- 1Alfred-Wegener-Institut Helmholtz-Zentrum für Polar- und Meeresforschung, Department of Climate Science, Bremerhaven, Germany
- 2Ocean Atmosphere Systems GmbH, Hamburg, Germany
- 3University Grenoble Alpes, Université de Toulouse, CNRM, Centre d’Études de la Neige, Grenoble, France
Reliable and detailed measurements of atmospheric and snow conditions in the Arctic are limited. While modern atmospheric reanalyses could potentially replace the former, the latter can be principally simulated by dedicated snow modelling. However, because the uncertainties of reanalyses and modelling are still exceptionally large at high latitudes, a thorough analysis of the performance of atmospheric reanalyses and the snow model simulations are required. Specifically, we aim to answer the following questions for Villum Research Station (VRS), northeast Greenland: (1) What are the predominant snow and meteorological conditions? (2) What are systematic differences between the modern atmospheric reanalysis ERA5 and in situ measurements? (3) Can the snow model Crocus simulate reliably snow depth and stratigraphy? We systematically compare atmospheric in situ measurements and ERA5 reanalysis (November 2015–August 2018) and evaluate simulated and measured snow depth (October 2014–September 2018). Moreover, modelled and measured vertical profiles of snow density and snow specific surface area (SSA) are analysed for two days where a survey had taken place. We found good agreement between in situ and ERA5 atmospheric variables with correlation coefficients >0.84 except for precipitation, wind speed, and wind direction. ERA5’s resolution is too coarse to resolve the topography in the study area adequately, leading presumably to the detected biases. Crocus can simulate satisfactorily the evolution of snow depth, but simulations of SSA and density profiles, whether driven by ERA5 or in situ measurements are biased compared to measurements. Unexpectedly, measured snow depth agrees better with ERA5 driven simulation than with simulation forced with in situ measurements (explained variance: 0.73 versus 0.23). This is due to differences in snowfall, humidity and air temperature between both forcing datasets. In conclusion, ERA5 has great potential to force snow models but the use of Crocus in the Arctic is affected by limitations such as inappropriate parametrisations for Arctic snowpack evolution, but also by lack of process formulations such as vertical water vapour transport. These limitations strongly affect the accuracy of the vertical profiles of physical snow properties.
1 Introduction
The availability of snow data, especially snow depth and physical snow properties in the Arctic, is temporally and spatially limited. However, snow plays a crucial role in the Arctic, as it covers the ground most of the year as well as the sea ice during the cold season. Snow depth, snow cover duration as well as snow properties such as density, specific surface area (SSA: Ice-air interface surface area divided by snow mass; in Calonne et al., 2020), thermal conductivity and albedo have climatic, ecology and socioeconomic impacts (Sturm et al., 1997; Hall, 2004; Callaghan et al., 2011; Box et al., 2012). Changes in snow cover alter the exchange of energy and mass between the land surface and the atmosphere, e.g., by modifying the albedo of the surface as well as the sensible, latent and ground heat fluxes and thus also the length of the growing season (Stiegler et al., 2016). Snow also affects the flora and fauna. It is an important habitat for Arctic animals from the smallest like lemmings to the biggest animals like the polar bears (Schmidt et al., 2012; Liston et al., 2016; Domine et al., 2018; Boelman et al., 2019).
Despite its importance, the description of snow in current models, e.g., sea ice-ocean models, is simple as in most cases a fixed density for all layers is assumed (e.g., Uotila et al., 2019). These simplifications strongly limit the value of the output of these systems. In addition, for numerical weather predictions more accurate snow models allowing for snow metamorphism that work not only in the mid-latitudes but also in the Arctic are strongly needed to represent high-latitude–mid-latitude linkages that affect the weather over, e.g., Europe. Coupling of already existing snow models could help to overcome these issues (e.g., Day et al., 2020).
Snow models are important tools to gain information about the snowpack evolution where snow measurements are sparse (Bartelt and Lehning, 2002; Liston and Elder, 2006; Vionnet et al., 2012). One snow model used for the Arctic is Crocus (e.g., Jacobi et al., 2010; Carmagnola et al., 2014; Essery et al., 2016; Barrere et al., 2017. For this study, we use the snow model Crocus due to the implementation of light absorbing impurities and related processes in snow (Tuzet et al., 2017), which we aim to use for future studies. The assessment of the performance of Crocus in the Arctic in previous studies shows that bulk variables are reasonably simulated while vertical profiles of snow properties are deficient, e.g., due to the absence of water vapour transport (Domine et al., 2016b; Barrere et al., 2017; Domine et al., 2019).
However, complete and sufficiently long time series of meteorological measurements, especially reliable precipitation data, to force snow models are limited in the Arctic (Boelman et al., 2019). Therefore, snow models are often driven by atmospheric reanalyses delivering complete time series of meteorological data for given locations or areas (Barrere et al., 2017; Gouttevin et al., 2018; Domine et al., 2019). Such reanalysis data are physically consistent, i.e., the individual variables of the reanalysis are consistent to each other and energy, mass, and momentum are conserved as the dataset is generated using physical equations in the model, which cannot be guaranteed for in situ observations (European Centre, 2020; Hersbach et al., 2020). Since 2016, data from the next-generation global atmospheric reanalysis ERA5 (European Centre for Medium-Range Weather Forecasts (ECMWF) ReAnalysis-fifth Generation (ERA5) atmospheric reanalyses data set) are available (Hersbach et al., 2020). Some studies already evaluated the performance of ERA5 in the Arctic (Wang, 2019; Delhasse et al., 2020).
However, more studies are needed to investigate strengths and weaknesses of the reanalysis accurately. So far, nobody has investigated the prevailing snow and atmospheric conditions at Villum Research Station (hereafter VRS). VRS is one of the few permanent research stations north of 80° N. Due to its remote location, which also allows the study of contamination, the possibility to conduct sea-ice related field measurements in close proximity, and the comparably smooth topography (compared to, e.g., Ny Alesund, Svalbard), the station is an important location for studying the effects of climate change in the Arctic. The station provides a complete set of atmospheric variables needed to force snow models including precipitation measurements, which are rarely measured in the Arctic. In addition, vertical snow profiles of snow density and SSA during an expedition in spring 2018 are available. For the first time, ERA5 is being used to force the snow model Crocus in the Arctic. Therefore, in this paper we aim to answer the following main questions.
(1) What are the snow and meteorological conditions at VRS, northeast Greenland?
(2) What are systematic differences between ERA5 and in situ measurements in northeast Greenland regarding variables needed to drive snow models at an Arctic site?
(3) Can Crocus simulate reliably snow depth evolution and the vertical profiles of snow density and SSA at an Arctic site in northeast Greenland, when forced with atmospheric in situ data or ERA5?
2 Data and methods
First, measured atmospheric variables and snow conditions will be presented. Then differences in atmospheric in situ measurements and the modern reanalysis ERA5 will be analysed before both datasets are used to drive the snow model Crocus. Finally, the results of the simulations will be analysed and compared to in situ snow measurements.
2.1 Study site
The study site is located in the surroundings of the atmospheric measurement mast at VRS (81°34′ N, 16°38′ W, 37 m above sea level) in northeast Greenland (Figure 1). VRS is one of the northernmost permanent research stations in the Arctic with an extensive long term monitoring programme of atmospheric measurements. At the station all meteorological variables to force snow simulations as well as air pollutants have been measured officially since 2015 (Christensen et al., 2020). The Wandel Sea in the north, a local ice cap called Flade Isblink in the south and east and fjords in the west surround the low land (Rasch et al., 2016). The Greenland ice sheet is located at a distance of more than 100 km to the southwest (Gryning et al., 2021). For the simulations we used 60% sand fraction, 30% clay fraction and 10% silt based on general information about soil conditions of NE-Greenland along the coast.
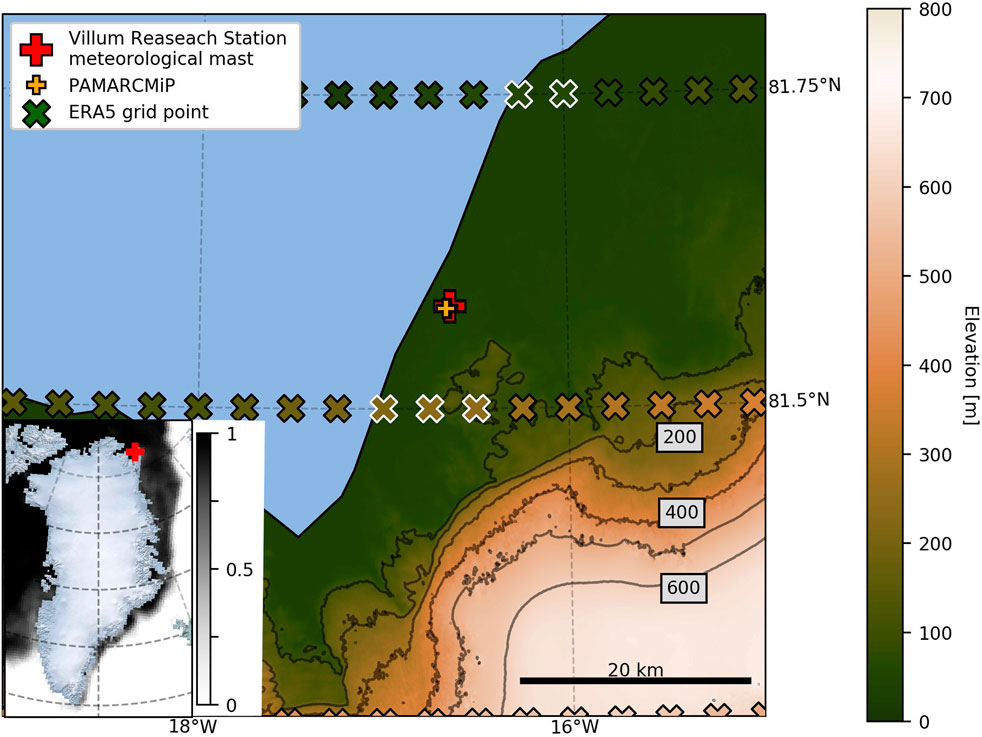
FIGURE 1. Map showing location of meteorological mast of Villum Research Station (red cross), measurement location during PAMARCMiP (yellow cross), ERA5 grid cell centres and terrain elevation (both using the same colour bar, the crosses are filled with colours according to the ERA5 elevation) in northeast Greenland (Digital elevation model: Howat et al., 2015; Howat et al., 2014). Crosses with white boundaries are the ERA5 grid cells used in this study. Small inserted panel at left bottom: Sea ice concentration on 15 March 2018 (Maslanik and Stroeve, 2018, National Snow and Data Center, updated yearly). Figure after Krampe et al. (2021) in TCD.
The observed annual mean temperature is −21°C. July is the warmest month (4°C) and March the coldest (−26°C) (Rasch et al., 2016; Gryning et al., 2021). The annual precipitation is 188 mm (Rasch et al., 2016). From mid-October to end of February there is polar night while from mid-April to beginning of September there is polar day (Nguyen et al., 2013).
2.2 In situ measurements
We use in situ observations from Greenland at VRS, where meteorological data from November 2015 to August 2018 and snow depth from August 2014 to September 2018 were measured to answer the research questions. In addition, during a campaign in spring 2018 numerous snow parameters, as snow depth, snow density and SSA were measured.
Meteorological data were measured at an automatic weather station (Villum Reserach Station, 2021). We chose the study period to compare atmospheric variables of ERA5 and in situ and simulated snow depth according to the availability of measured forcing variables between 27 November 2015 and 8 August 2018. Table 1 gives an overview on the installed meteorological sensors, their accuracy and the occurrence of missing data. In the Supplementary Appendix an overview about the different time periods used for validation and simulations in this study is delivered (Supplementary Appendix Table SA1) as well as a figure displaying the time series of most relevant atmospheric data (Supplementary Appendix Figure SA1).
