Spatiotemporal Dynamics and Geodetic Mass Changes of Glaciers With Varying Debris Cover in the Pangong Region of Trans-Himalayan Ladakh, India Between 1990 and 2019
- 1Department of Geoinformatics, University of Kashmir, Srinagar, India
- 2Department of Earth Sciences, University of Kashmir, Srinagar, India
Glaciers across the Himalayan arc are showing varying signs of recession. Glaciers in the eastern and western parts of the Himalayan arc are retreating more rapidly as compared to other regions. This differential retreat is often attributed to climatic, topographic, and geologic influences. The glaciers in the Trans-Himalayan region of Ladakh are believed to be relatively stable as compared to other parts of the western Himalaya. The present study ascertained the area changes and frontal retreat of 87 glaciers in the Pangong Region between 1990 and 2019 using satellite data. The geodetic mass changes were also assessed using SRTM and TanDEM-X digital elevation models of 2000 and 2012 respectively. Besides, the glacier outlines were delineated manually and compared with existing regional and global glacier inventories that are available over the region. The GlabTop model was used to simulate the glacier-bed overdeepenings of four glaciers that are associated with a proglacial lake. The study also analyzed the impact of topographic influences and varying debris cover on glacier recession. This analysis indicated deglaciation of 6.7 ± 0.1% (0.23% a−1) from 1990 to 2019 over the Pangong Region with clean-ice glaciers showing a higher retreat (8.4 ± 0.28%) compared to the debris-covered glaciers (5.7 ± 0.14%). However, the overall recession is lower compared to other parts of northwestern Himalayas. The glacier recession showed a positive correlation with mean glacier slope (r = 0.3) and debris cover (r = 0.1) with bigger size glaciers having retreated at a lesser pace compared to smaller ones. This underpins the need for in-situ data about debris thickness to precisely ascertain the role of debris on glacier recession in the Trans-Himalayan Ladakh where debris thickness data is absent. The mean glacier elevation did not indicate any influence on glacier recession. From 2000 to 12, the glaciers lost an ice mass amounting to 0.33 ± 0.05 m we. per year. The formation of four new proglacial lakes, although small (<6 ha), need to be monitored using remote sensing data while the infrastructure development activities should not be permitted given glacial lake outburst flood risk.
Introduction
Glaciers are important indicators of climate change (Haeberli and Hoelzle, 1995; Roe, 2011) and have significant impacts on water availability (Immerzeel et al., 2009). Changes in the glacier extent (Bolch et al., 2012; Kulkarni and Karyakarte, 2014; Rashid et al., 2017) and mass (Brun et al., 2017; Bandyopadhyay et al., 2019) have been very well documented in many parts of the Himalayas, but comparatively little attention has been paid to glacier monitoring in the Ladakh Region (Chudley et al., 2017; Schmidt and Nüsser, 2017), which besides being at a high altitude happens to be a rain-shadow region (Abdullah et al., 2020). For these reasons, it is also known as a cold desert (Romshoo et al., 2020). Owing to the remoteness of the area (Azam et al., 2018), there is only one field-based glaciological study (Soheb et al., 2020) that reported a negative mass balance of the neighbouring Stok Glacier between 1978 and 2019.
Remote sensing data products have been widely used to monitor the alpine cryosphere (Dar et al., 2014; Pratibha and Kulkarni, 2018; Chand and Watanabe, 2019; Liu et al., 2019; Sujakhu et al., 2019). Various approaches include automatic classification (Paul et al., 2002, 2004; Zhang et al., 2019; Lu et al., 2020), semi-automatic classification (Shukla et al., 2010; Rastner et al., 2014; Sahu and Gupta, 2018; Robson et al., 2020), object-based image algorithms, and manual digitization (Ye et al., 2006; Bhambri et al., 2011; Rashid and Majeed, 2020) have been widely used by remote sensing glaciologists. Manual digitization has been documented to be the most reliable since it takes the cognitive inputs from the analyst (Paul et al., 2013), however, it is time-consuming and subjective to the skill of the analyst. Besides areal changes, remote sensing has a capacity to map volumetric changes; the most important being the DEM products that are mostly stereo-products (Cogley, 2009; Gaddam et al., 2021). Owing to the lack of field data, rugged topography, and remote locations, the geodetic mass change assessments offer the easiest choice to estimate volume changes. However, such estimates are associated with uncertainties, which need to be quantified for reporting an accurate estimate.
While glacier recession is primarily attributed to atmospheric warming (Wang et al., 2017; Cook et al., 2019) and an increase in the anthropogenic footprint in the glacier and peri-glacier environments (Wang et al., 2019; Huggel et al., 2020), there could be several topographic and geological factors (Garg et al., 2017; Patel et al., 2018) that could affect glacier health. The variable debris cover on the glaciers could be a controlling factor (Barrand and Murray, 2006; Shukla and Qadir, 2016; Salerno et al., 2017), however, a recent study on the Karakoram (Muhammad et al., 2020) negates the role of thin debris cover on glacier recession.
The long-term records of the hydro-meteorological data are almost absent, which hampers quantifying any historic changes in the glaciers of the region. Although the use of gridded climate datasets for glaciohydrological assessments has been documented (Bhattacharya et al., 2021), such datasets need bias correction (Hussain et al., 2017; Kanda et al., 2020). The prevalent warming temperatures over the western Himalayas aid in glacier recession and also result in the formation of numerous proglacial lakes and expansion of already existing ones (Worni et al., 2013; Wang et al., 2015). These lakes are growing in both area and number (Nie et al., 2017; Rashid and Majeed, 2018). One of the most dangerous hazards that these proglacial lakes pose is that of a glacial lake outburst flood (Vuichard and Zimmermann, 1987; Liu et al., 2013; Allen et al., 2019). This calls for increased attention, as they can prove to be fatal in case of a sudden outburst. There are just a few studies (Govindha Raj, 2010; Mir et al., 2018; Rashid and Majeed, 2018) of these proglacial lakes in the region. This necessitates a need for continuous monitoring and detailed risk assessment for precisely quantifying the risk that these lakes pose to the downstream communities and infrastructure. To our knowledge, there is only one detailed study that reconstructed a GLOF event in the Ladakh region (Majeed et al., 2021). The receding glaciers and the damming of water behind the moraines could alter the hydrological regimes (Singh and Bengtsson, 2004; Immerzeel et al., 2010, 2012) in the region and cause water availability issues (Miller et al., 2012). This could have serious implications on the socio-economy of the region and threaten food security (Misra, 2014; Bocchiola et al., 2019).
This study aims to map the glaciers of the Pangong region, Trans-Himalaya Ladakh. Multi-temporal satellite datasets were used to assess the area changes and frontal retreat of the glaciers in the region. A qualitative comparison was carried out by comparing the glacier outlines delineated in this study with three existing global and regional glacier inventories. The topographic characteristics and debris cover were correlated with glacier area changes. The geodetic mass changes were quantified using multi-date DEMs of 2000 and 2012. In addition, the GlabTop model was used to simulate the proglacial lake expansion utilizing information about glacier bed overdeepenings.
Study Area
This study focused on the glaciers of the Pangong Mountain Range (PMR), in the northern region of Ladakh. The PMR runs parallel to the Ladakh Range (Godwin-Austen, 1867) ∼100 km northwest from Chushul. The highest elevation in the range is 6,700 m and the northern slopes are heavily glaciated. This area is situated on the left bank of Pangong Lake but lies at an elevation of 1,400 m above the lake. Pangong Lake is an endorheic lake in the Himalayas (Rathour et al., 2020) situated at a height of about 4,350 m. It is 134 km long and extends from India to China. Approximately 60% of the length of the lake lies in China. The lake is 5 km wide at its broadest point and covers a total area of 604 km2. Geographically, it is not a part of the Indus River basin area and is a separate land-locked river basin without any outlet. While the total area of the watershed is 844 km2, the glaciated area is ∼60 km2 which amounts to a total of just ∼7%. There are a total of 87 glaciers with a cumulative area of 59.45 ± 6.86 km2 as mapped from satellite data of 1990. These glaciers are relatively small with an average size of 0.8 km2. The smallest glacier has an area of 0.29 ± 0.01 km2 while the largest has an area of 4.31 ± 0.35 km2. Most of the glaciers are debris-covered. The watershed lies between latitudes 33°36′ N—34°2′ N and longitudes 78°14′ E—78°39′ E (Figure 1). The mean elevation of the watershed is 5,109 m. The summers are mild, while the winters are extreme in the region (Srivastava et al., 2020). The lake freezes completely in winters. Pangong receives winter precipitation mostly by Mediterranean influences.
