Climate adjusted projections of the distribution and frequency of poor air quality days for the contiguous United States
- First Street Foundation, Brooklyn, NY, United States
Unhealthy air quality conditions can strongly affect long-term human health and wellbeing, yet many air quality data products focus on near real-time alerts or short-term forecasts. Understanding the full state of air quality also requires examining the longer term frequency and intensity of poor air quality at ground level, and how it might change over time. We present a new modeling framework to compute climate-adjusted estimates of air quality hazards for the contiguous United States (CONUS) at 10 km horizontal resolution. The framework blends results from statistical, machine-learning, and climate-chemistry models—including a bias-adjusted version of the EPA Community Multiscale Air Quality Model (CMAQ) time series as described in (Wilson et al., 2022)—for ground-level ozone, anthropogenic fine particulate matter (PM2.5), and wildfire smoke PM2.5 into consistent estimates of days exceeding the “unhealthy for sensitive groups” (orange colored) classification on the EPA Air Quality Index for 2023 and 2053. We find that joint PM2.5 and ozone orange+ days range from 1 day to 41 days across CONUS, with a median value of 2 days, across all years. Considering all properties across CONUS, we find that 63.5% percent are exposed to at least one orange or greater day in 2023, growing to 72.1% in 2053. For a 7-day threshold, 3.8% and 5.7% of properties are exposed in 2023 and 2053, respectively. Our results also support the identification of which parts of the country are most likely to be impacted by additional climate-related air quality risks. With growing evidence that even low levels of air pollution are harmful, these results are an important step forward in empowering individuals to understand their air quality risks both now and into the future.
1 Introduction
Air pollution poses a major threat to human wellbeing, with well documented adverse health effects (Pope et al., 2002; Schwartz et al., 2002; Bell et al., 2007; Shah et al., 2013; Kim et al., 2015; Karanasiou et al., 2021) and impacts to quality of life (Kim et al., 2020). The United States has made significant strides in curbing air pollution since the passing of the Clean Air Act in 1963, with the U.S. Environmental Protection Agency (EPA) leading the design and implementation of policies and standards for regulating air quality levels. Since the implementation of the Clean Air Act, the combined emissions of criteria pollutants and their precursors have dropped by 78% (US EPA, 2016). However, climate change threatens to reduce these gains by altering meteorology in ways that are either directly conducive to pollutant formation or indirectly conducive through secondary factors like wildfires (Spracklen et al., 2009; Val Martin et al., 2015; Cohen et al., 2017; Liu et al., 2021). This counteracting effect, often referred to as the “climate penalty”, threatens to offset planned emissions reduction strategies and make achieving rigorous air quality standards more difficult.
Ground-level ozone and fine particulate matter (PM2.5) have two of the stronger connections to climate change of any major pollutants. Ozone, which forms when nitrogen oxides and volatile organic compounds chemically interact with sunlight, is at least partially modulated by temperature, humidity, and vapor pressure deficit, among other factors (Mahmud et al., 2008; Nolte et al., 2008; Shen et al., 2016; Kavassalis and Murphy, 2017; Arnold et al., 2018; Wells et al., 2021), all of which are projected to shift under climate change. While there has been a significant decline in the national average ozone concentrations since 1980 (from 0.094 ppm in 1980 to 0.066 ppm in 2022; US EPA, 2016), estimates of the magnitude of the summer ozone climate penalty are on the order of 2–8 ppb by the mid-century, although estimates varying by season and climate scenario (Nolte et al., 2008; Weaver et al., 2009; Kelly et al., 2012; Trail et al., 2013; Pfister et al., 2014; Fann et al., 2015; He et al., 2016).The same meteorological variables also influence the production and transport of anthropogenic and biogenic PM2.5, which combined has been observed to decline by 5–8 μg/m3 since 2000 (from a national average of 13.53 μg/m3 in 2000 to 7.82 μg/m3 in 2022; US EPA, 2016). However, because particulate matter is typically directly emitted, models that find a strong climate connection for ozone often find less success for PM2.5 (Ryan, 2016). A few studies have shown small, but statistically significant effects of climate on PM2.5 on the order of 0.5–2.0 μg/m3, but broader research finds inconsistent trends (Tai et al., 2010; Kelly et al., 2012; Dawson et al., 2014; Day and Pandis, 2015; Fiore et al., 2015; Shen et al., 2017).
Conversely, increased wildfire activity—which currently represents approximately 15%–30% of PM2.5 concentrations in the United States—is likely to significantly impact PM2.5 production into the future (Jacob and Winner, 2009; Spracklen et al., 2009; Liu et al., 2021) and put at risk any improvements gained from controls on anthropogenic emissions. The importance of this point is underscored given the fact that wildfire is the fastest-growing natural disaster associated with climate change and is expected to continue to increase over the next 30 years (Kearns et al., 2022). While not a direct correlation, fire emission increases of 50% more by mid-century are projected for CONUS in multiple studies (Spracklen et al., 2009; Val Martin et al., 2015; Ford et al., 2018). Historically, an estimated 20%–25% of all PM2.5 events exceeding the 24-h national standard have occurred when wildfire smoke was present (Kaulfus et al., 2017). Long-range transport of PM2.5 via large-scale flow is known to substantially increase PM2.5 concentrations, sometimes by a factor of 2–3 (Mueller et al., 2020; Lin et al., 2021; Mardi et al., 2021). Local fires’ influence can dominate, with prescribed fire burning found to explain about 25% of the variance in overall PM2.5 concentrations in the southeast United States (Afrin and Garcia-Menendez, 2020), with small fires’ smoke dominating within 2 km of the source (Pearce et al., 2012). In severe fire years, wildfire smoke pollution is even more significant. One estimate found 41% of CONUS pollution in 2020 could be attributed solely to west coast fires (Lin et al., 2021). Another found an estimated 25% of air quality gains since at least 2016 have already been eroded by increases in wildfire-related PM2.5 concentrations (Burke et al., 2023).
Appropriately characterizing and communicating potential reversals in air quality progress is important for addressing public health and quality of life challenges across the United States. Ozone and particulate matter have many well documented associations with long and short-term adverse health effects, including chest pain, coughing, asthma, respiratory diseases and infections, and premature deaths (Bell et al., 2007; Kinney et al., 2008; Tagaris et al., 2009; Fann et al., 2015; Fann et al., 2021; Garcia-Menendez et al., 2015; Orru et al., 2017; Silva et al., 2017). Air pollution also more broadly has the potential to decrease happiness and satisfaction in life, increase many mental disorders, trigger behavioral responses, and hurt productivity (Lu, 2020). Currently, governments rely on individuals to protect themselves from poor air quality by issuing alerts and recommending people to stay indoors and wear masks. AirNow—a flagship EPA product—collates and applies rapid quality control to sensor observations across the United States. to provide near real-time estimation of the official Air Quality Index (AQI), a color-coded index focused on communicating whether air quality is healthy or unhealthy at a given point in time. Similarly, BreezoMeter (acquired by Google in late 2022) combines monitoring station and satellite data with real-time traffic information, meteorological conditions, European Union’s Copernicus Atmosphere Monitoring Service (CAMS) to provide both historic, real-time, and forecasted air quality. BreezoMeter data is widely consumed via an integration into the Apple Weather app on iOS devices. These alert-based approaches may have small benefits that are unequal across populations, as wealthy populations are more likely to take precautions during a bad air quality event (Burke et al., 2022).
Surprisingly, there is a dearth of data products aimed at providing a representative picture of typical air quality levels for any given location. Because poor air quality alerts can drive secondary effects like school cancellations, individuals might be interested in knowing the number of these alerts they might expect in a given year, and how that is expected to change into the future. Several organizations, including the EPA and the American Lung Association, do summarize official station data into yearly reports on air quality levels (American Lung Association, 2023), but face inherent limitations from the coarse geographic coverage of monitoring stations. Improving the spatial resolution of air quality summaries requires incorporating data from multiple sources, including climate chemistry, statistical, or machine learning modeling approaches, each coming with their own set of limitations. Here, we outline an approach to combine assessments of ozone and fine-particulate matter both presently and 30 years into the future to provide high resolution estimates of annual poor air quality days across the contiguous United States (CONUS).
The Community Multiscale Air Quality Model (CMAQ) results from EQUATES (EPA’s Air QUAlity TimE Series Project) form the foundation for current year projections of ozone and anthropogenic particulate matter (EPA, 2023). CMAQ is an open-source, state-of-the-art, suite of programs to simulate air quality by using the latest knowledge in atmospheric sciences and air quality modeling to produce concentrations of ozone, particulates, and more across spatially continuous layers (Appel et al., 2021). These results are supplemented with EPA air quality monitoring station observations for bias correction and wildfire-specific concentrations from a machine learning model from Childs et al. (2022). Ozone results are climate-adjusted using previously published methodology in Wilson et al. (2022), and smoke results are climate-adjusted using simulated fires’ output from the First Street Foundation Wildfire Model (Kearns et al., 2022). The integrated modeling approach provides a consistent set of projections for the number days for both the current conditions and for projected conditions 30 years into the future reaching an “unhealthy for sensitive groups” (orange colored) or above threshold on the EPA Air Quality Index.
