoulin, J. A., Harris, A. G., Moore, T. E., Bleick, H. A., and Siwiec, B. R. (2008). Bedrock Geologic Map of the Southern Brooks Range, Alaska, and Accompanying Conodont Data, 70.
Tjallingii, R., Röhl, U., Kölling, M., and Bickert, T. (2007). Influence of the Water Content on X-ray Fluorescence Core-Scanning Measurements in Soft marine Sediments: XRF Core Scanning. Geochem. Geophys. Geosystems 8. doi:10.1029/2006gc001393
Tomirdiaro, S. V., and Chernen’kiy, O. (1987). Cryogenic Deposits of East Arctic and Sub Arctic. SSSR Far-East-Sci. Cent., 196.
Turetsky, M. R., Abbott, B. W., Jones, M. C., Anthony, K. W., Olefeldt, D., Schuur, E. A. G., et al. (2020). Carbon Release through Abrupt Permafrost Thaw. Nat. Geosci. 13, 138–143. doi:10.1038/s41561-019-0526-0
Ulrich, M., Grosse, G., Strauss, J., and Schirrmeister, L. (2014). Quantifying Wedge-Ice Volumes in Yedoma and Thermokarst Basin Deposits. Permafrost Periglac. Process. 25, 151–161. doi:10.1002/ppp.1810
Velichko, A. A., Catto, N., Drenova, A. N., Klimanov, V. A., Kremenetski, K. V., and Nechaev, V. P. (2002). Climate Changes in East Europe and Siberia at the Late Glacial-Holocene Transition. Quat. Int. 91, 75–99. doi:10.1016/S1040-6182(01)00104-5
Walter Anthony, K., Schneider von Deimling, T., Nitze, I., Frolking, S., Emond, A., Daanen, R., et al. (2018). 21st-century Modeled Permafrost Carbon Emissions Accelerated by Abrupt Thaw beneath Lakes. Nat. Commun. 9, 3262. doi:10.1038/s41467-018-05738-9
Walter, K. M., Edwards, M. E., Grosse, G., Zimov, S. A., and Chapin, F. S. (2007). Thermokarst Lakes as a Source of Atmospheric CH4 during the Last Deglaciation. Science 318, 633–636. doi:10.1126/science.1142924
Wang, X., Toner, B. M., and Yoo, K. (2019). Mineral vs. Organic Matter Supply as a Limiting Factor for the Formation of mineral-associated Organic Matter in forest and Agricultural Soils. Sci. Total Environ. 692, 344–353. doi:10.1016/j.scitotenv.2019.07.219
Weindorf, D. C., Bakr, N., and Zhu, Y. (2014a). Advances in Portable X-ray Fluorescence (PXRF) for Environmental, Pedological, and Agronomic Applications. in Advances in Agronomy. Elsevier, 1–45. doi:10.1016/B978-0-12-802139-2.00001-9
Weindorf, D. C., Bakr, N., Zhu, Y., Mcwhirt, A., Ping, C. L., Michaelson, G., et al. (2014b). Influence of Ice on Soil Elemental Characterization via Portable X-Ray Fluorescence Spectrometry. Pedosphere 24, 1–12. doi:10.1016/S1002-0160(13)60076-4
Weiss, N., Blok, D., Elberling, B., Hugelius, G., Jørgensen, C. J., Siewert, M. B., et al. (2016). Thermokarst Dynamics and Soil Organic Matter Characteristics Controlling Initial Carbon Release from Permafrost Soils in the Siberian Yedoma Region. Sediment. Geology 340, 38–48. doi:10.1016/j.sedgeo.2015.12.004
Weltje, G. J., and Tjallingii, R. (2008). Calibration of XRF Core Scanners for Quantitative Geochemical Logging of Sediment Cores: Theory and Application. Earth Planet. Sci. Lett. 274, 423–438. doi:10.1016/j.epsl.2008.07.054
Wetterich, S., Grosse, G., Schirrmeister, L., Andreev, A. A., Bobrov, A. A., Kienast, F., et al. (2012). Late Quaternary Environmental and Landscape Dynamics Revealed by a Pingo Sequence on the Northern Seward Peninsula, Alaska. Quat. Sci. Rev. 39, 26–44. doi:10.1016/j.quascirev.2012.01.027
Wetterich, S., Kuzmina, S., Andreev, A. A., Kienast, F., Meyer, H., Schirrmeister, L., et al. (2008). Palaeoenvironmental Dynamics Inferred from Late Quaternary Permafrost Deposits on Kurungnakh Island, Lena Delta, Northeast Siberia, Russia. Quat. Sci. Rev. 27, 1523–1540. doi:10.1016/j.quascirev.2008.04.007