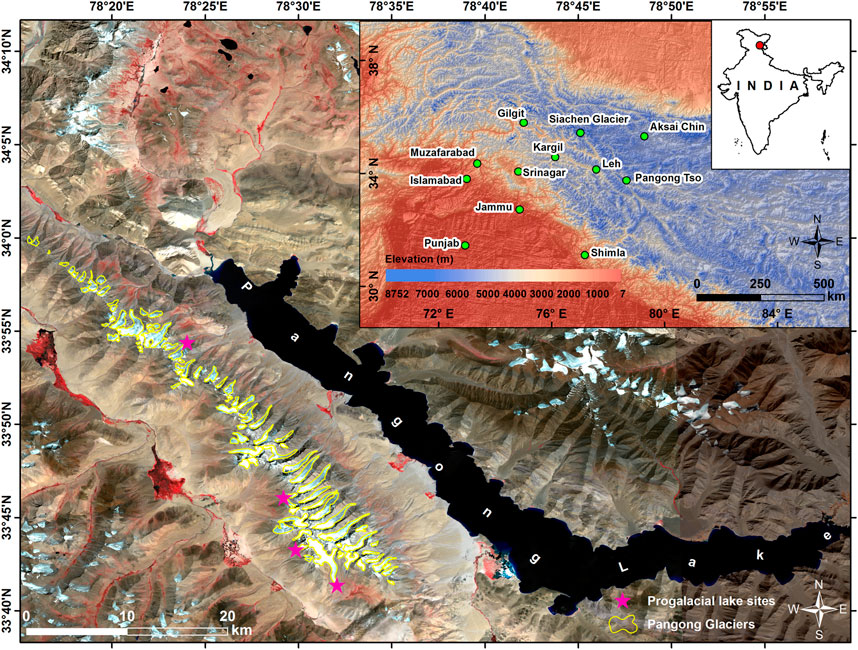
FIGURE 1. Location of the study area. Glaciers (yellow outlines) and proglacial lakes (pink stars) of the Pangong Mountain Range draped on the Sentinel 2A image (August 28, 2017). Inset image: Major locations around Pangong Region draped on 30-arc second GTOPO DEM. Red dot in the India map indicates Pangong Region.
Materials and Methods
Data
A repository of satellite data and DEMs were used to carry out this study. The snow-free images from 1990 to 2019 were used for areal changes of the glaciers while two different DEMs were used for quantifying the mass changes. The details of these datasets are provided in Table 1.
Methods
Glacier Changes
The standard geometric correction (Jensen, 2005) was used to coregister the images. The images from 1990 to 2019 were coregistered to arrive at an accuracy of one pixel. The glaciers were manually digitized at a scale of 1:30,000. Mountain shadows, cloud cover, and debris cover limit the accuracy of the glacier mapping. However, alternate images from ±2 years were used to overcome this. All the glacier boundaries were validated using high-resolution Google Earth data. Proglacial lakes are also mapped from the same images. The precision of the glacier boundary delineation is within half a pixel (Bolch et al., 2010; Paul et al., 2013). For the 1990s outlines, we assumed a mapping inaccuracy of half a pixel (∼15 m) for Landsat TM data and one pixel (∼3 m) for Planet Cubesat data (Pieczonka and Bolch, 2015; Bhattacharya et al., 2016).
The uncertainties related to the area changes (EAC) was calculated considering the law of error propagation as (Hall et al., 2003):
where EAC is the uncertainty related to the change in the area between two time periods, EA1 is the uncertainty of glacier area at one point in time and EA2 is the uncertainty of glacier area at the second point in time.
The uncertainties related to snout changes between two times (ESC) is given by the following formula (Hall et al., 2003):
where ESC is the uncertainty in snout change, λ1 and λ2 are the spatial resolutions of the two images used for calculating the snout changes.
Glacier Debris and Topographic Parameters
The supraglacial debris was manually delineated from the high-resolution Planet Cubesat images for the year 2019. Besides high-resolution Google Earth imagery was used to validate the debris cover. The topographic parameters that include slope, elevation, and southerly aspect of the glaciers were estimated using TanDEM-X in a GIS environment. The topographic parameters and debris cover were co-related with glacier recession rates to arrive at trends.
Geodetic Mass Balance
The glaciological method to estimate mass loss is a challenging task given the costs involved and also the remoteness of glaciers (Rashid et al., 2021). Many glaciers in the Jammu and Kashmir region are inaccessible for direct measurements due to the ruggedness of the terrain (Immerzeel et al., 2009; Bolch et al., 2019). It is pertinent to mention that long-term glaciological mass balance measurements in the trans-Himalayan region of Ladakh do not exist, however, there have been some initiatives very recently that attempt to establish long-term field-based glacial mass balance records in Stok Kangri (Soheb et al., 2020) and Zanskar regions (Mehta et al., 2021). On the contrary, the geodetic mass balance assessment is a quick method to access mass losses in the glaciers. It simply involves the DEMs of two times and differencing to arrive at thickness changes (Braithwaite, 2002). This thickness change can be converted to corresponding mass loss using glacier area and density assumptions (Cogley, 2009; Muhammad et al., 2019; Rashid and Majeed, 2020). For quantifying the geodetic mass changes over the Pangong region, SRTM C band DEM of 2000 and TanDEM-X of 2012 were used. A correction factor of 3.4 m for SRTM C band penetration in glacier ice was used as suggested by Gardelle et al. (2013). TanDEM-X is a stacked product with data acquired over multiple seasons/years. Hence, a uniform radar penetration is unlikely. The potential radar penetration of the X band was ignored due to its high frequency and non-uniform penetration across different seasons (Huber et al., 2020). The DEMs were coregistered using the universal co-registration algorithm (Nuth and Kääb, 2011). Further, the outliers in the off-glacier (Supplementary Figure S1) and on-glacier elevation (Supplementary Figure S3) were identified and removed using the inter-quartile range approach suggested by Barbato et al. (2011) and adopted by Huber et al. (2020). The DEM differencing results were interpolated using Inverse Distance Weighted (IDW) algorithm over the voided areas (13.49%) of SRTM DEM. The elevation-dependent outliers in the on-glacier DEM difference image were ignored since the glaciers lie in a homogenous topographic zone (Mean glacier elevation: 5,613–6,534 m asl).
The uncertainty in geodetic mass balance (∇M) related to glacier area (A), ice density (ρ) assumed to be 850 ± 60 kg m−3, and surface elevation change (h) is expressed as (Ramsankaran et al., 2019):
where ∇ represents the uncertainty in estimates.
The uncertainty in elevation change (σΔh) is calculated based on the following formula Huber et al. (2020):
where σem is the uncertainty in DEM, σvoidfill is the uncertainty introduced by the void-filling approach, and σTDXdate is the date uncertainty of TanDEM-X and estimated to be ± 2 times the change of elevation per year. The uncertainty in the void filling was assumed negligible since the observed and predicted values for non-voided areas showed an insignificant bias (0.02 m).
The σdem was calculated after the approach suggested by McNabb et al. (2019) as:
where σΔz is the mean of glacier difference between the two DEMs after coregistration, A is the glacier area, σarea is the uncertainty in glacier area and ΔZ is the mean elevation difference of on-glacier pixels.
Glacier Bed Overdeepenings
Glacier-bed overdeepenings and ice thickness were estimated for four glaciers associated with proglacial lakes in the Pangong region using the GlabTop model to check the possibility of expansion of existing proglacial lakes in the future. The input parameters to the model are glacier outlines, DEM, and the glacier flowlines. In this study, the glacier outlines and flowlines were manually delineated from the satellite data and also using a 3D perspective from Google Earth. The glacier bed overdeepenings were modeled using glacier outlines and flowlines and ALOS PALSAR DEM. The model has been extensively used for predicting ice thickness and glacier bed overdeepenings in the Himalayas with an uncertainty of 30% (Linsbauer et al., 2016; Rashid and Majeed, 2020; Sattar et al., 2021), however, the uncertainty in the ice thickness estimates could be reduced to 17% using better quality DEMs and improved shape factor (Ramsankaran et al., 2018; Majeed et al., 2021). The model assumes constant basal stress along the central flowline of a glacier (Linsbauer et al., 2012) and quantifies ice-thickness using a slope-dependent approach (Haeberli and Hoelzle, 1995).
Comparison With Existing Glacier Inventories
The various glacier inventories available for this region include the Randolph Glacier Inventory (RGI), Glacier Area Mapping for Discharge from the Asian Mountains (GAMDAM), and International Council for Integrated Mountain Development (ICIMOD) Glacier Inventories. A comparative analysis is made between these inventories and the glacier inventory generated in this study.
Results
Glacier Area and Frontal Changes
Satellite data revealed a total of 87 glaciers in the study area covering an area of 54.8 ± 2.53 km2 in 1990. The glacier cover reduced to 51.1 ± 1.03 km2 by 2019 indicating a 6.7 ± 0.1% area loss at a rate of 0.23% a−1 (Figure 2; Table 2). While the clean-ice glaciers retreated by 8.4 ± 0.28% (1.6 km2), the debris-covered glaciers lost 5.7 ± 0.14% (2.03 km2) at a rate of 0.29% a−1 and 0.2% a−1 respectively. The glacier size varies between 3.5 and 353.4 ha with an average size of 58.8 ha. The snout recession, with an uncertainty of ± 30.1 m, varied between 0 m (GLIMS Id G33717E78538N) to 557 m (GLIMS Id G33725E78536N) at an average of 126 m (4.3 m a−1).
