- 1School of Ecological Technology and Engineering, Shanghai Institute of Technology, Shanghai, China
- 2Shanghai Municipal Landscape Management and Guidance Station, Shanghai, China
The traditional visual tree assessment method is subjective in evaluating tree risks and therefore not effective in precisely detecting internal decay in tree trunk and root systems. To improve the accuracy of street tree risk assessment, a new nondestructive testing method was proposed. This new tree risk assessment method combines different non-destructive testing technologies, such as sonic tomography and ground-penetrating radar, which could significantly increase the accuracy of risk assessment in tree trunks and roots. The method was applied to evaluate the risk of 1,001 street trees in Shanghai’s historical feature protection area. The results revealed that despite most street trees having low branch and trunk risk levels, more than one-third had high root risk levels. The risk factors of street trees were mainly in the trunk and root system, with a significant correlation between the street tree risk level and tree cavities, diseases, and insect pests, as well as the depth and range of the root distribution, leaning, and internal decay in trunks. With the help of non-destructive testing and risk assessment analysis, as well as targeted prevention measures, the possibility of street risk damage was largely reduced, including street trees tilting and collapsing during typhoons, etc.
1 Introduction
As crucial element of urban greening, street trees provide numerous benefits to urban environment and city residents (Nowak et al., 2006; Sreetheran et al., 2011; Kardan et al., 2015; Mullaney et al., 2015), and play an irreplaceable role in building resilient cities (Chevallerie, 1983; Li et al., 2009; Lopes et al., 2009; Roy et al., 2012; Jia et al., 2021) and in preserving natural and cultural heritage in cities (Li et al., 2012). Street trees are exposed to a variety stresses, such as complex urban climate (Burton, 2002; Jim, 2003;Jia et al., 2021), buildings with high density (Wong, 2010; Tan et al., 2016), and poor soil conditions (Loeb, 1992; Jim, 2003; Jim and Zhang, 2013; Tang, 2018). As a result, street trees may show various unhealthy conditions, including large crown deviation, leaning, internal decay, and root damage (Li et al., 2009; Shu et al., 2011; Gao and Liu, 2014; Jia, 2014; Meunpong et al., 2019). These conditions may pose risks under extreme weather conditions, such as the breaking and falling of branches, trunks, or the entire tree (Gao and Liu, 2014; Jia et al., 2021), therefore affecting urban traffic and the safety of city residents (Lopes et al., 2009; Lazim and Misni, 2016; Jia et al., 2021). By conducting organized tree risk assessment, urban managers can identify the potential risks of street trees and take specific measures to eliminate or mitigate these risks. Tree risk assessment systems that are used in most cases include the International Society of Arboriculture (ISA) Tree Hazard Evaluation (Matheny and Clark, 1994), the United States Department of Agriculture Forest Services Community Tree Risk Evaluation Method (Pokorny, 2003), and the ISA Tree Risk Assessment Best Management Practice (BMP) Method (Smiley and Lilly, 2017). Although these methods share similarities in evaluating tree structure, defects, and potential hazards, they differ in the measurement of each particular risk factor (Norris, 2007; Matheny and Clark, 2009). In many cities in China, visual tree assessment (VTA) had the commonly used method (Zhan et al., 2007; Cai, 2014; Gao and Liu, 2014; Liu et al., 2017; Wang et al., 2017). However, the lack of quantitative assessment of certain indicators in VTA made the assessment results highly subjective. Particularly, this method cannot be used to accurately identify the internal decay of trunks and roots, making the risks of these parts unclear (Li et al., 2009; He et al., 2021). Therefore, it is necessary to explore more accurate diagnosis and assessment methods, especially for the risk detection of ancient or large trees (Hu et al., 2000; Jim, 2005; Zhan et al., 2007; Zheng et al., 2013; Xiao et al., 2016; Ma et al., 2017; Júnior et al., 2019).
Non-destructive testing (NDT) is an emerging tree/wood testing technology, which mainly includes sonic tomography technology and ground penetrating radar technology. The tomography techniques that were developed for engineering or medical applications have been evaluated for their applicability in urban trees. It is found that acoustic methods could be effective to detect the internal decay of urban trees (Wang and Allison, 2008). Nicolotti et al. (2003) applied three different types of tomography methods (electric, ultrasonic, and georadar) on urban trees and found that ultrasonic tomography was the most effective one, which could locate the position of the anomalies and estimate their sizes, shapes, and characteristics. Gilbert and Smiley (2004) evaluated the ability of acoustic tomography to quantitatively detect the decay size in two types of urban trees. PiCUS tomography and visual inspection were also applied to evaluate 27 cross-sections from 13 trees, which showed high accuracy in detecting decaying areas and decaying amount. Brazee et al. (2011) compared the measurement of tree internal decay using acoustic and electrical impedance tomography. Similarly, Rinn (2015) verified acoustic tomography with resistograph testing. Qin et al. (2018) combined the acoustic-laser technique and acoustic tomography to assess Cinnamomum camphora. As confirmed by other studies, sonic tomography has also been proven to be highly effective in detecting the internal decay of trees (Liu and Li, 2018; Baláš et al., 2020), even at early stages of wood decomposition (Dudkiewicz and Durlak, 2021). Salvatore et al. (2022) used four different devices to measure decay: a microsecond timer, an electric resistivity tomograph, an acoustic tomograph and a resistograph. This study indicated high correlations between resistance drilling and stress wave, which provided an opportunity to replace the resistograph with a less invasive microsecond timer or the acoustic tomograph. These studies demonstrated that sonic tomography can accurately locate the internal damage of tree trunks and, therefore, can be applied to urban tree risk evaluation. It is worth noting that the “Picus sonic tomography” method is not completely non-invasive, as it requires the installation of measuring nails and sensors in the tree trunk (Xiao et al., 2016). Although these wounds will gradually heal, there is a possibility of fungi and bacteria infiltrating into the tree trunk, making it a “conditional” NDT (Júnior et al., 2019). Ground-penetrating radar (GPR), as a recognized geophysical technology with high efficiency and resolution, can detect decay and layer interfaces in tree trunk by recording reflected and diffracted signals from anomalies in the trunks. However, the interpretation of GPR data is challenging, as the irregular outline of the tree trunk makes it difficult to perform the processing algorithms (Alani et al., 2019). At present, GPR technology has become increasingly popular for detecting and analyzing the roots of urban trees, as well as for studying the stability and resistance of trees. This method can monitor the root structure of trees for a long period without interfering with their growth, yet the GPR data is largely dependent on the underground spatial structure and soil composition (Alani et al., 2020).
