- 1School of Design and China Institute for Urban Governance, Shanghai Jiao Tong University, Shanghai, China
- 2University of California, Santa Barbara, Department of Geography, Santa Barbara, CA, United States
Identifying key ecological nodes/corridors and priority restoration areas (KENPRA) is the key link for optimizing land use and ecological security patterns (ESPs). However, few studies have considered future land use/cover change (LUCC) and urban sprawl in identifying KENPRA for ESP maintenance. To optimize KENPRA, we took Quanjiao County, Anhui Province, China as a case study area, a typical unit for Chinese Land Spatial Planning and a suburb of Yangtze River Delta agglomeration challenging LUCC and ecological security pattern maintenance. A comprehensive framework for optimizing KENPRA has been established by integrating ESP and land use conflict (LUC) to adapt to land use change for corresponding urbanization processes. A CA-Markov model was used to predict future land use under different KENPRA-based scenarios in 2030. The results found that the total area of 4,357.2 ha priority restoration areas and 17 key ecological nodes was KENPRA, which were approximately 50% and concentrated in intensive LUC areas. The result of the simulation model showed that KENPRA-based scenarios integrating LUC indicated less urban expansion and better effectiveness for maintaining ESPs in 2030. The findings and proposed framework provide new and important information and implications for planners and policymakers to understand and improve land planning/policy; the results also can provide better understanding of the coupled human–nature system linking LUCC, ecosystem services, and land and restoration planning.
1 Introduction
Rapid urbanization, characterized by urban population agglomeration and the transformation of natural or semi-natural surface to artificial surface (Dong et al., 2020), has become one of the key drivers of global land use/cover changes (LUCC) and environmental problems (Haddad et al., 2015), especially in the suburbs of urban agglomeration zones (Ziv and Davidowitz, 2019). Increasing LUCC has destroyed ecological corridors and networks across suburbs globally. They are among the most serious factors in the increase of landscape fragmentation (Haddad et al., 2015), ecosystem service decline (Cai and Peng, 2021), wildlife habitat degradation (Haddad et al., 2015; Hong et al., 2017; Yang et al., 2019), and biodiversity loss (Perino et al., 2019). Therefore, it is crucial for planners and policymakers to develop a new method which optimizes key corridor/nodes and restoration areas in urban–suburban land and adapts to LUCC in response to urbanization processes (Yu, 1996; Hepcana et al., 2009; Ghosh and Chifos, 2017; Hong et al., 2017). It is important to better understand the coupled human–nature system of suburbs by linking with LUCC, ecosystem services, and land planning (An and López-Carr, 2012). LUCC, development, and planning/governance for ecological priority areas were each identified by Kramer et al. (2017) as priority CHANS questions. There is a need, however, to integrate these concepts as they do not exist in a vacuum, and only in their integration can we understand causation in coupled human–natural systems (Ferraro et al., 2019).
Over the past century, there have been efforts to conserve and restore core habitat patches and ecological corridors undergoing habitat deterioration and landscape fragmentation caused by LUCC (Ando et al., 1998; Forman, 1998; Cook, 2002). These basic spatial approaches originate from landscape ecology theories and paradigms, for example, patch–corridor–matrix paradigm (Forman, 1998) and source–sink theory (Schier and Needleman, 2009). Cook (2002) demonstrated that using patch content analysis, corridor content analysis, and network structure analysis for ecological network planning is important to improve the integrity, productivity, and biodiversity of ecological systems. Furthermore, Yu (1996)) raised the concept of security patterns (SPs) in landscape planning. Many scholars refined this concept and developed a widely recognized concept of ecological security patterns (ESPs) in the fields of landscape ecology, urban and landscape planning, and land management (Ma et al., 2004; Waldheim, 2006; Wang et al., 2019b; Peng et al., 2019). It defined ESP as the spatial pattern that comprises critical ecological components, patches, and corridors of the ecosystem. It emphasized guaranteeing certain ecological processes, protecting ecosystem structures and functions, and maintaining health and integrity of the ecosystem (Yu, 1996; Su et al., 2016; He et al., 2020). ESP has formed a general paradigm including identification of ecological source (ES) and extraction of ecological corridors (ECs) (Yu, 1996; Peng et al., 2018a). It has been applied in land planning and urban–suburban environmental policy for decision makers to identity key corridors and priority restoration areas (Dai et al., 2021; Fu et al., 2021) and implement ecological restoration (Wang et al., 2019a; Wang et al., 2020a; Ghosh et al., 2021).
For balancing environmental protection and economic development, ESP has become one of the important national environmental policies throughout China, with its high population pressure and rapid urbanization process (Peng et al., 2018a; He et al., 2019). In addition, optimization of key ecological nodes and priority restoration areas (KENPRA) is a key step that translates ESP planning into practice. Publication of “National Land Planning of China (2016–2030)” and “National Master Plan for Major Ecological Protection and Restoration Projects, China (2021–2035)” has set a goal of building a national ESP system across the country by 2035. Later, an increasing demand for ESP and KENPRA identification through “National Land Spatial Planning,” “Spatial Ecological Restoration Planning,” and restoration schemes from province to county scale (Office of the Ministry of Natural Resources, 2021) could lead to improved land planning processes and greater ecological restoration in a new era after the Grain-to-Green Program, China’s Natural Forest Conservation Program (NFCP) (Chen et al., 2009; Peng et al., 2020b). Moreover, the county scale is the basic administrative management unit for building fundamental ESP and implementing ecological restoration in key nodes and corridors in China. Understanding how to effectively identify ESP and priority restoration areas at the county scale is important for improving China’s land planning and policy design.
Many KENPRA identification methods have been used in previous studies (Peng et al., 2018b; Fu et al., 2021). For example, the minimum cumulative resistance (MCR) model (Peng et al., 2018a; Peng et al., 2019; Li et al., 2020) and circuit theory (Wang et al., 2020b; Fu et al., 2021) have been used to build ecological corridors (Su et al., 2016; Dong et al., 2020) and identify key ecological nodes for priority restoration (Fang et al., 2020; Fu et al., 2021). However, most of these approaches view KENPRA from a “critical for connectivity” lens (Gonzalez Ovando et al., 2016; Zhang X. et al., 2020; Martins silva and Guimaraes Vieira, 2020), often ignoring the dynamics of land use and landscape pattern change into KENPRA identification processes. Land use conflict (LUC), also named land use competitiveness, referring to the conflict of various land uses for scarce land resources (Brown and Raymond, 2014; Zou et al., 2021), is a favorably performing indicator to predict and indicate the potential future LUCC (Zou et al., 2019). LUC has been used to reflect the actual situation of land use in fast-growing areas, predict future LUCC (Jiang et al., 2020), and guide sustainable land use planning and management (Kim and Arnhold, 2018). Questionnaire interview and participatory mapping GIS (Brown and Raymond, 2014), mathematical statistical analysis method (Coccoli et al., 2018), and multicriteria evaluation systems have been used to measure LUC (Iojă et al., 2014; Jiang et al., 2020). Multicriteria evaluation systems constructed from suitability and driving forces have been recognized as a promising method of LUC potential identification (Mubareka and Ehrlich, 2010). Notwithstanding, integrating predictor LUCC factors into KENPRA for adapting to urbanization and LUCC has been called for (Waldheim, 2006; Zhang D. et al., 2020; Cheng et al., 2022); yet, studies on how to apply it into ESPs to improve KENPRA identification are still limited.
To fill the gap, we developed a framework for integrating ESP and LUC for optimizing KENPRA at a county scale study of Quanjiao County, Anhui Province, China. Quanjiao is located in the southern part of Chuzhou City, Anhui Province, and it is also a suburb of Chuzhou and a part of the Yangtze River Delta (YRD) region, one of the urban agglomerations with the highest urbanization level in China. Surrounded by these extensive urbanization cities, Quanjiao is faced with the pressure of being urbanized with implications for its maintaining an optimal ecological security pattern. The aims of this study are 1) to present a comprehensive framework to optimize KENPRA by integrating ESP and LUC; 2) to develop ESP by identifying ecological sources and extracting ecological corridors and to identify KENPRA by integrating ESP and LUC in Quanjiao; and 3) to assess the effects of future LUCC under two KENPRA-based scenarios and to provide implications for land planning and policy. This study could not only be helpful to facilitate land planning and restoration policy by better adapting to LUCC through the process of KENPRA identification but also could provide a unique perspective to understanding of the coupled human–nature system by linking LUCC, ecosystem services, and land planning.
2 Methods
2.1 Study Area and Data Sources
Quanjiao County (117°49′~118°25′N, 31°51′~32°15′E) is a suburban county of Chuzhou City, located in the southern part of Chuzhou, Anhui Province, China (Figure 1), with a total land area of 1,568 km2. Quanjiao is a typically hilly suburb county of eastern China, and it has all kinds of natural elements such as hill, forest, grassland, farmland, wetland, and river. The total population of Quanjiao County was 452,000 by the end of 2019, with the urbanization rate of 56.9% (SOQC, 2020). It is also a part of the Yangtze River Delta (YRD) region (Figure 1), which is one of the regions with the highest urbanization levels in China. Quanjiao County is 48 kilometers to Nanjing with an 83.2% urbanization rate, 98 kilometers to Hefei with a 76.3% urbanization rate, and 360 kilometers to Shanghai with an 88.1% urbanization rate. Surrounded by these extensive urban sprawls, Quanjiao is faced with the pressure of being intensively urbanized, with LUCC and ecological corridor loss.