2 Materials and methods
Our modeling framework was intentionally designed with a focus on days with “poor air quality” at ground level for common pollutants that have a demonstrated connection to climate change. Towards this goal, we built the present model with the following key decisions. First, we focused on the two most widespread pollutants under the National Ambient Air Quality Standards (NAAQS) with the strongest climate connection, PM2.5 and ozone. The Clean Air Act established NAAQS for six common “criteria” air pollutants with known health risks that include particle pollution (PM2.5 and PM10), ground-level ozone, sulfur dioxide, nitrogen dioxide, carbon monoxide, and lead. As of 2022, the population in counties where air quality levels exceeded the NAAQS was the greatest for particulate matter and ground-level ozone, with 78.3 million people living in counties where ozone levels exceeded NAAQS, 20.2 million people living in counties where PM2.5 exceeded the standard (US EPA, 2016). Second, we used an orange and above (henceforth orange+) classification on the EPA Air Quality Index (AQI) as the definition of a poor air quality day, or when air quality is at a level that starts to plausibly affect the health of a significant subset of the population. The EPA uses the AQI as a tool for communicating air quality levels from the criteria pollutants to the public. Each AQI breakpoint is assigned a color that is used in the EPA’s “Air Now” system and other public messaging around daily air quality safety levels. The six AQI categories and corresponding colors are as follows: good (green), moderate (yellow), unhealthy for sensitive groups (orange), unhealthy (red), very unhealthy (purple), and hazardous (maroon). The EPA provides general recommendations for activity levels under the AQI categories, which are broken out into the “general population” and “sensitive groups”. Sensitive groups refers to populations that may experience heightened symptoms from exposure to air pollutants, including but not limited to: people with cardiovascular or lung disease (including asthma and COPD), children and teenagers, older adults, new or expectant mothers, people with diabetes or obesity, and outdoor workers (US EPA, 2014).
Figure 1 shows a flowchart diagram of all modeling components and the general workflow. All modeling components were designed to produce independent predictions of annual orange+ days in both 2020s and 2050s climate conditions. We then integrated the results from each component into a joint result of orange+ days by adding the days from each component together. The following sections describe the individual modeling methodologies for ozone, wildfire smoke particulate matter, and anthropogenic particulate matter along with their corresponding climate projections, if relevant.
2.1 Ozone
To generate an annualized expected number of ozone exceedance days, we follow the ozone modeling methodology described in Wilson et al. (2022) that uses a point process extreme value model with CMAQ data and climate-projected temperature, relative humidity, and vapor pressure deficit as the meteorological controls on extreme ozone levels. Extreme Value Theory (EVT)is a frequently used technique for modeling ozone levels (Smith, 1989; Thompson, 2001; Rieder et al., 2010; Shen et al., 2016) and allows for estimating both the frequency and intensity of ozone exceedances. The methodology is summarized below, along with several minor changes.
2.1.1 Bias correction
To account for biases in the CMAQ time series (Appel et al., 2021), we merged station observations and CMAQ model output into a single data layer via a spatial regression model with time varying bias coefficients. These biases in CMAQ output, as discussed explicitly in (Appel et al., 2021), include a persistent underestimation of ozone across CONUS in springtime and in California throughout the year, as well as a tendency for CMAW to underestimate PM2.5 across the western and southern States. This merging approach is conceptually similar to the EPA fused data product methodology described in Berrocal et al. (2012), and serves the purpose of synthesizing the best available air quality information at a given location. For each daily time step, observed ozone concentrations were estimated using CMAQ concentrations and a spatially varying random field modeled with a Gaussian Random Field (GRF) process. To avoid propagating significant concentration changes in the merged product across areas bracketed by EPA stations but without any observations in between, we implemented an intermediate step that created “synthetic observational stations” representative of CMAQ output at approximately 100 km grid spacing, while removing any synthetic gridded stations within 50 km of real AQS stations. Each synthetic station was assigned the modeled concentration value from the corresponding CMAQ grid cell. This process results in a conservative spatial bias correction where AQS observations are favored in locations where they exist, otherwise the original CMAQ concentrations are kept as unaltered as possible. This scheme avoids edge cases where large discrepancies between modeled and observed results are propagated over long distances, while the modeled concentrations away from the observations should be considered to be consistent yet conservative estimates. The bias correction model was fitted using the INLA-SPDE approach in R-INLA (Rue et al., 2009; Lindgren et al., 2011) for every day in the EQUATES dataset between 2006 and 2019.
2.1.2 Current and future climate time series
The ozone component used a consistent set of daily meteorological covariates from the University of Idaho Gridded Surface Meteorological Dataset (GridMET), which are derived from a climatically aided interpolation process that blends gridded climate data and regional reanalysis datasets into a spatially and temporally complete set of surface meteorological variables (Abatzoglou, 2013). We used maximum temperature, minimum humidity, and vapor pressure deficit data for model fitting, consistent with the covariates recommended in Wilson et al. (2022). Daily observations were downloaded for the years 2006–2019 (over 350,000 observations per year; EPA-AQSweb) to match the ozone observations and resampled onto a 10 km grid using a weighted average of each covariate value.
We generated a mid-century (2046–2059) ozone weather time series by statistically adjusting the GridMET data to reflect the distribution changes between current and future conditions across an ensemble of downscaled SSP245 climate models produced by the NASA Earth Exchange (NASA-NEX) (Thrasher et al., 2022). We excluded the “hot models” (Hausfather et al., 2022) from the ensemble mean, leaving 11 GCMs in total. Because the NASA-NEX data only contained projected values for mean relative humidity, we derived minimum humidity and vapor pressure deficit using the Clausius Clapeyron equation to get a saturated vapor pressure at the maximum and minimum temperatures, assuming a vapor pressure derived from mean humidity data. Modified quantile and scaled distribution mapping bias correction techniques from the bias correction Python3 package were used to correct modeled projections by adjusting them with statistical scaling factors derived between current and future model output (Bai et al., 2016; Switanek et al., 2017). This process preserves the underlying variability in the current time series while shifting the overall trend towards a future climate. Supplementary Figure S1 shows the mean changes across temperature, humidity, and vapor pressure deficit between 2050s and 2020s climate conditions.
2.1.3 Point process model
Following the Poisson point process formulation from (Gilleland and Katz, 2016) and the recommendations in (Wilson et al., 2022), we define an extreme value theory model for ozone intensity (Λ) in Eq. 1 with location (μ), scale (σ), and shape parameters (ξ) on the time frame [t1, t2] as [0,1] and parametrize the “season” length as 365.25 days:
Conditional on the scale parameter being greater than zero, the Poisson rate parameter in Eq. 2 defines the frequency of exceeding a given threshold:
For model fitting, we selected a threshold equal to the 90th percentile value of ozone concentrations at each grid cell, respectively. We use the preferred model specification from Wilson et al. (2022), using a log transformed scale parameter and meteorological covariates and ‘year’ as covariates on the location parameter but not the scale parameter. All model fitting was completed using the maximum likelihood approach within the R package extRemes version 2.1–2 (Gilleland and Katz, 2016). Each grid cell was treated independently and the time series were declustered using an algorithm from (Coles et al., 2001) built into the extRemes package. Current and future predictions for annual orange+ days were estimated using Eq. 2 with a threshold set at 71 parts per billion (EPA-NAAQS) and the daily time series of current and future meteorological conditions, respectively. We fixed the value of the year covariate equal to the time frame of 2017–2019 to hold anthropogenic precursor emissions constant over time.
2.2 Wildfire smoke PM2.5
Wildfire emissions, along with other anthropogenic and biogenic emissions, are represented in EQUATES CMAQ modeled PM2.5 concentrations alongside ozone. However, there are known limitations in modeling wildfire smoke in climate chemistry models (Garcia-Menendez et al., 2014; Nikonovas et al., 2017), including differing wildfire emissions inventories (Koplitz et al., 2018), adequately capturing the influence of local meteorology on transport dynamics (Herron-Thorpe et al., 2014; Gunsch et al., 2018; Carter et al., 2020), and accurately modeling plume injection height (Paugam et al., 2016; Ye et al., 2021; Li et al., 2023). As a result, climate chemistry model output of wildfire PM2.5 can differ significantly from ground observations.
Therefore in our modeling approach, with a focus on proper attribution of wildfire PM2.5, the daily gridded wildfire smoke PM2.5 concentration dataset from Childs et al. (2022) is used in combination with simulated ELMFIRE fire emissions (Kearns et al., 2022; Melecio-Vázquez et al., 2023) to characterize current and future impacts to air quality conditions for CONUS due to PM2.5. The Childs et al. (2022) data, hereafter “Childs data”, which are openly available via Github, were produced using a machine learning (ML) model that combines ground, satellite, and reanalysis data sources to arrive at PM2.5 concentration estimates. The PM2.5 estimates were calculated from those data sources via the ML model on a 10 km grid across CONUS between the years of 2006 and 2020, and compared against observations from EPA monitoring stations (from over 130,000 to 500,000 observations per year across the 2006–2020 time series; EPA-AQSweb). The comparison produced an CONUS-average R2 of 0.67, with the strongest correlation evident in the Pacific Northwest and the weakest model performance in the desert Southwest. The Childs model performance did show significant spatial variation across CONUS, something which the authors partially attribute to spatially variable relationships between satellite AOD and ground PM2.5 due to land cover (particularly shrubland). Areas with less smoke PM2.5 variance and more variation in non-smoke PM2.5 were associated with the lowest performance.