While advanced tree detection technologies have been shown to be effective in quantifying internal decay, it is not clear how their data impact tree risk assessments (Koeser et al., 2017). Shanghai has a subtropical monsoon climate with four distinct seasons. The city receives more than 60% of its annual rainfall from May to September, with typhoons affecting the area from July to September. The downtown areas of Shanghai are densely populated with high-rise buildings, leaving limited space for urban greenery. The rapid pace of urban development has put significant pressure on urban trees in the area. In this study, we conducted a risk assessment of street trees in the Hengshan Road-East Fuxing Road historical feature protection area in Shanghai using a precise diagnosis technique that combines VTA with NDT methods. We also built a comprehensive risk assessment system based on a risk matrix method to evaluate street tree risks and analyzed the risk spatial distribution and the main risk factors of street trees in the study area. Then, targeted prevention measures were taken for street trees with high risk levels. In July 2021, Severe Typhoon In-Fa (International Code: 2106) had a significant impact on the southeast coast of China. The strong winds and heavy rain brought by the typhoon upon its landing in Shanghai caused extensive damage to buildings and outdoor facilities throughout the city. After the “Severe Typhoon In-Fa”, further analysis was carried out to verify the risk damage of street trees in the study area.
2 Materials and methods
2.1 Study area and subjects
The Hengshan Road–East Fuxing Road historical feature protection area was the largest protected area of historical and cultural features in Shanghai. Street trees in this area are an important component of the historical and cultural protection strategy. From September 2020 to March 2021, 1,001 street trees with a diameter at breast height greater than 40 cm were subjected to risk assessment on 14 main roads in the Hengshan Road-East Fuxing Road Historical Feature Protection Area (Figure 1). There are various species of trees in the study area, such as Platanus orientalis L., Pterocarya stenoptera (C. DC), Sophora japonica L., Quercus acutissima Carruth and Sapium sebiferum (L.) Roxb. Among them, P. orientalis was the most abundant one, with a total number of 884 (88.31%), and P. stenoptera ranked the second, with a total of 109 (10.89%). Platanus orientalis is the most planted street tree in Shanghai, accounting for approximately 65% of the total trees.
Over the past century, the street tree species in the study area have changed. However, P. orientalis and P. stenoptera have been preserved due to their strong resistance and longevity, thus becoming an important part of the area’s historical and cultural heritage (Yan et al., 2012; Yang and Yan, 2013). Regrettably, the growth of street trees has been continually disrupted due to the construction of high-density buildings, roads, and pipelines. Aging and other factors, such as diseases and insect pests, have posed risks of falling or breaking of trunks and branches of street trees under extreme weather conditions (Wu, 2016; Jin et al., 2019).
2.2 Research methods
2.2.1 Visual tree assessment
The acquired risk assessment data were analyzed and used to generate graphs using ArcGIS 10.8 software. The data included tree species, coordinates, tree height, diameter at breast height (DBH), trunk inclination and tree’s potential growth indicators.
The street trees with a DBH greater than 40 cm were selected for VTA. The assessment covered the branches, trunk, root system, and their surrounding environment. To detect the main risk points of street trees, previous research results and ISA recommendations were referred (Matheny and Clark, 1994; He et al., 2021). The branch assessment was focused on large crown deviation, the presence of fungal fruit bodies, diseases and insect pests, large dead branches, and dieback in the tip. The trunk assessment included trees leaning, tree trunk cavities and mechanical damage. The root assessment was focused on intertwined roots and underground pipe gallery construction. The surrounding environment assessment included possible falling sites, road grade, and the density of surrounding buildings.
2.2.2 Non-destructive testing
Non-destructive testing (NDT) was used to detect trunk internal decay and the root distribution of street trees in a non-invasive manner. Among them, sonic tomography (PiCUS-3, Angus, Germany) was used to detect the internal decay in trunks of street trees. This method used high-precision tree geometric information software to calculate sound velocity and to draw tree sound wave transmission rate or density images, thereby accurately describing the internal structure of trees. According to the manufacturer’s instructions, three cross-sections were selected from each tree for detection. Ideally, the three cross-sections are located 1) near the root system, 2) at the measurement point of DBH, and 3) below the first bifurcation point of the tree. For convenience, the three cross-section selected in this study were: 1) 0.3 m above ground the level, 2) 1.6 m above ground level (0.3 m above the point of DBH), and 3) 2.1 m below branch height (Figure 2). All data were imported into the computer that was connected to the sensors and main control unit, followed by data analysis and visualization using PiCUS-3 software.
Tree radar unit (TRU™, TreeRadar, US) was used to detect the roots distribution of street trees without interfering with their growth. This method utilized ground-penetrating radar technology (GPR) to perform non-destructive scanning of the root system from both vertical and horizontal directions. In the vertical direction, the root distribution was categorized into three zones: 0–20 cm, 21–40 cm, and 41–100 cm below the ground level. The horizontal distribution was detected using the concentric circle method, where the trunk of the tree was considered the center of the circle. The scanning process included three semicircular cross-sections on the sidewalk side and two on the roadway side, with the radii set as 1.0 m, 1.5 m, and 2.0 m. This is because street tree pools in Shanghai are all within 1.6 m of side length, and the areas outside tree pools are sidewalks or roadways, while underground parts are concrete structures. The root system is rarely able to extend beyond a circular area with a radius of 2.0 m around the trunk (Figure 2). The obtained scanning data were analyzed using Tree WinTM PRO software (TreeRear, US) to generate root density maps in both horizontal and vertical directions and three-dimensional virtual model of tree roots.
2.2.3 Establishment of assessment indicator system
The risk matrix method could be used for risk ranking, risk sources identification, and risk responses. As the operation is simple and intuitive, it was widely used in various fields of risk management. The risk matrix method combined both qualitative and quantitative analysis to classify the impact of risk consequences and the possibility of risk occurrence (Dunster et al., 2017). The calculation formula for risk assessment was:
The risk matrix method was used to evaluate the impact and possibility of risk consequences and to determine the risk level of street trees. The parameters used in the street tree risk assessment are shown in Tables 1–4. Table 1 represented the risk occurrence possibility(P), with values from i to iv representing low to extremely high-risk possibility. Table 2 described the severity of the consequences(S), with a to d represented negligible to extreme severity. Table 3 showed the comparison values of tree risk level(R), with X-axis representing the risk severity and Y-axis representing the risk possibility. Table 4 listed the risk classification criteria in the risk matrix.