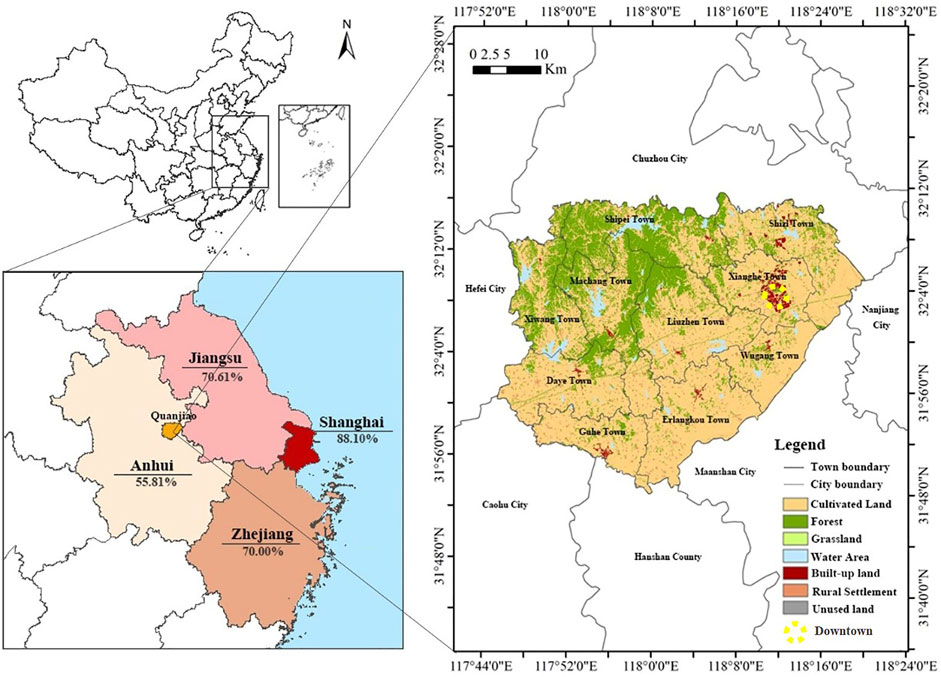
FIGURE 1. Location of the study area. (The numbers below province names refer to the urbanization rate of each province in 2019.)
The following data sources were used in this study: 1) seven land cover types, including cultivated land, forest, grassland, water area, built-up land, rural settlement, and unused land, extracted from 2010, 2015, and 2018 land use maps obtained from the National Geomatics Center of China (NGCC) with a 30-m resolution. The Third National Land Survey of the study area was used to calibrate the land use map in 2018; 2) precipitation and rainfall erosivity data were extracted from Chinese National Average Annual Rainfall and the Chinese National Rainfall Erosivity Map (Xun and Zhang, 2021), which were downloaded from the Resource and Environment Science and Data Center, China (https://www.resdc.cn/); 3) soil data were obtained from The Second National Soil Census Data, China. Soil characteristics, for example, soil texture and organic matter content were used to determine the standard content of sand, silt, and clay and calculate soil erosion factor by the EPIC model formula; 4) a 20-m resolution digital elevation model (DEM) was downloaded from the Geospatial Data Cloud site, Computer Network Information Center, Chinese Academy of Sciences (http://www.gscloud.cn), from which were extracted or computed elevation, slope, and slope length.
2.2 Framework for Optimizing Key Ecological Nodes/Corridors and Priority Restoration Areas
A comprehensive framework for optimizing KENPRA by integrating ESP and LUC was proposed (Figure 2), and four main technical steps were identified. Multisource data collection and data set building were conducted before the main methodological steps. The first step was to identify and establish ESPs with three sub-steps. The first sub-step was to identify an ecological source through an evaluation system, including habitat quality, ecosystem services, and landscape connectivity. The second sub-step was to construct a resistance surface by assigning resistance coefficients to different land use types and geomorphological factors. Ecological corridors were generated with circuit theory by using the results of ecological source and resistance surface. The third sub-step was to develop ecological security patterns by determining main ecological sources and ecological corridors. Moreover, a multicriteria evaluation system was built to evaluate the intensity of LUC from different land use functions, for example, ecological land conflict, cultivated land conflict, and construction land conflict. Each land use function was assessed based on suitability and driving forces of land use conversion. The first sub-step of assessing habitat quality, ecosystem services, and landscape connectivity laid the foundation for evaluating ecological land suitability for this sub-step. The third step was to optimize KENPRA from the three perspectives of ecological security: importance, criticality, and timeliness. Key ecological nodes (KENs) and priority restoration areas (PRAs) were identified through the assessment results of ecological pinch, ecological barrier, and LUC index.
2.3 Ecological Security Pattern Identification
2.3.1 Ecological Source Area Identification
An ecological source area is not only the habitat of native species but also the flow of ecological elements and the provision of products and ecosystem services (Asgarian et al., 2015; Peng et al., 2018b). Provisioning of regional ecosystem services and maintaining of ecological processes in the landscape have been considered in identifying ecological security source areas in recent studies (Peng et al., 2019; Zhang D. et al., 2020; Kang et al., 2021). Three of the most important provision ecosystem services in study areas, that is, carbon storage and sequestration, soil conservation, and water yield were selected as main ecosystem services (Supplementary Appendix A). The habitat quality model of the Integrated Valuation of Ecosystem Services and Tradeoffs (InVEST) tool was developed to calculate habitat quality (Zhang D. et al., 2020). Soil conservation and water yield were calculated by using the Revised Universal Soil Loss Equation (RUSLE) (Bamutaze et al., 2021) and the water balance equation (Jiang et al., 2021), respectively. Carbon storage and sequestration were assessed by using InVEST of the carbon storage and sequestration model. The probability of connectivity index evaluated the landscape connectivity with extracted core areas (Saura and Pascual-Hortal, 2007). Recently, an increasing number of studies have identified landscape connectivity as an important indicator of the landscape ecological process in identifying the ecological source (Peng et al., 2018a; Wang et al., 2020b). Thus, habitat quality, ecosystem services, and landscape connectivity were selected to identify the ecological source (Table 1; Supplementary Appendix A). The Delphi method was used to weight each indicator of habitat quality, ecosystem services, and landscape connectivity at 0.25, 0.50, and 0.25, respectively (Zhang D. et al., 2020). The results were standardized to four classes (extremely high, high, medium, and low) using the Jenks natural breaks classification method (Peng et al., 2018a; Zhang D. et al., 2020). Given the uneven distribution of natural conditions, we selected ecological resources by categorizing the study area into the plain area (7–15 m), hilly area (15–50 m), and low mountain area (50–395.4 m) according to landform types (Nhri, 2016). In low mountain regions and hilly areas with relatively high density of ecological patches, we selected the extremely high class as potential ecological resources, whereas in plain areas, both extremely high and high classes were considered potential ecological resources. Based on the contiguous correction, the Aggregate tool in ArcGIS 10.6 was used to aggregate dispersive potential ecological patches with different buffers in each area. Finally, the areas with not less than 1 km2 were delimitated as ecological source areas (Fang et al., 2020).
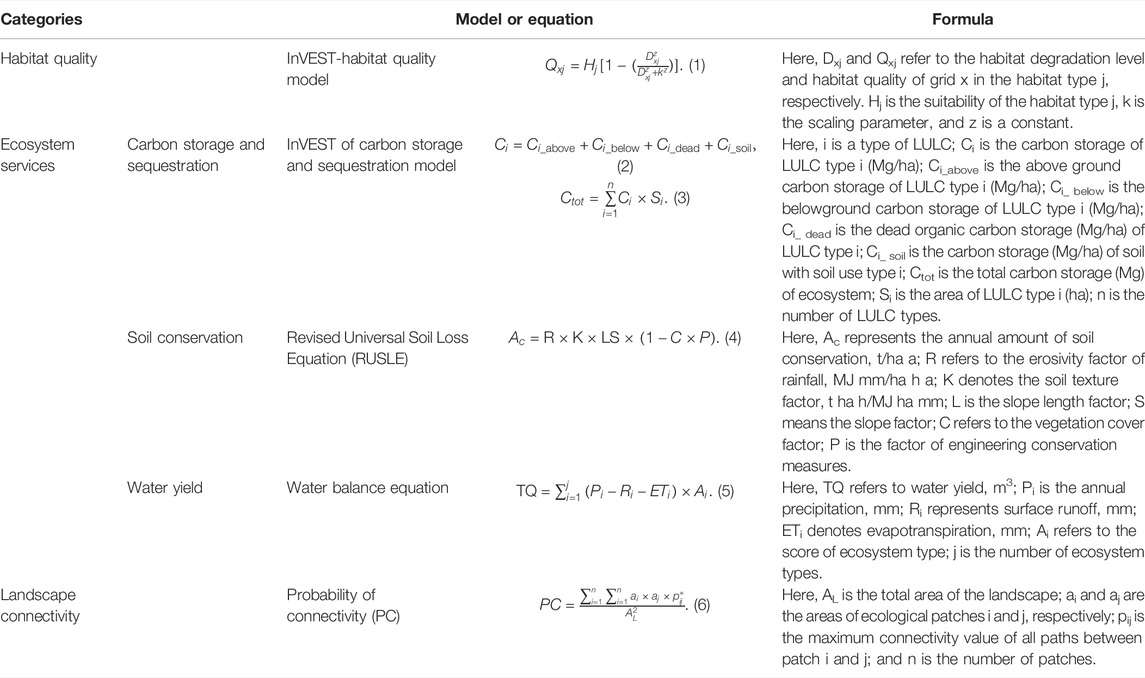
TABLE 1. Methods to assess habitat quality, ecosystem services, and landscape connectivity for ecological sources.
2.3.2 Ecological Corridor Identification
The ecological resistance surface was constructed for generating ecological corridors. Ecological functions and processes flow through the patch-corridor landscape structure. The resistance of their spatial flow is highly affected by landscape heterogeneity (Spear et al., 2010; Pickett et al., 2017). The resistance coefficients were assigned to different land use types and other geomorphological factors (Supplementary Appendix Table SB1) according to previous studies (Spear et al., 2010; Wang et al., 2020b). We used invisible resistance factors with Kriging interpolation to modify the ecological resistance surface. Slope and other topographic factors were extracted or computed from the digital elevation model (DEM) in ArcGIS 10.6 (ESRI, 2018). Finally, an ecological resistance surface was created by the minimum cumulative resistance (MCR) model, and the final results were normalized to 0–1. The circuit theory has been used to create and identify ecological corridors (Fu et al., 2021). It shares the similar principle of ecology such as the possibility of gene flow or species movement between ecological sources and nodes (Wang et al., 2020b). Based on the circuit theory, we identified the ecological corridors using Linkage Mapper software.