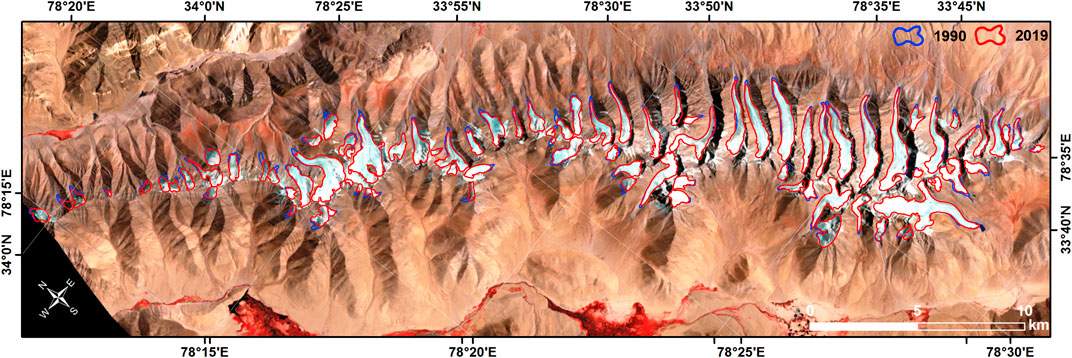
FIGURE 2. Area changes and frontal retreat of Pangong group of glaciers from 1990 to 2019. Background image: Sentinel 2A of August 28, 2017.
The mean glacier elevation for all the glaciers is more than 5,600 m asl ranging from 5,613 m asl to 6,534 m asl. Analysis of glacier area changes does not show any significant correlation (r = -0.14) with the mean glacier elevation (Figure 3A). The mean glacier slope varied between 13.6° and 40.9°. The mean glacier slope showed a positive correlation (r = 0.3) with glacier recession (Figure 3B) which was further researched by ascertaining the frontal slope of each glacier. The frontal slope was calculated for the 400 m area from the snout of each glacier. The mean frontal slope for the glaciers of the Pangong Region varied between 6.5° and 39.9°. The frontal slope also showed a similar positive correlation (r = 0.31) with glacier recession.
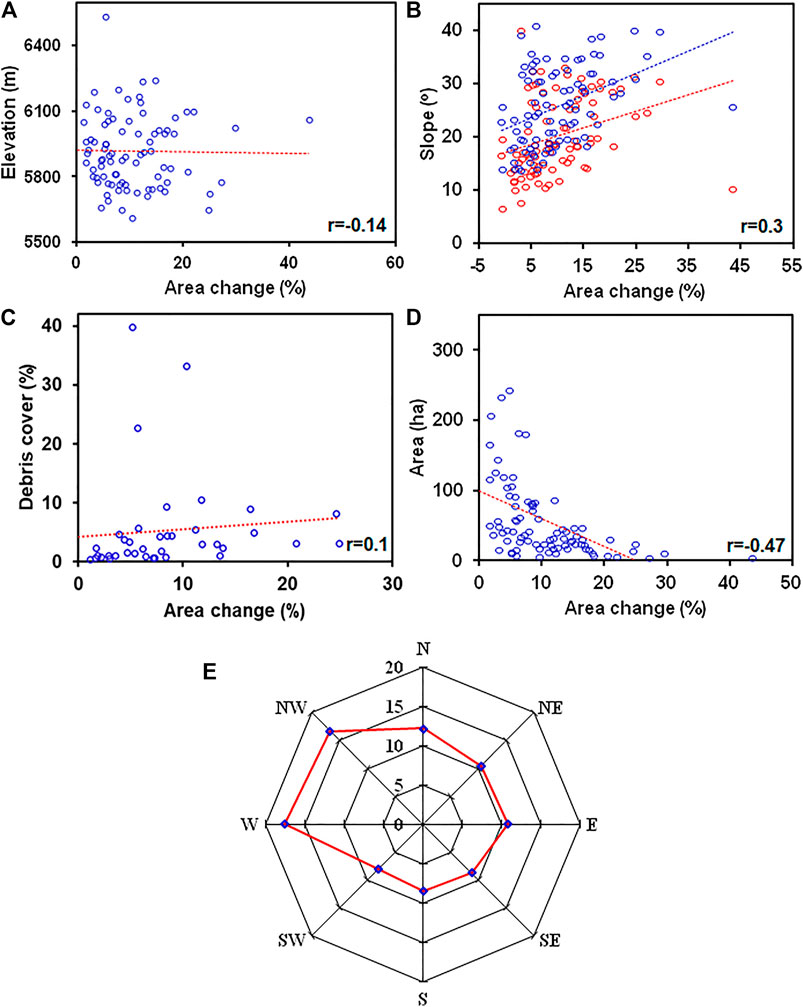
FIGURE 3. Correlation of glacier area changes with (A) Mean glacier elevation, (B) Mean glacier slope, (C) Debris cover, (D) Glacier area, and (E) Slope-aspect.
Based on the debris cover, the glaciers were categorized into clean-ice and debris-covered glaciers (Figure 3C). This analysis indicated 48 (∼55%) out of 87 glaciers were clean-ice glaciers and 39 (45%) were debris-covered with debris-cover varying from 0.2–39.8%. This analysis indicated that glacier recession has a slightly positive correlation with debris cover (r = 0.1) which needs to be further investigated. The glacier area showed an inverse relation (r = -0.47) with glacier recession (Figure 3D) indicating smaller glaciers recede faster than larger ones. Moreover, the role of slope-aspect was correlated with glacier recession (Figure 3E). Analysis of slope-aspect indicated that glaciers having west and northwest-facing slopes lose area slightly faster compared to south-facing glaciers.
Geodetic Mass Balance
DEM differencing indicated that the Pangong group of glaciers, on average, lost 4.59 ± 0.64 m at a rate of 0.38 ± 0.05 m a−1 between 2000 and 2012 with the highest thickness loss of 1.45 m a−1 and the highest gain of 0.22 m a−1 (Figure 4). While the surface of clean-ice glaciers lowered by 0.28 m a−1 the ice loss for debris-covered glaciers was estimated to be 0.43 m a−1. Glacier-wise the highest mean surface lowering of 0.78 m a−1 was observed in TS ID 30 (GLIMS ID G33921E78371N) and the highest surface gain of 0.002 m a−1 was observed for TS ID 75 (GLIMS ID G33854E78436N). The mass loss corresponding to surface lowering for these glaciers is calculated to be 199.48 Mt from 2000 to 2012. This is indicative of ice loss at the rate of 16.62 Mt a−1. The equivalent water loss is estimated to be 3.9 ± 0.54 m we. at a rate of 0.33 m w. e. per year. For clean-ice glaciers, the estimated equivalent water loss is 0.24 m we. per year. However, for debris-covered glaciers, the equivalent water loss is 0.37 m we. per year.
Glacier Thickness and Bed Overdeepenings
Out of the total 87 glaciers mapped in the Pangong Region, four glaciers (GLIMS ID: G33715E78523N, G33723E78503N, G33763E78492N, and G33897E78392N) are associated with a proglacial lake. These lakes have an area of 5.74 ± 0.16 ha, 0.42 ± 0.04 ha, 0.23 ± 0.03 ha and 0.56 ± 0.05 ha, respectively, GlabTop model analysis simulated a mean glacier ice thickness of 58 m with a maximum and minimum thickness of 1 and 145 m, respectively (Figure 5). It further indicated that the proglacial lake associated with G33715E78523N can further grow by 1.72 ha reaching a maximum area of 7.46 ha. The model suggests the formation of eight additional glacier bed overdeepenings for the glacier most of which lie in the upper ablation zone with areas less than a hectare. For G33723E78503N the ice thickness varies between 1 and 48 m with a mean thickness of 19 m. The model does not simulate any expansion of the proglacial lake, however, it predicted the formation of a new lake with an area of 1.06 ha around the middle of the ablation zone of the glacier. For G33763E78492N the ice thickness varies between 1 and 34 m with a mean of 17 m. The model predicted expansion of the existing proglacial lake by 0.36 ha reaching a maximum area of 0.59 ha. The model indicated the formation of two more bed overdeepenings with an area of 0.03 and 0.06 ha in the upper ablation zone. For G33897E78392N the ice thickness varies between 1 and 75 m with a mean of 31 m. The model pointed to the expansion of the existing proglacial lake by 0.87 ha reaching a maximum area of 1.43 ha. Further, the model predicted three additional bed overdeepenings in the upper ablation zone.