Previous studies revealed that tree collapse due to root/trunk rot and branch breakage due to trunk/branch decay (Roson-Szeryńska et al., 2014) could damage vehicles and buildings and harm pedestrians (Lazim and Misni, 2016). The latest research findings and non-destructive testing techniques were employed in this study to ensure that the assessment items and indicators were up-to-date and accurate (Dunster et al., 2017; He et al., 2021). The assessment items were focused on the branches, trunks, and roots of street tree, and the assessment indicators included internal decay in trunks, the depth and range of the root distribution, as well as the VTA indices mentioned earlier. Based on these factors, we constructed a comprehensive and accurate street tree risk assessment system that included the risk occurrence possibility (P) and the risk consequence severity (S) (Table 5).
The risk occurrence possibility (P) assessment mainly considered the problem of structural failure of trees.13 indicators (C1-C13) for B1-B4 were scored, then the total score was converted to the possibility level in Table 1, using the calculation method as follows: 5–15 points, 16–18 points, 19–22 points, 23–45 points were converted to the scores of i (Low), ii (Moderate), iii (High), or iv (Extremely high), respectively. The severity of risk consequences (S) mainly considered growth conflicts. Three indicators (C14-C16) for B5-B6 were scored, then the total score was converted to the severity (S) level in Table 2, with a (2<S ≤ 4 points), b (4<S ≤ 6 points), c (6<S ≤ 8 points), d (8<S ≤ 10 points).
By looking up to the risk matrix (Table 3) with the obtained results P and S level, the risk level R of a single tree could be obtained (Table 4), with I (<3), II (3–6), III (8–9), and IV (12–16) corresponding to “Negligible risk”, “Acceptable risk”, “Medium risk”, and “Significant risk”, respectively.
2.2.4 Data and statistical analysis
The spatial distribution of street tree risks was visualized using ArcGIS10.7 software. The correlation between the street tree risk level and each indicator was examined using R 4.1.0. Kendall rank correlation coefficient was calculated using stats (v. 4.1.2) and corrplot (v. 0.84) package to assess the relationship of ordinal data (The codes are available in Supplementary Material). The stats package was used to calculate the kendall correlation coefficient and the significance test, and the corrplot package was used to plot the correlation heatmap. The size and color of the bubble represented the Kendall rank correlation coefficient value. The number of asterisks represented the significance level of the correlation between the variables, with * p-value <0.05, * * p-value <0.01, * * * p-value <0.001.
3 Results
3.1 Street tree risk assessment and analysis
3.1.1 Accurate diagnosis of the street tree risk
As shown in Figure 3, more than 93% of the street trees in the study area had a tree branch risk occurrence possibility (P) of level II or below. Only 5.99% and 0.60% of the trees were found to have a level III and a level IV branch risk, respectively. Specifically, fifty P. orientalis had a level III branch risk, and three had a level IV branch risk, while nine P. stenoptera had a level III branch risk, and two had a level IV branch risk. Only one S. sebiferum had a level IV branch risk. Hence, the regular monitoring of tree risks is necessary.
More than 85% of the street trees were rated at level II or below for trunk risk occurrence possibility (P) (Figure 3), while 12.59% and 1.80% of the trees were rated at level III and level IV, respectively. Approximately 89% of the P. orientalis had a trunk risk level of II or below, while 47% of the P. stenoptera had a trunk risk level of III or above.
More than 62% of the street trees had a root system risk occurrence possibility (P) level of II or below, while 37% of trees had a level III or higher root system risk (Figure 3). Most street tree root systems were distributed within the 0–50 cm depth range, and there was a serious imbalance between the canopy and root system. A high proportion of street trees had a high risk of root system damage. Among P. orientalis, 288 had level III root system risk p values, and 51 had level IV root system risk p values. Among P. stenoptera, 27 had level III root system risk p values, and 3 had level IV root system risk p values. Thus, the health condition of street tree root systems needs to be closely monitored.
3.1.2 Overall risk assessment of street trees
Figure 4 illustrated that more than 74% of street trees had a risk occurrence possibility (P) level of II or below, yet the majority had a risk consequence severity (S) level of II and III, comprising 25.17% and 63.14%, respectively. More than 76% of the trees had a risk consequence level (R) of II or below; 194 trees had a risk consequence level (R) of III, and 42 trees had a risk consequence level (R) of IV. These trees need to be closely monitored, and appropriate management measures should be taken to ensure their safety.
As depicted in Figure 5, the risk occurrence possibility level of P. orientalis was moderate, with 78% having a level of II or below. More than 56% of the P. stenoptera exhibited a risk occurrence possibility level of III or higher, indicating a high risk. One S. sebiferum and one S. japonica were identified to have a risk occurrence possibility level (P) of IV. It was observed that 83% of the falling/breaking parts were in the branches of P. orientalis and 91% were in the trunk of P. stenoptera.
3.2 Overall spatial pattern of street tree risk
Figure 6 shows that Dongping Road and Fenyang Road had the highest average branch (B1) risk occurrence possibility level, while Baoqing Road and Taojiang Road had the lowest risk occurrence possibility level (Figure 6A). The high branch risk was due to severe disease, insect pests, and dieback in the upper portion and large crown deviation. Besides, Dongping Road and Wukang Road had the highest average trunk (B2) risk occurrence possibility level, while Gao’an Road and Gaoyou Road had the lowest average trunk (B2) risk occurrence possibility level (Figure 6B). The presence of tree cavities and internal decay in trunks was the main cause of high trunk (B2) risk occurrence possibility level p-value, while leaning of trees was the minor cause. Moreover, Gao’an Road and Gaoyou Road had the highest average root system (B3) risk occurrence possibility level p-value, while Baoqing Road and Yueyang Road had the lowest average root system (B3) risk occurrence possibility level p-value (Figure 6C). The high root system (B3) risk occurrence possibility level p-value was mainly caused by belowground pipe gallery construction, which damaged the root system on the motorway side and led to an uneven distribution of the range and depth of the root system.