2.4 Land Use/Cover Change Assessment and Identification
Land use conflict arises from the scarcity of resources with obvious spatial attribution (Zou et al., 2019). A hierarchical multicriteria evaluation system (Jiang et al., 2020) was developed for land use conflict (Table 2) from land function suitability and potential driving forces (Liu et al., 2014; Iojă et al., 2014). Cultivated LUC of study areas is mainly influenced by natural factors (Liu et al., 2014; Chen et al., 2021), for example, slope, surface soil texture, and the potential driving forces of land use conversions and mainly include social and location factors (Table 3). Developed LUC was assessed by suitability factors, including natural, social, and economic factors, and driving forces of land use conversion (Supplementary Appendix Table SB2). Ecological land suitability was evaluated by the results of ecosystem service provision and habitat quality. The LUC index can be calculated using the following Eq. 7 (Jiang et al., 2020):
where Pi refers to the LUC of grid I; W1, W2, and W3 are the weights of indicators at different levels; and fij is the score of index j in grid i. We used the natural breaks method to divide the conflict into three scales: strong, medium, and weak. LUCs were divided into 27 categories (Jiang et al., 2020) and assigned corresponding to land use conflict intensities (Table 2). The LUC values ranged from 0 to 1, where 1 stands for the highest conflict and 0 indicates no conflict.
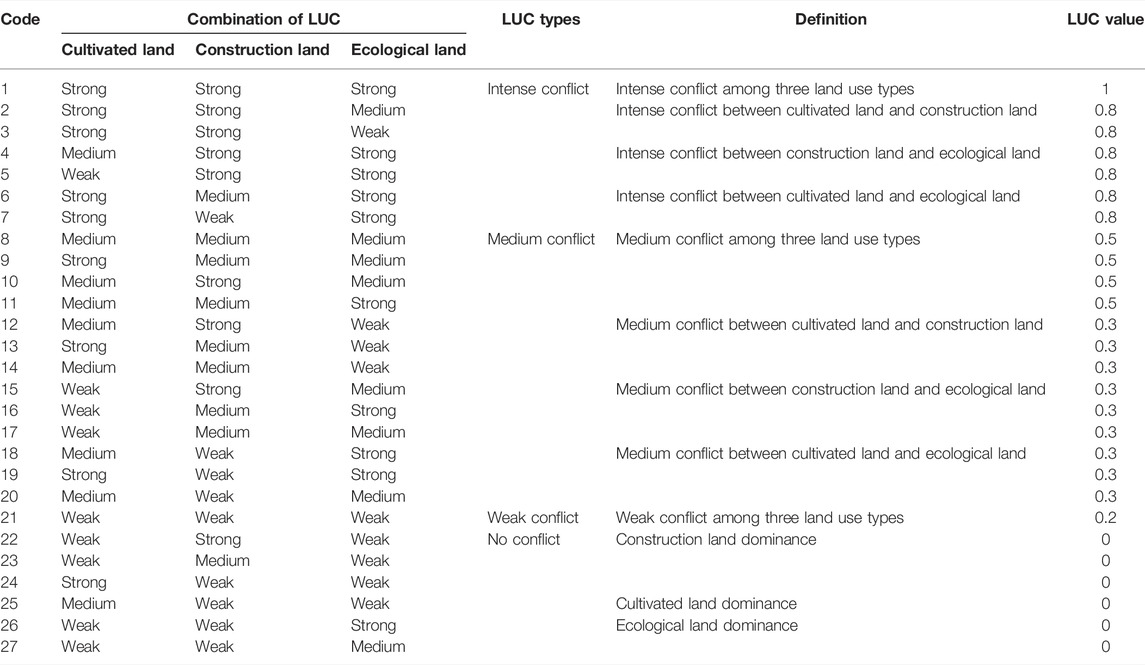
TABLE 2. Types of land conflict types and definition based on all combinations of three conflict ranks and three land use types.
2.5 Optimizing Key Ecological Nodes and Priority Restoration Areas
KENPRA identification is based on the importance, criticality, and time for ecological nodes and restoration areas to ESP (Fu et al., 2020; Wang et al., 2021). Ecological pinchpoint, ecological barrier, and LUC (as an index) were selected to indicate these three aspects (Zhang D. et al., 2020; Jiang et al., 2020). Currently, ecological pinchpoints are highly intensive areas, indicating that there are few or no alternative paths for species and other ecological processes, which are irreplaceable and important in landscape connectivity (Peng et al., 2018b). Ecological barriers, which have a high possibility of impeding species movement between patches of habitats, are critical areas for implementing restoration projects that could increase the likelihood of ecological corridor connectivity by restoring these nodes. If there are no timely protections or restoration projects involved, LUC areas will have a higher probability of having intense land use conflict and being urbanized.
Ecological pinchpoints and ecological barrier nodes and areas were identified by using the Pinchpoint Mapper Toolbox of ArcGIS and Circuitscape tool (http://www.circuitscape.org/linkagemapper). The ecological pinchpoints were generated using current intensity data (Peng et al., 2018b). Ecological barrier nodes were created by using the Barrier Mapper module of the Circuitscape tool, which found the value of important connectivity nodes by calculating the connectivity recovery value after the removal of barrier points (Mcrae et al., 2012). LUC node identification was based on the assessment of LUC. Each layer of ecological pinchpoint, ecological barrier, and LUC index was divided into four classes: 1) extremely high, 2) high, 3) medium, and 4) low. Priority restoration areas were extracted from each layer of extremely high areas and areas which were not less than 1 km2. Key ecological nodes were optimized and extracted from the top 10% of the high-value areas with not less than 1 km2 (Fang et al., 2020) and were transferred from polygon to point in ArcGIS 10.6.
2.6 Simulation of Future Land Use Scenario (CA-Markov Model)
To better understand the effects of KENPRA on future land use land cover (LULC) and conservation, a CA-Markov model was used to predict future LULC under different KENPRA-based scenarios. The CA-Markov model combines Cellular Automata and Markov Chain, which focuses on quantitatively assessing and predicting LULC (Ghosh and Chifos, 2017). In our study, a CA-Markov model was used to predict and assess the land use scenarios in 2030 based on the historical land use data. Two scenarios were developed based on ecological security source area identification under different schemes. In Scenario A, the protection of the ecological nodes and key areas of restoration, together with extremely high current density and LUC areas, should be restricted. In Scenario B, KENPRA identified and protected only extremely high current density areas.
The CA-Markov model predicted the LULC of future years based on the transition probability matrix from at least two past time periods. It was calibrated by using suitability maps with spatially explicit factors and constraint variables for each LULC type. We used the transition probability matrix created by LULC of 2015 and 2018 and suitability maps to predict the LULC in 2030 under Scenario A and Scenario B. LULC maps of 2015 and 2018 were prepared as raster layers with a cell size of 10 × 10 m. We converted LULC raster layers to rst form in IDRISI Selva, and the transition probability matrix was initially created by using a Markov model from LULC of 2015 and 2018 as the base years. A multicriteria evaluation module was applied to calibrate the model by creating suitability maps of all LULC types integrated with an initial transition probability matrix in CA-Markov to produce a future growth potential surface (Ghosh et al., 2021). Suitability maps were considered suitable factors and constraints for each LULC type. Constraint factors serve to limit the LULC under alternatives. Land policy and land condition slope, for example, permanent prime farmland have been considered to be constraints factors (Han et al., 2009; Ghosh and Chifos, 2017). All LULC and suitability layers were converted into 10 m*10 m grid cells according to their original resolution size. The spatial data preparation and raster analyses were implemented in ArcGIS v. 10.6 (ESRI, 2018), and the Markov and CA-Markov model were implemented in IDRISI Selva (Eastman, 2012).We used a transition probability matrix created in 2015 and 2018 LULC and suitability maps to predict the LULC until 2030 under Scenario A and Scenario B. The Kappa coefficient was used for verifying the prediction accuracy of the CA-Markov model (Pontius an d Millones, 2011), which was calculated by the CROSSTAB tool in IDRISI software. Models with a Kappa coefficient greater than 0.80 were considered achieving a high consistency (Feinstein and Cicchetti, 1990), which was found to be 0.861 and 0.836 under Scenario A and Scenario B, respectively, suggesting a good performance.
Where, T is the test pixels, C is the correctly classified pixels observations, G is the sum of multiplied total value.
3 Results
3.1 Ecological Security Pattern Identification and Construction
The results of habitat quality, ecosystem services, and landscape connectivity in 2018 showed spatial heterogeneity across the study area (Figure 3), while the extremely high areas of these three indictors were concentrated in the northwest comprising hilly areas. Specifically, areas with extremely high values of habitat quality and ecosystem services were mostly distributed in the northwest, accounting for 34.1 and 8.1% of the total area, respectively. The same as the result of landscape connectivity that extremely high and high areas were distributed among continuous forests in the northwest, which had a national forest park and a headwater reserve with high species movement connectivity capacity. Finally, 15 patches of ecological sources were selected in the study area (Figure 3), with a total area of 21,497.75 ha, which were mainly distributed in the northern hilly areas and accounted for 84.8% of the total area of ecological sources. To verify the identification result of ecological sources, we compared the selected ecological sources with the existing national and provincial nature reserves, the results of which showed good spatial consistency.