Comparison With Existing Glacier Inventories
There is a huge uncertainty concerning the total number of glaciers in the Himalayas (Bolch et al., 2012; Azam et al., 2021), the objective of comparison of existing global and regional inventories was made with the inventory generated in this study. Of the three glacier inventories—CIMOD, GAMDAM, and RGI—available for the region, GAMDAM glacier inventory captured all the glaciers that were delineated in this study (Figure 6; Table 3). The total number of glaciers in the GAMDAM inventory for the Pangong Region is 132 which cover an area of 72.7 km2 indicating an overestimation by 51% (45 glaciers) in the number of glaciers and 42.3% (21.6 km2) in glacier area. The smallest glacier area mapped in this study has an area of 3.49 ha compared to 1.03 ha mapped in the GAMDAM inventory. Similarly, the largest glacier area mapped in this study is 353.4 ha compared to 418.5 ha in GAMDAM. The other two inventories, ICIMOD and RGI, grossly underestimate the total number of glaciers and total glacier area in the Pangong Region. The ICIMOD glacier inventory suggests 44 glaciers spread over 19.9 km2 indicating an underestimation of 49.4% (43 glaciers) total number of glaciers and 61.1% (31.3 km2) in the glacier area. The RGI suggests 50 glaciers spread over 26.6 km2 indicating an underestimation of 42.5% (37 glaciers) total number of glaciers and 61.1% (24.6 km2) in the glacier area.
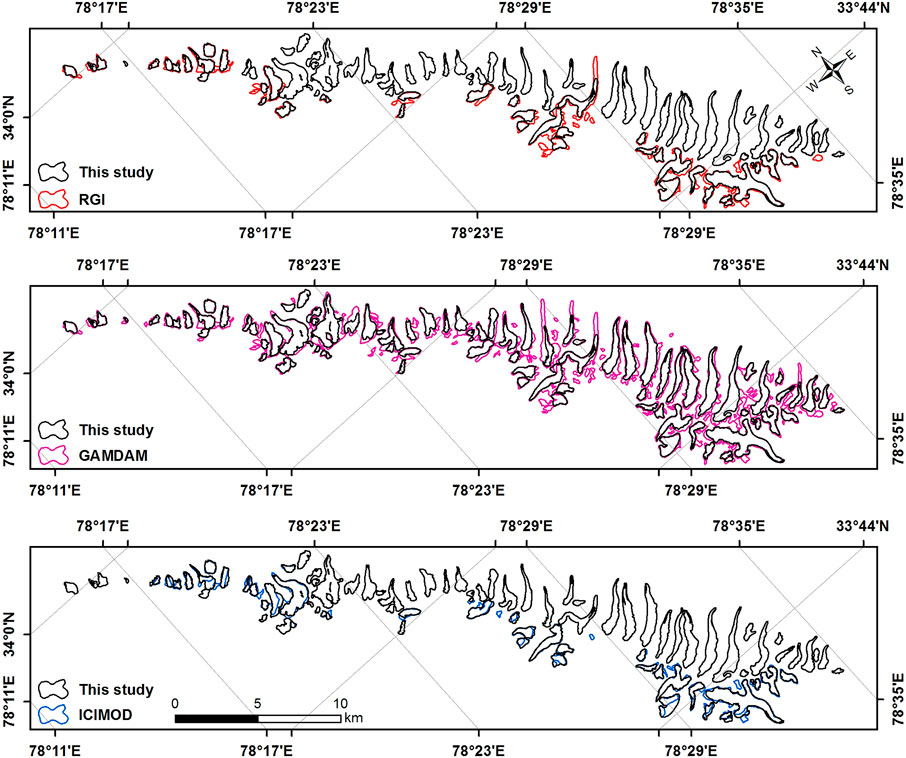
FIGURE 6. Comparison of glacier outlines delineated in this study with Randolph Glacier Inventory (RGI), GAMDAM glacier inventory, and ICIMOD glacier inventory.
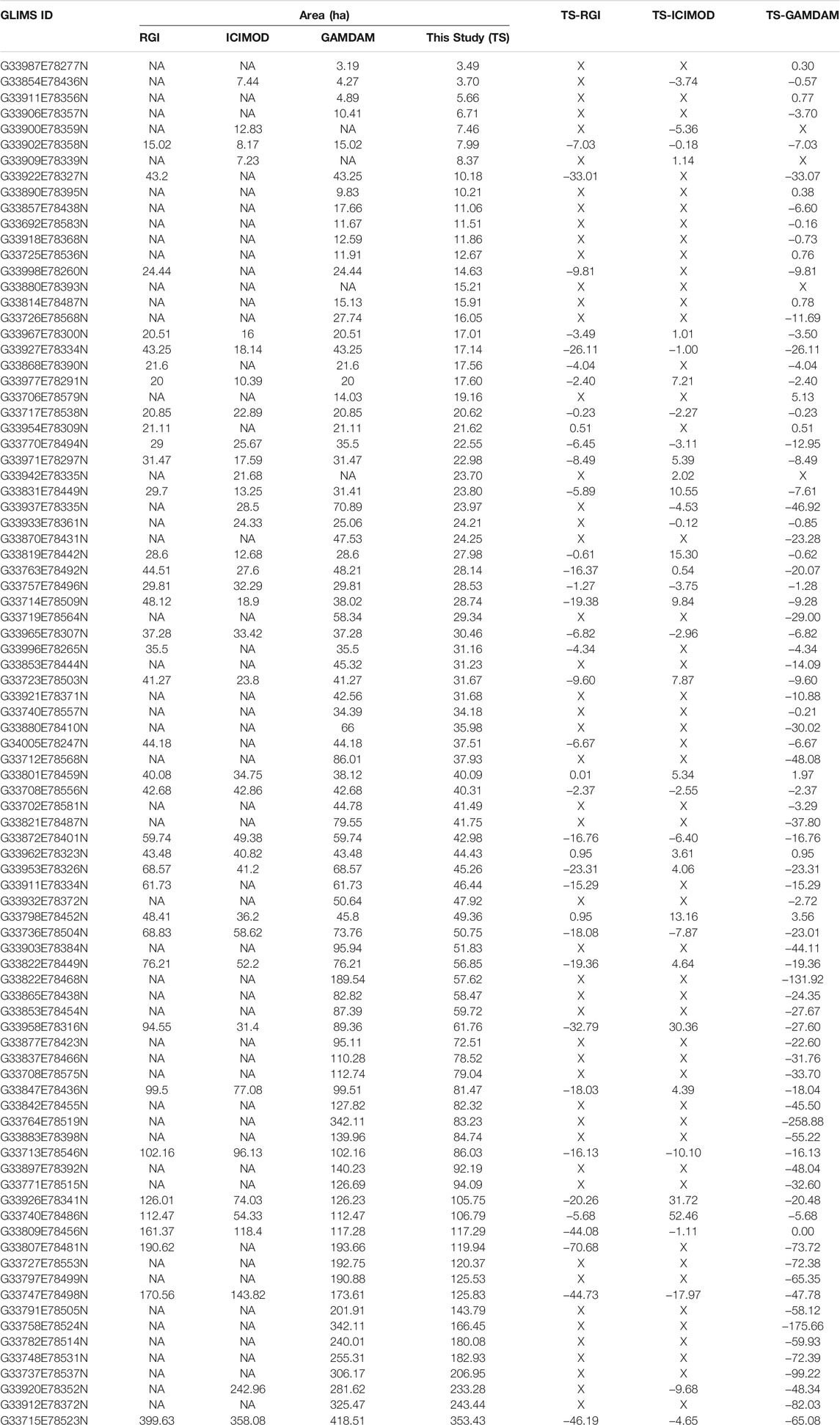
TABLE 3. Comparison of the present inventory (TS) with the existing inventories (RGI, ICIMOD and GAMDAM).
Discussion
This analysis indicated that the glaciers in Pangong Region have been retreating like the other glaciated basins in northwestern Himalaya. However, the rate of retreat is lower since the study area is located in the Trans-Himalayan Ladakh region-a rain shadow area (Raj and Sharma, 2013; Kumar et al., 2017). Based on the existing knowledge and compared to other Trans-Himalayan glaciated regions, the area experienced the second-lowest retreat (Figure 7, Supplementary Table S1). The frontal changes of glaciers in the Pangong Region, also on the lower side, are consistent with the previously documented studies over the region (Majeed et al., 2021).
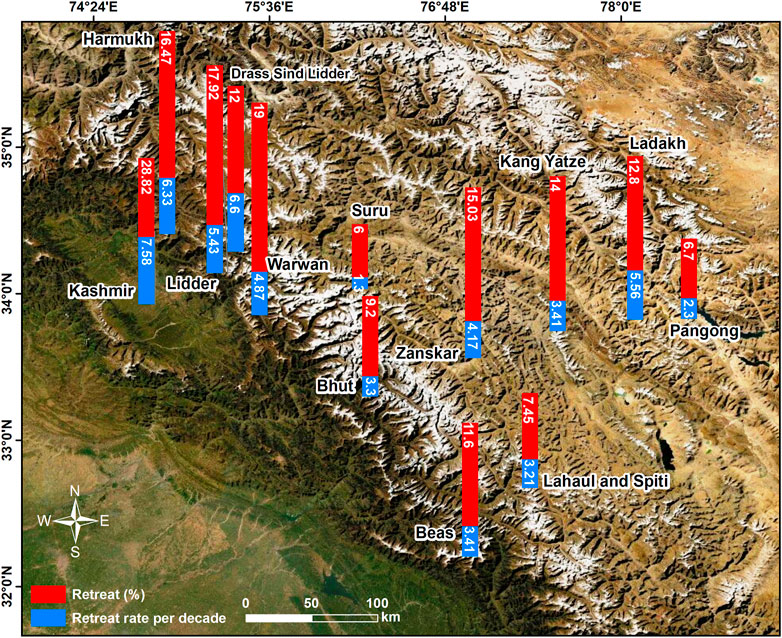
FIGURE 7. Comparison of glacier retreat of Pangong region with neighbouring areas in western Himalaya. Red bar indicates total loss (as %) and blue bar indicates loss per decade (as %).