To calculate annual orange+ days using the Childs data, we first applied a 5 pixel gaussian filter to the daily concentration data to smooth out coarse gridded artifacts in the source data. We then added a year-specific baseline anthropogenic concentration (described in Section 2.3) to the daily smoke PM2.5 estimates and summed the number of total number days above the 35.4 μg/m3 threshold per year. Adding in an average anthropogenic contribution accounts for the fact that the AQI is calculated off total PM2.5, not just the wildfire smoke contributing portion. We then summed the number of total number days above the 35.4 μg/m3 threshold per year and derived the average, average in non-zero smoke years, and maximum annual number of orange+ days across the entire time range. The “average in non-zero” and “maximum” years are a helpful additional characterizations in this context because wildfire time series are zero-inflated and orange+ days tend to be heavily concentrated in specific years with heightened fire activity. We use the average in non-zero year metric for the primary results in this paper, with the alternative versions included in the supplementary information.
2.2.1 Future wildfire smoke estimation
To estimate how air quality due to wildfire-driven PM2.5 may evolve with climate change in the future, our modeling framework leverages the output from First Street’s wildfire modeling effort. The wildfire modeling used a Monte Carlo simulation approach to drive a wildfire behavior model at 30 m horizontal using a 10yr NOAA surface weather record, adjusted USFS/DOI LANDFIRE fuel estimates, and historical ignition locations across CONUS, resulting in approximately 50 M simulated wildfires for 2023 (Kearns et al., 2022). Simulations were also conducted with the weather time series scaled to future atmospheric conditions using CMIP6 SSP245 outputs, resulting in a corresponding collection of approximately 50 M simulated wildfires for 2053. For each simulated wildfire, we retained information on the fuel-class-dependent mass output, fire size, and other statistics to estimate simulated smoke output from ELMFIRE. ELMFIRE’s combustion process estimates the total mass-released and the PM2.5 fractional yield is taken from the Fire Inventory from NCAR (Wiedinmyer et al., 2011). ELMFIRE runs at a sub-hourly time resolution for the emission rate and the results are aggregated to an average hourly rate, from which a sum of the hourly emission rates (at each hour) results in an estimate of the total mass-released for single fire (see full details in Melecio-Vazquez et al., 2023). Each individual fire’s PM2.5 emissions output is aggregated with others within 48 km bins, as defined by the underlying fire model’s computation scheme. Note that the results from Kearns et al. (2022) showed that more wildfires are likely across CONUS in the future under climate change, but not that those fires are likely to be larger or more intense than the current distribution of fires.
To model potential changes in poor air quality days at ground-level from the larger number of fires anticipated in the future across CONUS, we used a “change factor” approach that uses the ratio of future emissions to current emissions as a proxy for how much additional mass could expected be injected into the atmosphere due to those additional wildfires in the future. To calculate the change factor, we first used a threshold of 1000 acres for simulated fire size to filter out the many simulated small fires that are less likely to have a large effect on orange+ day occurrence. We then aggregated the remaining fires of size greater than 1000 acres in 48 km bins defined by the underlying fire model and calculated the average mass of PM2.5 released in current (2023) and future (2053) simulations (Supplementary Figure S2). In doing so, these average masses of emitted PM2.5 focus on local wildfire effects and do not incorporate any advection in or out of adjacent 48 km bins. This focus recognizes the aforementioned dominance of local fires on ground-level PM2.5 conditions (e.g. Pearce et al., 2012) and other studies such as (Jaffe et al., 2020) that have indicated that 80% of wildfires’ emissions are injected into the local atmospheric boundary layer instead of reaching higher layers at which advection over larger distances at high altitude may play a role in PM2.5 distribution. We then applied the ratio of future to current emissions as a multiplier to the daily PM2.5 wildfire concentrations from the Childs data to create a representative 2050s time series, and repeated the summation of orange+ AQI days to estimate the likely number of such days under future climate conditions.
2.3 Anthropogenic PM2.5
Our modeling approach incorporates anthropogenic PM2.5 estimates to provide a baseline expectation of poor air quality from non-wildfire related sources. To maintain consistency across model components, we used EQUATES CMAQ output as the basis for ground-surface-level anthropogenic PM2.5 estimates. However, because the EQUATES model output does not separate out wildfire related PM2.5 from other PM2.5 sources, we performed an additional processing routine to estimate wildfire-exclusive PM2.5 concentrations. We used this post-processed data to estimate purely anthropogenic orange+ days to add a baseline PM2.5 concentration for smoke orange+ day calculations without double counting smoke contributions.
Our processing routine is described as follows. First, we implemented a cap on daily PM2.5 concentrations at 1,400 μg/m3 (the highest single station observation recorded) to account for a known error in wildfire ignitions in CMAQ output. We then created a new daily concentration time series by combining CMAQ output with EPA station observations using the same fusion methodology described in Section 2.1.1 to reduce biases in underlying CMAQ model output. For first order smoke-removal, we matched the “smoke days” (non-zero values) in the Childs dataset to the CMAQ grid on a day/year per-pixel basis and excluded those days from the fused CMAQ output. The machine learning approach in the Childs data typically has a broader spatial representation of smoke due to the inclusion of satellite-based products like aerosol optical depth. Visual inspections showed that this step removed a majority of wildfire activity, but some clear fire activity remained (indicated by large, isolated spikes in PM2.5) due to methodological differences between the two data products. To account for this, we implemented a median filtering process to the daily data to remove large spikes in the CMAQ data that the Childs data did not capture. Using a window of 9 grid cells, we replaced a pixel’s PM2.5 value with the spatial median value if the difference between the two was more than 43.5 μg/m3 (the difference between a “green” and “red” AQI day). We used the processed data to calculate a yearly ‘smoke-exclusive’ mean PM2.5 concentration and the number of orange+ days for 2015–2019.
3 Results
Figure 2 shows the projected total number of orange+ days from ozone (a-b), wildfire smoke (c-d), and all sources (e-f) in 2023 and 2053. Results for the alternate smoke specifications are included in Supplementary Figures S3, S4. We find that joint PM2.5 and ozone orange+ days range from 1 to 41 across CONUS, with a median value of 2. Ozone and wildfire-related PM2.5 produce similar numbers of orange+ days at their respective maxima. At a high level, wildfire smoke is the predominant contributing pollutant on the west coast compared to ozone in the Midwest and on the East Coast. Central and Southern California are notable exceptions with consistently high values across both pollutants. Ozone orange+ days range from wildfire smoke are spread across the Western United States, with hotspots in Northern and Central California, the Idaho-Montana border, and the Washington-Canada border. Mean days in smoke years are non-zero across the entirety of most western states, ranging up to 5–10 days in fire-affected areas. Parts of the Mountain-west and Southeast show orange+ days from wildfire smoke, although typically only a few days. These spatial trends between 2023 and 2053 are consistent regardless of what wildfire smoke metric (mean, mean in non-zero years, max) is used, but the magnitude of smoke orange+ days is significantly higher in the maximum year (Supplementary Figure S1). Ozone orange+ days are spatially concentrated in California, the Great Lakes, and the Northeast Corridor, with isolated patches in places like Salt Lake City, the Greater Denver Region, and various cities in Texas. The magnitude of ozone orange+ days is typically 1-5, with the highest projected count at 27 days in Southern California. The spatial distributions associated with the future 2053 projections are strongly associated with areas of existing ozone pollution with the largest rates of increases associated with Ozone increases in California, the Great Plains, the Midwest, Tennessee Valley, and Northeastern regions of the country.
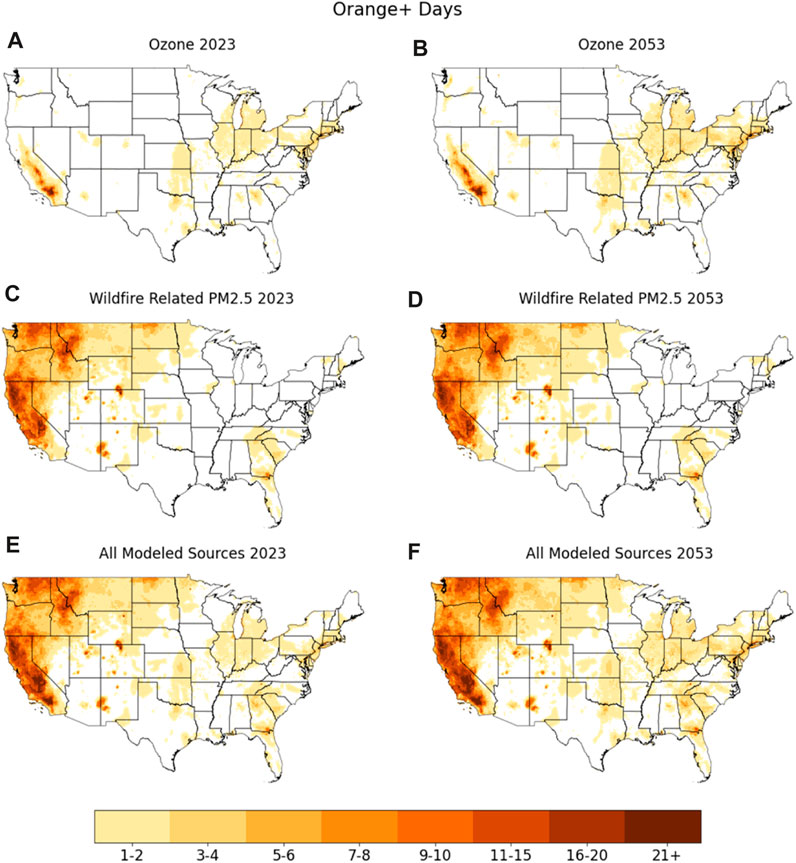
FIGURE 2. Estimated number of orange+ days in 2023 and 2053 conditions driven by ozone (A, B), wildfire smoke (C, D), and all modeled sources (E, F).