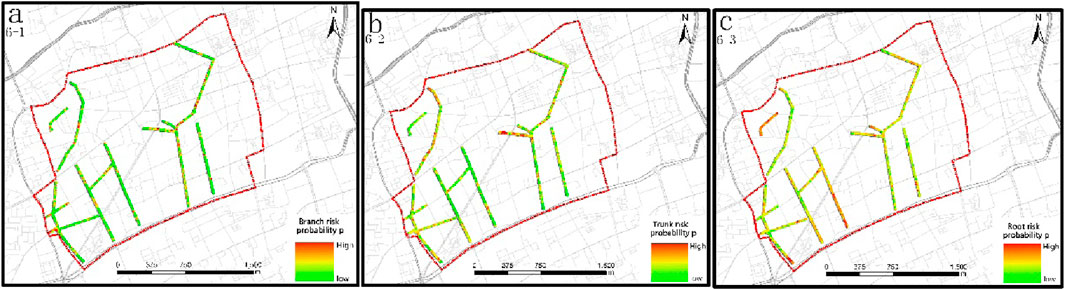
Figure 6. Spatial distribution of street tree safety risk assessment items Note: (A–C) are the spatial distributions of branch, trunk, and root assessment items, respectively.
According to Figure 7A, Dongping Road and Wukang Road had the highest average overall risk occurrence possibility level (P) values, while Baoqing Road and Gaoan Road had the lowest. Figure 7B showed that Taiyuan Road and Tianping Road had the highest risk consequence severity level (S) values, while Donghu Road and Taojiang Road had the lowest. Guangyuan Road and Tianping Road had the highest average risk level (R) values, while Donghu Road and Taojiang Road had the lowest (Figure 7C). Tianping Road had the highest risk consequence severity level (S) and risk level (R) values due to its high road grade, high building density, damage to the root systems caused by the construction of an underground pipeline, branches with diseases and insect pests, and the presence of cavities and decay within tree trunks.
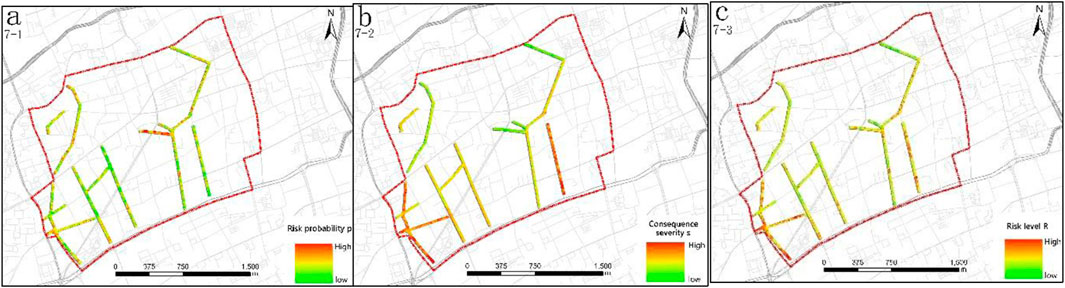
Figure 7. Spatial distribution of safety risk assessment of street trees Note: (A–C) are the spatial distributions of possibility level P, severity level S, and risk level R, respectively.
3.3 Street tree risk point analysis and risk prevention
3.3.1 Street tree risk point analysis
The risk assessment point is a crucial factor affecting the risk occurrence possibility of street trees. Among the risk factors, root depth distribution (C11) was identified in 873 trees with a moderate risk severity, root range distribution (C10) was found in 754 trees with a moderate risk severity, and belowground pipe gallery construction (C13) was detected in 473 trees (Figure 8). These three factors were the most prevalent indicators of street tree risk, and they were all in the root system. The frequency of risk factors associated with trunks and branches (C1-C9) of street trees was lower than those related to roots. Internal decay in trunks (C9) was identified in 468 trees with a medium severity level, while trunk cavities (C7) were found in 322 trees, mostly with a high severity level. Tree leaning (C6) and diseases and insect pests (C4) were also common. Other factors only had limited influence on the risk possibility.
The risk level of street trees exhibited a significantly positive correlation with several factors related to trunks and roots, including tree trunk cavities (C7), diseases and insect pests (C4), the root range distribution (C10), the root depth distribution (C11), the tree leaning (C6), and internal decay in trunks (C9) (Figure 9). There was also a positive correlation between the risk level and factors such as large crown deviation (C1), large dead branches (C2), and root intertwined (C12), but there was no significant correlation with fungal fruiting bodies (C3). The results also exhibited that the presence of large dead branches (C2) was positively correlated with dieback in the tip (C5), while the presence of fungal fruiting bodies (C3) was positively correlated with both dieback in the tip (C5) and mechanical damage (C8). The presence of internal decay in trunks (C9) was positively correlated with both the tree leaning (C6) and trunk cavities (C7).
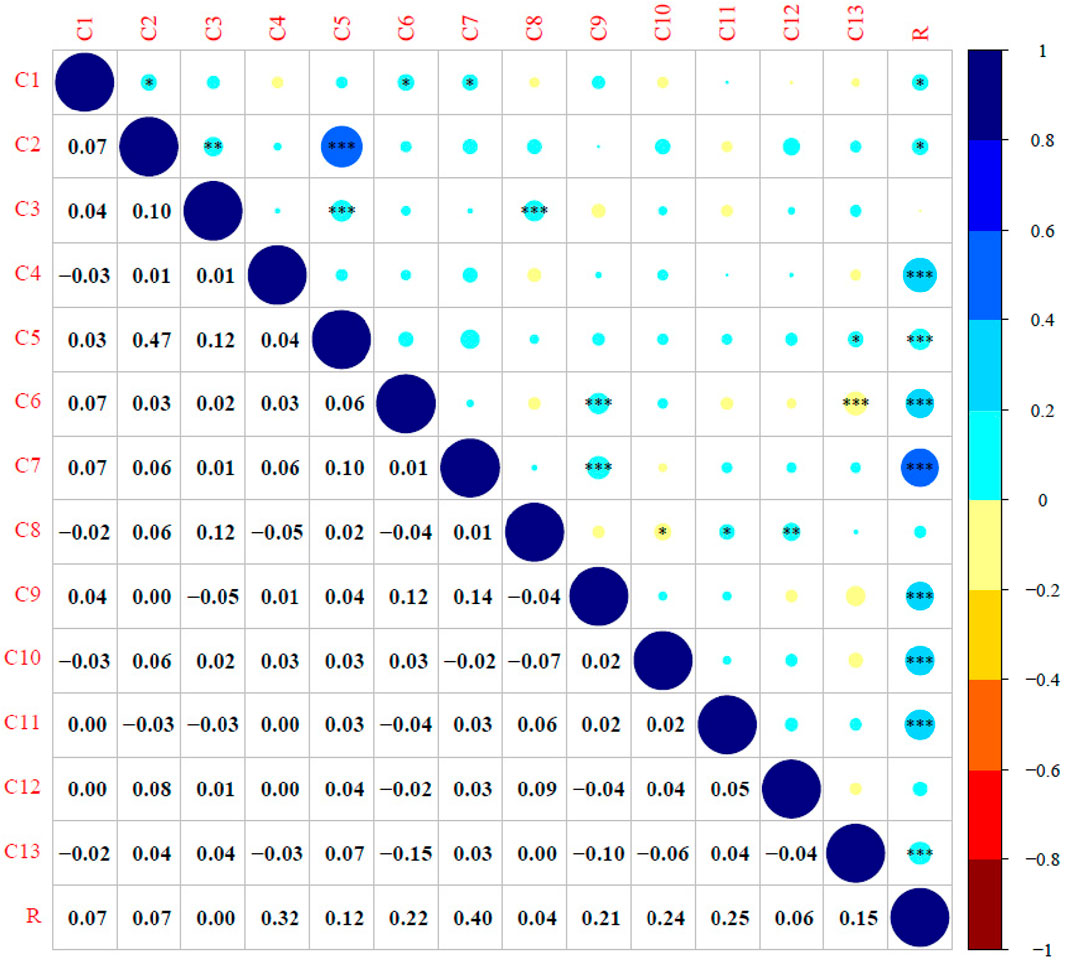
Figure 9. Correlation analysis of the 13 indicators and the R values (Note: * p-value <0.05, * * p-value <0.01, * * * p-value <0.001)
3.3.2 Risk prevention and analysis during typhoons
Based on the results of the tree risk assessment, targeted prevention and control measures were implemented for trees with higher risk consequence levels in the study area from April to June 2022 before the arrival of typhoon season. The focus was on trees with a risk occurrence possibility (P) level of II or above and a risk consequence severity (S) level of III or above. The specific measures included: 1) pruning and shaping of trees, with particular attention given to the diseased, weak, and dead branches; 2) prevention and control of wood-boring pests, such as longhorn beetles and termite; 3) straightening and pruning of tilted trees; and 4) key reinforcement treatment, including the installation of protective support for street trees with high root risk before the typhoon season. Besides, appropriate risk prevention measures were implemented to prevent damage to buildings, vehicles, and pedestrians caused by falling branches or trees.