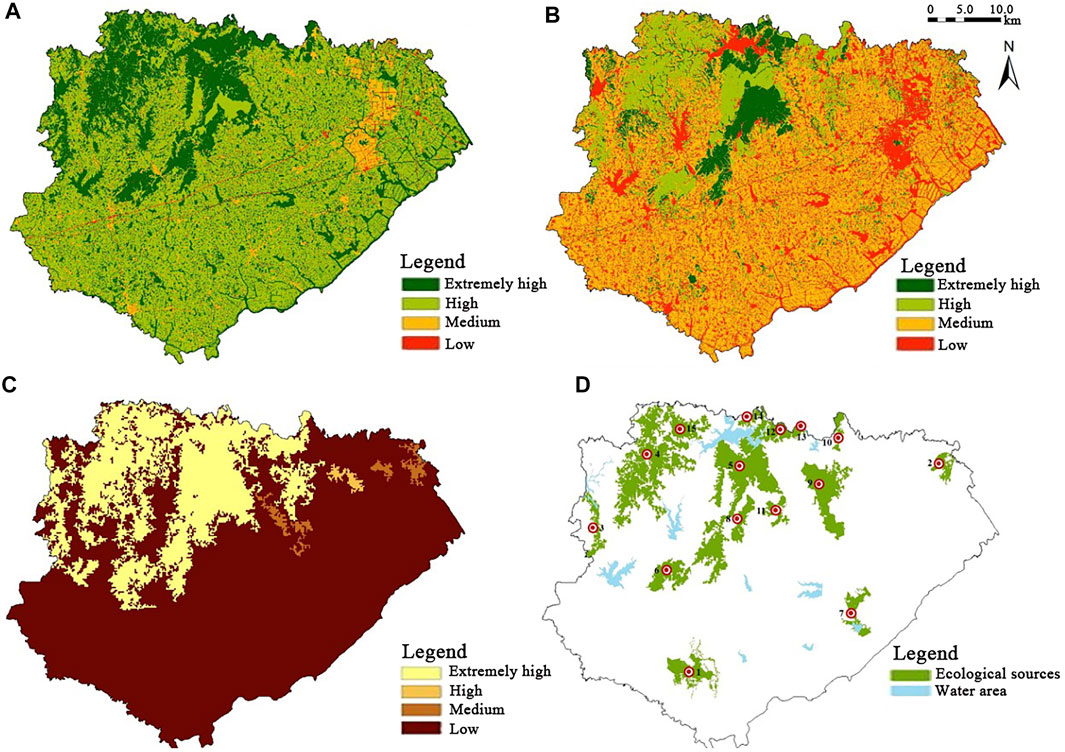
FIGURE 3. Ecological source areas in Quanjiao County. (a. Habitat quality; b. ecosystem services; c. landscape connectivity; d. ecological source.)
The ecological resistance surface modified by dominant resistance and recessive resistance is shown in Figure 4A. Overall, the built-up areas had the highest value of ecological resistance, and their value decreased gradually from downtown centers to other areas. The intensive cumulative current corridors mean high current density and value of connectivity as shown in Figure 4B. High and extremely high values of cumulative current constituted only 6.8 and 1.0% of the total length of the cumulative current surface, respectively, and were found only in the corridors of cultivated land in the southeast and corridors connecting cultivated land and forest in the middle of Quanjiao County. Finally, 31 ecological corridors were identified with a total length of 214.28 km (Figure 4C), with a maximum, minimum, and average length of 25.00, 0.73, and 8.93 km, respectively.
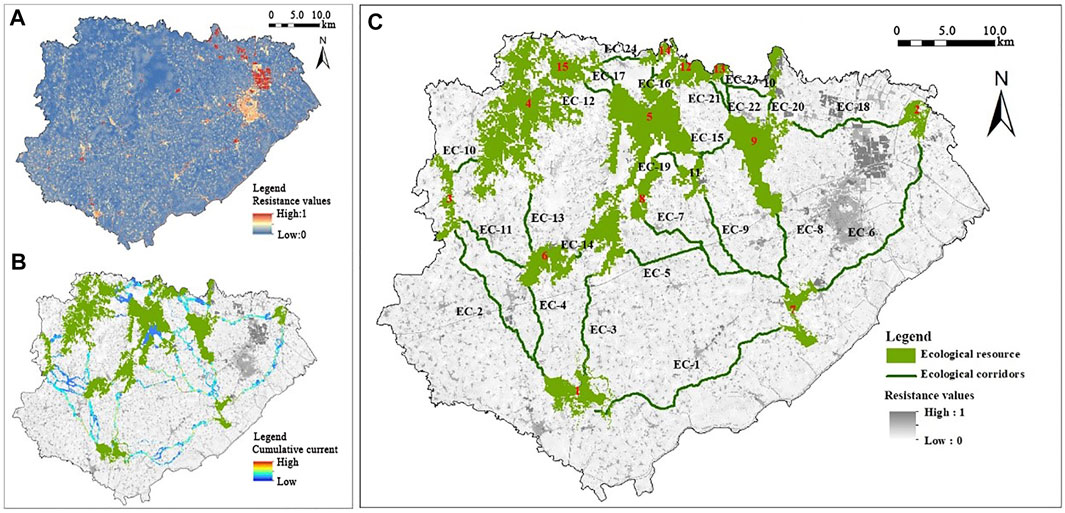
FIGURE 4. Ecological security patterns in Quanjiao County. (a. Ecological resistance surface; b. cumulative current; d. ecological corridors.)
3.2 Land Use Conflict Analysis
The assessment of LUC in 2018 showed that 82.56% of the study area had potential conflict, including extremely intense, intense, medium, and weak levels. Only 17.44% of the study area had no land use conflict (Figure 5A). Areas with extremely intense and intense LUC were mainly distributed in southeast areas (7,179.03 m2 and 40,825.09 ha, respectively), which were dominated by cultivated lands. Apparently, areas of no land use conflict were distributed in the northern hilly areas, which were inconsistent with the spatial patterns of high habitat quality and multiecosystem services provision, indicating that these areas are highly suitable and critical for ecological protection.
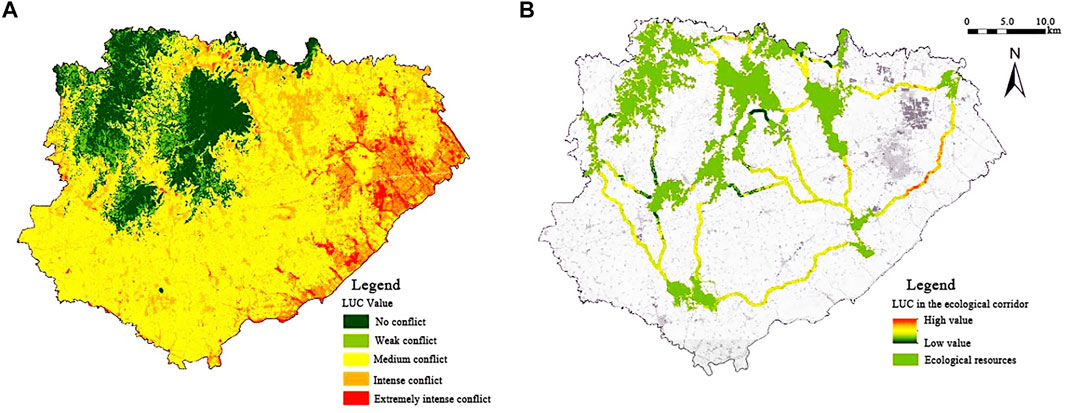
FIGURE 5. LUC in Quanjiao County and ecological corridors. (a. LUC in overall area of Quanjiao County; b. LUC in ecological corridors of Quanjiao County.)
LUC in ecological corridors is shown in Figure 5B. Areas with high values of LUC were mainly distributed in the southeast of Quanjiao County, which were close to the downtown and accounted for 5.1% of the total length of ecological corridors. These areas were mainly in ecological corridors EC-6, EC-8, and EC-18. Of special note, corridor EC-6 achieved approximately 50% of the total extremely intense areas of corridors.
3.3 Key Ecological Nodes and Priority Restoration Areas Optimization
A total of 34 key ecological nodes were found (Figure 6), including 17 key LUC nodes, 11 pinchpoints, and six barrier nodes. Key ecological nodes of LUC points were distributed mainly in the southeast and surrounded by downtown across intense LUC cultivated land. Surprisingly, we also found three nodes in the northern forest, adjacent to the north boundary, which may be due to the conflict between conservation and rural building development. By contrast, key ecological nodes of pinchpoints and barriers were mainly distributed in the north and middle of Quanjiao County, which played important roles in connecting ecological sources between cultivated land and forest.
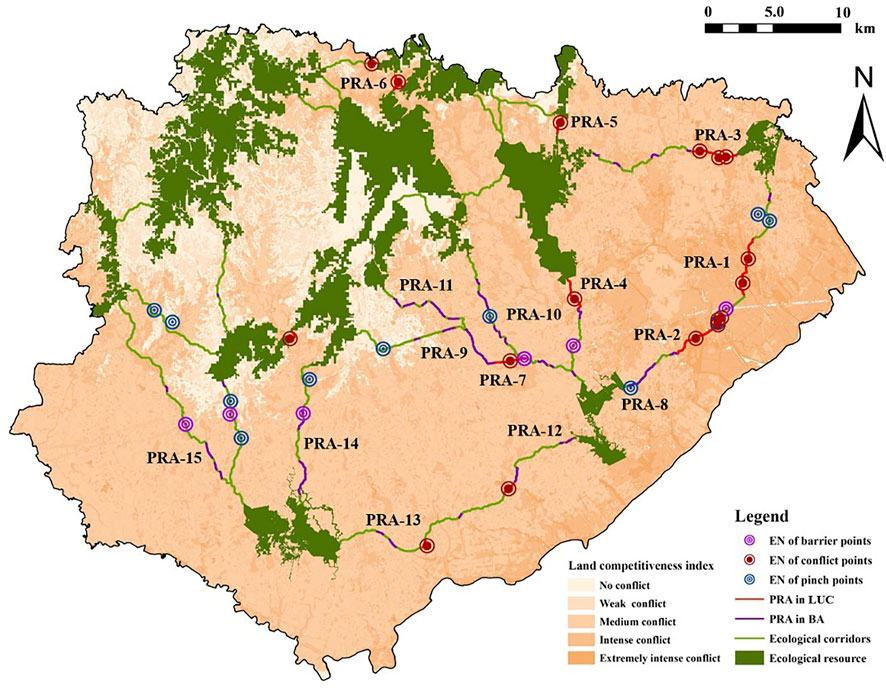
FIGURE 6. Key ecological nodes and priority restoration areas in Quanjiao County. (KEN: Key ecological nodes; PRA: priority restoration areas)
In addition, a total area of 4,357.2 ha were identified as priority corridors (Figure 6), including 2,485.2 ha of intensive land conflict corridors and 1,872 ha of high probability of barrier corridors, comprising 28.2 and 21.3% of the total corridor areas, respectively. Dense priority restoration areas were located in southeast Quanjiao county, resulting in potentially intensive land use conflicts. Several priority restoration areas in the middle of Quanjiao were found due to its importance of connectivity for species migration and ecological process flow between southern cultivated land and northern hilly areas. The strategies for land management for each priority restoration area were proposed (Supplementary Appendix Table SB3).