The glacier recession in Pangong was correlated with topographic parameters and debris cover to better understand the influence of these parameters on glacier melt. This analysis indicated that the mean glacier elevation does not have any impact on the recession at higher elevations. It is pertinent to mention that the mean glacier elevation of the Pangong group of glaciers lies above ∼5,600 m asl. Analysis of mean glacier slope shows a positive correlation with glacier recession. This anomalous behavior was further analyzed by taking into consideration the frontal slope of each glacier which was generally flatter than the mean glacier slope indicating that the glaciers with flatter frontal slopes retreated faster. Analysis of debris cover indicated a weak positive correlation with glacier melt. Although there are no in-situ debris thickness data the visual analysis generally indicated a thin debris layer on the Pangong group of glaciers. It is pertinent to mention that Muhammad et al. (2020) also suggested that the thin debris layers do not influence the melting of glaciers in the high-altitude Karakoram. Glacier area showed a negative correlation with glacier recession which has been established by many previous studies (Ceballos et al., 2006; Debnath et al., 2019; Zhao et al., 2020).
While this analysis indicated that the Pangong group of glaciers thinned at a rate of 0.38 ± 0.05 m a−1 between 2000 and 2012, Abdullah et al. (2020) report a similar retreat of 0.46 m a−1 for the Pangong region. The uncertainty estimates of surface lowering and mass change in this study are higher given the fact that the ice loss is lower. Some other mass balance studies over the region suggest comparatively lesser mass changes. For instance, a study by Brun et al. (2017), who used ASTER stereo pairs for estimating the mass changes of the glaciers, suggested a loss of ∼0.5 m a−1 of glacier ice. A relatively older study by Kääb et al. (2012) also puts the ice loss estimate for this region at ∼0.5 m a−1. Vijay and Braun (2018) have put up similar estimates for the glaciers located towards the eastern part of the Pangong region. The differences in the ice loss estimates could be a manifestation of the different DEMs, analysis techniques and the time period for which the mass changes were estimated.
GlabTop model simulations indicate that out of the four glaciers associated with a proglacial lake, three lakes could expand. While the proglacial lake associated with G33723E78503N might not expand, there is a formation of a 1.06 ha lake in the midst of the ablation zone also evident on satellite data and Google Earth imagery of August 2013 and June 2016. It is pertinent to mention that the model has been tested in different glaciated regions of the European Alps (Linsbauer et al., 2012; Magnin et al., 2020) and Himalayas (Linsbauer et al., 2016; Pandit and Ramsankaran, 2020) including Trans-Himalayan Ladakh (Rashid and Majeed, 2018; Majeed et al., 2021) for ice-thickness and glacier-bed overdeepenings. Given the prevailing glacier melt scenario over the seismically-active Jammu and Kashmir region, the development of new proglacial lakes and the formation of already existing ones would pose a significant glacial lake outburst (GLOF) risk to the downstream communities and infrastructure. Studies indicate that there have already been a few incidences of GLOF (Schmidt et al., 2020) and cloudburst (Kumar et al., 2012) in the Trans-Himalayan Ladakh.
Another important aspect of this study was to compare the glacier inventory generated in this study with the existing global and regional inventories. This analysis indicated that only the GAMDAM glacier inventory captured all the glaciers that were delineated in the current effort. This could be attributed to the fact that while ICIMOD and RGI inventories have been synthesized by automatic and semiautomatic methods, the glaciers in GAMDAM inventory have been delineated manually which allows for cognitive inputs from the analyst, unlike automated methods. The overestimation in both area and number of glaciers in GAMDAM glacier inventory could be attributed to the mapping of smaller seasonal or perennial snowpacks without any proper ablation and accumulation zones having been delineated as glaciers in the region.
Conclusion
This analysis utilized remote sensed data including satellite images and DEMs to quantify glacier recession, frontal retreat, and geodetic mass changes of the Pangong group of glaciers in Trans-Himalayan Ladakh. Satellite data analysis revealed that the glaciers retreated conservatively at 0.23% per year between 1990 and 2019 as compared to other regions of the western Himalaya. The geodetic mass change estimates for the selected glaciers indicated a surface lowering of 0.38 ± 0.05 m a−1. While clean-ice and debris-covered glaciers respectively lost 8.4 and 5.7% of the area, the surface lowering was more for debris-covered glaciers (0.43 m a−1) compared to clean-ice glaciers (0.28 m a−1). However, this needs to be researched further through extensive ground surveys aimed at collecting debris thickness measurements in the study area. It is pertinent to mention that debris-thickness measurements are absent in Trans-Himalayan Ladakh and scanty in the Indian Himalaya. Further, glacier recession was correlated with topographic parameters and debris cover which indicated that mean glacier elevation does not influence recession patterns in the Pangong region owing to the relatively higher elevation of glaciers (∼5,600 asl) in the study area. There is an inverse relation between glacier size and recession which has been widely reported in previous studies. The mean glacier slope showed a positive correlation with glacier recession indicating that the glaciers with flatter tongues are retreating faster compared to steeper ones. The glacier outlines mapped manually in this study were compared with the existing three regional/global glacier inventories (ICIMOD, GAMDAM, and RGI). Although GAMDAM glacier inventory captured most of the glaciers it overestimated the glacier area and number owing to the fact that smaller snowpacks were delineated as glaciers. The GlabTop model simulations indicated expansion of three proglacial lakes and formation of a new periglacial lake which could be potential lake outburst hotspots given that the region is experiencing a temperature warming scenario and the glaciers lie in a seismically active area. There is a potential for catastrophic glacial floods and any infrastructure development in the downstream areas should not proceed without a detailed land-use plan.
Data Availability Statement
The raw data supporting the conclusion of this article will be made available by the authors, without undue reservation.
Author Contributions
IR conceptualized and designed the study. UM, IR, NN, and NG performed data analysis. IR wrote the first draft of the manuscript. UM and NN wrote sections of the manuscript. All authors contributed to manuscript revision, read, and approved the submitted version.
Conflict of Interest
The authors declare that the research was conducted in the absence of any commercial or financial relationships that could be construed as a potential conflict of interest.
Publisher’s Note
All claims expressed in this article are solely those of the authors and do not necessarily represent those of their affiliated organizations, or those of the publisher, the editors and the reviewers. Any product that may be evaluated in this article, or claim that may be made by its manufacturer, is not guaranteed or endorsed by the publisher.
Acknowledgments
The authors are thankful to USGS for making the Landsat data freely available to users. The authors also express gratitude to Andreas Linsbauer, University of Zurich for providing the GlabTop model. The first author also acknowledges the support of Department of Science and Technology, Government of India (DST, GoI) for INSPIRE fellowship (Grant Number: IF180682) for pursuing Ph.D. The comments and suggestions from the two reviewers greatly improved the manuscript structure and content.