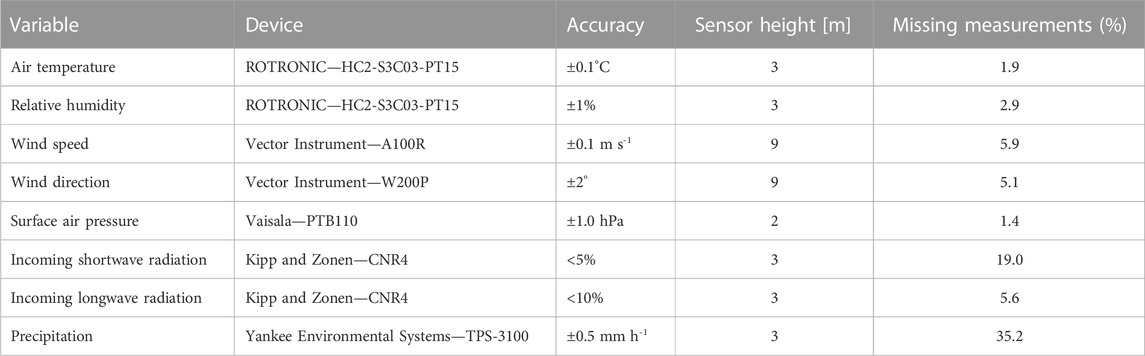
TABLE 1. Forcing data in the period 27 November 2015 to 8 August 2018—Meteorological sensors installed at Villum Research Station (Yankee Environmental Systems, 2012; ASIAQ, 2014). Table after Krampe et al. (2021) in TCD.
The precipitation sensor used is a hot plate sensor, which has several advantages over commonly used bucket gauges. It has a high catch-efficiency because the snow vaporises directly when it hits the hot plate, rather than being vaporised before it reaches the bottom of a bucket gauges sensor and thus not being measured. In addition, the sensor provides real time measurements from a rate of 0.1 mm h-1 within 60 s and is thus capable of measuring light precipitation and has an accuracy of ±0.5 mm h−1 (Yankee Environmental Systems, 2012).
In situ snow depth was measured at VRS from 26 August 2014 to 30 September 2018 (ASIAQ, 2014). In addition, we conducted snow measurements during the Polar Airborne Measurements and Arctic Regional Climate Model Simulation Project (PAMARCMiP) campaign, in a distance less than 500 m away from VRS, between 10 March and 8 April 2018. Measurements included snow density, SSA and snow depth. The meteorological situation during and shortly before the campaign is described in Section 3.2.2.
Vertical profiles of snow density and SSA were retrieved from data measured with a SnowMicroPen (SMP) Version 4, a snow penetrometer measuring the bonding force between snow grains with a vertical resolution of 1.25 mm (Schneebeli et al., 1999). Note that there are different parametrisations to obtain density and SSA from penetration force and hence calculating snow density and SSA from penetration force introduces uncertainties.
While the parametrisation from Proksch et al. (2015) was developed for an older SMP version, the parametrisations from Calonne et al. (2020) and King et al. (2020) were developed for the SMP version 4, used here. However, calibrations of the individual parametrisations were made for different regions and utilised different instruments. Proksch et al. (2015) used microcomputed tomography (micro-CT) data from the European Alps and the Arctic for the development of the parametrisation, while Calonne et al. (2020) employed IceCube and density cutter measurements from the Swiss Alps, i.e., only warm snow measurements. The density parametrisation of King et al. (2020) was developed using density cutter measurements from Arctic snow on sea ice.
As snow from different regions (e.g., Arctic and Alps, land and sea ice) is not similar, we tested all these parametrisations. Three parametrisations were applied for density and SSA was obtained based only on Calonne et al. (2020) and Proksch et al. (2015), as King et al. (2020) did not provide any SSA parametrisation.
Comparisons are shown in Figure 2. While densities calculated after Calonne et al. (2020) and King et al. (2020) differ only slightly, densities after Proksch et al. (2015) were considerably higher in the upper part of the profiles and generally more variable over the entire profile (Figure 2 shaded areas). However, density after King et al. (2020) is lower than obtained from the other parametrisations especially in the lower part. SSA after Calonne et al. (2020) differs strongly from Proksch et al. (2015) and is clearly higher and more variable over the entire profile.
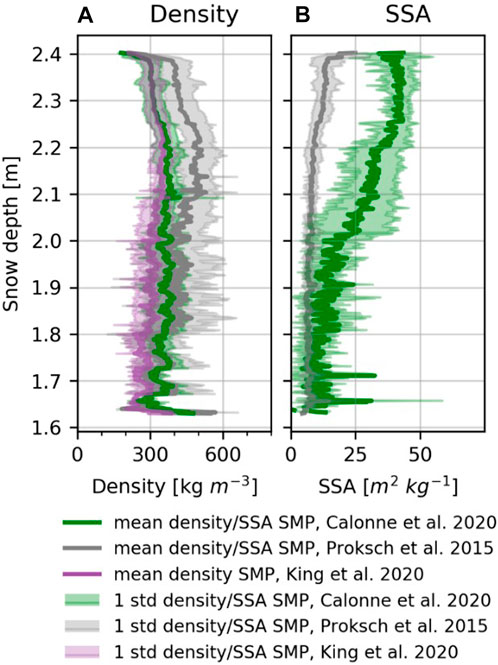
FIGURE 2. Comparison of SnowMicroPen (SMP) parametrisations of Calonne et al. (2020); Proksch et al. (2015); King et al. (2020) (only density) to obtain (A) snow density and (B) specific surface area (SSA) from penetration force measured on 23 March 2018. The shaded areas represent the standard deviation range. Figure from Krampe et al. (2021) in TCD.
After comparing the results for all parametrisations, we used results after Calonne et al. (2020) for the comparison with simulations. Calonne et al. (2020) provides parametrisations for density and SSA and is developed for the SMP version we used in the field. Further, calculated density is overall in good agreement with density calculated after King et al. (2020). However, our comparison shows that results should be interpreted with care.
Due to thick ice layers within the snowpack, the SMP could not penetrate the full vertical profile and snow depth measurements were not possible. The maximal penetration depth of the SMP measurements on 23 March 2018 was about 0.8 m.
In addition, on 3 April 2018, total snow depth was measured with a ruler, and every 0.1 m of the profile, snow density was measured with a density cutter (60 mm × 30 mm x 56 mm) and SSA with an IceCube3 at five locations. The optical system IceCube measures the hemispherical infrared reflectance of snow and converts the reflectance into SSA (Zuanon, 2013). Table 2 provides further details on the instruments used for the measurements.
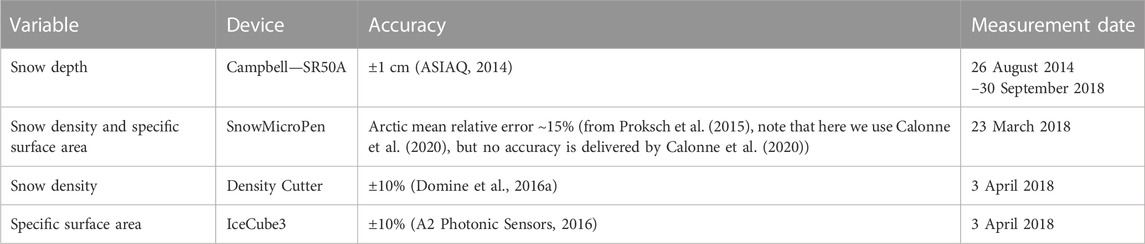
TABLE 2. Validation data—Instruments used to measure snow physics at Villum Research Station and during PAMARCMiP campaign. Krampe et al. (2021) in TCD.
2.3 Modelling
2.3.1 The snow model Crocus
We used the multilayer snow model Crocus (Brun et al., 1992; Vionnet et al., 2012) in single-column mode, i.e., we simulated only the temporal evolution of one column of one grid cell. The snow model is embedded in the surface scheme SURFEX (version 8.1) and is coupled to the soil model ISBA-DF (Boone et al., 2000). The snow model can deal with up to 50 dynamical snow layers. Layer thickness, heat content, density and age characterise each snow layer. The number of the snow layers is dynamical, i.e., layers can run empty (zero thickness).
Crocus describes the evolution of the snowpack by taking the energy- and mass balance of the snowpack into account. Implemented processes are surface melting, internal melting and refreezing, snow metamorphism, near-surface densification due to wind, enhanced sublimation for strong winds, fragmentation and compaction due to snowdrift, settling due to the weight of overlying snow layers and solar absorption in the snowpack. Snow compaction and microstructural changes due to wind drift in Crocus occur when wind speed exceeds a transport threshold depending on snow properties. Commonly, this threshold is at 6 m s−1 (Vionnet et al., 2013). These wind drift effects are passed on to the underlying layers with an exponential decay until the precalculated layer’s transport threshold falls below the wind speed. Snow redistribution is not taken into account (Vionnet et al., 2013).
Crocus is driven by surface fluxes derived from air temperature, specific humidity, wind speed, incoming direct and diffuse shortwave radiation, incoming longwave radiation, rain- and snowfall rate and surface air pressure. In addition to the atmospheric forcing, the model uses the terrain information aspect, slope and altitude as well as optionally wet and dry deposition coefficients of black carbon (BC) or other light absorbing impurities (Tuzet et al., 2017). A detailed description of the model can be found in Vionnet et al. (2012). Supplementary Appendix Table SA2 gives an overview about the employed process formulations.
2.3.2 Model simulations setup
2.3.2.1 ERA5
The global atmospheric reanalysis ERA5 is available in the Climate Data Store of Copernicus on a regular latitude-longitude grid at 0.25° × 0.25° resolution and has a temporal resolution of 1 hour (Hersbach et al., 2020). For VRS the grid cell size is 5 km along the longitude and 31 km along the latitude. The output of the simulations driven by ERA5 are representable on spatial scales of at least one grid cell size, i.e., on 5 km × 31 km.
Instead of interpolating spatially to the location of VRS, we took the nearest available grid cell (mid-point at 81.5° N 16.75° W, 185 m above sea level), which is about 10 km south from VRS. This is done to avoid spatial interpolation of the atmospheric variables, which might destruct their physical consistency. We ran the model with atmospheric input from the four other nearest neighbour grid cells to VRS located on land (Figure 1). The results of the additional simulations were taken as a metric for the co-location error. i.e., were deemed to give information on the representativeness of the ERA5 data (see Loew et al., 2017 for an introduction of the terminology used).
Due to a constant negative offset throughout the years in ERA5’s surface air pressure, probably caused by orographic effects (see Section 3.2.1), we adjusted ERA5 surface air pressure by adding the mean difference between in situ air pressure and ERA5 air pressure. To reduce spin-up effects, introduced through the unknown initial conditions of the soil, we run a first pass from January 2010 to December 2019 and used the initial conditions on 31 December 2019 for a second pass of this period, i.e., we use the 31 December 2019 conditions for the initial conditions of January 2010 in the second pass. This second pass simulation is called ERA5 control run (ERA5-CTRL). An overview of all model simulations performed is given in Table 3. Simulations to analyse the impact of individual forcing variables on the simulated snow depth (ERA5-sens, see Section 2.3.3) used ERA5-CTRL forcing but with disturbances on each individual forcing variable. Please see the Supplementary Appendix for more information on the preparation of the forcing data.

TABLE 3. Overview of all conducted model simulations (LWdown: Longwave radiation downwards, PSurf: Surface air pressure). Table after Krampe et al. (2021) in TCD.
2.3.2.2 In situ measurements
For the simulations driven by measured atmospheric data, meteorological data from VRS were used. The time step of the atmospheric data varies over time, except for precipitation. Until 24 April 2017, the time step is 30 min and 5 min thereafter. For the precipitation measurements, the time step is 5 min over the entire period. To force Crocus, we resampled the data to hourly mean or hourly accumulated data, respectively.