3.4 Changes of Land Use/Cover Change in Different Key Ecological Nodes/Corridors and Priority Restoration Areas–Based Simulation Scenarios
The change in LULC showed that the built-up areas increased slightly from 0.6% in 2010 to 0.7% in 2015 and increased rapidly to 2.36% in 2018 (Supplementary Appendix Table SB4). In contrast, the overall vegetation covers of the study area decreased gradually by 2.6% from 2010 to 2018, indicating that the built-up expansion was at the cost of cultivated land, forest, and grassland. The changes in LULC showed a similar trend, with different changes in different LULC type under these two KENPRA-based simulation scenarios from 2018 to 2030 (Figure 7; Supplementary Appendix Table SB4). Specifically, built-up areas are projected to have a larger expansion under Scenario B (310.23 ha) than Scenario A (857.53 ha) during the period of 2018–2030. Moreover, 1.46 and 2.48 ha of ecological corridors are likely to be occupied by built-up land, while −6.19 and 17.7 ha of ecological corridors will expand to rural settlement under Scenario A and Scenario B, respectively. The area of forest showed a significant increase, accounting for 24.1%% and 22.31%% of land. In contrast, the area of cultivated land decreased to 63.81 and 64.46% under Scenario A and Scenario B, respectively. Moreover, the overall growth of forest, grassland, and water areas in ecological corridors will have a greater growth under Scenario A (3,479.05 ha) than under Scenario B (3,259.76 ha) during the 2018–2030 period. In brief, the simulation result revealed that ecological security patterns will achieve better conservation efficiency with less ESP destruction under Scenario A than Scenario B in the period between 2018 and 2030.
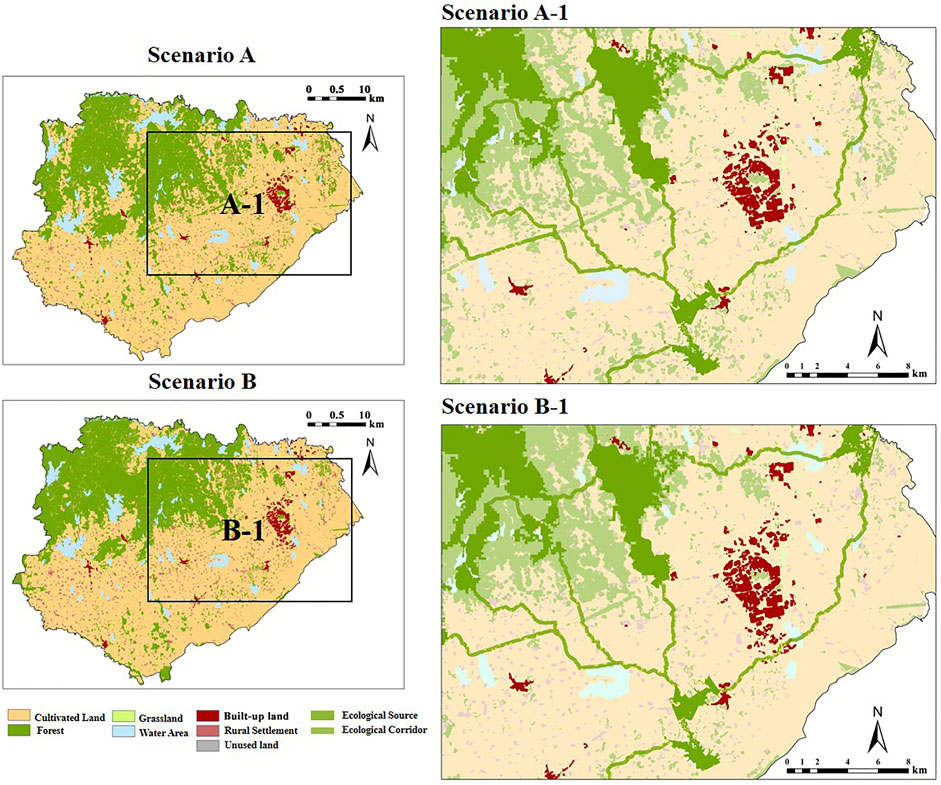
FIGURE 7. Simulated LULC change from 2018 to 2030 under Scenario A and Scenario B in Quanjiao County.
4 Discussion
This study demonstrates that the framework of integrating ESP and LUC is an effective approach to optimize KENPRA for improving land planning and management. This approach combines habitat quality, multiple ecosystem services, and landscape connectivity into ecological source identification. It integrates LUC into KENPRA optimization which adapts to rapid urbanization and LUCC for KENPRA optimization. In this case study, the simulated future land use by the CA-Markov model confirmed the hypothesis that KENPRA incorporating LUC could decrease corridor destruction and landscape fragmentation under land expansion pressure and better protect core wildlife habitat, conserve ecological corridors, and maintain ecological security.
4.1 Ecological Security Pattern Identification
Our study demonstrated a way to develop ESPs through ecological sources and ecological corridors to facilitate KENPRA identification at Quanjiao County. The results suggested that ESP linking between ecological processes and landscape patterns could help us better understand ecological processes of movement and fluxes and improve ecological integrity by conserving and restoring core patches and ecological corridors, especially in suburban areas where there is great pressure of rapid LUCC and land expansion (Cook, 2002; Peng et al., 2018b)
We identified ecological sources from multiple aspects, including habitat quality, ecosystem services, and landscape connectivity, the results of which all showed good spatial consistency with existing high ecological value areas, for example, nature reserves. The result is consistent with Fu’s study (Fu et al., 2020), which found that assessing habitat maintenance, ecosystem service importance, and landscape connectivity showed a good performance in delimiting ecological patches in Loess Plateau, China. Compared with previous paradigms that addressed ecological source identification on representative species or target group habitat (Hepcana et al., 2009; Luque et al., 2012), our approach embraced multiple important ecological processes by assessing critical ecosystem services and internal landscape connectivity. Ecosystem services, which were translated from the structure and function of ecosystems into the provision of important services (Daily et al., 2009), could better predict critical ecosystem process and function by quantitatively assessing certain ecosystem services (Costanza and Folke, 1997; Daily, 1997). For example, assessment of water yield service tells us the capacity of water provision and flux process (Cai et al., 2017), which is important for wildlife, for example, fish and amphibian, ecosystems, and human well-being. The results also could be explained from source–sink theory. “Sources” are known as patches that support local species population growth and contribute positively to the ecological process (Chen et al., 2008). These critical ecosystem service areas play an importance role as “sources” to maintain and facilitate the ecological process. In addition, integrating internal landscape connectivity into ecological source identification to strengthen the importance of patches could be better understood from this theory on how patch connectivity promotes species migration across heterogenous landscapes (Hansen, 2011).
In our study, the principle of MCR was used in building an ecological resistance surface, whereas circuit theory was used to generate ecological corridors to overcome the shortcomings of the MCR model which often fails to clarify species spread randomly and identify a specific range of corridors (Peng et al., 2018b; Dai et al., 2021). It can be understood from circuit theory that electric currents share the random walk property, which is similar to the ecological flow of movements of animals and ecological process flux landscape patches (Mcrae et al., 2012). Ecological corridors could be generated in heterogenous landscapes by calculating the “resistance” or “current.” A similar result was found in Peng’s (Peng et al., 2018b) study, in which circuit theory was used to successfully identify ecological corridors and key ecological nodes in Yunnan Province. Our study proved that this method could also be applied effectively at the county scale.
4.2 A Comprehensive Framework to Optimized Key Ecological Nodes/Corridors and Priority Restoration Areas and Implications
KENPRA optimization is a critical step that moves land planning into restoration practice, which provides the scientific-based evidence for decision makers to implement restoration projects and environmental policy. Our study presented a comprehensive framework of KENPRA optimization by integrating ESP and LUC to adapt to rapid urbanization and future LUCC. This study showed that LUC was a promising indicator for quantitative assessment of potential LUCC. Incorporating LUC to KENPRA optimization could improve the identification of priority restoration areas. Jiang’s study (Jiang et al., 2020) demonstrated that areas with intense conflict had a high possibility of LUCC and tended to be converted into the type of higher competitiveness. Similarly, we found in our study that cultivated land areas, which were high intensive LUC areas, had high potential to be converted into built-up areas. This finding is consistent with the perspective of geography (Coccoli et al., 2018; Zou et al., 2019). LUC is a geographical phenomenon which originated from the scarcity of spatial land resources and the spillover of spatial functions. It is also an antagonistic phenomenon in the process of spatial resource allocation in the process of human–land relationships and conflict over spatial resources (Zou et al., 2020).
The results of the CA-Markov model indicated that by integrating LUC, KENPRA-based scenarios showed better response to urban expansion in 2030, when there will be less ecological corridor destruction and better conservation efficiency for ESP. Different from Zhang’s study in the Yangtze River Delta region, China (Zhang D. et al., 2020), in which they incorporated land use/cover predictions into ecological security source areas, our study integrated potential land use/cover change by integrating LUC into the overall ESP process to improve KENPRA identification. By doing so, it is more effective for decision makers to build ESP construction and KENPRA identification in urban or suburban areas where they have high pressure of rapid urbanization. Another advantage of integrating LUC is that the framework can be improved by taking natural conditions, socioeconomic activities, and environmental policy into account (Iojă et al., 2014). Compared with traditional ways of ESP construction which focused on natural conditions (Yu, 1996), this framework could be more resilient to future development change. Simultaneously, the forward-looking KENPRA identification will be helpful for managers in developing land planning agendas and in implementing restoration projects.
4.3 Implications to Land Planning and Policy
Our study explored how to bridge the gap between practical needs and research limitations by proposing a new approach of integrating ESP and LUC into KENPRA optimization, which will facilitate the ongoing and forthcoming China’s Ecosystem Restoration Projects at the basic administration unit of the county. It also could be applied at other regions and countries across different scales. In addition, our proposed framework of incorporating ESP and LUC, which combines essentially land spatial planning of land function suitability assessment and landscape pattern analysis, could be more approachable and efficient when applied in Chinese National Land Spatial Planning and Spatial Ecological Restoration Planning. It also could improve the understanding of the coupled human–nature system of suburbs by improving linkages among LUCC, ecosystem services, and land planning.