After the Severe Typhoon In-Fa, it was found that only 9 street trees on 6 of the 14 roads that have been subjected to tree risk assessments had incidents of broken branches and fallen trees. Among them, there were broken branches only in Tianping Road, while there were fallen trees in Gao’an Road, Wukang Road, Taiyuan Road and Kangping Road. Damages from street tree risk on the above roads occurred where there had relatively high risk in the comprehensive spatial pattern analysis of street tree risk. In this typhoon, only 0.9% of all surveyed trees were damaged, and there were no losses to people, buildings, or vehicles. This implied street tree risk can be significantly reduced by identifying potential risks of trees through the street tree risk assessment and taking necessary preventive measures. Whether necessary preventive measures were taken or not, regular risk detecting of street trees is necessary. After importing tree risk data into the urban tree management platform, it is necessary to continuously monitor the growth status of high-risk trees.
4 Discussion
In recent years, climate changes and tree diseases that cause trees to fall have increased the possibility of street trees’ harm to citizens (De Petris et al., 2020). Accidents related to trees or branches’ sudden falls have drawn people’s attention. As a result, the theories and application in evaluating tree risk have been the subject of debates among researchers and technicians. A thorough inspection of trees’ branches, trunks and root collars is essential in detecting hazardous conditions of street trees (Smiley et al., 2000).
The results indicated that the tree branch risk occurrence possibility level was generally low. This was probably because branches that may affect public facilities were removed annually to reduce the possibility of large crown deviation, large dead branches, and diseases and insect pests. Similarly, tree trunk risk occurrence possibility level was relatively low, especially for most P. orientalis. However, large trees planted in the downtown area might have been affected by long-time development in the site environment, leading to various risks such as large crown deviation, internal decay in the trunks, and leaning in the branches and trunks, as revealed by most VTA-based assessment (He et al., 2021). The difference between regular street trees and trees in important protected area in Shanghai might result from the relatively standardized management of trees with high historical value, thereby reducing the risk of branch and trunk risk occurrence possibility. The high-risk level of root system is mainly due to the limited area available for tree growth, poor soil fertility, and inadequate growth space for root systems, making it difficult for roots to extend and grow to the surrounding area to enhance their wind resistance (Nicoll and Ray, 1996). Additionally, road adjustments and the frequent belowground pipeline construction resulted in the direct cutting of street tree roots, making it difficult to support the trees in all directions. More than 74% of street trees in the protected area had a risk consequence severity level of III or above, mainly due to the interaction between high building density, pedestrian and vehicular traffic, narrow sidewalks, and power lines.
The risk of street trees was closely related to their growth environment, especially in developed urban areas, where the high flow of people and vehicles, high-density buildings, and road construction could continuously endanger the growth of street trees and thus cause serious risk consequences (Júnior et al., 2019). Studies conducted in cities such as Hong Kong and Xiamen have demonstrated that street trees are susceptible to the negative effects of narrow growth spaces and municipal engineering projects such as frequent construction and underground trenching (Jim, 2003; Tang, 2018). Additionally, excessive soil compaction caused by heavy vehicle traffic can damage tree roots, ultimately increasing the possibility of tree risk (Jim and Zhang, 2013; North et al., 2017). Therefore, high-risk roads that had the highest risk consequence severity level and risk level values, were those with high road grades, high building density, small building spaces, and high traffic or pedestrian flow, such as Tianping Road.