Our current year results validate moderately well against station observations. Figure 3 compares the model predicted number of orange+ days to EPA station derived orange+ days. To generate approximately comparable statistics, we selected stations with 75% of available yearly observations for a minimum of 3 years between 2014-2021. For PM2.5, our model results tend to be slight underestimates, with the exception of the western Coastline. For ozone, we show the opposite trend with slight overestimates in the East and underestimates in the West, especially in California. The largest differences (10+ days) occur in urban centers with ozone pollution (e.g. Denver, Salt Lake City, Los Angeles) and hotspots of activity for both ozone and wildfire PM2.5 like the central valley. These underestimations originate from the low bias in the underlying CMAQ datasets used for model training.
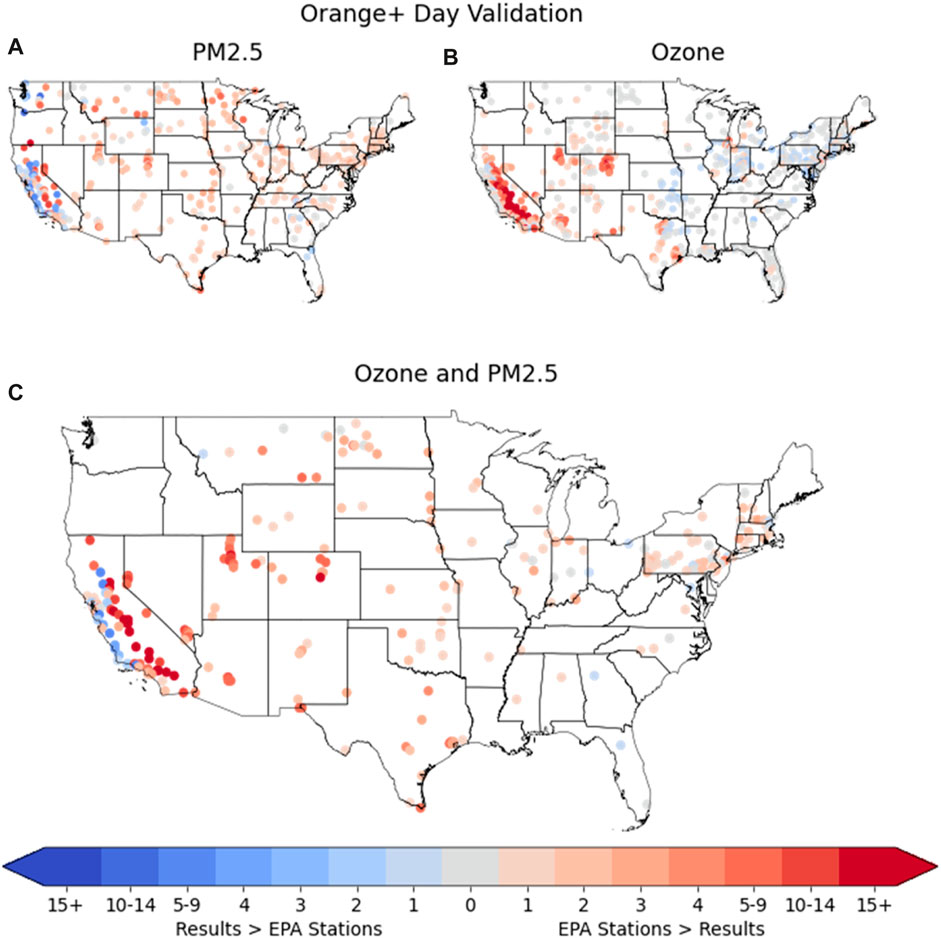
FIGURE 3. Comparison of model predictions versus 2014–2021 EPA station observations for Orange+ Days from (A) ozone, (B) PM2.5 and (C) both pollutants.
Figure 4 isolates the number of additional orange+ days resulting from climate change for ozone (24) and wildfire smoke (4b), respectively. We find the impact of climate change on orange+ days to be relatively modest, with increases of 1–5 days in most locations across both pollutants. For ozone, we find that nearly all our projected increases are in areas that already experience ozone pollution, consistent with the findings in Wilson et al. (2022). This suggests that changes in meteorological conditions into the 2050s are generally not significant enough on their own to create new areas of concern for ozone pollution. However, if anthropogenic emission of ozone precursors does not decrease in the future, climate change will exacerbate ozone pollution in areas with existing concern, indicating the magnitude of the ozone “climate penalty”. In our results this is seen in places like Central and Southern California—regions which are known to have some of the worst ozone pollution in the United States—projected to see increases of 10 orange+ days by mid-century. For wildfire smoke, we show increases 1–3 days across most of the western United States, with concentrated pockets of higher changes. Because the FSF-WFM resolves wildfire activity at very high horizontal resolution, increases in PM2.5 are more localized to areas with increased emissions. Our projected increases trend significantly higher in severe fire seasons, with an extra 10–14 days in many locations out west (Supplementary Figure S3).
In addition to the aforementioned comparisons of our results against observations, we also can observe the sensitivity of our results to concentration estimates that have been bias-adjusted and then compared against a threshold to arrive at annual orange+ exceedance day estimates. To guard against a systematic overestimation of the impacts of climate change on concentrations, we have taken a conservative approach which weights the observations appropriately but limits their spatial influence. The influence of the bias-adjustment procedure on results relative to a threshold concentration can be examined by considering a “worst case” scenario, for each day and for each pixel within the study domain, where the bias-adjusted estimates would result in an exceedance of the orange+ threshold—but the non-adjusted concentrations would not. This worst-possible influence for ozone is observed to range from only 0.25% of additional orange+ exceedance days annually, to under 0.02% additional days, for an average of 64 grid points per year for all of CONUS, which is negligible overall. The possible impact on annual orange+ days’ spatial distribution as derived from the isolation of the bias adjustment process for ozone and PM2.5 (bias-adjusted minus non-adjusted) is shown in Figure 5, and indicates that in the “worst case” the exceedance days would be coincident with those locations with the highest ozone concentrations. The bias-adjustment method previously described appears to be effectively emphasizing the impact of the actual observations while allowing the modeled results to provide coherent patterns of orange+ days distribution, without systematically over-extending or overestimating these relative to the ozone and PM2.5 thresholds. These patterns are similar in distribution and magnitude in both the 2023 and 2053 results.
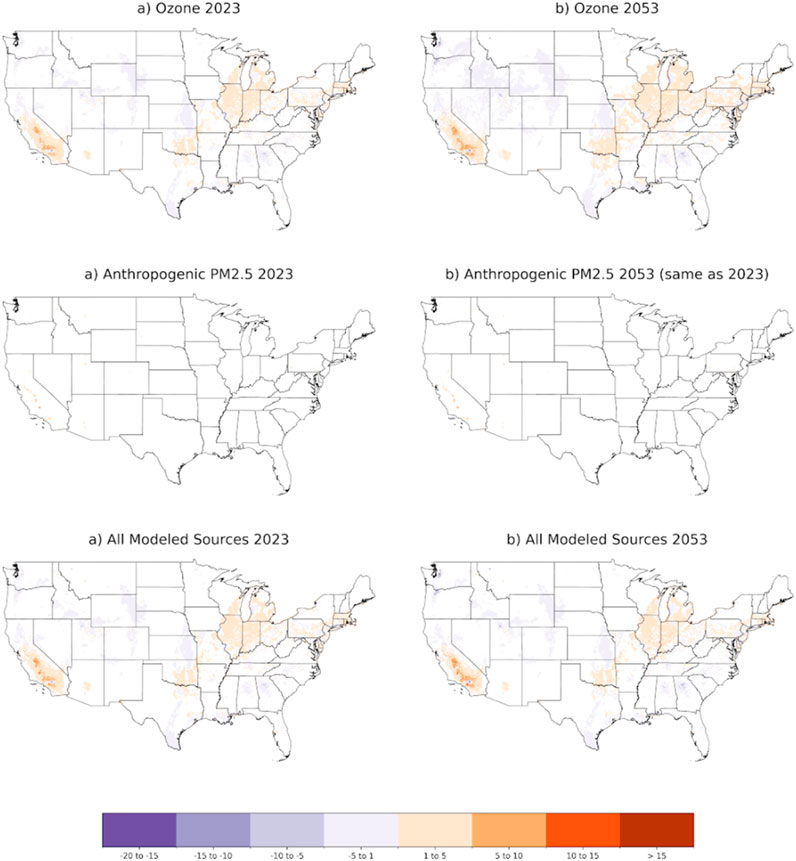
FIGURE 5. The difference in annual orange+ exceedance days of the bias-adjusted and non-biased adjusted ozone (top), PM2.5 (center), and combined (bottom) for 2023 (left) and 2053 (right).