We used ERA5 data to fill in missing data (about 5%) for all in situ variables, except for precipitation. The amount and timing of precipitation events were too diverse between ERA5 and in situ measurements to fill the missing data. In addition, an overestimation of precipitation would be introduced when filling missing precipitation data with ERA5 precipitation, most likely because of the different spatial scales of precipitation in ERA5 and the in situ data (the latter containing much smaller scales). Therefore, we deliberately set missing precipitation data to zero knowing that this is causing an underestimation of the accumulated precipitation. Additionally, we examined the timing and length of the precipitation data gaps. There are many short gaps over the entire study period, as visible in Supplementary Appendix Figure SA2.
The in situ control simulation (Insitu-CTRL) is forced with in situ measurements from VRS from 27 November 2015 to 8 August 2018, except for the measured incoming longwave radiation, which was replaced by the corresponding ERA5 variable for the whole study period because of the systematic errors in this measurements (see Supplementary Appendix Figure SA1 for a comparison of measured and ERA5 longwave radiation). To reduce spin-up effects for the in situ simulation, we used the archived initial conditions from 26 November 2015 of ERA5-CTRL, as initial conditions for the in situ simulation. Please see the Supplementary Appendix for more information on the preparation of the forcing data.
2.3.3 Sensitivity simulations
To understand the difference between the simulated snow depths we used the approach of partial disturbances. With this approach we were able to quantify the contribution of every forcing variable to the difference in the simulated snow depth between ERA5-CTRL and Insitu-CTRL.
We used ERA5-CTRL as baseline simulation for our sensitivity survey because of its consistency and completeness of all forcing variables. From the ERA5 data, we calculated the daily standard deviation from January 2010 to August 2020 for every forcing variable. We used this longer time period to get more robust estimates of the standard deviations. Let m(xi) be the model state that is controlled by the forcing xi, whereby the index i enumerates the individual forcing variables. We added one-tenth of the standard deviation to the individual forcing variable as typical disturbance di while all other variables were unchanged in each individual simulation of ERA5-sens. Then, we determined the difference between the simulated snow depth for each of the ERA5-sens simulations m(xi + di) and the ERA5-CTRL simulation m(xi). The sensitivity e(xi) for every forcing variable is defined as:
with di being one-tenth of the standard deviation from the ERA5 ith forcing variable.
We estimated the influence of each forcing variable on the snow depth difference between Insitu-CTRL and ERA5-CTRL as:
with m(xi′)—m(xi) being the mean bias of in situ and ERA5 simulated snow depth and xi′—xi the mean difference between the ith in situ and the corresponding ERA5 forcing variable. For a perfect linear model, the sum of m(xi′)—m(xi) over all forcing variables xi would be equal to the snow depth difference between Insitu-CTRL and ERA5-CTRL. However, because of the non-linearities in the model the sum approximates the “real” difference of both simulations but allows us to identify the main sources of the difference between Insitu-CTRL and ERA5-CTRL with respect to the simulated snow depth.
3 Results
In the following, the results to answer the research questions raised in the introduction are presented. The measured conditions of the atmosphere and snow at the VRS are provided. Next, measurements of the required atmospheric forcing data are compared with ERA5. These two sections are needed as preparation to finally analyse the snow simulations in the last section of the results.
3.1 Measured conditions at Villum Research Station
3.1.1 In situ atmospheric measurements in general
An overview about the atmospheric conditions of the study period (27 November 2015–8 August 2018) is given in Supplementary Appendix Figure SA1. The mean near surface air temperature during the study period was −14.5°C. Thereby, the maximum air temperature was 14.1°C (26 July 2016) and the minimum air temperature was −41.1°C (10 March 2017). Mean air temperatures for the seasons were: December to February: −25.0°C, March to May: −20.4°C, June to August: 2.6°C, September to November: −13.7°C. Thereby, direct incoming shortwave radiation was present from February until October, whereby maximum incoming radiation of about 345 W m−2 was reached in June in all years.
Rainfall was present in 2016 in the months June to September, in 2017 in May to September and in 2018 in February, May, July and August. Thereby, maximum rainfall occurred in June and July where more than 30 mm rainfall was measured.
Snowfall can appear year round. Minimum snowfall occurred in July in all 3 years (lower than 5 mm). Snowfall higher than 100 mm w.e. (water equivalent) was measured in December 2015 (135.3 mm w.e.) and May 2017 (140.2 mm w.e.). But the most extreme snowfall event was measured in February 2018 (571.0 mm w.e.).
3.1.2 Measured snow conditions
Snow depth measurements at VRS are available from 26 August 2014 to 30 September 2018. As can be seen in Figure 3 and Table 4, snow cover started to form in late August in all years. Snow cover lasted until mid-July of the following year for the snow seasons 2014/15 and 2015/16, until late June for the snow season 2016/17, and until early August for 2017/18.
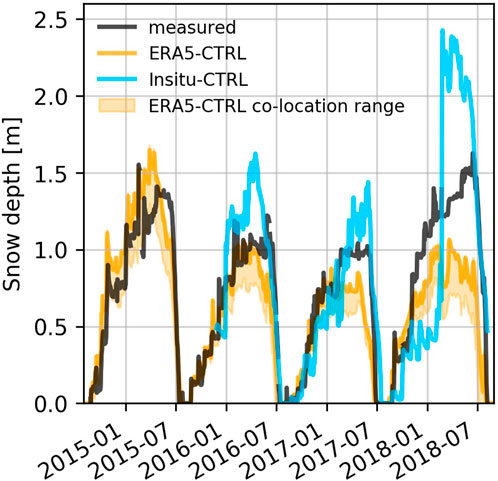
FIGURE 3. Snow depth at Villum Research Station from August 2014 to August 2018 covering four entire snow seasons. Measured snow depth (black line) and simulated by ERA5-CTRL (orange line—closest ERA5 grid cell to Villum Research Station) and by Insitu-CTRL (blue line–no data available before 27 November 2015 and after 7 August 2018). The orange shaded area visualises minimum and maximum snow depth of simulations at the four additional ERA5 grid cells nearest to Villum Research Station located on land and serve to illustrate the potential influence of the co-location error. Figure from Krampe et al. (2021) in TCD.

TABLE 4. Measured start and end dates of snow season for each year of the study period, defined as the first date on which snow depth exceeds or falls below 0.05 m, respectively. Height and date of maximum snow depth.
The date and height of the maximum snow depth varied considerably between the snow seasons with the highest maximum during the entire study period of 1.62 m (15 June 2018) and the lowest maximum snow depth of 1.03 m (23 April 2017). The long-term mean snow depth during the snow season was 0.74 m.
Snow depth varies considerably even on small spatial scales. With the SR50A-sensor at VRS, we can only measure snow depth at one point. On 3 April 2018, snow depth in the vicinity of VRS was additionally measured. It varied between 0.60 m and 1.17 m (standard deviation: 0.22 m). This might be caused by several small depressions and bumps that influenced local snow depth.
Mean snow density over all profiles measured with the SMP on 23 March 2018 was 307 kg m−3 (standard deviation: 33 kg m−3), while the SMP was only able to penetrate a maximum of about 0.8 m from the top of the snow layer due to thick ice lenses. The SMP measurements (Figure 2) showed a pronounced increase in the density from about 180 kg m−3 to about 300 kg m−3 in only a few centimetres down from the snow surface. This density peak could reflect a surface wind slab layer, which density cutter measurements cannot resolve.
The snow density cutter measurements on 3 April 2018 (Figure 4) result in a mean density of 291 kg m−3 (standard deviation: 67 kg m−3). During the snowpit surveys, measurements over the full profiles were possible and we found thick ice layers in the lower 0.4 m in 40% of the snowpits and 0.3 m–0.4 m thick compacted snow layers about 0.2 m below the snow-atmosphere interface in another 40% of the snowpits.
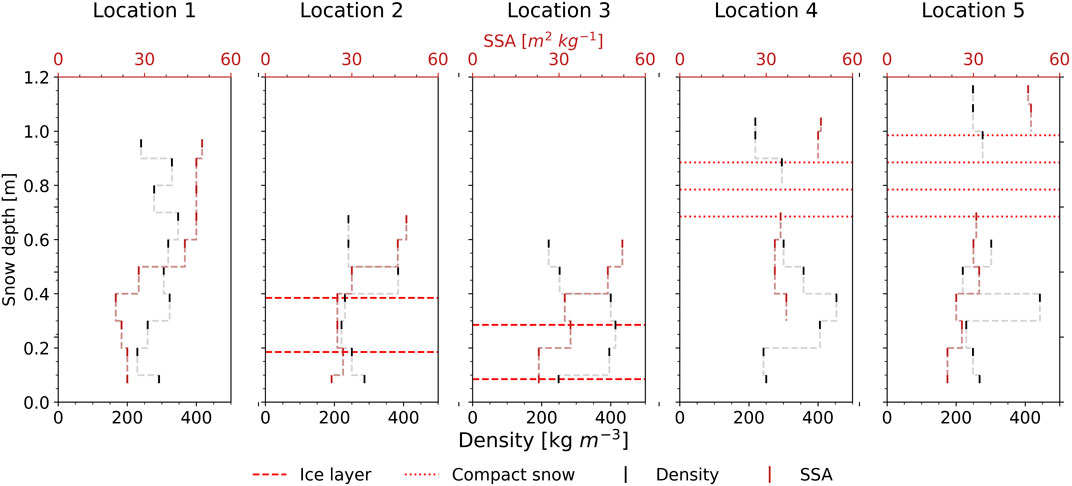
FIGURE 4. Observed snow densities with the density cutter in black and specific surface area with the IceCube in brown. Measurements from 3 April 2018 from five locations located every 50 m on a 200 m transect. Note the difference in snow depth of the five profiles.
We observed a decrease in density towards the basal layers. In addition, observations during fieldwork indicate a bottom depth hoar layer in our profiles. Our density observations reflect the typical stratigraphy of an Arctic snowpack: a basal depth hoar layer with low density (150–200 kg m−3) and high density at the top wind slab snow layers (exceeding 400 kg m−3).
On 23 March 2018 the mean SSA measured with the SMP (Figure 2) was 24 m2 kg−1 (standard deviation: 12 m2 kg−1) for the top 0.8 m. The mean surface SSA was 44 m2 kg−1 (standard deviation: 7 m2 kg−1). SSA was stable over the top 0.15 m and halved over the next 0.4 m (25 m2 kg−1) while it was stable again over the following 0.35 m.
Also, the IceCube measurements from 3 April 2018 (Figure 4) showed a decrease in SSA from the surface towards the middle part of the snow layers continued by an almost constant course to greater depth. Thereby, mean SSA was 34 m2 kg−1 (standard deviation: 11 m2 kg−1). However, amplitudes of the decrease and the absolute value of SSA between all snow profiles differed considerably.
3.2 Comparison of atmospheric in situ measurements and reanalysis data
3.2.1 Entire study period
Figure 5 shows scatter plots of daily anomalies of the atmospheric forcing variables from ERA5 and the in situ data. Anomalies are depicted because they drive the inter-annual variability in the snow variables to be analysed. Observed anomalies of direct shortwave radiation, diffuse shortwave radiation, specific humidity and air temperature agreed well. Mean biases were small and the Pearson correlation coefficients were between 0.84 and 0.91. ERA5 underestimated the air temperature by 1.0°C and the specific humidity by 0.0002 kg kg−1 compared to the in situ data. Longwave radiation showed a poor correlation (r: 0.28) and a high root mean square difference (RMSD: 60.5 W m−2), which we attribute to measurement errors in the in situ longwave radiation clearly visible in Supplementary Appendix Figure SA1.