Supplementary Material
The Supplementary Material for this article can be found online at: https://www.frontiersin.org/articles/10.3389/feart.2021.748107/full#supplementary-material
References
Abdullah, T., Romshoo, S. A., and Rashid, I. (2020). The Satellite Observed Glacier Mass Changes over the Upper Indus Basin during 2000-2012. Sci. Rep. 10, 14285. doi:10.1038/s41598-020-71281-7
Allen, S. K., Zhang, G., Wang, W., Yao, T., and Bolch, T. (2019). Potentially Dangerous Glacial Lakes across the Tibetan Plateau Revealed Using a Large-Scale Automated Assessment Approach. Sci. Bull. 64, 435–445. doi:10.1016/j.scib.2019.03.011
Azam, M. F., Kargel, J. S., Shea, J. M., Nepal, S., Haritashya, U. K., Srivastava, S., et al. (2021). Glaciohydrology of the Himalaya-Karakoram. Science 373, eabf3668. doi:10.1126/SCIENCE.ABF3668
Azam, M. F., Wagnon, P., Berthier, E., Vincent, C., Fujita, K., and Kargel, J. S. (2018). Review of the Status and Mass Changes of Himalayan-Karakoram Glaciers. J. Glaciol. 64, 61–74. doi:10.1017/jog.2017.86
Bandyopadhyay, D., Singh, G., and Kulkarni, A. V. (2019). Spatial Distribution of Decadal Ice-Thickness Change and Glacier Stored Water Loss in the Upper Ganga basin, India during 2000-2014. Sci. Rep. 9, 16730. doi:10.1038/s41598-019-53055-y
Barbato, G., Barini, E. M., Genta, G., and Levi, R. (2011). Features and performance of some outlier detection methods. J. Appl. Stat. 38, 2133–2149. doi:10.1080/02664763.2010.545119
Barrand, N. E., and Murray, T. (2006). Multivariate Controls on the Incidence of Glacier Surging in the Karakoram Himalaya. Arctic, Antarctic, Alpine Res. 38, 489–498. doi:10.1657/1523-0430(2006)38[489:mcotio]2.0.co;2
Bhambri, R., Bolch, T., and Chaujar, R. K. (2011). Mapping of Debris-Covered Glaciers in the Garhwal Himalayas Using ASTER DEMs and thermal Data. Int. J. Remote Sensing 32, 8095–8119. doi:10.1080/01431161.2010.532821
Bhattacharya, A., Bolch, T., Mukherjee, K., King, O., Menounos, B., Kapitsa, V., et al. (2021). High Mountain Asian Glacier Response to Climate Revealed by Multi-Temporal Satellite Observations since the 1960s. Nat. Commun. 12, 4133. doi:10.1038/s41467-021-24180-y
Bhattacharya, A., Bolch, T., Mukherjee, K., Pieczonka, T., Kropáček, J., and Buchroithner, M. F. (2016). Overall Recession and Mass Budget of Gangotri Glacier, Garhwal Himalayas, from 1965 to 2015 Using Remote Sensing Data. J. Glaciol. 62 (236), 1115–1133. doi:10.1017/jog.2016.96
Bocchiola, D., Brunetti, L., Soncini, A., Polinelli, F., and Gianinetto, M. (2019). Impact of Climate Change on Agricultural Productivity and Food Security in the Himalayas: A Case Study in Nepal. Agric. Syst. 171, 113–125. doi:10.1016/j.agsy.2019.01.008
Bolch, T., Kulkarni, A., Kääb, A., Huggel, C., Paul, F., Cogley, J. G., et al. (2012). The State and Fate of Himalayan Glaciers. Science 336, 310–314. doi:10.1126/science.1215828
Bolch, T., Menounos, B., and Wheate, R. (2010). Landsat-based Inventory of Glaciers in Western Canada, 1985-2005. Remote Sensing Environ. 114, 127–137. doi:10.1016/j.rse.2009.08.015
Bolch, T., Shea, J. M., Liu, S., Azam, F. M., Gao, Y., Gruber, S., et al. (2019). “Status and Change of the Cryosphere in the Extended Hindu Kush Himalaya Region,” in The Hindu Kush Himalaya Assessment (Springer International Publishing), 209–255. doi:10.1007/978-3-319-92288-1_7
Braithwaite, R. J. (2002). Glacier Mass Balance: The First 50 Years of International Monitoring. Prog. Phys. Geogr. Earth Environ. 26, 76–95. doi:10.1191/0309133302pp326ra
Brun, F., Berthier, E., Wagnon, P., Kääb, A., and Treichler, D. (2017). A Spatially Resolved Estimate of High Mountain Asia Glacier Mass Balances from 2000 to 2016. Nat. Geosci 10, 668–673. doi:10.1038/ngeo2999
Ceballos, J. L., Euscátegui, C., Ramírez, J., Cañon, M., Huggel, C., Haeberli, W., et al. (2006). Fast Shrinkage of Tropical Glaciers in Colombia. Ann. Glaciol. 43, 194–201. doi:10.3189/172756406781812429
Chand, M. B., and Watanabe, T. (2019). Development of Supraglacial Ponds in the Everest Region, Nepal, between 1989 and 2018. Remote Sensing 11, 1058. doi:10.3390/rs11091058
Chudley, T. R., Miles, E. S., and Willis, I. C. (2017). Glacier Characteristics and Retreat between 1991 and 2014 in the Ladakh Range, Jammu and Kashmir. Remote Sensing Lett. 8, 518–527. doi:10.1080/2150704X.2017.1295480
Cook, A. J., Copland, L., Noël, B. P. Y., Stokes, C. R., Bentley, M. J., Sharp, M. J., et al. (2019). Atmospheric Forcing of Rapid marine-terminating Glacier Retreat in the Canadian Arctic Archipelago. Sci. Adv. 5, eaau8507. doi:10.1126/sciadv.aau8507
Dar, R. A., Rashid, I., Romshoo, S. A., and Marazi, A. (2014). Sustainability of winter Tourism in a Changing Climate over Kashmir Himalaya. Environ. Monit. Assess. 186, 2549–2562. doi:10.1007/s10661-013-3559-7
Debnath, M., Sharma, M. C., and Syiemlieh, H. J. (2019). Glacier Dynamics in Changme Khangpu Basin, Sikkim Himalaya, India, between 1975 and 2016. Geosciences 9, 259. doi:10.3390/GEOSCIENCES9060259
Gaddam, V. K., Kulkarni, A. V., Bjornsson, H., Gullapalli, S., and Ballina, M. (2021). Applications of SPOT-7 Tri-stereo Imagery in Deriving the Surface Topography and Mass Changes of Glaciers in Indian Himalaya. Geocarto Int. 36 (13), 1512–1532. doi:10.1080/10106049.2019.1648567
Gardelle, J., Berthier, E., Arnaud, Y., and Kääb, A. (2013). Region-wide Glacier Mass Balances over the Pamir-Karakoram-Himalaya during 1999-2011. The Cryosphere 7 (4), 1263–1286. doi:10.5194/tc-7-1263-2013
Garg, P. K., Shukla, A., and Jasrotia, A. S. (2017). Influence of Topography on Glacier Changes in the central Himalaya, India. Glob. Planet. Change 155, 196–212. doi:10.1016/j.gloplacha.2017.07.007
Godwin-Austen, H. H. (1867). Notes on the Pangong lake District of Ladakh, from a Journal Made during a Survey in 1863. J. R. Geographical Soc. Lond. 37, 343–363. doi:10.2307/1798534
Govindha Raj, K. B. (2010). Remote Sensing Based hazard Assessment of Glacial Lakes: a Case Study in Zanskar basin, Jammu and Kashmir, India. Geomatics, Nat. Hazards Risk 1, 339–347. doi:10.1080/19475705.2010.532973
Graham Cogley, J. (2009). Geodetic and Direct Mass-Balance Measurements: Comparison and Joint Analysis. Ann. Glaciol. 50, 96–100. doi:10.3189/172756409787769744
Haeberli, W., and Hoelzle, M. (1995). Application of Inventory Data for Estimating Characteristics of and Regional Climate-Change Effects on Mountain Glaciers: a Pilot Study with the European Alps. Ann. Glaciol. 21, 206–212. doi:10.3189/s0260305500015834
Hall, D. K., Bayr, K. J., Schöner, W., Bindschadler, R. A., and Chien, J. Y. L. (2003). Consideration of the Errors Inherent in Mapping Historical Glacier Positions in Austria from the Ground and Space (1893-2001). Remote Sensing Environ. 86, 566–577. doi:10.1016/S0034-4257(03)00134-2
Huber, J., McNabb, R., and Zemp, M. (2020). Elevation Changes of West-central Greenland Glaciers from 1985 to 2012 from Remote Sensing. Front. Earth Sci. 8, 35. doi:10.3389/feart.2020.00035
Huggel, C., Carey, M., Emmer, A., Frey, H., Walker-Crawford, N., and Wallimann-Helmer, I. (2020). Anthropogenic Climate Change and Glacier lake Outburst Flood Risk: Local and Global Drivers and Responsibilities for the Case of lake Palcacocha, Peru. Nat. Hazards Earth Syst. Sci. 20, 2175–2193. doi:10.5194/nhess-20-2175-2020
Hussain, S., Song, X., Ren, G., Hussain, I., Han, D., and Zaman, M. H. (2017). Evaluation of Gridded Precipitation Data in the Hindu Kush-Karakoram-Himalaya Mountainous Area. Hydrological Sci. J. 62 (14), 2393–2405. doi:10.1080/02626667.2017.1384548
Immerzeel, W. W., Droogers, P., de Jong, S. M., and Bierkens, M. F. P. (2009). Large-scale Monitoring of Snow Cover and Runoff Simulation in Himalayan River Basins Using Remote Sensing. Remote Sensing Environ. 113, 40–49. doi:10.1016/j.rse.2008.08.010
Immerzeel, W. W., Van Beek, L. P. H., and Bierkens, M. F. P. (2010). Climate Change Will Affect the Asian Water Towers. Science 328, 1382–1385. doi:10.1126/science.1183188
Immerzeel, W. W., van Beek, L. P. H., Konz, M., Shrestha, A. B., and Bierkens, M. F. P. (2012). Hydrological Response to Climate Change in a Glacierized Catchment in the Himalayas. Climatic Change 110, 721–736. doi:10.1007/s10584-011-0143-4
Jensen, J. R. (2005). Introductory Digital Image Processing: A Remote Sensing Perspective. Saddle River, NJ: Prentice Hall.