To better understand how our projected additional orange+ days impact the United States population, we also calculated the property-level exposure using property data from the commercial data provider Lightbox, which has aggregated property and building level data from across the US from public sources including local tax assessor offices (https://www.lightboxre.com/). Figure 6 shows the total count of properties at the grid-cell level stratified by the number of orange+ days from all modeled sources in 2023 and 2053, and broken out by pollutant. Among all 140 million properties nationally, 91.5 million have at least one orange+ day in 2023, increasing to 103.7% in 2053. The number of properties experiencing each additional orange+ day monotonically decreases in both time periods, although a greater proportion of properties are in higher bins (3+) in the mid-century data. Consistent with current estimates of population under air quality nonattainment, we find that more properties experience orange+ days from ozone pollution rather than PM2.5 pollution. When considering risk by pollutant, more properties experience orange+ days due to Ozone exposure at the lower end of the “number of days” metric,. By contrast, PM2.5 is the predominant pollutant in areas that see a week (7+ days) of poor air quality day. This seems to indicate that there are two national pictures emerging, one in which a large amount of the country is exposed to high levels of Ozone, PM2.5, or both up to a handful of days a year; and another where a smaller portion of the country is exposed to high levels of PM2.5 driven predominately by wildfire smoke. While the properties in this most at risk area are proportionally very small, it is important to note that they are qualitatively different in regard to the characteristics of their exposure from the rest of the country.
4 Discussion
We presented an integrated estimate of unhealthy air quality days (orange+ on the EPA AQI) from ground-level ozone and fine particulate matter across the contiguous United States both presently and 30 years into the future under CMIP6 SSP245. Our work combined representative data sources from climate-chemistry, machine learning, and statistical models with climate modeling to produce estimates for orange+ (unhealthy for sensitive groups) AQI days across CONUS. Despite substantial progress in reducing air pollution in the U.S. through emission control strategies, we find that 64% of United States properties of the country experience poor air quality days currently, growing to 72% by midcentury. To our knowledge, this work represents the first large-scale integration of air quality models aimed at providing long-term, climate adjusted estimates of poor air quality days. With recent evidence suggesting that individuals alter their behavior in response to air quality events (Burke et al., 2022), this research hopefully enables individuals and communities to better understand their air quality risks both now and into the future.
We find that the “climate penalty” under simulated 2050s meteorological conditions is on the order of one or two additional poor air quality days at ground level for most of the United States, increasing up to a week or two in the most severe locations. These increases are consistent with previous literature on the magnitude of the ozone climate penalty (e.g. Shen et al., 2016) and are similar in magnitude to the increase in poor air quality days from wildfire smoke days over the past two decades (Burke et al., 2022; Childs et al., 2022). While these increases might appear modest, there is a growing body of evidence suggesting that even small increases in air pollution can negatively affect health outcomes, especially over longer time periods (Brauer et al., 2019; Brunekreef et al., 2021; Dominici et al., 2022). These results are particularly meaningful for the smaller proportion of areas and across CONUS that see 7+ orange+ days. For those properties, the increase in exposure is disproportionately driven by PM2.5 from wildfire smoke. Furthermore, some research indicates that current “acceptable” levels of air pollution are possibly too high, and adverse health effects from air pollution can be observed down to very low concentrations (Hoffmann et al., 2021). In 2021, this led the World Health Organization to ambitiously recommend lowering annual mean concentrations of PM2.5–5 μg/m3 and peak season MDA8 ozone concentrations to 60 μg/m3. Using these standards would result in more poor air quality days than we estimated in this study, underscoring the importance of characterizing any climate-related impacts to air quality.
Several recent studies have focused specifically on the impacts of wildfire smoke on counteracting progress in reducing anthropogenic PM2.5 levels in the United States (Burke et al., 2022; Burke et al., 2023; Childs et al., 2022). With results showing upwards of 25% of progress has been undone, it is important to understand how these trends might continue into the future. One of the challenges in translating this type of research to a high-resolution, climate-projected context are numerous uncertainties in coupled climate and chemical transport models (Garcia-Menendez et al., 2014; Nikonovas et al., 2017) that can lead to an underestimation of PM2.5 conditions. Therefore in this study, we focused on proper attribution of wildfire PM2.5 using the (Childs et al., 2022) dataset and ELMFIRE fire emissions (Kearns et al., 2022; Melecio-Vázquez et al., 2023) to characterize current and future impacts to surface air quality conditions for CONUS due to PM2.5. Using ELMFIRE fire emissions from the FSF-WFM anchors our analysis with a deterministic representation of simulated fire behavior rather than fire weather indices or other proxy measures. This provides a high resolution realistic representation of where fires have started and how they have spread across millions of Monte Carlo simulations under the same range of meteorological forcings, rather than just meteorological conditions alone that might be prone to more fire activity. Using this approach, we found that the doubling of wildfire risk is associated with an increase in average fire emissions of up to 50% across CONUS, translating to 1-5 additional orange+ days in an average smoke year and 10–20 orange+ days in a bad smoke year (Supplementary Figure S4). With similar increases in poor air quality days over the past two decades associated with up to 25% reductions in air quality progress (Burke et al., 2023), our results indicate that climate change is a significant barrier to achieving PM2.5 air quality goals in the future.
We note a few limitations to our future smoke estimates. The wildfire behavior model used (Lautenberger, 2013), cannot simulate the relatively rare large plume fires that have coupled atmospheric behaviors, so the very largest and severe fires are not captured in the simulation. Since each simulated wildfire is simulated independently, this approach also cannot resolve the increased correlated impact on air quality from groups of concurrent ignitions or “cluster fires”, such as the ones outside of Montreal, Canada that produced poor air quality across the central and eastern US in June 2023. The FSF-WFM is also geographically constrained to CONUS, so any changes to wildfire activity and corresponding smoke from Canadian, Alaskan, or Mexican wildfires is not captured in the future projections of the current version of our air quality model. Our future projections of increased air quality risk do not include any changes in any advected wildfire smoke contributions to PM2.5, and instead are confined to essentially local contributions. These limitations imply that our current projections should be considered as conservative estimates, particularly in those areas in the U.S. where large, advective smoke events are known to contribute to surface PM2.5 air quality. Finally, the FSF-WFM assumes no changes in the built environment, fire management practices, fire suppression resources, future wind patterns, or significant anthropogenically-influenced shifts in wildfire ignition locations into the 2050s in order to isolate the effect of climate change.
Our modeling framework holds all anthropogenic factors outside of those indirectly built into the shared socio-economic pathways data constant into the future to isolate the effect of climate change. While this approach is not uncommon in air quality literature, it is unlikely that emissions in the 2050s will be identical to those in the current period, especially if policies aimed at achieving broader climate goals are effectively reducing emissions. The trend for future expectations of PM2.5 is a decrease into the future as anthropogenic sources are better handled and reduced. However the portion that may be attributed to wildfires is expected to increase, potentially leading to 50% of all PM2.5 sources coming from wildfires (Val Martin et al., 2015; Ford et al., 2018) especially in western CONUS. This increase is expected to come mostly from increasing fires and fuel loadings, and minimally from changes in fuel moisture into the future (Liu et al., 2021). In our analysis, we found the smoke signal outweighs the anthropogenic PM2.5 signal for orange+ days in almost all areas, with the exception of major metro areas (e.g. Los Angeles). For ozone, reductions in precursor emissions or changes in local VOC-to-NOx ratios could temper the climate-related increases, but the relative impact compared to climate-related increases is uncertain. Understanding the relative contribution of policy-related improvements in air quality versus climate-related reductions is an important area of continued research.
5 Conclusion
Over the last half-century, a tremendous amount of improvement has been made in the reduction of harmful anthropogenically-sourced pollutants. In fact, per the EPA (EPA-Trends, 2021), the combined emissions of criteria pollutants and their precursors have dropped by 78% since 1970. This trend provides clear empirical evidence that the Clean Air Act, and other policies geared towards the reduction of harmful air pollutants, have been effective. Furthermore, those reductions have occurred across a number of different pollutants, but have generally all had the positive benefit of reducing various health risks. The outcome has been a healthier environment, less pollutant exposure for the population, and an overall improvement in quality of life across a number of different associated dimensions. The implications of these improvements are exceedingly important as exposure to high levels of Ozone and PM2.5 have been consistently shown to be linked to lower levels of physical and mental health, at even low levels of occurrence (Bell et al., 2007; Kinney et al., 2008; Tagaris et al., 2009; Fann et al., 2015; Fann et al., 2021; Garcia-Menendez et al., 2015; Orru et al., 2017; Silva et al., 2017).
Unfortunately for US communities, recent changes in the environment are also driving increases in some pollutants and improvements made under the Clean Air Act are coming undone per the “climate penalty” (Rasmussen et al., 2013; Burke et al., 2023). While the country is still less exposed to high levels of Ozone and PM2.5, a reversal at the national level is worrisome and worth the attention of scientists and policymakers. Moreover, this research shows that while the average “climate penalty” across the country is relatively small, in some areas the reversal is much more dramatic. Those areas are also seeing the most impacts of the role the changing climate is having in driving the “climate penalty” with direct exposure in increasing levels of extreme heat (and associated conditions) driving up exposure to Ozone and increasing rates of wildfire (and associated conditions) driving up exposure to PM2.5. The carry-on effects of this hyperlocal exposure also bring with them potential for larger scale socioeconomic consequences. In these localized areas, there are likely to be spikes in the negative physical and mental health implications that we know are associated with exposure to poor air quality. Additionally, we are already seeing residents leave areas associated with high rates of exposure to environmental conditions that directly impact “quality of life”. In coastal Florida for instance, research has shown that areas with high exposure to nuisance tidal flooding are growing at slower rates and seeing negative property value impacts (McAlpine and Porter, 2018; Gourevitch et al., 2023). Nationwide the availability of climate change impact data have been shown to influence the home buying process for specific homes that have been assessed to have high climate risks (Fairweather et al., 2023). These trends indicate that people respond to increasing climate risk, and the awareness of that risk, once exposure reaches a tipping point that negatively impacts their quality of life. The national model documented throughout this research allows for the identification of the areas most likely to be impacted by increasing exposure to pollutants and what parts of the country are most at risk for seeing the negative health, economic, and quality of life impacts that come with that increasing exposure.