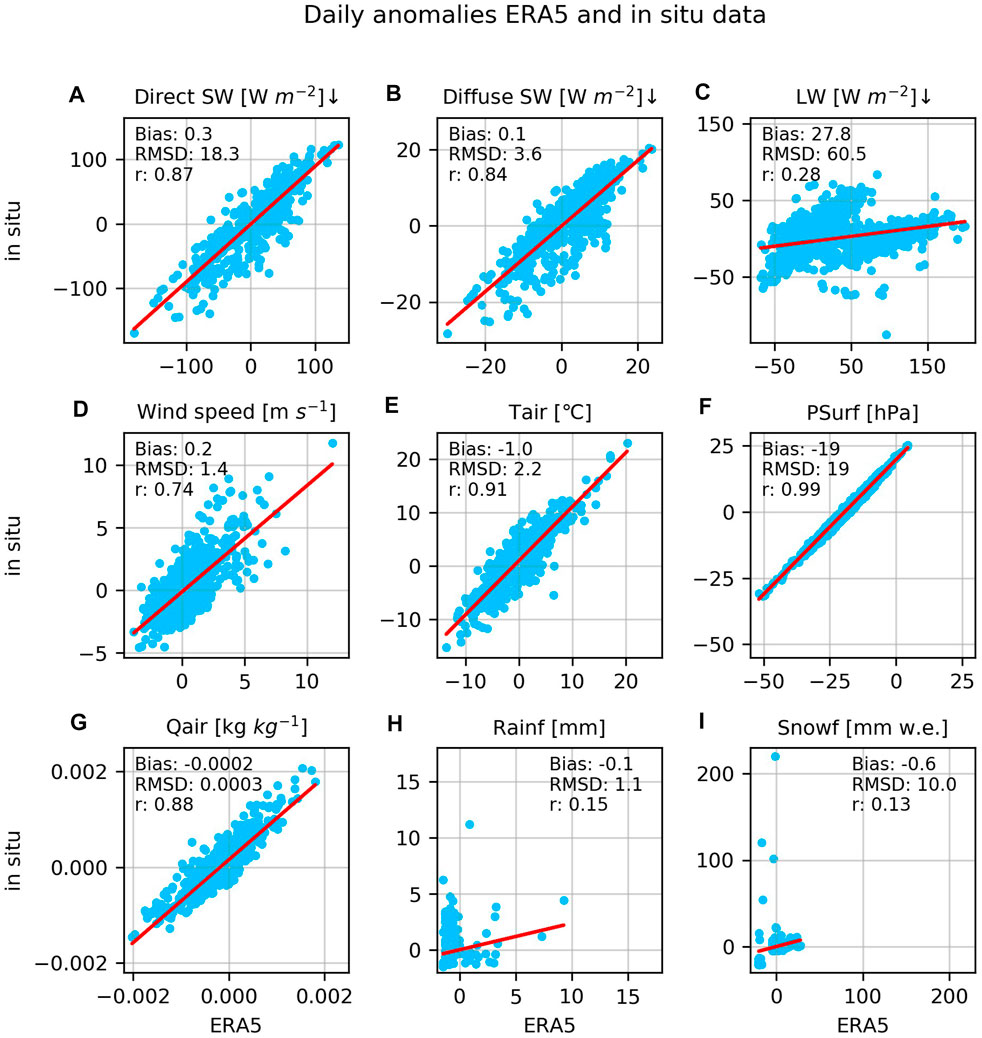
FIGURE 5. Scatter plots of ERA5 and in situ daily anomalies. Metrics inserted into each panel are the mean bias defined as ERA5–in situ, root mean squared difference (RMSD) and correlation coefficient (r). The red line depicts the regression line. (A) Direct incoming shortwave radiation (SW), (B) diffuse incoming SW, (C) incoming longwave radiation (LW), (D) wind speed, (E) air temperature (Tair), (F) surface air pressure (PSurf), (G) specific humidity (Qair), (H) rainfall (Rainf) and (I) snowfall (Snowf) in mm w.e. (water equivalent). Figure from Krampe et al. (2021) in TCD.
Atmospheric surface pressure correlated well with in situ surface pressure (r: 0.99) but showed a systematic offset of 19 hPa. We attribute this bias to the altitude difference of the ERA5 nearest grid cell to VRS of about 150 m caused by the relatively coarse resolution of ERA5.
Rain- and snowfall showed mean biases of 0.1 mm day−1 and 0.6 mm day−1 w.e. and poor correlations of 0.15 and 0.13, respectively. Observed and ERA5 precipitation differed strongly not only in the amount but also in the timing of extreme events. In situ precipitation was generally higher than ERA5 precipitation.
In situ measurements showed clearly more snowfall over the entire study period than ERA5 (70% of total measured snowfall). In contrast, the frequency of ERA5 snowfall was more than three times higher than the frequency of in situ snowfall, i.e., ERA5 showed many small snowfall events.
ERA5 and measured wind direction showed large deviations. Measured preferred wind direction was from the south-west while ERA5’s preferred wind direction was from the south (Supplementary Appendix Figure SA3). The mean wind speed from ERA5 over the entire study period was slightly higher than measured (0.2 m s−1). However, ERA5 underestimated the occurrence of wind speeds higher than 6 m s−1.
In summary, there was a good match between atmospheric measurements and ERA5 for the incoming direct and diffuse shortwave radiation, incoming longwave radiation (except the period of obvious measurement errors) and specific humidity. Air temperature and surface air pressure agreed well in general but altitudinal differences between actual topography and ERA5 resolved topography have to be taken into account. Lower wind speeds were reproduced well by ERA5 but there were underestimations of higher wind speeds. Rainfall and snowfall showed considerable differences in frequency and amount.
3.2.2 During and shortly before the PAMARCMiP campaign
Exceptional weather conditions lasting for about 2 weeks were present in the second half of February 2018 (Figure 6). Even air temperatures above freezing temperature occurred. The mean daily air temperatures between 17 and 27 February 2018 were about 20°C warmer than in the weeks before and after.
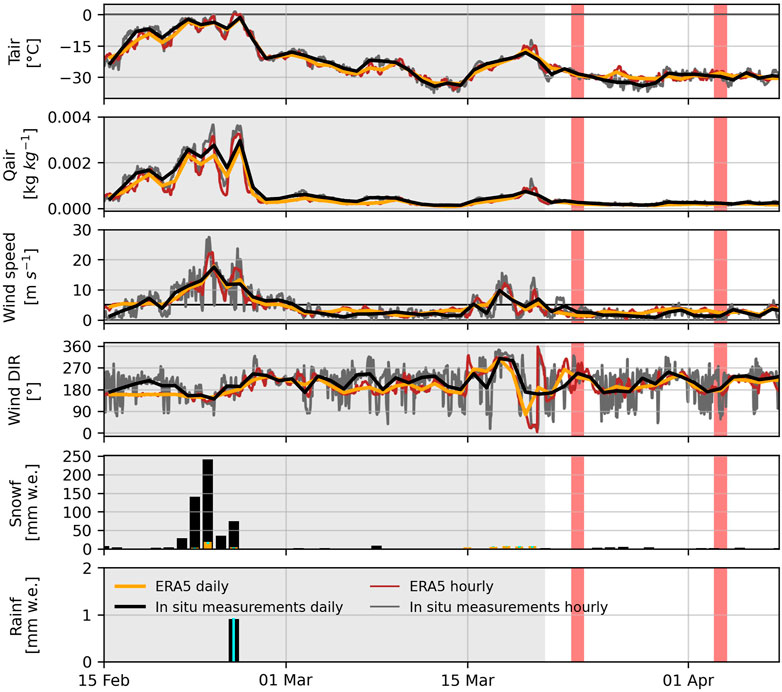
FIGURE 6. Atmospheric development from 15 February 2018 to end of PAMARCMiP campaign 8 April 2018. PAMARCMiP time is shown as the white and red area, whereby the red area is the time of the snowpit measurements. Cyan vertical lines in the panels for snow- and rainfall show the co-location error of ERA5. In case of rainfall the vertical line indicates the occurrence of rainfall on neighbouring grid cells (see text). Figure after Krampe et al. (2021) in TCD.
During the PAMARCMiP campaign (10 March to 8 April 2018), air temperature was about −20°C. A high pressure system located over the North Pole together with several weak low pressure systems over northeast Greenland were present (see also Nakoudi et al., 2020). The warm event was accompanied by remarkably strong winds. In situ wind speeds exceeded 20 m s−1. During the time of the snowpit survey observed wind speeds were about 6 m s−1.
ERA5 strongly underestimated snowfall during the warm event. Between 17 February 2018 and 27 February 2018, ERA5 snowfall was about 5% of the in situ snowfall (ERA5: 28 mm w.e., in situ: 530 mm w.e.). Due to the short periods of air temperature above the freezing temperature there was also some rainfall measured. ERA5 rainfall during that time was close to zero at 81.5° N, 16.75° W, while neighbouring grid cells showed a similar amount of rainfall of 1 mm as the in situ measurements.
Apart from the warm event the amount of precipitation in both datasets were similar, but the timing of precipitation events differed strongly. During PAMARCMiP, in situ measurements showed a snowfall event lasting over several days, which is not present in ERA5. Instead, ERA5 showed snowfall about a week before the campaign. Field observations during PAMARCMiP confirmed strong snowfall events.
In summary, ERA5 is able to catch the heat waves and strong winds in the second half of February 2018. Most variables match well during this period apart from precipitation and some minor deviations in wind direction and wind speed during peak times, where ERA5 underestimates.
Since ice layers were detected in the snowpack during PAMARCMiP we analysed the occurrence and timing of rain on snow events to investigate whether rain on snow events were responsible. For this purpose, we looked at rainfall events where there was ≥0.05 m of snow on the ground for the period where precipitation was measured (26 November 2015 to 8 August 2018, 987 days). During this period, there were 861 days with snow depth ≥0.05 m. On 52 days, i.e., in about 5% of the time, there were rain on snow events. They occurred dominantly at the end of the snow season (see Supplementary Appendix Figure SA4). However, 2018 was the only year during the study period, where rain and therefore rain on snow events were present already in February. Therefore, we assume that the ice layers found are caused not only by melting and refreezing of snow but also by rain on snow events.
3.3 Snow simulations at Villum Research Station
In the last subsections, we examined the differences between atmospheric measurements and ERA5. This first step is important to understand the differences in snow simulation results that arise from the different forcings. With this background, in the following subsections we examined the simulated snow properties using these atmospheric data to force the snow model Crocus and compared it with the presented snow measurements.
3.3.1 Simulated snow depth
Overall ERA5-CTRL simulated the snow depth for the winter-centred years 2015/16, 2016/17 and 2017/18 in good agreement with the measurements (RMSD: 0.29 m) (Figure 7A). Until early spring, the measured snow depth was within the co-location error of the ERA5-CTRL simulations. Melting started too early compared to the measurements (Figure 3). Date and amount of the maximum snow depth showed some moderate differences.
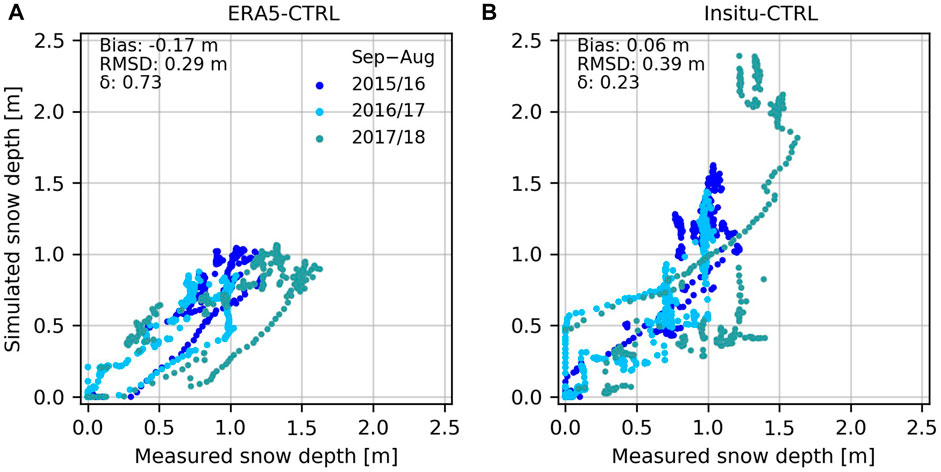
FIGURE 7. Scatter diagram of measured and simulated daily snow depth between 27 November 2015 and 8 August 2018. For (A) ERA5-CTRL simulation and (B) Insitu-CTRL simulation. The different colours visualise different years. Metrics displayed are the mean bias, root mean squared difference (RMSD) and explained variance δ. Figure after Krampe et al. (2021) in TCD.