Tree risk assessment is a process for identifying, analyzing, and evaluating risks (Koeser et al., 2017). The traditional and internationally recognized visual assessment methods mainly focus on growing of tree crowns and trunks. Arboriculturists consider VTA as an essential practice that serves as a starter for evaluating tree defects and provides basic information for assessing tree growth performance and stability (Lin and Yang, 2015). However, VTA method cannot detect the internal decay of tree trunks and roots, resulting in high subjectivity in the risk assessment (Li et al., 2009). The instrumental investigation could quantitatively describe any anomalies found. Concerns related to public safety support the development and application of rapid and precise diagnostic technologies to detect decay and other types of structural defects in trees (Brashaw et al., 2009). Both the instruments used in this study demonstrated the ability to effectively detect tree trunks and roots’ internal decay. It is found that the main risk point of street trees is in the root system, followed by internal decay/cavities in the trunk. However, considering the complex and time-consuming data detection procedure, it is suggested to acquire more detailed data only in trees with known decay levels or in trees that require monitoring over time (Han, 2013; Jia et al., 2021). The results of this study showed that there was a correlation between the VTA indicators (such as tree leaning) and the risk indicators of advanced instrument detection (such as internal decay in trunks). In a survey of decay among oak trees in Tampa, (Florida, United States), Koeser et al. (2016) found that 90% of laurel oak (Quercus laurifolia) with measured stem decay greater than 30% of the diameter had external indicators of decay. Similarly, in an assessment of maple (Acer spp.), linden (Tilia spp.), and birch (Betula spp.) trees, Terho (2009) found that most of decayed trees had visual symptoms such as cracks, mechanical wounds, and fungal fruiting bodies. Therefore, to improve the speed and accuracy of street tree risk detection, future studies are required to explore the correlation between these two methods.
According to the assessment of tree damage after a typhoon, effective risk assessment and targeted restoration measures for street trees could significantly reduce the possibility of tree risks occurring. To further improve the accuracy of tree risk assessment, a comparative analysis of the trees damage in areas that have not been assessed are still needed. For large-scale street trees in the research area, regular observation of high-risk trees to obtain multi-year detection data is also necessary to provide detailed recommendations for improving street tree safety.
5 Conclusion
To reduce the subjectivity caused by traditional visual tree assessment and improve the accuracy of street tree risk assessment, a new assessment method based on non-destructive testing has been developed. A comprehensive indicator system for tree risk assessments has been established using a combination of qualitative and quantitative methods. The non-destructive testing technique enables the acquisition of information regarding internal trunk decay and the distribution depth and range of the root system. Such information, which cannot be obtained from conventional visual assessments, can provide more precise quantitative indicators for street tree risk assessments.
The results of risk detection and assessment on 1,001 street trees located in the center of Shanghai indicated that most trees (over 80%) had low-risk occurrence possibility (P) level for their branches and trunks. However, nearly one-third of the street trees had a higher risk occurrence possibility (P) level for their root system due to limited space for growth. While most street trees posed low risk occurrence possibility and risk level, a significant proportion of them had high-risk consequence severity level due to the impact of the surrounding environment. The main risk points of street trees consist of the root system’s distribution depth and range, underground pipeline construction, and internal decay in the trunks and tree cavities.
Based on the non-destructive testing and risk assessment results, the implementation of targeted prevention had been taken for street trees with high-risk level in the study area. For future study of tree risks, the relationship between climate factors such as typhoons and tree risk assessment will be an important direction of tree risk mechanisms. Taking preventive measures based on the results of risk assessment and climatic characteristics is of great significance in reducing the occurrence of street tree collapse and other accidents.
Data availability statement
The datasets presented in this study can be found in online repositories. The names of the repository/repositories and accession number(s) can be found in the article/Supplementary Material.
Author contributions
KH: Conceptualization, Project administration, Validation, Writing–original draft. LW: Data curation, Investigation, Methodology, Visualization, Writing–review and editing. BW: Conceptualization, Resources, Funding acquisition, Supervision, Writing–review and editing.
Funding
The author(s) declare that financial support was received for the research, authorship, and/or publication of this article. This study was supported by the Shanghai Science and Technology Project (No. 19DZ1203701).
Acknowledgments
We thank three reviewers for the constructive comments on our manuscript.
Conflict of interest
The authors declare that the research was conducted in the absence of any commercial or financial relationships that could be construed as a potential conflict of interest.
Publisher’s note
All claims expressed in this article are solely those of the authors and do not necessarily represent those of their affiliated organizations, or those of the publisher, the editors and the reviewers. Any product that may be evaluated in this article, or claim that may be made by its manufacturer, is not guaranteed or endorsed by the publisher.
Supplementary material
The Supplementary Material for this article can be found online at: https://www.frontiersin.org/articles/10.3389/feart.2024.1338316/full#supplementary-material
Abbreviations
GIS, geographic information system; ISA, international society of arboriculture; BMP, best management practice; VTA, visual tree assessment.
References
Alani, A. M., Giannakis, I., Zou, L., Lantini, L., and Tosti, F. (2020). Reverse-time migration for evaluating the internal structure of tree-trunks using ground-penetrating radar. NDT E Internat 115, 102294. doi:10.1016/j.ndteint.2020.102294
Alani, A. M., Soldovieri, F., Catapano, I., Giannakis, I., Gennarelli, G., Lantini, L., et al. (2019). The use of ground penetrating radar and microwave tomography for the detection of decay and cavities in tree trunks. Remote Sens. 11 (18), 2073. doi:10.3390/rs11182073
Baláš, M., Gallo, J., and Kuneš, I. (2020). Work sampling and work process optimization in sonic and electrical resistance tree tomography. J. For. Sci. 66 (1), 9–21. doi:10.17221/66/2019-JFS
Brashaw, B. K., Bucur, V., Divos, F., Goncalves, R., Lu, J., Meder, R., et al. (2009). Nondestructive testing and evaluation of wood: a worldwide research update. For. Prod. J. 59 (3), 7–14.
Brazee, N. J., Marra, R. E., Göcke, L., and Van Wassenaer, P. (2011). Non-destructive assessment of internal decay in three hardwood species of northeastern North America using sonic and electrical impedance tomography. Forestry 84 (1), 33–39. doi:10.1093/forestry/cpq040
Burton, E. (2002). Measuring urban compactness in UK towns and cities. Environ. Plann B Plann. Des. 29 (2), 219–250. doi:10.1068/b2713
Cai, Y. (2014). Application of VTA method in risk assessment of street trees in fuzhou city fujian. Fujian, China: Agriculture and Forestry University.
Chevallerie, H. (1983). “The ecology and preservation of street trees,” in Ecology and design in landscape, 24th symposium of the british ecological society. Editors A. D. Bradshaw, D. A. Goode, and E. Thorp (Oxford: Blackwell Scientific Publication), 345–355.