Wetterich, S., Rudaya, N., Tumskoy, V., Andreev, A. A., Opel, T., Schirrmeister, L., et al. (2011). Last Glacial Maximum Records in Permafrost of the East Siberian Arctic. Quat. Sci. Rev. 30, 3139–3151. doi:10.1016/j.quascirev.2011.07.020
Wetterich, S., Schirrmeister, L., Andreev, A. A., Pudenz, M., Plessen, B., Meyer, H., et al. (2009). Eemian and Late Glacial/Holocene Palaeoenvironmental Records from Permafrost Sequences at the Dmitry Laptev Strait (NE Siberia, Russia). Palaeogeogr. Palaeoclimatol. Palaeoecol. 279, 73–95. doi:10.1016/j.palaeo.2009.05.002
Wetterich, S., Tumskoy, V., Rudaya, N., Andreev, A. A., Opel, T., Meyer, H., et al. (2014). Ice Complex Formation in Arctic East Siberia during the MIS3 Interstadial. Quat. Sci. Rev. 84, 39–55. doi:10.1016/j.quascirev.2013.11.009
Wilson, M. J. (2004). Weathering of the Primary Rock-Forming Minerals: Processes, Products and Rates. Clay miner. 39, 233–266. doi:10.1180/0009855043930133
Wilson, S. A. (1997). Data Compilation for USGS Reference Material BHVO-2, Hawaian Basalt. US Geol. Surv. Open-file Rep. 2.
Windirsch, T., Grosse, G., Ulrich, M., Schirrmeister, L., Fedorov, A. N., Konstantinov, P. Y., et al. (2020). Organic Carbon Characteristics in Ice-Rich Permafrost in Alas and Yedoma Deposits, central Yakutia, Siberia. Biogeosciences 17, 3797–3814. doi:10.5194/bg-17-3797-2020
Yang, Z. B., You, J. F., and Yang, Z. M. (2008). Manganese Uptake and Transportation as Well as Antioxidant Response to Excess Manganese in Plants. Zhi Wu Sheng Li Yu Fen Zi Sheng Wu Xue Xue Bao 33, 480–488.
Yool, A., and Tyrrell, T. (2003). Role of Diatoms in Regulating the Ocean's Silicon Cycle. Glob. Biogeochem. Cycles 17, a–n. doi:10.1029/2002GB002018
Young, K. E., Evans, C. A., Hodges, K. V., Bleacher, J. E., and Graff, T. G. (2016). A Review of the Handheld X-ray Fluorescence Spectrometer as a Tool for Field Geologic Investigations on Earth and in Planetary Surface Exploration. Appl. Geochem. 72, 77–87. doi:10.1016/j.apgeochem.2016.07.003
Keywords: thaw, alas, thermokarst, mineralogy, late pleistocene – holocene, arctic, X-ray fluorescence, bootstrapping technique
Citation: Monhonval A, Mauclet E, Pereira B, Vandeuren A, Strauss J, Grosse G, Schirrmeister L, Fuchs M, Kuhry P and Opfergelt S (2021) Mineral Element Stocks in the Yedoma Domain: A Novel Method Applied to Ice-Rich Permafrost Regions. Front. Earth Sci. 9:703304. doi: 10.3389/feart.2021.703304
Received: 30 April 2021; Accepted: 18 August 2021;
Published: 03 September 2021.
Edited by:
Ko Van Huissteden, Vrije Universiteit Amsterdam, NetherlandsReviewed by:
Trofim Maximov, Institute for Biological Problems of Cryolithozone, Siberian Branch (RAS), RussiaEvgeny Abakumov, Saint Petersburg State University, Russia
Copyright © 2021 Monhonval, Mauclet, Pereira, Vandeuren, Strauss, Grosse, Schirrmeister, Fuchs, Kuhry and Opfergelt. This is an open-access article distributed under the terms of the Creative Commons Attribution License (CC BY). The use, distribution or reproduction in other forums is permitted, provided the original author(s) and the copyright owner(s) are credited and that the original publication in this journal is cited, in accordance with accepted academic practice. No use, distribution or reproduction is permitted which does not comply with these terms.
*Correspondence: Arthur Monhonval, YXJ0aHVyLm1vbmhvbnZhbEB1Y2xvdXZhaW4uYmU=