While the onset of snow accumulation in 2017/18 of ERA5-CTRL was in good agreement with the observation, the simulation underestimated the snow depth from November 2017 onwards strongly. From late February to mid-March 2018, ERA5-CTRL showed even a slight reduction while the measured snow depth is growing, which further increased the difference between simulated and measured snow depth. Over the entire study period, the mean bias between ERA5-CTRL’s snow depth and the observation was −0.17 m (Figure 7A).
In contrast, the snow depth simulated by Insitu-CTRL overestimated the maximum snow depth in all 3 years. However, for the in situ forced simulation the initial values (e.g., snow depth) was taken from the spin-up run with ERA5-CTRL forcing on 26 November 2015 as no snow depth measurements were available before 27 November 2015. Therefore, in 2015/16 Insitu-CTRL was certainly impacted by the initial values and was difficult to evaluate.
The end of the snow season of 2015/16 agreed well with the snow depth measurements (Figure 3). In Insitu-CTRL the onset of snow accumulation and snow depth of the year 2016/17 matched well until late spring. Later in the year the measured accumulation events were too strong leading to an overestimation of the snow depth. The end of the snow season occurred somewhat later than observed in 2017.
In 2017/18, Insitu-CTRL overestimated the maximum snow depth by almost 1 m and showed a later than measured increase in snow depth. Also, the in situ simulation clearly underestimated the snow depth at the beginning of the snow season 2017/18 until the strong precipitation event in February. From late February to early March, there was a distinct increase in snow depth of about 1.5 m within a few days. This event led to an overestimation of snow depth after the event (see Supplementary Appendix Figure SA5). Even if over the entire study period the mean bias between Insitu-CTRL and the observed snow depth was low (0.06 m), the RMSD is larger as in ERA5-CTRL (0.39 m versus 0.29 m). The explained variance, which is the proportion of variance in the observation that can be explained by the simulation was 23% (Figure 7B) while it was 73% in the ERA5-CTRL simulation.
Supplementary Appendix Figure SA1 shows that the monthly snow depth disagreements between measured and both simulated snow depths were highest during the melting season. Furthermore, the snow depth deviations were higher for the simulations driven by atmospheric in situ data than for the ERA5 driven simulations.
As the sensitivity survey (Table 5) showed, the differences between the in situ and ERA5 forcing in snowfall and specific humidity but also to a lower degree air temperature were dominantly causing the deviation between the simulated snow depth of Insitu-CTRL and ERA5-CTRL. The remaining forcing variables had minor impact.
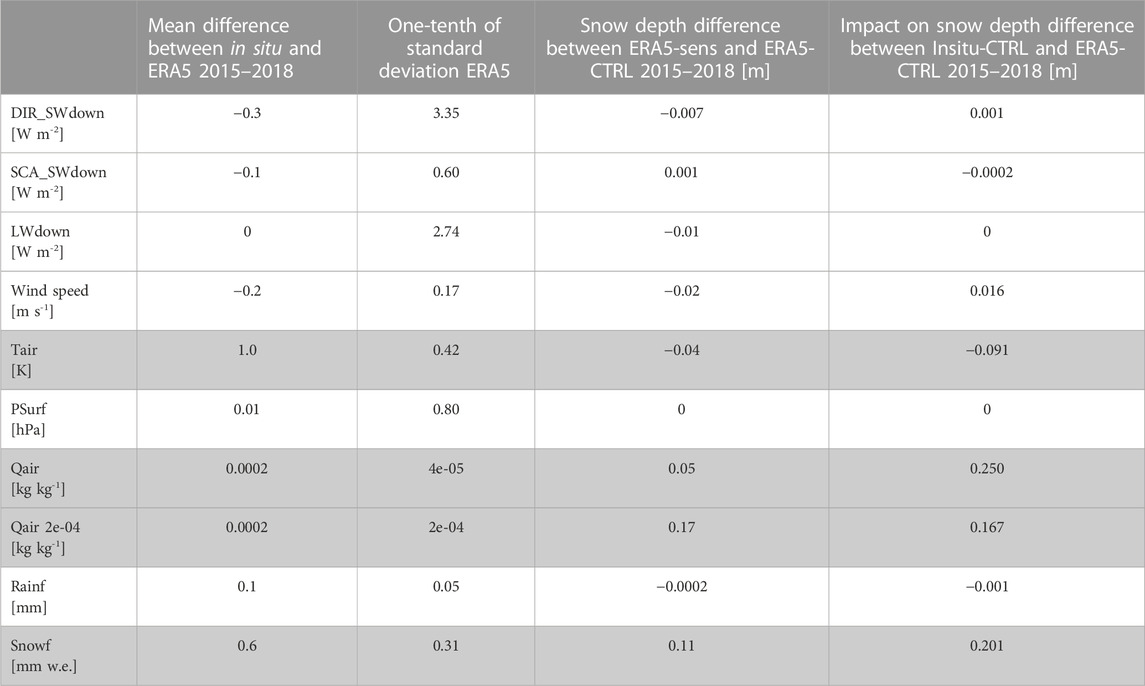
TABLE 5. Results of sensitivity survey. CTRL refers to the control simulations described in Section 2.3.2. Mean difference between in situ and ERA5 2015–2018 refers to the atmospheric forcings while remaining columns refer to the simulations. DIR_SWdown: Direct incoming shortwave radiation, SCA_SWdown: Diffuse incoming shortwave radiation, LWdown: Incoming longwave radiation, Tair: Air temperature, PSurf: Surface air pressure, Qair: Specific humidity, Rainf: Rainfall and Snowf: Snowfall in water equivalent (w.e.). Absolute values below 10−4 are set to zero. Table after Krampe et al. (2021) in TCD
The large response to the differences in specific humidity turned out to be an artefact of the linearisation. The experiment was rerun with a disturbance of 0.0002 kg−1 and resulted in a difference in snow depth of 0.17 m. This shows the limits of our approach–the sensitivity calculated for a disturbance of one-tenth of the standard deviation of the variability of the specific humidity was overestimated. Adding up the impacts on the snow depths of the perturbations on snowfall, specific humidity (0.0002 kg−1) and air temperature amounted to 0.28 m and compared well with the mean difference in snow depths between Insitu-CTRL and ERA5-CTRL of 0.23 m, i.e., these forcing variables were causing the difference.
In summary, ERA5 simulated snow depth surprisingly clearly outperformed in situ simulated snow depth. Taking into account the considerable spatial snow depth variability, ERA5 simulated snow depth, which represents a mean of the grid cell, agreed unexpectedly well with the point measurements at VRS. We found that up to 73% of the variance measured could be described by the ERA5 forced simulation. This is a high value for any kind of simulation of the earth system. The in situ simulated snow depth showed larger deviations from the measured snow depth than the ERA5 simulation (only up to 23% explained variance).
Another reason for differences in snow depth between ERA5 and in situ forced simulations and the measurements is attributed to variations in the snow densities. Hence, we calculated the snow water equivalent from measured snow depth by assuming a snow density of 250 kg m−3 and compared it with the simulated snow water equivalent. This confirmed that main differences in simulated and measured snow depth are due to different precipitation rates. However, we would need snow water equivalent measurements or higher vertically and temporally resolved snow density measurements to compare accurately snow water equivalents of simulations and measurements.
3.3.2 Simulated snow density profiles
Overall, simulated and measured density profiles showed a poor agreement for the CTRL simulations (Figures 8A, 9A). Simulated snow densities, both in ERA5-CTRL and Insitu-CTRL were much lower than measured. This was especially true for the upper part of the profiles.
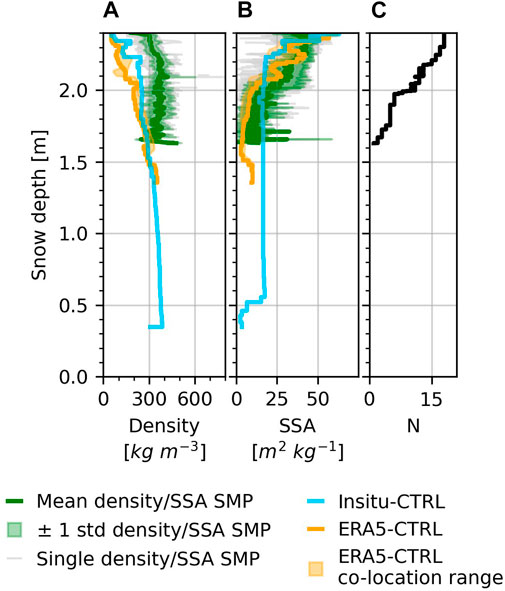
FIGURE 8. Simulated and measured snow density and specific surface area (SSA) on 23 March 2018. Measured density and SSA are derived after Calonne et al. (2020) from the SnowMicroPen (SMP) from 13 SMP measurements on a 150 m transect. (A) Displays snow density, (B) SSA and (C) the sampling frequency of SMP measurements. Snow depth baseline is the maximum snow depth during PAMARCMiP campaign of all measurements and simulations (2.4 m) as the SMP did not penetrate the entire snowpack (see also explanation in the Supplementary Appendix). Single density/SSA SMP shows measured single SMP profiles. The meaning of the colours are expressed in the legend. Figure after Krampe et al. (2021) in TCD.
Simulated snow densities showed a clear densification with depth and did not capture the snow density decrease from 388 kg m−3 to 270 kg m−3 measured with the density cutter in the lower part of the snowpack (e.g., below 0.3 of normalised snow depth, Figure 9A).
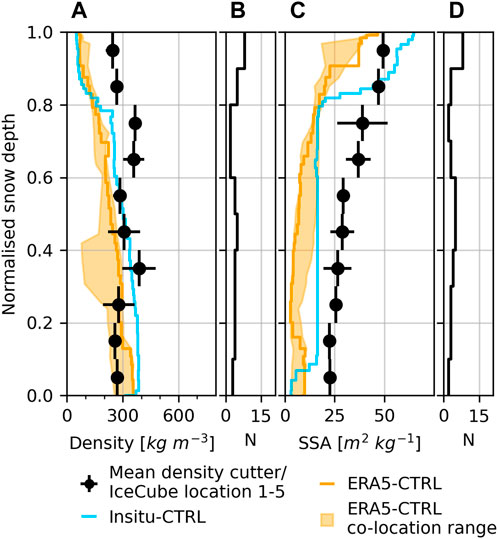
FIGURE 9. Simulated and measured snow density from density cutter and specific surface area (SSA) from IceCube on 3 April 2018. (A) Snow density, (C) SSA and (B) and (D) the sampling frequency of density cutter measurements and IceCube measurements, respectively. Figure after Krampe et al. (2021) in TCD.
SMP measurements showed a pronounced increase in the density from 180 kg m−3 to 300 kg m−3 over a few centimetres below the snow surface, which density cutter measurements could not resolve. Both simulations (ERA5-CTRL and Insitu-CTRL) were not able to capture this density increase near the surface (Figure 8A).
Vertical mean density in Insitu-CTRL was higher than in ERA5-CTRL on 23 March 2018 and 3 April 2018. In Insitu-CTRL (Figure 9A) thick snow layer of recent snowfall was visible at the top of the profile (1–0.85 of normalised snow depth). Also, density in ERA5-CTRL indicated some recent snowfall but less pronounced compared to Insitu-CTRL (Figure 9A, 1–0.9 of normalised snow depth).