De Petris, S., Sarvia, F., and Borgogno-Mondino, E. (2020). RPAS-based photogrammetry to support tree stability assessment: longing for precision arboriculture. Urban For. Urban Green 55, 126862. doi:10.1016/j.ufug.2020.126862
Dudkiewicz, M., and Durlak, W. (2021). Sonic Tomograph as a tool supporting the sustainable management of historical greenery of the UMCS Botanical Garden in Lublin. Sustainability 13 (16), 9451. doi:10.3390/su13169451
Dunster, J. A., Smiley, E. T., Matheny, N., and Lilly, S. (2017). Tree risk assessment manual. Atlanta, US: International Society of Arboriculture.
Gao, M., and Liu, J. (2014). Overview of security research on the landscape trees. J. Northwest For. Univ. 29 (4), 278–281+292.
Gilbert, E. A., and Smiley, E. T. (2004). Picus sonic tomography for the quantification of decay in white oak (Quercus alba) and hickory (Carya spp.). J. Arboric. 30, 277–281. doi:10.48044/jauf.2004.033
Han, F. (2013). Overview of tree risk assessment studies. J. Shandong For. Sci. Technol. 43 (5), 90–94+80.
He, K., Song, P., Wang, B., Yan, W., and Song, T. (2021). Research on safety risk assessment of urban street trees in Shanghai. Chin. Landsc. Archit. 37 (9), 106–111.
Hu, Y., Zheng, S., Guan, D., and Wang, L. (2000). Evaluation of old and famous trees in guangzhou city. China Popul. Resour. Environ. (S2), 45–47.
Jia, X. (2014). Reasons and countermeasures for the partial crown and narrow crown of garden trees. China Flowers Hort. (18), 46–47.
Jia, Y., Lei, J., and Huang, S. (2021). Safety risk assessment and management of street trees on huanshi road in guangzhou. Guangdong Landsc. Archit. 43 (4), 93–96.
Jim, C. Y. (2003). Protection of urban trees from trenching damage in compact city environments. Cities 20 (2), 87–94. doi:10.1016/S0264-2751(02)00096-3
Jim, C. Y. (2005). Monitoring the performance and decline of heritage trees in urban Hong Kong. J. Environ. Manage. 74 (2), 161–172. doi:10.1016/j.jenvman.2004.08.014
Jim, C. Y., and Zhang, H. (2013). Defect-disorder and risk assessment of heritage trees in urban Hong Kong. Urban For. Urban Green 12 (4), 585–596. doi:10.1016/j.ufug.2013.06.003
Jin, H., Huang, S., and Luo, Y. (2019). Emergency management system of garden trees under typhoon disaster. Guangdong Landsc. Archit. 41 (4), 4–8.
Júnior, S. I., Biondi, D., Ximenes, E., dos Reis, A. R. N., and Bomm, B. F. H. (2019). Risk assessment of trees protected by law in Curitiba squares, Paraná, Brazil. Bosque 40 (3), 347–354. doi:10.4067/S0717-92002019000300347
Kardan, O., Gozdyra, P., Misic, B., Moola, F., Palmer, L. J., Paus, T., et al. (2015). Neighborhood greenspace and health in a large urban center. Sci. Rep. 5 (1), 11610. doi:10.1038/srep11610
Koeser, A. K., Hauer, R. J., Klein, R. W., and Miesbauer, J. W. (2017). Assessment of likelihood of failure using limited visual, basic, and advanced assessment techniques. Urban For. Urban Green 24, 71–79. doi:10.1016/j.ufug.2017.03.024
Koeser, A. K., McLean, D. C., Hasing, G., and Allison, R. B. (2016). Frequency, severity, and detectability of internal trunk decay of street tree Quercus spp. in Tampa, Florida, U.S. U. S. Arboric. Urban For. 42 (4), 217–226. doi:10.48044/jauf.2016.020
Lazim, R. M., and Misni, A. (2016). Public perceptions towards tree risk management in subang jaya municipality, Malaysia. Procedia Soc. Behav. Sci. 222, 881–889. doi:10.1016/j.sbspro.2016.05.210
Li, C., Weng, S., Lin, Y., and Pang, R. (2009). Advance on assessment of landscaping tree health and hazard. Guangdong Agric. Sci. (7), 186–189.
Li, C., Weng, S., and Pang, G. R. (2012). An investigation and assessment of the health of the landscape trees in the green land in Guangzhou. J. Foshan Univ. 30 (6), 8–13.
Lin, C. J., and Yang, T. H. (2015). Detection of acoustic velocity and electrical resistance tomographies for evaluation of peripheral-inner wood demarcation in urban royal palms. Urban For. Urban Green 14 (3), 583–589. doi:10.1016/j.ufug.2015.05.010
Liu, L., and Li, G. (2018). Acoustic tomography based on hybrid wave propagation model for tree decay detection. Comput. Electron. Agric. 151, 276–285. doi:10.1016/j.compag.2018.06.020
Liu, X., Li, H., Zheng, Y., and Yuan, T. (2017). Safety evaluation of street trees in northern cities: taking dongwangzhuang strip greenbelt and college road street trees as examples. Xiandai Hortic. (14), 142.
Lopes, A., Oliveira, S., Fragoso, M., Andrade, J. A., and Pedro, P. (2009). “Wind risk assessment in urban environments: the case of falling trees during windstorm events in lisbon,” in Bioclimatology and natural hazards. Editors K. Střelcová, C. Mátyás, A. Kleidon, M. Lapin, F. Matejka, M. Blaženecet al. (Dordrecht: Springer Netherlands), 55–74.
Ma, L., Liu, H., Peng, Z., Xu, W., and Yuan, T. (2017). Safety assessment of trees in public green spaces of universities in northern China——a case study of Beijing forestry university zhongguo yuanyi wenzhai 33, (5), 55–56+157.
Matheny, N., and Clark, J. (2009). Tree risk assessment: what we know (and what we don't know). Arborist news 18, 28–33.
Matheny, N. P., and Clark, J. R. (1994). A photographic guide to the evaluation of hazard trees in urban areas. Int. Soc. Arboric.
Meunpong, P., Buathong, S., and Kaewgrajang, T. (2019). Google Street View virtual survey and in-person field surveys: an exploratory comparison of urban tree risk assessment. Arboric. J. 41 (4), 226–236. doi:10.1080/03071375.2019.1643187
Mullaney, J., Lucke, T., and Trueman, S. J. (2015). A review of benefits and challenges in growing street trees in paved urban environments. Landsc. Urban Plann. 134, 157–166. doi:10.1016/j.landurbplan.2014.10.013
Nicoll, B. C., and Ray, D. (1996). Adaptive growth of tree root systems in response to wind action and site conditions. Tree Physiol. 16 (11-12), 891–898. doi:10.1093/treephys/16.11-12.891
Nicolotti, G., Socco, L. V., Martinis, R., Godio, A., and Sambuelli, L. (2003). Application and comparison of three tomographic techniques for detection of decay in trees. J. Arboric. 29 (2), 66–78. doi:10.48044/jauf.2003.009
Norris, M. (2007). Tree risk assessments–what works–what does not–can we tell. In A review of a range of existing tree risk assessment methods. Perth, Australia: ISAAC Conference Perth (Vol., pp. 1–31).