Kääb, A., Berthier, E., Nuth, C., Gardelle, J., and Arnaud, Y. (2012). Contrasting Patterns of Early Twenty-First-century Glacier Mass Change in the Himalayas. Nature 488, 495–498. doi:10.1038/nature11324
Kanda, N., Negi, H. S., Rishi, M. S., and Kumar, A. (2020). Performance of Various Gridded Temperature and Precipitation Datasets over Northwest Himalayan Region. Environ. Res. Commun. 2 (8), 085002. doi:10.1088/2515-7620/ab9991
Kulkarni, A. V., and Karyakarte, Y. (2014). Observed Changes in Himalayan Glaciers. Curr. Sci. 106, 237–244.
Kumar, A., Srivastava, P., and Meena, N. K. (2017). Late Pleistocene Aeolian Activity in the Cold Desert of Ladakh: A Record from Sand Ramps. Quat. Int. 443, 13–28. doi:10.1016/J.QUAINT.2016.04.006
Linsbauer, A., Frey, H., Haeberli, W., Machguth, H., Azam, M. F., and Allen, S. (2016). Modelling Glacier-Bed Overdeepenings and Possible Future Lakes for the Glaciers in the Himalaya-Karakoram Region. Ann. Glaciol. 57, 119–130. doi:10.3189/2016AoG71A627
Linsbauer, A., Paul, F., and Haeberli, W. (2012). Modeling Glacier Thickness Distribution and Bed Topography over Entire Mountain Ranges with GlabTop: Application of a Fast and Robust Approach. J. Geophys. Res. 117, a–n. doi:10.1029/2011JF002313
Liu, J.-j., Tang, C., and Cheng, Z.-l. (2013). The Two Main Mechanisms of Glacier Lake Outburst Flood in Tibet, China. J. Mt. Sci. 10, 239–248. doi:10.1007/s11629-013-2517-8
Liu, Y., Wang, N., Zhang, J., and Wang, L. (2019). Climate Change and its Impacts on Mountain Glaciers during 1960-2017 in Western China. J. Arid Land 11, 537–550. doi:10.1007/s40333-019-0025-6
Lu, Y., Zhang, Z., and Huang, D. (2020). Glacier Mapping Based on Random Forest Algorithm: A Case Study over the Eastern Pamir. Water 12, 3231. doi:10.3390/w12113231
Magnin, F., Haeberli, W., Linsbauer, A., Deline, P., and Ravanel, L. (2020). Estimating Glacier-Bed Overdeepenings as Possible Sites of Future Lakes in the De-glaciating Mont Blanc Massif (Western European Alps). Geomorphology 350, 106913. doi:10.1016/j.geomorph.2019.106913
Majeed, U., Rashid, I., Sattar, A., Allen, S., Stoffel, M., Nüsser, M., et al. (2021). Recession of Gya Glacier and the 2014 Glacial lake Outburst Flood in the Trans-himalayan Region of Ladakh, India. Sci. Total Environ. 756, 144008. doi:10.1016/j.scitotenv.2020.144008
McNabb, R., Nuth, C., Kääb, A., and Girod, L. (2019). Sensitivity of Glacier Volume Change Estimation to DEM Void Interpolation. The Cryosphere 13, 895–910. doi:10.5194/tc-13-895-2019
Mehta, M., Kumar, V., Garg, S., and Shukla, A. (2021). Little Ice Age Glacier Extent and Temporal Changes in Annual Mass Balance (2016-2019) of Pensilungpa Glacier, Zanskar Himalaya. Reg. Environ. Change 21, 38. doi:10.1007/s10113-021-01766-2
Miller, J. D., Immerzeel, W. W., and Rees, G. (2012). Climate Change Impacts on Glacier Hydrology and River Discharge in the Hindu Kush-Himalayas. Mountain Res. Develop. 32, 461–467. doi:10.1659/mrd-journal-d-12-00027.1
Mir, R. A., Jain, S. K., Lohani, A. K., and Saraf, A. K. (2018). Glacier Recession and Glacial lake Outburst Flood Studies in Zanskar basin, Western Himalaya. J. Hydrol. 564, 376–396. doi:10.1016/j.jhydrol.2018.05.031
Misra, A. K. (2014). Climate Change and Challenges of Water and Food Security. Int. J. Sustain. Built Environ. 3, 153–165. doi:10.1016/j.ijsbe.2014.04.006
Muhammad, S., Tian, L., Ali, S., Latif, Y., Wazir, M. A., Goheer, M. A., et al. (2020). Thin Debris Layers Do Not Enhance Melting of the Karakoram Glaciers. Sci. Total Environ. 746, 141119. doi:10.1016/j.scitotenv.2020.141119
Muhammad, S., Tian, L., and Khan, A. (2019). Early Twenty-First century Glacier Mass Losses in the Indus Basin Constrained by Density Assumptions. J. Hydrol. 574, 467–475. doi:10.1016/j.jhydrol.2019.04.057
Nie, Y., Sheng, Y., Liu, Q., Liu, L., Liu, S., Zhang, Y., et al. (2017). A Regional-Scale Assessment of Himalayan Glacial lake Changes Using Satellite Observations from 1990 to 2015. Remote Sensing Environ. 189, 1–13. doi:10.1016/j.rse.2016.11.008
Nuth, C., and Kääb, A. (2011). Co-registration and Bias Corrections of Satellite Elevation Data Sets for Quantifying Glacier Thickness Change. The Cryosphere 5, 271–290. doi:10.5194/tc-5-271-2011
Pandit, A., and Ramsankaran, R. (2020). Identification of Potential Sites for Future Lake Formation and Expansion of Existing Lakes in Glaciers of Chandra Basin, Western Himalayas, India. Front. Earth Sci. 8, 382. doi:10.3389/feart.2020.500116
Patel, L. K., Sharma, P., Fathima, T. N., and Thamban, M. (2018). Geospatial Observations of Topographical Control over the Glacier Retreat, Miyar basin, Western Himalaya, India. Environ. Earth Sci. 77, 190. doi:10.1007/s12665-018-7379-5
Paul, F., Barrand, N. E., Baumann, S., Berthier, E., Bolch, T., Casey, K., et al. (2013). On the Accuracy of Glacier Outlines Derived from Remote-Sensing Data. Ann. Glaciol. 54, 171–182. doi:10.3189/2013AoG63A296
Paul, F., Huggel, C., and Kääb, A. (2004). Combining Satellite Multispectral Image Data and a Digital Elevation Model for Mapping Debris-Covered Glaciers. Remote Sensing Environ. 89, 510–518. doi:10.1016/j.rse.2003.11.007
Paul, F., Kääb, A., Maisch, M., Kellenberger, T., and Haeberli, W. (2002). The New Remote-Sensing-Derived Swiss Glacier Inventory: I. Methods. Ann. Glaciol. 34, 355–361. doi:10.3189/172756402781817941
Pieczonka, T., and Bolch, T. (2015). Region-wide Glacier Mass Budgets and Area Changes for the Central Tien Shan between ∼1975 and 1999 Using Hexagon KH-9 Imagery. Glob. Planet. Change 128, 1–13. doi:10.1016/j.gloplacha.2014.11.014
Pratibha, S., and Kulkarni, A. V. (2018). Decadal Change in Supraglacial Debris Cover in Baspa basin, Western Himalaya. Curr. Sci. 114, 792–799. doi:10.18520/cs/v114/i04/792-799
Ramsankaran, R., Pandit, A., and Azam, M. F. (2018). Spatially Distributed Ice-Thickness Modelling for Chhota Shigri Glacier in Western Himalayas, India. Int. J. Remote Sensing 39, 3320–3343. doi:10.1080/01431161.2018.1441563
Ramsankaran, R., Pandit, A., and Parla, A. (2019). “Decadal Estimates of Surface Mass Balance for Glaciers in Chandra basin, Western Himalayas, India-A Geodetic Approach,” in Climate Change Signals and Response (Singapore: Springer), 109–125. doi:10.1007/978-981-13-0280-0_7
Rashid, I., Majeed, U., Najar, N. A., and Bhat, I. A. (2021). Retreat of Machoi Glacier, Kashmir Himalaya between 1972 and 2019 Using Remote Sensing Methods and Field Observations. Sci. Total Environ. 785, 147376. doi:10.1016/j.scitotenv.2021.147376
Rashid, I., and Majeed, U. (2018). Recent Recession and Potential Future lake Formation on Drang Drung Glacier, Zanskar Himalaya, as Assessed with Earth Observation Data and Glacier Modelling. Environ. Earth Sci. 77, 429. doi:10.1007/s12665-018-7601-5
Rashid, I., and Majeed, U. (2020). Retreat and Geodetic Mass Changes of Zemu Glacier, Sikkim Himalaya, India, between 1931 and 2018. Reg. Environ. Change 20, 125. doi:10.1007/s10113-020-01717-3
Rashid, I., Romshoo, S. A., and Abdullah, T. (2017). The Recent Deglaciation of Kolahoi valley in Kashmir Himalaya, India in Response to the Changing Climate. J. Asian Earth Sci. 138, 38–50. doi:10.1016/j.jseaes.2017.02.002
Rastner, P., Bolch, T., Notarnicola, C., and Paul, F. (2014). A Comparison of Pixel- and Object-Based Glacier Classification with Optical Satellite Images. IEEE J. Sel. Top. Appl. Earth Observations Remote Sensing 7, 853–862. doi:10.1109/JSTARS.2013.2274668