3.3.3 Simulated SSA profiles
Overall, SSA measured by the SMP and simulated were in some agreement when the sample variability of the measurements is taken into account (the simulated SSA was within the range spanned by ±1 standard deviation of the sampling variability). SSA in ERA5-CTRL was much more variable in depth than Insitu-CTRL on 23 March 2018 (Figure 8B). SSA in ERA5-CTRL showed three peaks while SSA in Insitu-CTRL was more uniform in depth. SSA measured with the SMP showed a huge standard deviation, which led to some match with simulated SSA even if both simulated profiles differed by about 10 m2 kg−1 in average.
The vertical profiles of the SSA of both simulations were similar in form to the observations taken with the IceCube on 3 April 2018 (Figure 9C). All profiles showed a decrease in SSA from the surface towards the middle part of the snow profile continued by an almost constant course to greater depth.
However, amplitudes of the decrease and the absolute value of SSA differed considerably. ERA5-CTRL started at the surface with a realistic SSA of 47 m2 kg−1 but decreased stronger than the measurements with depth. Therefore, ERA5-CTRL underestimated SSA over the entire snow profile and showed mostly values below Insitu-CTRL. It decreased from the top of the snowpack until 0.6 m, followed by a constant middle part with SSA lower than 10 m2 kg−1. SSA increased in the lower part of the profile until the ground, which contradicts the measurements. SSA in Insitu-CTRL showed an overestimation of surface SSA of about 15 m2 kg−1 but it decreased stronger than measured before it became rather stable from 0.8 to 0.1 m while the measurement showed a slight decrease over the whole profile with higher SSA than Insitu-CTRL.
Overall, ERA5-CTRL and Insitu-CTRL could capture the gross profile of measured SSA. However, both simulations failed to reproduce the details in the measured SSA profiles. Moreover, the SSA decreasing rates from top to bottom of the profiles were different for simulations and measurements.
4 Discussion
The first research question is already addressed in the results section and a further discussion is redundant. The first subsection discusses the systematic differences between in situ and reanalysis datasets with respect to variables needed to model simulations (research question 2) and the second subsection treats the performance of the snow model Crocus (research question 3).
4.1 Comparison of atmospheric in situ measurements and reanalysis data at Villum Research Station
Atmospheric variables measured at VRS are point measurements representing local conditions. ERA5 on the other hand represents mean values in a grid cell. Due to the relatively coarse resolution of ERA5, the orographic relief is much smoother than in reality. This causes discrepancies in altitude and leads to differences, e.g., in surface air pressure. After correcting for this ERA5 air pressure agrees well with measurements, which is in line with the findings of Delhasse et al. (2020).
We find a good correspondence between measured and ERA5 air temperatures, shortwave and longwave radiation (excluding the erroneous time period) in agreement with other studies (Betts et al., 2019; Wang et al., 2019; Delhasse et al., 2020). Differences in observed and ERA5 surface air temperature can also be explained by the elevation difference between VRS and the ERA5 grid cell.
Differences in topography also affect wind speed and wind direction (Delhasse et al., 2020). Higher obstacles to the southeast from VRS, not resolved by ERA5, are impacting wind direction. In addition, 10 m winds of ERA5 are analysed from the respective profile with the vertical resolution of the atmospheric model which causes differences in wind speed and wind direction between the reanalysis and in situ measurements. This can be problematic in terms of snow modelling, as wind is an important variable to simulate snow density due to wind compaction, enhanced sublimation and wind redistribution at higher wind speeds.
In our study, generally ERA5 overestimates wind speed (mean over the whole study period) while Betts et al. (2019) found an underestimation for the Canadian Prairies; Delhasse et al. (2020) identified an underestimation over stations located in the ablation area of the Greenland Ice Sheet but mostly overestimation for stations located in the accumulation area (Delhasse et al., 2020). However, we identify wind speed underestimation for higher wind speeds (>∼6 m s−1) in agreement with Betts et al. (2019) and Delhasse et al. (2020).
Our analyses of the wind direction show strong differences between reanalysis and in situ data, although without impact on our simulations because we do not prescribe an aspect ratio. However, the difference between measured and ERA5 wind direction might have to be taken into account for other studies. Especially when it comes, e.g., to modelling of snow redistribution by snowdrift, wind direction plays an important role and has to be handled with care.
The reanalysis is only able to resolve processes which are within the scales of the model resolution or even larger (Minola et al., 2020). Precipitation on a kilometre scale can already vary significantly (Betts et al., 2019). Therefore, ERA5 cannot resolve local precipitation events as for instance visible in the atmospheric data during PAMARCMiP where ERA5 underestimates precipitation during the warm event in the second half of February 2018.
Large measurement gaps in in situ precipitation are problematic. We underestimate the precipitation accumulated over time because we replaced these gaps by zero precipitation. Nonetheless, the in situ simulations clearly overestimate the snow depth, i.e., our simulations suggest that the in situ precipitation is not consistent to the measured snow depth. False precipitation measurements can be caused by snowdrift, which is falsely detected as local precipitation.
Because the snow season 2017/18 shows the most pronounced deviation of measured and simulated snow depth (larger for the in situ than for the reanalysis simulations), we had a closer look into this season. We compared the monthly cumulative in situ precipitation with the monthly cumulative precipitation of ERA5 and GPCP (Global Precipitation Climatology Project, Adler et al., 2016) (see Supplementary Figure SA5). During the first five months (September 2017 to January 2017), in situ precipitation is slightly lower than GPCP precipitation and ERA5 precipitation. This can be explained by the setting of gaps in measured precipitation to zero that we performed. In February 2018 there is high precipitation during the exceptional warm air intrusion event lasting over several days. This event is not seen in ERA5 or GPCP. It is also not reflected in the measured snow depth, which only rises slightly during that time. However, as also wind speed was extreme and the precipitation instrument in general is known as reliable even in windy conditions (Nitu et al., 2018), we cannot exclude that this was a local precipitation event which was not captured by the reanalyses products and that additional snowfall was blown away. The high snowfall event in February 2018 leads to the sudden increase of snow depth in the simulations forced by in situ data not seen in the simulations forced by ERA5.
We consider the uncertainties caused by filling gaps of the remaining forcing variables with ERA5 as neglectable. ERA5 shortwave radiation agrees well with observations (19.0% missing values) and Crocus shows a minor impact of differences in in situ and ERA5 shortwave radiation. The measurement gaps of the other variables are below 6.0% and therefore considered as unproblematic.
4.2 Snow simulations at Villum Research Station
4.2.1 Simulated snow depth
We can show that Crocus is in principle able to simulate reliably snow depth evolutions for an Arctic site when uncertainties of the atmospheric forcing are small. If the results can be transferred to other locations is, of course, questionable and will be part of future research. Other studies have come to the same conclusion but in contrast to our study most studies also adjusted the forcing data (Jacobi et al., 2010; Sauter and Obleitner, 2015; Barrere et al., 2017; Luijting et al., 2018), e.g., scale measured snowfall to the maximum measured snow water equivalent measurement. This does not allow for a proper analysis of the quality of the model.
We found that ERA5 considerably underestimates snow accumulation compared to in situ measurements during the warm air intrusion in spring 2018 and for other major precipitation events, which accumulates as well to a strong underestimation of snow depth throughout the snow season in 2018. In our study, deviations in simulated and measured snow densities contributed to differences in snow depth between simulations and measurements. Also, Luijting et al. (2018) found accumulated over- and underestimations of snow depth of about 1 m over a snow season.
We found highest impacts on the in situ and ERA5 forced simulations with regard to the snow depths for snowfall rate, specific humidity and air temperature. Sauter and Obleitner (2015) showed that uncertainties in forcing can lead to more than 3 m deviation in snow depth after one year. This again highlights how problematic biases due to missing data, snowdrift and riming on instruments and measurement uncertainties in the measured data are.
Snow redistribution due to strong winds is not implemented in the model and could be another reason for deviations between observed and simulated snow depth (see also Luijting et al., 2018). In the Arctic, snow redistribution is important. However, we investigated the correlation of wind speed above 6 m s−1 and snowfall for both forcing datasets and calculated a Pearson correlation of 0.46 and 0.51 for in situ and ERA5 data, respectively. From this we conclude that the impact of snowdrift at our location is probably not large and that therefore the timing of snow incorporated into the snowpack is not a decisive process for the snow density profile at VRS. Otherwise, we would have expected a higher correlation between high wind speeds and precipitation for in situ data (containing the effect of wind drift) than for the ERA5 data.
However, the impact on density and increased sublimation due to snowdrift is implemented into Crocus and leads to distinctively lower snow depths in our simulations. Nevertheless, the parametrisation of increased sublimation during snowdrift requires more attention, which is beyond the scope of this paper.
Crocus reproduces well the observed onset of snow accumulation in our study, while melting starts earlier than observed. Reasons for the discrepancies in the timing and development of thawing could be a higher simulated thermal conductivity of the basal snow layer and a lower simulated thermal conductivity of the near-surface layers but also the ageing of snow.
Further, the measured snow depth is a point measurement. Already the measured snow depth in the detailed snow profiles close to the location of atmospheric measurements showed deviations due to spatial variability. Also other studies found differences of 0.45 m to more than 1 m in snow accumulation within a radius of a few kilometres (Domine et al., 2016a; Pedersen et al., 2016). Reasons for this include snow redistribution by wind, microtopographic relief and vegetation (Liston and Sturm, 2002; Barrere et al., 2017).
4.2.2 Simulated snow density and SSA profiles
We find considerable differences between measured density profiles and both CTRL simulations while Essery et al. (2016) found a strong correlation between simulated and observed snow density profiles (r=0.74). We see a constant increase in density with depth. This is typical for Alpine snowpacks, where dense basal layers are common while density in Arctic snowpacks usually decreases with depth (Domine et al., 2019).
Our simulations overestimate density for upper snow layers except at the surface and underestimate density for basal snow layers. This correspondents with results from other studies (Jacobi et al., 2010; Gascon et al., 2014; Barrere et al., 2017; Domine et al., 2019). Our results show clearly that an important process determining the snow stratigraphy in the Arctic is missing in Crocus. Barrere et al. (2017) and Jacobi et al. (2010) considered the main reason for the inverted density profiles in the lack of a parametrisation of upward water vapour transport due to strong temperature gradients occurring in the Arctic.
Moreover, the presumably too high precipitation in the in situ data, which was measured about one month before the measurements of the vertical snow profiles, is not visible in the snow depth measurements. In the in situ simulations, however, it leads to higher snow masses and consequently to increased compaction compared to the ERA5 density profile, which was forced by much lower precipitation.
A realistic simulation of snow density is crucial since there are strong functional relationships within the model parametrisations between individual variables. For example, snow density, SSA, thermal conductivity and temperature gradient are linked (Vionnet et al., 2012; Carmagnola et al., 2014; Domine et al., 2019). Thus, one incorrectly simulated variable affects the reliability of other physical snow variables (Domine et al., 2019). As density is a key variable, we anticipate that differences in simulated and measured densities can partly explain differences in simulated and measured SSA. SSA is parameterised in Crocus as an inverse function of the optical diameter (Carmagnola et al., 2014). Therefore, an inverted stratification of snow density leads to a lower SSA and thus to a lower albedo simulated with Crocus (Domine et al., 2019).
In addition, flaws in the forcing data can lead to erroneous simulated densities and SSA as visible in deviations in ERA5-CTRL and Insitu-CTRL in SSA (Figure 9). We find discrepancies between simulations of SSA and IceCube measurements, which is in agreement with Carmagnola et al. (2014). Strong temperature gradients in the Arctic’s snow layers not captured by Crocus (Domine et al., 2019) could partly explain differences in our simulated and measured SSA profiles.
5 Conclusion
Working with snow in the Arctic is challenging due to limited availability of measured snow data. Detailed snow models can help to overcome this problem and can assist to interpret the measurements but require carefully prepared forcing data to ensure high quality of simulated snow data. We can show that already relatively small deviations in solid precipitation, specific humidity and air temperature lead to considerable differences in simulated snow depth.