North, E. A., D’Amato, A. W., Russell, M. B., and Johnson, G. R. (2017). The influence of sidewalk replacement on urban street tree growth. Urban For. Urban Green. 24, 116–124. doi:10.1016/j.ufug.2017.03.029
Nowak, D. J., Crane, D. E., and Stevens, J. C. (2006). Air pollution removal by urban trees and shrubs in the United States. Urban For. Urban Green 4 (3), 115–123. doi:10.1016/j.ufug.2006.01.007
Pokorny, J. D. (2003). Urban tree risk management: a community guide to program design and implementation. USDA For. Serv. Northeast. Area, State Private For. 3 (No. 3).
Qin, R., Qiu, Q., Lam, J. H., Tang, A. M., Leung, M. W., and Lau, D. (2018). Health assessment of tree trunk by using acoustic-laser technique and sonic tomography. Wood Sci. Technol. 52 (4), 1113–1132. doi:10.1007/s00226-018-1016-z
Roson-Szeryńska, E., Sikorski, P., and Zara-Januszkiewicz, E. (2014). The effectiveness of the visual method of hazard tree assessment (WID method) in the management of urban trees. Plants Urban Areasl., 89–93. doi:10.15414/2014.9788055212623.89-93
Roy, S., Byrne, J., and Pickering, C. (2012). A systematic quantitative review of urban tree benefits, costs, and assessment methods across cities in different climatic zones. Urban For. Urban Green. 11 (4), 351–363. doi:10.1016/j.ufug.2012.06.006
Salvatore, F. P., Maria, F. C., Giuseppe, Z., and Andrea, P. R. (2022). Comparative evaluation of inspection techniques for decay detection in urban trees. Sens. Actuators A Phys. doi:10.1016/j.sna.2022.113544
Shu, Q., Cao, Z., and Zhang, X. (2011). Relationship between tree health (Life-span and disease development) and environmental factors. Anhui For. Sci. Technol. 37 (1), 42–44.
Smiley, E. T., Fraedrich, B. R., and Fengler, P. H. (2000). “Hazard tree inspection, evaluation, and management,” in Handbook of urban and community forestry in the Northeast (Boston, MA: Springer US), 243–260.
Smiley, N. M., and Lilly, S. (2017). BMP best management practices: tree risk assessment. BMP best management practices: tree risk assessment. 2.
Sreetheran, M., Adnan, M., and Khairil Azuar, A. (2011). Street tree inventory and tree risk assessment of selected major roads in Kuala Lumpur, Malaysia. Arboric. Urban For. 37 (5), 226–235. doi:10.48044/jauf.2011.030
Tan, Z., Lau, K. K. L., and Ng, E. (2016). Urban tree design approaches for mitigating daytime urban heat island effects in a high-density urban environment. Energy Build. 114, 265–274. doi:10.1016/j.enbuild.2015.06.031
Tang, L. (2018). Problems in the planting of street trees in cities and their maintenance and management measures. For. Xinjiang (3), 36–37.
Terho, M. (2009). An assessment of decay among urban Tilia, Betula, and Acer trees felled as hazardous. Urban For. Urban Green. 8, 77–85. doi:10.1016/j.ufug.2009.02.004
Wang, X., and Allison, R. B. (2008). Decay detection in red oak trees using a combination of visual inspection, acoustic testing, and resistance micro drilling. Arboric. Urban For. 34 (1), 1–4. doi:10.48044/jauf.2008.001
Wang, Y., Luo, Y., and Lin, Z. (2017). Judging the health risks of campus trees in Chinese Culture University by visual tree assessment method. Huagang Agric. Sci. 40, 61–74.
Wong, J. F. (2010). Factors affecting open building implementation in high density mass housing design in Hong Kong. Habitat Int. 34 (2), 174–182. doi:10.1016/j.habitatint.2009.09.001
Wu, Y. (2016). Tree fracture mechanism and risk assessment and protection under wind and snow disasters. Anhui, China: Anhui Agricultural University.
Xiao, J., Tan, H., Chen, J., and Wang, A. (2016). Preliminary study on risk assessment model of ancient and famous trees. Hunan For. Sci. Technol. 43 (1), 61–65+79. (in Chinese with English abstract).
Yan, W., Yang, R., and Zhang, S. (2012). Thinking of the present and development of avenues in Shanghai. Acta Agric. Shanghai 28 (2), 106–109.
Yang, R., and Yan, W. (2013). Status and future of construction and management for street trees in Shanghai. J. Jiangsu For. Sci. Technol. 40 (3), 34–37.
Zhan, M., Cai, Q., Li, J., and Chen, F. (2007). Using visual trees to assess risk and health: a case study of old trees in Taichung County. Cross-strait Young Scientists Forum. Taiwan, China: Chinese Forestry Society.
Keywords: ground-penetrating radar, risk matrix, risk prevention, sonic tomography, spatial pattern, tree detection
Citation: He K, Wei L and Wang B (2024) Construction of street tree risk assessment system and empirical analysis based on non-destructive testing technologies. Front. Earth Sci. 12:1338316. doi: 10.3389/feart.2024.1338316
Received: 14 November 2023; Accepted: 26 February 2024;
Published: 08 April 2024.
Edited by:
Marcelo Cohen, Federal University of Pará, BrazilReviewed by:
Andrea R. Proto, Mediterranea University of Reggio Calabria, ItalyMaria Sudakova, Lomonosov Moscow State University, Russia
Raquel Gonçalves, State University of Campinas, Brazil
Copyright © 2024 He, Wei and Wang. This is an open-access article distributed under the terms of the Creative Commons Attribution License (CC BY). The use, distribution or reproduction in other forums is permitted, provided the original author(s) and the copyright owner(s) are credited and that the original publication in this journal is cited, in accordance with accepted academic practice. No use, distribution or reproduction is permitted which does not comply with these terms.
*Correspondence: Benyao Wang, d2FuZ2Jlbnlhb0AxNjMuY29t