It is worth noting that the stimulation result indicates a significant increase of forest with cultivated land decline in the period of 2018–2030 under ENKAR-based scenarios. The stimulated LULC change could be explained by LULC constrain factor of ecological redline policy, which is carried out by Chinese government to strictly protect natural areas with high value of ecosystem services and ecological sensitive areas. The farmland in mountain regions could be used for afforestation with ecological redline policy and restoration projects. Therefore, undergoing and upcoming environmental project and land policy should fully consider in KENPRA for responding future LULC. In addition, based on our results from KENPRA identification and a field site visit, site-specific strategies for land management in the study area were proposed. Building and maintaining ecological corridors were suggested to be incorporated into urban planning. Developed land expansion was limited in PRA-1 to PRA-3 by delineating development control lines to limit built-up land expansion. Strict conservation policies, including the ecological redline policy, and prohibited activities of deforestation and mining were encouraged in core patches and ecological corridors in PRA-4 to PRA-6. Essential ecological restoration was suggested, including reclamation in PRA-5, wetland restoration in PRA-2, and building ecological corridor bridges in PRA-14 and PRA-15 to help wildlife migration when ecological corridors are interrupted by artificial roads. These refined strategies could better protect the ecological security pattern and balance ecosystem protection and economic development.
Our result is consistent with that of previous studies that suggests that considering land use transition in spatial planning is conducive to facilitating suburban spatial governance (Ge et al., 2020) and achieve SDGs (Vörösmarty et al., 2018). ESP was originally proposed in the urban context to facilitate landscape design and urban planning for conserving habitat and safeguarding important ecological networks (Yu, 1996; Waldheim, 2006). Though approximately 15.2% of the world’s terrestrial area has been established as protected areas to protect global wildlife habitats and ecological networks (Yang et al., 2019; Peng et al., 2020a), urbanization is a global trend with momentum. Therefore, our process to guide ESP establishment and restoration implementation in urban and suburban areas could effectively conserve and restore terrestrial and inland freshwater ecosystems and their services, which contribute to sustainable land use and the achievement of SDGs in semi-natural and artificial areas.
Our study was, however, limited to one single context studying a Chinese county. Future work could usefully apply this framework to other regions at different scales. With the increasing demand for “Landscape Planning for Ecological Restoration” and with ever more restoration programs being implemented, more such studies are needed. The proposed approach could be used to improve land planning and management by better adapting to rapid LUCC and could also be helpful in reducing landscape fragmentation and conserve wildlife habitat and biodiversity in semi-natural or artificial contexts (Peng et al., 2018a; Zhang D. et al., 2020). Furthermore, the spatial composition of ecological corridors need to be explored in the future studies; ecological corridors with different widths, for example, may have different influences on ESP maintenance (van Schalkwyk et al., 2020), which should be considered in optimizing KENPRA. Last, our research is limited by data availability. Future research would benefit from further spatiotemporal data enhancement of the variables analyzed here and the incorporation of variables absent in this study.
5 Conclusion
Our study demonstrated that incorporating ESP and LUC into KENPRA identification can be an effective approach to respond to future LUCC and land expansion. More importantly, the proposed framework could be helpful for planners and decision makers to identify priority restoration areas and implement restoration projects in other urban and suburban areas, especially in areas facing intensive LUCC pressure. More importantly, our study provided an innovative perspective to understand the coupled human–nature system by linking LUCC, ecosystem services, and land planning. The results showed that 15 patches with a total of 21,497.75 ha were identified ecological sources, accounting for 13.7% of the total area of Quanjiao, and they were mainly distributed in the north of hilly areas. In addition, 31 ecological corridors were found with a total length of 220.04 km, distributed evenly across the county. Finally, 17 key ecological nodes and a total area of 4,357.2 ha priority restoration areas were identified, constituting approximately half of KENPRA distributing in intensive LUC areas. The results of the simulation model showed that KENPRA-based scenarios integrating LUC indicated less urban expansion and better conservation effectiveness for ESP in future scenarios. Future work will further explore how to apply this framework to other regions at different scales and how to integrate this proposed process of KENPRA into “Landscape Planning for Ecological Restoration” and other land planning. This study not only suggests improvements in the process of KENPRA identification and the proposed framework but also has implications for better understanding of the coupled human–nature system linking LUCC, ecosystem services, and environmental and restoration planning.
Data Availability Statement
The original contributions presented in the study are included in the article/Supplementary Material, further inquiries can be directed to the corresponding author.
Author Contributions
WP: conceptualization; data curation; methodology; software; validation; visualization; and writing—original draft, review, and editing. ZZ: formal analysis; investigation; methodology; software; and visualization. GH: investigation; formal analysis; and methodology. XL: data curation; formal analysis; and methodology. WW: writing—review and editing. YC: data curation; project administration; resources; supervision; and writing—review and editing. DL-C: supervision and writing—review and editing.
Funding
This work was supported by the Key Philosophy and Social Science Foundation of Ministry of Education of China (Grant number 19JZD023), Shanghai Sailing Program (Grant number 21YF1419600), and Faculty Initiation Programs of Shanghai Jiao Tong University (Grant number 2121X010500744).
Conflict of Interest
The authors declare that the research was conducted in the absence of any commercial or financial relationships that could be construed as a potential conflict of interest.
Publisher’s Note
All claims expressed in this article are solely those of the authors and do not necessarily represent those of their affiliated organizations, or those of the publisher, the editors, and the reviewers. Any product that may be evaluated in this article, or claim that may be made by its manufacturer, is not guaranteed or endorsed by the publisher.
Supplementary Material
The Supplementary Material for this article can be found online at: https://www.frontiersin.org/articles/10.3389/feart.2022.875433/full#supplementary-material
References
Abdelgalil, E. A., and Cohen, S. I. (2007). Economic Development and Resource Degradation: Conflicts and Policies. Socio-Economic Plann. Sci. 41, 107–129. doi:10.1016/j.seps.2005.10.002
An, L., and López-Carr, D. (2012). Understanding Human Decisions in Coupled Natural and Human Systems. Ecol. Model. 229, 1–4. doi:10.1016/j.ecolmodel.2011.10.023
Ando, A., Camm, J., Polasky, S., and Solow, A. (1998). Species Distributions, Land Values, and Efficient Conservation. Science 279, 2126–2128. doi:10.1126/science.279.5359.2126
Asgarian, A., Amiri, B. J., and Sakieh, Y. (2015). Assessing the Effect of green Cover Spatial Patterns on Urban Land Surface Temperature Using Landscape Metrics Approach. Urban Ecosyst. 18, 209–222. doi:10.1007/s11252-014-0387-7
Bamutaze, Y., Mukwaya, P., Oyama, S., Nadhomi, D., and Nsemire, P. (2021). Intersecting RUSLE Modelled and Farmers Perceived Soil Erosion Risk in the Conservation Domain on Mountain Elgon in Uganda. Appl. Geogr. 126, 102366. doi:10.1016/j.apgeog.2020.102366
Brown, G., and Raymond, C. M. (2014). Methods for Identifying Land Use Conflict Potential Using Participatory Mapping. Landscape Urban Plann. 122, 196–208. doi:10.1016/j.landurbplan.2013.11.007
Cai, W., Gibbs, D., Zhang, L., Ferrier, G., and Cai, Y. (2017). Identifying Hotspots and Management of Critical Ecosystem Services in Rapidly Urbanizing Yangtze River Delta Region, China. J. Environ. Manage. 191, 258–267. doi:10.1016/j.jenvman.2017.01.003
Cai, W., and Peng, W. (2021). Exploring Spatiotemporal Variation of Carbon Storage Driven by Land Use Policy in the Yangtze River Delta Region. Land 10, 1120. doi:10.3390/land10111120
Chen, L., Fu, B., and Zhao, W. (2008). Source-sink Landscape Theory and its Ecological Significance. Front. Biol. China 3, 131–136. doi:10.1007/s11515-008-0026-x
Chen, L., Zhao, H., Song, G., and Liu, Y. (2021). Optimization of Cultivated Land Pattern for Achieving Cultivated Land System Security: A Case Study in Heilongjiang Province, China. Land Use Policy 108, 105589. doi:10.1016/j.landusepol.2021.105589
Chen, X., Lupi, F., He, G., Ouyang, Z., and Liu, J. (2009). Factors Affecting Land Reconversion Plans Following a Payment for Ecosystem Service Program. Biol. Conservation 142, 1740–1747. doi:10.1016/j.biocon.2009.03.012
Cheng, H., Zhu, L., and Meng, J. (2022). Fuzzy Evaluation of the Ecological Security of Land Resources in mainland China Based on the Pressure-State-Response Framework. Sci. Total Environ. 804, 150053. doi:10.1016/j.scitotenv.2021.150053
Coccoli, C., Galparsoro, I., Murillas, A., Pınarbaşı, K., and Fernandes, J. A. (2018). Conflict Analysis and Reallocation Opportunities in the Framework of marine Spatial Planning: A Novel, Spatially Explicit Bayesian Belief Network Approach for Artisanal Fishing and Aquaculture. Mar. Pol. 94, 119–131. doi:10.1016/j.marpol.2018.04.015
Cook, E. A. (2002). Landscape Structure Indices for Assessing Urban Ecological Networks. Landscape Urban Plann. 58, 269–280. doi:10.1016/s0169-2046(01)00226-2
Costanza, R., and Folke, C. (1997). “Valuing Ecosystem Services with Efficiency, Fairness, and Sustainability as Goals,” in Nature's Services: Societal Dependence on Natural Ecosystems (Washington, DC: Island Press).
Dai, L., Liu, Y., and Luo, X. (2021). Integrating the MCR and DOI Models to Construct an Ecological Security Network for the Urban Agglomeration Around Poyang Lake, China. Sci. Total Environ. 754, 141868. doi:10.1016/j.scitotenv.2020.141868
Daily, G. C. (1997). Nature's Services: Societal Dependence on Natural Ecosystems. Yale, USA: Island PressYale University Press.