Data availability statement
The original contributions presented in the study are included in the article/Supplementary Material, further inquiries can be directed to the corresponding author.
Author contributions
BW: Conceptualization, Formal Analysis, Methodology, Software, Validation, Writing–original draft, Writing–review and editing. MP: Conceptualization, Formal Analysis, Methodology, Software, Validation, Writing–original draft. DM-V: Conceptualization, Formal Analysis, Methodology, Software, Validation, Writing–original draft. HH: Software, Validation, Writing–original draft. MA: Software, Validation, Writing–original draft. ES: Writing–original draft, Writing–review and editing. JP: Writing–original draft, Writing–review and editing. EK: Conceptualization, Methodology, Writing–original draft, Writing–review and editing.
Funding
The author(s) declare financial support was received for the research, authorship, and/or publication of this article. This research received no external funding, and was entirely supported by the philanthropic donors to the First Street Foundation.
Acknowledgments
This research leans heavily on previously published datasets. We’d like to thank Marissa Childs and her co-authors for her generosity in making the Childs et al. (2022) data publicly available, and the Pyrgence Consortium including Chris Lautenberger for the simulated wildfire data from Kearns et al. (2022). We’d also like to thank Dr. Daniel Tong, Dr. Emma Knowland, and Dr. Bryan Duncan for their helpful feedback during the model development process.
Conflict of interest
The authors declare that the research was conducted in the absence of any commercial or financial relationships that could be construed as a potential conflict of interest.
Publisher’s note
All claims expressed in this article are solely those of the authors and do not necessarily represent those of their affiliated organizations, or those of the publisher, the editors and the reviewers. Any product that may be evaluated in this article, or claim that may be made by its manufacturer, is not guaranteed or endorsed by the publisher.
Supplementary material
The Supplementary Material for this article can be found online at: https://www.frontiersin.org/articles/10.3389/feart.2024.1320170/full#supplementary-material
References
Abatzoglou, J. T. (2013). Development of gridded surface meteorological data for ecological applications and modelling. Int. J. Climatol. 33, 121–131. doi:10.1002/joc.3413
Achakulwisut, P., Mickley, L. J., and Anenberg, S. C. (2018). Drought-sensitivity of fine dust in the US Southwest: implications for air quality and public health under future climate change. Environ. Res. Lett. 13, 054025. doi:10.1088/1748-9326/aabf20
Afrin, S., and Garcia-Menendez, F. (2020). The influence of prescribed fire on fine particulate matter pollution in the southeastern United States. Geophys. Res. Lett. 47, e2020GL088988. doi:10.1029/2020GL088988
American Lung Association (2023). State of the air. Available at: https://www.lung.org/getmedia/338b0c3c-6bf8-480f-9e6e-b93868c6c476/SOTA-2023.pdf (Accessed October 9, 2023).
Appel, K. W., Bash, J. O., Fahey, K. M., Foley, K. M., Gilliam, R. C., Hogrefe, C., et al. (2021). The Community Multiscale Air Quality (CMAQ) model versions 5.3 and 5.3.1: system updates and evaluation. Geosci. Model. Dev. 14, 2867–2897. doi:10.5194/gmd-14-2867-2021
Arnold, S. R., Lombardozzi, D., Lamarque, J.-F., Richardson, T., Emmons, L. K., Tilmes, S., et al. (2018). Simulated global climate response to tropospheric ozone-induced changes in plant transpiration. Geophys. Res. Lett. 45, 13070–13079. doi:10.1029/2018GL079938
Bai, K., Chang, N.-B., Yu, H., and Gao, W. (2016). Statistical bias correction for creating coherent total ozone record from OMI and OMPS observations. Remote Sens. Environ. 182, 150–168. doi:10.1016/j.rse.2016.05.007
Bell, M. L., Goldberg, R., Hogrefe, C., Kinney, P. L., Knowlton, K., Lynn, B., et al. (2007). Climate change, ambient ozone, and health in 50 US cities. Clim. Change 82, 61–76. doi:10.1007/s10584-006-9166-7
Brauer, M., Brook, J. R., Christidis, T., Chu, Y., Crouse, D. L., Erickson, A., et al. (2019). Mortality–air pollution associations in low-exposure environments (MAPLE): phase 1. Res. Rep. Health Eff. Inst., 203.
Brunekreef, B., Strak, M., Chen, J., Andersen, Z. J., Atkinson, R., Bauwelinck, M., et al. (2021). Mortality and morbidity effects of long-term exposure to low-level PM2.5, BC, NO2, and O3: an analysis of European cohorts in the ELAPSE Project. Res. Rep. Health Eff. Inst. 2021, 1–127.
Burke, M., Childs, M. L., de la Cuesta, B., Qiu, M., Li, J., Gould, C. F., et al. (2023). The contribution of wildfire to PM2.5 trends in the USA. Nature 622, 761–766. doi:10.1038/s41586-023-06522-6
Burke, M., Heft-Neal, S., Li, J., Driscoll, A., Baylis, P., Stigler, M., et al. (2022). Exposures and behavioural responses to wildfire smoke. Nat. Hum. Behav. 6, 1351–1361. doi:10.1038/s41562-022-01396-6
Carter, T. S., Heald, C. L., Jimenez, J. L., Campuzano-Jost, P., Kondo, Y., Moteki, N., et al. (2020). How emissions uncertainty influences the distribution and radiative impacts of smoke from fires in North America. Atmos. Chem. Phys. 20, 2073–2097. doi:10.5194/acp-20-2073-2020
Childs, M. L., Li, J., Wen, J., Heft-Neal, S., Driscoll, A., Wang, S., et al. (2022). Daily local-level estimates of ambient wildfire smoke PM 2.5 for the contiguous US. Environ. Sci. Technol. 56, 13607–13621. doi:10.1021/acs.est.2c02934
Cohen, A. J., Brauer, M., Burnett, R., Anderson, H. R., Frostad, J., Estep, K., et al. (2017). Estimates and 25-year trends of the global burden of disease attributable to ambient air pollution: an analysis of data from the Global Burden of Diseases Study 2015. Lancet 389, 1907–1918. doi:10.1016/S0140-6736(17)30505-6
Coles, S., Bawa, J., Trenner, L., and Dorazio, P. (2001). An introduction to statistical modeling of extreme values. Cham: Springer.
Dawson, J. P., Bloomer, B. J., Winner, D. A., and Weaver, C. P. (2014). Understanding the meteorological drivers of U.S. Particulate matter concentrations in a changing climate. Bull. Am. Meteorological Soc. 95, 521–532. doi:10.1175/BAMS-D-12-00181.1
Day, M. C., and Pandis, S. N. (2015). Effects of a changing climate on summertime fine particulate matter levels in the eastern U.S. J. Geophys. Res. Atmos. 120, 5706–5720. doi:10.1002/2014JD022889
Dominici, F., Zanobetti, A., Schwartz, J., Braun, D., Sabath, B., and Wu, X. (2022). Assessing adverse health effects of long-term exposure to low levels of ambient air pollution: implementation of causal inference methods. Res. Rep. Health Eff. Inst. 2022, 1–56.
EPA-Trends (2021). Our nation’s air trends through. Available at: https://gispub.epa.gov/air/trendsreport/2022/#home (Accessed January 26, 2024).
Fairweather, D., Kahn, M. E., Metcalfe, R. D., and Sandoval-Olascoaga, S. (2023). PRELIMINARY: the impact of climate risk disclosure on housing search and buying dynamics: evidence from a nationwide field experiment with redfin.