Rathour, R., Gupta, J., Mishra, A., Rajeev, A. C., Dupont, C. L., and Thakur, I. S. (2020). A Comparative Metagenomic Study Reveals Microbial Diversity and Their Role in the Biogeochemical Cycling of Pangong lake. Sci. Total Environ. 731, 139074. doi:10.1016/j.scitotenv.2020.139074
Robson, B. A., Bolch, T., MacDonell, S., Hölbling, D., Rastner, P., and Schaffer, N. (2020). Automated Detection of Rock Glaciers Using Deep Learning and Object-Based Image Analysis. Remote Sensing Environ. 250, 112033. doi:10.1016/j.rse.2020.112033
Roe, G. H. (2011). What Do Glaciers Tell Us about Climate Variability and Climate Change. J. Glaciol. 57, 567–578. doi:10.3189/002214311796905640
Romshoo, S. A., Bashir, J., and Rashid, I. (2020). Twenty-first century-end Climate Scenario of Jammu and Kashmir Himalaya, India, Using Ensemble Climate Models. Climatic Change 162, 1473–1491. doi:10.1007/s10584-020-02787-2
Sahu, R., and Gupta, R. D. (2018). Conceptual Framework of Combined Pixel and Object-Based Method for Delineation of Debris-Covered Glaciers. ISPRS Ann. Photogramm. Remote Sens. Spat. Inf. Sci. IV-5, 173–180. doi:10.5194/isprs-annals-IV-5-173-2018
Salerno, F., Thakuri, S., Tartari, G., Nuimura, T., Sunako, S., Sakai, A., et al. (2017). Debris-covered Glacier Anomaly? Morphological Factors Controlling Changes in the Mass Balance, Surface Area, Terminus Position, and Snow Line Altitude of Himalayan Glaciers. Earth Planet. Sci. Lett. 471, 19–31. doi:10.1016/j.epsl.2017.04.039
Sattar, A., Haritashya, U. K., Kargel, J. S., Leonard, G. J., Shugar, D. H., and Chase, D. V. (2021). Modeling lake Outburst and Downstream hazard Assessment of the Lower Barun Glacial Lake, Nepal Himalaya. J. Hydrol. 598, 126208. doi:10.1016/j.jhydrol.2021.126208
Schmidt, S., Nüsser, M., Baghel, R., and Dame, J. (2020). Cryosphere Hazards in Ladakh: the 2014 Gya Glacial lake Outburst Flood and its Implications for Risk Assessment. Nat. Hazards 104, 2071–2095. doi:10.1007/s11069-020-04262-8
Schmidt, S., and Nüsser, M. (2017). Changes of High Altitude Glaciers in the Trans-himalaya of Ladakh over the Past Five Decades (1969-2016). Geosciences 7, 27. doi:10.3390/GEOSCIENCES7020027
Shukla, A., Arora, M. K., and Gupta, R. P. (2010). Synergistic Approach for Mapping Debris-Covered Glaciers Using Optical-thermal Remote Sensing Data with Inputs from Geomorphometric Parameters. Remote Sensing Environ. 114, 1378–1387. doi:10.1016/j.rse.2010.01.015
Shukla, A., and Qadir, J. (2016). Differential Response of Glaciers with Varying Debris Cover Extent: Evidence from Changing Glacier Parameters. Int. J. Remote Sensing 37, 2453–2479. doi:10.1080/01431161.2016.1176272
Singh, P., and Bengtsson, L. (2004). Hydrological Sensitivity of a Large Himalayan basin to Climate Change. Hydrol. Process. 18, 2363–2385. doi:10.1002/hyp.1468
Soheb, M., Ramanathan, A., Angchuk, T., Mandal, A., Kumar, N., and Lotus, S. (2020). Mass-balance Observation, Reconstruction and Sensitivity of Stok Glacier, Ladakh Region, India, between 1978 and 2019. J. Glaciol. 66, 627–642. doi:10.1017/jog.2020.34
Sravana Kumar, M., Shekhar, M. S., Rama Krishna, S. S. V. S., Bhutiyani, M. R., and Ganju, A. (2012). Numerical Simulation of Cloud Burst Event on August 05, 2010, over Leh Using WRF Mesoscale Model. Nat. Hazards 62, 1261–1271. doi:10.1007/S11069-012-0145-1
Srivastava, P., Kumar, A., Singh, R., Deepak, O., Kumar, A. M., Ray, Y., et al. (2020). Rapid lake Level Fall in Pangong Tso (lake) in Ladakh, NW Himalaya: a Response of Late Holocene Aridity. Curr. Sci. 119 (2), 219–231. doi:10.18520/cs/v119/i2/219-231
Sujakhu, N. M., Ranjitkar, S., He, J., Schmidt-Vogt, D., Su, Y., and Xu, J. (2019). Assessing the Livelihood Vulnerability of Rural Indigenous Households to Climate Changes in Central Nepal, Himalaya. Sustainability 11, 2977. doi:10.3390/su11102977
Vijay, S., and Braun, M. (2018). Early 21st century Spatially Detailed Elevation Changes of Jammu and Kashmir Glaciers (Karakoram-Himalaya). Glob. Planet. Change 165, 137–146. doi:10.1016/j.gloplacha.2018.03.014
Vuichard, D., and Zimmermann, M. (1987). The 1985 Catastrophic Drainage of a Moraine-Dammed lake, Khumbu Himal, Nepal: Cause and Consequences. Mountain Res. Develop. 7, 91–110. doi:10.2307/3673305
Wang, P., Li, Z., Li, H., Wang, W., Zhou, P., and Wang, L. (2017). Characteristics of a Partially Debris-Covered Glacier and its Response to Atmospheric Warming in Mt. Tomor, Tien Shan, China. Glob. Planet. Change 159, 11–24. doi:10.1016/j.gloplacha.2017.10.006
Wang, S., Du, J., Li, S., He, H., and Xu, W. (2019). Impact of Tourism Activities on Glacial Changes Based on the Tourism Heat Footprint (THF) Method. J. Clean. Prod. 215, 845–853. doi:10.1016/j.jclepro.2019.01.120
Wang, W., Xiang, Y., Gao, Y., Lu, A., and Yao, T. (2015). Rapid Expansion of Glacial Lakes Caused by Climate and Glacier Retreat in the Central Himalayas. Hydrol. Process. 29, 859–874. doi:10.1002/hyp.10199
Worni, R., Huggel, C., and Stoffel, M. (2013). Glacial Lakes in the Indian Himalayas - from an Area-wide Glacial lake Inventory to On-Site and Modeling Based Risk Assessment of Critical Glacial Lakes. Sci. Total Environ. 468-469, S71–S84. doi:10.1016/j.scitotenv.2012.11.043
Ye, Q., Kang, S., Chen, F., and Wang, J. (2006). Monitoring Glacier Variations on Geladandong Mountain, central Tibetan Plateau, from 1969 to 2002 Using Remote-Sensing and GIS Technologies. J. Glaciol. 52, 537–545. doi:10.3189/172756506781828359
Zhang, J., Jia, L., Menenti, M., and Hu, G. (2019). Glacier Facies Mapping Using a Machine-Learning Algorithm: The Parlung Zangbo Basin Case Study. Remote Sensing 11, 452. doi:10.3390/rs11040452
Zhao, X., Wang, X., Wei, J., Jiang, Z., Zhang, Y., and Liu, S. (2020). Spatiotemporal Variability of Glacier Changes and Their Controlling Factors in the Kanchenjunga Region, Himalaya Based on Multi-Source Remote Sensing Data from 1975 to 2015. Sci. Total Environ. 745, 140995. doi:10.1016/J.SCITOTENV.2020.140995
Keywords: glacier recession, geodetic mass changes, glacier inventories, remote sensing, pangong lake, ladakh himalaya
Citation: Majeed U, Rashid I, Najar NA and Gul N (2021) Spatiotemporal Dynamics and Geodetic Mass Changes of Glaciers With Varying Debris Cover in the Pangong Region of Trans-Himalayan Ladakh, India Between 1990 and 2019. Front. Earth Sci. 9:748107. doi: 10.3389/feart.2021.748107
Received: 28 July 2021; Accepted: 22 November 2021;
Published: 23 December 2021.
Edited by:
Aparna Shukla, Ministry of Earth Sciences, IndiaReviewed by:
Martin Truffer, University of Alaska Fairbanks, United StatesAtanu Bhattacharya, University of Zurich, Switzerland
Copyright © 2021 Majeed, Rashid, Najar and Gul. This is an open-access article distributed under the terms of the Creative Commons Attribution License (CC BY). The use, distribution or reproduction in other forums is permitted, provided the original author(s) and the copyright owner(s) are credited and that the original publication in this journal is cited, in accordance with accepted academic practice. No use, distribution or reproduction is permitted which does not comply with these terms.
*Correspondence: Irfan Rashid, irfangis@gmail.com, irfangis@kashmiruniversity.ac.in