Daily, G. C., Polasky, S., Goldstein, J., Kareiva, P. M., Mooney, H. A., Pejchar, L., et al. (2009). Ecosystem Services in Decision Making: Time to Deliver. Front. Ecol. Environ. 7, 21–28. doi:10.1890/080025
De Groot, R. S., Alkemade, R., Braat, L., Hein, L., and Willemen, L. (2010). Challenges in Integrating the Concept of Ecosystem Services and Values in Landscape Planning, Management and Decision Making. Ecol. Complexity 7, 260–272. doi:10.1016/j.ecocom.2009.10.006
Dong, J., Peng, J., Liu, Y., Qiu, S., and Han, Y. (2020). Integrating Spatial Continuous Wavelet Transform and Kernel Density Estimation to Identify Ecological Corridors in Megacities. Landscape Urban Plann., 103815. doi:10.1016/j.landurbplan.2020.103815
Eastman, J. R. (2012). IDRISI Selva Tutorial. IDRISI Production. Worcester, USA: Clark Labs-Clark University.
Fang, Y., Wang, J., Huang, L. Y., and Zhai, T. L. (2020). Determining and Identifying Key Areas of Ecosystem Preservation and Restoration for Territorial Spatial Planning Based on Ecological Security Patterns: A Case Study of Yantai City. J. Nat. Resour. 35, 190–203.
Feinstein, A. R., and Cicchetti, D. V. (1990). High Agreement but Low Kappa: I. The Problems of Two Paradoxes. J. Clin. Epidemiol. 43, 543–549. doi:10.1016/0895-4356(90)90158-l
Ferraro, P. J., Sanchirico, J. N., and Smith, M. D. (2019). Causal Inference in Coupled Human and Natural Systems. Proc. Natl. Acad. Sci. U.S.A. 116, 5311–5318. doi:10.1073/pnas.1805563115
Forman, T. T. (1998). “Land Mosaics,” in The Ecology of Landscapes and Regions (New YorkUSA: Cambridge University Press).
Fu, F. J., Liu, Z. H., and Liu, H. (2021). Identifying Key Areas of Ecosystem Restoration for Territorial Space Based on Ecological Security Pattern: a Case Study in Hezhou City. Acta Ecologica Sinica 41. doi:10.5846/stxb202005241316
Fu, Y., Shi, X., He, J., Yuan, Y., and Qu, L. (2020). Identification and Optimization Strategy of County Ecological Security Pattern: A Case Study in the Loess Plateau, China. Ecol. Indicators 112, 106030. doi:10.1016/j.ecolind.2019.106030
Ge, D., Zhou, G., Qiao, W., and Yang, M. (2020). Land Use Transition and Rural Spatial Governance: Mechanism, Framework and Perspectives. J. Geogr. Sci. 30, 1325–1340. doi:10.1007/s11442-020-1784-x
Ghosh, S., and Chifos, C. (2017). The 1985 Siting of a Toyota Manufacturing Plant in Rural Kentucky, USA: The Ensuing Land Use Change and Implications for Planning. Landscape Urban Plann. 167, 288–301. doi:10.1016/j.landurbplan.2017.07.006
Ghosh, S., Das Chatterjee, N., and Dinda, S. (2021). Urban Ecological Security Assessment and Forecasting Using Integrated DEMATEL-ANP and CA-Markov Models: A Case Study on Kolkata Metropolitan Area, India. Sustain. Cities Soc. 68, 102773. doi:10.1016/j.scs.2021.102773
Gong, S. H., Xiao, Y., Zheng, H., Xiao, Y., and Ouyang, Z. Y. (2017). Spatial Patterns of Ecosystem Water Conservation in China and its Impact Factors Analysis. Acta Ecol. Sinica 37, 2455–2462. doi:10.5846/stxb201512012406
González Ovando, M. L., Plascencia Escalante, F. O., and Martínez-Trinidad, T. (2016). Áreas prioritarias para restauración ecológica y sitios de referencia en la región Chignahuapan-Zacatlán. Myb 22, 41–52. doi:10.21829/myb.2016.2221323
Haddad, N. M., Brudvig, L. A., Clobert, J., Davies, K. F., Gonzalez, A., Holt, R. D., et al. (2015). Habitat Fragmentation and its Lasting Impact on Earth's Ecosystems. Sci. Adv. 1, e1500052. doi:10.1126/sciadv.1500052
Hamilton, K., Sjardin, M., Marcello, T., and Xu, G. (2008). Ecosystem Marketplace and New Carbon Finance. Washington, D.C.
Han, J., Hayashi, Y., Cao, X., and Imura, H. (2009). Application of an Integrated System Dynamics and Cellular Automata Model for Urban Growth Assessment: A Case Study of Shanghai, China. Landscape Urban Plann. 91, 133–141. doi:10.1016/j.landurbplan.2008.12.002
Hansen, A. J. (2011). “Contribution of Source-Sink Theory to Protected Area Science,” in Sources, Sinks, and Sustainability across Landscapes. Editors J. Liu, A. Morzillo, and J. Wiens (Cambridge: Cambridge University Press).
He, J., Shi, X., Fu, Y., and Yuan, Y. (2020). Evaluation and Simulation of the Impact of Land Use Change on Ecosystem Services Trade-Offs in Ecological Restoration Areas, China. Land Use Policy 99. doi:10.1016/j.landusepol.2020.105020
He, S., Wang, D., Li, Y., Fang, Y., Lan, H., and Chen, W. (2019). Implementation of a Landscape Ecological Use Pattern Model: Debris Flow Waste-Shoal Land Use in the Yeyatang Basin, Yunnan Province, China. Land Use Policy 81, 483–492. doi:10.1016/j.landusepol.2018.11.024
Hepcan, Ş., Hepcan, Ç. C., Bouwma, I. M. I., Jongman, R. H. G., and Özkan, M. B. (2009). Ecological Networks as a New Approach for Nature Conservation in Turkey: A Case Study of İzmir Province. Landscape Urban Plann. 90, 143–154. doi:10.1016/j.landurbplan.2008.10.023
Hong, W., Guo, R., Su, M., Tang, H., Chen, L., and Hu, W. (2017). Sensitivity Evaluation and Land-Use Control of Urban Ecological Corridors: A Case Study of Shenzhen, China. Land Use Policy 62, 316–325. doi:10.1016/j.landusepol.2017.01.010
Iojă, C. I., Niţă, M. R., Vânău, G. O., Onose, D. A., and Gavrilidis, A. A. (2014). Using Multi-Criteria Analysis for the Identification of Spatial Land-Use Conflicts in the Bucharest Metropolitan Area. Ecol. Indicators 42, 112–121.
Jiang, S., Meng, J., and Zhu, L. (2020). Spatial and Temporal Analyses of Potential Land Use Conflict under the Constraints of Water Resources in the Middle Reaches of the Heihe River. Land Use Policy 97. doi:10.1016/j.landusepol.2020.104773
Jiang, W., Hu, Y., Wang, J., Sun, P., Zhang, Q., Huang, N., et al. (2021). Natural Rainfall Affects Soil Erosion of Typical Vegetationin Dabie Mountains, Anhui Province. J. Beijing Normal University(Natural Science) 57, 265–274.
Kang, J., Zhang, X., Zhu, X., and Zhang, B. (2021). Ecological Security Pattern: A New Idea for Balancing Regional Development and Ecological protection. A Case Study of the Jiaodong Peninsula, China. Glob. Ecol. Conservation 26, e01472. doi:10.1016/j.gecco.2021.e01472
Kim, I., and Arnhold, S. (2018). Mapping Environmental Land Use Conflict Potentials and Ecosystem Services in Agricultural Watersheds. Sci. Total Environ. 630, 827–838. doi:10.1016/j.scitotenv.2018.02.176
Kramer, D. B., Hartter, J., Boag, A. E., Jain, M., Stevens, K., Nicholas, K. A., et al. (2017). Top 40 Questions in Coupled Human and Natural Systems (CHANS) Research. Ecol. Soc. 22. doi:10.5751/es-09429-220244
Li, S., Xiao, W., Zhao, Y., and Lv, X. (2020). Incorporating Ecological Risk index in the Multi-Process MCRE Model to Optimize the Ecological Security Pattern in a Semi-arid Area with Intensive Coal Mining: A Case Study in Northern China. J. Clean. Prod. 247, 119143. doi:10.1016/j.jclepro.2019.119143
Liu, Q., Zhao, H., and Wu, K. (2014). Identifying Potential Land Use Conflict Based on Competitiveness of Different Land Use Types in Beijing, China. Resour. Sci. 36, 1579–1589.
Luque, S., Saura, S., and Fortin, M.-J. (2012). Landscape Connectivity Analysis for Conservation: Insights from Combining New Methods with Ecological and Genetic Data. Landscape Ecol. 27, 153–157. doi:10.1007/s10980-011-9700-5
Ma, K. M., Fu, B. J., and Li, X. Y. (2004). The Regional Pattern for Ecological Security (RPES): the Concept and Theoretical Basis. Acta Ecol. Sin. 24, 761–768.
Mahoney, D., Blandford, B., and Fox, J. (2021). Coupling the Probability of Connectivity and RUSLE Reveals Pathways of Sediment Transport and Soil Loss Rates for forest and Reclaimed Mine Landscapes. J. Hydrol. 594, 125963. doi:10.1016/j.jhydrol.2021.125963
Martins silva, T. C., and Guimaraes Vieira, I. C. (2020). Identification of Priority Areas for Ecological Restoration in Eastern Para, Brazil. Floresta E Ambiente 27. doi:10.1590/2179-8087.014418
Mcrae, B. H., Hall, S. A., Beier, P., and Theobald, D. M. (2012). Where to Restore Ecological Connectivity? Detecting Barriers and Quantifying Restoration Benefits. PLoS One 7, e52604. doi:10.1371/journal.pone.0052604
Mubareka, S., and Ehrlich, D. (2010). Identifying and Modelling Environmental Indicators for Assessing Population Vulnerability to Conflict Using Ground and Satellite Data. Ecol. Indicators 10, 493–503. doi:10.1016/j.ecolind.2009.09.002
Nhri, N. H. R. I. (2016). Comprehensive Water Resources Planning of Quanjiao County. Quanjiao County, China: Nanjing Hydraulic Research Institute.