Fann, N., Nolte, C. G., Dolwick, P., Spero, T. L., Brown, A. C., Phillips, S., et al. (2015). The geographic distribution and economic value of climate change-related ozone health impacts in the United States in 2030. J. Air & Waste Manag. Assoc. 65, 570–580. doi:10.1080/10962247.2014.996270
Fann, N. L., Nolte, C. G., Sarofim, M. C., Martinich, J., and Nassikas, N. J. (2021). Associations between simulated future changes in climate, air quality, and human health. JAMA Netw. Open 4, e2032064. doi:10.1001/jamanetworkopen.2020.32064
Fiore, A. M., Naik, V., and Leibensperger, E. M. (2015). Air quality and climate connections. J. Air & Waste Manag. Assoc. 65, 645–685. doi:10.1080/10962247.2015.1040526
Ford, B., Val Martin, M., Zelasky, S. E., Fischer, E. V., Anenberg, S. C., Heald, C. L., et al. (2018). Future fire impacts on smoke concentrations, visibility, and health in the contiguous United States. GeoHealth 2, 229–247. doi:10.1029/2018GH000144
Garcia-Menendez, F., Hu, Y., and Odman, M. T. (2014). Simulating smoke transport from wildland fires with a regional-scale air quality model: sensitivity to spatiotemporal allocation of fire emissions. Sci. Total Environ. 493, 544–553. doi:10.1016/j.scitotenv.2014.05.108
Garcia-Menendez, F., Saari, R. K., Monier, E., and Selin, N. E. (2015). U.S. Air quality and health benefits from avoided climate change under greenhouse gas mitigation. Environ. Sci. Technol. 49, 7580–7588. doi:10.1021/acs.est.5b01324
Gilleland, E., and Katz, R. W. (2016). extRemes 2.0: an extreme value analysis package in R. J. Stat. Soft. 72. doi:10.18637/jss.v072.i08
Gourevitch, J. D., Kousky, C., Liao, Y. (P. ), Nolte, C., Pollack, A. B., Porter, J. R., et al. (2023). Unpriced climate risk and the potential consequences of overvaluation in US housing markets. Nat. Clim. Chang. 13, 250–257. doi:10.1038/s41558-023-01594-8
Gunsch, M. J., May, N. W., Wen, M., Bottenus, C. L. H., Gardner, D. J., VanReken, T. M., et al. (2018). Ubiquitous influence of wildfire emissions and secondary organic aerosol on summertime atmospheric aerosol in the forested Great Lakes region. Atmos. Chem. Phys. 18, 3701–3715. doi:10.5194/acp-18-3701-2018
Hausfather, Z., Marvel, K., Schmidt, G. A., Nielsen-Gammon, J. W., and Zelinka, M. (2022). Climate simulations: recognize the ‘hot model’problem. Nature 605, 26–29. doi:10.1038/d41586-022-01192-2
He, H., Liang, X.-Z., Lei, H., and Wuebbles, D. J. (2016). Future U.S. ozone projections dependence on regional emissions, climate change, long-range transport and differences in modeling design. Atmos. Environ. 128, 124–133. doi:10.1016/j.atmosenv.2015.12.064
Herron-Thorpe, F. L., Mount, G. H., Emmons, L. K., Lamb, B. K., Jaffe, D. A., Wigder, N. L., et al. (2014). Air quality simulations of wildfires in the Pacific Northwest evaluated with surface and satellite observations during the summers of 2007 and 2008. Atmos. Chem. Phys. 14, 12533–12551. doi:10.5194/acp-14-12533-2014
Hoffmann, B., Boogaard, H., de Nazelle, A., Andersen, Z. J., Abramson, M., Brauer, M., et al. (2021). WHO air quality guidelines 2021–aiming for healthier air for all: a joint statement by medical, public health, scientific societies and patient representative organisations. Int. J. Public Health 66, 1604465. doi:10.3389/ijph.2021.1604465
Jacob, D. J., and Winner, D. A. (2009). Effect of climate change on air quality. Atmos. Environ. 43, 51–63. doi:10.1016/j.atmosenv.2008.09.051
Jaffe, D. A., O’Neill, S. M., Larkin, N. K., Holder, A. L., Peterson, D. L., Halofsky, J. E., et al. (2020). Wildfire and prescribed burning impacts on air quality in the United States. J. Air & Waste Manag. Assoc. 70, 583–615. doi:10.1080/10962247.2020.1749731
Karanasiou, A., Alastuey, A., Amato, F., Renzi, M., Stafoggia, M., Tobias, A., et al. (2021). Short-term health effects from outdoor exposure to biomass burning emissions: a review. Sci. Total Environ. 781, 146739. doi:10.1016/j.scitotenv.2021.146739
Kaulfus, A. S., Nair, U., Jaffe, D., Christopher, S. A., and Goodrick, S. (2017). Biomass burning smoke climatology of the United States: implications for particulate matter air quality. Environ. Sci. Technol. 51, 11731–11741. doi:10.1021/acs.est.7b03292
Kavassalis, S. C., and Murphy, J. G. (2017). Understanding ozone-meteorology correlations: a role for dry deposition. Geophys. Res. Lett. 44, 2922–2931. doi:10.1002/2016GL071791
Kearns, E. J., Saah, D., Levine, C. R., Lautenberger, C., Doherty, O. M., Porter, J. R., et al. (2022). The construction of probabilistic wildfire risk estimates for individual real estate parcels for the contiguous United States. Fire 5, 117. doi:10.3390/fire5040117
Kelly, J., Makar, P. A., and Plummer, D. A. (2012). Projections of mid-century summer air-quality for North America: effects of changes in climate and precursor emissions. Atmos. Chem. Phys. 12, 5367–5390. doi:10.5194/acp-12-5367-2012
Kim, H., Kim, W.-H., Kim, Y.-Y., and Park, H.-Y. (2020). Air pollution and central nervous system disease: a review of the impact of fine particulate matter on neurological disorders. Front. Public Health 8, 575330. doi:10.3389/fpubh.2020.575330
Kim, K.-H., Kabir, E., and Kabir, S. (2015). A review on the human health impact of airborne particulate matter. Environ. Int. 74, 136–143. doi:10.1016/j.envint.2014.10.005
Kinney, P. L., O’Neill, M. S., Bell, M. L., and Schwartz, J. (2008). Approaches for estimating effects of climate change on heat-related deaths: challenges and opportunities. Environ. Sci. Policy 11, 87–96. doi:10.1016/j.envsci.2007.08.001
Lautenberger, C. (2013). Wildland fire modeling with an Eulerian level set method and automated calibration. Fire Saf. J. 62, 289–298. doi:10.1016/j.firesaf.2013.08.014
Li, Y., Tong, D., Ma, S., Freitas, S. R., Ahmadov, R., Sofiev, M., et al. (2023). Impacts of estimated plume rise on PM2.5 exceedance prediction during extreme wildfire events: a comparison of three schemes (Briggs, Freitas, and Sofiev). Atmos. Chem. Phys. 23, 3083–3101. doi:10.5194/acp-23-3083-2023
Lin, C.-A., Lu, C.-H., Chen, S.-P., Hung, W.-T., Civerolo, K. L., and Rattigan, O. V. (2021). Characterization of intra-continental smoke transport and impact on New York State air quality using aerosol reanalysis and multi-platform observations. Atmos. Pollut. Res. 12, 154–166. doi:10.1016/j.apr.2021.01.021
Lindgren, F., Rue, H., and Lindström, J. (2011). An explicit link between Gaussian fields and Gaussian Markov random fields: the stochastic partial differential equation approach. J. R. Stat. Soc. Ser. B Stat. Methodol. 73, 423–498. doi:10.1111/j.1467-9868.2011.00777.x
Liu, Y., Liu, Y., Fu, J., Yang, C.-E., Dong, X., Tian, H., et al. (2021). Projection of future wildfire emissions in western USA under climate change: contributions from changes in wildfire, fuel loading and fuel moisture. Int. J. Wildland Fire 31, 1–13. doi:10.1071/WF20190
Lu, J. G. (2020). Air pollution: a systematic review of its psychological, economic, and social effects. Curr. Opin. Psychol. 32, 52–65. doi:10.1016/j.copsyc.2019.06.024
Mahmud, A., Tyree, M., Cayan, D., Motallebi, N., and Kleeman, M. J. (2008). Statistical downscaling of climate change impacts on ozone concentrations in California. J. Geophys. Res. 113, D21103. doi:10.1029/2007JD009534
Mardi, A. H., Dadashazar, H., Painemal, D., Shingler, T., Seaman, S. T., Fenn, M. A., et al. (2021). Biomass burning over the United States East coast and western north atlantic ocean: implications for clouds and air quality. JGR Atmos. 126, e2021JD034916. doi:10.1029/2021JD034916
McAlpine, S. A., and Porter, J. R. (2018). Estimating recent local impacts of sea-level rise on current real-estate losses: a housing market case study in miami-dade, Florida. Popul. Res. Policy Rev. 37, 871–895. doi:10.1007/s11113-018-9473-5
Melecio-Vázquez, D., Lautenberger, C., Hsieh, H., Amodeo, M., Porter, J. R., Wilson, B., et al. (2023). A coupled wildfire-emission and dispersion framework for probabilistic PM2.5 estimation. Fire 6, 220. doi:10.3390/fire6060220
Mueller, S., Tarnay, L., O’Neill, S., and Raffuse, S. (2020). Apportioning smoke impacts of 2018 wildfires on eastern sierra Nevada sites. Atmosphere 11, 970. doi:10.3390/atmos11090970
Nikonovas, T., North, P. R. J., and Doerr, S. H. (2017). Particulate emissions from large North American wildfires estimated using a new top-down method. Atmos. Chem. Phys. 17, 6423–6438. doi:10.5194/acp-17-6423-2017
Nolte, C. G., Gilliland, A. B., Hogrefe, C., and Mickley, L. J. (2008). Linking global to regional models to assess future climate impacts on surface ozone levels in the United States. J. Geophys. Res. 113, D14307. doi:10.1029/2007JD008497