In situ atmospheric and snow measurements in the Arctic are rare, incomplete, and have to cope with difficult measurement conditions such as riming on the instruments and strong winds. However, models need complete time series to drive them. Here we present in situ atmospheric measurements for 2015 to 2018 and compare them with the modern atmospheric reanalysis ERA5 before driving the snow model Crocus with these datasets. We also analyse snow depth measurements (2014–2018) and detailed vertical density and SSA profiles of a campaign in spring 2018. These profiles show the typical stratification of Arctic snow and the influence of a recent warm air intrusion event with high wind speeds on the snow stratification.
Our study demonstrates that the reanalysis ERA5, except for precipitation, wind speed, and wind direction, agrees well with atmospheric measurements at VRS in northeast Greenland. ERA5 is also “physically consistent” because it is itself an output of a numerical atmospheric model. This does not necessarily apply for the observations due to measurement errors and co-location errors. At least partly this explains higher agreement of simulated and measured snow depth under ERA5 forcing than under in situ forcing, a result we had not expected.
Overall, our study shows that ERA5 is capable of replacing in situ measurements to force snow models where observations are limited, considering the highlighted systematic differences and limitations of in situ data and the reanalysis. We stress the importance of quality control of all in situ data in order to take advantage of observational data over reanalyses in modelling meaningful snow related quantities. Our study site is located in an area influenced by orography. We expect higher agreement of ERA5 and observations for flat areas as widely common in the Arctic such as over sea ice.
Concerning our research question, whether the snow model Crocus can reliably simulate snow depth evolution as well as snow density and SSA profiles, we cannot give a conclusive answer. Crocus can simulate the gross evolution of snow depth, but not of SSA and snow density of an Arctic snowpack sufficiently. Thereby the ERA5 simulation outperforms the in situ simulation at least with regard to the snow depth evolution.
Here, we present for the first time snow and atmospheric conditions for VRS and use ERA5 to force the snow model Crocus in the Arctic. Our study shows that Crocus has great potential for simulating snow conditions in the Arctic. The model can contribute to complement temporally and spatially limited observed snow depth measurements through representative simulations. Simulated and observed snow densities and SSA show deviations of which the user needs to be aware. Having that in mind, Crocus can give added value to the evolution and the range of the prevailing density and SSA.
In addition to the comparison carried out between ERA5 and measured atmospheric data and the performance of the snow model Crocus, we present measured snow and atmospheric conditions prevailing at one of the most northerly research stations. These analyses and measurements are rare this far north in the Arctic and therefore valuable to a wider community.
The available atmospheric time series used to assess the performance of ERA5 is from 27 November 2015 to 8 August 2018. Implications of this relatively short time period are relatively large uncertainties of the metrics used for the validation of this study. Using longer time series for comparison would provide more robust metrics of mean biases, RMSD and correlation coefficient between measurements and ERA5. Temporal variability and extreme events such as the warm air intrusion event in spring 2018 can lead to a deviating performance in such exceptional years compared to average years and distort the metrics.
Regarding the validation data used for snow simulations, validation was performed for a time series (26 August 2014 to 30 September 2018) for snow depth evolution, while single day measurements from spring 2018 were used for snow density and SSA. The limited single day measurements make it difficult to evaluate the performance of the model over the year and over different seasons. However, it can be shown that substantial model formulations are not suitable for snow simulations in the Arctic, as the spring snow profiles in 2018 show clear deviations from the simulated snow profiles. These discrepancies are the result of false simulated snowpack evolution from the beginning of the snow season onwards, which lead to the build-up of the spring snowpack, which we compare with measurements.
The atmospheric measurements and snow depth measurements at VRS are continued but quality controlled data were not available for this study. Also, in the near future revisits of the station for a prolongation of the snow density and specific surface area measurements are planned. These measurements should be used in future modelling studies for re-evaluation of our results.
Data availability statement
The raw data supporting the conclusion of this article will be made available by the authors, without undue reservation on request from the corresponding author.
Author contributions
DK carried out the analysis, performed the model simulations, drafted the manuscript and prepared the figures. FK helped with fruitful discussions of the analysis and designed the methodology of the sensitivity survey. All authors contributed to the editing of the manuscript. AH helped with the observational design of the study. FK and MD assisted with the numerical design. AH was leading the PAMARCMiP campaign.
Funding
CNRM/CEN is part of Labex OSUG@2020 (ANR-10-LABX-0056). MD has received funding from the European Research Council (ERC) under 20 the European Centre 2020 research and innovation programme (grant agreement No. 949516 and IVORI).
Acknowledgments
We gratefully acknowledge the funding by the Deutsche Forschungsgemeinschaft (DFG—German Research Foundation; project ID 268020496—TRR 172) within the Transregional Collaborative Research Center project of “ArctiC Amplification: Climate Relevant Atmospheric and SurfaCe Processes, and Feedback Mechanisms (AC)3”. We thank Marco Zanatta for the snow sampling during the campaign and Villum Research Station for general support. Special thanks go to Keld Mortensen and Andreas Massling for providing observational meteorological data. The authors acknowledge the cooperation and the productive scientific discussion with Christian Haas, Stefanie Arndt, Marco Zanatta and Olaf Eisen.
Conflict of interest
Author FK was employed by the company Ocean Atmosphere Systems GmbH.
The remaining authors declare that the research was conducted in the absence of any commercial or financial relationships that could be construed as a potential conflict of interest.
The reviewer DV declared a past collaboration with the author MD to the handling editor.
Publisher’s note
All claims expressed in this article are solely those of the authors and do not necessarily represent those of their affiliated organizations, or those of the publisher, the editors and the reviewers. Any product that may be evaluated in this article, or claim that may be made by its manufacturer, is not guaranteed or endorsed by the publisher.
Supplementary material
The Supplementary Material for this article can be found online at: https://www.frontiersin.org/articles/10.3389/feart.2023.1053918/full#supplementary-material
References
A2 Photonic Sensors (2016). A2 photonic sensors: IceCube: Innovative optical system for measurement of the specific surface area (SSA) of snow. Available at: http://www.a2photonicsensors.com/medias/A2PS_IceCube_EN.pdf.
Adler, R., Wang, J.-J., Sapiano, M., Huffman, G., Chiu, L., Xie, P. P., et al. (2016) Global precipitation climatology project (GPCP) climate data record (CDR), version 2.3 (monthly). National Centers for Environmental Information. doi:10.7289/V56971M6
ASIAQ (2014). Weather station at Station Nord technical documentation. Obtained by personal contact with Villum Research Station.
Barrere, M., Domine, F., Decharme, B., Morin, S., Vionnet, V., and Lafaysse, M. (2017). Evaluating the performance of coupled snow–soil models in SURFEXv8 to simulate the permafrost thermal regime at a high Arctic site. Geosci. Model Dev. 10, 3461–3479. doi:10.5194/gmd-10-3461-2017
Bartelt, P., and Lehning, M. (2002). A physical SNOWPACK model for the Swiss avalanche warning. Cold Regions Sci. Technol. 35, 123–145. doi:10.1016/S0165-232X(02)00074-5
Betts, A. K., Chan, D. Z., and Desjardins, R. L. (2019). Near-surface biases in ERA5 over the Canadian prairies. Front. Environ. Sci. 7, 129. doi:10.3389/fenvs.2019.00129
Boelman, N. T., Liston, G. E., Gurarie, E., Meddens, A. J. H., Mahoney, P. J., Kirchner, P. B., et al. (2019). Integrating snow science and wildlife ecology in Arctic-boreal North America. Environ. Res. Lett. 14, 010401. doi:10.1088/1748-9326/aaeec1
Boone, A., Masson, V., Meyers, T., and Noilhan, J. (2000). The influence of the inclusion of soil freezing on simulations by a soil–vegetation–atmosphere transfer scheme. J. Appl. Meteorology Climatol. 39, 1544–1569. doi:10.1175/1520-0450(2000)039<1544:tiotio>2.0.co;2
Box, J. E., Fettweis, X., Stroeve, J. C., Tedesco, M., Hall, D. K., and Steffen, K. (2012). Greenland ice sheet albedo feedback: Thermodynamics and atmospheric drivers. Cryosphere 6, 821–839. doi:10.5194/TC-6-821-2012
Brun, E., David, P., Sudul, M., and Brunot, G. (1992). A numerical model to simulate snow-cover stratigraphy for operational avalanche forecasting. J. Glaciol. 38, 13–22. doi:10.3189/S0022143000009552
Callaghan, T. V., Johansson, M., Brown, R. D., Groisman, P. Y., Labba, N., Radionov, V., et al. (2011). Multiple effects of changes in Arctic snow cover. AMBIO 40, 32–45. doi:10.1007/S13280-011-0213-X
Calonne, N., Richter, B., Löwe, H., Cetti, C., Schure, J. ter, van Herwijnen, A., et al. (2020). The RHOSSA campaign: Multi-resolution monitoring of the seasonal evolution of the structure and mechanical stability of an Alpine snowpack. Cryosphere 14, 1829–1848. doi:10.5194/tc-14-1829-2020
Carmagnola, C. M., Morin, S., Lafaysse, M., Domine, F., Lesaffre, B., Lejeune, Y., et al. (2014). Implementation and evaluation of prognostic representations of the optical diameter of snow in the SURFEX/ISBA-Crocus detailed snowpack model. Cryosphere 8, 417–437. doi:10.5194/tc-8-417-2014
Christensen, K. (2020). Measuring the impact of a new snow model using surface energy budget process relationships. Available at: https://villumresearchstation.dk/about-the-station.
Day, J. J., Arduini, G., Sandu, I., Magnusson, L., Beljaars, A., Balsamo, G., et al. (2020). Measuring the impact of a new snow model using surface energy budget process relationships. J. Adv. Model. Earth Syst. 12. doi:10.1029/2020MS002144
Delhasse, A., Kittel, C., Amory, C., Hofer, S., van As, D., Fausto, S. R., et al. (2020). Brief communication: Evaluation of the near-surface climate in ERA5 over the Greenland ice sheet. Cryosphere 14, 957–965. doi:10.5194/tc-14-957-2020
Domine, F., Barrere, M., and Morin, S. (2016b). The growth of shrubs on high Arctic tundra at Bylot Island: Impact on snow physical properties and permafrost thermal regime. Biogeosciences 13, 6471–6486. doi:10.5194/bg-13-6471-2016
Domine, F., Barrere, M., and Sarrazin, D. (2016a). Seasonal evolution of the effective thermal conductivity of the snow and the soil in high Arctic herb tundra at Bylot Island, Canada. Cryosphere 10, 2573–2588. doi:10.5194/tc-10-2573-2016
Domine, F., Gauthier, G., Vionnet, V., Fauteux, D., Dumont, M., and Barrere, M. (2018). Snow physical properties may be a significant determinant of lemming population dynamics in the high Arctic. Arct. Sci. 4, 813–826. doi:10.1139/as-2018-0008
Domine, F., Picard, G., Morin, S., Barrere, M., Madore, J.-B., and Langlois, A. (2019). Major issues in simulating some arctic snowpack properties using current detailed snow physics models: Consequences for the thermal regime and water budget of permafrost. J. Adv. Model. Earth Syst. 11, 34–44. doi:10.1029/2018MS001445
Essery, R., Kontu, A., Lemmetyinen, J., Dumont, M., and Ménard, C. B. (2016). A 7-year dataset for driving and evaluating snow models at an Arctic site (Sodankylä, Finland). Geosci. Instrum. Method. Data Syst. 5, 219–227. doi:10.5194/gi-5-219-2016
European Centre (2020). For medium-range weather forecasts (ECMWF): Fact sheet: Earth system data assimilation. Available at: https://www.ecmwf.int/en/about/media-centre/focus/2020/fact-sheet-earth-system-data-assimilation (Accessed March 13, 2023).