Nie, X., Lu, B., Chen, Z., Yang, Y., Chen, S., Chen, Z., et al. (2020). Increase or Decrease? Integrating the CLUMondo and InVEST Models to Assess the Impact of the Implementation of the Major Function Oriented Zone Planning on Carbon Storage. Ecol. Indicators 118, 106708. doi:10.1016/j.ecolind.2020.106708
Peng, J., Pan, Y., Liu, Y., Zhao, H., and Wang, Y. (2018a). Linking Ecological Degradation Risk to Identify Ecological Security Patterns in a Rapidly Urbanizing Landscape. Habitat Int. 71, 110–124. doi:10.1016/j.habitatint.2017.11.010
Peng, J., Yang, Y., Liu, Y., Hu, Y. n., Du, Y., Meersmans, J., et al. (2018b). Linking Ecosystem Services and Circuit Theory to Identify Ecological Security Patterns. Sci. Total Environ. 644, 781–790. doi:10.1016/j.scitotenv.2018.06.292
Peng, J., Zhao, S., Dong, J., Liu, Y., Meersmans, J., Li, H., et al. (2019). Applying Ant colony Algorithm to Identify Ecological Security Patterns in Megacities. Environ. Model. Softw. 117, 214–222. doi:10.1016/j.envsoft.2019.03.017
Peng, W., Kong, D., Wu, C., Møller, A. P., and Longcore, T. (2020a). Predicted Effects of Chinese national park Policy on Wildlife Habitat Provisioning: Experience from a Plateau Wetland Ecosystem. Ecol. Indicators 115, 106346. doi:10.1016/j.ecolind.2020.106346
Peng, W., López-Carr, D., Wu, C., Wang, X., and Longcore, T. (2020b). What Factors Influence the Willingness of Protected Area Communities to Relocate? China's Ecological Relocation Policy for Dashanbao Protected Area. Sci. Total Environ. 727, 138364. doi:10.1016/j.scitotenv.2020.138364
Perino, A., Pereira, H. M., Navarro, L. M., Fernández, N., Bullock, J. M., Ceaușu, S., et al. (2019). Rewilding Complex Ecosystems. Science 364. doi:10.1126/science.aav5570
Pickett, S. T. A., Cadenasso, M. L., Rosi-Marshall, E. J., Belt, K. T., Groffman, P. M., Grove, J. M., et al. (2017). Dynamic Heterogeneity: a Framework to Promote Ecological Integration and Hypothesis Generation in Urban Systems. Urban Ecosyst. 20, 1–14. doi:10.1007/s11252-016-0574-9
Pontius, R. G., and Millones, M. (2011). Death to Kappa: Birth of Quantity Disagreement and Allocation Disagreement for Accuracy Assessment. Int. J. Remote Sensing 32, 4407–4429. doi:10.1080/01431161.2011.552923
Saura, S., and Pascual-Hortal, L. (2007). A New Habitat Availability index to Integrate Connectivity in Landscape Conservation Planning: Comparison with Existing Indices and Application to a Case Study. Landscape Urban Plann. 83, 91–103. doi:10.1016/j.landurbplan.2007.03.005
SOQC (2020). “Quanjiao County of National Economic and Social Development Statistical Bulletin in the Year of 2019,” in Quanjiao County,China: Statistics Office of Quanjiao County. Editor S. O. O. Q. County.
Spear, S. F., Balkenhol, N., Fortin, M.-J., Mcrae, B. H., and Scribner, K. (2010). Use of Resistance Surfaces for Landscape Genetic Studies: Considerations for Parameterization and Analysis. Mol. Ecol. 19, 3576–3591. doi:10.1111/j.1365-294x.2010.04657.x
Su, Y., Chen, X., Liao, J., Zhang, H., Wang, C., Ye, Y., et al. (2016). Modeling the Optimal Ecological Security Pattern for Guiding the Urban Constructed Land Expansions. Urban For. Urban Green. 19, 35–46. doi:10.1016/j.ufug.2016.06.013
Sun, W., Shao, Q., and Liu, J. (2014). Assessment of Soil Conservation Function of the Ecosystem Services on the Loess Plateau. J. Nat. Resour. 29, 365–376.
Tian, P., Zhu, Z., Yue, Q., He, Y., Zhang, Z., Hao, F., et al. (2021). Soil Erosion Assessment by RUSLE with Improved P Factor and its Validation: Case Study on Mountainous and Hilly Areas of Hubei Province, China. Int. Soil Water Conservation Res. 9, 433–444. doi:10.1016/j.iswcr.2021.04.007
Van Schalkwyk, J., Pryke, J. S., Samways, M. J., and Gaigher, R. (2020). Corridor Width Determines Strength of Edge Influence on Arthropods in Conservation Corridors. Landscape Ecol. 35, 1175–1185. doi:10.1007/s10980-020-01008-6
Vörösmarty, C. J., Osuna, V. R., Cak, A. D., Bhaduri, A., Bunn, S. E., Corsi, F., et al. (2018). Ecosystem-based Water Security and the Sustainable Development Goals (SDGs). Ecohydrology & Hydrobiology 18, 317–333.
Wang, C., Yu, C., chen, T., Feng, Z., Hu, Y., and Wu, K. (2020a). Can the Establishment of Ecological Security Patterns Improve Ecological protection? an Example of Nanchang, China. Sci. Total Environ. 740, 140051. doi:10.1016/j.scitotenv.2020.140051
Wang, C., Yu, C., chen, T., Feng, Z., Hu, Y., and Wu, K. (2020b). Can the Establishment of Ecological Security Patterns Improve Ecological protection? an Example of Nanchang, China. Sci. Total Environ. 740, 140051. doi:10.1016/j.scitotenv.2020.140051
Wang, S., Wu, M., Hu, M., Fan, C., Wang, T., and Xia, B. (2021). Promoting Landscape Connectivity of Highly Urbanized Area: An Ecological Network Approach. Ecol. Indicators 125, 107487. doi:10.1016/j.ecolind.2021.107487
Wang, S., Zhang, X., Wu, T., and Yang, Y. (2019a). The Evolution of Landscape Ecological Security in Beijing under the Influence of Different Policies in Recent Decades. Sci. Total Environ. 646, 49–57. doi:10.1016/j.scitotenv.2018.07.146
Wang, S., Zhang, X., Wu, T., and Yang, Y. (2019b). The Evolution of Landscape Ecological Security in Beijing under the Influence of Different Policies in Recent Decades. Sci. Total Environ. 646, 49–57. doi:10.1016/j.scitotenv.2018.07.146
Xun, X., and Zhang, Y. (2021). “China Meteorological Background Dataset,” in Resource And Environment Science And Data Center. Editor C. A. China.
Yang, R., Cao, Y., Hou, S., Peng, Q., Wang, X., Wang, F., et al. (2019). Cost-effective Priorities for the Expansion of Global Terrestrial Protected Areas: Setting post-2020 Global and National Targets. Sci. Adv. 6, eabc3436. doi:10.1126/sciadv.abc3436
Yu, K. (1996). Security Patterns and Surface Model in Landscape Ecological Planning. Landscape Urban Plann. 36, 1–17. doi:10.1016/s0169-2046(96)00331-3
Zhang, D., Wang, X., Qu, L., Li, S., Lin, Y., Yao, R., et al. (2020a). Land Use/cover Predictions Incorporating Ecological Security for the Yangtze River Delta Region, China. Ecol. Indicators 119, 106841. doi:10.1016/j.ecolind.2020.106841
Zhang, X., Jin, X., Liang, X., Zhao, Q., Ren, J., and Zhou, Y. (2020b). Identification and Optimization of Hierarchical Ecological Nodes Based on Multi-Target Genetic Algorithm: Take Jintan District of Changzhou as an Example. J. Nat. Resour. 35, 174–189.
Ziv, Y., and Davidowitz, G. (2019). When Landscape Ecology Meets Physiology: Effects of Habitat Fragmentation on Resource Allocation Trade-Offs. Front. Ecol. Evol. 7. doi:10.3389/fevo.2019.00137
Zou, L., Liu, Y., Wang, J., and Yang, Y. (2021). An Analysis of Land Use Conflict Potentials Based on Ecological-Production-Living Function in the Southeast Coastal Area of China. Ecol. Indicators 122, 107297. doi:10.1016/j.ecolind.2020.107297
Zou, L., Liu, Y., Wang, J., Yang, Y., and Wang, Y. (2019). Land Use Conflict Identification and Sustainable Development Scenario Simulation on China's Southeast Coast. J. Clean. Prod. 238. doi:10.1016/j.jclepro.2019.117899
Keywords: ecological security patterns, ecosystem services, land use conflicts, priority restoration areas, coupled human–nature system
Citation: Peng W, Zhang Z, He G, Liu X, Wang W, Cai Y and López-Carr D (2022) Integrating Potential Land Use Conflict Into Ecological Security Pattern in Response to Land Use/Cover Changes at a County Scale in Yangtze River Delta, China. Front. Earth Sci. 10:875433. doi: 10.3389/feart.2022.875433
Received: 14 February 2022; Accepted: 21 March 2022;
Published: 13 April 2022.
Edited by:
Chunyang He, Beijing Normal University, ChinaReviewed by:
Yuanyuan Zhao, Beijing Forestry University, ChinaJinghu Pan, Northwest Normal University, China
Libang Ma, Northwest Normal University, China
Copyright © 2022 Peng, Zhang, He, Liu, Wang, Cai and López-Carr. This is an open-access article distributed under the terms of the Creative Commons Attribution License (CC BY). The use, distribution or reproduction in other forums is permitted, provided the original author(s) and the copyright owner(s) are credited and that the original publication in this journal is cited, in accordance with accepted academic practice. No use, distribution or reproduction is permitted which does not comply with these terms.
*Correspondence: Yongli Cai, eWxjYWkyMDIwQHNqdHUuZWR1LmNu