Orru, H., Ebi, K. L., and Forsberg, B. (2017). The interplay of climate change and air pollution on health. Curr. Envir Health Rpt 4, 504–513. doi:10.1007/s40572-017-0168-6
Paugam, R., Wooster, M., Freitas, S., and Val Martin, M. (2016). A review of approaches to estimate wildfire plume injection height within large-scale atmospheric chemical transport models. Atmos. Chem. Phys. 16, 907–925. doi:10.5194/acp-16-907-2016
Pearce, J. L., Rathbun, S., Achtemeier, G., and Naeher, L. P. (2012). Effect of distance, meteorology, and burn attributes on ground-level particulate matter emissions from prescribed fires. Atmos. Environ. 56, 203–211. doi:10.1016/j.atmosenv.2012.02.056
Pfister, G. G., Walters, S., Lamarque, J.-F., Fast, J., Barth, M. C., Wong, J., et al. (2014). Projections of future summertime ozone over the U.S. J. Geophys. Res. Atmos. 119, 5559–5582. doi:10.1002/2013JD020932
Pope, C. A., Burnett, R. T., Thun, M. J., Calle, E. E., Krewski, D., Ito, K., et al. (2002). Lung cancer, cardiopulmonary mortality, and long-term exposure to fine particulate air pollution. JAMA 287, 1132. doi:10.1001/jama.287.9.1132
Rieder, H. E., Staehelin, J., Maeder, J. A., Peter, T., Ribatet, M., Davison, A. C., et al. (2010). Extreme events in total ozone over Arosa – Part 1: application of extreme value theory. Atmos. Chem. Phys. 10, 10021–10031. doi:10.5194/acp-10-10021-2010
Rue, H., Martino, S., and Chopin, N. (2009). Approximate Bayesian inference for latent Gaussian models by using integrated nested Laplace approximations. J. R. Stat. Soc. Ser. B Stat. Methodol. 71, 319–392. doi:10.1111/j.1467-9868.2008.00700.x
Ryan, W. F. (2016). The air quality forecast rote: recent changes and future challenges. J. Air & Waste Manag. Assoc. 66, 576–596. doi:10.1080/10962247.2016.1151469
Schwartz, J., Laden, F., and Zanobetti, A. (2002). The concentration-response relation between PM(2.5) and daily deaths. Environ. Health Perspect. 110, 1025–1029. doi:10.1289/ehp.021101025
Shah, A. S., Langrish, J. P., Nair, H., McAllister, D. A., Hunter, A. L., Donaldson, K., et al. (2013). Global association of air pollution and heart failure: a systematic review and meta-analysis. Lancet 382, 1039–1048. doi:10.1016/S0140-6736(13)60898-3
Shen, L., Mickley, L. J., and Gilleland, E. (2016). Impact of increasing heat waves on U.S. ozone episodes in the 2050s: results from a multimodel analysis using extreme value theory. Geophys. Res. Lett. 43, 4017–4025. doi:10.1002/2016GL068432
Shen, L., Mickley, L. J., and Murray, L. T. (2017). Influence of 2000–2050 climate change on particulate matter in the United States: results from a new statistical model. Atmos. Chem. Phys. 17, 4355–4367. doi:10.5194/acp-17-4355-2017
Silva, R. A., West, J. J., Lamarque, J.-F., Shindell, D. T., Collins, W. J., Faluvegi, G., et al. (2017). Future global mortality from changes in air pollution attributable to climate change. Nat. Clim. Change 7, 647–651. doi:10.1038/nclimate3354
Smith, R. L. (1989). Extreme value analysis of environmental time series: an application to trend detection in ground-level ozone. Stat. Sci. 4, 367–377. doi:10.1214/ss/1177012400
Spracklen, D. V., Mickley, L. J., Logan, J. A., Hudman, R. C., Yevich, R., Flannigan, M. D., et al. (2009). Impacts of climate change from 2000 to 2050 on wildfire activity and carbonaceous aerosol concentrations in the western United States. J. Geophys. Res. 114, D20301. doi:10.1029/2008JD010966
Switanek, M. B., Troch, P. A., Castro, C. L., Leuprecht, A., Chang, H.-I., Mukherjee, R., et al. (2017). Scaled distribution mapping: a bias correction method that preserves raw climate model projected changes. Hydrol. Earth Syst. Sci. 21, 2649–2666. doi:10.5194/hess-21-2649-2017
Tagaris, E., Liao, K.-J., DeLucia, A. J., Deck, L., Amar, P., and Russell, A. G. (2009). Potential impact of climate change on air pollution-related human health effects. Environ. Sci. Technol. 43, 4979–4988. doi:10.1021/es803650w
Tai, A. P. K., Mickley, L. J., and Jacob, D. J. (2010). Correlations between fine particulate matter (PM2.5) and meteorological variables in the United States: implications for the sensitivity of PM2.5 to climate change. Atmos. Environ. 44, 3976–3984. doi:10.1016/j.atmosenv.2010.06.060
Thompson, M. (2001). A review of statistical methods for the meteorological adjustment of tropospheric ozone. Atmos. Environ. 35, 617–630. doi:10.1016/S1352-2310(00)00261-2
Thrasher, B., Wang, W., Michaelis, A., Melton, F., Lee, T., and Nemani, R. (2022). NASA global daily downscaled projections, CMIP6. Sci. Data 9, 262. doi:10.1038/s41597-022-01393-4
Trail, M., Tsimpidi, A. P., Liu, P., Tsigaridis, K., Hu, Y., Nenes, A., et al. (2013). Downscaling a global climate model to simulate climate change over the US and the implication on regional and urban air quality. Geosci. Model. Dev. 6, 1429–1445. doi:10.5194/gmd-6-1429-2013
US EPA (2023). EQUATESv1.0: emissions, WRF/MCIP, CMAQv5.3.2 data - 2002-2019 US_12km and NHEMI_108km. doi:10.15139/S3/F2KJSK
US EPA-AQSweb (2024). AQS web downloads. Available at https://aqs.epa.gov/aqsweb/airdata/download_files.html#AQI (Accessed January 26, 2024).
US EPA-NAAQS (2015). Air quality modeling final rule technical support document 2015 ozone NAAQS good neighbor plan. Available at https://www.epa.gov/system/files/documents/2023-03/AQ%20Modeling%20Final%20Rule%20TSD.pdf (Accessed January 26,lts from a multimodel analysis using extr 2024).
Us Epa, O. (2014). Patient exposure and the air quality index. Available at: https://www.epa.gov/pmcourse/patient-exposure-and-air-quality-index (Accessed October 9, 2023).
Us Epa, O. (2016). Air quality - national summary. Available at: https://www.epa.gov/air-trends/air-quality-national-summary (Accessed October 6, 2023).
Val Martin, M., Heald, C. L., Lamarque, J.-F., Tilmes, S., Emmons, L. K., and Schichtel, B. A. (2015). How emissions, climate, and land use change will impact mid-century air quality over the United States: a focus on effects at national parks. Atmos. Chem. Phys. 15, 2805–2823. doi:10.5194/acp-15-2805-2015
Weaver, C. P., Liang, X.-Z., Zhu, J., Adams, P. J., Amar, P., Avise, J., et al. (2009). A preliminary synthesis of modeled climate change impacts on U.S. Regional ozone concentrations. Bull. Am. Meteorological Soc. 90, 1843–1864. doi:10.1175/2009BAMS2568.1
Wells, B., Dolwick, P., Eder, B., Evangelista, M., Foley, K., Mannshardt, E., et al. (2021). Improved estimation of trends in U.S. ozone concentrations adjusted for interannual variability in meteorological conditions. Atmos. Environ. 248, 118234. doi:10.1016/j.atmosenv.2021.118234
Wiedinmyer, C., Akagi, S. K., Yokelson, R. J., Emmons, L. K., Al-Saadi, J. A., Orlando, J. J., et al. (2011). The Fire INventory from NCAR (FINN): a high resolution global model to estimate the emissions from open burning. Geosci. Model. Dev. 4, 625–641. doi:10.5194/gmd-4-625-2011
Wilson, B., Pope, M., Porter, J. R., Kearns, E., Shu, E., Bauer, M., et al. (2022). Characterizing changes in extreme ozone levels under 2050s climate conditions: an extreme-value analysis in California. Atmos. Environ. X 16, 100195. doi:10.1016/j.aeaoa.2022.100195
Keywords: air quality, particulate matter, ozone, wildfire smoke, climate change
Citation: Wilson B, Pope M, Melecio-Vazquez D, Hsieh H, Alfaro M, Shu E, Porter J and Kearns EJ (2024) Climate adjusted projections of the distribution and frequency of poor air quality days for the contiguous United States. Front. Earth Sci. 12:1320170. doi: 10.3389/feart.2024.1320170
Received: 11 October 2023; Accepted: 14 February 2024;
Published: 07 March 2024.
Edited by:
Ana G. Rappold, United States Environmental Protection Agency (EPA), United StatesReviewed by:
Amit Kumar Mishra, Jawaharlal Nehru University, IndiaJayanarayanan Kuttippurath, Indian Institute of Technology Kharagpur, India
Copyright © 2024 Wilson, Pope, Melecio-Vazquez, Hsieh, Alfaro, Shu, Porter and Kearns. This is an open-access article distributed under the terms of the Creative Commons Attribution License (CC BY). The use, distribution or reproduction in other forums is permitted, provided the original author(s) and the copyright owner(s) are credited and that the original publication in this journal is cited, in accordance with accepted academic practice. No use, distribution or reproduction is permitted which does not comply with these terms.
*Correspondence: Edward J. Kearns, ed@firststreet.org