Gascon, G., Sharp, M., Burgess, D., Bezeau, P., Bush, A. B., Morin, S., et al. (2014). How well is firn densification represented by a physically based multilayer model? Model evaluation for Devon Ice Cap, Nunavut, Canada. J. Glaciol. 60, 694–704. doi:10.3189/2014JoG13J209
Gouttevin, I., Langer, M., Löwe, H., Boike, J., Proksch, M., and Schneebeli, M. (2018). Observation and modelling of snow at a polygonal tundra permafrost site: Spatial variability and thermal implications. Cryosphere 12, 3693–3717. doi:10.5194/tc-12-3693-2018
Gryning, S.-E., Batchvarova, E., Floors, R., Münkel, C., Skov, H., and Sørensen, L. L. (2021). Observed and modelled cloud cover up to 6 km height at Station Nord in the high Arctic. Intl J. Climatol. 41, 1584–1598. doi:10.1002/joc.6894
Hall, A. (2004). The role of surface albedo feedback in climate. J. Clim. 17, 1550–1568. doi:10.1175/1520-0442(2004)017<1550:TROSAF>2.0
Hersbach, H., Bell, B., Berrisford, P., Hirahara, S., Horányi, A., Muñoz-Sabater, J., et al. (2020). The ERA5 global reanalysis. Q. J. R. Meteorol. Soc. 146, 1999–2049. doi:10.1002/qj.3803
Howat, I. M., Negrete, A., and Smith, B. E. (2014). The Greenland Ice Mapping Project (GIMP) land classification and surface elevation data sets. Cryosphere 8, 1509–1518. doi:10.5194/tc-8-1509-2014
Howat, I., Negrete, A., and Smith, B. (2015). MEaSUREs Greenland ice mapping project (GIMP) digital elevation model, version 1. gimpdem5_5_v01.1. NASA Natl. Snow Ice Data Cent. Distributed Act. Archive Cent. 29, 1. doi:10.5067/NV34YUIXLP9W
Jacobi, H.-W., Domine, F., Simpson, W. R., Douglas, T. A., and Sturm, M. (2010). Simulation of the specific surface area of snow using a one-dimensional physical snowpack model: Implementation and evaluation for subarctic snow in Alaska. Cryosphere 4, 35–51. doi:10.5194/tc-4-35-2010
Krampe, D., Kauker, F., Dumont, M., and Herber, A. (2021). On the performance of the snow model Crocus driven by in situ and reanalysis data at Villum research station in northeast Greenland, the cryosphere discuss. [preprint]. doi:10.5194/tc-2021-100
King, J., Howell, S., Brady, M., Toose, P., Derksen, C., Haas, C., et al. (2020). Local-scale variability of snow density on Arctic sea ice. Cryosphere 14, 4323–4339. doi:10.5194/tc-14-4323-2020
Libois, Q., Picard, G., Dumont, M., Arnaud, L., Sergent, C., Pougatch, E., et al. (2014). Experimental determination of the absorption enhancement parameter of snow. J. Glaciol. 60 (222), 714–724. doi:10.3189/2014JoG14J015
Libois, Q., Picard, G., France, J. L., Arnaud, L., Dumont, M., Carmagnola, C. M., et al. (2013). Influence of grain shape on light penetration in snow. Cryosphere 7, 1803–1818. –1818. doi:10.5194/tc-7-1803-2013
Liston, G. E., and Elder, K. (2006). A distributed snow-evolution modeling system (SnowModel). J. Hydrometeor 7, 1259–1276. doi:10.1175/JHM548.1
Liston, G. E., Perham, C. J., Shideler, R. T., and Cheuvront, A. N. (2016). Modeling snowdrift habitat for polar bear dens. Ecol. Model. 320, 114–134. doi:10.1016/j.ecolmodel.2015.09.010
Liston, G. E., and Sturm, M. (2002). Winter precipitation patterns in arctic Alaska determined from a blowing-snow model and snow-depth observations. J. Hydrometeor 3, 646–659. doi:10.1175/1525-7541(2002)003<0646:WPPIAA>2.0
Loew, A., Bell, W., Brocca, L., Bulgin, C. E., Burdanowitz, J., Calbet, X., et al. (2017). Validation practices for satellite-based Earth observation data across communities. Rev. Geophys. 55, 779–817. doi:10.1002/2017RG000562
Luijting, H., Vikhamar-Schuler, D., Aspelien, T., Bakketun, Å., and Homleid, M. (2018). Forcing the SURFEX/Crocus snow model with combined hourly meteorological forecasts and gridded observations in southern Norway. Cryosphere 12, 2123–2145. doi:10.5194/tc-12-2123-2018
Maslanik, J., and Stroeve, J. (2018). Near-real-time DMSP SSMIS daily polar gridded Sea Ice concentrations, version 1 2018-03-15. Available: at: https://nsidc.org/data/NSIDC-0081/versions/1 (Accessed February 24, 2021).
Minola, L., Zhang, F., Azorin-Molina, C., Pirooz, A. A. S., Flay, R. G. J., Hersbach, H., et al. (2020). Near-surface mean and gust wind speeds in ERA5 across Sweden: Towards an improved gust parametrization Clim. Dyn. 55, 887–907. doi:10.1007/s00382-020-05302-6
Nakoudi, K., Ritter, C., Böckmann, C., Kunkel, D., Eppers, O., Rozanov, V., et al. (2020). Does the intra-arctic modification of long-range transported aerosol affect the local radiative budget? (A case study). Remote Sens. 12, 2112. doi:10.3390/rs12132112
Nguyen, Q. T., Skov, H., Sørensen, L. L., Jensen, B. J., Grube, A. G., Massling, A., et al. (2013). Source apportionment of particles at station nord, north east Greenland during 2008–2010 using COPREM and PMF analysis. Atmos. Chem. Phys. 13, 35–49. doi:10.5194/acp-13-35-2013
Nitu, R., Roulet, Y.-A., Wolff, M., Earle, M., Reverdin, A., Smith, C., et al. (2018). WMO solid precipitation intercomparison experiment. Geneva: SPICE.
Pedersen, S. H., Tamstorf, M. P., Abermann, J., Westergaard-Nielsen, A., Lund, M., Skov, K., et al. (2016). Spatiotemporal characteristics of seasonal snow cover in Northeast Greenland from in situ observations. Arct. Antarct. Alp. Res. 48, 653–671. doi:10.1657/AAAR0016-028
Proksch, M., Löwe, H., and Schneebeli, M. (2015). Density, specific surface area, and correlation length of snow measured by high-resolution penetrometry. J. Geophys. Res. Earth Surf. 120, 346–362. doi:10.1002/2014JF003266
Rasch, M., Frandsen, E. R., Skov, H., and Hansen, J. L. (2016).Site manual. Villum research station. Station Nord. Greenland. Available at: https://villumresearchstation.dk/fileadmin/villumresearchstation/Generelle/SITE_MANUAL.pdf (Accessed May 28, 2020).
Sauter, T., and Obleitner, F. (2015). Assessing the uncertainty of glacier mass-balance simulations in the European Arctic based on variance decomposition. Geosci. Model Dev. 8, 3911–3928. doi:10.5194/gmd-8-3911-2015
Schmidt, N. M., Ims, R. A., Høye, T. T., Gilg, O., Hansen, L. H., Hansen, J., et al. (2012). Response of an Arctic predator guild to collapsing lemming cycles. Proc. Biol. Sci. 279, 4417–4422. doi:10.1098/rspb.2012.1490
Schneebeli, M., Pielmeier, C., and Johnson, J. B. (1999). Measuring snow microstructure and hardness using a high resolution penetrometer. Cold Regions Sci. Technol. 30, 101–114. doi:10.1016/S0165-232X(99)00030-0
Stiegler, C., Lund, M., Christensen, T. R., Mastepanov, M., and Lindroth, A. (2016). Two years with extreme and little snowfall: Effects on energy partitioning and surface energy exchange in a high-Arctic tundra ecosystem. Cryosphere 10, 1395–1413. doi:10.5194/tc-10-1395-2016
Sturm, M., Holmgren, J., König, M., and Morris, K. (1997). The thermal conductivity of seasonal snow. J. Glaciol. 43, 26–41. doi:10.3189/S0022143000002781
Tuzet, F., Dumont, M., Lafaysse, M., Picard, G., Arnaud, L., Voisin, D., et al. (2017). A multilayer physically based snowpack model simulating direct and indirect radiative impacts of light-absorbing impurities in snow. Cryosphere 11, 2633–2653. doi:10.5194/tc-11-2633-2017
Tuzet, F., Dumont, M., Picard, G., Lamare, M., Voisin, D., Nabat, P., et al. (2020). Quantification of the radiative impact of light-absorbing particles during two contrasted snow seasons at Col du Lautaret (2058 m a.s.l., French Alps). Cryosphere 14, 4553–4579. doi:10.5194/tc-14-4553-2020
Uotila, P., Goosse, H., Haines, K., Chevallier, M., Barthélemy, A., Bricaud, C., et al. (2019). An assessment of ten ocean reanalyses in the polar regions. Clim. Dyn. 52, 1613–1650. doi:10.1007/s00382-018-4242-z
Villum Reserach Station (2021). Villum Reserach Station: Meteorological data, asiaqmet. Available at: https://www2.dmu.dk/asiaqmet/Default.aspx (Accessed February 5, 2021).
Vionnet, V., Brun, E., Morin, S., Boone, A., Faroux, S., Le Moigne, P., et al. (2012). The detailed snowpack scheme Crocus and its implementation in SURFEX v7.2. Model Dev. 5, 773–791. doi:10.5194/gmd-5-773-2012
Vionnet, V., Guyomarc’h, G., Naaim Bouvet, F., Martin, E., Durand, Y., Bellot, H., et al. (2013). Occurrence of blowing snow events at an Alpine site over a 10-year period: Observations and modelling. Adv. Water Resour. 55, 53–63. doi:10.1016/j.advwatres.2012.05.004
Wang, C., Graham, R. M., Wang, K., Gerland, S., and Granskog, M. A. (2019). Comparison of ERA5 and ERA-interim near-surface air temperature, snowfall and precipitation over Arctic sea ice: effects on sea ice thermodynamics and evolution. Cryosphere 13, 1661–1679. doi:10.5194/TC-13-1661-2019
Wang, Z. (2019). “The solar resource and meteorological parameters,” in Design of solar thermal power plants Z. Wang (Amsterdam, Netherlands: Elsevier), 47. doi:10.1016/B978-0-12-815613-1.00002-X
Yankee Environmental Systems (2012). TPS_3100 total precipitation sensor. Installation & user guide version 2 01.
Keywords: snow measurements, meteorological measurements, snow modelling, Crocus, ERA5, Arctic, PAMARCMiP
Citation: Krampe D, Kauker F, Dumont M and Herber A (2023) Snow and meteorological conditions at Villum Research Station, Northeast Greenland: on the adequacy of using atmospheric reanalysis for detailed snow simulations. Front. Earth Sci. 11:1053918. doi: 10.3389/feart.2023.1053918
Received: 26 September 2022; Accepted: 21 April 2023;
Published: 19 May 2023.
Edited by:
Satoru Yamaguchi, National Research Institute for Earth Science and Disaster Resilience (NIED), JapanReviewed by:
Francesco Avanzi, CIMA Research Foundation, ItalyDeborah Verfaillie, Centre Européen de Recherche et d’enseignement de Géosciences de l’environnement (CEREGE), France
Copyright © 2023 Krampe, Kauker, Dumont and Herber. This is an open-access article distributed under the terms of the Creative Commons Attribution License (CC BY). The use, distribution or reproduction in other forums is permitted, provided the original author(s) and the copyright owner(s) are credited and that the original publication in this journal is cited, in accordance with accepted academic practice. No use, distribution or reproduction is permitted which does not comply with these terms.
*Correspondence: Daniela Krampe, RGFuaWVsYS5LcmFtcGVAYXdpLmRl